- 1Department of Soil Science and Agriculture Chemistry, College of Agriculture, Gwalior, Madhya Pradesh, India
- 2ICAR- Indian Institute of Soil Science, Nabibagh, Bhopal, Madhya Pradesh, India
- 3Department of Agricultural and Biosystems Engineering, Iowa State University, Ames, IA, United States
Optimum soil moisture and high crop residue return (RR) can increase the active pool of soil organic carbon and nitrogen, thus modulating the magnitude of greenhouse gas (GHG) fluxes. To determine the effect of soil moisture on the threshold level of RR for the wheat production system, we analyzed the relationship between GHG fluxes and RR at four levels, namely 0, 5, 10, and 15 Mg ha−1 (R0, R5, R10, and R15) under two soil moisture content (80% FC and 100% FC) and three levels of nutrient management (NS0: no nutrient; NS1, NS2= 3x NS1). Nutrient input (N and P) in NS1 balanced the residue C/nutrient stoichiometry to achieve 30% stabilization of the residue C input in RR (R5). All RR treatments (cf. R0) were found to significantly reduce N2O emission in moderate soil moisture content (80% FC) by 22–56% across nutrient management due to enhanced soil C mineralization, microbial biomass carbon, and N immobilization. However, averaged across nutrient management, a linear increase in N2O emission was observed with increasing RR under 100% FC soil moisture. A significant decrease in CH4 emission by ca. 46% in most RR treatments was observed in 100% FC compared with the R0. The N2O emission was negatively correlated (p=<0.001) with nutrient stoichiometry. Partial least square (PLS) regression indicated that GHG emissions were more responsive (values > 0.8) to management variables (RR rate, nitrogen (N) input rate, soil moisture, and nutrient stoichiometry of C: N) and post-incubation soil properties (SMBC and NO3-N) in Alfisols. This study demonstrated that the mechanisms responsible for RR effects on soil N2O, CH4 fluxes, and carbon mineralization depend on soil moisture and nutrient management, shifting the nutrient stoichiometry of residue C: N: P.
1 Introduction
Crop residues, the major byproduct of the agricultural sector, have a higher production rate in India (500 million metric tons, Mt), of which the share of wheat residue is more than 50% in India. For several reasons (short time span for sowing the next crop, limited farm mechanization, scarce manpower, and poor acceptability of paddy straw as fodder) crop residue of ~87 Mt is burned annually in India, contributing to GHG emissions and carbonaceous aerosols (Deshpande et al., 2023). Among the crop residues, wheat residue burning contributes the maximum, i.e., 65% of total GHG emissions due to residue burning, followed by rice and maize (Lenka et al., 2014). The most sustainable and cost-effective method to manage wheat residue could be its recycling and incorporation into the soil (Wang et al., 2018). This approach and other sustainable agricultural practices hold significant promise for reducing emissions and improving soil health. Harvesting wheat residues with a combined harvester leaves different proportions (varying from 30% to 100%) of residues in farmers' fields with different rates of wheat RR to the soil (Singh et al., 2024). Crop RR has multiple-fold benefits in maintaining soil health, crop productivity, and reduced emissions from residue burning (Kumar and Goh, 1999; Fang et al., 2018b). However, the different rates of crop RR could be a source or sink to soil emission of GHGs (CO2, N2O, and CH4). Several factors, including management and soil properties, could affect the responses to GHG emissions. However, the relative importance of management (RR rate, soil moisture, and nutrient input) factors and the soil parameters to the change in the magnitude of GHG emissions is still uncertain (Wang et al., 2018; Zhou et al., 2021; Mirzaei et al., 2022; Zhang et al., 2022).
The imperative to mitigate GHG emissions from agricultural activities has spurred intensive research to understand the factors that govern emissions dynamics, particularly in India, where wheat cultivation is prevalent during the Rabi season. However, the extent to which GHG emissions can be mitigated through crop residue management hinges on a multitude of factors, with soil moisture emerging as a pivotal determinant in this context (Liu et al., 2019; Korkiakoski et al., 2022; Fairbairn et al., 2023). The decomposition of crop residues, a process central to GHG emissions, is profoundly influenced by soil moisture levels, which regulate microbial activity and decomposition rates (Lenka et al., 2022). Furthermore, variations in soil moisture, driven by factors such as precipitation patterns and irrigation practices, can modulate the efficacy of different residue management strategies in mitigating GHG emissions. Previous research has shown that higher residue inputs can enhance microbial activity by providing an immediate and accessible energy source for soil microbes, which boosts microbial respiration and overall activity (Wang et al., 2018; Fang et al., 2020; Zhou et al., 2021; Abalos et al., 2022b; Mirzaei et al., 2022; Zhang et al., 2022). However, the relationship between soil moisture and carbon mineralization is complex, with studies reporting a bell-shaped relationship where moderate moisture levels optimize microbial activity and carbon mineralization (Fairbairn et al., 2023). Excessive moisture can create anaerobic conditions that inhibit microbial activity and decomposition (Korkiakoski et al., 2022). Interaction of soil moisture with other management practices like nitrogen demonstrated that compared with the high soil moisture content (45 mm irrigation), irrigation with 36 mm and 27 mm increased the soil organic carbon by 15.70%−34.46%, with N180-N240 (Li C. et al., 2023).
Nutrient input also plays a significant role in modulating these processes (Li et al., 2021). Elemental stoichiometry resulting from residue C input modulates the humification efficiency of residue C, soil nutrient cycling, and GHG emissions (Kirkby et al., 2013; Fang et al., 2019, 2020; Liu et al., 2021; Singh et al., 2024). The microbial utilization of crop residue-derived labile C sources, microbial biomass growth, and microbial efficiency may be enhanced by balancing the residue C-nutrient stoichiometry through the external nutrient supply, thereby enhancing the formation of soil organic carbon (Fang et al., 2019). In contrast, excess nutrient application and narrowing of the C: N and C:P ratio below 12:1 and 50:1, respectively, relieved the P-shortage of microbial activity and stimulated N2O emissions upon straw addition (Li P. et al., 2023; Wu et al., 2020). The interaction between RR rates, soil moisture, and nutrient input can shift microbial communities from being nutrient-limited to substrate-limited, highlighting the importance of balanced carbon and nutrient inputs (Fang et al., 2018a; Shen et al., 2021). The resource stoichiometry between hot water-extracted organic carbon and mineral nitrogen and the ratio of mineral nitrogen to Olsen-P were crucial in influencing N2O emissions (Wu et al., 2020). This study aims to elucidate these complex interactions, providing insights into sustainable agricultural practices that enhance soil carbon dynamics and mitigate GHG emissions.
The functional relationship between N2O emissions and the rate of crop residue application can be both linear (Singh et al., 2024) and nonlinear (Wang et al., 2012), indicating the influence of other controlling factors on the nature of the relationship. This relationship is influenced by several factors, including the nitrogen content of the residues (Abalos et al., 2022b), and management factors, including soil nutrients and moisture (Mirzaei et al., 2022). There are several studies reporting non-linear responses of N2O to increasing nitrogen fertilizer rates across various cropping systems (Xu et al., 2019; Liyanage et al., 2020; Abalos et al., 2022a; Zhang et al., 2022). The addition of organic carbon input could alter the nonlinear response of N2O to a linear constant emission with increasing N fertilization rates (Xu et al., 2019). Residue incorporation and N fertilization rate linearly increased the loss of reactive N (denitrification, volatilization, leaching, and runoff) in rice-wheat crop rotation (Xia et al., 2020). Studies have shown that high wheat RR (0.5%) and high N fertilization rate (0.96 g·N·pot−1) can increase CO2 emission by 9% compared with no residue however, N2O emission was the highest at medium RR (0.25%) (Wang et al., 2012). Soil moisture, being a key driving variable of soil GHG emission (Stark and Firestone, 1995; Liu et al., 2019; Fairbairn et al., 2023), has been less studied in understanding the functional relationship between GHG emission and RR rates.
Therefore, this study aimed to investigate the effects of varying wheat RR rates (0, 5, 10, and 15 Mg/ha) and nutrient management practices (NS0, NS1, NS2) under two different soil moisture conditions (100% FC and 80% FC) on carbon mineralization and GHG emissions (N2O and CH4) in Alfisols. Alfisols are the dominant soil order in India and occupy 24.25% (79.7 Mha) of the total geographical area in India (Chandrakala et al., 2021). The soil moisture levels were chosen to mimic wet and optimal moisture environments to assess their impact on GHG emissions and global warming potential. We hypothesized that (1) the combined effect of soil moisture levels at 80% field capacity (cf. 100% FC) and increased wheat RR rates (5, 10, and 15 Mg/ha) (cf. R0) will result in a significant reduction in GHG emissions (N2O and CH4) in Alfisols, (2) Nutrient management (NS1 and NS2) shifting the nutrient stoichiometry of C: N: P significantly influence the efficacy of different rates of wheat RR in reducing GHG emissions (cf. NS0), and (3) Soil N immobilization from high RR and residue C/nutrient input stoichiometry (C: N >12:1 and C: P > 50:1) will positively impact N2O reduction compared with RR and residue C/nutrient input stoichiometry (C: N <=12:1; C:P <= 50:1).
2 Materials and methods
2.1 Soil and wheat residue
The surface soil (0–15 cm) used to conduct this investigation was collected from the farmer's field (21.96°N latitude and 77.74°E longitude) that follows a maize-fallow cropping sequence, presenting an Alfisol according to the USDA soil taxonomy. The soil samples were meticulously processed to remove visible roots and big residual bits and then stored at a temperature of 4°C until further investigation. The soil is characterized by a sandy loam texture, 0.68% total organic carbon (TC), 0.06% total nitrogen (TN), and 0.014% total phosphorus (TP) with a C: N ratio of 11.87 and a C: P ratio of 49.61. An elemental analyzer (NC analyzer, Thermofisher, Flash 2000 model) was used to determine total organic C and N concentrations in soil and residues. We used sulphuric acid-perchloric acid digestion and molybdenum antimony colorimetric estimation (Page et al., 1982) to determine total P concentration in soil and residue. The wheat crop residue was collected after the harvest of wheat from an experimental plot under a long-term soybean-wheat cropping system at a recommended dose of fertilizer in the research farm of the Indian Institute of Soil Science. For the residue treatment, the wheat residues were pulverized after air drying and sieved to a size of 2 mm. The relevant properties of wheat residue were 44.78% TC, 0.56% TN, and 0.023% TP, having a C: N ratio of 79.54 and a C: P ratio of 1,946.96.
2.2 Incubation experiment
Soil microcosms were established using 461 ml glass jars with an aluminum screw-cap lid fitted with a septum for gas sampling. The soil was pre-incubated for 10 days at 70% of the two moisture levels and room temperature. Following pre-incubation, an incubation experiment was set up with varied levels of residue and nutrient input under two soil moisture contents (80% and 100% FC). Wheat residues were applied at four levels: 0, 90, 180, and 270 mg (dry weight), which was equivalent to 0 (R0), 5 (R5), 10 (R10), and 15 (R15) Mg ha−1, respectively. The crop residues (<2 mm) were completely mixed with soil (<2 mm) for incubation based on a 10 cm incorporation depth in the field. After residue addition, the nutrient solution was added to all RR treatments at three levels: (1) NS0: no nutrient, (2) NS1, nutrients (N and P) were added to balance the residue C/nutrient stoichiometry of C: N: P:: 1,000:83.3: 20.0 (Fang et al., 2020) to achieve 30% humification of added residue C input at 5 Mg/ha (R5), and 3) NS2= 3x NS1. Therefore, the achieved nutrient stoichiometry varied with RR rate, and the values were 12:1 (C: N) and 50:1 (C: P) in R5 NS1, 24:1 (C: N), and 100:1 (C: P) in R10 NS1 and 36:1 (C: N) and 150:1 (C: P) in R15 NS1. Additionally, stoichiometry was 4:1 (C: N) and 17:1 (C: P) in R5 NS2, 8:1 (C: N), and 33:1 (C: P) in R10 NS2, and 12:1 (C: N) and 50:1 (C: P) in R15 NS2. Nutrient solutions corresponding to nutrient levels were prepared using AR grade urea and potassium dihydrogen phosphate, and 10 M sodium hydroxide solution was used to modify the pH of the nutrient solution to 7. All treatments were replicated three times and incubated at 30°C incubation temperature at 80% FC and 100% FC soil moisture content. This temperature was selected because the aboveground mean annual surface temperature was ca. 30°C in sub-tropical and semi-arid regions during different crop-growing seasons (Lenka et al., 2022). Triplicate empty glass jars were also incubated to account for the headspace GHGs. Field capacity (FC) was measured at matric potentials of −33 kPa using sieved (<2 mm) soil samples in pressure plate extractors from Soil Moisture Equipment Corp., Santa Barbara, CA, with FC moisture content at 0.14 m3m−3. Soil moisture was maintained through regular weighing and water addition to compensate for evaporation losses during gas sampling intervals.
2.3 GHG sampling and measurements
The GHG fluxes from different treatments were measured in gas chromatography (Agilent Technologies model 7890A). Headspace gases were drawn from the incubation jars using a syringe and immediately transferred to a 10 ml evacuated glass at frequent intervals for 116 days of incubation. The CH4/CO2/N2O flux rate was calculated as the change in headspace N2O/CH4/CO2 concentration using the ideal gas law and molecular weight of the gases. Cumulative N2O, CH4, and CO2 emissions were determined by linear integration of daily fluxes. The global warming potential of CO2-equivalent was calculated by multiplying the cumulative N2O and CH4 emissions by their respective radiative forcing potentials using the following equation (Singh et al., 2024):
GWP (mg CO2 eq. kg−1 soil) = CH4 (mg kg−1 soil) × 27.2 + N2O (mg kg−1 soil) × 273 + CO2 (mg kg−1 soil) × 1
2.4 Post incubation soil properties
After the incubation period of 116 days, soil samples were analyzed for relevant soil properties such as soil minerals nitrogen (NO3-N and NH4-N), alkaline phosphatase, soil microbial biomass carbon (SMBC), and labile SOC following standard analytical procedures. The moisture content was determined gravimetrically using the oven-dry method in a part of the moist composite soil samples. Soil mineral nitrogen was estimated after extraction in 2M KCl, and subsequent analysis employed standard methods (Kempers, 1974). Labile SOC calculations utilized the potassium permanganate oxidation method (Blair et al., 1995; Islam et al., 2003) and measured alkaline phosphatase as an indicator of P demand (Alef and Nannipieri, 1995). Soil MBC was determined by a fumigation-extraction method, and a conversion factor of 0.45 was applied to determine SMBC (Joergensen and Brookes, 1990).
2.5 Statistical analysis
All data underwent tests for normality and homogeneity of variance, with transformations applied as needed. Statistical analyses were performed using SPSS software, setting a significance threshold of p=0.05. The general linear model univariate ANOVA was employed, followed by Tukey's HSD test for multiple comparisons of means. Curve fittings were applied to model the response of N2O, CH4, and CO2 emissions to residue rates. The coefficient of determination (R2) and variance metrics (SST, sum of squares for total; SSR, sum of squares for regression; SSE, sum of squares for error) were used to assess model fit. The function with the highest R2 value at P <0.05 was chosen for the least significant differences (LSD) test. In cases where R2 values were identical among models, the function with the lower SSE value, indicating reduced systematic error, was selected as the best representation of the GHG response. The relative effect of management variables (residue and nutrient input, soil moisture) and post-harvest soil parameters (NO3-N, NH4-N, SMBC, labile C, and alk. PO4) on soil GHG emission (CO2/CH4/N2O) was evaluated using Pearson correlation (two-tailed significance) and partial least squares (PLS) regression models. The variable influence projection (VIP) score of value > 0.8 (Gómez-Gener et al., 2018) was used to identify the potential drivers of GHG emission in Alfisols under various managements. PLS is a regression multivariate analytical technique that enables the assessment of relationships between multiple and collinear independent (X; potential explanatory variables: RR rate, soil moisture, nutrient stoichiometry of C: N, C: P, N input, SMBC, NO3-N, NH4-N, SMBC, labile C, and alk. PO4) and dependent (Y; N2O, CH4, and CO2 flux) data matrices. The model's predictive ability was enhanced by decomposing the X and Y variables into new, fewer uncorrelated orthogonal latent factors, and the optimal PLS model was selected based on the minimum cross-validation root PRESS to produce a linear regression model. The predicted residual sum of squares of the model was extracted using the optimal number of factors in the cross-validation. The model with the lowest root mean predicted residual sum of squares is the optimal number of factors. The model performance was analyzed using the root mean square error (RMSE), adjusted (R2) coefficient of determination, and p-value between observed and predicted values due to their robustness, simplicity, and widespread use. All the graphs were constructed using Origin Pro, version 2024b (Origin Lab Corporation, Northampton, MA, USA).
3 Results
3.1 Carbon mineralization
The carbon mineralization in control soil and soil plus crop residue measured as magnitude of soil CO2 flux was significantly the highest in the treatment receiving R15 + NS2 at 100% FC (445.61 mg C kg−1 soil) and the lowest R0 + 100% FC + NS2 (38.70 mg C kg−1 soil) (Table 1). The factor effect of wheat RR increased (P <0.001), but nutrient input (NSL) and soil moisture did not change cumulative carbon mineralization with significant interactions between RR rate x soil moisture x NS (P=0.008), RR rate x soil moisture, and RR rate x NS (Supplementary Table S1). Increasing soil moisture from 80% FC to 100% FC relatively decreased the carbon mineralization though not significantly at all RR rates averaged across nutrient input (Figure 1). The response of carbon mineralization to increasing RR changed from linear (y = 21.57x + 45.16, R2 = 0.99) to curvilinear (y = −1.51x2 + 40.61x + 48.01, R2=0.99) with increasing soil moisture from 80% FC to 100% FC across nutrient management (Figure 2). Across soil moisture, adding nutrients with crop residue had no significant effect on carbon mineralization at R0 and R5. However, C mineralization decreased at a RR rate of R10 and increased at R15 with increasing nutrient input shifting the stoichiometry (Supplementary Figure S1).
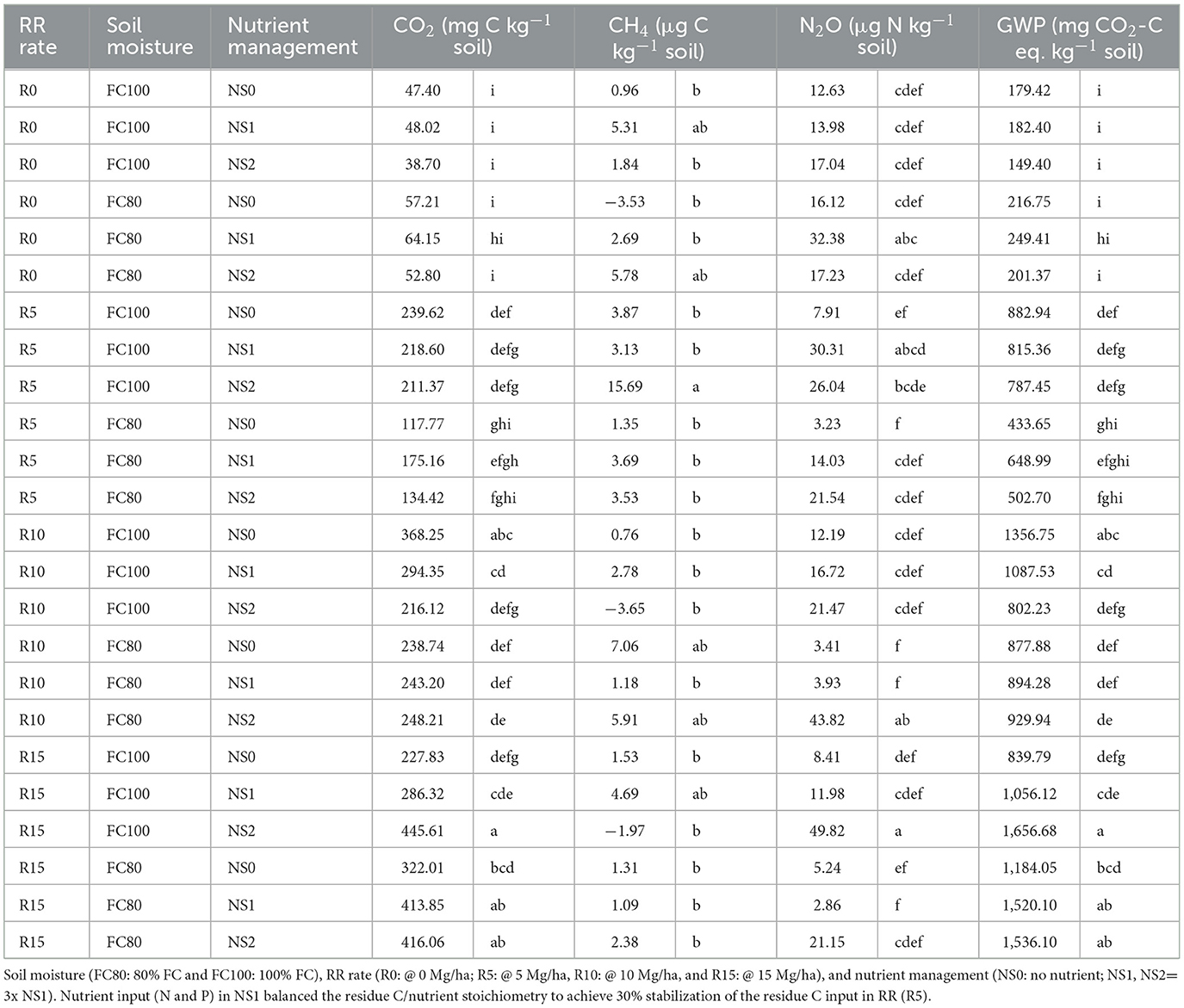
Table 1. Effect of varied residue return (RR) and nutrient input (NSL) on cumulative soil GHG emission (CO2, CH4, and N2O) and global warming potential under different soil moisture content for 116 days of incubation.
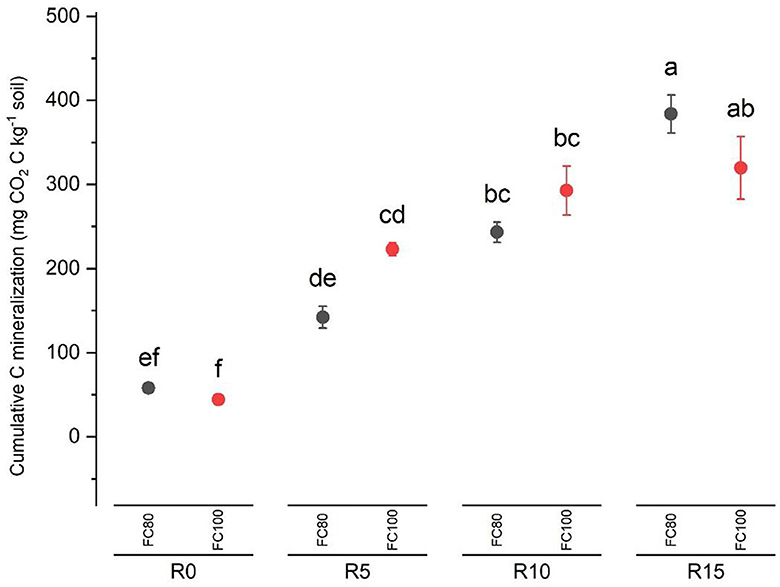
Figure 1. Effect of soil moisture on cumulative carbon mineralization (mg CO2 C kg−1 soil) under different rates of wheat residue return (R0: @ 0 Mg/ha; R5: @ 5 Mg/ha, R10: @ 10 Mg/ha, and R15: @ 15 Mg/ha) averaged across nutrient input over116 days of incubation. Vertical bars represent the mean ± standard error (n=3). Different lower-case letters indicate significant differences among treatments at α < 0.05.
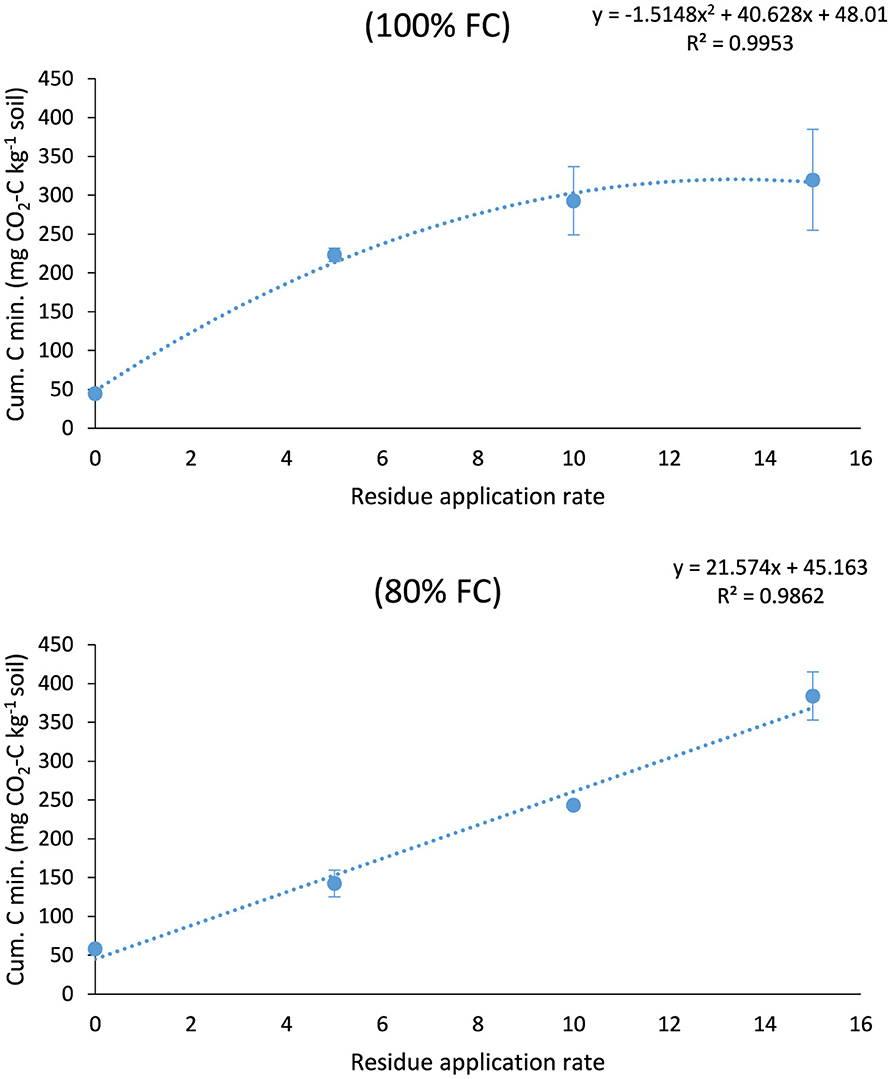
Figure 2. The response of cumulative carbon mineralization (mg CO2 C kg−1 soil) to different application rates of wheat residue (R0: @ 0 Mg/ha; R5: @ 5 Mg/ha, R10: @ 10 Mg/ha, and R15: @ 15 Mg/ha) under 80 and 100% FC averaged across nutrient input over116 days of incubation.
3.2 Nitrous oxide (N2O) fluxes
Increasing soil moisture from 80% to 100% FC increased the N2O fluxes across residue and nutrient management, and the magnitude was significantly the highest in the treatment receiving R15 + NS2 at 100% FC (49.82 μg N kg−1 soil) and the lowest R15 + 80% FC + NS1 (2.85 μg N kg−1 soil) (Table 1). Nutrient input shifting the stoichiometry had a significant effect only at higher RR rates (R10 and R15) (Supplementary Figure S2). Only the main effect of nutrient input (NS, P < 0.0001) was significant, with significant interactions between RR rate x soil moisture x NS (P < 0.0001), RR rate x soil moisture, and RR rate x NS (Supplementary Table S1, Figure 3). Regression analysis revealed a linear response in N2O emissions toward RR rates (y = 0.4364x + 15.463 R2 = 0.47) at 100% FC. In contrast, the response was quadratic (y = 0.0167x2 – 0.8971x + 20.68 R2 = 0.63) at 80% FC, i.e., wheat residue application with an increment of @ 5 Mg/ha from 5 to 15 Mg/ha decreased the N2O emission from 12.9 to 9.7 μg N kg−1 compared to soil without residue (21.9 μg N kg−1) (Figure 4). Aditionaly the sunburst constructed indicated the decreasing proportion of N2O emission with RR rate at 80% FC (Figure 5). However, increasing nutrient input, significantly increased the N2O fluxes NS2 (27.26 μg N kg−1 soil) >NS1 (15.77 μg N kg−1 soil) >NS0 (8.64 μg N kg−1 soil) averaged across residue management and soil moisture.
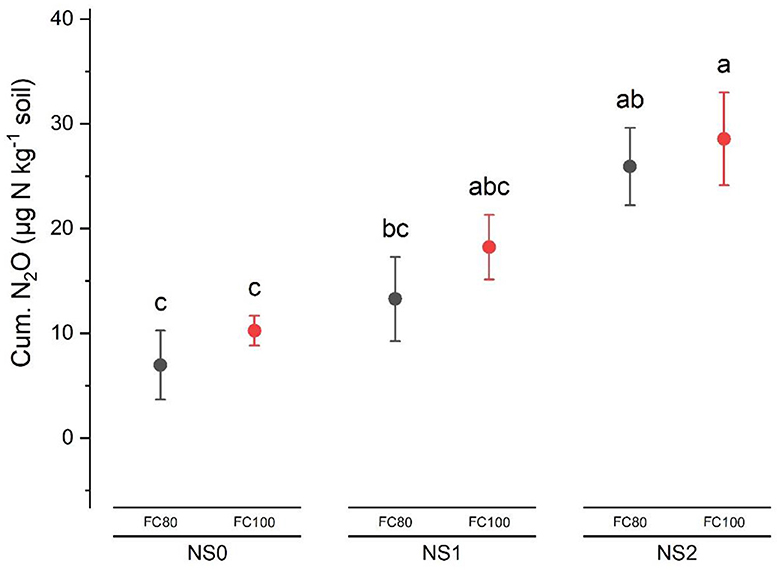
Figure 3. Effect of soil moisture on cumulative N2O fluxes (μg N kg−1 soil) under different nutrient management averaged across RR rates over 116 days of incubation. Vertical bars represent the mean ± standard error (n=3). Different lower-case letters indicate significant differences among treatments at α < 0.05.
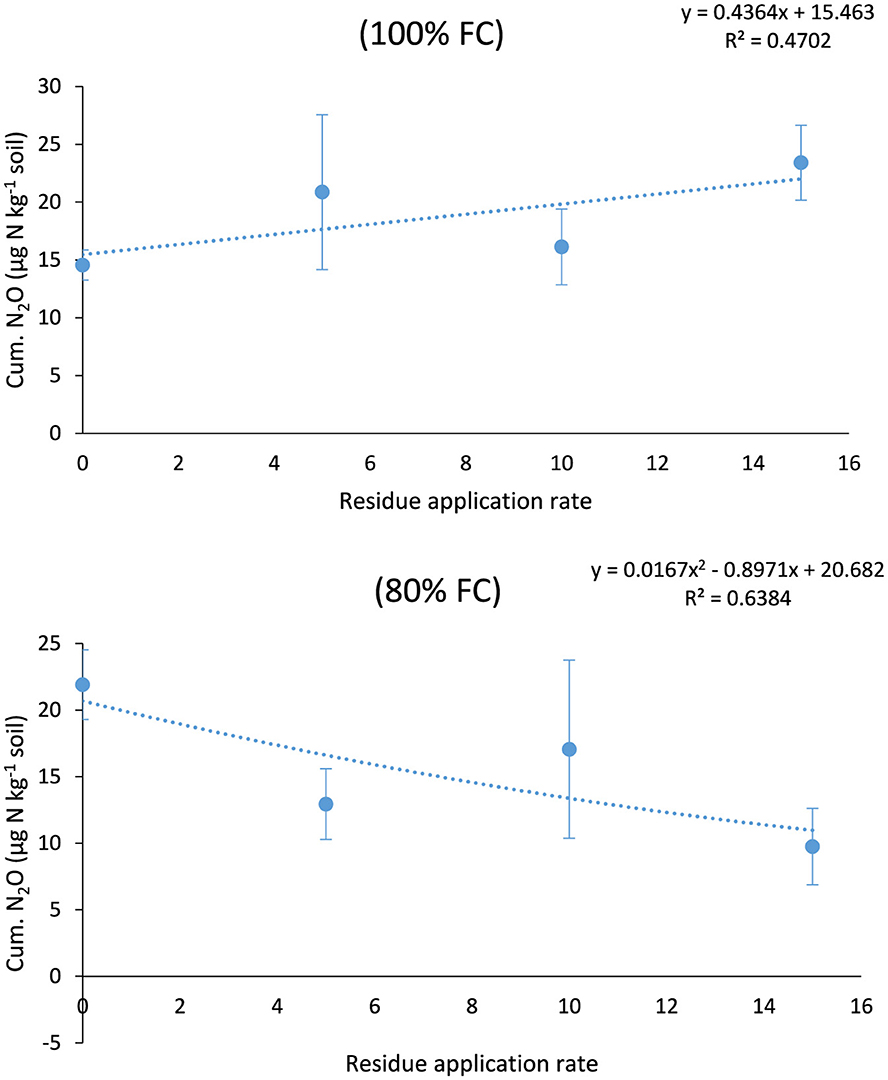
Figure 4. The response of N2O fluxes (μg N kg−1 soil) to different application rates of wheat residue (R0: @ 0 Mg/ha; R5: @ 5 Mg/ha, R10: @ 10 Mg/ha, and R15: @ 15 Mg/ha) under 80 and 100% FC across nutrient management over 116 days of incubation.
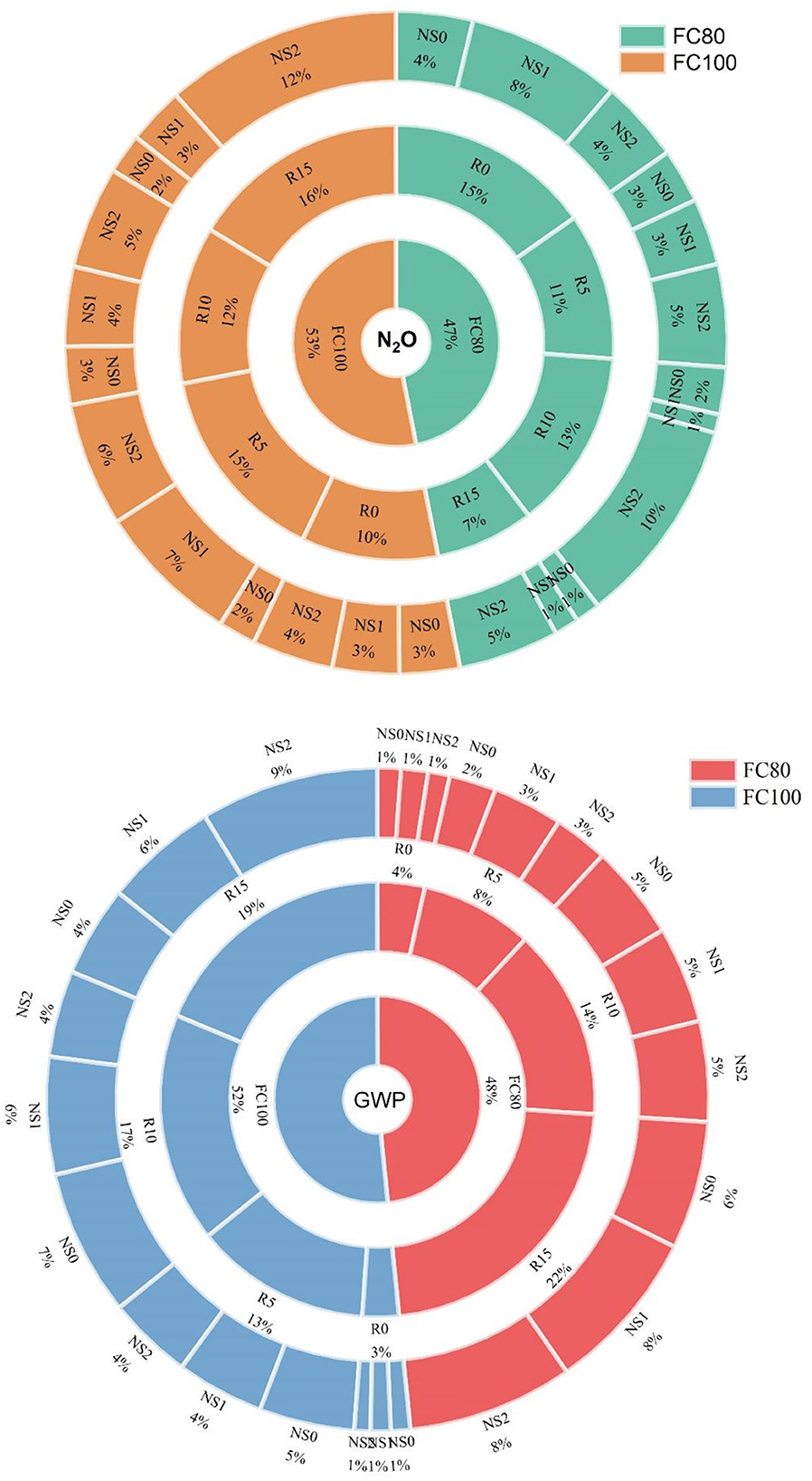
Figure 5. A sunburst chart illustrating the effect of soil moisture (80% FC and 100% FC), residue return rates (R0: @ 0 Mg/ha; R5: @ 5 Mg/ha, R10: @ 10 Mg/ha, and R15: @ 15 Mg/ha), and nutrient management (NS0, NS1 and NS2) on cumulative (a) N2O emissions and (b) GWP over 116 days of incubation.
3.3 Methane (CH4) fluxes
The average cumulative CH4 fluxes ranged from 15.69 μg C kg−1 soil (R5+NS2 at 100% FC) and the lowest in −3.65 μg C kg−1 soil (R10+NS2 at 100% FC) (Table 1). Changing soil moisture influenced the response of CH4 fluxes to the rate of wheat residue application. Averaged across nutrient input at 100% FC soil moisture, the trend of CH4 fluxes was linear, and maximum emissions occurred at an RR rate of 5 Mg/ha (Figure 6). In contrast, at 80% FC averaged across nutrient management, cumulative CH4 fluxes had a curvilinear response to increasing RR rate, with emissions increasing sharply from 0 (R0) to 10 Mg/ha (R10) and then declining at an RR rate of 15 Mg/ha (R15). The methane fluxes were the lowest mean value of 1.5 μg C kg−1 soil in the treatment, receiving RR rate of 15 Mg/ha in both the soil moisture content and averaged across nutrient management (Figure 6).
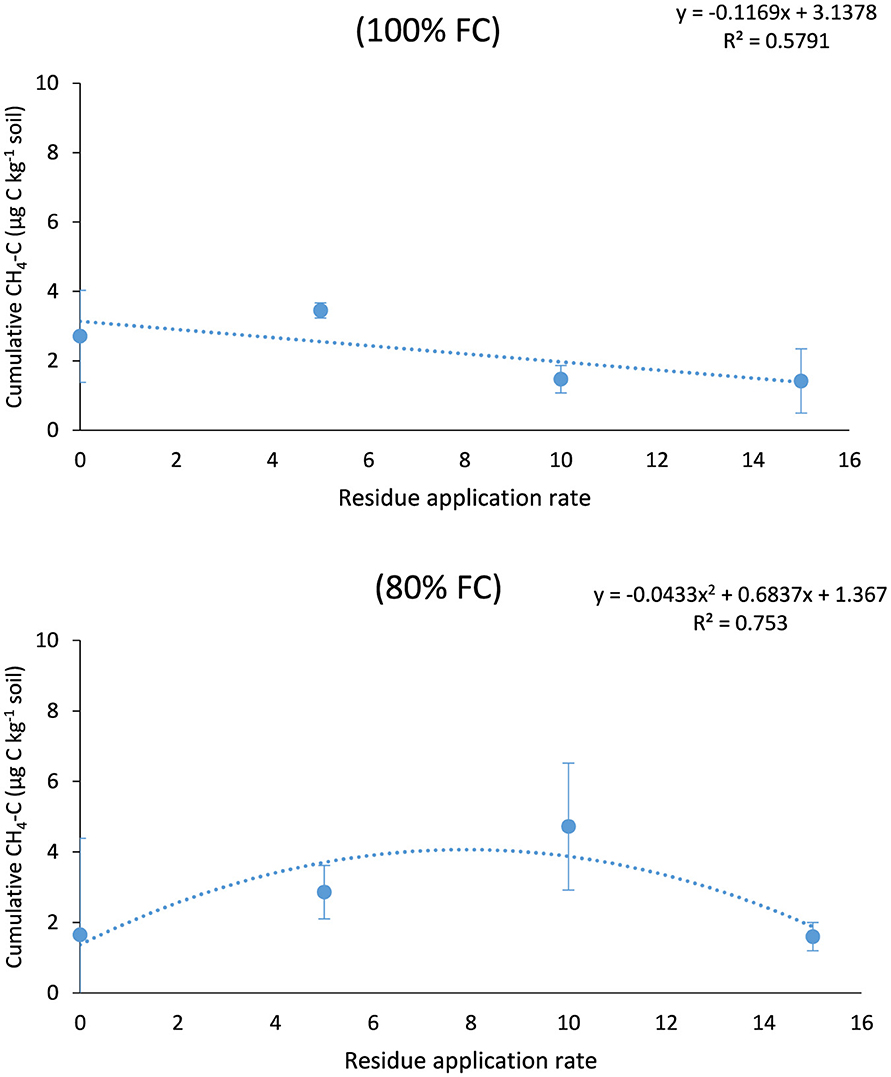
Figure 6. The response of CH4 fluxes (μg C kg−1 soil) to different application rates of wheat residue (R0: @ 0 Mg/ha; R5: @ 5 Mg/ha, R10: @ 10 Mg/ha, and R15: @ 15 Mg/ha) under 80 and 100% FC across nutrient management over116 days of incubation.
3.4 Global warming potential
The interactive effects of RR rate, soil moisture, and nutrient management significantly influenced SOC mineralization (Supplementary Table S1). The interactive effect of R15 + NS2 at 100% FC was the highest (1656.82 mg CO2 C eq. kg−1 soil), followed by R0 + NS2 at 100% FC (149.40 mg CO2 C eq. kg−1 soil) (Table 1). Increasing wheat RR rates triggered the GWP across soil moisture and nutrient management and had a relatively lesser GWP at 80% FC compared with 100% FC (Figure 5). The effect of soil moisture was inconsistent on GWP, for example, at 80% FC the GWP increased with application rates of wheat residue (R15 > R10 > R0); however, the RR rates were comparable at 100% FC (R5≈R10≈R15).
3.5 Post-incubation soil properties
The post-incubation labile soil carbon, soil microbial biomass, ammoniacal N (NH4-N), and alkaline phosphatase responded significantly to the interaction of wheat RR rate, soil moisture, and nutrient management except NO3-N (Table 2, Supplementary Table S1). With treatment, receiving R15+NS2 at 80% FC had the highest labile soil C (528.87 mg C kg−1) and the lowest in R0 + NS0 at 100% FC (323.29 mg C kg−1). Higher rates of wheat RR significantly reduced the soil NO3-N by 1.55 times and 2.49 times at R10 and R15 compared to R0 and R5. Contrarily, the concentration increased with increasing soil moisture and nitrogen input. The interactive effect of R5 + NS1 at 100% FC (50.58 mg N Kg−1) was the highest, and R15 + NS0 at 80% FC (6.80 mg N kg-1) was the lowest on soil NO3-N. In contrast, soil NH4-N and SMBC increased significantly with increasing wheat RR, the order being R15>R10>R5>R0 (NH4-N) R10> R15≈R5> R0 (SMBC), and increasing soil moisture and nutrient concentration decreased NH4-N. The interactive effect of R5 + NS0 at 100% FC (39.31 mg N Kg−1) was the highest, and R5 + NS1 at 80% FC (1.63 mg N kg−1) was the lowest on soil NH4-N. In contrast, with increasing wheat RR, NS, and soil moisture, the SMBC increased significantly, the order being R10> R15≈, R5> R0, FC100> FC80, and NS1>NS2> NS0. The highest SMBC was in treatment R5 + NS1 at FC100 (1,503.01 mg kg−1) and the lowest in R5 + NS0 at FC 80 (299.37 mg kg−1). The alkaline phosphatase activity was higher in wheat RR treatments at 80% FC with NS1 compared with R0 NS0 at 100% FC.
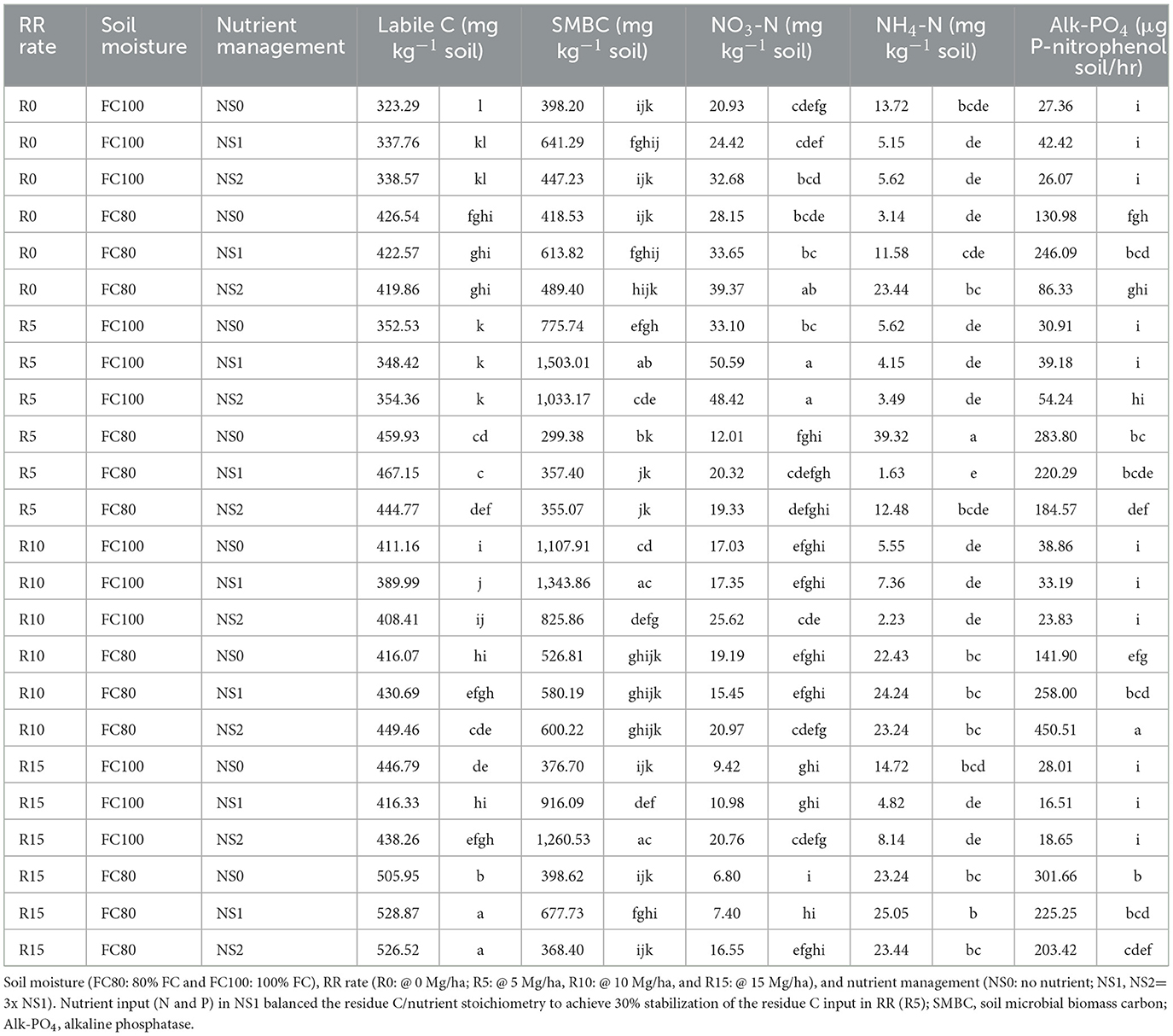
Table 2. Effect of varied residue return (RR) and nutrient input (NSL) on relevant post incubation soil properties under different soil moisture content after 116 days of incubation.
The Pearson correlation analysis was carried out to explore the relationship between GHG emissions and the PLS model predictor variables. Pearson correlation (Figure 7) showed a significant (p ≤ 0.01) positive correlation between N2O and N input, NO3-N, and SMBC, whereas nutrient stoichiometry of C: N and C: P was negative. However, CH4 was only correlated with NO3-N (p ≤ 0.05). Post-incubation soil properties viz., SMBC, labile C, and management variables (RR rate, N input, and nutrient addition altering the nutrient stoichiometry of C: N) were significantly (p ≤ 0.01) positively correlated with CO2 and GWP, whereas NO3-N was negative. The PLS model for GHG emissions extracted two factors from the data matrix that explained 55.49% of the cumulative predictor (X) variance and 38.34% of GHG (Y) responses (Supplementary Table S2). The factor loading plot (Supplementary Figure S3) and the relative importance of predictor variables (Figure 8) in the partial least square (PLS) model indicated that N2O emission was more responsive (values >0.8) to management variables (RR rate, nitrogen (N) input rate, soil moisture, and nutrient stoichiometry of C: N) and post-incubation soil properties (SMBC and NO3-N) in Alfisols. The ranking of the variables important for the projection of GHG fluxes in the Alfisols was as follows: RR rate > N input > soil moisture > NO3-N > SMBC > C: N > Alk.PO4 > C: P> labile C > NH4-N. The model performance was significant (p < 0.05) for the estimation of all three GHGs; however, the model was most effective for the estimation of CO2 (r2 = 0.83) followed by N2O (r2 = 0.54) and CH4 (r2 = 0.17) (Supplementary Figure S4).
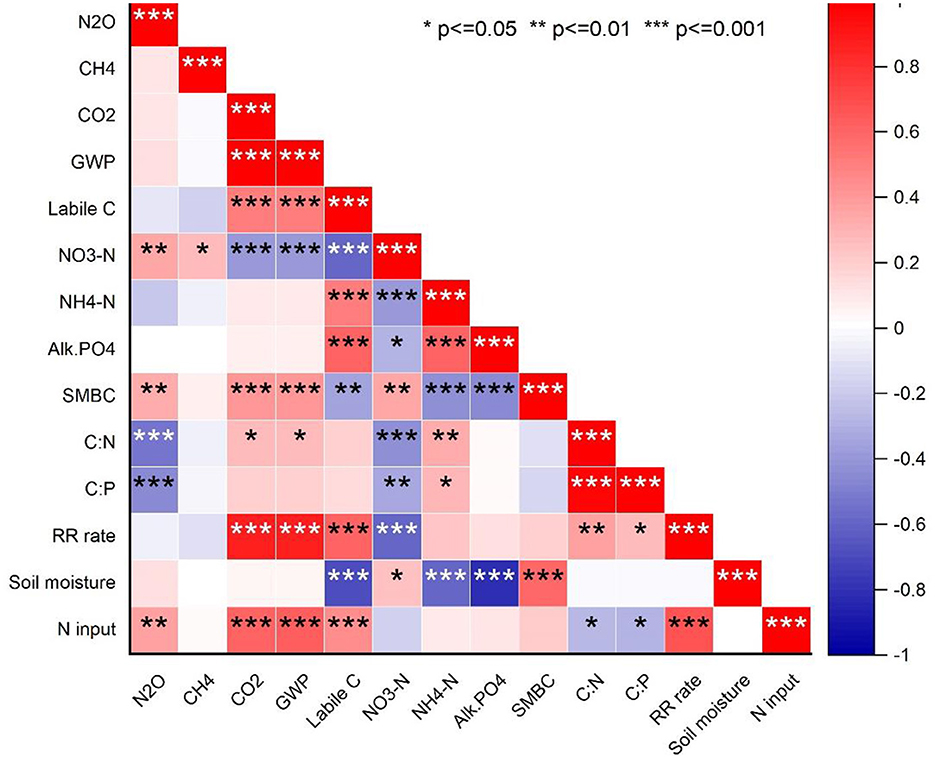
Figure 7. Pearson correlation between observed variables (labile soil carbon, soil microbial biomass, nitrate N: NO3-N; ammoniacal N: NH4-N, and alkaline phosphatase) and responses (CO2, CH4, N2O, and GWP), C: N: ratio of carbon and nitrogen; C: P: ratio of carbon and phosphorus.
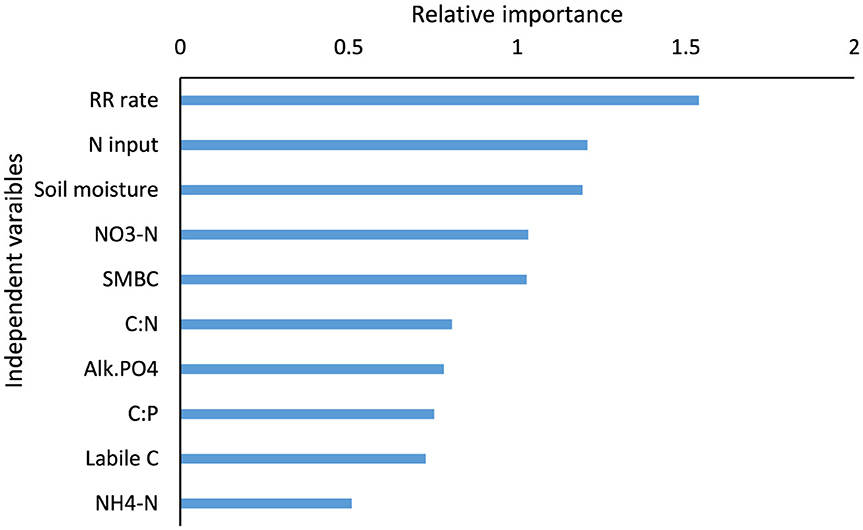
Figure 8. Variable importance of predictors based on partial least square modeling for the effect of management practices and related soil properties on GHG emission. NO3-N: Nitrate N; NH4-N: ammoniacal N; Alk.PO4: alkaline phosphatase; SMBC: soil microbial biomass carbon; RR rate: wheat residue return rate; C: N: stoichiometry of residue C to N input/soil C to N; C: P: stoichiometry of residue C to P input/soil C to P.
4 Discussion
4.1 Soil carbon mineralization
In this study, we aimed to mimic wet (100% FC) and optimum (80% FC) soil moisture in GHG emissions from increasing wheat residue inputs (RR rate 0, 5, 10, and 15 Mg/ha) under three different nutrient management (NS0, NS1, NS2) in Alfisols. The study elucidates the intricate mechanisms underlying carbon mineralization and its interaction with RR rates, soil moisture, and nutrient management. Carbon mineralization, the process of converting organic carbon to carbon dioxide, is primarily driven by microbial activity in soil ecosystems (Lenka et al., 2019). The highest carbon mineralization observed in the treatment with R15 + NS2 at 100% FC suggests that high residue input and nutrient availability significantly enhance microbial activity and soil CO2 fluxes. The positive correlation observed between RR rate and labile C (p <=0.001) and CO2 and labile C (p <=0.001) justifies that labile C from decomposing crop residues might have provided an immediate and accessible energy source for soil microbes (Lenka et al., 2022). That boosted microbial respiration and overall activity as they decompose the organic matter.
In a study, Fairbairn et al. (2023) reported a bell-shaped relationship between soil moisture and CO2 fluxes. This effect is strongly supported by the results obtained in our study, which showed the highest soil carbon mineralization at 80% FC (Ca. 100% FC) after 116 days of incubation. Soil moisture modulated the functional relationship of carbon mineralization to wheat RR rates. The curvilinear response to RR rates at 100% FC indicates that moderate moisture content (80% FC) would be ideal for microbial activity and decomposition, whereas excessive moisture (100% FC) and high RR rate (R15) may lead to anaerobic conditions, inhibiting these processes (Liu et al., 2019). Our experiment results agree with previous studies that have reported decreased carbon mineralization under excess soil moisture content and organic input (Unger et al., 2010; Korkiakoski et al., 2022; Fairbairn et al., 2023). There could be a few possible explanations for these responses to carbon mineralization; firstly, high soil moisture content and residue input could inhibit the diffusion of O2 and CO2 (Fairbairn et al., 2023), secondly decreased soil NO3-N and its negative correlation with CO2 fluxes (Figure 7) (Unger et al., 2010) and third the decomposing crop residue input might have triggered O2 depletion (Lussich et al., 2024) that inhibit microbial activity and decomposition. However, a linear relationship was observed between carbon mineralization and RR rates at 80% FC indicating moderate moisture content could be optimum for maximal microbial activity at all RR rates, as commonly reported (Fairbairn et al., 2023).
At low RR rates (R0 and R5), the addition of nutrients did not significantly influence carbon mineralization. This could be attributed to the limited availability of substrate for microbial activity. The microbial community might not experience sufficient stimulation with zero or minimal organic inputs, thereby rendering nutrient additions ineffective in altering mineralization rates (Li et al., 2021). However, at an intermediate RR rate (R10), a decrease in carbon mineralization with nutrient supplementation was observed. This could be due to nutrient-induced microbial immobilization (Table 2). When nutrients are readily available, microbes might prioritize biomass growth over respiration, leading to lower CO2 release from mineralization (Singh et al., 2024). Carbon mineralization increased with nutrient input at the highest RR rate (R15). This suggested that the microbial community becomes substrate-limited rather than nutrient-limited at high substrate levels. Supplementary nutrients can enhance microbial activity and decomposition, producing higher CO2 emissions (Fang et al., 2018b). Our results align with previous studies highlighting the importance of balancing carbon and nutrient inputs to optimize soil carbon dynamics and microbial activity (Lai et al., 2017). For instance, studies have shown that labile carbon inputs can significantly enhance microbial respiration and nutrient cycling, particularly when supported by adequate nutrient availability (Lan et al., 2017). Post incubation soil results further supported responses of soil carbon mineralization that treatments with higher RR and balanced moisture and nutrient inputs (R15 at 80% FC and NS2) showed the highest labile carbon and microbial biomass, essential for soil fertility and microbial functions.
4.2 N2O emission
In line with previous studies (Abalos et al., 2022a; Khoshru et al., 2023; Korkiakoski et al., 2022; Liu et al., 2019; Liyanage et al., 2020; Mathivanan et al., 2021; Xu et al., 2019), we found a significant stimulation of soil N2O emission following the application of high residues return, nutrient supplement, and soil moisture (R15 + NS2 at 100% FC, 49.82 μg N kg−1 soil) could be attributed to enhanced microbial activity and denitrification processes under wetter conditions (Mehnaz et al., 2019; Liu et al., 2021). The linear response of N2O emissions toward RR rates at 100% FC (y = 0.4364x + 15.463, R2 = 0.47) suggests that higher residue inputs directly contribute to greater N2O emissions due to increased substrate availability for microbial processes. In contrast, the quadratic response observed at 80% FC (y = 0.0167x2 - 0.8971x + 20.68, R2 = 0.63) indicates that moderate residue (R10) return may optimize conditions for N2O production, whereas excessively high or low residue inputs are favorable for N2O consumption. Averaged across soil moisture and RR rate carbon nutrient stoichiometry (C: N and C: P) further amplified N2O fluxes, with NS2 treatments showing the highest emissions (27.26 μg N kg−1 soil) compared to NS1 (15.77 μg N kg−1 soil) and NS0 (8.64 μg N kg−1 soil). This effect is consistent with studies reporting lowering carbon nutrient stoichiometry removed microbial nutrient limitation, stimulating nitrification and denitrification processes, leading to increased N2O production (Mehnaz et al., 2019; Liyanage et al., 2020; Li et al., 2021). Post-incubation analyses revealed a reduction in nitrate (NO3-N) levels with high RR and nutrient stoichiometry could be attributed to increased microbial immobilization, while ammonium (NH4-N) concentrations rise, indicating shifts in nitrogen forms during decomposition (Kumar et al., 2018; Lenka et al., 2021; Khoshru et al., 2023) resulting in the lowest N2O emission in R15 + 80% FC + NS1 (2.85 μg N kg−1 soil). Conversely, the increase in NH4-N and SMBC with higher RR and moisture levels indicates that these conditions favor ammonification and microbial biomass growth (Fang et al., 2020). The highest SMBC was recorded in the R5+NS1 treatment at 100% FC (1,503.01 mg kg−1), emphasizing the importance of balanced residue and nutrient management for maintaining soil microbial health (Mehnaz et al., 2019). The positive correlations observed between N2O emissions and SMBC, NO3-N, and N input highlight the interconnectedness of microbial processes, nitrogen cycling, and GHG emissions (Yang et al., 2022). Additionally, the significant negative correlation between nutrient stoichiometry (C: N and C: P) and N2O emission supported our results of the lowest N2O emission in treatment R15 + 80% FC + NS1 (C: N >12:1 and C: P > 50:1). The variable importance plot from the partial least square (PLS) model further underscores the significant influence of nutrient stoichiometry (C: N and C: P), N input, soil microbial biomass, soil mineral N (NO3-N and NH4-N), on soil N2O emissions.
4.3 CH4 emission
Our results showed that a combination of a low RR rate (@5 Mg/ha) and high soil moisture (100% FC) significantly increased the cumulative CH4 fluxes compared with a high RR rate (R10, R15) and medium soil moisture (80% FC) with high nutrient input (NS2), This indicates a non-linear response of CH4 emissions to residue application rates (Figure 6), with emissions initially increasing and then decreasing at higher RR. The curvilinear trend at 80% FC suggests that intermediate levels of RR may create conditions that enhance methanogenic activity (Bhattacharyya et al., 2012), likely due to optimal substrate availability and microbial activity, consistent with findings from other studies that show similar non-linear patterns in GHG emissions from soil residue management (Yang et al., 2022; Singh et al., 2024). The study's findings are underpinned by several key mechanisms. The response of CH4 fluxes to RR suggests a balance between methanogenesis and methanotrophy, regulated by substrate availability and soil moisture (Liu et al., 2021; Korkiakoski et al., 2022; Yang et al., 2022; Khoshru et al., 2023). High RR may initially promote methanogenesis by providing substrates, but excessive residue might create anaerobic conditions less favorable for methanotrophy, explaining the decline in CH4 emissions at higher RR rates (Battaglia et al., 2022; Yang et al., 2022). The significant correlation of CH4 emission with soil NO3-N further supports the results of lesser emission at a high RR rate (R10 and R15) because the RR rate was negatively correlated with NO3-N (Figure 7).
4.4 Global warming potential
The PLS model revealed that soil management factors (RR rate, nitrogen (N) input rate, and soil moisture) were more important than the post-incubation soil properties as all of them reached the threshold of 0.8 (Jiang et al., 2023). Therefore, a significant increase in GWP with high RR and nutrient input at 100% field capacity (FC) enhanced SOC mineralization (Figure 1), while lower residue and nutrient inputs at 100% FC showed the lowest mineralization. Increasing wheat RR rates generally increased GWP, particularly at higher soil moisture levels (100% FC), due to enhanced microbial activity and decomposition of organic (Abalos et al., 2022b). Our results showed a significant (p ≤ 0.001) positive correlation between RR rate, N input, SMBC, and soil moisture with GWP, further supporting enhanced GWP with RR. Previous studies corroborate these findings, showing that soil moisture content and nutrient availability critically influence the magnitude of GWP by affecting microbial processes involved in GHG production (Pareja-Sánchez et al., 2019; Abalos et al., 2022a; Battaglia et al., 2022; Mirzaei et al., 2022; Jiang et al., 2023). Therefore, our results underscore the need to optimize crop RR rate with soil moisture and residue C/nutrient stoichiometry for reduced GHG emissions. Managing these factors can optimize residue management practices to mitigate climate change impacts while enhancing soil health and productivity. Researchers and farmers can use nutrient stoichiometry (C: N balance) to fine-tune nitrogen and phosphorus inputs, maximizing residue stabilization while controlling N2O fluxes. This can lead to more efficient fertilizer use, reducing environmental impacts and costs. The results highlighted the importance of integrating soil moisture and nutrient inputs in residue management guidelines, providing a framework for policymakers to develop strategies for climate-smart agriculture. We recommend further studies to explore site-specific residue management practices, particularly under varying climatic and soil conditions, to enhance their effectiveness in reducing greenhouse gas emissions. Future research should focus on long-term field studies to validate these findings and develop practical guidelines for sustainable residue management.
5 Conclusions
Post-incubation soil properties such as labile soil carbon, soil microbial biomass carbon (SMBC), and soil mineral N (NO3-N and NH4-N) were significantly impacted by the interaction of wheat RR, soil moisture and nutrient input that, in turn, affected the differential responses of GHG emissions from Alfisols. Soil moisture altered the responses of GHG emissions to RR rate. Quadratic non-linear responses of N2O and CH4 were observed at medium soil moisture content (80% FC) across nutrient management. The curvilinear response to RR rate at 100% FC indicates that moderate moisture content (80% FC) would be ideal for microbial activity and soil carbon mineralization. Nitrous oxide emissions were significantly influenced by soil moisture and nutrient management, with the highest fluxes recorded in the R15 + NS2 (C: N:: 12:1 and C: P:: 50:1) at 100% FC and the lowest in treatment receiving R15 + 80% FC + NS1 (C: N > 12:1 and C: P > 50:1). A strong positive correlation was found between N2O emissions and post-incubation soil properties, while CH4 fluxes were mainly correlated with NO3-N. The relative variable importance analysis using the partial least squares (PLS) model identified the profound effect of RR rate followed by N input, soil moisture, NO3-N, SMBC, and nutrient stoichiometry C: N in estimating GHG emissions from Alfisols. This study underscores the intricate interactions between soil moisture, residue, and nutrient management in reducing GHG emissions and improving soil health, highlighting the need for optimized residue and nutrient input strategies to mitigate climate change impacts and enhance soil fertility in Alfisols.
Data availability statement
The original contributions presented in the study are included in the article/Supplementary material, further inquiries can be directed to the corresponding author.
Author contributions
DS: Writing – original draft, Methodology, Investigation, Formal analysis. SL: Writing – review & editing, Writing – original draft, Supervision, Project administration, Methodology, Funding acquisition, Conceptualization. NKL: Writing – review & editing, Resources, Investigation, Formal analysis. DKY: Data curation, Software, Writing – original draft. SSY: Conceptualization, Supervision, Writing – original draft. RSK: Writing – review & editing, Software, Formal analysis. AS: Writing – original draft, Resources, Investigation. MS: Writing – original draft, Software.
Funding
The author(s) declare financial support was received for the research, authorship, and/or publication of this article. This research was financially supported by the Science and Engineering Research Board, POWER Fellowship (Grant No. SPF/2020/000022) and the instrument from National Agricultural Science Fund of the Indian Council of Agricultural Research, New Delhi (Grant No. NASF/CA-7019/2018-19).
Acknowledgments
The Sangeeta Lenka expresses sincere gratitude to the USDA-Foreign Agricultural Service for granting the Scientific Exchange Program Fellowship on Climate Smart Agriculture. We gratefully acknowledge the editor's and reviewers' invaluable contributions in shaping this research.
Conflict of interest
The authors declare that the research was conducted in the absence of any commercial or financial relationships that could be construed as a potential conflict of interest.
Publisher's note
All claims expressed in this article are solely those of the authors and do not necessarily represent those of their affiliated organizations, or those of the publisher, the editors and the reviewers. Any product that may be evaluated in this article, or claim that may be made by its manufacturer, is not guaranteed or endorsed by the publisher.
Supplementary material
The Supplementary Material for this article can be found online at: https://www.frontiersin.org/articles/10.3389/fsufs.2024.1490523/full#supplementary-material
References
Abalos, D., Recous, S., Butterbach-Bahl, K., De Notaris, C., Rittl, T. F., Topp, C. F. E., et al. (2022a). A review and meta-analysis of mitigation measures for nitrous oxide emissions from crop residues. Sci. Total Environ. 828:154388. doi: 10.1016/j.scitotenv.2022.154388
Abalos, D., Rittl, T. F., Recous, S., Thiébeau, P., Topp, C. F. E., van Groenigen, K. J., et al. (2022b). Predicting field N2O emissions from crop residues based on their biochemical composition: a meta-analytical approach. Sci. Total Environ. 812:152532. doi: 10.1016/j.scitotenv.2021.152532
Alef, K., and Nannipieri, P. (1995). Methods in Applied Soil Microbiology and Biochemistry. Academic Press.
Battaglia, M. L., Thomason, W. E., Fike, J. H., Evanylo, G. K., Stewart, R. D., Gross, C. D., et al. (2022). Corn and wheat residue management effects on greenhouse emissions in the mid-Atlantic USA. Land 11:846. doi: 10.3390/land11060846
Bhattacharyya, P., Roy, K. S., Neogi, S., Adhya, T. K., Rao, K. S., and Manna, M. C. (2012). Effects of rice straw and nitrogen fertilization on greenhouse gas emissions and carbon storage in tropical flooded soil planted with rice. Soil Tillage Res. 124, 119–130. doi: 10.1016/j.still.2012.05.015
Blair, G. J., Lefroy, R. D., and Lisle, L. (1995). Soil carbon fractions based on their degree of oxidation, and the development of a carbon management index for agricultural systems. Aust. J. Agric. Res. 46, 1459–1466. doi: 10.1071/AR9951459
Chandrakala, M., Lakhsman, K., and Maske, S. P. (2021). Indian soils: characteristics, distribution, potentials and constraints. Chron. Bioresour. Manag. 4, 121−127.
Deshpande, M. V., Kumar, N., Pillai, D., Krishna, V. V., and Jain, M. (2023). Greenhouse gas emissions from agricultural residue burning have increased by 75% since 2011 across India. Sci. Total Environ. 904:166944. doi: 10.1016/j.scitotenv.2023.166944
Fairbairn, L., Rezanezhad, F., Gharasoo, M., Parsons, C. T., Macrae, M. L., Slowinski, S., et al. (2023). Relationship between soil CO2 fluxes and soil moisture: anaerobic sources explain fluxes at high water content. Geoderma 434:116493. doi: 10.1016/j.geoderma.2023.116493
Fang, Y., Nazaries, L., Singh, B. K., and Singh, B. P. (2018a). Microbial mechanisms of carbon priming effects revealed during the interaction of crop residue and nutrient inputs in contrasting soils. Glob. Chang. Biol. 24, 2775–2790. doi: 10.1111/gcb.14154
Fang, Y., Singh, B. P., Collins, D., Armstrong, R., Van Zwieten, L., and Tavakkoli, E. (2020). Nutrient stoichiometry and labile carbon content of organic amendments control microbial biomass and carbon-use efficiency in a poorly structured sodic-subsoil. Biol. Fertil. Soils 56, 219–233. doi: 10.1007/s00374-019-01413-3
Fang, Y., Singh, B. P., Collins, D., Li, B., Zhu, J., and Tavakkoli, E. (2018b). Nutrient supply enhanced wheat residue-carbon mineralization, microbial growth, and microbial carbon-use efficiency when residues were supplied at high rate in contrasting soils. Soil Biol. Biochem. 126, 168–178. doi: 10.1016/j.soilbio.2018.09.003
Fang, Y., Singh, B. P., Cowie, A., Wang, W., Arachchi, M. H., Wang, H., et al. (2019). Balancing nutrient stoichiometry facilitates the fate of wheat residue-carbon in physically defined soil organic matter fractions. Geoderma 354:113883. doi: 10.1016/j.geoderma.2019.113883
Gómez-Gener, L., Gubau, M., von Schiller, D., Marcé, R., and Obrador, B. (2018). Effect of small water retention structures on diffusive CO2 and CH4 emissions along a highly impounded river. Inl. Waters 8, 449–460. doi: 10.1080/20442041.2018.1457846
Islam, K. R., Stine, M. A., Gruver, J. B., Samson-Liebig, S. E., and Weil, R. R. (2003). Estimating active carbon for soil quality assessment: a simplified method for laboratory and field use. Am. J. Altern. Agric. 18, 3–17. doi: 10.1079/AJAA2003003
Jiang, Z., Yang, S., Smith, P., and Pang, Q. (2023). Ensemble machine learning for modeling greenhouse gas emissions at different time scales from irrigated paddy fields. F. Crop. Res. 292:108821. doi: 10.1016/j.fcr.2023.108821
Joergensen, R. G., and Brookes, P. C. (1990). Ninhydrin-reactive nitrogen measurements of microbial biomass in 0.5 m K2SO4 soil extracts. Soil Biol. Biochem. 22, 1023–1027. doi: 10.1016/0038-0717(90)90027-W
Kempers, A. J. (1974). Determination of sub-microquantities of ammonium and nitrates in soils with phenol, sodium nitroprusside and hypochlorite. Geoderma 12, 201–206. doi: 10.1016/0016-7061(74)90068-8
Khoshru, B., Khoshmanzar, E., Asgari Lajayer, B., and Ghorbanpour, M. (2023). “Chapter 29 - Soil moisture-mediated changes in microorganism biomass and bioavailability of nutrients in paddy soil,” in Plant Stress Mitigators, eds. M. Ghorbanpour and M. Adnan Shahid (Academic Press), 479–494. doi: 10.1016/B978-0-323-89871-3.00005-7
Kirkby, C. A., Richardson, A. E., Wade, L. J., Batten, G. D., Blanchard, C., and Kirkegaard, J. A. (2013). Carbon-nutrient stoichiometry to increase soil carbon sequestration. Soil Biol. Biochem. 60, 77–86. doi: 10.1016/j.soilbio.2013.01.011
Korkiakoski, M., Määttä, T., Peltoniemi, K., Penttilä, T., and Lohila, A. (2022). Excess soil moisture and fresh carbon input are prerequisites for methane production in podzolic soil. Biogeosciences 19, 2025–2041. doi: 10.5194/bg-19-2025-2022
Kumar, K., and Goh, K. M. (1999). Crop residues and management practices: effects on soil quality, soil nitrogen dynamics, crop yield, and nitrogen recovery. Adv. Agron. 68, 197–319. doi: 10.1016/S0065-2113(08)60846-9
Kumar, M., Kundu, D. K., Ghorai, A. K., Mitra, S., and Singh, S. R. (2018). Carbon and nitrogen mineralization kinetics as influenced by diversified cropping systems and residue incorporation in Inceptisols of eastern Indo-Gangetic Plain. Soil Tillage Res. 178, 108–117. doi: 10.1016/j.still.2017.12.025
Lai, R., Arca, P., Lagomarsino, A., Cappai, C., Seddaiu, G., Demurtas, C. E., et al. (2017). Manure fertilization increases soil respiration and creates a negative carbon budget in a Mediterranean maize (Zea mays L.)-based cropping system. Catena 151, 202–212. doi: 10.1016/j.catena.2016.12.013
Lan, Z. M., Chen, C. R., Rashti, M. R., Yang, H., and Zhang, D. K. (2017). Stoichiometric ratio of dissolved organic carbon to nitrate regulates nitrous oxide emission from the biochar-amended soils. Sci. Total Environ. 576, 559–571. doi: 10.1016/j.scitotenv.2016.10.119
Lenka, S., Choudhary, R., Lenka, N. K., Saha, J. K., Amat, D., Patra, A. K., et al. (2022). Nutrient management drives the direction and magnitude of nitrous oxide flux in crop residue-returned soil under different soil moisture. Front. Environ. Sci. 10:857233. doi: 10.3389/fenvs.2022.857233
Lenka, S., Lenka, N. K., Singh, R. C., Subba Rao, A., Kundu, S., Raghuwansi, J., et al. (2014). Greenhouse gas emission and soil properties as influenced by wheat biomass burning in Vertisols of central India. Curr. Sci. 107, 1150–1154.
Lenka, S., Malviya, S. K., Lenka, N. K., Sahoo, S., Bhattacharjya, S., Jain, R. C., et al. (2021). Manure addition influences the effect of tillage on soil aggregation and aggregate associated carbon in a Vertisol of central India. J. Environ. Biol. 41, 1585–1593. doi: 10.22438/jeb/41/6/SI-221
Lenka, S., Trivedi, P., Singh, B., Singh, B. P., Pendall, E., Bass, A., et al. (2019). Effect of crop residue addition on soil organic carbon priming as influenced by temperature and soil properties. Geoderma 347, 70–79. doi: 10.1016/j.geoderma.2019.03.039
Li, C., Wang, G., Han, Q., Sun, J., Ning, H., and Feng, D. (2023). Soil moisture and water-nitrogen synergy dominate the change of soil carbon stock in farmland. Agric. Water Manag. 287:108424. doi: 10.1016/j.agwat.2023.108424
Li, P., Zhang, A., Huang, S., Han, J., Jin, X., Shen, X., et al. (2023). Optimizing Management Practices under Straw Regimes for Global Sustainable Agricultural Production. Agronomy 13:710. doi: 10.3390/agronomy13030710
Li, Z., Reichel, R., and Brüggemann, N. (2021). Effect of C:N:P stoichiometry on soil nitrous oxide emission and nitrogen retention. J. Plant Nutr. Soil Sci. 184, 520–529. doi: 10.1002/jpln.202000416
Liu, L., Estiarte, M., and Peñuelas, J. (2019). Soil moisture as the key factor of atmospheric CH4 uptake in forest soils under environmental change. Geoderma 355:113920. doi: 10.1016/j.geoderma.2019.113920
Liu, X., Peñuelas, J., Sardans, J., Fang, Y., Wiesmeier, M., Wu, L., et al. (2021). Response of soil nutrient concentrations and stoichiometry, and greenhouse gas carbon emissions linked to change in land-use of paddy fields in China. Catena 203:105326. doi: 10.1016/j.catena.2021.105326
Liyanage, A., Grace, P. R., Scheer, C., de Rosa, D., Ranwala, S., and Rowlings, D. W. (2020). Carbon limits non-linear response of nitrous oxide (N2O) to increasing N inputs in a highly-weathered tropical soil in Sri Lanka. Agric. Ecosyst. Environ. 292:106808. doi: 10.1016/j.agee.2019.106808
Lussich, F., Dhaliwal, J. K., Faiia, A. M., Jagadamma, S., Schaeffer, S. M., and Saha, D. (2024). Cover crop residue decomposition triggered soil oxygen depletion and promoted nitrous oxide emissions. Sci. Rep. 14, 1–14. doi: 10.1038/s41598-024-58942-7
Mathivanan, G. P., Eysholdt, M., Zinnbauer, M., Rösemann, C., and Fu,ß, R. (2021). New N2O emission factors for crop residues and fertiliser inputs to agricultural soils in Germany. Agric. Ecosyst. Environ. 322:107640. doi: 10.1016/j.agee.2021.107640
Mehnaz, K. R., Corneo, P. E., Keitel, C., and Dijkstra, F. A. (2019). Carbon and phosphorus addition effects on microbial carbon use efficiency, soil organic matter priming, gross nitrogen mineralization and nitrous oxide emission from soil. Soil Biol. Biochem. 134, 175–186. doi: 10.1016/j.soilbio.2019.04.003
Mirzaei, M., Gorji Anari, M., Taghizadeh-Toosi, A., Zaman, M., Saronjic, N., Mohammed, S., et al. (2022). Soil nitrous oxide emissions following crop residues management in corn-wheat rotation under conventional and no-tillage systems. Air, Soil Water Res. 15:8789. doi: 10.1177/11786221221128789
Page, A. L., Millar, R. H., and Keeney, D. R. (1982). Methods of Soil Analysis, Part 2. Madison WI: American Society of Agronomy and Soil Sciences Society of America.
Pareja-Sánchez, E., Cantero-Martínez, C., Álvaro-Fuentes, J., and Plaza-Bonilla, D. (2019). Tillage and nitrogen fertilization in irrigated maize: key practices to reduce soil CO2 and CH4 emissions. Soil Tillage Res. 191, 29–36. doi: 10.1016/j.still.2019.03.007
Shen, H., Zhang, Q., Zhu, S., Duan, P., Zhang, X., Wu, Z., et al. (2021). Organic substitutions aggravated microbial nitrogen limitation and decreased nitrogen-cycling gene abundances in a three-year greenhouse vegetable field. J. Environ. Manage. 288, 112379. doi: 10.1016/j.jenvman.2021.112379
Singh, D., Lenka, S., Lenka, N. K., Yadav, D. K., Yadav, S. S., Kanwar, R. S., et al. (2024). Residue management and nutrient stoichiometry control greenhouse gas and global warming potential responses in Alfisols. Sustain. 16, 3997. doi: 10.3390/su16103997
Stark, J. M., and Firestone, M. K. (1995). Mechanisms for soil moisture effects on activity of nitrifying bacteria. Appl. Environ. Microbiol. 61, 218–221. doi: 10.1128/aem.61.1.218-221.1995
Unger, I. M., Muzika, R. M., and Motavalli, P. P. (2010). The effect of flooding and residue incorporation on soil chemistry, germination and seedling growth. Environ. Exp. Bot. 69, 113–120. doi: 10.1016/j.envexpbot.2010.03.005
Wang, J., Sainju, U. M., and Barsotti, J. L. (2012). Residue placement and rate, crop species, and nitrogen fertilization effects on soil greenhouse gas emissions. J. Environ. Prot. (Irvine,. Calif). 03, 1238–1250. doi: 10.4236/jep.2012.329141
Wang, N., Yu, J. G., Zhao, Y. H., Chang, Z. Z., Shi, X. X., Ma, L. Q., et al. (2018). Straw enhanced CO2 and CH4 but decreased N2O emissions from flooded paddy soils: Changes in microbial community compositions. Atmos. Environ. 174, 171–179. doi: 10.1016/j.atmosenv.2017.11.054
Wu, L., Hu, R., Tang, S., Shaaban, M., Zhang, W., Shen, H., et al. (2020). Nitrous oxide emissions in response to straw incorporation is regulated by historical fertilization. Environ. Pollut. 266:115292. doi: 10.1016/j.envpol.2020.115292
Xia, L., Lam, S. K., Wang, S., Zhou, W., Chen, D., and Yan, X. (2020). Optimizing nitrogen fertilization rate to enhance soil carbon storage and decrease nitrogen pollution in paddy ecosystems with simultaneous straw incorporation. Agric. Ecosyst. Environ. 298:106968. doi: 10.1016/j.agee.2020.106968
Xu, C., Han, X., Ru, S., Cardenas, L., Rees, R. M., Wu, D., et al. (2019). Crop straw incorporation interacts with N fertilizer on N2O emissions in an intensively cropped farmland. Geoderma 341, 129–137. doi: 10.1016/j.geoderma.2019.01.014
Yang, L., Muhammad, I., Chi, Y. X., Liu, Y. X., Wang, G. Y., Wang, Y., et al. (2022). Straw return and nitrogen fertilization regulate soil greenhouse gas emissions and global warming potential in dual maize cropping system. Sci. Total Environ. 853:158370. doi: 10.1016/j.scitotenv.2022.158370
Zhang, L., Qin, R., Chai, N., Wei, H., Yang, Y., Wang, Y., et al. (2022). Optimum fertilizer application rate to ensure yield and decrease greenhouse gas emissions in rain-fed agriculture system of the Loess Plateau. Sci. Total Environ. 823:153762. doi: 10.1016/j.scitotenv.2022.153762
Keywords: residue return rate, stoichiometry, soil moisture, relative variable importance, nitrous oxide, methane
Citation: Singh D, Lenka S, Lenka NK, Yadav DK, Yadav SS, Kanwar RS, Sarkar A and Saha M (2024) Optimizing residue return with soil moisture and nutrient stoichiometry reduced greenhouse gas fluxes in Alfisols. Front. Sustain. Food Syst. 8:1490523. doi: 10.3389/fsufs.2024.1490523
Received: 18 September 2024; Accepted: 22 October 2024;
Published: 06 November 2024.
Edited by:
Yue Li, Kunming University of Science and Technology, ChinaCopyright © 2024 Singh, Lenka, Lenka, Yadav, Yadav, Kanwar, Sarkar and Saha. This is an open-access article distributed under the terms of the Creative Commons Attribution License (CC BY). The use, distribution or reproduction in other forums is permitted, provided the original author(s) and the copyright owner(s) are credited and that the original publication in this journal is cited, in accordance with accepted academic practice. No use, distribution or reproduction is permitted which does not comply with these terms.
*Correspondence: Sangeeta Lenka, c2FuZ2VldGEubGVua2FAaWNhci5nb3YuaW4=; Narendra Kumar Lenka, bmtsZW5rYTc0QGdtYWlsLmNvbQ==