- 1Department of Plant, Food and Environmental Sciences, Faculty of Agriculture, Dalhousie University, Truro, NS, Canada
- 2Department of Agricultural Economics, Obafemi Awolowo University, Ile-Ife, Nigeria
- 3Disaster Management Training and Education Centre for Africa, University of the Free State, Bloemfontein, South Africa
- 4Department of Microbiology, Hezekiah University, Umudi, Nigeria
- 5Department of Agricultural Extension and Rural Society, Faculty of Agriculture, Mansoura University, Mansoura, Egypt
- 6Department of Plant Production, College of Food and Agriculture Sciences, King Saud University, Riyadh, Saudi Arabia
Climate change is a major challenge impacting food security globally. Sub-Saharan African (SSA) countries including Nigeria has experienced the negative effect of climate vagaries most especially on agricultural production, thus, leading to food insecurity. However, sustainable land management (SLM) practices have a huge potential to minimize the impacts on food security in a rapidly changing climate. This study estimates the determinants of the adoption of SLM practices and the impact of adoption on household food security among smallholder rice farmers in Ogun State, Nigeria. A multistage sampling procedure was used to select 120 respondents. A Poisson endogenous treatment (PET) model was employed to analyse the determinants of level of adoption of SLM and impact of SLM adoption on household food security level of smallholder rice farmers in the study area. To account for counterfactuals, a doubly-robust augmented-probability-weighted regression adjustment (APWRA) was also used. In the same vein, the study employed the marginal treatment effects (MTE) approach to estimate the treatment effects heterogeneity. The results showed that socio-economic factors greatly influenced the adoption of SLM practices, such as age and educational level of farmers. The effect of SLM adoption on food security of smallholder farmers was found to be improved when they used SLM package consisting of variety of practices, hence, SLM practices have the potential to alleviate food insecurity among rice farmers if well combined and used to a large extent. The study concluded that knowledge in form of formal education, some form of vocational training, and trainings to access weather information were key to influencing SLM adoption among smallholder farmers in the study area. The treatment effects on untreated (ATU) are lower than that of ATE and ATT, confirming the positive selection on unobserved gains. In particular, the ATU results show that for an average non-adopting household, adoption of SLM practices would significantly improve dietary diversity by about 27%. Farm-level policy efforts that aims to equip farmers through education, trainings and disseminating information on climate change would be a huge step towards the promotion of SLM practice which eventually leads to increased food security. The study recommended that continuous adoption and extensive use can be fostered by encouraging farmers to join a social organisation where related and relevant information on sustainable land management practices is shared through trained agricultural extension officers.
1 Introduction
In developing nations, agricultural output needs to rise in order to feed a growing population. Studies indicate that managing land sustainably could boost food production without harming water and soil resources (Hlatshwayo et al., 2023; Xiong and Li, 2024; Sheikh et al., 2024). Agroforestry, terraces, minimal soil disturbance, organic fertilization, water harvesting and conservation, and the integration of residues are examples of improved agronomic techniques (Leng et al., 2024; Srivastav et al., 2024). Nearly half of the world’s population, including 50–90% of those in developing nations, resident in rural regions and depend heavily on agriculture for their livelihood (Munaweera et al., 2022; Ben Hassen and El Bilali, 2022). As posited by Xu et al. (2021), an estimated 821 million people in the world were undernourished in 2017; at least one person out of every nine in the world (FAO et al., 2018). In Africa, the situation is more pressing in the region of sub-Saharan Africa (SSA) where an estimated 23.2% of the population or between one out of four and one out of five people in the region may have suffered from chronic food deprivation in 2017 (World Health Organization, 2018). The number of undernourished people in SSA countries, Nigeria inclusive, rose from 212.2 million in 2014 to almost 256.5 million in 2017, an increase of 20.9 percent in 3 years (FAO et al., 2018).
Nigeria is one of the West African countries experiencing food insecurity (Ukonu et al., 2024). The estimated that 12.1 million Nigerians are in a food insecurity crisis, and it is feared to increase to 16.9 million people if humanitarian support and government interventions are not scaled up. Although endowed by nature with extensive land mass, varieties of crops in different ecological zones with optimal yield, oil wells and increasing population, harnessing these resources to provide national food sufficiency has proved problematic (Oriola, 2009). This has been exacerbated by the COVID-19 pandemic, civil insecurity (Krishnamurthy et al., 2020), bad governance and corruption (Igbinedion and Aihie, 2015) and land degradation in Nigeria (Adenle et al., 2022). In accordance with the 2020 global food security index, Nigeria’s food insecurity status is considered serious in the severity chart (von Grebmer et al., 2020). The Federal Ministry of Agriculture of Nigeria in 2014 estimated that 65% of the population is food insecure despite having more than half of all employment’s dependent on agriculture (El-ladan, 2014). Among several other factors, heightened food insecurity among farm households is caused by limited access to credit, poor storage and improved agricultural facilities, and negative environmental influences such as erosions and floods (El-ladan, 2014). Other reasons include the lower household income necessary for food purchases needed to attain food security (Osabuohien et al., 2018; Ogunpaimo et al., 2021; Ojo et al., 2022), a huge reliance on imported food items (Adeniyi and Dinbabo, 2019) and land degradation (Adenle et al., 2022). The 2030 United Nations Sustainable Development Goals are new global policies with the objective to restructure regional and national development plans over the next 10 years. The global policy aims to put an end to poverty and hunger, food insecurity, sustaining natural resources and the environment, and promote food and agriculture sustainability (SDG, 2019).
Nigeria, in West Africa, experiences one of the highest rates of land degradation with biomass decline amounting to about 400,000 ha/year and losses of agricultural productivity (Ridder, 2007; main report). Demand for agricultural land displaces forests or leads to agricultural productivity losses (Arowolo et al., 2018), which drives degradation in remote areas (Adenle and Ifejika Speranza, 2020). Land degradation has been the critical challenge for SSA countries. The causes of land degradation are complex and vary from place to place. Land degradation is considered major threat to the survival and livelihoods of millions of people in SSA (Maja and Ayano, 2021; Amoako Johnson and Hutton, 2014). Besides, addressing the intricate causes of the prevailing land degradation problems remains a critical policy challenge for Nigeria since its economy greatly relies on subsistence agriculture. These land degradation problems have also far-reaching economic, social, and environmental influences (Pender and Gebremedhin, 2008). With regard to cost of land degradation, various estimates show that it costs a considerable proportion of a country’s national income. Accordingly, sustainable land management practices (SLMPs) play a vital role in the sustenance of food production by addressing the effect of climate change on soil and land use, and improving land degradation (Hermans and McLeman, 2021; Sekaran et al., 2021).
In addition, in the absence of effective sustainable land management (SLM) practice, it is less likely to eradicate poverty. Sustainable land management practices (SLMPs) are important for ensuring environmental protection, food security, poverty alleviation, and economic growth in a nation. While Sustainable Land Management (SLM) has emerged as a widely accepted approach for addressing land degradation in agroecosystems, the use of SLM practices remains low among smallholder farmers. The ability of agricultural innovation, including appropriate technologies, to improve long-term food security in Africa is closely related to diffusion progression and the conditions for adoption that smallholders face. However, adoption of SLMPs is hampered by institutional factors such as credit and adequate extension services and information (Thinda et al., 2020). High costs paid for chemical inputs are also beyond the financial capacities of the poor smallholder farmers across the continent (Mthethwa et al., 2022; Gwacela et al., 2024).
As reviewed, despite the abundance of research works in SLM and its crop productivity effect, the studies are extensively oriented towards the initial adoption but with no attention to the intensity of adoption of SLM practices (Mutenje et al., 2019; Ojo et al., 2022; Oduniyi et al., 2023; Mthethwa et al., 2022; Mncube et al., 2023; Nyam et al., 2024). These studies explored the determinants and intensity of SLM practices and climate -smart agriculture (CSA), respectively in South Africa and Nigeria. However, the studies failed to simultaneously consider the impact of adoption of either SLM on household food security. Therefore, this present study evaluates the factors affecting smallholder rice farmers’ decision and intensity of adoption of SLM practices and the impact of adoption on household food security. Thus, studying the simultaneous adoption behavior of farmers and the intensity of the use of SLM practices vis-à-vis the impact of adoption on household food security would be helpful to the existing body of knowledge. This study is imperative as it would help to better understand the households’ decision behavior towards land management practices on farm plots as well as institutional and biophysical factors that affect such decisions. The study will also provide information about impact of adoption of SLMPs on household food security in Ogun State area of Nigeria by accounting for selection bias (Cornelissen et al., 2016; Shahzad and Abdulai, 2021), marginal treatment effects approach. The MTE approach considers both observable and unobservable factors influencing the adoption of SLM practices. It also helps estimate treatment effect heterogeneities by showing the MTE curve against various degrees of unobservable resistance to SLM adoption.
2 Conceptual framework
2.1 The determinants of adoption of sustainable land management practices by smallholder rice farmers
Empirical studies have hypothesized that both adoption and intensity of SLMP are influenced by household socio-demographic characteristics and other forms of institutional factors (Adeagbo et al., 2021; Oduniyi et al., 2023). The framework of the double-hurdle model incorporates a first stage adoption of SLMP based on the same set of covariates determining the adoption of SLM practices.
With the assumption of the error terms in the equations is uncorrelated conditional on all covariates, the standard errors from separate estimations are also valid for conducting statistical inference. If the conditionally uncorrelated errors assumption does not hold, coefficient estimates from separate regressions will be biased (Heckman, 1977; Harding et al., 2020). According to testing for conditionally uncorrelated errors follows the same method as well as the Heckman test for selection bias. Although it is not technically necessary for identification, it is standard to impose at least one justifiable exclusion restriction when estimating the second stage. The null hypothesis that the first and second stage errors are conditionally uncorrelated is tested using the standard t-statistic for the coefficient estimate on inverse mill ration (IMR). If the coefficient estimate is statistically significantly different than zero, the null hypothesis is rejected and the model must be re-estimated to conduct valid inference (De Luca and Perotti, 2011). Failure to reject the null, the second stage parameters excluding IMR is re-estimated. A probit model of SLM for selection equations is estimated using a function of explanatory variables that are likely also determine SLM intensity, vis-a-vis one or more exclusion variables. The IMR predicted from the first-stage probit regression is added as a regressor to account for the selection bias in the second hurdle. Following Adeagbo et al. (2021), adoption of SLM practices can be stated as the stage at which a household decides to adopt one or more adaptive option in mitigating the effect of climate change. The underlying latent variable that captures the true farmers’ socio-economic characteristics is hypothesized to determine the probability of adoption of SLM by a smallholder farmer. The regression Equation 1 indicates the latent variable :
and, if
if
where is a categorical variable that takes the value of 1 if a smallholder farmer adopts SLM practices and 0 otherwise. α is a vector of parameters to be estimated. In line with Wooldridge (2002), a probit model of which follows random utility is as expressed in Equation 2:
where, equals 1 for households that adopts SLM practices and 0 otherwise; represents the vector of independent variables; α, vector of parameters to be estimated; , standard normal cumulative distribution function; ei is a random error term hypothesized to be distributed normally with unit variance and zero mean.
2.2 The intensity of SLM practices among smallholder rice farmers
Ordinary least squares (OLS) regression cannot accurately estimate count data since they are non-normal (Maddala et al., 2001). The Poisson regression model (PRM), the negative binomial regression model (NBRM), the zero-inflated Poisson (ZIP), and the zero-inflated negative binomial model are the most popular regression models used to assess count data models (ZINB). Regression models with non-negative integer responses are typically analyzed using the PRM and NBRM models (Greene, 2008; Kirui et al., 2013). In contrast to the situation in this study, the final two (ZIP and ZINB) are specifically employed to account for scenarios with frequent zero counts (i.e., where there are more zeros than would be expected). Only the PRM is therefore discussed here since the response variables were non-negative integers and with only a few zero counts.
In implementing any given technology, smallholder farmers frequently make logical selections Zhang and Zeng (2021). Farmers typically consider the advantages of a specific technology before adopting it since their goal is to maximize predicted (discounted) profits over time subject to input and commodity prices and technological constraints. A farmer will rationally accept new technology if the projected (discounted) value of profits from employing it exceeds utility from the existing technique (Channa et al., 2019). Using a Poisson model, the intensity of SLM’s determinants were estimated. The Poisson model is the most straightforward and likely the most popular approach for modeling counts variables (Cameron and Trivedi, 2001; Siegfried and Hothorn, 2020). In this study, Poisson regression was adopted since diagnostic tests showed that there was neither overdispersion or under dispersion. Following Wooldridge (2002) and Greene (2008), the density function of the Poisson regression model is given in Equation 3 by:
Where; and M = 0,1,…, is the number of SLM used by the farmers and D vector of predictor variables and Υ and 𝛩 are the parameters to be estimated.
Greene (2008) show that the expected number of events 𝛿 (in this case, number of SLM practices adopted by the farmers) is given in Equation 4;
2.3 Impact of SLM practices adoption on household food and nutrition security
This section presents a framework to illustrate how adoption decisions of farm household’s impact on household food security. The main hypothesis of the study is premised on the adoption of SLMP on food and nutrition status of small-scale crop farmers in the study area. The adoption decision is expected to impact food and nutrition security status of households. Considering the outcome variable, HDDS, as a linear function of the treatment variable (i.e., SLMP) and a set of other covariates X, then the linear equation (5) is expressed as:
Where indicates a measure of food and nutrition security, is set of explanatory variables, SLMPi is an indicator variable for SLMP adoption, and denote parameter vectors and is an error term. The estimated parameter measures the causal effect of SLMP adoption on farm performance if and only if adoption and non-adoption of SLMP is random (Bello et al., 2024). However, the decision to adopt SLMP is mostly influenced by unobservable characteristics such as motivation/inherent abilities, managerial skills and risk preferences. As a result, it cannot be random as these observed and unobserved characteristics may have correlations with error term of the outcome variables. For example, a skewed bias is most likely to occur if farmers with high managerial skills or inherent abilities adopt SLMP. Similarly, farmers with high educational attainment may easily access information leading to SLMP adoption, which can result in upward biasedness. This is because farmers self-select themselves into adoption and farmers with high educational level and highly motivated are likely to have undue advantage over their counterparts with low educational attainment and low self-esteem or managerial skills. This also suggests that adoption of SLMP is potentially endogenous. Consequently, a double-robust AIPW postestimation test different from PET model, following Cattaneo et al. (2013), was performed to obtain consistent estimates. Thus, the AIPW can ensure consistent results, as it permits the treatment and the outcome model to account for misspecification due to its double-robust property.1 However, there is strong evidence to support the notion that the adoption of SLMP is likely to be influenced by unobservable characteristics like, risk aversion, technical proficiency, and social capital, which may be correlated with the HDDS (Oseni and winters, 2009). Therefore, impact in the outcome variable’s distribution between the adopters and non-adopters may not only reflect the treatment’s effects but also variations as a result of selection process (Abadie, 2003). The marginal treatment effects can be defined as a function of the quantiles, or the treatment effect at a particular value of error term (Cornelissen et al., 2016). In estimating the marginal treatment model, this study follows the mathematical model employed by (Shahzad and Abdulai, 2021). The MTE method accounts for both observable and unobservable factors influencing adoption of SLMP practices based on propensity scores and plots the MTE curve against varying levels of unobservable resistance to SLMP adoption.
3 Research methodology
3.1 Study area
The study is conducted in Ogun State, a Southwestern part of Nigeria. Rice is one of the most important staple foods in Nigeria. Smallholder rice farming has an important role in achieving a sustainable livelihood and food and nutrition security in Nigeria. Rice is a staple food in Nigeria and the main source of agricultural income (Sanusi and Dries, 2024). Its production is dominated by smallholder farmers who are vulnerable to the risks of changing weather patterns, and land and environmental degradation (Vivek, 2019; Akanbi et al., 2022). Rising temperatures and changes in rainfall patterns have direct effects on crop yields and indirect effects through irrigation water availability, thus exacerbating the impacts of droughts, soil degradation and the decline in biodiversity (FAO, 2016). Economic activities in relation to the production and consumption of rice are being widely regarded a panacea for economic development and reducing poverty (Demont and Ndour, 2015). However, only about 57% of the 6.7 million metric tonnes of rice consumed in Nigeria annually is locally produced, leading to a supply deficit of about 3 million metric tonnes. Consequently, Nigeria is the leading importer of rice in the global market (Idris et al., 2024).
Ogun State in Nigeria is one of the major rice producing States in the nation with heavy reliance on rain-fed agriculture. This is evident as some areas in Lagos and Ogun States were said to have experienced an uncommon rainfall with thunderstorms in the early days of 2021, drawing attention to the fact that these regions record the highest number of industries in Nigeria. The challenge of climate unpredictability makes subsistence farming difficult (Etim et al., 2021; Ojo et al., 2022). South West Nigeria is one of the geopolitical zones of Nigeria consisting of Ekiti, Lagos, Ogun, Ondo, Osun and Oyo States. The zone lies between Latitude 4°North and 9°North of the equator and Longitude 3°East and 6.2° East of the Greenwich meridian, it is bounded on the North by Kwara and Kogi states, in the South by the Atlantic Ocean and in the West by the republic of Benin. The Southwest is characterized by a tropical climate with weather conditions varying between the dry and rainy seasons, the wet season runs from March till October while the dry season is shorter, it runs from November to February. The temperature ranges between 21 and 34°C while the annual rainfall ranges between 1,500 and 3,000 mm. The major inhabitants of South-West Nigeria are the Yorubas but there are also a lot of immigrants from neighbouring countries and other geopolitical zones, this is due to the conducive economic attributes that supports agricultural practices. The combined population of the zone is estimated at 32.5million people.
Ogun State borders Lagos State to the South, Osun and Oyo States to the North, Ondo State and the Republic of Benin to the West. Ogun State comprises varying dialects of the Yoruba language. Some important cities and towns in the state include Abeokuta, Ijebu-Ode, Sagamu, Ikenne Remo, Ilaro, Ijebu-Igbo, Aiyetoro, Ota etc. Abeokuta being the State’s capital is the most populous city. The State generally is noted for its high concentration of industrial estates being a major manufacturing hub in Nigeria, major factories in Ogun includes the Dangote Cement in Ibese, Nestle, May and Baker, Lafarge Cement factory in Ewekoro, amongst others. Ogun state is also noted for being an exclusive site of Ofada rice production. There are 20 local government areas in the state with each headed by a chairman. However, only eight out of these 20 local government are involved in rice production, Abeokuta North, Yewa North, Ewekoro Ifo, Ijebu-North, Ikenne, Obafemi Owode, and Ogun Waterside (Figure 1).
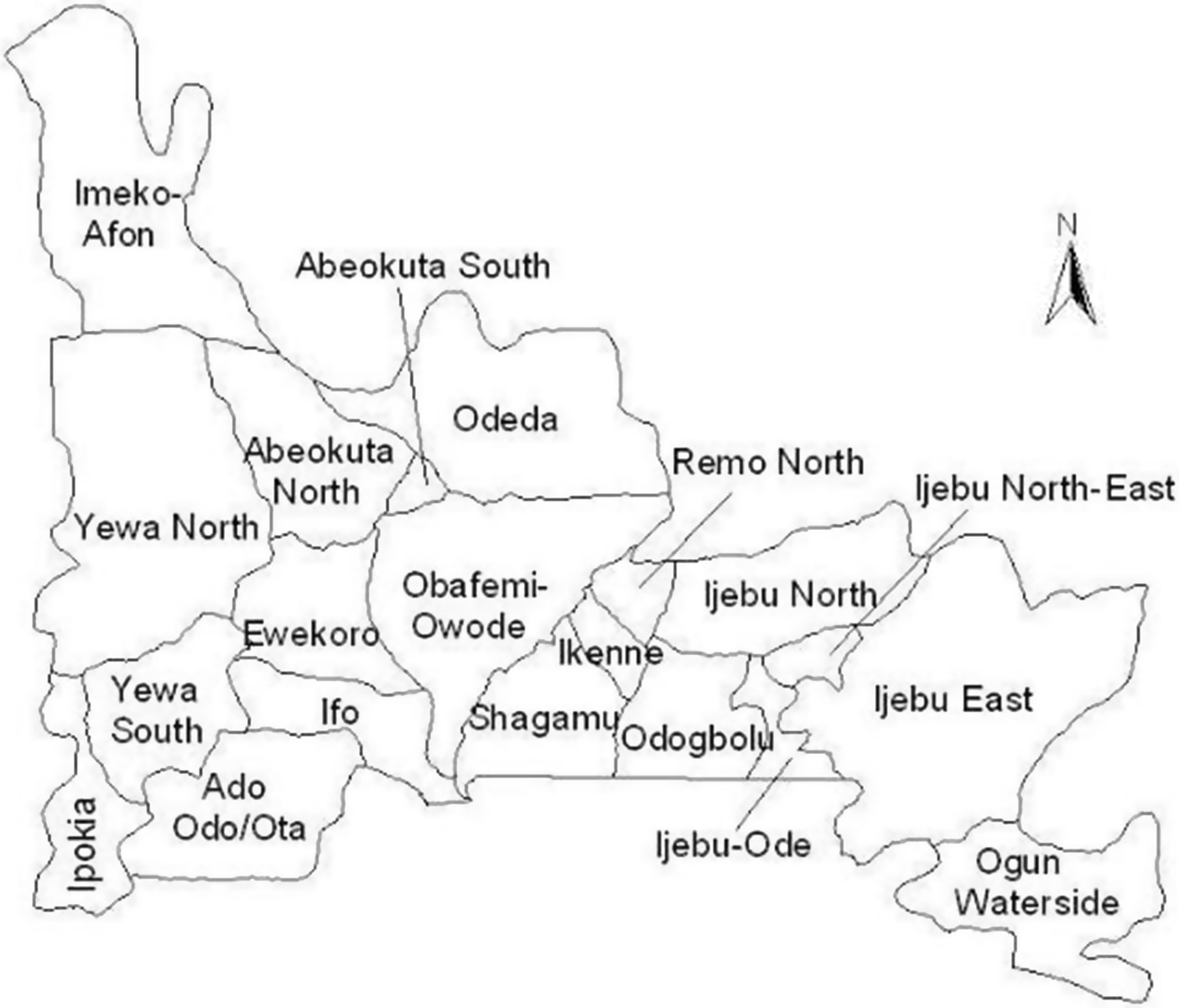
Figure 1. Map of Ogun State showing the local government areas. Source: Gbadebo (2012). Geochemical analysis of groundwater quality in Agbara and environs.
3.2 Sampling technique and sample size
The study employed a multistage sampling procedure that combined purposive and proportionate random sampling methods. The first stage involved the purposive selection of Abeokuta North, Yewa North, Ewekoro Ifo, Ijebu-North and Obafemi Owode local governments areas (LGAs) based on the predominance of rice farmers in the region. At the second stage, 3 villages in each local government were randomly selected from the rice-producing communities in the LGAs. At the final stage, 8 rice-farmers were selected from each of the communities using a simple random technique. A quick census of rice-farming households in the selected communities was carried out to get the sampling frame for each community and the targeted sample size of rice-farming households in the community. To determine the sample size, the study followed Slovin’s (1974) and Tejada and Punzalan (2012) formula. Slovin’s formula is as expressed:
Where n = sample size, N is total population, and e, margin of error. This study determined its sample size with 95% confidence level. Hence, margin of error is 5%. A total sum of one hundred and twenty respondents (120) was used for the study.
Data was obtained by carrying out both on-farm and home interviews. Because of the dynamics of rice farming in Ogun state which involves a huge form of accountability to the State’s government, it was necessary to liaise with extension officers in certain local governments in order to hasten the data collection process. Questionnaires were primarily made use of; schedule interviews and field observation were also employed. Primary data was collected from the respondents (rice farmers in Ogun State) with the aid of well-structured questionnaire. The survey questionnaire assessed the relevant socioeconomic characteristics of the farmers including their age, gender, primary and secondary occupation, data on the household dietary diversity score (HDDS) which speaks of the consumption frequency of diverse food groups was used to estimate the level of farmers’ food security through the measurement of household food access. Information on farmers’ choices of SLM practices were also collected.
4 Results and discussion
4.1 The socio-demographic characteristics of the smallholder rice farmers in Ogun state
This section reports the description of both dependent and the explanatory variables included in the model estimations (Table 1).
Age is a significant factor in agricultural output, according to Hoffert et al. (2002), because it affects the family head’s level of farming experience. The sampled household heads are 43 years old on average. Given that the average life expectancy in Nigeria is 51.9 years, this indicates that the respondents are still of an age where they can work. This adds to the findings of Musemwa et al. (2007), which suggested that the longer the household head has been in charge, the more sustainable the household economy has been. This is because older people have a relative wealth of experience in both the social and physical environment, as well as more farming experience. According to the gender analysis, men make up 80% of household heads. This suggests that men predominate in the research area’s rice production. The outcome also shows that 90% of household leaders are married. This demonstrates that if farmers had sufficient responsibilities, they would be required to commit to solutions for coping with climate change, which would eventually lessen their status of food insecurity. Zenda (2002), who stated that married households benefit from having partners who can work and help them in agricultural operations, supports the findings.
The average respondents in the study area spent approximately 14 years in school. This is in line with the minimum prescription of 9 years of basic education under the Universal Basic Education Programme in Nigeria. In relation to the source of income within the study areas, some households reported having one main source (89%), however, some reported that they had more than one source of income (52%). These findings are supported by Vernooy (2022) who state that households in the rural areas turn to look for other income sources so as to increase their household income and that rural area can diversify their income. About 66% of the rice smallholder farmers had access to credit, which is a major determinant in choosing adaptation strategies. However, there was noticeable variation in the access to information. For example, about 48% of farmers who adopted at least one strategy had access to information related to climate change.
Following Oduniyi (2022), Kolapo et al. (2022), it is important to understand and identify SLMP used by the smallholder rice farmers for implementation of feasible practices at farm level. Smallholder farmers employed different practices against land degradation (Figure 2). In response to land degradation, households in the studied area have developed multiple SLMP to mitigate the negative impact of land degradation. The following various methods were mostly employed, including soil erosion management (92%), minimum soil disturbance (85%), vegetation management (85%), integrated soil management (80%). Others were animal waste (76%), water management (75%), integrated farming (71%). Some of these strategies were also identified in the studies of Salaisook et al. (2020); Oduniyi (2022), Kolapo et al. (2022) and Oduniyi (2022). In categorising the adoption of SLMPs into adopters and non-adopters, the mean value was determined and used as a threshold. A smallholder rice farmer is an adopter of SLMP if the mean value is greater than or equal to 5 and 0, otherwise. The dichotomous variable of SLMP was then used as the dependent variable in the first hurdle (Probit) model.
In line with the outcome variable, the household dietary diversity score (Table 2). The results show that the adopters of SLMPs had a significantly higher HDDS than the non-adopters. However, the significant mean difference in the HDDS between the adopters and the non-adopters, does not translate to causal inferences about the adoption of SLMPs as a result of the significant differences in observable characteristics between the two groups, which could lead to selection bias. Hence, the choice of poisson endogenous treatment effects to control for observed and unobserved heterogeneity between the adopters and the non-adopters to allow us to establish the causal effects of the adoption of SLMPs.
4.2 Household food security score based on HDDS
Food consumption of respondents was measured qualitatively through the use of dietary diversity. According to Leser (2013), dietary diversity can be defined as the number of different food groups consumed over a reference time period. The food groups consumed over a 24-h recall period was used as the basis of household dietary diversity. Table 3 represents the responses registered from the food groups consumed by the household of the farmers in the study areas 24 h before the questionnaires were administered. As shown in Figure 3, results on the household dietary diversity score indicated that the rice farmers’ households examined were 70% food insecure, 13% food secure, 10% mildly food secure and 7% moderately food secure. This agrees with the recent survey carried out by National Nutrition and Health Survey (2018) and affirmed by Otekunrin and Otekunrin (2022) which showed that the nutritional status of households in Ogun State is generally poor; stunting, wasting and underweights were revealed to be currently at 29.6, 6.6, and 20.4%, respectively.
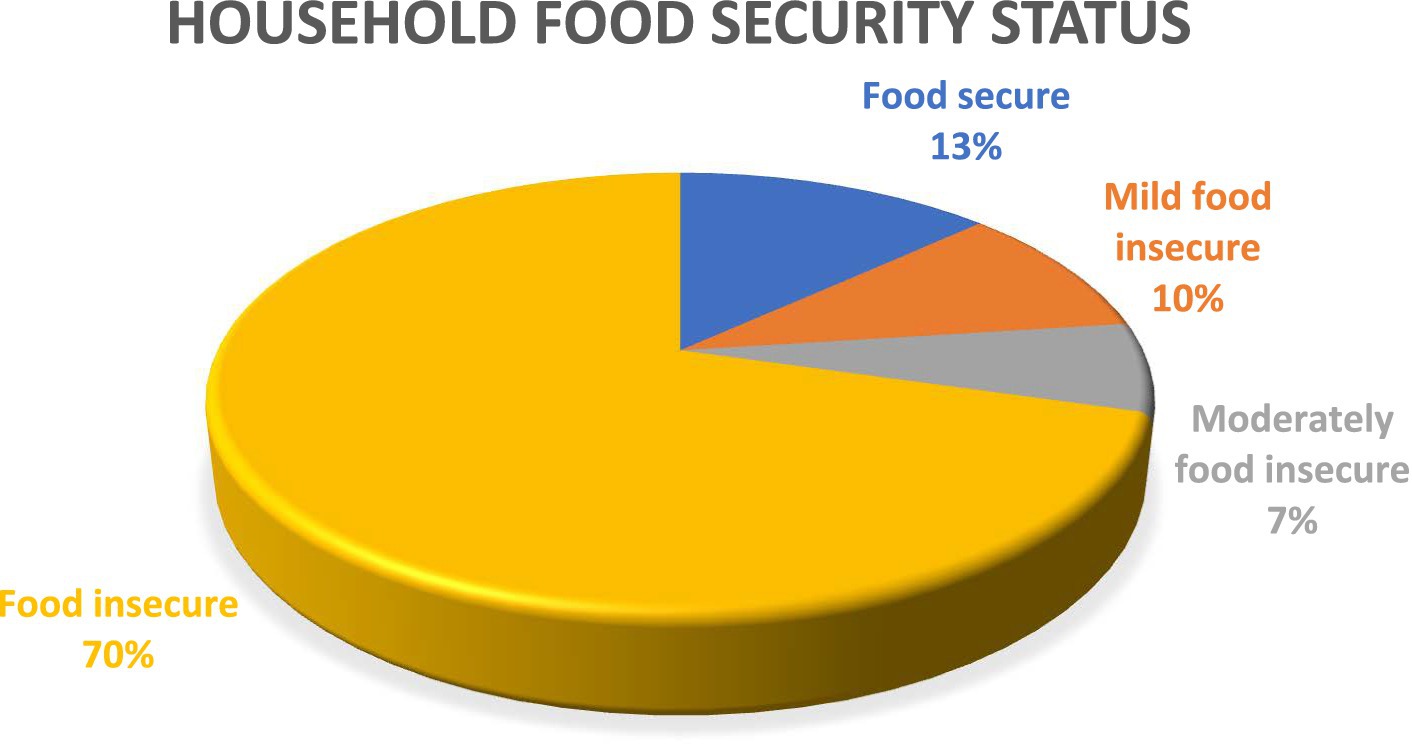
Figure 3. Household food security status. Responses with food items 0–3 are coded food insecure, 4–6, moderately food insecure, 7–9, mildly food insecure and 10–12 food items are coded food secure.
4.3 The household food security status in relation to adoption of SLMPS
The result of the relationship of household food security and adoption of SLMPs is presented in Figure 4. This result compares the household food security status of adopters and non-adopters of SLMPs.
The HDDS results score was categorized (food secure, mildly food insecure, moderately food insecure and food insecure). The results presented in Figure 4 show that adopters of SLMPs were more food secure compared to non-adopters. There are about 75% of the SLMPs adopters were food secure, 25% were mildly food insecure, 12.5% were found to be moderately food insecure with about 31.4% of the SLMP adopters were food insecure. In comparison to the food security status of non-adopters of SLMPs, the distribution of the food security status of adopters is better off than those households that did not use SLMP to mitigate the negative effects of land degradation.
4.4 The determinants of adoption of SLM practices among smallholder rice farmers (Probit regression model)
Table 4 showed the analysis and results of the Probit regression model (first hurdle).
The explanatory variables included in the model are: gender of respondent, age of respondent, availability of training by an organisation on how to access weather information, age of household head, marital status, household size, educational level, highest level of education received by any household member, years of farming experience, access to information, access to market, membership in any cooperative society, support from government on farm input, and access to good road networks. The probit analysis found six out of the 14 independent variables included in the model had relevant explanatory power on the determinants of SLM adoption. They included: availability of training by an organisation on how to access weather information, age of household head, household size, highest level of education received by any household member, support from government on farm input, and access to good road networks.
The coefficient of availability of training/education by an organisation on how to access weather information had a negative relationship with the adoption of SLM among smallholder farmers and it is significant at 10% level of significance. This implies that the adoption of SLM decreases with increased exposure to trainings on how to access SLMP information, this possible explanation could be based on the fact that farmers who assume that access to SLMP information are at their fingertips tend to be more laid back and not proactive in implementing SLM practices. This however contradicts the findings of Ndamani and Watanabe (2016) who argued that farmers who have been trained are more knowledgeable as a result of their ability to readily access climate change information and those involving adaptation options.
The coefficient of age of household head had a negative relationship with SLM adoption and it is significant at 5% level of significance. This implies that farmers’ capacity to adopt SLM decreases with age. This is in line with the findings of Oduniyi (2022) who established that young farmers are more energetic, risk-tolerant which gives them the platform to implement different and newer practices relating to climate change adaptation. The coefficient of household size (in numbers) had a negative relationship with the adoption of SLM and it is significant at 5% level of significance. This implies that as the farmers’ household size increases, the likelihood of adopting SLM would decrease. The negative and significant effects of the household size could be attributed to the reduced financial capacities of larger households, this is in line with the findings of Rochecouste et al. (2015) which opined that the technologies required for the successful implementation of SLM practices are often expensive, hence, smallholder farmers’ ability to access and use them are limited.
The coefficient of highest level of education attained by any household member had a positive relationship with the adoption of SLM and it is significant at 1% level of significance. This suggests that in increase in the education level of farmer or any member of his household such as the children significantly increases the probability of adopting SLM. Exposure to education and training is thought to enhance cognitive ability, which is consistent with numerous studies that show that farmers’ educational attainment generally correlates with their ability to adopt technological innovations due to the presumption that knowledge is linked to farmers’ ability to make wise decisions (Dung et al., 2021; Fadina and Barjolle, 2018; Kien et al., 2023; Santoso et al., 2023). As a result, in situations where a farmer is lacking in this area, the presence of a household member who is competent could give the farmer an advantage over his less educated competitors. This shows that education level significantly affects ability to receive and process technical information that is coherent with implementing SLM.
At the 5% level of significance, there is a statistically significant negative correlation between the availability of government subsidy for agricultural input and the adoption of SLM. This suggests that government assistance in the form of farm inputs provided to rice farmers in the study area reduces farmers’ propensity to use SLM. This is in contrast to the findings of Anuga et al. (2019), who found that because SLM investments have the ability to increase production capacity, the government’s provision of SLM inputs and finances to farmers is vital in boosting SLM investments. The adoption of SLM by smallholder farmers in the research area was positively correlated with access to a good road network, and this correlation is statistically significant at the 10% level of significance. This suggests that access to a good road network enhances SLM adoption.
4.5 Determinants of level of adoption of sustainable land management practices among smallholder rice farmer (Poisson regression)
The empirical results of the Poisson regression (second hurdle) while estimating the determinants of the level of adoption of SLM among rice farmers in the study area is presented in Table 5.
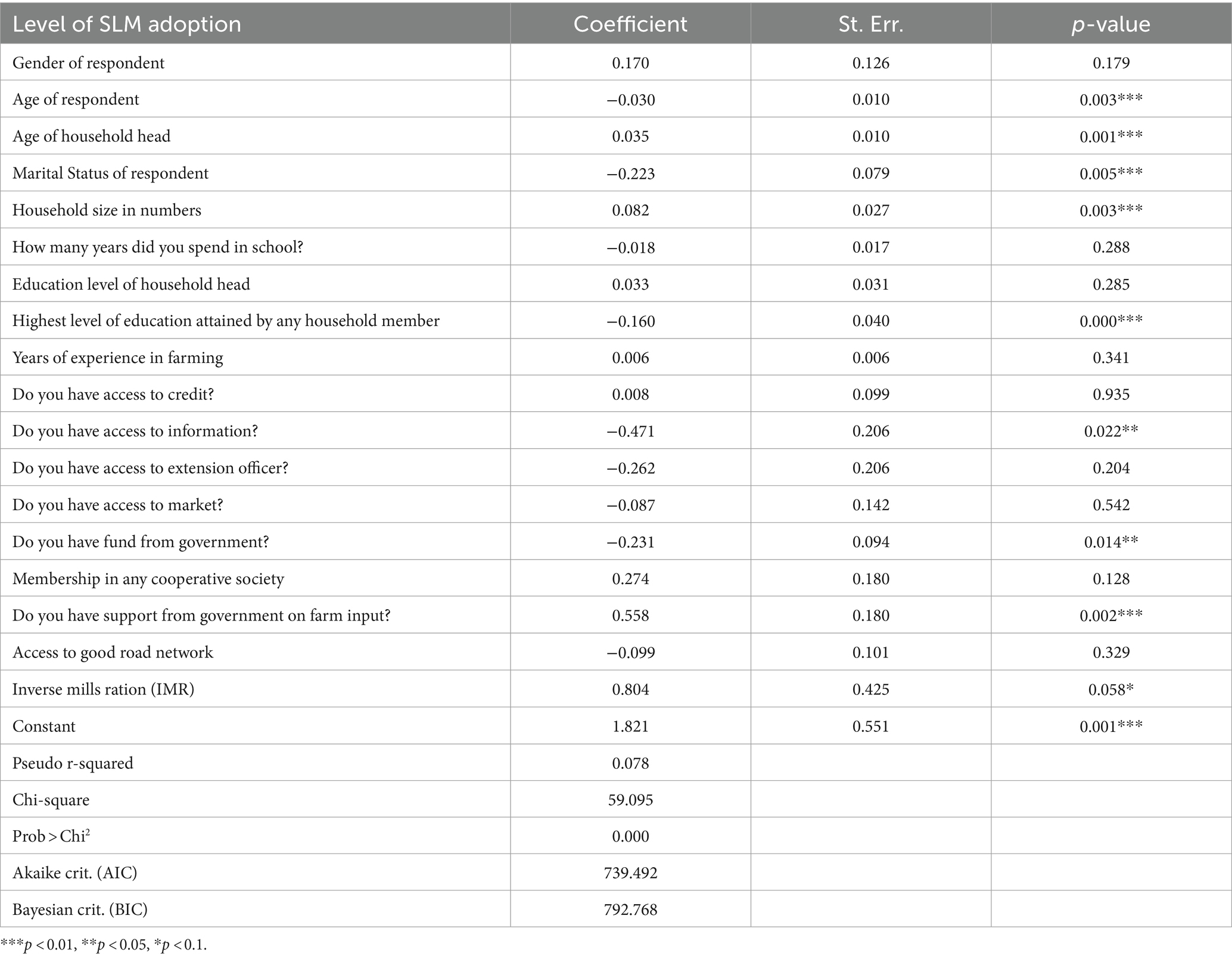
Table 5. Determinants of level of adoption of SLM among smallholder rice farmers-Second-hurdle (Poisson regression).
The explanatory variables included in the model are: gender of respondent, age, marital status, household size, years spent in school, education level of household head, highest level of education attained by any household member, years of farming experience, access to credit, access to information, access to extension officer, access to market, availability of funds from the government, membership in a cooperative society, support from government on farm inputs and access to good roads. The Poisson regression model found that eight out of the 17 independent variables included in the model were significant in influencing the intensity of adoption of SLM. These factors include the respondent’s age, the age of the household head, marital status, the size of the household, the highest education level obtained by any household member, access to information, availability of government funding, and government support for farm supplies. The selectivity issue was indicated by the inverse mill ratio (IMR), which is depicted in the table, and by its significance, which is 10%. The selectivity issue was fixed in the first hurdle and fitted in the second hurdle of the model by the introduction of the IMR.
The level of SLM adoption by smallholder farmers was negatively correlated with the respondent’s age, and this correlation is statistically significant at the 1% level of significance. This suggests that the degree at which SLM is adopted lowers as respondent age increases. Age does undoubtedly have a role in how motivated and interested farmers are to adopt new technologies like SLM. This shows that younger farmers are more likely to do so than their older counterparts. This is in line with the findings of Fahad and Wang (2018); Begum et al. (2023). which support the idea that farmers’ ages are unfavorably correlated with their use of adaptation techniques.
The level of SLM adoption was positively correlated with the household head’s age, and this correlation is statistically significant at the 1% level of significance. This suggests that the possibility of SLM adoption increases as the household head’s age rises. Contrary to the findings of Goli et al. (2023) which imply that household heads’ ages are negatively and not favorably correlated with their degree of adoption, this suggests that older farmers will perform better in their level of SLM adoption. It is however worthy of note that the effect of farmers’ age on the adoption of SLM practices has been accompanied by varying results; found a positive correlation, a negative association was deduced by Wang et al. (2023); Zeleke et al. (2023). The influence of age on adoption of SLM practices was ruled out as an insignificant correlation according to the findings of (Mirzabaev et al., 2023).
The coefficient of the respondent’s marital status had a negative relationship with the level of adoption of SLM and is statistically significant at the 1% level of significance. This indicates that the respondent’s marital status lowers the level of adopting SLM, which may be related to the responsibilities associated with excelling in new innovations like SLM (Fentahun et al., 2023). For instance, it was discovered that younger men in the study area went above and above to embrace SLM techniques and make sure they were productive. In terms of level of adoption of SLM, the household size had a positive correlation that was statistically significant at the 1% level of significance (Asfew et al., 2023). This suggests that adoption of SLM was more prevalent in households with bigger populations. This may be related to more readily available and less expensive labor in the form of household members’ assistance with the completion of pertinent tasks.
The coefficient of the highest level of education attained by any household member had a negative relationship with the level of adopting SLM and it is statistically significant at 1%. This suggests that the chance of SLM adoption is decreased by the level of education gained by the farmer or anyone living in his household. This finding runs counter to the majority of studies that link educational exposure to higher levels of SLM adoption. For example, Nkegbe et al. (2017) indicated that higher education levels maintain household heads well-informed and aware of available better agricultural technology like SLM. In the same vein, Asfaw et al. (2018) established that well educated farmers have better understanding of climate change and related issues and are better able to adapt to it by taking up new innovations readily.
Smallholder farmers’ adoption of SLM was negatively correlated with their level of information access, and this association is statistically significant at a 5% level of significance. This suggests that access to information lowers the level of SLM adoption. This is at odds with research that claim farmers are more likely to embrace climate change adaptation measures if they have access to radio, mobile phones, and the media than if they do not (Thinda et al., 2020). Mulwa et al. (2017) further supported the notion that having access to trustworthy information sources helps farmers become more climate-adaptable (Shilomboleni et al., 2024). The coefficient of access to fund from government had a negative relationship with the level of SLM adoption and it is statistically significant at 5% level of significance. This suggests that the likelihood of SLM adoption decreases when farmers gain access to government funding. Farmers’ propensity to use these monies for their own projects and to meet personal needs may be one explanation for this. The findings of Anuga et al. (2019) and Ndamani and Watanabe (2016), which suggested that funding from the government is a crucial institutional determinant to allow simple acceptance and upgrading of SLM methods at all levels, are at odds with this negative association. This connection was confirmed by Teshome et al. (2016), who noted that the improper use of public funds might be a problem that would hinder the implementation of SLM.
The level of SLM adoption was negatively correlated with the coefficient of government support for farm inputs, which is statistically significant at the 1% level of significance. This suggests that government assistance for farm inputs lowers the rate of SLM adoption. The plausible reason for this result could be attributed to the fact that access to new improved varieties that are suitable for a particular ecology requires a huge sum of money which could highly expensive for an average farmer to afford. However, the government is yet to meet up with the demand of procuring these varieties and making it available to the farmers at subsidized rate as a means assisting the local farmers in boosting rice production in Ogun State (Osabuohien et al., 2018).
4.6 Impact of SLM adoption on household food security—poisson regression with endogenous selection
Table 6 showed the analysis and result of the Poisson regression with endogenous treatment.
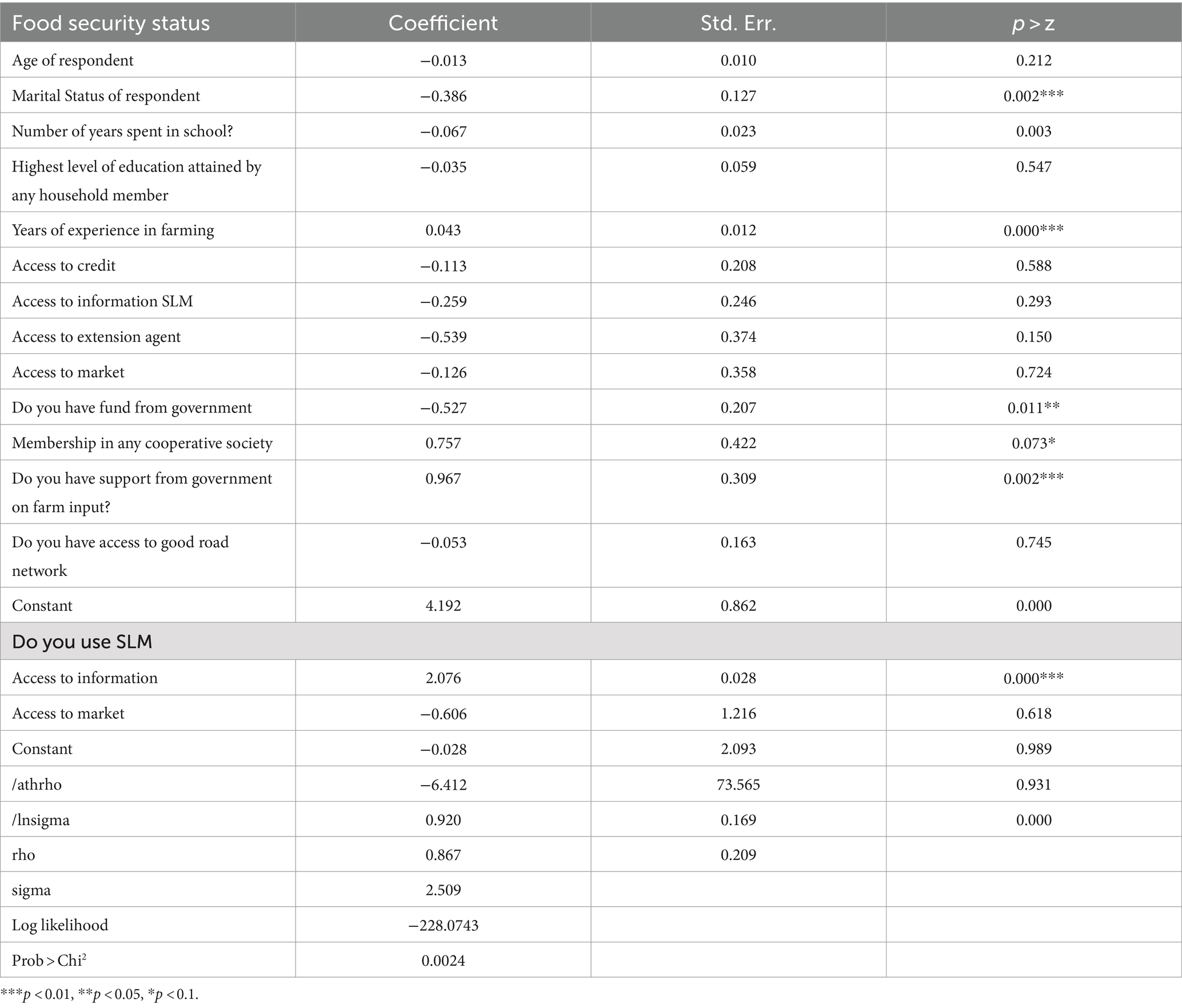
Table 6. Impact of SLM adoption on household food security—poisson regression with endogenous selection.
The predicator variables included in the model are: age of respondents, marital status, years spent in school, highest level of education attained by any household member, years of experience in farming, access to credit, access to information, access to extension officer, access to market, fund from government, membership in any cooperative society, support from government on farm inputs, access to good road network and access to information, From the 15 predicator variables fitted in this model, six predictor variables had a statistically significant influence on household food security. They include: marital status of respondents, years of farming experience, availability of fund from government, membership in cooperative society, support from government on farm input and access to information.
Farmers’ food security was negatively correlated with their marital status, and this correlation is statistically significant at the 1% level of significance. This suggests that the likelihood of the farmers having access to food is decreased by marital status. The plausible reason could be traceable to the fact that most of the respondents are in the rural area where household food preparation decisions are often left to wives to execute. However, this differential can also be attributed to the types of food grown and consumed in rural areas. This result resonates with the studies of Cordero-Ahiman et al. (2021) and Kolliesuah et al. (2023) who posited that rural households are mostly accustomed to the limited number of foods they grow which tend to also limit their understanding. However, this is in variance to the assumption that married households do better because they have the chance to decide together on the production and consumption of a wide variety of meals (Ojo et al., 2022). According to Ngema et al. (2018), married households have better food security for their caregiver than single households do.
At a 1% level of significance, the years of agricultural experience demonstrated a positive correlation with food security. This suggests that having more years of farming experience enhances the possibility of the farmers having a stable supply of food. This may be related to increased financial capability due to years of resource accumulation, which then allows for a variety of consumption options. This supports the research by Danso-Abbeam et al. (2022), which highlights the value of agricultural expertise in raising farmers’ chances of adapting. Farmers’ adoption can be linked to increase in productivity which then transcend to increased level of food security. Availability of fund from government is statistically significant at a 5% level of significance and a negative relationship exists between it and the food security of farmers. This implies that farmers’ access to government funds diminishes their chances of being food secure. A possible explanation could be based on the misuse of funds which is bound to occur among smallholder farmers who have large families and income non-proportional to fend for them.
The coefficient of farmers’ membership in cooperative society had a positive relationship with food security and it is statistically significant at 10% level of significance. This implies that farmers’ belonging in cooperative societies tend to improve their chances of being food secure. The importance of social networks is such that farmers can rely on their association for support especially in times when there is critical need for them and also access relevant information that aims to improve their productivity and food security status. The findings of Kehinde and Ogundeji (2022) reiterates the significance of farmers’ membership in an association.
The coefficient of support from government on farm input had a positive relationship with the level of food security of rice farmers in the study area and is statistically significant at 1% level of significance. This implies that government’s support to farmers in form provision of inputs to them increases the food security status of the farmers including that of their households. This is in line with the findings of Khatri-Chhetri et al. (2017) who opined that the cost of implementing technologies could influence farmers’ preferences and willingness to pay. This suggests that farmers may be prevented from adopting technologies which carry the potential of increasing their level of food security due to financial incapacity, hence, support from farmers in form of farm inputs could lessen the possibility of farmers being excluded from the possibility of being food secure. The coefficient of access to information had a positive relationship with the food security status of the farmers, it is statistically significant at 1% level of significance. This implies that access to information increases the chances of being food secure. In fact, proper awareness is the first step towards fully utilizing SLM which then transcend to increased productivity, hence, food security.
4.7 Treatment effects for the adoption of SLM practices on household food security—poisson regression with endogenous treatment effect
In order to address the endogeneity issue, this study estimated endogenous Poisson regression while taking smallholder farmers’ adoption of SLM into account. The adoption of SLM was positively and significantly impacted by the results. It is misleading to compare the food security status of SLM adoption impact study adopters and non-adopters because most adoption impact studies do not account for potential differences in the characteristics of the two groups. Despite taking endogeneity into account, the estimate from the endogenous Poisson regression model may also be insufficient. This is due to the fact that the counterfactual scenario’s missing data prevents the direct coefficients from the model from being regarded as ATT. In order to address this, the study used ATE and ATT to examine the causal effects of SLM adoption on household food security status. Poisson regression with endogenous treatment was employed in this analysis, and AIPW was added as a robustness check. Therefore, we start by talking about the Poisson estimates. Following the fitting of the Poisson regression with endogenous treatment effects, the ATE and ATT were calculated.2 Table 7 shows that the adoption of SLM resulted in an estimated potential outcome mean (ATE) of approximately 6.8 and statistical significance at 1% for the number of food items consumed by households.According to the ATE estimate, if a farmer in the study area implements SLM practices, their average farm household will likely consume approximately three extra food items. The ATT of implementing SLM on the quantity of food items consumed by households was measured by the conditional treatment effects, which was 2.5 and statistically significant at 1%. As a result, compared to non-adopters, the average household in the adopters group would consume roughly three extra food items.

Table 7. Treatment effects for the adoption of SLMP on food security—poisson regression with endogenous treatment effect.
AIPW significantly increases household food security as a result of SLM adoption in tandem with the Poisson regression. The ATE and POM are roughly three (3) and seven (7), respectively, based on Table 8. Therefore, if every farmer in our sample adopted the SLM as a form of climate smart agriculture technology, the average number of foods consumed would be three times higher than the average of seven that would occur if no farmer adopted the SLM. Similarly, the group that adopted SLM practices experienced an increase in food consumption of 5.2 times greater than the group that did not adopt SLM practices.

Table 8. Treatment effects for the adoption of SLMP on food security—augmented-probability-weighted regression adjustment.
The two estimation techniques’ results showed that farmers used a significantly higher number of strategies to mitigate the negative effects of climate change when they received on-farm demonstration training. The average causal effects, as presented in Tables 7, 8, showed that there was a divergence in the magnitudes of the outcome variable estimates between AIPW and Poisson endogenous treatment regression. Differences in unobserved heterogeneity among smallholder rice farmers may be the cause of this discrepancy in the two sets of results. Adoption of SLM has a positive effect on household nutrition security, which is consistent with the findings of Worku et al. (2020) in Ghana and Martey et al. (2020) in Eastern Africa. The study’s findings imply that SLM adoption enhances household food security in the investigated area. The significance of SLM adoption in helping smallholder farmers adjust to the unpredictable effects of climate change is highlighted by the implications of these findings.
4.8 Frequency distribution of the propensity score by adoption status of smallholder rice farmers
The findings in Figure 5 demonstrate that smallholder rice farmers share a common support for the adoption of SLM.
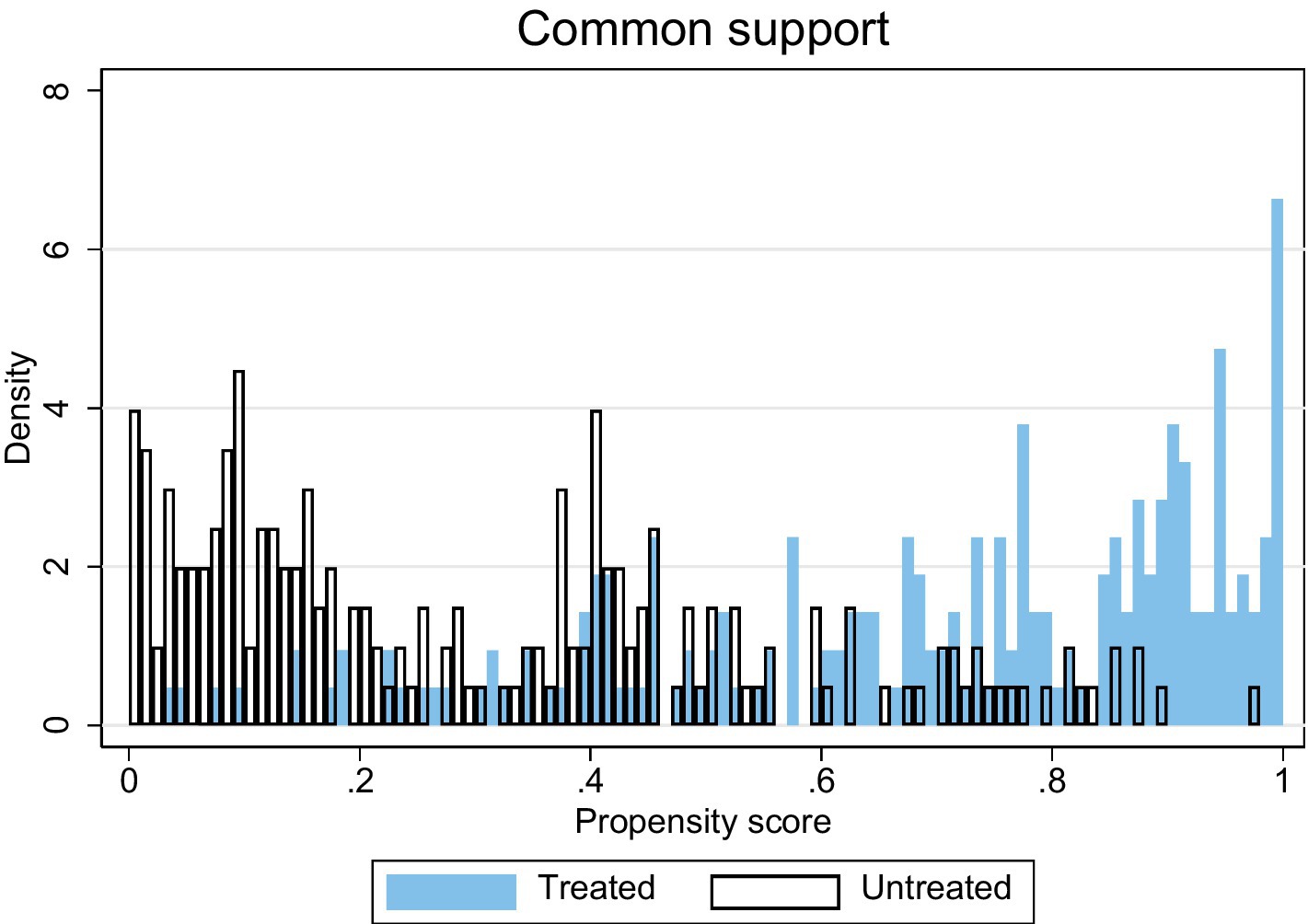
Figure 5. The common support showing the frequency distribution of the propensity score by adoption status of small-scale crop farmers.
The propensity scores are forecast using the baseline first stage probit regression in order to estimate the factors influencing the adoption of SLM practices using the probit model. Figure 4 makes it clear that the first regression stage yields a range for common support of 0.1 to 0.99. It even demonstrates the mutual support that results from variations in the second stage’s covariates and instruments. This satisfies the general assumption in MTE applications, according to Shahzad and Abdulai (2021), that the MTE curve’s shape is constant with respect to control variables.
4.9 Marginal treatment effects results of causal effects of adoption of SLM practices on household food security
Figure 6 clearly illustrates the direct correlation between unobserved characteristics and selection based on gains from, as higher food consumption scores values imply a higher likelihood of adoption. As was already mentioned, higher SLM values suggest a higher likelihood of adoption and can be interpreted as an adoption propensity.
A pattern of direct selection on gains found for observed farm household characteristics is depicted by the MTE curve in Figure 6. The MTE curve for HDDS can be seen in Figure 6. The downward slope of the curve indicates that adoption resistance increases with a decrease in adoption benefits. This suggests that gains are driving a trend of positive selection. Therefore, in terms of diversified food, farmers who are more likely to implement SLM practices stand to gain more. This result supports those in Table 9, which show that the pattern of heterogeneity (slope of MTE curve), which is shown to be statistically significant at the 5% level in the lower part of Table 9, benefits farmers who are most likely to implement SLM practices.
4.10 Summary of causal effects of adoption of SLM on food and nutrition security
The results of the causal impact of adoption of SLM practices on food and nutrition security based on our baseline specification are presented in Table 9.
The adoption of SLM practices and its estimated treatment effects on the outcome variable are reported. The findings demonstrate household dietary follow the same pattern of selection, with farmers who are most likely to adopt benefiting from adoption to the greatest extent (i.e., treatment effects on the treated (ATT) being higher than the other parameters). Hence, unobserved gains that indicate positive selection are statistically significant. The estimates of average treatment effects (ATE) indicate that the treatment effects are positive and statistically significant in terms of both sign and magnitude. According to the ATE results, implementing SLM practices improves food diversity by 35%. These improvements are significant (at the 1% level). According to ATT estimates, adoption of SLM practices improves dietary diversity by 52% for an average adopting household. The treatment effects on untreated (ATU) are lower than that of ATE and ATT, confirming the positive selection on unobserved gains. In particular, the ATU results show that for an average non-adopting household, adoption of SLM practices would significantly improve dietary diversity by about 27%. The local average treatment effects (LATE), which shows that households who adopted SLM practices as a result of more access to extension services, improve dietary diversity by about 34%.
5 Conclusion and policy recommendations
This study estimates the determinants of the adoption of SLM practices and the impact of adoption on household food security among smallholder rice farmers in Ogun State, Nigeria. A multistage sampling procedure was used to select 120 respondents. A double-hurdle count data and poisson endogenous treatment (PET) models were employed to analyse the determinants of level of adoption of SLMP and impact of SLMP adoption on household food security level of smallholder rice farmers, respectively in the study area. The results showed that socio-economic factors greatly influenced the adoption of SLMP practices, such as age and educational level of farmers. SLM practices have the potential to reduce food insecurity among rice farmers if they are well-combined and utilized extensively. It was discovered that the adoption of SLMP, which consists of a variety of practices, improved the effect of SLMP adoption on food security of smallholder farmers. To address potential bias in the estimates of ATT, ATET, and POM of the Poisson endogenous treatment model, a doubly robust augmented inverse probability weighted (AIPW) was employed as a reliable solution. The average causal effects results showed that there was a divergence in the magnitudes of the outcome variable estimates between AIPW and Poisson endogenous treatment regression. The possible cause of this discrepancy between the two sets of results could be variations in the unobserved heterogeneity among smallholder rice farmers. In order to demonstrate heterogeneity in adoption gains in both observed and unobserved factors that influence adoption of SLM practices, the study used the marginal treatment effects (MTE) approach. The study’s empirical findings demonstrate a considerable degree of heterogeneity in the advantages of implementing SLM practices. In instance, a pattern of positive selection on the gain that was observed from adopting SLM practices for household diet. This observation stems from the fact that adoption typically has a greater positive impact on households that are more likely to adopt SLM practices. The average treatment effects on the treated (ATT) demonstrate that implementing SLM practices greatly increases farm households’ food and nutrition security. The study found that the adoption of SLMP by smallholder farmers in the study area was significantly influenced by knowledge gained through formal education, some type of vocational training, and trainings to access weather information. The study recommended that continuous adoption and extensive use can be fostered by encouraging farmers to join a social organisation where related and relevant information on sustainable land management practices is shared through trained agricultural extension officers. Farm-level policy initiatives that seek to empower farmers by providing them with knowledge, training, and education about land degradation would be a significant step toward encouraging SLM practices, which in turn leads to improved food security. Overall, the study’s findings indicate that increasing the adoption of SLM techniques can aid in enhancing the food and nutrition security of Nigerian farm households. Therefore, utilizing information and communication technologies, the government, stakeholders, and donor agencies must offer capacity-building innovations, trainings for on-farm demonstrations, and sustainable land management education. For future research in a coordinated effort to identify yield and mitigation effects from sustainable land management for several agro-ecological zones and farming systems is needed to fill the gaps in our understanding identified in this study.
Data availability statement
Publicly available datasets were analyzed in this study. This data can be found at: https://data.worldbank.org/.
Ethics statement
Ethical review and approval was not required for the study on human participants in accordance with the local legislation and institutional requirements. Written informed consent from the patients/ participants or the patients'/participants' legal guardian/next of kin was not required to participate in this study in accordance with the national legislation and the institutional requirements.
Author contributions
TO: Methodology, Writing – review & editing, Conceptualization, Funding acquisition, Resources. OA: Conceptualization, Visualization, Methodology, Writing – review & editing. OI: Methodology, Visualization, Data curation, Writing – review & editing. CE: Conceptualization, Resources, Validation, Writing – review & editing. HK: Funding acquisition, Methodology, Resources, Writing – review & editing. KE: Funding acquisition, Resources, Validation, Visualization, Writing – review & editing. FK: Conceptualization, Methodology, Data curation, Formal Analysis, Writing – original draft. JS: Conceptualization, Data curation, Visualization, Writing – original draft.
Funding
The author(s) declare that financial support was received for the research, authorship, and/or publication of this article. The authors would like to thank Researchers Supporting Project number (RSPD2024R952), King Saud University, Saudi Arabia.
Conflict of interest
The authors declare that the research was conducted in the absence of any commercial or financial relationships that could be construed as a potential conflict of interest.
Publisher’s note
All claims expressed in this article are solely those of the authors and do not necessarily represent those of their affiliated organizations, or those of the publisher, the editors and the reviewers. Any product that may be evaluated in this article, or claim that may be made by its manufacturer, is not guaranteed or endorsed by the publisher.
Footnotes
1. ^The mathematical equations of estimating AIPW can be found in (Glynn and Quinn, 2010).
2. ^ATE and ATT were estimated as a post-estimation after fitting the Stata command etpoisson for Poisson regression with endogenous treatment. The ATE estimated after etpoisson is the potential outcome means while ATT is the conditional treatment effect.
References
Abadie, A. (2003). Semiparametric instrumental variable estimation of treatment response models. J. Econ. 113, 231–263.
Adeagbo, O. A., Ojo, T. O., and Adetoro, A. A. (2021). Understanding the determinants of climate change adaptation strategies among smallholder maize farmers in south-west, Nigeria. Heliyon 7:e06231. doi: 10.1016/j.heliyon.2021.e06231
Adeniyi, D. A., and Dinbabo, M. F. (2019). Factors influencing household food security among irrigation smallholders in north West Nigeria. J. Rev. Glob. Econ. 8, 291–304. doi: 10.6000/1929-7092.2019.08.25
Adenle, A. A., Boillat, S., and Speranza, C. I. (2022). Key dimensions of land users’ perceptions of land degradation and sustainable land management in Niger state, Nigeria. Environ. Challeng. 8:100544. doi: 10.1016/j.envc.2022.100544
Adenle, A. A., and Ifejika Speranza, C. (2020). Social-ecological archetypes of land degradation in the Nigerian Guinea Savannah: insights for sustainable land management. Remote Sens. 13:32.
Akanbi, S.-U. O., Olatunji, O. S., Oladipo, O. S., Adeyemi, U. T., and Akinyoade, A. (2022). Vulnerability of rice farmers to climate change in Kwara state, Nigeria. Turk. J. Agric. Food Sci. Technol. 10, 374–380. doi: 10.24925/turjaf.v10i2.374-380.4648
Amoako Johnson, F., and Hutton, C. W. (2014). Dependence on agriculture and ecosystem services for livelihood in Northeast India and Bhutan: vulnerability to climate change in the Tropical River basins of the upper Brahmaputra. Clim. Chang. 127, 107–121. doi: 10.1007/s10584-012-0573-7
Anuga, S. W., Gordon, C., Boon, E., and Surugu, J. M. I. (2019). Determinants of climate smart agriculture (CSA) adoption among smallholder food crop farmers in the Techiman municipality, Ghana. Ghana J. Geography 11, 124–139.
Arowolo, A. O., Deng, X., Olatunji, O. A., and Obayelu, A. E. (2018). Assessing changes in the value of ecosystem services in response to land-use/land-cover dynamics in Nigeria. Sci. Total Environ. 636, 597–609.
Asfaw, S., Pallante, G., and Palma, A. (2018). Diversification strategies and adaptation deficit: evidence from rural communities in Niger. World Dev. 101, 219–234. doi: 10.1016/j.worlddev.2017.09.004
Asfew, M., Bakala, F., and Fite, Y. (2023). Adoption of soil and water conservation measures and smallholder farmers' perception in the bench-Sheko zone of Southwest Ethiopia. J. Agric. Food Res. 11:100512. doi: 10.1016/j.jafr.2023.100512
Begum, M. E. A., Hossain, M. I., and Mainuddin, M. (2023). Climate change perceptions, determinants and impact of adaptation strategies on watermelon farmers in the saline coastal areas of Bangladesh. Lett. Spat. Resour. Sci. 16, 1–31. doi: 10.1007/s12076-022-00324-6
Bello, L. O., Awotide, B. A., and Sakurai, T. (2024). Climate change adaptation and smallholder farmers welfare: Empirical evidence from the Sahelian Region of West Africa. Land Use Policy 142:107181.
Ben Hassen, T., and El Bilali, H. (2022). Impacts of the Russia-Ukraine war on global food security: towards more sustainable and resilient food systems? Food Secur. 11:2301. doi: 10.3390/foods11152301
Cameron, A. C., and Trivedi, P. K. (2001). Essentials of count data regression. A companion to theoretical econometrics, 331.
Cattaneo, O., Gereffi, G., Miroudot, S., and Taglioni, D. (2013). Joining, upgrading and being competitive in global value chains: a strategic framework. World Bank Policy Research Working Paper, 6406.
Channa, H., Chen, A. Z., Pina, P., Ricker-Gilbert, J., and Stein, D. (2019). What drives smallholder farmers’ willingness to pay for a new farm technology? Evidence from an experimental auction in Kenya. Food Policy 85, 64–71. doi: 10.1016/j.foodpol.2019.03.005
Cordero-Ahiman, O. V., Vanegas, J. L., Franco-Crespo, C., Beltrán-Romero, P., and Quinde-Lituma, M. E. (2021). Factors that determine the dietary diversity score in rural households: the case of the Paute River basin of Azuay Province, Ecuador. Int. J. Environ. Res. Public Health 18:2059. doi: 10.3390/ijerph18042059
Cornelissen, G., Pandit, N. R., Taylor, P., Pandit, B. H., Sparrevik, M., and Schmidt, H. P. (2016). Emissions and char quality of flame-curtain" Kon Tiki" Kilns for Farmer-Scale charcoal/biochar production. PLoS One 11:e0154617.
Danso-Abbeam, G., Asale, M. A., and Ogundeji, A. A. (2022). Determinants of household food insecurity and coping strategies in northern Ghana. GeoJournal 88, 2307–2324. doi: 10.1007/s10708-022-10742-0
De Luca, G., and Perotti, V. (2011). Estimation of ordered response models with sample selection. Stata J. 11, 213–239. doi: 10.1177/1536867X1101100204
Demont, M., and Ndour, M. (2015). Upgrading rice value chains: Experimental evidence from 11 African markets. Glob. Food Secur. 5, 70–76.
Dung, T. Q., Bonney, L. B., Adhikari, R., and Miles, M. P. (2021). Entrepreneurial orientation and vertical knowledge acquisition by smallholder agricultural firms in transitional economies: The role of interfirm collaboration in value-chains. J. Bus. Res. 137, 327–335.
El-ladan, I. (2014). “Climate change and food security in Nigeria.” in Proceedings of the International Conference on Possible Impacts of Climate Change on Africa, Institute of African Research and Studies. Cairo University, Cairo, Egypt. 18–20 May 2014. p. 16.
Etim, M. A., Babaremu, K., Lazarus, J., and Omole, D. (2021). Health risk and environmental assessment of cement production in Nigeria. Atmosfera 12:1111. doi: 10.3390/atmos12091111
Fadina, A. M. R., and Barjolle, D. (2018). Farmers’ adaptation strategies to climate change and their implications in the Zou Department of South Benin. Environments. 5:15.
Fahad, S., and Wang, J. (2018). Farmers’ risk perception, vulnerability, and adaptation to climate change in rural Pakistan. Land Use Policy 79, 301–309. doi: 10.1016/j.landusepol.2018.08.018
FAO (2016). FAOSTAT Database. FAO. Available at: www.fao.org/faostat (Accessed December 01, 2016).
FAO, WFP, and EU (2018). Global report of food crises 2018. Food security information Network (FSIN). Available at: http://www.fao.org/fleadmin/user_upload/fsin/docs/global_report/2018/GRFC_2018_Full_reporEN.pdf (Accessed July 18, 2024).
Fentahun, G., Amsalu, T., and Birhanie, Z. (2023). Farmers’ perceptions about the influence of land fragmentation and land quality on sustainable land management in the upper lake Tana Basin: evidence from Dera District. Cogent Econ. Finan. 11:2160132. doi: 10.1080/23322039.2022.2160132
Gbadebo, A. M. (2012). Groundwater fluoride and dental fluorosis in southwestern Nigeria. Environ. Geochem. Health 34, 597–604.
Glynn, A. N., and Quinn, K. M. (2010). An introduction to the augmented inverse propensity weighted estimator. Polit. Anal. 18, 36–56.
Goli, I., Azadi, H., Najafabadi, M. O., Lashgarara, F., Viira, A. H., Kurban, A., et al. (2023). Are adaptation strategies to climate change gender neutral? Lessons learned from paddy farmers in northern Iran. Land Use Policy 125:106470. doi: 10.1016/j.landusepol.2022.106470
Greene, W. (2008). Functional forms for the negative binomial model for count data. Econ. Lett. 99, 585–590. doi: 10.1016/j.econlet.2007.10.015
Gwacela, M., Ngidi, M. S. C., Hlatshwayo, S. I., and Ojo, T. O. (2024). Analysis of the contribution of home gardens to household food security in Limpopo Province, South Africa. Sustainability 16:2525. doi: 10.3390/su16062525
Harding, M., Lamarche, C., and Pesaran, M. H. (2020). Common correlated effects estimation of heterogeneous dynamic panel quantile regression models. J. Appl. Econ. 35, 294–314. doi: 10.1002/jae.2753
Heckman, J. J. (1977). Sample selection bias as a specification error (with an application to the estimation of labor supply functions) (No. w0172). National Bureau of Economic Research.
Hermans, K., and McLeman, R. (2021). Climate change, drought, land degradation and migration: exploring the linkages. Curr. Opin. Environ. Sustain. 50, 236–244. doi: 10.1016/j.cosust.2021.04.013
Hlatshwayo, S. I., Ngidi, M. S. C., Ojo, T. O., Modi, A. T., Mabhaudhi, T., and Slotow, R. (2023). The determinants of crop productivity and its effect on food and nutrition security in rural communities of South Africa. Front. Sustain. Food Syst. 7:1091333. doi: 10.3389/fsufs.2023.1091333
Hoffert, M. I., Caldeira, K., Benford, G., Criswell, D. R., Green, C., Herzog, H., et al. (2002). Advanced technology paths to global climate stability: energy for a greenhouse planet. Science 298, 981–987. doi: 10.1126/science.1072357
Idris, A., Lenshie, N. E., and Miapyen, B. S. (2024). Border closure and border governance dialectics in Nigeria. Afr. Secur. Rev. 33, 1–15. doi: 10.1080/10246029.2023.2253209
Igbinedion, S., and Aihie, J. (2015). Towards good governance and sustainable food security in Nigeria: challenges and the way forward. OIDA International J. Sustain. Dept. 8, 41–52.
Kehinde, A. D., and Ogundeji, A. A. (2022). The simultaneous impact of access to credit and cooperative services on cocoa productivity in South-Western Nigeria. Agric. Food Secur. 11:11. doi: 10.1186/s40066-021-00351-4
Khatri-Chhetri, A., Aggarwal, P. K., Joshi, P. K., and Vyas, S. (2017). Farmers' prioritization of climate-smart agriculture (CSA) technologies. Agric. Syst. 151, 184–191. doi: 10.1016/j.agsy.2016.10.005
Kien, N. D., Dung, T. Q., Oanh, D. T. K., An, L. T., Dinh, N. C., Phan, N. T., et al. (2023). Climate-resilient practices and welfare impacts on rice-cultivating households in Vietnam: does joint adoption of multiple practices matter? Aust. J. Agric. Resour. Econ. 67, 263–284. doi: 10.1111/1467-8489.12506
Kirui, O. K., Okello, J. J., Nyikal, R. A., and Njiraini, G. W. (2013). Impact of mobile phone-based money transfer services in agriculture: evidence from Kenya. Quart. J. Int. Agric. 52, 141–162. doi: 10.22004/ag.econ.173644
Kolapo, A., Didunyemi, A. J., Aniyi, O. J., and Obembe, O. E. (2022). Adoption of multiple sustainable land management practices and its effects on productivity of smallholder maize farmers in Nigeria. Resourc. Environ. Sustain. 10:100084. doi: 10.1016/j.resenv.2022.100084
Kolliesuah, N. P., Olum, S., and Ongeng, D. (2023). Status of household dietary diversity and associated factors among rural and urban households of northern Uganda. BMC Nut. 9:83. doi: 10.1186/s40795-023-00739-4
Krishnamurthy, P. K., Choularton, R. J., and Kareiva, P. (2020). Dealing with uncertainty in famine predictions: How complex events affect food security early warning skill in the Greater Horn of Africa. Glob. Food Secur. 26:100374.
Leng, A., Wang, K., Bai, J., Gu, N., and Feng, R. (2024). Analyzing sustainable development in Chinese cities: a focus on land use efficiency in production-living-ecological aspects. J. Clean. Prod. 448:141461. doi: 10.1016/j.jclepro.2024.141461
Leser, S. (2013). The 2013 FAO report on dietary protein quality evaluation in human nutrition: Recommendations and implications. Nutrition Bulletin, 38, 421–428.
Maddala, G. S., Li, H., and Srivastava, V. K. (2001). A comparative study of different shrinkage estimators for panel data models. Ann. Econ. Financ. 2, 1–30.
Maja, M. M., and Ayano, S. F. (2021). The impact of population growth on natural resources and farmers’ capacity to adapt to climate change in low-income countries. Earth Syst. Environ. 5, 271–283. doi: 10.1007/s41748-021-00209-6
Martey, E., Etwire, P. M., and Kuwornu, J. K. (2020). Economic impacts of smallholder farmers’ adoption of drought-tolerant maize varieties. Land Use Policy 94:104524.
Mirzabaev, A., Strokov, A., and Krasilnikov, P. (2023). The impact of land degradation on agricultural profits and implications for poverty reduction in Central Asia. Land Use Policy 126:106530. doi: 10.1016/j.landusepol.2022.106530
Mncube, L. N., Ojo, T. O., and Nyam, Y. S. (2023). Addressing food insecurity in Richmond area of KwaZulu-Natal, South Africa: the role of cash transfers. Sci. Afr. 19:e01485. doi: 10.1016/j.sciaf.2022.e01485
Mthethwa, K. N., Ngidi, M. S. C., Ojo, T. O., and Hlatshwayo, S. I. (2022). The determinants of adoption and intensity of climate-smart agricultural practices among smallholder maize farmers. Sustain. For. 14:16926. doi: 10.3390/su142416926
Mulwa, C., Marenya, P., and Kassie, M. (2017). Response to climate risks among smallholder farmers in Malawi: A multivariate probit assessment of the role of information, household demographics, and farm characteristics. Clim. Risk Manag. 16, 208–221.
Munaweera, T. I. K., Jayawardana, N. U., Rajaratnam, R., and Dissanayake, N. (2022). Modern plant biotechnology as a strategy in addressing climate change and attaining food security. Agric. Food Secur. 11, 1–28. doi: 10.1186/s40066-022-00369-2
Musemwa, L., Chagwiza, C., Sikuka, W., Fraser, G., Chimonyo, M., and Mzileni, N. (2007). Analysis of cattle marketing channels used by small scale farmers in the eastern Cape Province, South Africa. Livest. Res. Rural. Dev. 19:131.
Mutenje, M. J., Farnworth, C. R., Stirling, C., Thierfelder, C., Mupangwa, W., and Nyagumbo, I. (2019). A cost-benefit analysis of climate-smart agriculture options in Southern Africa: Balancing gender and technology. Ecol. Econ. 163, 126–137.
National Nutrition and Health Survey (2018). Report on the Nutrition and Health Situation of Nigeria. Accessed at: https://www.unicef.org/nigeria/reports/national-nutrition-and-health-survey-nnhs-2018 (Accessed July 18, 2024).
Ndamani, F., and Watanabe, T. (2016). Determinants of farmers’ adaptation to climate change: a micro level analysis in Ghana. Sci. Agric. 73, 201–208. doi: 10.1590/0103-9016-2015-0163
Ngema, P. Z., Sibanda, M., and Musemwa, L. (2018). Household food security status and its determinants in Maphumulo local municipality, South Africa. Sustainability 10:3307. doi: 10.3390/su10093307
Nkegbe, P. K., Abu, B. M., and Issahaku, H. (2017). Food security in the Savannah accelerated development authority zone of Ghana: an ordered probit with household hunger scale approach. Agric. Food Secur. 6, 1–11. doi: 10.1186/s40066-017-0111-y
Nyam, Y. S., Modiba, N. T. S., Ojo, T. O., Ogundeji, A. A., Okolie, C. C., and Selelo, O. T. (2024). Analysis of the perceptions of flood and effect of adoption of adaptation strategies on income of informal settlements of Mamelodi in South Africa. Clim. Serv. 34:100468. doi: 10.1016/j.cliser.2024.100468
Oduniyi, O. S. (2022). Factors driving the adoption and use extent of sustainable land management practices in South Africa. Circ. Econ. Sustain. 2, 589–608.
Oduniyi, O. S., Ojo, T. O., and Nyam, Y. S. (2023). Awareness and adoption of sustainable land management practices among smallholder maize farmers in Mpumalanga province of South Africa. Afr. Geograph. Rev. 42, 217–231. doi: 10.1080/19376812.2021.2018661
Ogunpaimo, O. R., Oyetunde-Usman, Z., and Surajudeen, J. (2021). Impact of climate change adaptation on household food security in Nigeria—a difference-in-difference approach. Sustain. For. 13:1444. doi: 10.3390/su13031444
Ojo, T. O., Ogundeji, A. A., and Emenike, C. U. (2022). Does adoption of climate change adaptation strategy improve food security? A case of rice farmers in Ogun state, Nigeria. Land 11:1875. doi: 10.3390/land11111875
Oriola, E. O. (2009). A framework for food security and poverty reduction in Nigeria. Eur. J. Soc. Sci. 8, 132–139.
Osabuohien, E. S., Okorie, U. E., and Osabohien, R. A. (2018). “Rice production and processing in Ogun state, Nigeria: qualitative insights from farmers' association” in Food systems sustainability and environmental policies in modern economies: IGI GLOBAL. 188–215.
Oseni, G., and Winters, P. (2009). Rural nonfarm activities and agricultural crop production in Nigeria. Agric. Econ. 40, 189–201.
Otekunrin, O. A., and Otekunrin, O. A. (2022). Nutrition outcomes of under-five children of smallholder farm households: do higher commercialization levels Lead to better nutritional status? Child Indic. Res. 15, 2309–2334. doi: 10.1007/s12187-022-09960-2
Pender, J., and Gebremedhin, B. (2008). Determinants of agricultural and land management practices and impacts on crop production and household income in the highlands of Tigray, Ethiopia. J. Afr. Econ. 17, 395–450. doi: 10.1093/jae/ejm028
Ridder, R. M. (2007). Global forest resources assessment 2010: options and recommendations for a global remote sensing survey of forests. FAO For. Resour. Assess. Programme Work. 141.
Rochecouste, J. F., Dargusch, P., Cameron, D., and Smith, C. (2015). An analysis of the socio-economic factors influencing the adoption of conservation agriculture as a climate change mitigation activity in Australian dryland grain production. Agric. Syst. 135, 20–30. doi: 10.1016/j.agsy.2014.12.002
Salaisook, P., Faysse, N., and Tsusaka, T. W. (2020). Reasons for adoption of sustainable land management practices in a changing context: a mixed approach in Thailand. Land Use Policy 96:104676. doi: 10.1016/j.landusepol.2020.104676
Santoso, A. B., Girsang, S. S., Raharjo, B., Pustika, A. B., Hutapea, Y., Kobarsih, M., et al. (2023). Assessing the challenges and opportunities of agricultural information systems to enhance farmers’ capacity and target Rice production in Indonesia. Sustain. For. 15:1114. doi: 10.3390/su15021114
Sanusi, M. M., and Dries, L. (2024). Weather-related shocks, livelihood assets and coping strategies of water-insecure smallholder rice farmers: a case study from Ogun state, Nigeria. Environ. Dev. 51:101040. doi: 10.1016/j.envdev.2024.101040
Sekaran, U., Lai, L., Ussiri, D. A., Kumar, S., and Clay, S. (2021). Role of integrated crop-livestock systems in improving agriculture production and addressing food security–a review. J. Agric. Food Res. 5:100190. doi: 10.1016/j.jafr.2021.100190
Shahzad, M. F., and Abdulai, A. (2021). The heterogeneous effects of adoption of climate-smart agriculture on household welfare in Pakistan. Appl. Econ. 53, 1013–1038.
Sheikh, A. T., Chaudhary, A. K., Mufti, S., Davies, S., and Rola-Rubzen, M. F. (2024). Soil fertility in mixed crop-livestock farming systems of Punjab, Pakistan: the role of institutional factors and sustainable land management practices. Agric. Syst. 218:103964. doi: 10.1016/j.agsy.2024.103964
Shilomboleni, H., Epstein, G., and Mansingh, A. (2024). Building resilience in Africa’s smallholder farming systems: contributions from agricultural development interventions—a scoping review. Ecology and Society, 29.
Siegfried, S., and Hothorn, T. (2020). Count transformation models. Methods Ecol. Evol. 11, 818–827. doi: 10.1111/2041-210X.13383
Slovin, M. B. (1974). On the relationships among monetary aggregates. J. Money Credit Bank. 6, 353–366.
Srivastav, A. L., Patel, N., Rani, L., Kumar, P., Dutt, I., Maddodi, B. S., et al. (2024). Sustainable options for fertilizer management in agriculture to prevent water contamination: a review. Environ. Dev. Sustain. 26, 8303–8327. doi: 10.1007/s10668-023-03117-z
Tejada, J. J., and Punzalan, J. R. B. (2012). On the misuse of Slovin’s formula. Philip. Statist. 61, 129–136.
Teshome, A., de Graaff, J., Ritsema, C., and Kassie, M. (2016). Farmers' perceptions about the influence of land quality, land fragmentation and tenure systems on sustainable land management in the north western Ethiopian highlands. Land Degrad. Dev. 27, 884–898. doi: 10.1002/ldr.2298
Thinda, K. T., Ogundeji, A. A., Belle, J. A., and Ojo, T. O. (2020). Understanding the adoption of climate change adaptation strategies among smallholder farmers: evidence from land reform beneficiaries in South Africa. Land Use Policy 99:104858. doi: 10.1016/j.landusepol.2020.104858
Ukonu, I. C., Wallace, C. A., and Lowe, N. M. (2024). Household food security and dietary diversity in South-Eastern Nigeria. Matern. Child Nutr. 20:e13599. doi: 10.1111/mcn.13599
Vernooy, R. (2022). Does crop diversification lead to climate-related resilience? Improving the theory through insights on practice. Agroecol. Sustain. Food Syst. 46, 877–901. doi: 10.1080/21683565.2022.2076184
Vivek, K.S. (2019). Assessment of livelihoods of smallholder Rice farmers in Aurangabad District, Bihar, India. Van Hall Larenstein University of Applied Sciences, the Netherlands [MSc thesis].
von Grebmer, K., Bernstein, J., Alders, R., Dar, O., Kock, R., Rampa, F., et al. (2020). 2020 global hunger index: One decade to zero hunger: Linking health and sustainable food systems; Welthungerhilfe: Bonn. Germany; Concern Worldwide: Dublin, Iraland.
Wang, J., Liu, L., Zhao, K., and Wen, Q. (2023). Farmers’ adoption intentions of water-saving agriculture under the risks of frequent irrigation-induced landslides. Climate. Risk Manag. 39:100484. doi: 10.1016/j.crm.2023.100484
Wooldridge, J. M. (2002). Inverse probability weighted M-estimators for sample selection, attrition, and stratification. Port. Econ. J. 1, 117–139. doi: 10.1007/s10258-002-0008-x
Worku, M., De Groote, H., Munyua, B., Makumbi, D., Owino, F., Crossa, J., et al. (2020). On-farm performance and farmers’ participatory assessment of new stress-tolerant maize hybrids in Eastern Africa. Field Crop Res. 246:107693.
World Health Organization (2018). The state of food security and nutrition in the world 2018: building climate resilience for food security and nutrition. Food & Agriculture Organization.
Xiong, L., and Li, R. (2024). Assessing and decoupling ecosystem services evolution in karst areas: a multi-model approach to support land management decision-making. J. Environ. Manag. 350:119632. doi: 10.1016/j.jenvman.2023.119632
Xu, M., Luan, Y., Zhang, Z., and Jiang, S. (2021). Dietary pattern changes over Africa and its implication for land requirements for food. Mitig. Adapt. Strateg. Glob. Chang. 26:13. doi: 10.1007/s11027-021-09939-4
Zeleke, G., Teshome, M., and Ayele, L. (2023). Farmers' livelihood vulnerability to climate-related risks in the north Wello zone, northern Ethiopia. Environ. Sustain. Indicat. 17:100220. doi: 10.1016/j.indic.2022.100220
Zenda, S. M. (2002). A systems approach to marketing in less developed agriculture with reference to Bululwane irrigation scheme, J-STAGE.
Keywords: poisson endogenous treatment, food security, rice farming, marginal treatment effects, sustainable land management practice
Citation: Ojo TOO, Adesiyan OF, Ige AO, Emenike CU, Kassem HS, Elhindi KM, Kitole FA and Sesabo JK (2024) The role of sustainable land management practices in alleviating household food insecurity in Nigeria. Front. Sustain. Food Syst. 8:1414243. doi: 10.3389/fsufs.2024.1414243
Edited by:
Andrew John Dougill, University of York, United KingdomReviewed by:
Akinyinka Akinyoade, ASCL, NetherlandsTemitayo Adenike Adeyemo, University of Ibadan, Nigeria
Adeola Oluwabunmi Adeagbo, University of Ibadan, Nigeria
Copyright © 2024 Ojo, Adesiyan, Ige, Emenike, Kassem, Elhindi, Kitole, and Sesabo. This is an open-access article distributed under the terms of the Creative Commons Attribution License (CC BY). The use, distribution or reproduction in other forums is permitted, provided the original author(s) and the copyright owner(s) are credited and that the original publication in this journal is cited, in accordance with accepted academic practice. No use, distribution or reproduction is permitted which does not comply with these terms.
*Correspondence: Temitope Oluwaseun Oluwaseun Ojo, b2pvdGVtaXRvcGU3MEB5YWhvby5jb20=