- 1College of Life Sciences, Inner Mongolia Agriculture University, Hohhot, Inner Mongolia, China
- 2Inner Mongolia Agronomy and Animal Husbandry Technology Extension Center, Hohhot, China
- 3Xing'an League Agricultural and Animal Husbandry Science Research Institute, Ulanhot, China
Introduction: The rhizosphere microbiome plays a critical role in plant growth and productivity. However, the genotype-specific dynamics and functional influence of these microbial communities on soybean (Glycine max (L.) Merri.) yield traits remain underexplored. This study investigated the composition and functional implications of rhizosphere microbial communities in conventional and genetically modified (GM) soybean varieties under field conditions.
Methods: A comparative analysis was conducted on rhizosphere microbial communities associated with conventional H0269 and transgenic Z1510 and M579 soybean varieties at seedling and flowering stages. High-throughput sequencing of 16S rDNA and ITS regions was used to profile bacterial and fungal communities. Alpha and beta diversity metrics were assessed, and correlation analyses were performed to identify microbial taxa linked to yield traits.
Results: Distinct microbial community structures were observed across soybean genotypes and growth stages. Proteobacteria, Acidobacteriota, and Actinobacteria were dominant bacterial phyla, while Ascomycota predominated among fungi. Genotype-specific differences were evident, with M579 exhibiting the highest bacterial alpha diversity. Beta diversity analysis revealed significant shifts in microbial composition between growth stages, particularly for bacterial communities. Correlation analysis identified 15 bacterial and 13 fungal species significantly associated with yield traits such as plant density, grain weight, and theoretical yield.
Discussion: The results highlight genotype-dependent recruitment of rhizosphere microorganisms and their potential impact on soybean productivity. GM soybean varieties selectively enriched beneficial microbes that may enhance nutrient uptake, stress tolerance, and disease resistance. These findings provide valuable insights into plant-microbe interactions, paving the way for developing microbial inoculants and breeding strategies to optimize soybean yield in sustainable agriculture.
1 Introduction
The rhizosphere microorganisms played a crucial role in plant growth, development, and health (Weng et al., 2024; Sharma et al., 2024). These beneficial bacteria, fungi, and archaea formed complex communities around plant roots, creating a dynamic environment. It has been reported that certain Bacillus strains have the potential to partially replace chemical fertilizers in rice production (Tripathi et al., 2022). The rhizosphere microorganisms can not only provide phosphorus and nitrogen for promoting plant growth, but also help plant tolerate abiotic stresses such as drought and salinity (Abrahão et al., 2018; Bandara and Kang, 2024). The interactions between plant and rhizosphere microorganisms were bidirectional and led to sustainable agricultural practices, including the use of plant growth-promoting rhizobacteria as biofertilizers and biopesticides (Olanrewaju et al., 2019; Ferreyra-Suarez et al., 2024; Vejan et al., 2016). Recent advances in multi-omics approaches have further revealed how plants actively shape their rhizobiome through root exudate profiles, creating genotype-specific microbial recruitment patterns (Levy et al., 2017; Zhalnina et al., 2018).
Soybean (Glycine max (L.) Merri.), a globally significant food and cash crop, serves as a primary source of high-quality protein and oil for human consumption. While traditional breeding has focused on improving aboveground agronomic traits, there is growing interest in the rhizosphere microbiome’s potential to enhance crop productivity and sustainability (Kumar and Dubey, 2020). Soybean and legume microbiomes are shaped by plant genetics, environmental factors, and agricultural practices. Genetically related cowpea cultivars develop similar rhizobacterial communities (de Albuquerque et al., 2022), while soybean genotypes modulate microbial assembly via root exudates and nodulation (Liu et al., 2019; Zhong et al., 2019). Seasonal shifts and soil types alter peanut rhizosphere microbiota (Daraz et al., 2023), and organic farming enhances peanut microbiome diversity compared to conventional methods (Paudel et al., 2023). Soybean nodulation enriches nitrogen-cycling microbes, improving subsequent crop yields (Liu et al., 2024), while intercropping creates unique microbiome configurations (Lanzavecchia et al., 2024). Functional diversity in soybean rhizospheres supports stress resilience and nitrogen fixation (Ajiboye et al., 2022; Mayhood and Mirza, 2021).
With the increasing adoption of genetically modified (GM) soybeans in agricultural fields, field experiments have become crucial in assessing the interactions between GM soybeans and rhizosphere microbiomes. Global research reveals geographically distinct responses. North American studies in the U.S. Midwest show environmental factors outweigh GM traits in shaping bacterial diversity (Liu et al., 2019; Chang et al., 2021), while Argentine trials identify transient Pseudomonadales shifts post-glyphosate application mediated by soil organic content (Bento et al., 2019). Nigerian organic GM systems match conventional yields with rainfall-driven microbial shifts (Olowe et al., 2023), whereas Romanian field trials demonstrate microbiome stability patterns aligning with Central European observations (Ichim, 2019; Noack et al., 2024). Despite progress, critical gaps persist in understudied regions like Eastern European chernozems and Africa’s Sahel, where unique genotype-environment-microbiome relationships emerge (Sarkar et al., 2022). Crops shape rhizosphere microbiomes via root exudates, selecting bacteria that aid stress resilience and nutrient cycling. While environmental factors and management practices drive community composition, functional microbial cores underpin plant health under local stressors (Cucio et al., 2016; Debenport et al., 2015). International comparisons further highlight system-specific dynamics. Brazilian glyphosate-resistant soybeans exhibit early Bradyrhizobium reductions (Zablotowicz and Reddy, 2004), Canadian systems show seasonal variability overriding genotypic effects, and Australian non-GM cultivars maintain stable variety-specific microbial signatures across soils (Jang et al., 2023). These disparities underscore the need for multinational consortia to disentangle genetic, environmental, and management factors shaping rhizosphere communities.
These findings highlight the need for continued research to fully understand long-term effects of GM soybeans on soil microbial communities in agricultural ecosystems (Ren et al., 2023). Our study addresses this through a comparative analysis of conventional and GM varieties in Inner Mongolia’s agriculturally critical yet understudied chernozem (chestnut soil zone), integrating rhizosphere microbiome profiles with soybean phenomic data across growth stages. We reveal genotype-specific microbial recruitment dynamics while contributing foundational datasets from this climate-vulnerable region, elucidating how pedoclimatic conditions interact with soybean genetics to shape yield-linked microbial communities. This work bridges continental research divides, offering insights into how regional pedoclimatic conditions interact with plant genetics to structure rhizosphere communities that influence yield outcomes.
2 Materials and methods
2.1 Plant materials
Three distinct soybean varieties, Zhongliandou (Z1510), Hejiao (H0269), and Maiyu (M579) were used in this study. Z1510 and M579 are both genetically modified soybeans. The Z1510 transformed from ZhongHuang 6,106 with the gat and eps(g2) gene, conferring glyphosate tolerance. M579 derived from DBN9004, contains epsps and pat gene, providing glyphosate and glufosinate-ammonium tolerance. In contrast, H0269 is a conventional soybean variety that does not possess these modifications and relies on traditional weed control methods.
2.2 Experimental design and site description
Three distinct soybean varieties cultivated at the experimental base of the Xing’an League Agriculture and Animal Husbandry Science, Institute in Inner Mongolia, a region of significant importance for soybean production in China. The site (45°43′-46°18’N, 121°53′-122°52′E) has a temperate continental climate with chernozem and chestnut soils (pH 8.03, organic matter 34.76 g/kg, total N 3.437 g/kg, total K 2.22%, alkaline N 252.54 mg/kg, available P 20.78 mg/kg, available K 88.75 mg/kg). A randomized complete block design with three replications was employed. Planting utilized a double-row ridge system (60 cm ridge spacing, 0.6 cm plant spacing) at 280,000 plants/ha. Z1510 and M579 received 2,250 mL/ha of 41% glyphosate isopropylamine salt, while H0269 received conventional herbicides. Base fertilizer was applied at >450 kg/ha.
2.3 Rhizosphere soil sampling and microbial community analysis
Samples were collected at seedling and flowering stages using a five-point sampling method. Rhizosphere soil was obtained from roots at 10–30 cm depth, sieved (2 mm), and stored at −80°C. DNA was extracted using HiPure Universal DNA Kit. The V3 and V4 region of 16S rRNA and ITS1 region were amplified and sequenced on a Qsep-400 platform (Persoon et al., 2017). Sequences were processed using QIIME 2 (version 2021.4) and DADA2 to obtain amplicon sequence variants (ASVs; Kesim et al., 2023). Taxonomic annotation used the SILVA 138 database (Bars-Cortina et al., 2023). Alpha diversity was assessed in QIIME 2. Beta diversity was evaluated using principal coordinates analysis (PCoA) based on Bray-Curtis distances. Linear discriminant analysis effect size (LEfSe) identified differentially abundant taxa (LDA > 3.5). Functional classification used FUNGuild (version 1.0; Djemiel et al., 2017).
2.4 Yield trait measurements
At maturity on September 28, yield components were measured following national standards. Plants per m2 were counted in 2 m2 areas, while single plant grain weight (g) was calculated by dividing total grain weight by the number of plants in the same 2 m2 area. The 100-grain weight (g) was determined as an average of 3 replicates, and moisture content (%) was measured using a moisture meter with 3 replicates. Finally, the theoretical yield (kg/ha) was calculated using the formula: plants/m2 × 10,000 × single plant grain weight× 10−5× 0.9. This comprehensive approach provides a standardized method for estimating crop yields based on key components measured in sample areas, with multiple replicates used for some measurements to enhance accuracy and reliability.
2.5 Statistical analysis
The 16S rRNA gene and ITS amplicon sequencing data were processed using the SILVA 138/16S rRNA database and Unite 8.0 database for taxonomic assignment. Quality control measures were implemented to ensure data integrity, including the detection and removal of chimeric sequences using the UCHIME (v1.9.1) software. Alpha diversity indices were calculated using the MOTHUR (v1.30.2) software to assess the richness and diversity of microbial communities across samples. To investigate the overall community composition and assess the similarities or dissimilarities between samples, beta diversity analysis was performed using principal coordinate analysis (PCoA) implemented in the QIIME (v1.9.1) software package (Caporaso et al., 2010). The resulting PCoA plots were generated using the R packages “vegan,” “ecodist,” and “ggplot2” in R (v4.1.3), enabling the visual representation and interpretation of beta diversity patterns (Schloss et al., 2009; Cole et al., 2014; Chen and Boutros, 2011). Correlations between microbial community composition (top 20 dominant bacterial taxa) and yield traits were examined using Pearson’s correlation coefficient. Data were subjected to a one-way analysis of variance with factors of treatments and expressed as means +SD. Comparisons between any two groups were performed by unpaired Student’s t-tests.
3 Results
3.1 Overall analysis of 16S rDNA
The 16S rDNA and ITS sequencing analyses revealed significant microbial diversity in rhizosphere soil samples. Using the 341F-806R primer pair, 404,470 denoised and non-chimeric ASVs were obtained from rhizosphere soil samples, averaging 67,411 ASVs per replicate (Supplementary Table S1). The ITSIF-ITSIR primer pair yielded 807,404 ASVs from surrounding soil samples, with an average of 134,567 ASVs per replicate (Supplementary Table S2). The higher ASV count in rhizosphere soil samples suggested potentially greater microbial diversity.
3.2 Alpha diversity of microbial community in rhizosphere soils
The alpha diversity of rhizosphere microbial communities in M579, Z1510, and H0269 soybean varieties revealed distinct patterns for bacterial and fungal populations (Table 1). At both seeding and flowering stages, bacterial abundance consistently followed the order M579 > Z1510 > H0269, with M579 exhibiting significantly higher Chao1 and ACE indices compared to H0269. M579 maintained 5.8% higher Shannon diversity than H0269 across growth stages. Notably, bacterial diversity indices remained stable within each soybean variety, suggesting that genetic factors have a stronger impact on bacterial community composition than developmental changes. In contrast, fungal communities showed more conserved traits, with 123 shared ASVs across all samples and no significant diversity differences among varieties or growth stages. However, fungal ASV abundance showed diverged between seedling and flowering stages. M579 and Z1510 decreased significantly, while H0269 remained stable. Shannon indices showed no significant differences among varieties. These findings revealed the complex dynamics of soybean rhizosphere microbiomes, showcasing distinct bacterial and fungal responses to plant genotype and developmental stage.
3.3 Beta diversity of microbial community in rhizosphere soils
Beta diversity analysis using PCoA revealed significant variations in rhizosphere microbial community composition across soybean growth stages and genotypes. The PCoA1 axis accounted for 18.55 and 33.78% of the total variance in bacterial and fungal community composition, respectively. At seedling stage, Z1510 and H0269 showed similar bacterial and fungal communities, distinct from M579. At flowering stage, bacterial communities of Z1510 and M579 converged, while H0269 diverged (Figure 1A). However, Fungal communities at flowering stage showed less distinct clustering among varieties, but M579 displayed high within-group variability (Figure 1B). These findings underscored the dynamic nature of rhizosphere microbiomes, influenced by both plant genotype and developmental stage.
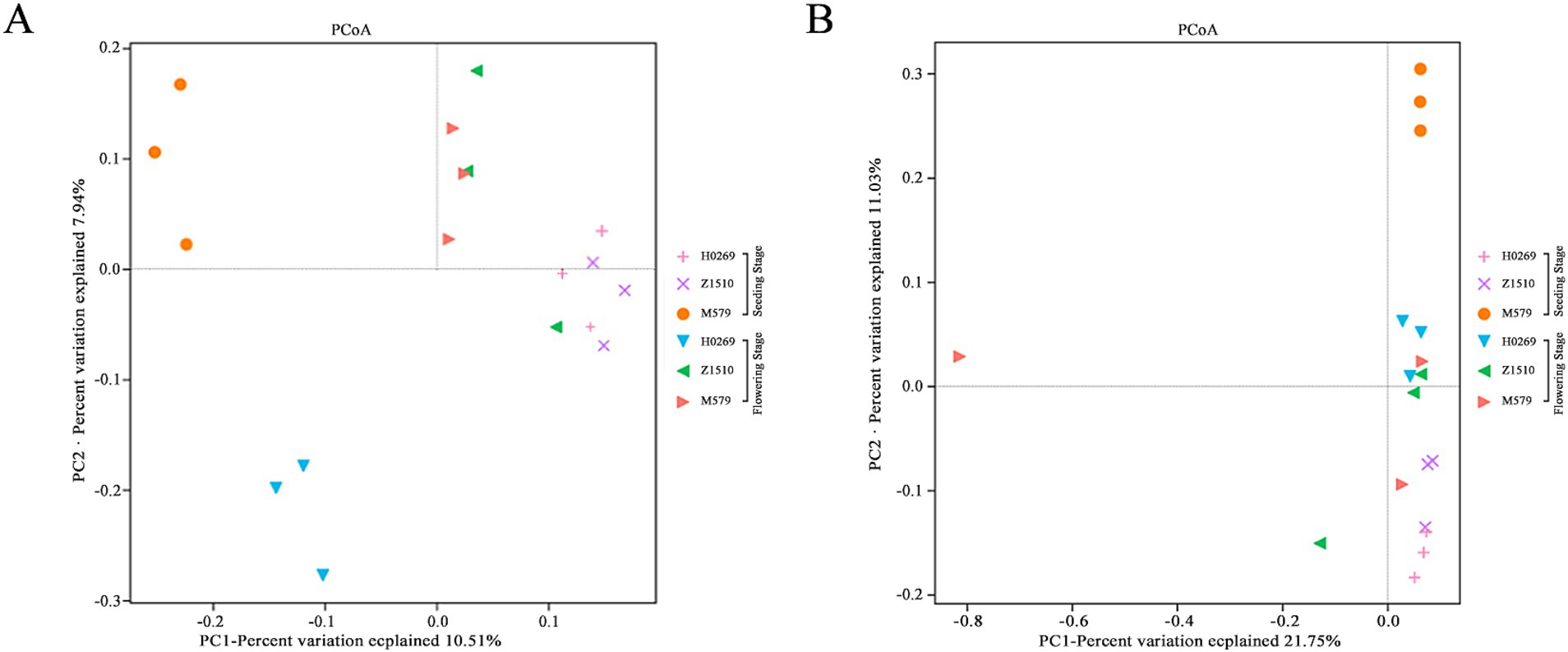
Figure 1. Principal component analysis based on the distance matrix calculated using the Bray–Curtis distance matrix for rhizosphere soil. The rhizosphere soil compartments cause the largest source of variance amongst bacterial (A) and fungal (B) communities of conventional soybean variety H0269 and genetically modified soybeans Z1510 and M579 at seeding stage and flowering stage.
3.4 Alterations in bacterial and fungal community composition
Analysis of soybean microbiomes across growth stages revealed significant variations in bacterial community structure. At the bacterial phylum level, the dominant groups identified were Proteobacteria, Acidobacteriota, and Actinobacteria. During the seedling stage, Proteobacteria exhibited the highest abundance in the H0269 variety, while M579 showed the lowest levels. Conversely, Acidobacteriota was most abundant in M579, with H0269 and Z1510 displaying similar abundances. Notably, the abundance of Actinobacteria remained relatively consistent across all three soybean varieties. The flowering period saw increased Proteobacteria and Acidobacteriota across all varieties, while Actinobacteria declined (Figure 2A). At the genus level, the dominant genera during both seedling and flowering periods were Sphingomonas, Gemmatimonas, RB41, Vicinamibacteraceae, and WD2101_soil_group (Figure 2B).
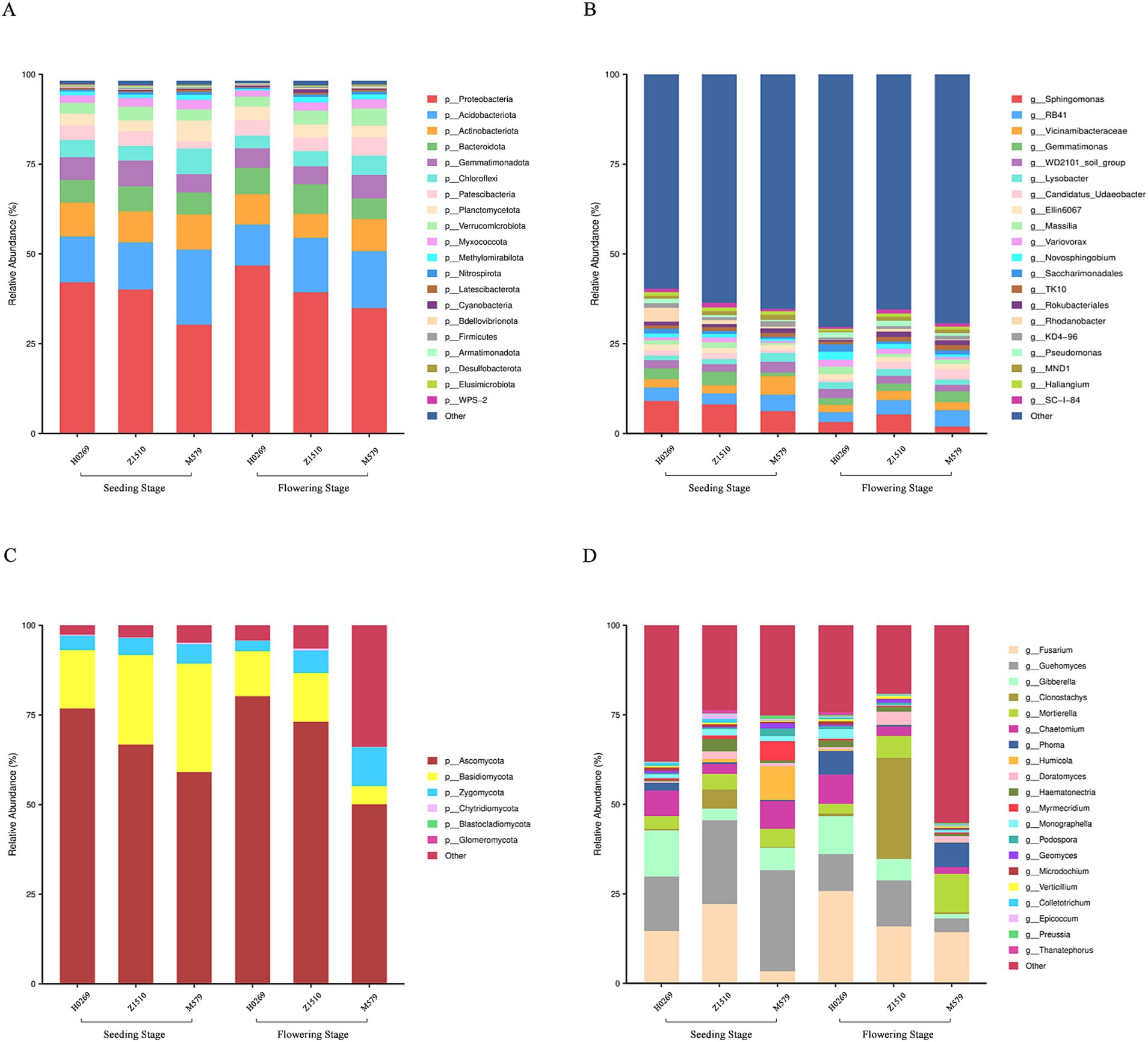
Figure 2. Composition and structure of bacterial and fungal communities of conventional soybean variety H0269 and genetically modified soybeans Z1510 and M579 at seeding stage and flowering stage. (A,B) Represent phylum and genus level of bacteria, respectively. (C,D) Represent phylum and genus level of fungi, respectively. The top 20 relative abundances are shown, and the remaining abundances are indicated as ‘Others’.
Fungal communities were dominated by Ascomycota, Basidiomycota, and Zygomycota phyla. At the seedling stage, Ascomycota was most abundant in H0269, followed by Z1510 and M579, while Basidiomycota peaked in M579. These abundance patterns persisted into the flowering stage, with Ascomycota increased in H0269 and Z1510 but decreased in M579 (Figure 2C). At the genus level, Fusarium and Guehomyces dominated, with Guehomyces showing higher abundance in M579 during the seedling stage. Conversely, Fusarium became more prevalent in H0269 during flowering (Figure 2D).
3.5 LEfSe analysis of bacterial and fungal communities
The LEfSe analysis of rhizosphere bacteria across three soybean varieties revealed significant differences in functional bacteria recruitment between seedling and flowering stages. At seedling stage, a total of 89 bacterial species were recruited, with M579 recruiting the highest number (48 species), followed by Z1510 (21 species), and H0269 (20 species). M579 was enriched with taxa such as P_Acidobacteria, c_Vicinamibacteria, and P_Chloroflexi. H0269 had o-Xanthomonaadales, f_Rhodanobaceae, and d_Rhodanobacte; and Z1510 had o-Gemmatimonadales and related taxa. During flowering stage, the total number of bacterial species decreased to 51, with H0269 recruiting 25 species, and both Z1510 and M579 recruiting 13 species (Figures 3A,B). H0269 showed enrichment in c_Alphasproteobacteria and c_Actinobacteria. Z1510 in o_Burkholderiales and f_Comamonadaceae, and M579 in f_Oxalobacteraceae and related taxa. From seedling to flowering stage, M579 experienced a substantial decrease of 33 bacterial species, Z1510 decreased by 8, while H0269 increased by 5.
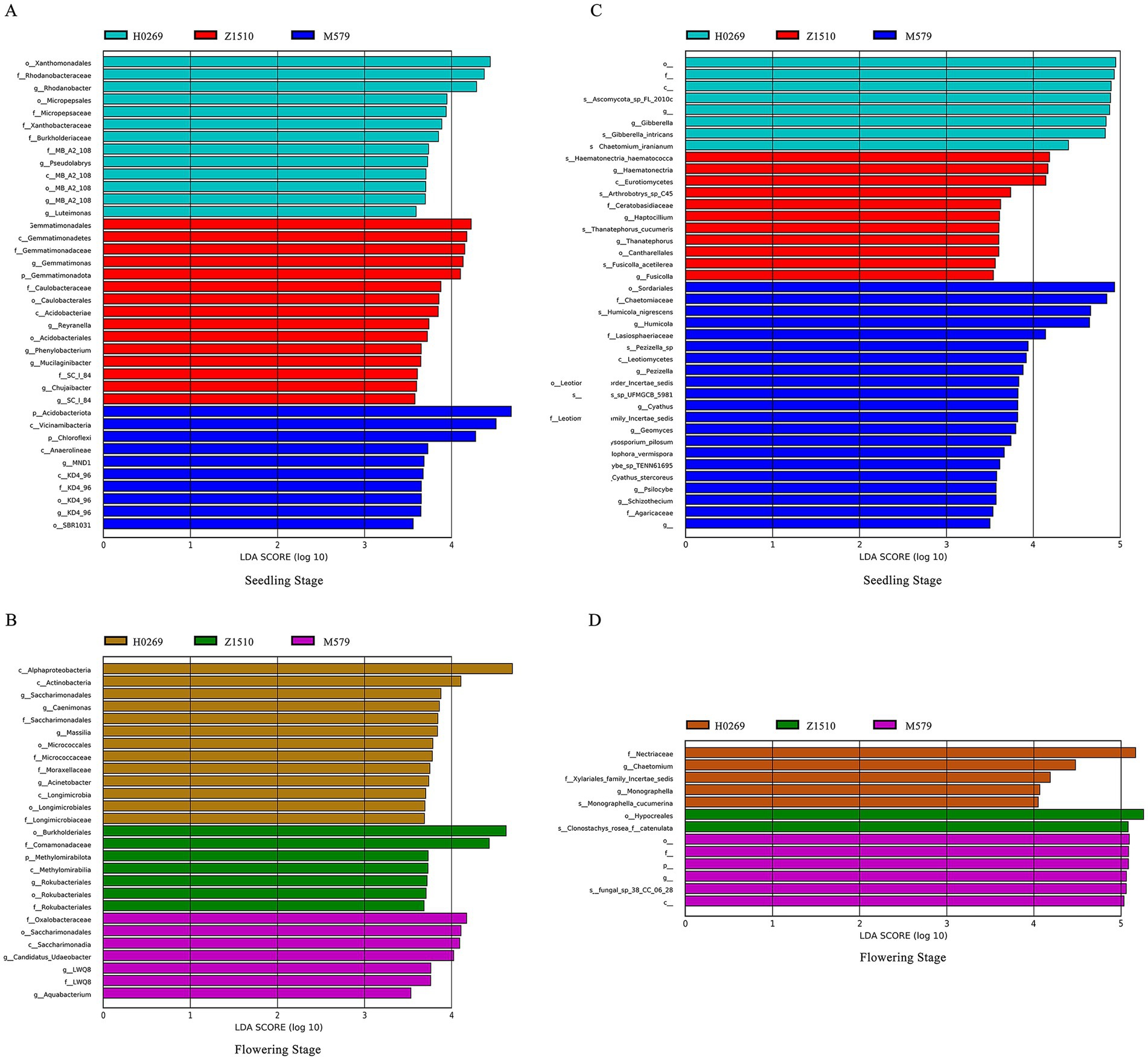
Figure 3. LEfSe analysis at multiple taxonomic levels comparing the rhizobiome soil of conventional soybean variety H0269 and genetically modified soybeans Z1510 and M579 at seeding stage and flowering stage. Histogram of the LDA scores greater than 3.5, computed for groups with differential abundance among the rhizobacterial communities at seeding stage (A) and flowering stage (B). Histogram of the LDA scores greater than 3.5, computed for groups with differential abundance among the rhizofungal communities at seeding stage (C) and tasseling stage (D). The length of each bar represents the contribution of species with significant differences in abundance.
Similarly, LEfSe analysis of rhizosphere fungi indicated significant differences in functional fungi recruitment between growth stages. In the seedling stage, a total of 67 fungal species were recruited: M579 recruited 36 species, Z1510 recruited 18 species, and H0269 recruited 13 species. During the flowering stage, this number dropped to 25 fungal species overall, with H0269 recruiting 12 species, M579 recruiting 8 species, and Z1510 recruiting 5 species. From seedling to flowering stages, M579 saw a decrease of 28 fungal species, Z1510 decreased by 13, and H0269 decreased by 6. Fungal taxa with LDA scores greater than 5 included f_Nutriaceae in H0269, o_Hypocrales and s_Clonostachys_rasea_f_catenulata in Z1510, and d_Ratobasidium and s_Ratobasidium-ramicola in M579 (Figures 3C,D).
3.6 Comparative analysis of soybean yield traits and rhizosphere microbial communities
Yield trait analysis across three soybean varieties revealed a consistent pattern, with Z1510 > M579 > H0269 for theoretical yield and grain number per plant. Z1510 showed superior yield indicators but lower 100-kernel weight and moisture content compared to M579 and H0269 (Table 2), suggesting a compensatory mechanism of increased grain number offsetting reduced grain weight.
The study examined relationships between rhizosphere microbes and five yield indicators: plant density, grains per plant, 100-grain weight, moisture content, and theoretical yield. The results revealed 15 bacterial and 13 fungal species exhibited significant associations with yield components. Among the bacteria, taxa such as s_wastewater metagenome and s_Taibaiella sp. demonstrated positive correlations with plant density but negative associations with grain weight. Conversely, species like S_Pedobacter panaciterrae showed positive correlations with theoretical yield, grain weight, and water content (Figure 4A). Fungal species, including s_Ascomycota sp. FL 2010c, negatively correlated with grain number and yield per plant while positively associating with water content. Conversely, S_Guehomyces pullulans exhibited positive correlations with yield components but negative associations with water content (Figure 4B).
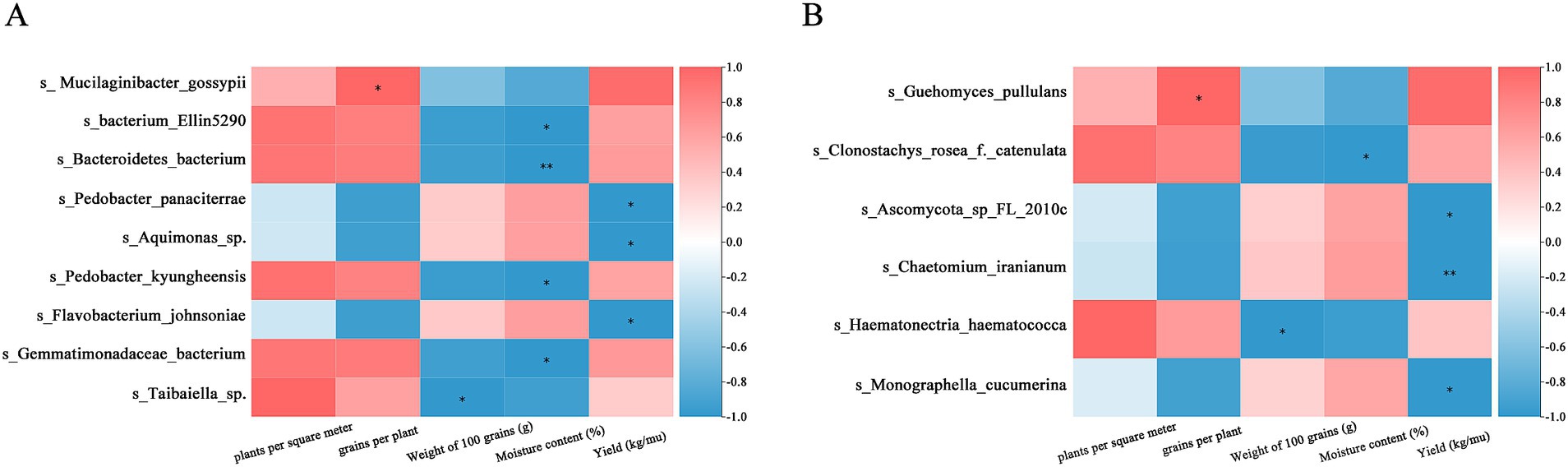
Figure 4. Correlation analysis between soybean rhizosphere microbial community and yield traits. (A) The rhizobacterial communities closely related to yield traits. (B) The rhizofungal communities closely related to yield traits.
4 Discussion
This study analyzed rhizosphere microbial dynamics in Z1510, M579, and H0269 soybean varieties across growth stages. Bacterial communities were dominated by Proteobacteria (40–60%) showing positive correlation with plant development, consistent with global patterns observed in soybean rhizospheres from North American chernozem soils (Wang et al., 2024). The 18–25% reduction in nitrogen-fixing Sphingomonas abundance in Z1510 and M579 compared to H0269 aligns with genotype-driven microbiome variations reported in Brazilian GM soybean cultivars, where Bradyrhizobium dominance persisted despite genetic modifications (Shen et al., 2025). These findings underscore that genotype effects on microbial recruitment are conserved across continents, though regional soil properties (pH, salinity) may modulate their magnitude, as demonstrated in comparative U.S.–China trials (Wang et al., 2023; Wei et al., 2021).
Fungal communities comprised 50–80% Ascomycota, with Guehomyces increasing 30–40% during reproductive stages. Z1510 and M579 showed 35% greater microbial fluctuations across growth phases than the H0269 soybean variety. This genotype-driven bacterial diversity patterns (M579 > Z1510 > H0269) contrast with Liu et al.’s identification of stronger genotype-specific recruitment in wild-type cultivars (Liu et al., 2019), but support Yang et al.’s conclusion that environmental factors outweigh GM traits in shaping soybean microbiomes (Yang et al., 2022). However, the impact of GM soybeans on rhizosphere microbiomes appears subtle and context-dependent, influenced by factors such as growth stage and environmental conditions (Wang et al., 2023; Yang et al., 2022). Another study on glyphosate-tolerant soybeans observed transient reductions in Bradyrhizobium abundance at the seedling stage but no long-term effects on microbial structure (Wei et al., 2021).
Fifteen bacterial and 13 fungal taxa correlated with yield traits, including Mucilaginibacter gossypii, Bacterium Ellin5290, Guehomyces pullulans, and Doratomyces sp. NG p07. Results suggest microbes influence yield through nitrogen cycling, water regulation, and plant density optimization. Soybean genetics impacted bacterial diversity twice as strongly as fungal diversity, aligning with Chang et al.’s demonstration of single-gene microbiome alterations, yet revealing broader genotype-level effects here (Chang et al., 2021). Simialy, high-yielding varieties showed rhizosphere microorganisms with higher activity and different carbon source utilization, suggesting superior recruitment and utilization of beneficial microbes, potentially enhancing nutrient absorption and yields (Popescu et al., 2022). Zheng et al. analyzed rhizosphere microbial community structures in three soybean genotypes (high oil, high protein, and high oil and high protein) further confirmed genotype-specific microbial communities and associated root characteristics (Zheng et al., 2019; Song et al., 2020).
While this field experiment provided valuable insights, further research was necessary to refine and expand upon these findings. These include addressing environmental variability, potential unaccounted microbial influences, and expanding the sample size to enhance statistical robustness. The single-site design (chernozem/ chestnut soils, pH 8.03) limited extrapolation to other agroecosystems, as environmental factors like soil type and regional climate influenced microbial recruitment. Future research should prioritize multi-location trials across soil types to assess microbiome stability, complemented by gnotobiotic systems validating causal relationships of key taxa (Mucilaginibacter gossypii). While identifying 15 bacterial and 13 fungal yield-associated taxa, taxonomic profiling through 16S/ITS sequencing captured microbial presence but not functional activity. The three-variety comparison with biological triplicates provides foundational insights, but expanded germplasm screening would better resolve subtle plant-microbiome interactions. Integration of metatranscriptomics could bridge taxonomic and functional analyses, while expanded sampling across growth stages would clarify temporal microbial dynamics.
In conclusion, this study illuminated the intricate interplay between soybean genotypes, developmental stages, and rhizosphere microbial communities. The findings advanced our understanding of plant-microbe interactions while revealing potential strategies for enhancing soybean yield and promoting sustainable agriculture. As this field progresses, it holds promise for significantly improving global food security and agricultural sustainability, paving the way for more efficient and eco-friendly soybean cultivation practices.
Data availability statement
The original contributions presented in the study are included in the article/Supplementary material, further inquiries can be directed to the corresponding authors.
Author contributions
XH: Conceptualization, Investigation, Writing – original draft, Formal analysis, Supervision, Validation. XZ: Investigation, Software, Validation, Visualization, Writing – original draft. MS: Investigation, Validation, Visualization, Writing – original draft. SY: Data curation, Formal Analysis, Investigation, Writing – original draft. SF: Investigation, Visualization, Writing – original draft. TT: Investigation, Writing – original draft, Resources. NC: Investigation, Writing – original draft, Resources. GZ: Conceptualization, Data curation, Project administration, Supervision, Writing – original draft. LH: Project administration, Supervision, Investigation, Writing – original draft. GW: Data curation, Funding acquisition, Resources, Supervision, Writing – review & editing, Investigation, Writing – original draft.
Funding
The author(s) declare that financial support was received for the research and/or publication of this article. This project is supported by the “Germplasm Innovation and Molecular Breeding of Forage Crops and Useful Microbiology” team, project number: TD202103.
Conflict of interest
The authors declare that the research was conducted in the absence of any commercial or financial relationships that could be construed as a potential conflict of interest.
Generative AI statement
The author(s) declare that no Gen AI was used in the creation of this manuscript.
Publisher’s note
All claims expressed in this article are solely those of the authors and do not necessarily represent those of their affiliated organizations, or those of the publisher, the editors and the reviewers. Any product that may be evaluated in this article, or claim that may be made by its manufacturer, is not guaranteed or endorsed by the publisher.
Supplementary material
The Supplementary material for this article can be found online at: https://www.frontiersin.org/articles/10.3389/fsufs.2025.1518597/full#supplementary-material
References
Abrahão, A., Ryan, M. H., Laliberté, E., Oliveira, R. S., and Lambers, H. (2018). Phosphorus- and nitrogen-acquisition strategies in two Bossiaea species (Fabaceae) along retrogressive soil chronosequences in South-Western Australia. Physiol. Plant. 163, 323–343. doi: 10.1111/ppl.12704
Ajiboye, T. T., Ayangbenro, A. S., and Babalola, O. O. (2022). Functional diversity of microbial communities in the soybean (Glycine max L.) rhizosphere from Free State, South Africa. Int. J. Mol. Sci. 23:9422. doi: 10.3390/ijms23169422
Bandara, A. Y., and Kang, S. (2024). Trichoderma application methods differentially affect the tomato growth, rhizomicrobiome, and rhizosphere soil suppressiveness against Fusarium oxysporum. Front. Microbiol. 15:1366690. doi: 10.3389/fmicb.2024.1366690
Bars-Cortina, D., Moratalla-Navarro, F., García-Serrano, A., Mach, N., Riobó-Mayo, L., Vea-Barbany, J., et al. (2023). Improving species level-taxonomic assignment from 16S rRNA sequencing technologies. Curr. Protoc. 3:e930. doi: 10.1002/cpz1.930
Bento, C. P. M., van der Hoeven, S., Yang, X., Riksen, M., Mol, H. G. J., Ritsema, C. J., et al. (2019). Dynamics of glyphosate and AMPA in the soil surface layer of glyphosate-resistant crop cultivations in the loess pampas of Argentina. Environ. Pollut. 244, 323–331. doi: 10.1016/j.envpol.2018.10.046
Caporaso, J. G., Kuczynski, J., Stombaugh, J., Bittinger, K., Bushman, F. D., Costello, E. K., et al. (2010). QIIME allows analysis of high-throughput community sequencing data. Nat. Methods 7, 335–336. doi: 10.1038/nmeth.f.303
Chang, H. X., Noel, Z. A., and Chilvers, M. I. (2021). A beta-lactamase gene of Fusarium oxysporum alters the rhizosphere microbiota of soybean. Plant J. 106, 1588–1604. doi: 10.1111/tpj.15257
Chen, H., and Boutros, P. C. (2011). VennDiagram: a package for the generation of highly-customizable Venn and Euler diagrams in R. BMC Bioinform. 12:35. doi: 10.1186/1471-2105-12-35
Cole, J. R., Wang, Q., Fish, J. A., Chai, B., McGarrell, D. M., Sun, Y., et al. (2014). Ribosomal database project: data and tools for high throughput rRNA analysis. Nucleic Acids Res. 42, D633–D642. doi: 10.1093/nar/gkt1244
Cucio, C., Engelen, A. H., Costa, R., and Muyzer, G. (2016). Rhizosphere microbiomes of European + seagrasses are selected by the plant, but are not species specific. Front. Microbiol. 7:440. doi: 10.3389/fmicb.2016.00440
Daraz, U., Erhunmwunse, A. S., Dubeux, J. C. B. Jr., Mackowiak, C., Guerra, V. A., Hsu, C. M., et al. (2023). Soil bacterial communities across seven Rhizoma Peanut cultivars (Arachis glabrata Benth.) respond to seasonal variation. Microb. Ecol. 86, 2703–2715. doi: 10.1007/s00248-023-02277-9
de Albuquerque, T. M., Mendes, L. W., Rocha, S. M. B., Antunes, J. E. L., Oliveira, L. M. S., Melo, V. M. M., et al. (2022). Genetically related genotypes of cowpea present similar bacterial community in the rhizosphere. Sci. Rep. 12:3472. doi: 10.1038/s41598-022-06860-x
Debenport, S. J., Assigbetse, K., Bayala, R., Chapuis-Lardy, L., Dick, R. P., and McSpadden Gardener, B. B. (2015). Association of shifting populations in the root zone microbiome of millet with enhanced crop productivity in the Sahel region (Africa). Appl. Environ. Microbiol. 81, 2841–2851. doi: 10.1128/AEM.04122-14
Djemiel, C., Grec, S., and Hawkins, S. (2017). Characterization of bacterial and fungal community dynamics by high-throughput sequencing (HTS) Metabarcoding during flax dew-retting. Front. Microbiol. 8:2052. doi: 10.3389/fmicb.2017.02052
Ferreyra-Suarez, D., Garcia-Depraect, O., and Castro-Munoz, R. (2024). A review on fungal-based biopesticides and biofertilizers production. Ecotoxicol. Environ. Saf. 283:116945. doi: 10.1016/j.ecoenv.2024.116945
Ichim, M. C. (2019). The Romanian experience and perspective on the commercial cultivation of genetically modified crops in Europe. Transgenic Res. 28, 1–7. doi: 10.1007/s11248-018-0095-9
Jang, S., Choi, S. K., Zhang, H., Zhang, S., Ryu, C. M., and Kloepper, J. W. (2023). History of a model plant growth-promoting rhizobacterium, Bacillus velezensis GB03: from isolation to commercialization. Front. Plant Sci. 14:1279896. doi: 10.3389/fpls.2023.1279896
Kesim, B., Ülger, S. T., Aslan, G., Cudal, H., Üstün, Y., and Küçük, M. (2023). Amplicon-based next-generation sequencing for comparative analysis of root canal microbiome of teeth with primary and persistent/secondary endodontic infections. Clin. Oral Investig. 27, 995–1004. doi: 10.1007/s00784-023-04882-x
Kumar, A., and Dubey, A. (2020). Rhizosphere microbiome: engineering bacterial competitiveness for enhancing crop production. J. Adv. Res. 24, 337–352. doi: 10.1016/j.jare.2020.04.014
Lanzavecchia, G., Frascarelli, G., Rocchetti, L., Bellucci, E., Bitocchi, E., Di Vittori, V., et al. (2024). Genotype combinations drive variability in the microbiome configuration of the rhizosphere of maize/bean intercropping system. Int. J. Mol. Sci. 25:1288. doi: 10.3390/ijms25021288
Levy, A., Salas Gonzalez, I., Mittelviefhaus, M., Clingenpeel, S., Herrera Paredes, S., Miao, J., et al. (2017). Genomic features of bacterial adaptation to plants. Nat. Genet. 50, 138–150. doi: 10.1038/s41588-017-0012-9
Liu, Y., Han, Q., Zhang, J., Zhang, X., Chen, Y., Li, M., et al. (2024). Soybean nodulation shapes the rhizosphere microbiome to increase rapeseed yield. J. Adv. Res. 34, 1–16. doi: 10.1016/j.jare.2024.11.034
Liu, F., Hewezi, T., Lebeis, S. L., Pantalone, V., Grewal, P. S., and Staton, M. E. (2019). Soil indigenous microbiome and plant genotypes cooperatively modify soybean rhizosphere microbiome assembly. BMC Microbiol. 19:201. doi: 10.1186/s12866-019-1572-x
Mayhood, P., and Mirza, B. S. (2021). Soybean root nodule and rhizosphere microbiome: distribution of Rhizobial and Nonrhizobial endophytes. Appl. Environ. Microbiol. 87, 1–14. doi: 10.1128/AEM.02884-20
Noack, F., Engist, D., Gantois, J., Gaur, V., Hyjazie, B. F., Larsen, A., et al. (2024). Environmental impacts of genetically modified crops. Science 385:9340. doi: 10.1126/science.ado9340
Olanrewaju, O. S., Ayangbenro, A. S., Glick, B. R., and Babalola, O. O. (2019). Plant health: feedback effect of root exudates-rhizobiome interactions. Appl. Microbiol. Biotechnol. 103, 1155–1166. doi: 10.1007/s00253-018-9556-6
Olowe, V. I. O., Odueme, P. U., Fadeyi, O. J., Tolulope, O., and Somefun,. (2023). Agronomic response of soybeans (Glycine max (L.) Merrill) to different management practices in the humid tropics. Technol. Agron. 18, 263–271. doi: 10.48130/TIA-2023-0018
Paudel, D., Wang, L., Poudel, R., Acharya, J. P., Victores, S., de Souza, C. H. L., et al. (2023). Elucidating the effects of organic vs. conventional cropping practice and rhizobia inoculation on rhizosphere microbial diversity and yield of peanut. Environ. Microb. 18:60. doi: 10.1186/s40793-023-00517-6
Persoon, I. F., Buijs, M. J., Özok, A. R., Crielaard, W., Krom, B. P., Zaura, E., et al. (2017). The mycobiome of root canal infections is correlated to the bacteriome. Clin. Oral Investig. 21, 1871–1881. doi: 10.1007/s00784-016-1980-3
Popescu, S. C., Tomaso-Peterson, M., Wilkerson, T., Bronzato-Badial, A., Wesser, U., and Popescu, G. V. (2022). Metagenomic analyses of the soybean root Mycobiome and microbiome reveal signatures of the healthy and diseased plants affected by taproot decline. Microorganisms 10:856. doi: 10.3390/microorganisms10050856
Ren, H., Zhang, F., Zhu, X., Lamlom, S. F., Zhao, K., Zhang, B., et al. (2023). Manipulating rhizosphere microorganisms to improve crop yield in saline-alkali soil: a study on soybean growth and development. Front. Microbiol. 14:1233351. doi: 10.3389/fmicb.2023.1233351
Sarkar, S., Kamke, A., Ward, K., Rudick, A. K., Baer, S. G., Ran, Q., et al. (2022). Bacterial but not fungal rhizosphere community composition differ among perennial grass ecotypes under abiotic environmental stress. Microbiol. Spectr. 10:e0239121. doi: 10.1128/spectrum.02391-21
Schloss, P. D., Westcott, S. L., Ryabin, T., Hall, J. R., Hartmann, M., Hollister, E. B., et al. (2009). Introducing mothur: open-source, platform-independent, community-supported software for describing and comparing microbial communities. Appl. Environ. Microbiol. 75, 7537–7541. doi: 10.1128/AEM.01541-09
Sharma, V., Sharma, D. P., and Salwan, R. (2024). Surviving the stress: understanding the molecular basis of plant adaptations and uncovering the role of mycorrhizal association in plant abiotic stresses. Microb. Pathog. 193:106772. doi: 10.1016/j.micpath.2024.106772
Shen, W., Liu, L., Fang, Z., Zhang, L., Ren, Z., Yu, Q., et al. (2025). Cultivation of genetically modified soybeans did not Alter the overall structure of rhizosphere soil microbial communities. Plants (Basel) 14:457. doi: 10.3390/plants14030457
Song, Y., Li, X., Yao, S., Yang, X., and Jiang, X. (2020). Correlations between soil metabolomics and bacterial community structures in the pepper rhizosphere under plastic greenhouse cultivation. Sci. Total Environ. 728:138439. doi: 10.1016/j.scitotenv.2020.138439
Tripathi, S., Yadav, S., Sharma, P., Purchase, D., Syed, A., and Chandra, R. (2022). Plant growth promoting strain Bacillus cereus (RCS-4 MZ520573.1) enhances phytoremediation potential of Cynodon dactylon L. in distillery sludge. Environ. Res. 208:112709. doi: 10.1016/j.envres.2022.112709
Vejan, P., Abdullah, R., Khadiran, T., Ismail, S., and Nasrulhaq, B. A. (2016). Role of plant growth promoting Rhizobacteria in agricultural sustainability-a review. Molecules 21:573. doi: 10.3390/molecules21050573
Wang, M., Ge, A. H., Ma, X., Wang, X., Xie, Q., Wang, L., et al. (2024). Dynamic root microbiome sustains soybean productivity under unbalanced fertilization. Nat. Commun. 15:1668. doi: 10.1038/s41467-024-45925-5
Wang, P., Nie, J., Yang, L., Zhao, J., Wang, X., Zhang, Y., et al. (2023). Plant growth stages covered the legacy effect of rotation systems on microbial community structure and function in wheat rhizosphere. Environ. Sci. Pollut. Res. Int. 30, 59632–59644. doi: 10.1007/s11356-023-26703-0
Wei, B., Zhang, J., Wen, R., Chen, T., Xia, N., Liu, Y., et al. (2021). Corrigendum: genetically modified sugarcane intercropping soybean impact on rhizosphere bacterial communities and co-occurrence patterns. Front. Microbiol. 12:835633. doi: 10.3389/fmicb.2021.742341
Weng, L. Y., Luan, D. D., Zhou, D. P., Guo, Q. G., Wang, G. Z., and Zhang, J. L. (2024). Improving crop health by synthetic microbial communities: Progress and prospects. Ying Yong Sheng Tai Xue Bao 35, 847–857. doi: 10.13287/j.1001-9332.202403.028
Yang, M., Luo, F., Song, Y., Ma, S., Ma, Y., Fazal, A., et al. (2022). The host niches of soybean rather than genetic modification or glyphosate application drive the assembly of root-associated microbial communities. Microb. Biotechnol. 15, 2942–2957. doi: 10.1111/1751-7915.14164
Zablotowicz, R. M., and Reddy, K. N. (2004). Impact of glyphosate on the Bradyrhizobium japonicum symbiosis with glyphosate-resistant transgenic soybean: a minireview. J. Environ. Qual. 33, 825–831. doi: 10.2134/jeq2004.0825
Zhalnina, K., Louie, K. B., Hao, Z., Mansoori, N., da Rocha, U. N., Shi, S., et al. (2018). Dynamic root exudate chemistry and microbial substrate preferences drive patterns in rhizosphere microbial community assembly. Nat. Microbiol. 3, 470–480. doi: 10.1038/s41564-018-0129-3
Zheng, T., Liang, C., Xie, H., Zhao, J., Yan, E., Zhou, X., et al. (2019). Rhizosphere effects on soil microbial community structure and enzyme activity in a successional subtropical forest. FEMS Microbiol. Ecol. 95:fiz043. doi: 10.1093/femsec/fiz043
Keywords: rhizosphere, soybean, microbiome, 16S rDNA and ITS sequencing, yield trait
Citation: Han X, Zhang X, Su M, Yang S, Feng S, Tian T, Chen N, Zhao G, Huang L and Wang G (2025) Genotype-specific rhizosphere microbiome assembly and its impact on soybean (Glycine max (L.) Merri.) yield traits. Front. Sustain. Food Syst. 9:1518597. doi: 10.3389/fsufs.2025.1518597
Edited by:
Keston Njira, Lilongwe University of Agriculture and Natural Resources, MalawiReviewed by:
Mihaela Niculae, University of Agricultural Sciences and Veterinary Medicine of Cluj-Napoca, RomaniaVishal Srivastava, Cleveland Clinic, United States
Izhar Ali, Lanzhou University, China
Copyright © 2025 Han, Zhang, Su, Yang, Feng, Tian, Chen, Zhao, Huang and Wang. This is an open-access article distributed under the terms of the Creative Commons Attribution License (CC BY). The use, distribution or reproduction in other forums is permitted, provided the original author(s) and the copyright owner(s) are credited and that the original publication in this journal is cited, in accordance with accepted academic practice. No use, distribution or reproduction is permitted which does not comply with these terms.
*Correspondence: Guofen Zhao, Z3VvZmVuemhhb0AxMjYuY29t; Lichun Huang, MTUyNDg1OTM2NjBAMTYzLmNvbQ==; Guihua Wang, d2FuZ2d1aXVhekBzb2h1LmNvbQ==