- 1Economic Research Center of the Upper Yangtze River, Chongqing Technology and Business University, Chongqing, China
- 2School of Politics and Public Administration, Southwest University of Political Science and Law, Chongqing, China
This paper explores the impact of the Internet on the green development of agriculture in China. In the context of China’s agricultural development, this paper underscores the mounting significance of the Internet and explores the potential impact of environmental regulations on the relationship between the two. The analysis used a panel data set encompassing 31 Chinese provinces from 2007 to 2020. The empirical study found that the Internet significantly impacts the efficiency of green development in Chinese agriculture. Further analysis reveals that in comparison with central regions, eastern regions are better positioned to benefit from the information dividends of internet development. In addition, internet development exerts a more substantial promotional effect on the efficiency of green agricultural development in regions specializing in staple food production and balanced agriculture, as opposed to those specializing in staple food sales. The study posits that both command-and-control and market-incentive environmental regulations can enhance the promotional effect of internet development on the efficiency of green agricultural development in China. Furthermore, the study demonstrated that the moderating effects of command-and-control and market-incentive environmental regulations exhibit regional heterogeneity. Specifically, command-and-control environmental regulations have a more substantial moderating effect in eastern regions, while market-incentive environmental regulations have a more substantial moderating effect in western regions. Moreover, the moderating effects of environmental regulations vary across agricultural functional zones. The moderating effect of command-and-control environmental regulations is more substantial in eastern regions, while market-incentive environmental regulations have a more significant moderating effect on grain balance. The findings of this study provide valuable insights into the relationship between the Internet and green agricultural development in China. They also offer important implications for policy-makers.
1 Introduction
As one of the critical paths for constructing ecological civilization in China, green development in agriculture is an inevitable way to achieve the strategic goals of peak carbon and carbon neutrality in agriculture (Jiang, 2022) and is also a trend in the development of word agriculture (Jiang et al., 2022). However, with the development of China’s agricultural economy, the contradictions between agricultural development and environmental protection have become increasingly prominent, with many agricultural areas showing ecological and environmental problems such as declining groundwater levels, reducing soil fertility, increasing agricultural surface pollution, weakening agricultural ecological services and increasingly severe quality and safety of agricultural products. Consequently, reconciling sustained high agricultural economic growth with agroecological improvements has become a significant challenge that must be overcome on the road to high-quality economic development in China. Nevertheless, facing complex agricultural resources and environmental problems, it is no longer possible to cope with them using traditional governance, management, or financial and material investment. In recent years, the continuous integration of the Internet with the agricultural system has brought new opportunities for agricultural transformation and upgrading, accelerating the modernization of agriculture and rural development and contributing to the green development of agriculture. Therefore, clarifying the Internet’s impact on agricultural green development is of great practical significance in promoting the conversion of high-quality agricultural development.
In recent years, scholars have paid attention to green development in agriculture, mainly in the following three aspects. First, the concept of green development in agriculture is defined. Scholars point out that green development in agriculture is a complex concept that integrates the concepts of organic agriculture, recycling agriculture, low-carbon agriculture, and ecological agriculture (Chen et al., 2021). It is also a development approach based on ecological agriculture, whose production methods adopt technology and greening (Pimentel et al., 2005). Moreover, it can enhance the quality of the environment, make efficient use of resources and maintain economic sustainability, and contribute to reducing poverty and ensuring food security (Talukder et al., 2020). In addition, it can improve the safety and quality of agricultural products, scientifically adjust the industrial structure, optimize the ecological environment and enhance the brand influence of agricultural products (Miguel et al., 2012). Second, the efficiency measurement of agricultural green development. Based on theories such as green agriculture theory and sustainable development theory, scholars have established an evaluation index system for the green total factor productivity in agriculture by using evaluation methods such as AHP, Super-SBM, and DEA-Malmquist, from the perspectives of economic, social, and resource input and ecological benefits (Chen et al., 2021; Veisi et al., 2016; Shen et al., 2020) or selecting input–output indicators (Zhou et al., 2019; Liu et al., 2020). They comprehensively assessed the development status of agricultural green development efficiency and conducted a comparative analysis of agricultural green development efficiency in different regions. Third, the factors affecting agricultural green development. Scholars have found that factors such as the digital economy (Jiang et al., 2022), environmental regulation, financial support for agriculture (Xu et al., 2022), urbanization level, agricultural mechanization level, science and technology input (Guo et al., 2020) and agricultural science and technology innovation (Zhang et al., 2022) have different degrees of influence on agricultural green development.
However, there is a lack of research on the impact of the Internet as a new variable in agricultural green development. Existing articles mainly focus on qualitative research on the relationship between the Internet and the development of agricultural green development. Scholars have found that the Internet has become an important means of leading agricultural development and can provide new ways and means for agricultural modernization. The specific mechanism of the Internet’s effect on agricultural production is mainly reflected in breaking information asymmetry through information dissemination so that farmers can quickly obtain key information such as market dynamics, price information, and planting techniques, thereby optimizing production decisions (Roy and Aslekar, 2022). The Internet also helps to broaden the distribution channels of traditional agricultural operations and break through the limitations of the traditional agricultural business service model. This can then promote the development of rural e-commerce, make the trend of online marketing of agricultural products more prominent (Xiang and Wang, 2020), further promote the deepening of the division of labor in agricultural production, and increase farmers’ income (Duguma and Bai, 2025). At the same time, the Internet provides a new platform for the promotion and application of agricultural technology. Technologies such as the Internet of Things, big data, and artificial intelligence enable intelligent and precise management of agricultural production, accelerating the process of agricultural modernization (Sadiku et al., 2021). The Internet also optimizes the allocation of production factors such as land, labor, and capital by integrating upstream and downstream information in the industry chain, promoting the development of rural e-commerce and driving industrial upgrading (Huang et al., 2024). In addition, Internet technology helps promote green and sustainable development. Precision agriculture technology enables precise management of soil and water resources through the Internet of Things and big data (Duguma and Bai, 2025), reducing the use of fertilizers and pesticides and improving the quality and safety of agricultural products (Cihan, 2023). The Internet strengthens the social network relationships between farmers, promotes collaboration and cooperation, and forms a close-knit production community (Alshehri, 2023). Big data analysis provides scientific decision-making support for agricultural production, enabling precision irrigation, fertilization, and market forecasting, helping farmers cope with market fluctuations, and promoting the transformation of traditional agriculture into an information-based, large-scale, intelligent agriculture (Sinwar et al., 2020).
In summary, there is a wealth of research on green development in agriculture, but the relationship between the Internet and green development in agriculture is comparatively scarce. Therefore, the proposition that the Internet affects green development in agriculture must be studied in depth. Based on this, this paper will empirically test the relationship between the Internet and agricultural green development. The marginal contributions of this paper lie in the following. Firstly, this paper enriches the research on the factors influencing green development in agriculture. The existing literature on factors influencing agricultural green development concentrates on the impact of factors such as the digital economy, environmental regulation, and financial support on agriculture, but with little attention paid to the impact of the Internet. Based on this, this paper takes the Internet, a new generation of network information technology, as an entry point to empirically study the impact of the Internet on agricultural green development. Secondly, this paper examines the moderating effect of environmental regulation on the Internet’s influence on agricultural green development so that relevant government departments can take strong measures to fully play the Internet’s role in promoting agricultural green development.
2 Theoretical analysis and research hypothesis
2.1 Mechanisms of the impact of the Internet on green development in agriculture
In accordance with the principles of information search theory and the theory of new economic geography, traditional agricultural producers are constrained by high information search costs. However, the Internet has significantly reduced the marginal cost of information access, thereby enabling agricultural entities to overcome geographical boundaries and accurately access multiple pieces of information, such as the prices of production factors and fluctuations in market demand. This, in turn, has resulted in an increase in farmers’ income and the promotion of agricultural development. So, there is a significant positive correlation between the internet and the development of green agriculture. Specifically, green development in agriculture means less resource input, less environmental pollution, and more economic output. So, green development in agriculture encompasses both the agroecological environment and the agricultural economy. On this basis, this paper will elaborate on the impact of the Internet on agricultural green development from the ecological environment level and the economic level. At the ecological environmental level, the application of the Internet in agricultural production will accelerate agricultural production technology, optimizing the agricultural ecological environment. On the one hand, with the help of internet technology, it can vigorously promote soil formula fertilization, precise and scientific application of pesticides, agricultural water-saving irrigation, precise regulation of temperature, humidity and fertilizers, improve the resource efficiency of agricultural waste, and realize the long-term monitoring and analysis of the dynamic impact of the whole process of crop growth and development on the quality conditions of soil, water resources and other ecological environments. Those will are not only conducive to the rational use of agricultural resources, the reduction of carbon emissions and the improvement of the ecological environment (Dai et al., 2023), but also can promote the conservation and sustainable use of agricultural resources, grasp the green operation of agricultural production in a timely and accurate manner, and ensure the green health of agricultural products. On the other hand, the Internet has broken the barrier of geographical distance, which is beneficial to strengthening the exchange and cooperation between the supply and demand sides of agricultural technology. In the past, research institutes, universities, and other agricultural technology research and development units were generally situated in cities. So, farmers had few opportunities to communicate with them due to geographical and spatial distances. The Internet development has built a “bridge” between farmers and agricultural technology research units, enabling farmers to interact with agricultural technology research units without leaving their homes. It is favorable to promote the technological progress of agricultural production and the use of green production technology in the agricultural field.
At the economic level, the Internet catalyzes economic growth in agriculture, mainly in agricultural cultivation, agricultural production methods, and the marketing of agricultural products. In terms of agricultural cultivation, the Internet provides a new way for farmers to learn scientific cultivation techniques, which is conducive to cultivating professional farmers and improving their cultivation techniques, thereby improving the quality of agricultural products. From ancient times to the present, most farmers in China have relied on the experience left behind by their ancestors or accumulated by themselves to carry out agricultural production, lacking scientific planting techniques to guide them. The Internet can provide farmers with a convenient way to learn about the quality of the land, scientific farming and harvesting methods, appropriate amount of fertilizer application, and more. Then, they will improve the quality of agricultural products in learning. Thus, achieving the target of increasing income. In terms of agricultural production methods, the use of the Internet in agricultural production can be expressed as precise agricultural production methods to nurture agricultural products with quality. Specifically, using the Internet in agricultural production and operation can improve traditional agricultural farming methods, which are rough and scattered. Then, the efficient management of agricultural production and operation enterprises with large-scale, specialized, and refined will realized as quickly as possible. In turn, the quality of agricultural products is improved. In terms of agricultural product sales, the Internet facilitates effective linkage of the supply and demand markets for agricultural products, reduces transaction costs with agricultural business model innovation, and thus wins the first opportunity for agricultural development to compete in the market (Liu et al., 2021). In the traditional agricultural marketing model, due to the asymmetry of information, the market feedback is relatively late, and the initiative is not in the hands of the buyer. Therefore, it becomes difficult to protect the interests of most farmers and agricultural companies. The use of the Internet in the agricultural production materials and the sale of agricultural products can break the barrier between geography and information through the development of agricultural products e-commerce and agricultural materials e-commerce, which can effectively omit intermediate links, connect the supply of agricultural products with the outside market, promote the docking of production and marketing, reduce transaction costs and increase the income of farmers.
In this regard, this paper will propose hypothesis H1.
H1: The Internet and agricultural green development have a significant positive relationship.
2.2 The regulatory mechanism of environmental regulation
The theory of sustainable development and the “B model” theory proposed by American scholar Lester R. Brown provides a theoretical framework for the green development of agriculture. In this framework, technological innovation plays a key role in reducing resource consumption and pollution, offering hope for a more sustainable future. The Internet, with its capacity to precisely regulate environmental resources (such as soil, water, and fertilizer), can provide accurate environmental information and resource management solutions. These solutions help farmers use resources efficiently, reduce waste and pollution, and thus promote the transformation of agriculture toward high-quality green development. Therefore, in areas with a high level of environmental regulation, the positive impact of the Internet on the green development of agriculture is more significant. From a static perspective, according to the cost hypothesis, environmental regulation policy pressure will increase the environmental management costs of agricultural operators and crowd out agricultural input funds, which is detrimental to agricultural production. In contrast, the use of the Internet in agricultural production can not only significantly improve the efficiency of agricultural production but also greatly reduce the generation of agricultural pollution. Therefore, the pressure of environmental regulation policies will prompt agricultural production operators to deepen the use of the Internet in agricultural production, promote the integration of agriculture and obtain the maximum economic benefits under the minimum pollution standards of environmental regulation. From a dynamic perspective, based on the innovation compensation theory in Porter’s hypothesis, environmental regulation pressures agricultural producers to manage the environment, which will force agricultural producers to respond with technological innovation to improve resource utilization. In this process, through the technology exchange platform built by the Internet, agricultural producers can learn about the frontier technology of agricultural production faster, which means that the use of the Internet by agricultural operators will promote the innovation of agricultural production technology faster. Therefore, agricultural producers will be more inclined to use the Internet to promote agricultural green development under environmental regulation.
In this regard, this paper will propose hypothesis H2.
H2: In regions with high levels of environmental regulation, the positive impact of the Internet on agricultural green development is more robust.
3 Research design
3.1 Model setting
Firstly, this paper will construct a benchmark Equation 1 to explore the impact of the Internet on green development in agriculture.
Secondly, to further explore the moderating effects of environmental regulation between the Internet and agricultural green development. This paper will add the command-and-control environmental regulation variable, the interaction term between the Internet and the command-and-control environmental regulation to obtain Equation 2, based on Equation 1. Meanwhile, adding the market incentive-based environmental regulation variable, the interaction term between the Internet and the market incentive-based environmental regulation to obtain Equation 3, based on Equation 1.
In Equations 1–3, denotes the 31 provinces in China, t denotes the time from 2007 to 2020, is the intercept term, is the random error term, denotes green total factor productivity in agriculture, denotes the level of Internet development, denotes environmental regulation, denotes the control variables, , respectively denote the corresponding coefficients to be estimated.
3.2 Variable selection
3.2.1 Explained variables
Green development in agriculture (aee). According to neoclassical economic growth theory, factor accumulation and technological progress are the source of economic growth, and technological progress is essentially an increase in total factor productivity (Solow, 1956). So, increasing green total factor productivity is the key to promote the development of green economics. Improving green total factor productivity in agriculture is essential for green development, and its growth better reflects the development trend. Therefore, this paper considers agricultural green total factor productivity as a proxy variable for agricultural green development. In addition, this paper will select indicators to measure agricultural green total factor productivity in terms of both agricultural resource factor inputs and agricultural outputs. Then, referring to existing studies (Guo et al., 2022), using the Super-SBM model to measure green total factor productivity in agriculture.
Agricultural input variables mainly include the following eight categories: (1) Labor input, expressed in terms of the number of people employed in the plantation industry. The current statistics only count the number of people employed in the primary industry. Therefore, this paper will separate the number of people employed in agriculture, forestry, animal husbandry and fishery by the proportion of total agricultural output value to total agricultural, forestry, animal husbandry and fishery output value, and thus obtain data on the number of people employed in the plantation industry. (2) Agricultural machinery input, expressed in terms of the total power of agricultural machinery. (3) Land inputs, expressed in terms of the crop sown area. (4) Water input, expressed in terms of the effective irrigation area. (5) Pesticide input, expressed in terms of the number of pesticides applied. (6) Fertilizer input, expressed in terms of the amount of fertilizer applied to agriculture. (7) Agricultural film input, expressed in terms of the amount of agricultural film used. (8) Energy input, expressed in terms of the amount of agricultural diesel used. Meanwhile, the agricultural output consists of two aspects: (1) desired output, expressed in terms of the actual agricultural output excluding inflation in 2007 as the base period, considering the effect of price factors. (2) Non-desired output, selected from agricultural surface pollution and agricultural carbon emissions, with the accounting for the amount of agricultural surface pollution referring to the method of Xu et al. (2022) and the accounting for agricultural carbon emissions referring to the method of Dubey and Lal (2009) (Table 1).
3.2.2 Core explanatory variables
Internet (internet). The current method of measuring the level of Internet development mainly contains two kinds: one is a single indicator, and the other is a comprehensive indicator. At present, many scholars use a single indicator, such as the number of CN domain names (Kiiski and Pohjola, 2002) and Internet penetration rate (Koutroumpis, 2009), but a single indicator can only reflect the level of Internet development in a particular area. In order to reveal the level of Internet development more comprehensively, this paper will choose comprehensive indicators to measure the level of Internet development, mainly choosing the variables of the number of people accessing the Internet, the number of mobile phone subscribers, the number of Internet broadband access ports, the total volume of express delivery, the total volume of postal and telegraphic services, and GDP will be selected, and the level of Internet development will be measured by using the principal component analysis method. Among them, the variables are standardized using the total population of each province in order to circumvent the influence of different provinces’ geography and market size.
Before conducting principal component analysis, Barlett’s spherical test and KMO test were conducted on the above indicators. The results show that the approximate chi-square value of the spherical Bartlett’s test is 2761.009, with a p-value of less than 0.01, which means that the Barlett’s test of sphericity is passed; KMO = 0.853, which is greater than the threshold value of 0.7, which means that the KMO test is passed. It indicates that the above indicators are suitable for principal component analysis. The variance interpretation of the principal component analysis is shown in Table 2.
As shown in Table 2, only the first principal component has all the eigenroots greater than 1, and the contribution rate reaches 76.41%, which is more than 70%, so the first principal component is chosen. The weight of each indicator variable is obtained in Table 3 through the calculation. On this basis, the level of Internet development (Internet) in this paper is calculated.
At the same time, although Principal Component Analysis (PCA) effectively reduces the dimensionality of a dataset while retaining as much information as possible from the original data, this method may be criticized in practice for the subjective selection of variables and interpretation of loadings. Specifically, PCA may be subjective in determining which variables should be included in the analysis and how to interpret the loadings of each principal component, which may lead to potential measurement errors and thus affect the robustness of the model. In order to address these issues that may arise when using PCA to measure the level of Internet development and to avoid the resulting model non-robustness, this paper will also introduce the entropy method as a supplementary means to measure the level of Internet development. Using the entropy value method for measurement can not only overcome the problem of subjectivity in PCA but also test the robustness of the results based on PCA, thus ensuring the reliability and scientificity of the research conclusions.
3.2.3 Adjustment variables
3.2.3.1 Environmental regulation
The two main types of environmental regulation in China are command-and-control and market-incentive regulation, while public participation in informal environmental regulation is still at a preliminary stage. Therefore, this paper focuses on the two types of environmental regulation that play a significant role: command-and-control environmental regulation (er) and market-incentive environmental regulation (ep). Drawing on the study of Yang et al. (2017), command-and-control environmental regulation is equal to the multiplication of the number of the provincial environmental regulation policies and the relative level of agricultural pollution emissions. In contrast, market-incentive environmental regulation is equal to the multiplication of the incentive-based environmental regulation factors and the relative level of agricultural CO2 emissions based on the carbon emissions trading market. In this case, the incentive-based environmental regulation factor is characterized by whether the local carbon trading market is active, with a value of 1 if it is active and 0 if it is not. Moreover, the process of collecting the number of provincial environmental regulatory policies is as follows: the first step is to choose the data source: the Beida Fabo network was chosen as the data source. Peking University Legal Treasure Network is an authoritative and comprehensive legal database covering laws and regulations, judicial cases, and other resources of 31 provinces and cities in China. Step 2: Determine keywords and screen relevant documents: Based on the needs of the research topic, we identified keywords such as “environmental protection,” “pollution prevention,” “pollution,” “soil,” and so on. “Soil” and other keywords related to relevant environmental protection policies and regulations, as well as selecting regulations and policy texts with high relevance to the topic as research objects. The third step is to count the frequency of relevant words. From the screened relevant policies and regulations, we further count the frequency of words related to environmental regulation in 31 provinces and cities.
3.2.4 Control variables
Drawing on previous research (Xu et al., 2022; Guo et al., 2020; Zhang et al., 2022), we recognize that many other factors significantly impact agricultural green development. Based on this, the following control variables will be selected in this paper to ensure the comprehensiveness and accuracy of the analysis:
(1) Land Use Efficiency (landu), expressed by using the ratio of effective irrigated area to crop sown area. (2) Industrial Structure, select the ratio of plantation industry (ppif) and the ratio of primary industry (ppip) to measure. The proportion of plantation industry (ppif) is the proportion of the output value of plantation industry to the total output value of agriculture, forestry, animal husbandry, and fishery. The proportion of primary industry (ppip) is the ratio of the primary industry’s output value to the region’s total output value. (3) Household Business Income Ratio (hoi), this paper uses the proportion of household business income to total household income to express. (4) Agricultural Disaster rate (des), this paper uses the ratio of disaster area to crop sown area to measure. (5) Urbanization Level (city), the ratio of non-farm population to total urban population is chosen as the measure. (6) Human Capital (edu), the number of years of education per capita in rural areas, is selected as a proxy for human capital in rural areas. (7) Government Support (fd), the logarithm of fiscal expenditure on agriculture, is used. (8) Degree of Foreign Trade (open), the logarithm of the value of agricultural exports is used. (9) Infrastructure (trans), the logarithm of rural electricity consumption, is used to quantify rural infrastructure. (10) Climate Change. This paper will consider the impact of temperature, precipitation, air humidity, and sunshine on agriculture and select the annual average temperature (at), the logarithm of the annual rainfall (pret), the annual average relative humidity (arh), and the logarithm of the annual sunshine hours (sh) as the four indicators of climate change.
3.3 Data
This paper uses panel data for 31 provinces in China from 2007 to 2020. Internet-related data were obtained from the China Internet Network Information Center (CNNIC). The order-controlled environmental regulation data were selected from Beida Fabao.com by searching 31 provinces for environmental protection policy and regulatory documents related to “environment,” “pollution,” “prevention and control,” from which the number of policy texts highly relevant to the research topic was extracted. The data on market-incentivized environmental regulations were obtained from China’s carbon trading market. In contrast, the data on other variables were obtained from the China Population and Employment Statistical Yearbook (2008–2021), the eps database, the China Statistical Yearbook (2008–2021), and regional statistical yearbooks of provinces. The individual missing data were interpolated. The final, this paper obtains the balanced panel data. Table 4 shows the descriptive statistics. The results of total factor productivity in agriculture show that the mean value is 0.905, the maximum value is 1.524, and the minimum value is 0.297, with a gap of 1.227, indicating that there is a significant gap in the green total factor productivity in agriculture among provinces and municipalities in China from 2007 to 2020. The results of the Internet development level show that the mean value is 0.587, the maximum value is 1.010, and the minimum value is 0.297, with a gap of 0.692, meaning that the level of internet development in China’s 31 provinces also differed significantly during the study period.
4 Empirical tests and analysis of results
4.1 Results of the base regression model
Before regression estimation, this paper performs the Hausman test on the relationship between the Internet and agricultural green development. The results show that the p-values of the Hausman test were all less than 0.01, indicating that the original hypothesis of a random effect was rejected at the 1% level, so this paper will use the fixed-effects model to estimate models 1 and 2 in Table 5.
In Table 5, model 1 investigates the Internet’s impact on Chinese agricultural green development before adding control variables. The result show that the Internet has a significant positive impact on the green development of Chinese agriculture. In Table 5, model 2 examines the Internet’s impact on Chinese agricultural green development after adding control variables. The results show that after taking into account the effect of control variables, the Internet still contributed to the green development in Chinese agriculture. So, it can find that the internet did not change in the two models, and only its coefficient changed slightly, which indicates that the internet significantly promoted agricultural green development during the study period. Then, hypothesis H1 holds.
4.2 Heterogeneity test
4.2.1 Regional heterogeneity
In Table 6, models 1–3 show the regression results for the eastern, central, and western regions, respectively. The regression results show that the results show that Internet development has a positive impact on the efficiency of agricultural green development in all regions, but the central region fails to pass the test of significance (β = 1.742, p < 0.01; β = 0.077, p > 0.1; β = 4.741, p < 0.01), which suggests that eastern and western regions can obtain more information dividends from the development of the Internet. The reasons for this phenomenon may lie in the fact that the eastern region is economically developed and possesses rich professionals with knowledge of modern agricultural technology and the Internet, who are better able to apply Internet technology to agricultural production practices; the western region benefits from specific policies, such as the “One Belt and One Road” initiative and the “Western Development” initiative, which are favorable to the western region and the eastern region. The Western region benefits from specific policies such as the “Belt and Road” initiative and the “Great Western Development.” In addition, because of the low starting point of agricultural development in the western region, the application of new technologies often brings large marginal benefits. Therefore, in the western region, the promotion effect of the Internet on the green development of agriculture is particularly significant, which helps to rapidly improve the local level of agricultural modernization, while the central region. However, most provinces are largely agricultural and have relatively traditional agricultural production methods. There is greater resistance to the transition to a modern agricultural model that relies on Internet technology, which restricts the wide application of Internet technology in local agriculture and its effective enhancement of the efficiency of the green development of agriculture. Effective enhancement of agricultural green development efficiency.
4.2.2 Heterogeneity of agricultural functional areas
In Table 6, models 4–6, respectively, assess the impact of the Internet on agricultural green development in the major grain-producing, grain-marketing, and grain-balancing regions. The results show that the Internet positively contributes to the green development of agriculture in all regions; however, the major grain-marketing regions fails the significance test. The possible explanations for this phenomenon are as follows. On the one hand, the major grain-producing and grain-balancing regions bear the grain supply of most provinces in China. In order to ensure food security, the trend of using the Internet in agricultural production and developing smart agriculture is unstoppable, which will lead to the penetration of the Internet in all aspects and processes of agricultural production, thus promoting the green development of agriculture. On the other hand, the major grain-marketing regions are located in seven provinces, including Beijing, Tianjin, Shanghai, where the level of Internet development and the green total factor productivity in agriculture are among the highest in China. However, according to the law of diminishing marginal utility, when the Internet reaches a certain level of development, its positive impact will diminish. Thus, the internet has an insignificant impact on agricultural green development in the major grain-marketing regions.
4.3 Endogeneity test
Since changes in the efficiency of green development in agriculture may also impact Internet development, the endogeneity problem caused by reciprocal causality was examined by regressing the lagged term as the key explanatory variable, applying the instrumental variable method, and using the propensity score matching (PSM) method to overcome the endogeneity problem caused by reverse causality.
4.3.1 Core explanatory variables lagged one period
In this paper, the lag one period of Internet development is taken as the core explanatory variable for regression, and the results are shown in model 1 of Table 7. Model 1 in Table 4 shows that the lag one period of Internet development still shows a significant positive correlation (β = 2.851, p < 0.01) with the efficiency of China’s agricultural green development, which is consistent with the previous conclusion, indicating that the lag one period of Internet development has a significant positive impact on the efficiency of China’s agricultural green development in the current period. Therefore, the main cause is the development of the Internet in bidirectional causality. Based on this, hypothesis H1 is further verified.
4.3.2 Instrumental variable method
Referring to the existing, more general research results, this paper selects the first-order lag term of Internet development (L.internet) as the instrumental variable of current Internet development; the previous Internet development is the basis of current Internet development, which satisfies the principle of relevance of instrumental variable selection; the current green development of agriculture can not affect the past Internet development, which satisfies the principle of exclusivity of instrumental variable selection.
Meanwhile, referring to the research method of Nunn and Qian (2014), the interaction term is constructed using the national income from information technology services (is) in the previous year and the number of fixed-line telephones (lp) in 1984, which is used as an instrumental variable for the Internet development index of each province in this paper. The Internet came into people’s view from the beginning of telephone line dialing; before the widespread popularity of the Internet, the fixed telephone was an important medium for people’s communication, which meets the relevance requirement; with the promotion of mobile Internet, the influence of fixed telephone gradually diminishes, and for the time being, the number of historically fixed telephones is also difficult to influence the green development of agriculture, which meets the requirement of exogeneity. In order to test whether the instrumental variables have a weak instrumental variable problem, this paper uses a two-stage least squares approach, and the regression results are reported in Table 7, models 2–5. Among them, columns (2) and (4) are the first stage, the coefficients of the instrumental variable internet_IV are all significantly positive at the 1% level, and the results of the LM and F-test are significant. Therefore, there is no under-identification and weak instrumental variable problem for instrumental variables. Columns (3) and (5) show the results of the second stage.
After using different instrumental variables to mitigate the endogeneity of the model, the coefficients of Internet development are all significantly positive, and the conclusion that Internet development can promote green agricultural development is consistent with the results of the benchmark regression. In the instrumental variable applicability test, the coefficients of instrumental variables are all significantly positive in the first stage regression, indicating a strong correlation between instrumental variables and Internet development. The Kleibergen-Paap rk LM values are all significant in rejecting the under-identification hypothesis, and the Cragg-Donald Wald F-values are all greater than the 10% threshold of the Stock-Yogo test, rejecting the weak instrumental variables hypothesis.
4.3.3 Propensity score matching method
Provinces and cities with different levels of Internet development may also have significant differences in other aspects, which may affect agricultural green development and cause self-selection bias in the sample. This endogeneity problem is mitigated by the propensity score matching method (PSM). ① divide the sample into experimental and control groups using the median Internet development as the critical condition; ② use the 14 control variables in this study as covariates and match the propensity scores of the experimental and control groups through the kernel matching method to ensure that there is no significant difference between the matched samples in terms of other basic characteristics except for the Internet development; ③ use the matched samples to re-test the main effects, and the results are shown in Table 7, model 6. The coefficients of the Internet development (internet) coefficient is significantly positive at the 5% level, indicating that the promotion effect of Internet development on agricultural green development after mitigating the problem of self-selection bias endogeneity is still significant, and hypothesis H1 still holds.
4.4 Stability tests
This paper uses the robustness test for measurement error, omitted variables, and special sample problems, respectively. First, for the measurement error, this paper adopts the EBM-SGM index instead of the original super-efficiency DEA to measure the green development of agriculture and the entropy method instead of the original Principal Component Analysis (PCA) to recalculate the Internet development index data, which can weaken the measurement error to a certain extent. Secondly, this paper adds two variables for the omitted variables, production scale (ps) and planting structure (stru), as control variables in the fixed-effects model, which weakens the endogeneity problem caused by the omitted variables to a certain extent. Thirdly, to address the special sample problem, samples during the New Crown Pneumonia epidemic were excluded to rule out the potential interference of the epidemic on the research results.
4.4.1 Replacing the explanatory variable
Drawing on the research methodology of Fang et al. (2021), this paper will choose the SBM-GML index to measure agricultural green total factor productivity to serve as a proxy variable for the explanatory variable agricultural green development, which will further test the robustness of the previous model results. The results are shown in Table 8, model 3, which shows that after replacing the explanatory variable’s measurement method, the Internet’s regression coefficient is 1.185, which is at the 1% significant level. It Indicates that the Internet still has a significant positive impact on agricultural green development after replacing the research method of the explanatory variables, further validating hypothesis H1.
4.4.2 Replacing the core explanatory variables
Drawing on the practice of Narantuya and Otgonbayar (2020), the entropy method is chosen to measure the level of Internet development, to explore the impact of Internet development on the efficiency of China’s agricultural green development after replacing the core explanatory variables of the measurement method, and the results are shown in Table 8, model 2. The results show that after replacing the core explanatory variables, the impact coefficient of the internet is 1.048 and passes the 1% significance level, indicating that the Internet development’s impact on the efficiency of agriculture’s green development is still significant. Green development efficiency is still significant.
4.4.3 Missing variables
Since many factors may impact the efficiency of China’s agricultural green development, this paper may have the problem of omitted variables when selecting variables. Based on this, this paper will further add control variables based on the baseline model to explore whether Internet development will have a greater change on the green development of Chinese agriculture after adding control variables. In Table 8, model 3 shows that after adding the two control variables of production scale (ps) and planting structure (stru), Internet development plays a significant positive role in promoting the efficiency improvement of China’s agricultural green development (β = 2.513, p < 0.01). Although the regression coefficients and significance levels change, the overall results are consistent with those of the previous section, indicating no validation of the omitted variables in this paper. Problem, further validating the conclusions of model 2 in Table 2.
4.4.4 Excluding the special sample
Considering the significant impact of the New Crown Pneumonia epidemic on the economy, this paper excludes the 2020 data and re-runs the regression estimation to exclude the epidemic’s impact on the results; the results are shown in Table 8, model 4. The results show that the impact coefficient of the core explanatory variable internet development (internet) is 2.976 and passes the significance test at the 1% level. It shows that the regression results are still consistent with the previous conclusions after excluding special samples, which further indicates that the model conclusions in this paper have some stability.
5 Further testing
In Table 9, model 2 tests the moderating effect of command-and-control environmental regulation, which shows that the interaction term between command-and-control environmental regulation and the internet (er × internet) significantly affects agricultural green development. In Table 9, model 4 tests the moderating effect of market-incentive environmental regulation. The result shows that the interaction term between market-incentive environmental regulation and the internet (ep × internet) significantly positively affects agricultural green development. These findings indicate that both command-and-control environmental regulations and market-incentive environmental regulations can significantly enhance the role of the Internet in promoting agricultural green development, so hypothesis H2 hold.
At the same time, this paper also examines whether there are significant differences in the moderating effects of environmental regulation in different regions due to differences in the level of enforcement of environmental regulations in different regions. Table 10 shows the moderating effects of environmental regulations in different regions. Models 1–3 show the moderating effects of command-and-control environmental regulation in different regions. The results show that in the eastern region, the moderating effect of command-and-control environmental regulation is significantly positive (β = 0.109, p < 0.05), while in the central and western regions, the moderating effect is positive but not significant (β = 0.070, p > 0.1; β = 0.120, p > 0.1). Models 4–6 show the moderating effects of market-incentivized environmental regulation in different regions. The results show that in the western region, the moderating effect of market incentive-type environmental regulation is significantly positive (β = 0.010, p < 0.05), while in the central and western regions, the moderating effect is positive but not significant (β = 0.021, p > 0.1; β = 0.004, p > 0.1). This suggests regional heterogeneity in the moderating effects of both command-and-control and market-incentive environmental regulation, with command-and-control environmental regulation having a stronger moderating effect in the eastern region and market-incentive environmental regulation having a stronger moderating effect in the western region.
Secondly, there are significant differences between different agricultural functional areas regarding natural conditions, level of economic development, industrial structure, and policy environment. These differences affect the effectiveness of agricultural production methods and environmental protection measures in each region. Therefore, the moderating effect of environmental regulation on the relationship between Internet development and agricultural green development may also differ in different agricultural functional regions. Table 11 demonstrates the moderating effects of environmental regulation in different agricultural functional regions. Among them, models 1–3 are the results of command-and-control environmental regulation. The results show that the moderating effect of the command-and-control type of environmental regulation is significantly positive in the main grain marketing area (β = 0.114, p < 0.05), while the moderating effect in the main grain production area and the equilibrium area is positive but not significant (β = 0.310, p > 0.1; β = 0.138, p > 0.1). Models 4–6 show the results of market incentive-based environmental regulation. The results show that the moderating effect of market-incentivized environmental regulation is positive in all agricultural functional zones, but only the food balance zone passed the significance test. This suggests that there is heterogeneity in the regulatory effects of environmental regulation across functional agricultural regions, with command-and-control environmental regulation being stronger in the eastern region and market-incentive environmental regulation being more significant in the food balance region.
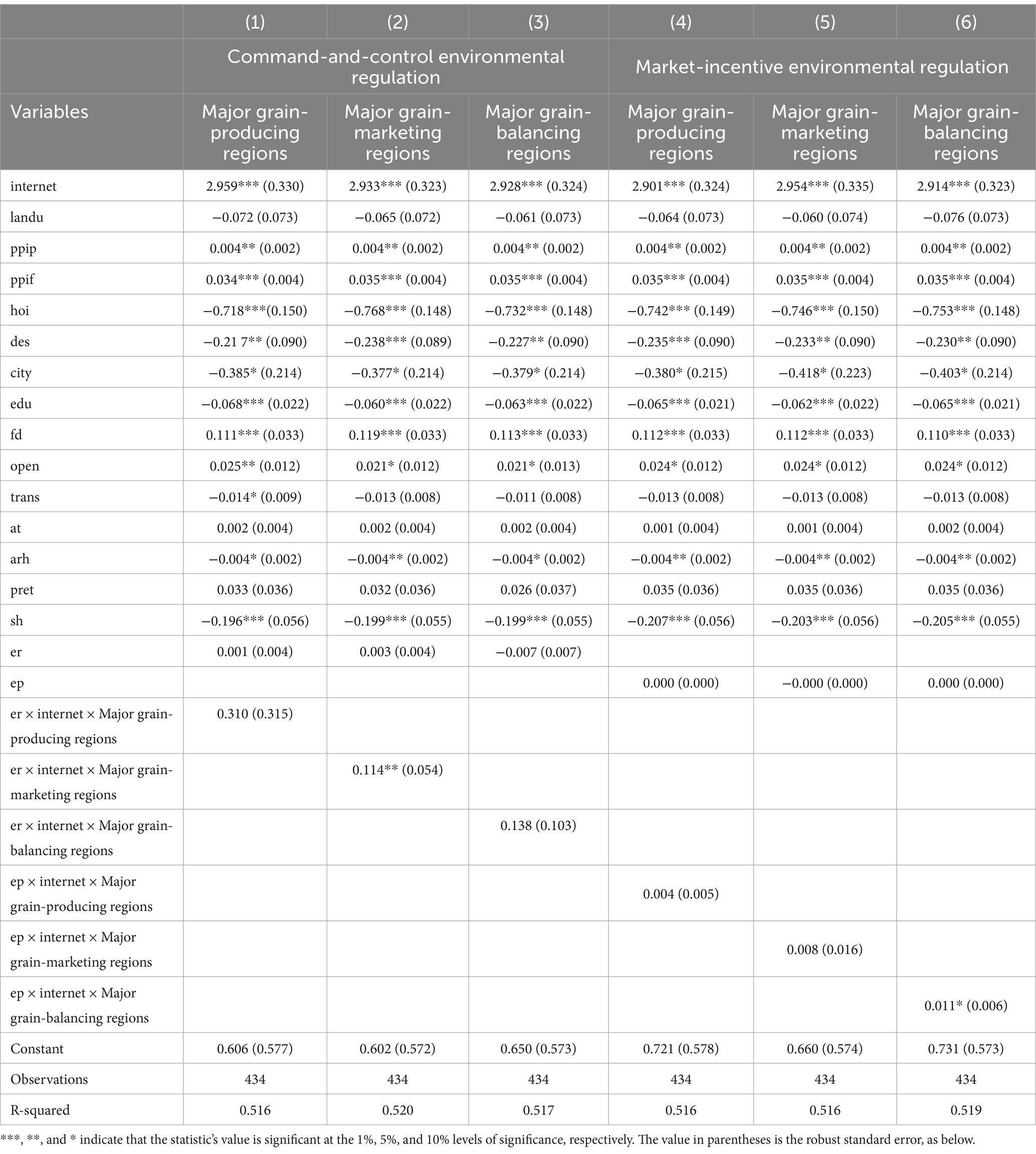
Table 11. Results of the moderating effect of environmental regulation in different agricultural functional areas.
6 Conclusions and implications
6.1 Conclusion
This paper analyses the impact of the Internet on the green development in agriculture by constructing panel data from 2007 to 2020, using 31 provinces in China as the research object. Firstly, using the panel linear regression model to tests the Internet’s impact on agricultural green development. Meanwhile, 31 provinces in China were divided according to geographical location and agricultural functional areas to test for heterogeneity. Then, based on the benchmark model, the moderating effects of command-and-control and market-incentive type environmental regulations on the Internet influencing agricultural green development were explored. The main research conclusions are as follows: (1) Internet development significantly impacts the efficiency of China’s agricultural green development. (2) By region, compared with the central theater region, the eastern region can obtain more information dividends from the Internet development; compared with the main grain marketing region, the Internet development promotes the agricultural green development efficiency of the main grain producing and balancing regions more. (3) Both command-and-control and market-incentive environmental regulations can strengthen the promotion effect of Internet development on China’s agricultural green development efficiency, indicating that with the increase in the intensity of environmental regulations, the promotion effect of Internet development on China’s agricultural green development efficiency is more obvious. (4) Regional heterogeneity exists in the moderating effects of both command-and-control environmental regulation and market-incentive environmental regulation, with command-and-control environmental regulation having a stronger moderating effect in the eastern region and market-incentive environmental regulation having a stronger moderating effect in the western region. (5) There is heterogeneity in the regulatory effect of environmental regulation in agricultural functional areas, in which command-and-control environmental regulation has a stronger regulatory effect in the eastern region. In contrast, market-incentive environmental regulation has a more significant regulatory effect on food balance.
6.2 Implications
Firstly, the government should further promote the construction of rural informatization, increase the penetration rate of Internet and mobile phones in rural areas, broaden the coverage of 5G network stations, broadband, and other network infrastructure in rural areas, facilitate the digital transformation of agricultural production, boost the implementation of the “Internet + agriculture” policy. At the same time, to create a favorable social environment for the “Internet + agriculture,” the government should also do an excellent job planning the strategic development of “Internet + agriculture” at the policy level, guiding the industrial development and application demonstration of “Internet + agriculture.” Then, promoting the opening and sharing of agricultural data by building an agricultural data-sharing platform, facilitating the flow of agricultural information between regions, and encouraging the flow of talents, scientific research results, and other vital elements to rural areas.
Secondly, the government should vigorously prompt the deep integration of the Internet and agriculture, further deepen the application of the Internet in agricultural production, and give full play to the Internet’s role in promoting the efficiency of agricultural green development as far as possible. More specifically, the government should vigorously promote the application of new technologies such as agricultural remote sensing and intelligent agricultural machinery in the whole agricultural industry chain, boost the digital transformation of the whole agricultural industry chain, make every effort to promote the construction of intelligent agriculture and rural areas, further strengthen the application of scientific research technology in agriculture, and drive the progress of cutting-edge agricultural production technology.
Third, the government should multiply and flexibly use the environmental regulation policy tools. On the one hand, most current environmental protection policies are designed for industrial point source pollution and may not fully apply to agricultural surface source pollution. So, the government should further improve the laws and regulations related to environmental protection in agriculture, clarify pollution standards, and control pollution sources at source by formulating relevant laws and regulations to promote green development in agriculture. On the other hand, it is essential to clarify further the detailed approach to regulating agricultural carbon emissions trading and other related regulations and to improve the agricultural carbon trading market by summarizing the lessons learned from regions where carbon trading markets have been opened.
Data availability statement
The original contributions presented in the study are included in the article/supplementary material, further inquiries can be directed to the corresponding author.
Author contributions
XY: Conceptualization, Data curation, Writing – original draft. XW: Methodology, Software, Validation, Writing – original draft. QJ: Funding acquisition, Writing – review & editing.
Funding
The author(s) declare that financial support was received for the research and/or publication of this article. This work was supported by the Humanities and Social Science Foundation of the Ministry of Education in China (grant no. 24YJC630089), the Student Research Innovation Project of Southwest University of Political Science and Law (grant no. 2024XZXS-299), and the Postdoctoral Fellowship Program of CPSF (grant no. GZC20241425).
Conflict of interest
The authors declare that the research was conducted in the absence of any commercial or financial relationships that could be construed as a potential conflict of interest.
Generative AI statement
The authors declare that no Gen AI was used in the creation of this manuscript.
Publisher’s note
All claims expressed in this article are solely those of the authors and do not necessarily represent those of their affiliated organizations, or those of the publisher, the editors and the reviewers. Any product that may be evaluated in this article, or claim that may be made by its manufacturer, is not guaranteed or endorsed by the publisher.
References
Alshehri, M. (2023). Blockchain-assisted internet of things framework in smart livestock farming. Internet Things 22:100739. doi: 10.1641/0006-3568(2005)055[0573:eeaeco]2.0.co;2
Chen, Z., Li, X., and Xia, X. (2021). Measurement and spatial convergence analysis of China’s agricultural green development index. Environ. Sci. Pollut. Res. 28, 19694–19709. doi: 10.1007/s11356-020-11953-z
Cihan, P. (2023). IoT technology in smart agriculture. Int Conf Recent Acad Studi 1, 185–192. doi: 10.59287/icras.693
Dai, X., Chen, Y., Zhang, C., He, Y., and Li, J. (2023). Technological revolution in the field: green development of Chinese agriculture driven by digital information technology (DIT). Agriculture 13:199. doi: 10.3390/agriculture13010199
Dubey, A., and Lal, R. (2009). Carbon footprint and sustainability of agricultural production systems in Punjab, India, and Ohio, USA. J. Crop Improv. 23, 332–350. doi: 10.1080/15427520902969906
Duguma, A. L., and Bai, X. (2025). How the internet of things technology improves agricultural efficiency. Artif. Intell. Rev. 58:63. doi: 10.1007/s10462-024-11046-0
Fang, L., Hu, R., Mao, H., and Chen, S. (2021). How crop insurance influences agricultural green total factor productivity: evidence from Chinese farmers. J. Clean. Prod. 321:128977. doi: 10.1016/j.jclepro.2021.128977
Guo, Y., Tong, L., and Mei, L. (2022). Spatiotemporal characteristics and influencing factors of agricultural eco-efficiency in Jilin agricultural production zone from a low carbon perspective. Environ. Sci. Pollut. Res. 29, 29854–29869. doi: 10.1007/s11356-021-16463-0
Guo, H., Xu, S., and Pan, C. (2020). Measurement of the spatial complexity and its influencing factors of agricultural green development in China. Sustain. For. 12:9259. doi: 10.3390/su12219259
Huang, T., Quan, Y., and Li, N. (2024). Reallocate to the right place: the heterogeneous effect of internet use on factor allocation of rural households in China. Econ Analy Policy 84, 1328–1346. doi: 10.1016/j.eap.2024.10.026
Jiang, G. (2022). How does agro-tourism integration influence the rebound effect of China’s agricultural eco-efficiency? An economic development perspective. Front. Environ. Sci. 10:689. doi: 10.3389/fenvs.2022.921103
Jiang, Q., Li, J., Si, H., and Su, Y. (2022). The impact of the digital economy on agricultural green development: evidence from China. Agriculture 12:1107. doi: 10.3390/agriculture12081107
Kiiski, S., and Pohjola, M. (2002). Cross-country diffusion of the internet. Inf. Econ. Policy 14, 297–310. doi: 10.1016/S0167-6245(01)00071-3
Koutroumpis, P. (2009). The economic impact of broadband on growth: a simultaneous approach. Telecommun. Policy 33, 471–485. doi: 10.1016/j.telpol.2009.07.004
Liu, Y., Sun, D., Wang, H., Wang, X., Yu, G., and Zhao, X. (2020). An evaluation of China’s agricultural green production: 1978–2017. J. Clean. Prod. 243:118483. doi: 10.1016/j.jclepro.2019.118483
Liu, D., Zhu, X., and Wang, Y. (2021). China's agricultural green total factor productivity based on carbon emission: an analysis of evolution trend and influencing factors. J. Clean. Prod. 278:123692. doi: 10.1016/j.jclepro.2020.123692
Miguel, A., Altieri, M. A., and Holt-Gimenez, E. (2012). Green agriculture: foundations for biodiverse, resilientand productive agricultural systems. Int J Agricult Sustain 10, 61–75. doi: 10.1080/14735903.2011.610206
Narantuya, E., and Otgonbayar, B. (2020). Entropy weight method for evaluating indicators of ICT development index. Int J Curr Adv Res 9, 23500–23505. doi: 10.24327/ijcar.2020.23504.4654
Nunn, N., and Qian, N. (2014). US food aid and civil conflict. Am. Econ. Rev. 104, 1630–1666. doi: 10.1257/aer.104.6.1630
Pimentel, D., Hepperly, P., HansonJ, D. D., and Seidel, R. (2005). Environmental, energetic and economic comparisons oforganic and conventional farming systems. BioScience 55, 573–582.
Roy, R., and Aslekar, A. (2022) IoT in farm productivity enhancement. In 2022 International Conference on Decision aid Sciences and Applications (DASA). IEEE, pp. 1034–1039
Sadiku, M. N. O., Ashaolu, T. J., Ajayi-Majebi, A., and Musa, S. M. (2021). Internet of things in agriculture: a primer. Int J Sci Adv 2, 215–220. doi: 10.51542/ijscia.v2i2.24
Shen, J., Zhu, Q., Jiao, X., Ying, H., Wang, H., Wen, X., et al. (2020). Agriculture green development: a model for China and the world. Front Agricult Sci Engineer 7, 5–13. doi: 10.15302/J-FASE-2019300
Sinwar, D., Dhaka, V. S., Sharma, M. K., and Rani, G. (2020). AI-based yield prediction and smart irrigation. Internet Things Anal Agric 67, 155–180. doi: 10.1007/978-981-15-0663-5_8
Solow, R. M. (1956). A contribution to the theory of economic growth. Q. J. Econ. 70, 65–94. doi: 10.2307/1884513
Talukder, B., Blay-Palmer, A., Vanloon, G. W., and Hipel, K. W. (2020). Towards complexity of agricultural sustainability assessment: Main issues and concerns. Environ Sustain Indicat 6:100038. doi: 10.1016/j.indic.2020.100038
Veisi, H., Liaghati, H., and Alipour, A. (2016). Developing an ethics-based approach to indicators of sustainable agriculture using analytic hierarchy process (AHP). Ecol. Indic. 60, 644–654. doi: 10.1016/j.ecolind.2015.08.012
Xiang, F., and Wang, D. Research on operation mode of “internet plus” agricultural products intelligent supply chain. In: 2020 International Conference on Urban Engineering and Management Science (ICUEMS). IEEE, (2020): 208–211.
Xu, L., Jiang, J., and Du, J. (2022). The dual effects of environmental regulation and financial support for agriculture on agricultural green development: spatial spillover effects and Spatio-temporal heterogeneity. Appl. Sci. 12:11609. doi: 10.3390/app122211609
Xu, B., Niu, Y., Zhang, Y., Chen, Z., and Zhang, L. (2022). China’s agricultural non-point source pollution and green growth: interaction and spatial spillover. Environ. Sci. Pollut. Res. 29, 60278–60288. doi: 10.1007/s11356-022-20128-x
Yang, Z., Fan, M., Shao, S., and Yang, L. (2017). Does carbon intensity constraint policy improve industrial green production performance in China? A quasi-DID analysis. Energy Econ 68, 271–282. doi: 10.1016/j.eneco.2017.10.009
Zhang, F., Wang, F., Hao, R., and Wu, L. (2022). Agricultural science and technology innovation, spatial spillover and agricultural green development—taking 30 provinces in China as the research object. Appl. Sci. 12:845. doi: 10.3390/app12020845
Keywords: Internet, green development in agriculture, environmental regulation, agricultural green transformation, digital agriculture
Citation: Yi X, Wang X and Jiang Q (2025) From fields to bytes: the Internet’s impact on China’s green agricultural revolution. Front. Sustain. Food Syst. 9:1547332. doi: 10.3389/fsufs.2025.1547332
Edited by:
Siphe Zantsi, Agricultural Research Council of South Africa (ARC-SA), South AfricaReviewed by:
Rudzani Nengovhela, University of Limpopo, South AfricaTawanda Marandure, Swedish University of Agricultural Sciences, Sweden
Copyright © 2025 Yi, Wang and Jiang. This is an open-access article distributed under the terms of the Creative Commons Attribution License (CC BY). The use, distribution or reproduction in other forums is permitted, provided the original author(s) and the copyright owner(s) are credited and that the original publication in this journal is cited, in accordance with accepted academic practice. No use, distribution or reproduction is permitted which does not comply with these terms.
*Correspondence: Qi Jiang, MTAzNjQ1MzU4MEBxcS5jb20=
†These authors have contributed equally to this work and share first authorship