- 1Veterinary Epidemiology, Economics and Public Health, Department of Pathobiology and Population Sciences, The Royal Veterinary College, Hatfield, Hertfordshire, United Kingdom
- 2Department of Public Health, Pharmacology and Toxicology, University of Nairobi, Nairobi, Kenya
This study investigated the economic performance of pastoralist small ruminant flocks in Kajiado county, Kenya, and the financial impact of mortalities and changes in performance indicators. A survey of 129 pastoralist small ruminant flocks captured production and economic data for a 12-month period via interview. Simulation models were then developed for flocks with different performance levels and trading activities. Their gross margins, and the financial impact from mortalities, were estimated. Sensitivity analyses were conducted for select performance indicators to assess their relative economic importance. On average, pastoralists who were not purchasing supplementary feed (79% of flocks) had gross margins of KSh 3,016 – KSh 3,123 per reproductive female. Goat production generated 2.43–2.51 times greater returns than sheep production. High efficiency mixed flocks achieved 2.04–2.06 times greater returns than medium efficiency flocks, and 12.19–14.25 times greater returns than low efficiency flocks. Pastoralist-only flocks had 3.82–4.01 times greater returns than pastoralist-traders. Financial losses due to mortality were on average 17.81% of the starting flock value. Fecundity and abortion rates in sheep, and parturition rate in goats, had the greatest economic impact. The developed models could support decision-making toward improving the economic performance of pastoralist flocks and the evaluation of interventions and external shocks.
1 Introduction
Pastoralist livestock systems represent extensive, mobile systems of rearing livestock on rangelands, or natural grasslands (Homewood, 2006; Davies et al., 2010). Their distinctive characteristics make them a critical economic activity in arid and semi-arid lands (ASALs), supporting the livelihoods and food security of approximately 120 million livestock owners and their dependents worldwide [Rass, 2006; Government of Kenya (GoK), 2012; Katiku et al., 2013]. Sub-Saharan Africa alone is home to 50 million pastoralists, yet this group remains one of the global poorest, with 25–50% believed to be living in extreme poverty (Rass, 2006). In addition to their economic importance, livestock hold profound socio-cultural value in pastoralist communities, serving as valuable assets and conferring cultural identity [Ayantunde et al., 2011; Government of Kenya (GoK), 2012]. These include their role as “mobile banks” due to their liquidity and as sources of wealth, prestige, gifts, and dowry (Nyariki and Ngugi, 2002). From a wider economic lens, pastoralist livestock systems contribute considerably to national economies and food supply. In African countries, they represent between 10 and 44% of gross domestic product (GDP) and directly support an estimated 20 million people, with benefits extending to 1.3 billion people through their value chains (Catley et al., 2013). In Kenya, the pastoralist agricultural sector contributes 13% to the national GDP, with a total worth of US$1.13 billion, primarily driven by the livestock sector, accounting for 92% (US$1.04 billion) of this value (Nyariki, 2017; Nyariki and Amwata, 2019). Notably, approximately 80% of Kenya’s land can be classified as ASALs where pastoralism is the predominant economic activity (Fitzgibbon, 2012; Amwata et al., 2016).
The Government of Kenya (GoK) has integrated plans for the ASALs into the country long-term development plan, Kenya Vision 2030, recognizing them as a focus area for poverty reduction and food insecurity [Government of Kenya (GoK), 2013a,b, 2018]. Under the ASALs Development Programme of Kenya Vision 2030, various initiatives are proposed, including the Livestock Production Strategy, the Range Management and Pastoralism Strategy, and the Livestock and Pastoralism Support Programme. These initiatives aim to empower pastoralists and ensure sustainability of their livelihoods. However, despite Kajiado County’s Integrated Development Plan (2013–2017) highlighting low livestock productivity as one of its major barriers to development, the region lacks a consitent monitoring system to measure pastoralist livestock productivity [Government of Kenya (GoK), 2013a,b].
While pastoralist livestock systems serve as significant national economic assets, they largely operate within “informal economies,” lacking comprehensive data on their production and economic performance. There have been numerous calls to strengthen data collection from these systems to better inform policy development (Chambwera et al., 2011; Schneider, 2011). Investing in research and data collection would certainly yield significant benefits, facilitating the development of interventions and policies aimed at enhancing pastoralist food production resilience and evaluating existing measures (MacGregor and Hesse, 2013). However, gathering the intricate production and economic data required for economic assessments presents significant challenges. Most pastoralists neither receive formal salaries nor pay income tax (MacGregor and Hesse, 2013), and their nomadic lifestyles make them difficult to access and monitor consistently over time. As a consequence, to date, economic studies of pastoralist livestock systems in Kenya have been limited to estimating national values of pastoralism, or the economic impacts of external shocks such as outbreaks of disease (Kihu et al., 2015; Nyariki and Amwata, 2019). Regardless, their comprehensive evaluation and an understanding of their economic performance at the farm-level remains poorly understood. Given that small ruminants serve as a prominent source of income and livelihoods for many pastoralists in Kajiado county (Abassa, 1995; Ojango et al., 2014), it is imperative that methodologies are developed that are capable of assessing their production and economic performance while addressing the unique challenges of data capture and analysis. Such methodologies can help gage their resilience to economic shocks and inform the formulation of effective policies to ensure their sustainability and improvement.
The objective of this study is to evaluate the economic performance of pastoralist small ruminant flocks in Kajiado, Kenya. Additionally, it seeks to analyze the financial impacts of mortalities and changes in select reproduction performance indicators. The methodology presented herein can serve as a framework for monitoring the economic viability of small ruminant flocks, facilitating better resource allocation, policy development, and the evaluation of financial ramifications associated with interventions, policies, or external shocks.
2 Materials and methods
2.1 Study site and selection of participants
Kajiado county was selected due to its large population of small ruminants and pastoralism representing the predominant source of livelihood of its rural communities [Government of Kenya (GoK), 2013a,b]. Additionally, it is one of the country’s arid and semi-arid lands (ASALs) targeted by the government of Kenya in their Kenya Vision 2030 for strategic development, and the County Integrated Development Plan (2013–2017) has highlighted low livestock productivity as a prominent development issue [Government of Kenya (GoK), 2013a,b]. Kajiado is located in southern Kenya and covers an area of 21,900 Km2 with a population of 1,120,649 sheep and 877,744 goats, the most abundant livestock followed by cattle (577,710) [Kenya National Bureau of Statistics (KNBS), 2019].
The county has five sub-counties: Kajiado North, Kajiado Central, Kajiado East, Kajiado West and Kajiado South. Each is divided into wards (the smallest administrative unit in the Kenya system of local government) of which there are 25 in Kajiado. A total of five wards were selected: two from Kajiado East (Kaputiei North and Kenyawa-Poka), two from Kajiado Central (Ildamat and Matapato South) and one from Kajiado West (Iloodokilani). These were selected following guidance from the county’s Directorate of Veterinary Services (DVS) based on their small ruminant livestock densities and ease of accessibility to pastoralists.
A cross-sectional survey of 130 small ruminant pastoralists was conducted in 2018. The detailed survey methods are presented by Ballesteros et al. (2021). The study focused on medium and large flocks, defined as those with more than 10 ewes and/or does and a minimum flock size of 50 small ruminants. These were considered sufficiently large to maintain self-replacement and operate commercially. Flocks were selected through systematic sampling using transect drivers along rural feeder roads in each of the study wards, and pastoralists were recruited with the assistance of DVS staff. Recruitment of more than one flock per manyatta (Maasai villages having several flocks belonging to different households) was avoided.
2.2 Data collection
Data were collected via face-to-face interviews with flock owners and/or the herdsman using a structured questionnaire. Prior to main data collection, the questionnaire was piloted with seven pastoralists in Kajiado to test its acceptability and understanding. A total of 129 flocks were analyzed with one flock excluded due to erroneous data entry by an enumerator at the time of survey.
All the data collected were obtained from participants’ recall and comprised information on flock dynamics, management practices, and economic data for the preceding annual (12 month) period of production, as well as data on owner characteristics. Interviews were conducted between June–August 2019 to ensure that data collected from different flocks referred to a similar retrospective period.
To assess population dynamics, data on flock size and age and sex structure were collected. For this, animals were categorized into four groups: young females (<2 years old), young males (<2 years old), adult females (>2 years old), and adult males (>2 years old). These categories were based on pastoralists’ preference and acceptability, as determined through pilot interviews, allowing capturing the required data more accurately (i.e., additional age categories were piloted and deemed unreliable or unfeasible). Retrospective data was captured on reproduction parameters (e.g., number of newborns, abortions, etc.), mortalities, offtakes, and intakes for the different age-sex categories for the preceding12-month period. The number of newborn lambs or kids, and their mortalities, were not captured by sex due to challenges in pastoralist’s recall. Mortality data included causes of mortality; disease, drought, predation, and “other” reasons. Offtake data were captured for; sales, animals slaughtered for home consumption, gifts/dowries/inheritances given, animals lost (i.e., missing) and “other” reasons. Intake data were captured for; purchases, gifts/dowries/inheritances received and “other” reasons. Other parameters included the number of newborn lambs or kids that survived, and the number of newborn lambs or kids that died, in the annual period.
Economic data were collected for the purchase and sale value of sheep and goats separately, and the four age-sex categories. The sources of livestock transaction were also obtained; market, broker, family member, pastoralist, and pastoralist outside of Kajiado. Variable cost data were collected on hay, concentrate feed, mineral supplementation, and water costs.
2.3 Flock modeling
Static production models were developed in Microsoft Excel to simulate flock dynamics over an annual period. Six flock types (or production systems) were modeled. Three related to the level of flock performance efficiency (low, medium, and high efficiency flocks), as described by Ballesteros et al. (2021) and based on a combination of net fecundity rate (average offspring born alive per reproductive female) and production rate (indicator that accounts for the balance of flock mortalities, intakes, and offtakes) for each species. These parameters were deemed the most reliable and best reflect a flocks’ production performance. Of the 129 flocks, Ballesteros et al. (2021) categorized 34 as low efficiency, 44 medium efficiency and 30 high efficiency. Data from these flocks were used for parametrization of their respective production models. Twenty-one flocks were not designated a flock efficiency category due to having either no sheep or no goats (20 flocks), or when a pastoralist declared to purposely remove goats from their flocks to uniquely own sheep (one flock).
Two models were also developed to reflect flocks where pastoralists also conducted trading activities (pastoralist-trader) or not (pastoralist-only). The distinction was based on responses from the survey questionnaire as to whether the farmer bought in animals or relied upon self-replacement. The two models were parametrized based on 68 flock categorized as pastoralist-only and 61 flocks categorized as pastoralist-traders, as indicated by Ballesteros et al. (2021).
Finally, one model was developed to represent the average flock in Kajiado and was based on all 129 flocks participating in our survey.
Each model had two sub-models, one for sheep and one for goats. To ensure consistency with the survey data, the models divided animals into four categories for each species: young females <2 years (YF), young males <2 years (YM), adult females >2 years (RF) and adult males >2 years (AM). The average number of animals in each category recorded at the time of survey (i.e., the end of the production year) for each flock-type was used to represent the flock structure at the start of the production year in the models (i.e., day 0 in the models). Using these values as Day 0 in the models, we simulated the population dynamics of the flock for a second cycle, but with all other parameters considered equal. The models therefore estimated what would occur if a flock from our survey ran for a second cycle with identical production and economic parameters. Here, the production cycle modeled is named the “model-cycle” and the production cycle analyzed based on the survey data the “data-cycle” (Figure 1).
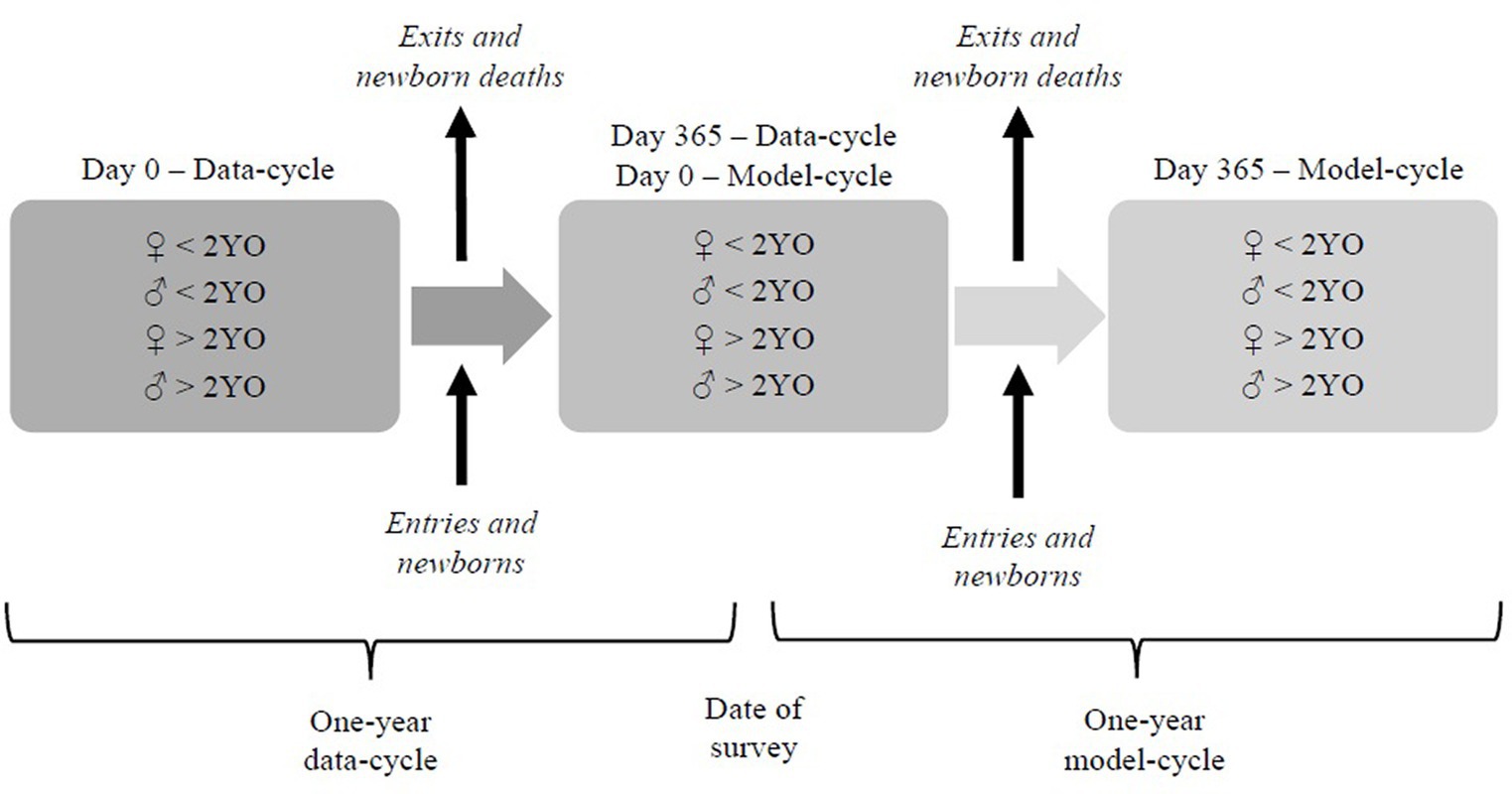
Figure 1. Diagram indicating the two cycles considered in this study; the data-cycle and model-cycle.
Several steps were required to obtain the number of newborns and the number of animals entering and exiting the flock, as well as to estimate the number of young animals transitioning to an adult age group. These steps are summarized in the following sections, and the complete methodology used to develop the models, including detailed calculations, are provided in the Supplementary material.
2.3.1 Calculation of population dynamics for the data-cycle
It was not possible to determine the number of RFs at the beginning of the data-cycle. Thus, for modeling purposes, many of the parameters were calculated as rates of RFs reported at the time of survey (i.e., at the end of the data-cycle). For example, fecundity rate was calculated as the number of animals born alive or dead divided by the number of RF at the end of the data-cycle. Rates were calculated also for newborn mortalities, total entries (the sum of individual intakes) and total exits (the sum of individual causes of mortalities and offtakes). Newborns were not included as entries, and newborn deaths are not included as exits, as these were considered separately. Rates were calculated for each flock in the survey, and mean rates for each type of flock was then used for the models.
2.3.2 Calculation of population dynamics for the model-cycle
The starting population (Day 0 model-cycle) for each of the production models was based on the mean population of animals in each age-sex category at the time of the interview (Day 365 data-cycle). The FR, entries rate, and exits rate values obtained for the data-cycle were used to gain an initial estimation of the number of number of newborn, total entries, and total exits in the model-cycle by multiplying them with the number of RFs at the start of the model cycle (or end of the data cycle). It was assumed that 50% of the total newborns were male or female. Since the number RFs at the start and at the end of the cycle are different, these initial rate values had to be corrected.
For this, it was first important to consider that over the production year, a number of sheep and goats in the <2-year-old category would be transitioning to the >2-year-old category. Naturally, only those aged 1–2 years old could possibly transition in an annual period. As the production models simulate the production year immediately prior to lambing or kidding season, it was assumed that all YFs and YMs at the start of the production year were between the ages of 1–2 years old. Another assumption was made that all YFs and YMs entering and exiting the flock were between the ages of 1–2 years old. Therefore, the number of young animals transitioning to adult category were all YFs and YMs at the start of the model cycle and those entering during the cycle, minus those YFs and YMs exiting during the cycle. This, together with the number of newborns, total entries and total exits allowed the calculation of the population numbers of each animal category at the end of the model-cycle. Subsequently, the FR, entries rate, and exits rate used were adjusted (fitted) until these were equal in the data-cycle and model-cycle.
To estimate the number of each type of each type of entry (i.e., purchased, gifts received and other entries) and exit (sale, gift provided, slaughtered, lost, disease death, predation death, drought death and death others) in the models, we first estimated their proportional contribution to the total entries and exit to each of the flock in the survey. Means were then obtained for each flock-type, and these were multiplied by the total number of entries and exit in the models (e.g., the mean proportion of purchased animals for a given flock-type was multiplied by the total number of entries in the corresponding model to estimate number of purchased animals in the model cycle). The models were developed to ensure that the sum of each type of entry and exit did not exceed the total number of entries or exits.
2.4 Economic modeling
Economic parameters were integrated into the models using sale and purchase prices of sheep and goats, and the variable costs data captured by the survey. The mean prices for each animal category were calculated and used to represent the value of entries, exits, newborns and the total flock value at the beginning and end of the model-cycle. Entries and newborn animals that survived were assigned the mean values of purchase, while exits were assigned the mean values of sale. The same prices were used across all flock types. Flock revenue was then calculated taking into consideration the total value of entries, exits and the change in flock value by the end of the model-cycle.
Variable cost data included hay, concentrates, minerals, water, and vaccination costs. For hay, concentrates, and minerals, data for their quantity purchased (in bales or kg) and price (KSh/bale of kg) over the year was captured. Data on water costs included the cost per month of water to the farmer (KSh/month), and the number of months where water was paid for. These costs were not reported for sheep and goats separately; thus it was assumed that feed and water were utilized equally. For each cost, a rate was calculated for each flock in the survey as a function of the number of RFs at the time of the survey (e.g., cost of water per RF), and the mean rate for each flock-type was used as the input for the economic models to calculate the annual costs for each feed and water cost. Data on annual veterinary costs, transactions of animal products (such as milk and wool) and labor costs, among others, were not captured by the survey questionnaire. However, estimations of vaccination costs, availability, and uptake, were obtained from co-author expertise of these systems and their contact with the DVS. Small ruminants are routinely vaccinated for caprine contagious pleuropneumonia (CCPP) and bluetongue. CCPP vaccination is estimated at a cost of 15KSh per animal and is readily available to pastoralists. Bluetongue vaccination under normal circumstances is government-provided at no cost. However, at times of limited supply, 50% of pastoralists reportedly purchase the vaccine at a cost of 10KSh per animal. Due to low uptake, FMD vaccination was not considered routine, and excluded from the vaccination costs. Vaccination against other infectious diseases also occurs but at zero cost. Peste des petits ruminants (PPR) and sheep and goat pox vaccination is provided by the government. Vaccination costs were hence calculated in the model by multiplying the cost of vaccinating each animal in a flock against CCPP and Bluetongue by the total number of sheep or goats in flocks at the start of the model-cycle for each flock type.
Estimates for other veterinary costs potentially incurred were unable to be accurately captured due to the high variability in reported health management practices, and the types and sources of medicines used. Therefore, they are excluded from the variable costs. Thus, the annual variable costs (VC) for the whole flock were calculated as the sum of the annual cost of hay, concentrates, minerals, water, and vaccination. Yet, given that flocks used different combinations of variable costs (e.g., some purchase hay or not), variable costs estimation were calculated for each variable cost structure.
Separate gross margins for sheep and goats populations were calculated by subtracting the variable costs from the revenue generated. Results were also collated to represent gross margins of mixed small ruminant flocks. Final gross margins were then presented per flock basis and per RF from day 0 in the model-cycle for comparison purposes. Gross margins of flocks for the data-cycle were also calculated. This was done by first estimating the gross margin per RF at the end of the model-cycle, and then multiplying this value by the number of RFs at the end of the data-cycle. The financial loss due to mortalities were then calculated. For this we first calculated the total population at risk of death for each animal category. The population at risk comprised the number of sheep or goats that had been in the flock at any point during the year, including those at the start of the production year and the total entries for each age and sex category. The sale price of animals in each category was used as proxy for the financial value of each death. The total financial loss due to mortality was then calculated as a proportion of the flock value at the start of the cycle.
2.5 Sensitivity analyses
Sensitivity analysis was conducted on selected RPIs and mortality variables to observe their impact on gross margins within the models. The four RPIs examined were fecundity rate, net prolificacy rate (average number of offspring born alive per parturition), parturition rate, and abortion rate. Mortality variables included mortality rates per animal category and for newborn lambs or kids. Separate sensitivity analyses were performed for sheep and goats by adjusting each input variable incrementally by 0.01 from its current value. The gross margin was used as the output variable to evaluate the impact adjusting variables. While fecundity rate was an input variable that could be easily adjusted, parturition rate, net prolificacy rate, and abortion rate were integrated into the models solely for the purpose of sensitivity analysis and were not considered input variables. Further details on the methods used for the sensitivity analysis can be found in the Supplementary material.
3 Results
3.1 Descriptive analyses
The following results describe the data captured and analyzed from the retrospective survey used to inform the development of the models. Therefore, it represents data from the data-cycle of production as described in our methods.
At the time of survey, pastoralists on average had more sheep in their flocks than goats (Table 1). RFs predominated, comprising approximately half of the total small ruminants in flocks. AMs comprised the smallest category. Complete data on the mean number of newborns, RPIs and mortalities are summarized in Supplementary Table S1 of the Supplementary material.
Average prices of sheep, goats, and feed and water costs are summarized in Supplementary Table S2 of the Supplementary material. Comparing average prices from all flocks, YF sheep (KSh 4,000) and goats (Ksh 3,000) had the lowest sale prices among the age/sex categories, while AM sheep (KSh 7,422.33) and goats (KSh 8,469.24) had the highest sale prices. Concerning purchases, YF sheep (KSh 3,026.09) and goats (KSh 4,166.67) had the lowest purchase prices, and YMs had the highest purchase price for sheep (KSh 5,666.67), while AMs (KSh 6,222.22) commanded the highest mean purchase price for goats. The mean sale and purchase prices of sheep and goats by source of their transaction is found in Supplementary Table S3. The findings suggest that markets were the predominant source of transaction for small ruminant pastoralists in Kajiado. However, several also utilized brokers, family members and other pastoralists (within and outside Kajiado county). Small ruminants appeared to fetch a lower price at market than if they were purchased or sold via another pastoralist or to family members, but prices were higher compared to brokers.
The type of feed and water costs small ruminant pastoralists incurred varied greatly. The majority had no supplementary feed costs (79.07%); almost half (48.84%) reported to only pay for water with no expenditure on feed, while almost a third (30.23%) reported that they had no water or feed costs. A complete breakdown of feed and water cost structures for all flocks and by flock-type is presented below in Table 2. Regarding water costs, for those who paid for water, it was obtained either by delivery by a water tanker (<1.00%% in the rainy season, 1.55% in the dry season), or by collection from a borehole/well (5.43% in the rainy season, 57.36% in the dry season). Those who had no water costs, water was sourced from either a river (41.86% in the rainy season, 0.78% in the dry season), rainfall (0.78% in the rainy season, <1.00% in the dry season), water pan (a traditional method of rain water collection and storage from depressions dug in low-lying land that collect water from surface runoff during rainfall) (42.64% in the rainy season, 14.73% in the dry season), their own borehole/well (5.43% in the rainy season, 2.33% in the dry season), from a free piped source (3.10% in the rainy season, 14.73% in the dry season), or from another pastoralist’s borehole/well (<1.00% in the rainy season, 2.33% in the dry season).
3.2 Model performance
3.2.1 Flock dynamics
The complete flock dynamics simulated by the models are summarized in Table 3. The average sheep flock size decreased by 10.58 sheep, losing 5.77 RFs annually. For goats, the average flock size also decreased but by 4.10 goats, losing approximately two RFs. Across all flock efficiency groups for sheep, the total number of sheep and RFs decreased. Only high efficiency goat flocks showed an increasing number of RFs. Pastoralist-only flock sizes and RFs increased, while pastoralist-trader flocks shrunk due to reductions in both sheep and goats, as well as their number of RFs.
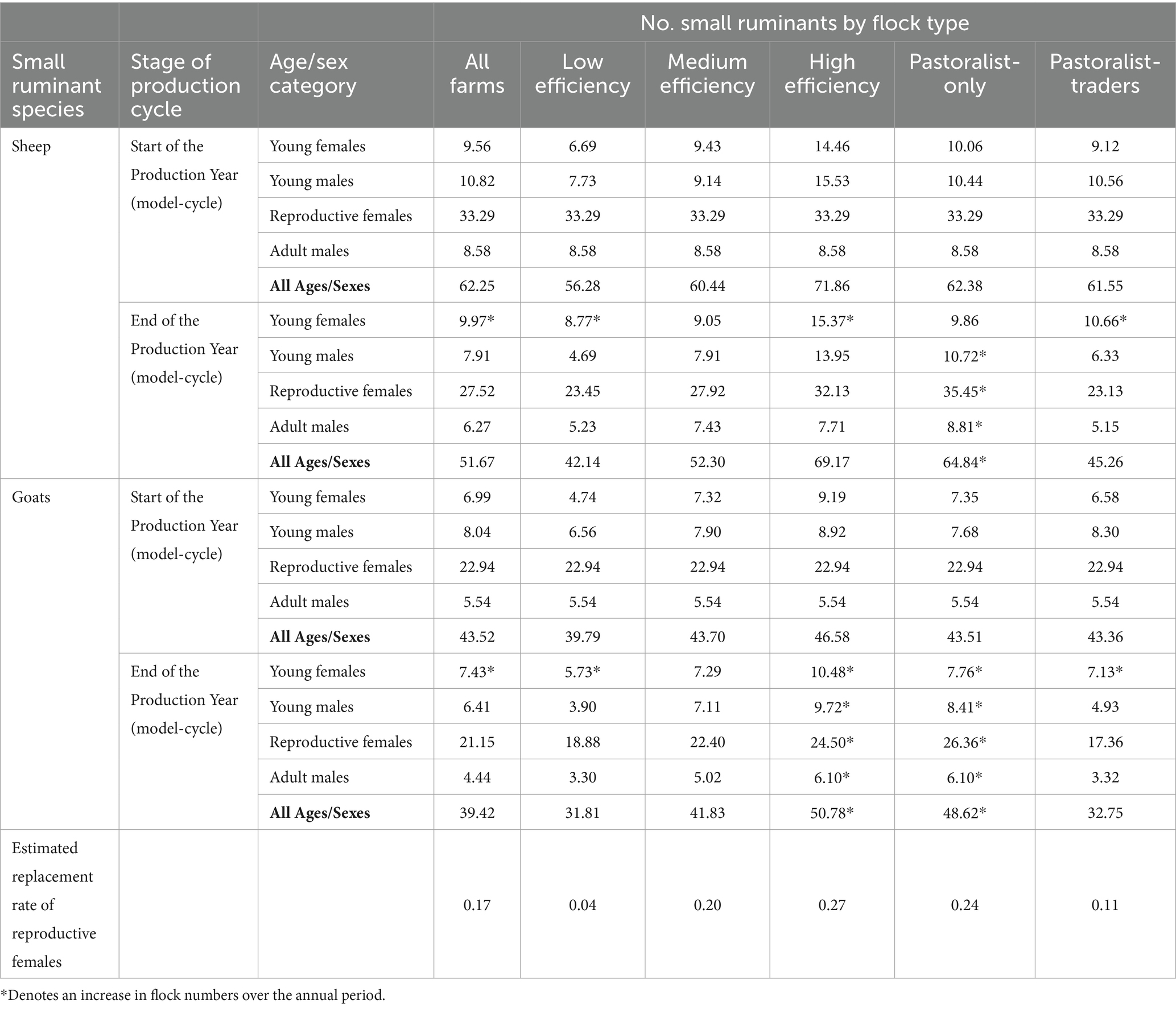
Table 3. Predicted flock dynamics of small ruminants over the annual period of analysis comparing flock efficiencies and enterprise type.
The average replacement rate was 17.5% for all farms, of which 49% was achieved using own young stock. Low efficiency and pastoralist-trader flocks did not have the capacity to replace animals with their own young stock and purchased the majority of their replacements. Medium and high efficiency flocks used their own young stock to replace over 70% of their replacements, and pastoralist-only flocks used own stock for 96% of their replacements.
3.2.2 Results of the gross margin analyses
A range of feed and water cost structures were reported, the most common being water costs only or no feed or water costs. When all feed and water costs are considered, the models estimated that the average pastoralist had a negative gross margin of −58.89 KSh per RF (Table 4). This figure comes predominantly from economic losses from sheep (−508.33 KSh/ ewe), while goats are observed to be more economically productive (593.32 KSh/doe). However, those flocks purchasing only water and no feed (the majority of flocks), the GM was 3015.55 KSh per RF. Overall, when considering the most common cost structures, goat production resulted in 2.43–2.51 times greater returns than sheep production. Although sheep provided greater value from sales than goats, differences are largely explained by a greater change in flock value in sheep than goats.
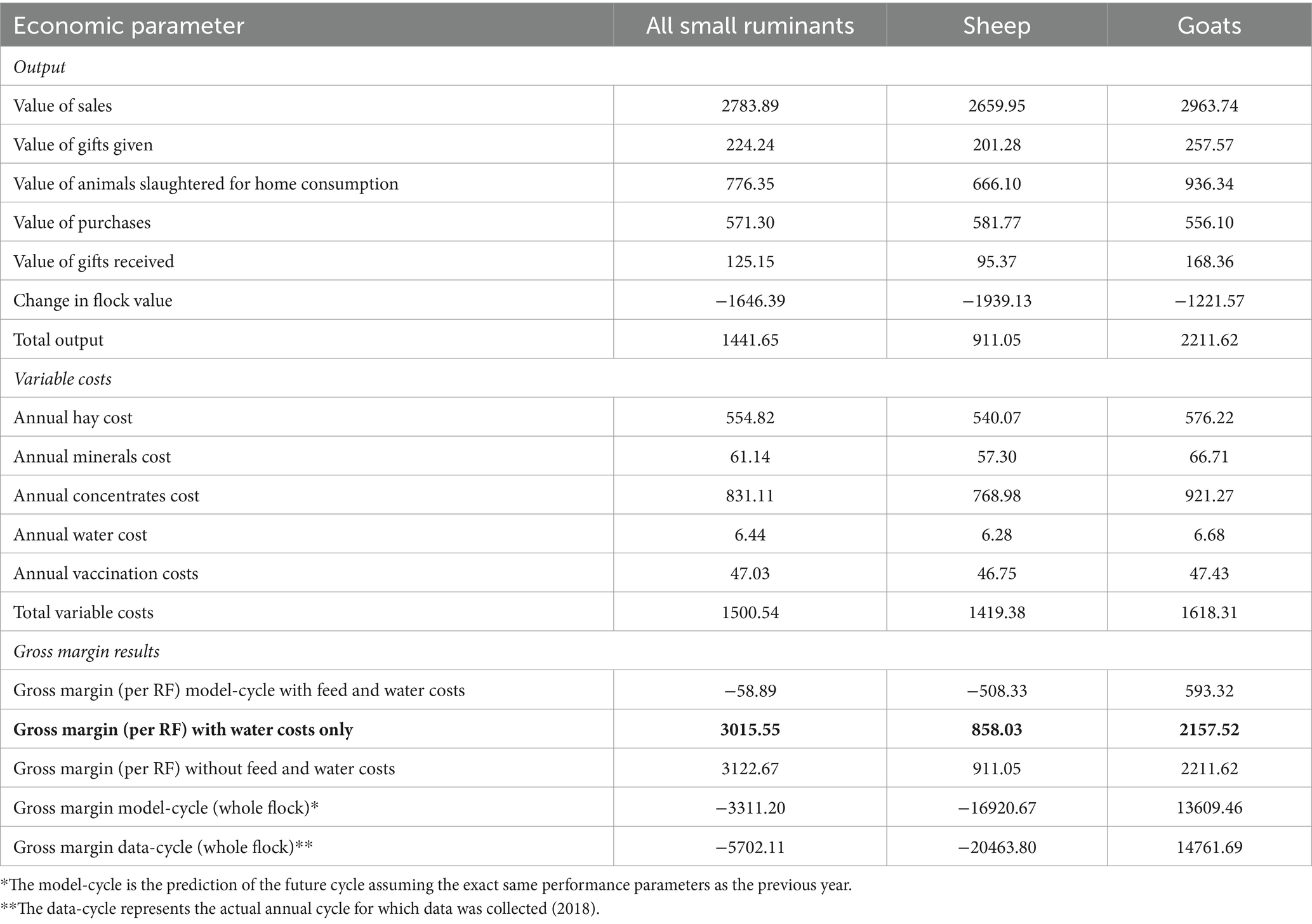
Table 4. Comparison of gross margins (in KSh) per reproductive female from sheep and goats and their contributions to the overall flock gross margin for the average farm in Kajiado.
Considering the most common feed and water cost structures again, high efficiency flocks achieved 2.04–2.06 times greater returns than medium efficiency flocks and 12.19–14.25 times greater returns than low efficiency flocks (for the average mixed flock). The returns for pastoralist-only was 3.82–4.01 times greater than pastoralist-traders (Figure 2). Improvements are largely explained by a reducing value of purchases and changes in flock value with improving flock efficiency. Complete gross margins by different flock-type can be found in Supplementary Tables S4, S5 of the Supplementary material.
Gross margins (per ewe or doe) were also estimated for all feed and water cost structures reported by respondents (Supplementary Table S6 of the Supplementary material). Furthermore, there was a difference between the gross margins generated from the data-cycle and the model-cycle. Those flock- types with negative gross margins in the model-cycle had larger deficits in gross margins in the data-cycle, and vice versa (Table 3). The average flock had an 72% increase in deficit in the data-cycle compared with the model-cycle.
3.2.3 Mortality rates and value of mortalities
The full mortality rates by age-sex category, newborns, and for all flock types, are summarized in Supplementary Table S7 of the Supplementary material. Notably, mortality rates were highest in young animals compared to adults for both sheep and goats and across all flock-types, reaching up to 70% in sheep YFs of low efficiency flocks. In goats, YFs of low efficiency flocks also had the highest mortality rates at 55%. Across all flock-types, YF and YM goats had lower mortality rates in comparison to sheep. Furthermore, mortality rates in young animals were observed to decrease in young animals with improving flock efficiency, and pastoralist-traders had higher mortality rates in young animals compared to pastoralist-only flocks.
Table 5 presents the number of mortalities at risk of mortality and their economic value, accounting that all flocks started the production year with the same number of animals for comparison purposes, for the different flock types studied On average, pastoralists lost KSh 1990.66 due to mortality, representing approximately17.8% of the flock value at the start of the model-cycle. A full breakdown by age and sex category, and by cause of mortality, can be found in Supplementary Table S8 in the Supplementary material.
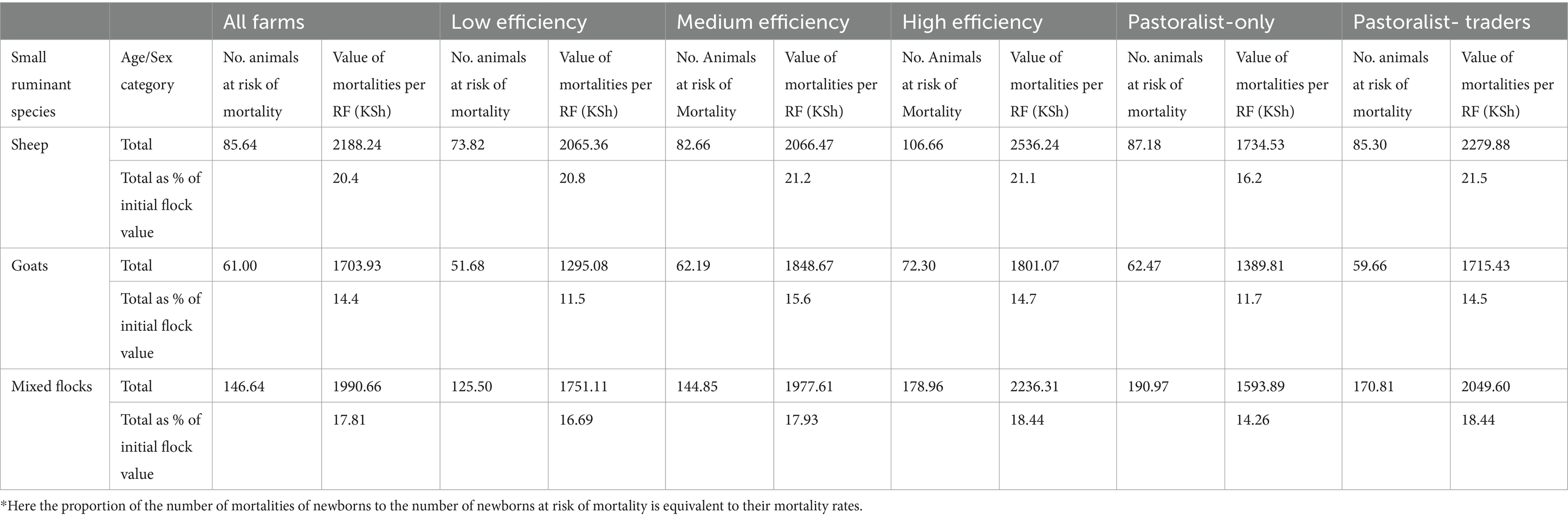
Table 5. Number of animals at risk of mortality and value of mortalities per reproductive female simulated by the models for all farms and by flock efficiency and trading activity group.
3.3 Sensitivity analyses
3.3.1 Impact of reproductive performance indicators
For the average pastoralist, changes in FR and AR had equally the greatest impact on gross margin per ewe of sheep with a 39.21KSh change in gross margin per ewe per 0.01 change in FR and AR. This was followed by PR with a 33.99KSh change in gross margin per ewe per 0.01 change in PR, and by NPR with a 23.98KSh change in gross margin per ewe per 0.01 change in NPR.
For goats, changes in PR had greatest impact on gross margin per doe with a 31.84KSh change in gross margin per doe per 0.01 change in PR. This was followed by FR and AR which also had an equivalent impact with a 30.74KSh change in gross margin per doe per 0.01 change in FR and AR. NPR had the least impact, with a change of 18.72KSh in gross margin per doe per doe per 0.01 change in NPR.
3.3.2 Impact of mortality rates
In sheep, changes in mortality rate in RFs had the greatest impact on gross margin per ewe with a 37.99KSh change in gross margin per ewe per 0.01 change in mortality rate in RFs. This was followed by newborn lambs, YMs, and AMs, respectively. Changes in YFs had the least impact (13.95KSh change in gross margin per ewe per 0.01 change in mortality rate in YFs).
Findings for goats also showed that changes in RFs had the greatest impact on gross margin per doe with a 52.74KSh change in gross margin per doe per 0.01 change in mortality rate in RFs. This was followed by YMs, newborn kids, and AMs, respectively. Similarly to sheep, changes in the mortality rate in YFs had the least impact (18.08KSh change in gross margin per doe per 0.01 change in mortality rate in YFs).
4 Discussion
This study presents a methodology for evaluating the economic performance of pastoralist small ruminant flocks in areas with limited accessibility and farm records, making it applicable to the global majority of pastoralists. The models serve as a valuable tool for generating benchmarking data, assessing the sector’s economic state, monitoring the effectiveness of interventions and policies, and estimating the impact of shocks on the system. We highlight the weak economic performance and fragile economic environment of these systems in Kajiado, with negative returns and significant financial losses due to mortality commonplace. These models identify systems at higher financial risk and demonstrate the potential benefits of improved reproduction and production performance. The findings are considered representative of the region, with average flock sizes observed aligning with those reported by Gitonga et al. (2016).
Modeling revealed that, except for high efficiency goat flocks or pastoralist-only flocks, maintaining the number of RFs in an annual cycle is challenging, highlighting the instability of their flock sizes and operations. Livestock numbers are crucial for pastoralist wealth and sustaining RF numbers is essential for generating offspring in the subsequent year for sale, asset accumulation, or self-replacement (Rass, 2006). According to our models, the primary factors impacting flock size are the rate of sales and the high mortalities of RFs, accounting for 45 and 36% of all exits in this category, respectively. Notably, 25% of exits were attributed to disease mortalities. This issue is exacerbated by the low replacement rates observed, particularly in low efficiency flocks and flocks managed by pastoralist-traders, who rely on purchases to replace RFs. It’s worth highlighting that the model calculations assume that all YFs at the start of the cycle, or those entering the flock, were over 1-year-old and that those remaining would transition into the RF category. Therefore, the models maximize the possible replacement rate, confirming declining RF populations in most flocks.
The models used in this study demonstrate a significant disparity in economic performance between farms operating at high and low efficiency. Only high efficiency flocks generated positive returns when accounting for variable costs. This difference in economic sustainability is also evident in the contrast between gross margins observed in the model-cycle compared to the data-cycle. Flocks with poor performance will continue to incur losses in subsequent years if they maintain the same practices. Highly efficient flocks and those managed by pastoralists-only can increase their gross margins by 2 and 11% the following cycle, respectively. The main factor contributing to these increased returns is the variation in flock value, which was negative in low efficiency flocks and positive in high efficiency flocks. This parameter accounts for losses resulting from mortality and changes in reproductive efficiency, highlighting their significance for financial sustainability. However, financial losses associated with mortality were substantial across all flock types investigated, which increased as flock efficiency improved. This is primarily due to more efficient flocks having a higher number of animals at risk of mortality. In fact, the mortality rate per animal at risk decreases as flock performance improves. Additionally, as efficiency increases, the number of animals entering the flock decreases, thus, the higher number of animals at risk is a result of improved reproductive performance. Although higher mortalities were observed in younger animals, RFs represented the age group with the most sales and, on average, commanded higher sale values. Sensitivity analyses further highlighted that changes in mortality rates in RFs would have the greatest economic impact, despite higher mortality rates observed in younger stock. However, the high mortality rate among young animals also has long-term implications for flock sustainability, as their survival enables improved self-replacement, asset accumulation, or future sale. On average, approximately 66% of mortalities were attributed to diseases. While numerous diseases contribute to small ruminant mortality, respiratory and intestinal diseases are considered the most significant (Peeler and Wanyangu, 1998). However, there appear to be gaps in the literature regarding the major causes of death in small ruminants in Kajiado, which should be investigated. The substantial difference in gross margins should serve as an incentive for pastoralists with lower efficiency to enhance their flock performance. The findings also suggest that pastoralists engaged in trading activities may be less concerned about flock health management, possibly because animals remain in their flocks for shorter periods. These results align with Roba et al. (2019) who reported that small ruminant traders from Marsabit County (Northern Kenya) had marginal profits and were at high risk of economic losses. Their vulnerability raises concerns for the sustainability of pastoralist small ruminant value chains in Kajiado, as they connect pastoralists to markets (Roba et al., 2019).
This study enhances our understanding of the cost structure distribution among pastoralists. Most pastoralists in this study practiced low-input small ruminant rearing, relying instead on natural resources, which is consistent with other studies (Kosgey et al., 2008; Gikaba et al., 2015). The majority (79.07%) did not purchase supplementary feed, with nearly half (48.84%) only incurring water costs. Approximately one-third (30.23%) had no costs for feed or water. These findings align with Kosgey et al. (2008), who reported that few Kenyan small ruminant pastoralists bought supplementary roughage, and even fewer purchased concentrate feed. Other studies conducted in Kajiado found that only 17.9% of pastoralists utilized supplementary feeding to enhance livestock productivity (Kipaya et al., 2020), and modern feeding strategies were reported to be low among Maasai pastoralists (Gikaba et al., 2015). Insufficient quantity and quality of feed are known to limit small ruminant production, affecting reproductive performance, productivity, and disease resilience (Gatenby, 1996). Thus, low adoption of supplementary feeding could be a significant factor contributing to the reported low livestock productivity in the ASALs of Kajiado [Government of Kenya (GoK), 2013a,b]. While livestock mobility is an important strategy for accessing better grazing and water sources, its relevance is relatively lower for small ruminants due to their improved drought resilience and adaptability to forage quality and availability (Mworia and Kinyamario, 2008; Gikaba et al., 2015). Therefore, increased adoption of modern feeding strategies could enhance productivity and long-term economic performance. However, our findings suggest that this option is currently inaccessible for those with lower efficiency due to negative returns when all costs are considered, and alternative strategies would likely be necessary. Importantly, our study showed that cost structures were similar across different flock efficiencies, making it challenging to determine the impact of feed on gross margins. Longitudinal and intervention studies are required to better evaluate the impact of feed and their potential to help pastoralists improve flock efficiency and returns.
The implications of these findings for the resilience of flocks to droughts in the region are of significant concern. ASALs are characterized by unpredictable rainfall patterns and cyclical droughts (Irungu et al., 1998; Gikaba et al., 2014), and Kajiado is considered vulnerable to climate change [Government of Kenya (GoK), 2017]. Population growth and changes in land tenure and usage have also contributed to the degradation and loss of natural pastures in the region (Galaty, 2013; Kimaru et al., 2021a,b). Degradation of natural pastures is recognized as the most limiting factor to livestock production in Kenyan ASALs and increases the vulnerability of pastoralist livelihoods [Government of Kenya (GoK), 2011]. During droughts, pastoralists in Kajiado reportedly adapt by purchasing more supplementary feed, particularly hay (Kimaru et al., 2021a,b). However, in our study, few purchased supplementary feed, possibly due to favorable rainfall in the year preceding the survey [Kenya Food Security Steering Group (KFSSG) and Kajiado County Steering Group (KCSG), 2017, 2018]. Nevertheless, during droughts, less efficient flocks may experience high mortalities, reduced liveweights, decreased animal sale values, reduced reproductive performance, and be compelled to increase their offtakes at reduced prices. Past droughts in the region, such as the 2009 drought that caused up to 70% losses of livestock in Kajiado [Government of Kenya (GoK), 2013a,b], highlight the potential impact of barriers to accessing feed. Traditionally, pastoralists accumulate livestock during favorable years to ensure sufficient breeding populations in anticipation of droughts (Campbell, 1984; Behnke and Kerven, 1994). Under normal circumstances, they can recover flock sizes and achieve resilience, but not when droughts occur every three years or less (van de Steeg et al., 2009). The situation is compounded by deficits of supplementary feed in Kajiado, particularly during drought when demand peaks. Reports indicate hay deficits of over 95% during the droughts of 2005, 2007, and 2009 (Kimaru et al., 2021a,b). Current Disaster Response and Relief (DRR) policies in Kajiado aim to promote feed (hay) supply, production, and storage to enhance pastoralist livestock productivity and resilience during droughts. However, these policies have been slow to gain momentum due to low demand in years with favorable rainfall, high costs associated with constructing storage facilities and hay production, lack of government support, and low profitability (Kimaru et al., 2021a,b). Furthermore, there is reported lack of awareness and dissatisfaction with DRR interventions. These issues, alongside financial limitations, inhibit pastoralists’ access to feed during drought, with implications for future sustainability in light of increasing drought frequency and severity.
Our findings demonstrate that goats are the more economically efficient species in all developed models. This difference is attributed, in part, to the higher price of adult goats compared to sheep. Additionally, goats experienced fewer mortalities, resulting in pastoralists losing 20.4 and 14.4% of the initial flock value for sheep and goats, respectively. Goats also exhibited higher rates of newborns per RF and improved RPIs. These findings suggest that promoting goat production over sheep in Kajiado may be a prudent strategy, considering goats’ adaptability due to their browsing behavior and heat tolerance, and Kajiado’s vulnerability to drought [Government of Kenya (GoK), 2017]. Currently, pastoralists in Kajiado heavily rely on sheep for their livelihoods, as reflected in their higher ownership. Promoting goat ownership could enhance pastoralists’ economic returns and bolster resilience to external shocks. Yet, the existing literature is unclear as to which species is more disease resistant. Conflicting findings are reported from studies investigating diseases such as peste des petits ruminants (PPR) and intestinal parasitic diseases, confounded further by specific breed differences (Taylor, 1984; Lefèvre and Diallo, 1990; Baker, 1998; Singh et al., 2004). Further studies are required to comprehensively assess disease resistance of each species, and the specific diseases affecting small ruminants in the region before definitive recommendations can be made. Furthermore, cultural, and social preferences, breed characteristics, and market demand should also be considered.
Economic modeling has been employed in Kenya to assess the impact of PPR (Kihu et al., 2015) and estimate the national economic value of pastoralism (Nyariki and Amwata, 2019). However, flock-level economics have not been evaluated, challenged by limited production and economic data availability, a lack of recording, and difficulty accessing these systems. Our methodology overcomes these barriers and was found to be efficient, easy to implement, and acceptable by pastoralists (Ballesteros et al., 2021). Given the absence of monitoring, our methodology can inform the implementation of programs assessing flock production and economic performance. Consistent data can reveal trends over time and enable the assessment and prediction of the impacts of interventions or policies. The model outputs can serve as baselines for estimating the cost of different diseases in the region. Ongoing monitoring can also unveil the impact of external shocks and assess resilience of pastoralists when faced with sudden and severe challenges. As a farm-level tool, it can help identify sources of financial loss and guide decision-making to enhance production returns. This includes evaluating the cost-effectiveness of management strategies that consider individual needs and financial constraints. Furthermore, we suggest expanding the methodology to other pastoralist livestock systems.
Certain assumptions compensated for data limitations and study constraints. Data collection relied on pastoralists’ recall, risking recall bias and potential over or under-estimation of economic performance (Raphael, 1987). However, a certain degree is expected and accepted with this methodology (Ejlertsen et al., 2013; Lesnoff et al., 2016). Nevertheless, the use of recall, the period of analysis, and our decision to target areas without formal record keeping also dictated the age and sex categories chosen for our study and limited a greater depth of analysis. The choice of age and sex category was extensively discussed in the design of our study, and more specific age categories were trialed during pilot interviews yet were found to be inaccurate or unfeasible due to the method of recall. Without formal record keeping, pastoralists were better and more accurately able to recall information regarding newborns, and animals <2 and > 2 years of age. Considering the entire study is based on developing a system that allows the rapid and feasible capture of data on flock production in these types of areas (ASALs), where it is very difficult to access flocks, capture data, and where record keeping is an uncommon practice, the methods used represent an optimal strategy on how to capture data and which took into consideration pastoralist feedback on how to capture this data most accurately. This is further explained in the paper by Ballesteros et al. (2021). Hence, our methodology offers a viable tool for their assessment, for which conventional tools are unsuitable and demanding, and facilitates large-scale programs for their evaluation. Another limitation is also related to the data collection period. Considering economic cycles of flocks, which span multiple years depending on drought frequency, longitudinal studies are recommended to comprehend economic performance over multi-year cycles.
Variable cost data were captured on a farm-level basis, considering quantity and cost. Separate models were developed for sheep and goats, assuming their equal utilization of resources. Sheep and goats have differing nutritional requirements, affecting their consumption of feed and water. Thus, variable costs may be mis-represented in either species. Certain variable costs were excluded from our models due to limited. However, the models could be simply modified to incorporate alternative costs if known by pastoralists.
In conclusion, our models evaluate the economic performance of pastoralists flocks and baseline economic information is generated, filling knowledge gaps and setting a benchmark for the region. The models presented can assist decision-making at farm-level, and guide decision-makers in identifying targets for interventions, assessing the impact of external shocks, and developing policies that support pastoralists in Kajiado.
Data availability statement
The raw data supporting the conclusions of this article will be made available by the authors, without undue reservation.
Ethics statement
The studies involving humans were approved by Social Sciences Research Ethical Review Board (the Royal Veterinary College) International Livestock Research Institute. The studies were conducted in accordance with the local legislation and institutional requirements. The participants provided their written informed consent to participate in this study.
Author contributions
J-CA: Data curation, Formal analysis, Investigation, Methodology, Software, Validation, Visualization, Writing – original draft, Writing – review & editing. JO: Conceptualization, Data curation, Funding acquisition, Investigation, Methodology, Project administration, Resources, Supervision, Validation, Writing – original draft, Writing – review & editing. CB: Data curation, Validation, Writing – review & editing. GA: Conceptualization, Data curation, Funding acquisition, Investigation, Project administration, Resources, Supervision, Validation, Writing – review & editing. PA: Conceptualization, Data curation, Formal analysis, Funding acquisition, Investigation, Methodology, Project administration, Resources, Software, Supervision, Validation, Visualization, Writing – original draft, Writing – review & editing.
Funding
The author(s) declare that financial support was received for the research, authorship, and/or publication of this article. The study was jointly funded by the Biotechnology and Biological Science Research Council (UK) and the National Research Funds (Kenya) under the Newton Fund program for Enhancing Ruminant Livestock Productivity (BB/S004866/1).
Acknowledgments
The authors are grateful to all the pastoralists of Kajiado county and their families, DVS staff and Kajiado CVO for their continuous support during the implementation of the project. The authors also thank the International Research Livestock Institute for their help and support and Barbara Wieland (ILRI), Bryony Jones (RVC), and Richard Koch (RVC) for their guidance throughout the project.
Conflict of interest
The authors declare that the research was conducted in the absence of any commercial or financial relationships that could be construed as a potential conflict of interest.
Publisher’s note
All claims expressed in this article are solely those of the authors and do not necessarily represent those of their affiliated organizations, or those of the publisher, the editors and the reviewers. Any product that may be evaluated in this article, or claim that may be made by its manufacturer, is not guaranteed or endorsed by the publisher.
Supplementary material
The Supplementary material for this article can be found online at: https://www.frontiersin.org/articles/10.3389/fsufs.2024.1406864/full#supplementary-material
References
Abassa, K. P. (1995). Reproductive losses in small ruminants in sub-Saharan Africa: a review [working paper]. International livestock Centre for Africa (ILCA).
Amwata, D. A., Nyariki, D. M., and Musimba, N. R. K. (2016). Factors influencing pastoral and agropastoral household vulnerability to food insecurity in the drylands of Kenya: a case study of Kajiado and Makueni counties. J. Int. Dev. 28, 771–787. doi: 10.1002/jid.3123
Ayantunde, A. A., de Leeuw, J., Turner, M. D., and Said, M. (2011). Challenges of assessing the sustainability of (agro)-pastoral systems. Livest. Sci. 139, 30–43. doi: 10.1016/j.livsci.2011.03.019
Baker, R. L. (1998). Genetic resistance to endoparasites in sheep and goats: a review of genetic resistance to gastrointestinal nematode parasites in sheep and goats in the tropics and evidence for resistance in some sheep and goat breeds in sub-humid coastal Kenya. Anim. Genet. Resour. Inform. 24, 13–30. doi: 10.1017/S1014233900001103
Ballesteros, C., Mwasi, A., Mungai, E., Ibarahim, A., Thuranina-McKeever, C., Aboge, G. O., et al. (2021). Developing and validating a rapid assessment tool for small ruminant reproduction and production in pastoralist flocks in Kajiado Kenya. Vet. Anim. Sci. 13:100186. doi: 10.1016/j.vas.2021.100186
Behnke, R., and Kerven, C. (1994). Redesigning for risk: tracking and buffering environmental variability in Africa's rangelands. Nat. Resour. Perspect. 1, 1–9,
Campbell, D. J. (1984). Response to drought among farmers and herders in southern Kajiado District, Kenya. Hum. Ecol. 12, 35–64. doi: 10.1007/BF01531283
Catley, A., Lind, J., and Scoones, I. (2013). Pastoralism and development in Africa: Dynamic change at the margins : Routledge: London, England.
Chambwera, M., MacGregor, J., and Baker, A. (2011). The informal economy: A primer for development professionals on the importance of the informal economy in developing countries [sustainable markets working paper]. International Institute for Environment and Development (IIED).
Davies, J., Niamir-Fuller, M., Kerven, C., and Bauer, K. (2010). “Extensive livestock production in transition: the future of sustainable pastoralism” in Livestock in a changing landscape, Vol.1: Vol. 1: Drivers, consequences, and responses. eds. H. Steinfeld, H. Mooney, F. Schneider, and L. Neville (Island Press: Washington D.C., United States).
Ejlertsen, M., Poole, J., and Marshall, K. (2013). Sustainable management of globally significant endemic ruminant livestock in West Africa: estimates of livestock demographic parameters in Mali. Anim. Genet. Resour. 52:168. doi: 10.1017/s2078633613000179
Fitzgibbon, C. (2012). Economics of resilience study – Kenya country report : Department for International Development (DFID), London, England.
Food and Agriculture Organization (FAO) (2017). Africa sustainable livestock 2050. Country brief. Kenya: Food and Agriculture Organization (FAO).
Galaty, J. G. (2013). The collapsing platform for pastoralism: land sales and land loss in Kajiado County, Kenya. Nomadic Peoples 17, 20–39. doi: 10.3167/np.2013.170204
Gikaba, J. M., Muthoni, K. S., and Bebe, B. O. (2014). Influence of drought duration on livestock feeding practices by Maasai pastoralists in Kajiado County, Kenya. Int. J. Innov. Appl. Stud. 8, 225–231,
Gikaba, J. M., Muthoni, K. S., and Bebe, B. O. (2015). Influence of changes in rainfall patterns on livestock feeding practices in Mailwa sub-location, Kajiado County, Kenya. Br. J. Appl. Sci. Technol. 7, 134–140. doi: 10.9734/bjast/2015/12527
Gitonga, P. N., Gachene, C. K., Njoroge, E., and Thumbi, S. M. (2016). Small ruminant husbandry practices amongst Kajiado and Marsabit pastoralists and their effects on peste des petits ruminants control strategies. Livest. Res. Rural. Dev. 28,
Government of Kenya (GoK). (2011). Vision 2030. Development strategy for northern Kenya and other arid lands. Government of Kenya.
Government of Kenya (GoK). (2013a). Kajiado County integrated development plan. Government of Kenya.
Government of Kenya (GoK). (2013b). Sector plan for agriculture second medium-term plan 2013–2017. Government of Kenya.
Government of Kenya (GoK). (2017). Climate risk profile for Kajiado County. Kenya County climate risk profile series. Government of Kenya.
Homewood, K. (2006). As pastoralists settle: social, health and economic consequences of pastoral sedentarization in Marsabit District, Kenya. Hum. Ecol. 34, 297–299. doi: 10.1007/s10745-006-9018-0
Irungu, P., Mbogo, S., Staal, S., Thorpe, W., and Njubi, D. (1998). Factors influencing adoption of Napier grass in smallholder dairy farming in highlands of Kenya. In Conference Proceedings of the 6th Bi-Annual Kenya Agricultural Research Institute (KARI) Scientific Conference.
Katiku, P. N., Kimitei, R. K., Korir, B. K., Muasya, T. K., Chengole, J. M., Ogillo, B. P., et al. (2013). Value chain assessment of small ruminant production, challenges, and opportunities: the case of southern rangelands of Kenya. Livest. Res. Rural. Dev. 25,
Kenya Food Security Steering Group (KFSSG) and Kajiado County Steering Group (KCSG). (2017). Kajiado County: 2017 short rains food security assessment report. Government of Kenya.
Kenya Food Security Steering Group (KFSSG) and Kajiado County Steering Group (KCSG). (2018). Kajiado County: 2018 long rains food security assessment report. Government of Kenya.
Kenya National Bureau of Statistics (KNBS). (2019). Kenya population and housing census, distribution of population by socio-economic circumstances.
Kihu, S. M., Gitao, G. C., Bebora, L. C., John, N. M., Wairire, G. G., Maingi, N., et al. (2015). Economic losses associated with peste des petits ruminants in Turkana County, Kenya. Pastoralism 5:9. doi: 10.1186/s13570-015-0029-6
Kimaru, J., Mutembei, H., and Muthee, J. K. (2021a). Effectiveness of drought risk reduction policies: case study of hay production in Kajiado County, Kenya. Am. J. Clim. Chang. 10, 512–532. doi: 10.4236/ajcc.2021.104026
Kimaru, J., Mutumbei, H., and Muthee, J. K. (2021b). Analysis of hay demand from pastoralism systems on the viability of hay production as a climate adaptation strategy in Kajiado County, Kenya. Agric. Sci. 12, 1089–1102. doi: 10.4236/as.2021.1210070
Kipaya, K. G., Nguhiu, P., and Munga, L. (2020). Socio-economic factors influencing the adoption of improved livestock farming practices among members of livestock cooperatives in Kajiado County, Kenya. World J. Innov. Res. 8, 1–9,
Köhler-Rollefson, I. (1997). Indigenous practices of animal genetic resource management and their relevance for the conservation of domestic animal diversity in developing countries. J. Anim. Breed. Genet. 114, 231–238. doi: 10.1111/j.1439-0388.1997.tb00509.x
Kosgey, I. S., Rowlands, G. J., van Arendonk, J. A. M., and Baker, R. L. (2008). Small ruminant production in smallholder and pastoral/extensive farming systems in Kenya. Small Rumin. Res. 77, 11–24. doi: 10.1016/j.smallrumres.2008.02.005
Lagat, P., and Nyangena, J. (2018). The effects of climate variability on livestock production in Kenya. J. Agric. Pol. 1, 58–79. doi: 10.47941/jap.105
Lefèvre, P. C., and Diallo, A. (1990). Peste des petits ruminants. Revue Scientifique et Technique (International Office of Epizootics) 9, 935–950. doi: 10.20506/rst.9.4.532
Lesnoff, M., Messad, S., and Juanès, X. (2016). 12MO: A cross-sectional retrospective method for estimating livestock demographic parameters in tropical small-holder farming systems v2.2. The French agricultural research Centre for International Development (CIRAD).
MacGregor, J., and Hesse, C. (2013). Pastoralism: Africa's invisible economic powerhouse? World Econ. J. 14, 35–63,
Mworia, J. K., and Kinyamario, J. I. (2008). Traditional strategies used by pastoralists to cope with La Nina induced drought in Kajiado, Kenya. Afr. J. Environ. Sci. Technol. 2, 10–14. doi: 10.5897/AJEST.9000125
Nyariki, D. (2017). Assessment of the total economic valuation of pastoralism in Kenya. The intergovernmental authority on development (IGAD).
Nyariki, D. M., and Amwata, D. A. (2019). The value of pastoralism in Kenya: application of total economic value approach. Pastoralism 9. doi: 10.1186/s13570-019-0144-x
Nyariki, D. M., and Ngugi, R. K. (2002). A review of African pastoral production systems: approaches to their understanding and development. J. Hum. Ecol. 13, 237–250. doi: 10.1080/09709274.2002.11905539
Ojango, J. M. K., Oyieng, E. P., Audho, J., and Okeyo, A. M. (2014). Indigenous sheep to help improve market access and livelihood security among pastoralists in Kenya: Results of a baseline survey : International Livestock Research Institute (ILRI).
Peeler, E. J., and Wanyangu, S. W. (1998). Infectious causes of small ruminant mortality in Kenya: a review. Small Rumin. Res. 29, 1–11. doi: 10.1016/S0921-4488(97)00120-X
Raphael, K. (1987). Recall bias: A proposal for assessment and Control. International Journal of Epidemiology 16, 167–170. doi: 10.1093/ije/16.2.167
Rass, N. (2006). Policies and strategies to address the vulnerability of pastoralists in sub-Saharan Africa [pro-poor livestock policy initiative (PPLPI) working paper series no. 37]. Food and agriculture organization (FAO).
Roba, G. M., Lelea, M. A., Hensel, O., and Kaufmann, B. (2019). Elusive profits: understanding the economic performance of local traders in the pastoral small ruminant value chain in northern Kenya. Nomadic Peoples 23, 78–105. doi: 10.3197/np.2019.230105
Schneider, F. G. (2011). The shadow economy and shadow economy labor force: What do we (not) know? [discussion paper 5769]. Institute for the Study of labor (IZA).
Singh, R. P., et al. (2004). ‘Prevalence and distribution of peste des petits ruminants virus infection in small ruminants in India’, Revue Scientifique et Technique de l’OIE, 23, pp. 807–819. doi: 10.20506/rst.23.3.1522
Taylor, W. P. (1984). The distribution and epidemiology of peste des petits ruminants. Prev. Vet. Med. 2, 157–166. doi: 10.1016/0167-5877(84)90059-X
Van de Steeg, J., Herrero, M., Kinyangi, J., Thornton, P., Rao, K. P. C., Stern, R., et al. (2009). The influence of climate variability and climate change on the agricultural sector in east and Central Africa - sensitizing the ASARECA strategic plan to climate change (research report no. 22). International livestock research institute (ILRI).
Keywords: economic performance, gross margin analysis, mortalities, pastoralist, small ruminants, simulation model
Citation: Arnold J-C, Onono J, Ballesteros C, Aboge G and Alarcon P (2024) Modeling the economic performance of small ruminant pastoralist flocks and financial impact of changes in reproductive performance and mortalities in Kajiado county, Kenya. Front. Sustain. Food Syst. 8:1406864. doi: 10.3389/fsufs.2024.1406864
Edited by:
Christian Bux, University of Bari Aldo Moro, ItalyCopyright © 2024 Arnold, Onono, Ballesteros, Aboge and Alarcon. This is an open-access article distributed under the terms of the Creative Commons Attribution License (CC BY). The use, distribution or reproduction in other forums is permitted, provided the original author(s) and the copyright owner(s) are credited and that the original publication in this journal is cited, in accordance with accepted academic practice. No use, distribution or reproduction is permitted which does not comply with these terms.
*Correspondence: Jean-Christophe Arnold, amFybm9sZDE4QHJ2Yy5hYy51aw==
†Senior author