- 1School of Economics, Shandong University of Technology, Zibo, China
- 2Institute of Business and Policy Research, Sher-e-Kashmir University of Agricultural Sciences and Technology of Kashmir, Srinagar, India
- 3School of Economics and Management, Southeast University, Nanjing, China
- 4School of Economics and Business, Western Caspian University, Baku, Azerbaijan
- 5Department of Green Economics, Tashkent State University of Economics, Tashkent, Uzbekistan
- 6Applied Science Research Center, Applied Science Private University, Amman, Jordan
Introduction: Promoting sustainable food systems requires advancing social and economic equity, particularly in rural areas with limited access to technology. Digital skills are increasingly vital in narrowing consumption disparities and fostering sustainable livelihoods.
Methods: This study investigates the impact of digital skills on consumption inequality among Chinese farmers using longitudinal data from the 2014–2020 China Family Panel Studies (CFPS). A Tobit regression model is employed, supplemented with robustness checks.
Results: The findings reveal that digital skills significantly reduce consumption inequality, especially in subsistence-related categories (such as food and healthcare) and enjoyment-oriented categories (such as education and leisure).
Discussion: Mechanism analysis identifies three key pathways through which digital skills operate: reducing mobility constraints, increasing non-farm employment opportunities, and improving access to information. The effects are particularly pronounced among farmers aged over 60 and those residing in central, western, northeastern, and northern regions. Policy implications include expanding digital skills training, enhancing regulation of rural credit institutions, and improving digital platforms for information dissemination. Tailored, region-specific strategies are recommended to accelerate digital inclusion and promote equitable rural development.
1 Introduction
Achieving sustainable food systems is not only about ensuring food security and improving agricultural productivity but also about addressing social inequalities that affect access to essential resources and overall well-being. The Outline of the Fourteenth Five-Year Plan for National Economic and Social Development of the People’s Republic of China and Vision 2035 emphasizes the objectives of “enhancing people’s well-being” and “raising the level of common governance and sharing.” These goals highlight China’s commitment to reducing social disparities and promoting inclusive growth. Consumption inequality, as a critical measure of social welfare disparities, reflects imbalances in access to food, nutrition, and other essential resources. Addressing these disparities is essential for ensuring equitable food systems where all population groups can benefit from economic progress. Studies by Meyer and Sullivan (2011), Reichlin (2020) and Attanasio and Pistaferri (2014) have demonstrated that consumption inequality not only represents economic disparities but also serves as an indicator of the uneven distribution of societal progress. In China, tackling consumption inequality is crucial not only for improving living standards but also for achieving social harmony, effective governance, and inclusive food systems that contribute to sustainable development.
Although rural residents in China have experienced a notable increase in consumption levels over recent years, the challenge of consumption inequality persists (Liu and Zhou, 2021). Data from the 2014–2020 China Family Tracking Survey (CFPS) shows that the gap between the richest and poorest rural households grew from 22.9 times to 26.7 times. This widening gap reveals that economic growth benefits have not been evenly shared among rural households (Zhong et al., 2022). Consumption inequality in rural areas poses significant challenges to achieving inclusive development, as it exacerbates socio-economic disparities, limits opportunities for upward mobility, and undermines efforts to foster a sense of shared prosperity among citizens (Yi et al., 2024).
In the 21st century, the rapid advancement of digital technologies, including the Internet, big data, and artificial intelligence, has transformed various aspects of social and economic life (Imamov and Semenikhina, 2021). These technologies have not only reshaped urban centers but have also begun to penetrate rural areas, bringing about new opportunities and challenges for rural development. According to the Statistical Report on the Development of the Internet in China, as of June 2024, over 90.1% of Internet users had acquired at least one form of digital literacy, indicating a widespread adoption of digital skills across the population (Li and Chen, 2024). Promoting digital villages and integrating digital technologies into rural economies have boosted household incomes, improved the consumption environment, and offered new ways to reduce consumption inequality. Digital tools help rural residents access goods and services, lower transaction costs, and participate more effectively in the digital economy (Tim et al., 2021). However, despite these advancements, the specific impact of digital skills application on consumption inequality among farmers remains insufficiently understood. This paper seeks to bridge this gap by examining the extent to which digital skills can mitigate consumption inequality, exploring the underlying mechanisms of this relationship, and identifying effective strategies for reducing disparities in rural consumption in the digital era.
The rest of the article is organized as follows: Section 2 reviews the literature, Section 3 covers theoretical analysis and hypothesis development, Section 4 explains the data and methods, Section 5 presents the results and discussion, and Section 6 concludes with policy implications.
2 Literature review
Consumption inequality refers to the differences in consumption patterns, levels, and structures among various income groups or social classes. It complements the study of economic inequality by focusing on how resources are used and welfare is experienced by individuals and households. Common measures like the Gini coefficient, Theil index, and quartile differences provide insights into overall consumption distribution but often miss finer details of individual welfare differences. To address this gap, concepts like “relative deprivation” have been introduced, measuring how individuals perceive their consumption compared to others in their reference groups. More precise indices such as the Yitzhaki index, Kakwani index, and Podder index offer a detailed view of these individual-level disparities, providing a deeper understanding of consumption inequality.
The relationship between digital skills application and consumption inequality has emerged as a key area of inquiry in recent years. Digital skills, encompassing the ability to use digital tools effectively, are recognized as a transformative factor in shaping consumption behavior. By reducing transaction costs and enhancing access to diverse goods and services, digital skills enable individuals to participate more actively in the economy. Research by Beheshti et al. (2024) and Purcărea et al. (2022) has highlighted how digital tools facilitate cost reductions, unlock latent consumption potential, and promote behavioral shifts toward more diversified consumption patterns (Hamari et al., 2016; Ertz et al., 2019) further emphasizes that digital platforms, such as e-commerce, online social networks, and digital entertainment services, not only broaden consumption opportunities but also influence individuals’ consumption concepts, encouraging them to allocate more resources to emerging consumption categories.
However, the impact of digital skills on consumption inequality is not uniform and often depends on contextual factors. These Studies (Cruz-Jesus et al., 2017; Gamede, 2022) have identified a non-linear relationship between digital skills application and consumption behavior, influenced by the stage of digital infrastructure development and socio-economic characteristics of the population. In the early stages of digital adoption, inadequate infrastructure and low levels of trust in digital technologies may limit their effectiveness (Hussain et al., 2024) argue that in such contexts, rural residents may resist utilizing digital tools for consumption due to concerns over privacy, security, and unfamiliarity with digital platforms. As digital infrastructure improves and residents become more accustomed to these technologies, their trust and willingness to engage in digital consumption increase, leading to significant improvements in consumption efficiency and structural optimization, as noted by Zhang Y. et al. (2023).
Conversely, for certain segments of the population, digital skills may have minimal impact on consumption patterns (French et al., 2021) point out the “knowledge threshold effect” of digital technologies, where individuals with low levels of education or digital literacy are unable to fully leverage digital tools for productive activities. Instead, they often use the internet for entertainment purposes, which does not translate into substantial changes in their overall consumption levels. Wang et al. (2023) highlighted that the effectiveness of digital skills application depends on individuals’ willingness and ability to engage meaningfully with digital tools, a factor that varies significantly across different demographic and socio-economic groups.
Some studies have focused on the understanding of digital transformation’s broader socio-economic impacts, which aligns with the mechanisms explored in this study. For instance, Zhao et al. (2024b) emphasized that digital transformation significantly contributes to pollution reduction in manufacturing enterprises, suggesting its capacity to reconfigure traditional production-consumption dynamics. Similarly, Zhao S. et al. (2023) showed that China’s low-carbon strategies have catalyzed digital upgrades in manufacturing, revealing how policy-induced digitalization can affect structural change. In the labor domain, Zhang et al. (2023b) and Zhang et al. (2023a) introduced models highlighted the importance of skill heterogeneity and factor matching in labor productivity and income distribution, supporting the notion that digital skills mediate employment outcomes. Moreover, Zhao et al. (2024a) underscored the influence of leadership’s digital background on innovation, which resonates with the argument about capacity-building as a key enabler of rural digital engagement. Wang et al. (2025) introduced evolutionary game theory to explore the diffusion of technologies under policy combinations, showing how strategic behavior affects adoption—an idea complementary to the heterogeneous digital uptake observed among farmers. Cheng et al. (2025) also highlighted the critical role of environmental uncertainty and entrepreneurial orientation, reinforcing the need to consider rural context variability. Finally, Luo et al. (2022) demonstrated how AI-based systems built on IoT can support agriculture, suggesting that digital platforms not only improve productivity but also information accessibility—one of the identified mechanisms.
Despite valuable insights from existing research, several gaps remain. Most studies on consumption inequality focus on overall patterns, overlooking the specific challenges faced by rural farmers. Additionally, while digital skills’ effects on consumption behavior have been studied, their impact on consumption inequality among farmers is rarely addressed. This study seeks to fill these gaps by using the Kakwani index to measure farmers’ consumption inequality across three categories—subsistence, development, and enjoyment—based on data from the China Family Tracking Survey (CFPS). A Tobit model is applied to analyze how digital skills affect consumption inequality, exploring mechanisms like mobility constraints, non-farm employment, and information access. The study also investigates regional and age-related differences in these effects, providing tailored recommendations to reduce consumption inequality among farmers.
This study offers three key contributions. First, it presents a new analytical approach using the Tobit model to assess how digital skills affect various aspects of consumption inequality. Second, it investigates how digital skills reduce consumption disparities by alleviating constraints, enhancing employment opportunities, and improving information access. Third, it provides evidence of how digital skills impact different regions and demographic groups, offering valuable insights for policymakers aiming to promote equitable rural development. By addressing these issues, this study enhances the understanding of digital inclusion and its role in promoting social equity and sustainable rural development.
3 Theoretical analysis and research hypotheses
Changes in consumption inequality depend on demand-side consumption ability and willingness to consume (Liu et al., 2024; Wilmers, 2017). The application of new-generation digital technologies, such as mobile Internet, e-commerce platforms, and digital finance, has provided farmers with abundant information resources and convenient consumption channels (Han and Kim, 2019), becoming an important driving force for improving farmers’ consumption ability and willingness.
In terms of consumption ability, according to Keynes’s absolute income hypothesis and Duesenberry’s relative income hypothesis, consumption depends on income in both the long and short term (Christelis et al., 2019). Thus, an increase in farmers’ income levels corresponds to an increase in their consumption ability. The application of digital skills can, on one hand, facilitate the transformation of traditional agricultural production, improve agricultural efficiency (Fabregas et al., 2019), and reduce production costs. At the same time, it enables farmers to overcome geographical limitations on selling agricultural products via e-commerce platforms, broadening sales channels and thereby improving their operating income (Li and Qin, 2022). On the other hand, digital skills help farmers adapt quickly to job positions requiring digital operations, increasing their wage income. Moreover, digital financial platforms enable farmers to engage in low-risk financial management activities, increasing their property income (Li et al., 2022), thus raising their overall income levels.
In terms of consumption willingness, farmers who use digital skills can access a variety of online consumption platforms, significantly reducing consumption costs (Rosário and Raimundo, 2021), improving convenience, and meeting their diverse consumption needs. Additionally, digital skills allow farmers to benefit from high-quality and comprehensive services, such as e-commerce, digital finance, and after-sales protection, ensuring that they receive high-quality products and services during the consumption process (Palese and Usai, 2018). This, in turn, enhances their willingness to consume. Therefore, the following hypothesis is proposed:
Hypothesis H1: Digital skills application can mitigate consumption inequality among farmers.
Digital skills applications can reduce farmers’ consumption inequality by addressing mobility constraints, non-farm employment opportunities, and access to information.
In terms of the liquidity constraint pathway, digital skills application can enhance farmers’ participation in and responsiveness to digital finance (Aziz and Naima, 2021), alleviating current liquidity constraints. Firstly, digital skills enable farmers to access and interpret financial market information more accurately (Kumar et al., 2023), match appropriate credit options and structural arrangements to their conditions and household needs, and flexibly organize capital withdrawal and repayment plans. Secondly, digital skills also allow residents to conveniently access digital credit and financial management services (Wang et al., 2023), such as online lending and digital banking, enabling them to quickly obtain credit resources and improve funding efficiency (Chen et al., 2024). Alleviating liquidity constraints not only allows residents to secure funds through credit to meet consumption needs (Yu C. et al., 2022) but also reduces the incentive for precautionary savings, raising current consumption levels (Holm, 2018). This alleviates farmers’ consumption inequality.
In terms of the non-farm employment pathway, digital skills applications can expand farmers’ employment options and increase the probability of non-farm employment. Firstly, digital skills reduce the social resource constraints faced by farmers (Correa, 2016), enabling them to access richer information about non-farm employment opportunities through online recruitment platforms, social media, and other channels. This reduces the difficulty of job searching (Zhan and Yang, 2024), increasing employment choices. Additionally, digital skills help farmers adjust their risk preferences, accumulate social capital, and acquire the human, technical, and market resources needed for entrepreneurship. This increases the likelihood of successful entrepreneurial ventures (Tan and Li, 2022). Secondly, digital skills allow farmers to acquire new knowledge, ideas, and skills through diverse channels, enhancing their competitiveness in the job market. This enables farmers to quickly and comprehensively obtain job details through the Internet and other resources, effectively reducing information asymmetry during the job search process (Kong et al., 2022). As a result, their probability of employment improves. Non-farm employment broadens income sources and reduces the risk of frictional unemployment (Reardon et al., 2000), thereby improving consumption levels and mitigating consumption inequality among farmers.
The application of digital skills improves farmers’ access to information, changing how they gather, process, and use it. Through smartphones, computers, and other digital devices, farmers can easily reach e-commerce platforms, social media, and agricultural websites. This helps them overcome geographical barriers and access more diverse and useful consumption-related information (Lin and Ma, 2022). Improved information access expands farmers’ consumption choices and enables them to utilize various online platforms, such as WeChat and Jieyin, to purchase goods (Nguyen et al., 2018), enjoying a wider selection of consumer goods (Vatsa et al., 2023). This compensates for the high search costs traditionally associated with rural consumption, thereby alleviating consumption inequality. Therefore, the following hypotheses are proposed:
Hypothesis H2: Digital skills application mitigates farmers’ consumption inequality by alleviating mobility constraints.
The application of digital skills can mitigate farmers’ consumption inequality by alleviating mobility constraints. Rural farmers face numerous challenges related to mobility, particularly in terms of limited access to broader consumer markets and essential financial services. The geographical isolation and logistical constraints often result in higher transaction costs and limited choices for rural consumers (Atalay and Edwards, 2022). Digital technologies like mobile phones, the internet, and digital financial platforms have been essential in closing the gap between rural and urban areas. With digital skills, farmers can access services that were previously out of reach. E-commerce platforms, for instance, enable them to buy goods that would have been too costly or inaccessible due to their remote locations (Yu C. et al., 2022).
Furthermore, the ability to access digital finance platforms enables farmers to engage in online transactions, credit facilities, and digital banking, thus overcoming the limitations posed by physical distance and financial exclusion (Aziz and Naima, 2021). These technological advancements provide farmers with tools to enhance their purchasing power and access a wider array of goods and services, thereby increasing their consumption choices. Moreover, the convenience of digital platforms enables farmers to bypass traditional barriers such as travel time and costs associated with shopping in urban centers (Nie et al., 2021). By reducing mobility constraints, digital skills allow farmers to engage more effectively in the market economy, leading to fairer consumption outcomes and helping to narrow the gap between rural and urban populations.
Hypothesis H3: Digital skills application mitigates farmers’ consumption inequality by promoting non-farm employment.
The application of digital skills mitigates farmers’ consumption inequality by promoting non-farm employment opportunities. In many rural areas, income inequality is exacerbated by the limited availability of non-agricultural employment opportunities, which often forces farmers to rely solely on seasonal or unstable agricultural income.
However, digital skills open a wide range of non-farm employment options, allowing farmers to diversify their income streams and increase their financial stability. The rise of online recruitment platforms, digital entrepreneurship, and remote working opportunities has made it possible for farmers to access jobs and business ventures beyond traditional agricultural sectors (Espinosa Zárate et al., 2023). For instance, digital platforms such as LinkedIn, Upwork, and regional job boards provide access to remote job opportunities, allowing farmers to engage in non-farm work and earn wages that complement their agricultural activities (Walker, 2019). Moreover, digital skills enable farmers to engage in e-commerce and other entrepreneurial activities that broaden income sources. By utilizing social media, e-commerce platforms, and digital marketing tools, farmers can sell agricultural products directly to consumers, increasing their market reach and profitability (Yang et al., 2022). The ability to engage in non-farm employment or entrepreneurial ventures enhances financial stability, reduces dependence on unpredictable agricultural income, and helps smooth consumption patterns over time. Furthermore, digital literacy allows farmers to enhance their competitiveness in the job market by acquiring new skills and knowledge that improve their employability (Cheng et al., 2024). As a result, non-farm employment opportunities, facilitated by digital skills, contribute to the diversification of income sources, which directly mitigates consumption inequality among farmers by providing more stable and higher income levels.
Hypothesis H4: Digital skills application mitigates farmers’ consumption inequality by enhancing access to information.
The application of digital skills mitigates farmers’ consumption inequality by enhancing access to information. One of the critical factors contributing to consumption inequality in rural areas is the lack of timely and accurate information about prices, product availability, and market trends. Farmers, especially those in remote areas, have traditionally faced significant information asymmetry, making it difficult to make informed purchasing decisions and to access products at competitive prices (Courtois and Subervie, 2015). Digital skills, however, have dramatically transformed how information is accessed and utilized in rural areas. Through smartphones, computers, and the internet, farmers can now access a wealth of information on e-commerce platforms, agricultural websites, and social media, significantly enhancing their ability to compare prices, evaluate product quality, and make informed consumption decisions (Ji et al., 2020). This reduction in information asymmetry allows farmers to access better deals on consumer goods and services, which in turn improves their overall consumption experience (Yu M. et al., 2022).
Moreover, digital platforms offer comprehensive information not only on consumer goods but also on agricultural practices, government subsidies, and financial products, which can help farmers make more informed decisions about investments in both consumption and production (Yu M. et al., 2022). By improving access to valuable resources, digital skills help rural farmers overcome traditional knowledge barriers, enabling them to make decisions that better suit their needs and preferences. This improved access to information leads to a fairer distribution of consumption opportunities, as farmers are no longer restricted by high search costs or unreliable local markets (Njuguna et al., 2025). As such, the application of digital skills improves consumption equality by providing farmers with the tools necessary to navigate and thrive in an increasingly information-driven economy.
To better illustrate the theoretical mechanisms underlying the relationship between digital skills and consumption inequality, Figure 1 presents a conceptual framework highlighting both direct and indirect pathways.
• The direct effects of digital skills include reducing production costs, increasing income, and improving consumer service quality, all of which enhance farmers’ spending power and contribute to a more equitable consumption structure.
• The indirect effects operate through eased liquidity constraints, expansion of non-farm employment opportunities, and elimination of information asymmetry, which collectively stimulate farmers’ willingness to consume and reduce disparities in consumption patterns.
These mechanisms provide the foundation for the empirical analysis, where we examine the extent to which digital skills reduce consumption inequality and assess the relative strengths of the direct and indirect effects. The conceptual model in Figure 1 serves as a theoretical guide for the hypothesis development and econometric strategy.
4 Materials and methods
4.1 Data source
This study selects data from the 2014–2020 China Family Tracking Survey (CFPS), a database with a survey sample covering 25 provinces across the country, providing comprehensive household demographic information as well as income and consumption expenditure data. Therefore, by taking the head of household as the research object and excluding urban residents and samples with missing primary information, a total of 15,268 observations were ultimately obtained.
4.2 Selection of variables
4.2.1 Explained variables
This study measures farmers’ consumption inequality across four categories: total consumption, subsistence consumption, developmental consumption, and enjoyment consumption. Firstly, referring to Guo et al. (2023), the expenditure on food, housing, and clothing is classified as subsistence consumption; the expenditure on transport and communication, health care, and culture and education is classified as developmental consumption; and the expenditure on culture, education, and recreation, as well as household equipment and durable goods, is classified as enjoyment consumption. Secondly, following the research of Luo and Li (2022), other individuals within the same county as the household are selected as the reference group. Household consumption expenditures are compared with those of other households within the same county to calculate the Kakwani index. This index measures the degree of individual consumption inequality, where a higher value indicates greater inequality. The Kakwani index is calculated using the formula provided in Equation 1.
where n is the number of observations in the reference cluster Y, is the mean value of consumption in the cluster, is a vector of consumption by households within the cluster and satisfies the inequality , is the mean value of consumption in the cluster for samples with consumption levels exceeding , is the mean value of the logarithm of consumption for samples with logarithms of consumption exceeding , and is the share of observations with consumption levels exceeding yi in the total number of observations.
4.2.2 Core explanatory variables
To comprehensively measure digital skills, a composite index is constructed, reflecting different levels of digital proficiency. This index considers three dimensions: (1) frequency of internet use for activities like socializing, shopping, studying, and working, (2) diversity of digital activities such as using digital financial services, participating in online training, and engaging in e-commerce, and (3) self-reported confidence in performing digital tasks. Principal Component Analysis (PCA) is used to assign weights to each dimension, ensuring a more precise assessment of digital skills. This approach provides a clearer understanding of how digital skills influence consumption inequality among farmers.
4.2.3 Mechanism variables
The mechanism variables in this paper are liquidity constraint and non-farm employment. For the measurement of liquidity constraint, drawing on the study of Zhao T. et al. (2023) “whether the household’s highly liquid assets are less than half of the household’s annual income” is used to measure the liquidity constraint, in which the highly liquid assets are measured by the net value of the financial assets, i.e., the difference between the financial assets and the non-housing liabilities. When the household’s highly liquid assets are less than half of the household’s annual income, the liquidity constraint is assigned a value of 1, otherwise it is 0. For the measurement of non-farm employment, drawing on the study by Huang et al. (2022), the question of ‘the nature of the current job’ is used to determine whether the non-farm employment is non-farm employment, and if the answer is a non-farm job, non-farm employment is assigned a value of 1, otherwise it is assigned a value of 1. For the measurement of information access, drawing on the methodology of Jiao et al. (2024), the question in the questionnaire ‘the importance of the Internet in accessing information’ was used as the measure, with the values ranging from 1 to 5, with the larger value representing the higher degree of importance of the application of digital skills to farmers’ information access.
4.2.4 Control variables
To control the influence of other variables on farmers’ consumption inequality, the selection of control variables is considered from two perspectives: individual and household. Among them, individual characteristics control gender, age, education, marital status and health level, while family characteristics mainly control family size, property purchase and family per capita income, which is logarithmic due to the large value of family per capita income. Descriptive statistics of variables are given in Table 1. The descriptive statistics reveal moderate consumption inequality among farmers (Kakwani index: 0.4149). Digital skills usage is low, with only 25.18% of respondents engaged in online activities. Liquidity constraints are widespread, affecting 69.24% of households, while non-agricultural employment remains limited at 23.79%. The average age is 51.81 years, education levels are low at 2.3 years, and per capita income disparities are evident (log average: 8.82). Despite high property ownership (92.85%) and a significant proportion of married respondents (85.22%), these findings highlight critical challenges, including low digital inclusion and limited income diversification.
4.3 Model construction
To construct the baseline model for testing the impact of digital skills application on farmers’ consumption inequality, a Tobit model is developed, shown in Equation 2, given that the measured farmers’ consumption inequality indexes are all restricted explanatory variables ranging between 0 and 1.
where is the explanatory variable farmers’ consumption inequality, is the core explanatory variable digital skills application, is the control variables at the individual and household level, and is the constant term. Similarly, and are the regression coefficients of the equation, and is the random disturbance term.
We employ the Tobit model to estimate the impact of digital skills on farmers’ consumption inequality, as the dependent variable is censored at a lower bound and cannot take negative values. Using ordinary least squares (OLS) in such cases can lead to biased estimates, whereas the Tobit model properly accounts for both the probability of nonzero consumption inequality and its magnitude.
While quantile regression and generalized linear models (GLMs) could be alternatives, the Tobit model is more suitable for handling censored data. Quantile regression is useful for analyzing heterogeneous effects but does not correct for truncation at the lower bound. Similarly, GLMs, such as Poisson or gamma regressions, are designed for skewed distributions but are less effective in cases where the dependent variable is naturally constrained. The Tobit model, by contrast, captures both the occurrence of consumption inequality and its intensity, making it the preferred approach.
5 Results and discussion
5.1 Results of benchmark regression
The empirical results strongly support the theoretical framework presented in Figure 1, confirming that digital skills influence farmers’ consumption inequality through both direct and indirect pathways. The direct effects of digital skills are reflected in higher income levels, reduced production costs, and improved service quality, all of which enhance farmers’ spending power. The indirect effects, including eased liquidity constraints, increased access to non-farm employment, and reduced information asymmetry, contribute to greater financial stability, thereby stimulating consumption and reducing disparities in spending capacity.
These findings substantiate the core hypothesis that digital skills play a crucial role in mitigating consumption inequality through multiple reinforcing mechanisms, as outlined in Figure 1. Moreover, the varying strengths of these mechanisms offer valuable policy insights, underscoring the importance of prioritizing employment-driven digital training programs and expanding financial inclusion efforts to maximize the economic benefits of digital adoption in rural communities.
The impact of digital skills application on farmers’ consumption inequality is further examined in Table 2. Columns (1)–(3) present the estimation results under different model specifications: without control variables, with individual controls, and with household controls, respectively. Across all specifications, digital skills application consistently exhibits a negative and statistically significant effect on consumption inequality at the 1% level, confirming hypothesis H1. This finding demonstrates that digital adoption significantly reduces consumption inequality by enhancing consumption convenience, improving the quality of goods and services, strengthening willingness to consume, and increasing income levels. Through these mechanisms, digital skills enable farmers to overcome financial barriers and expand their consumption capacity, thereby fostering a more equitable distribution of economic resources.
5.2 Robustness test
5.2.1 Replacement of the regression model
Considering inter-individual differences and trends over time, the Tobit model is replaced with a two-way fixed effects model for robustness testing, and the specific model is constructed as shown in Equation 3.
where is the inequality in farmers’ consumption, is the application of digital skills, is the control variable, and is the constant term. Similarly, are the regression coefficients of the equations. is the random error term. and are the individual fixed effects and time fixed effects, respectively.
Table 3 reports the results of this robustness test. The estimated coefficient of digital skills application is still significantly negative after replacing the regression model, which further indicates that digital skills application can improve farmers’ consumption inequality and proves the robustness of the baseline regression results.
5.2.2 Replacement of explanatory variables
In this study, the frequency of farmers’ use of the internet for activities such as social entertainment, online shopping, and work-study is used as an explanatory variable to measure digital skills application for robustness testing. Table 3 reports the results of this robustness test. After replacing the explanatory variables, the digital skills application estimates are still negative at the 5 percent significance level, again illustrating the robustness of the baseline regression results.
5.2.3 Impact of outlier adjustment
To address the potential influence of outliers on the regression results, a 3% upper and lower shrinkage treatment was applied to all variables in the original sample, followed by re-estimation. The regression results, presented in Table 3, show no significant changes in parameter estimates or significance levels. The regression coefficient for digital skills application remains significantly positive at the 1% level, confirming the robustness of the findings.
5.3 Endogeneity test
To study the impact of digital skills application on farmers’ consumption inequality, the model accounts for potential endogeneity issues stemming from omitted variables or unobserved factors. Two instrumental variables are selected: the coverage of mobile base stations (number per square kilometer in the household’s province) and the average level of digital skills application within the household’s district or county. To address potential endogeneity concerns, we employ two instrumental variables: (1) mobile base station coverage (number of base stations per square kilometer in a given province) and (2) regional digital skills levels (the average level of digital skills application in the household’s district or county). These instruments are justified based on two key conditions: (1) relevance and (2) exclusion restriction.
First, both instruments are strongly correlated with individual digital skills applications. Mobile base station coverage directly influences an individual’s ability to acquire and use digital tools, while regional digital skills levels reflect the broader digital environment, increasing the likelihood of personal digital adoption. Second, these variables satisfy the exclusion restriction, as they do not independently determine consumption inequality. Mobile base station coverage influences digital access but does not dictate personal consumption decisions. Likewise, regional digital skills levels may indicate a digitally advanced community but do not directly shape an individual’s consumption inequality unless they adopt digital skills. Weak instrument tests and overidentification tests further confirm their validity, with high F-statistics (626.11 and 230.65) indicating strong first-stage relevance and no overidentification bias. These results reinforce the robustness of the approach in mitigating endogeneity concerns.
The endogeneity test results are shown in Table 4. Columns (1) and (3) display the one-stage regression results, with F-values exceeding the critical value of 16.38 at the 10% bias level. The positive and significant coefficients for digital skills application validate the instrumental variables. Columns (2) and (4) present the two-stage regression results, indicating that digital skills application continues to have a significantly positive impact on consumption inequality, with improved coefficients of 0.0875 and 0.2060. These results confirm the model’s robustness and effectively address endogeneity concerns.
5.4 Results of dimensional regression
To assess the impact of digital skills application on various types of farmers’ consumption inequality, this study uses subsistence, development, and enjoyment-type consumption inequality as explanatory variables. The sub-dimensional regression results are presented in Table 5. The estimated coefficients of digital skills application for subsistence, development, and enjoyment-type consumption inequality are all negative and significant at the 1% level, with values of −0.0621, −0.0570, and −0.1166, respectively. These findings indicate that digital skills application helps reduce consumption inequality across different categories. The effect is particularly strong for subsistence and enjoyment-type consumption, suggesting that digital skills are especially effective in reducing inequality related to basic needs and leisure activities. Subsistence consumption involves essential goods and services that meet basic life needs. Digital skills make acquiring these necessities more convenient and efficient by reducing transaction costs, thereby helping to reduce inequality in subsistence consumption. Enjoyment consumption, which focuses on material satisfaction and personal enjoyment, also benefits from digital skills. Online platforms for travel booking, digital entertainment, and other services provide more options and convenience, making enjoyment consumption more accessible and helping to reduce inequality in this area. In contrast, developmental consumption involves long-term investments in education, training, and health, which are often influenced by factors such as resource availability, education level, and income. Due to these complex constraints, relying solely on digital skills is not sufficient to reduce inequality in developmental consumption.
5.5 Mechanism analysis
5.5.1 Mobility constraints
Table 6 presents the results of testing the mediating effect of mobility constraints on how digital skills application influences farmers’ consumption inequality. Column (1) shows that the estimated coefficient of digital skills application is −0.2564, which is significantly positive at the 1% level, indicating that digital skills help alleviate farmers’ mobility constraints. In column (2), the estimated coefficient of digital skills application is −0.0575, while the coefficient of mobility constraints is 0.0158, both significant at the 1% level. These results suggest that mobility constraints partially mediate the relationship between digital skills application and farmers’ consumption inequality. Therefore, hypothesis 2 is confirmed.
5.5.2 Non-farm employment
Table 6 presents the results of testing the mediating effect of non-farm employment on the relationship between digital skills application and farmers’ consumption inequality. Column (3) shows that the estimated coefficient of digital skills application is 0.4296, which is significantly positive at the 1% level, indicating that digital skills promote non-farm employment among farmers. Column (4) shows that the coefficient of digital skills application is −0.0487 and the coefficient of non-farm employment is −0.0587, both significant at the 1% level. These findings suggest that non-farm employment partially mediates the effect of digital skills application on farmers’ consumption inequality. Therefore, hypothesis 3 is confirmed.
5.5.3 Information access
Table 6 reports the results of testing the mediating effect of information access on the relationship between digital skills application and farmers’ consumption inequality. Column (5) shows that the estimated coefficient of digital skills application is 0.2449, which is significantly positive at the 1% level, indicating that digital skills enhance farmers’ access to information. Column (6) shows that the coefficient of digital skills application is −0.0537, while the coefficient of information access is −0.0037, both significant at the 1% level. These findings suggest that information access partially mediates the effect of digital skills application on farmers’ consumption inequality. Therefore, hypothesis 4 is confirmed.
5.6 Heterogeneity analysis
5.6.1 Regional heterogeneity analysis of the impact of digital skills application on farmers’ consumption inequality
The analysis of farmers’ consumption inequality reveals regional differences across China. The inequality indexes for the eastern, central, western, and northeastern regions are 0.4018, 0.4250, 0.4258, and 0.4137, respectively, while the southern and northern regions show indexes of 0.4112 and 0.4208. To explore the regional heterogeneity of the impact of digital skills application on consumption inequality, farmers were grouped into these regions, and group regression analyses were conducted. The regression results are detailed in Table 7. Columns (1) to (4) of Table 7 show the results for the East, Central, West, and Northeast regions. Digital skills application significantly mitigates consumption inequality in all regions, with coefficients of −0.0488, −0.0582, −0.0694, and −0.0548, respectively, all significant at the 1% level. The effects are more pronounced in the Midwest and Northeast, while the impact is relatively weaker in the East. Columns (5) and (6) display the results for the Southern and Northern regions. The coefficients are −0.0477 for the South and −0.0725 for the North, both significant at the 1% level. The stronger impact of digital skills in the central, western, and northeastern regions compared to the eastern region can be explained by key differences in digital infrastructure, economic development, employment structures, and income disparities.
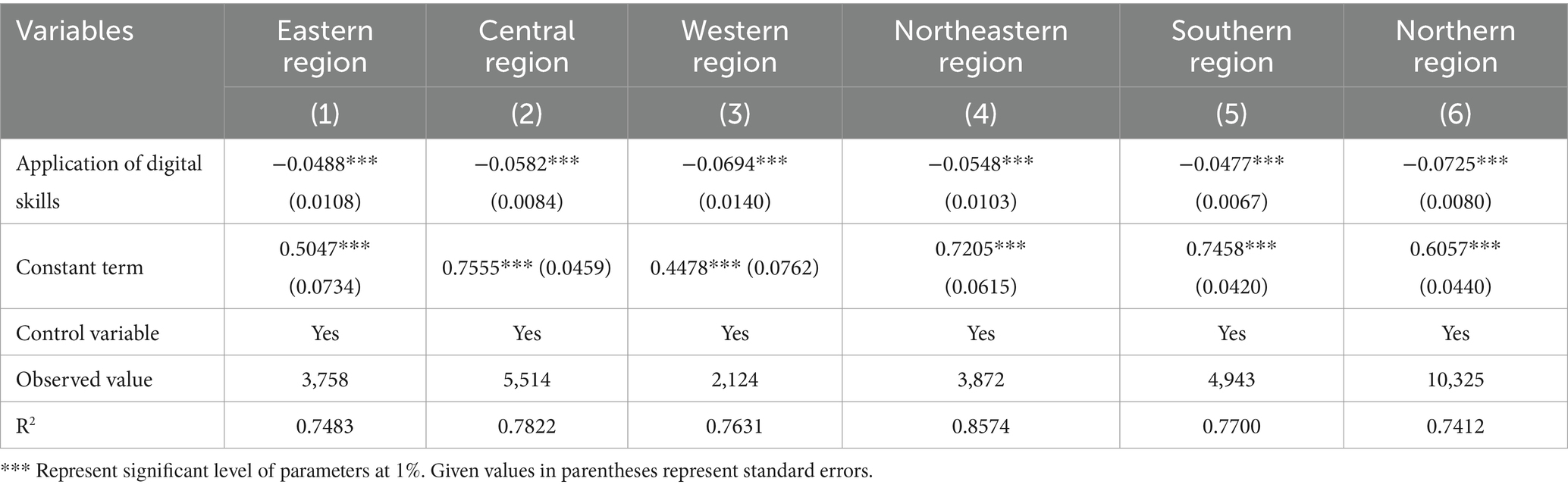
Table 7. Heterogeneity analysis: impact of digital skills application on farmers’ consumption inequality.
First, digital infrastructure gaps are more evident in the central, western, and northeastern regions, where internet penetration, broadband access, and digital service availability have historically lagged behind the eastern region. This means that when farmers in these regions develop digital skills, they experience a greater marginal benefit, as they gain access to previously unavailable online services, markets, and financial tools. Second, economic development in these regions is more dependent on agriculture, with fewer industrial and service-sector jobs compared to the highly urbanized eastern region. Digital skills allow farmers in these areas to diversify their income sources through e-commerce, digital financial inclusion, and non-farm employment opportunities, thereby increasing their consumption capacity and reducing consumption inequality. Third, income disparities play a critical role. In the eastern region, where rural households generally have higher incomes and better financial access, the additional benefits of digital skills on consumption equality are relatively lower. However, in the lower-income rural areas of the central, western, and northeastern regions, digital skills provide a crucial tool for economic mobility, allowing farmers to overcome barriers to employment, financial services, and consumption. Finally, government policies have played an active role in promoting digital adoption in underdeveloped regions. The Chinese government’s “Digital Rural Revitalization” strategy has focused on improving digital access and literacy in lagging areas, providing subsidized training and expanding rural broadband coverage. These initiatives have amplified the impact of digital skills in less developed regions, further contributing to the observed regional heterogeneity in consumption inequality reduction.
5.6.2 Age-based differences in the impact of digital skills on farmers’ consumption inequality
The analysis of farmers’ consumption inequality reveals significant differences across age groups. The consumption inequality index is 0.3078 for farmers aged 40 and below, 0.3942 for those aged 40–60, and 0.5310 for those aged 60 and above. To investigate the impact of digital skills application on consumption inequality among these age groups, regression analyses were conducted, with results presented in Table 8. Columns (1) to (3) in Table 8 show the regression results for the three age categories. The estimated coefficients for digital skills application on consumption inequality are −0.0520, −0.0659, and −0.0981 for farmers aged 40 and below, 40–60, and 60 and above, respectively, all significant at the 1% level. These results indicate that digital skills significantly reduce consumption inequality for all age groups, with the effect becoming more pronounced as age increases. The stronger effect of digital skills among older farmers can be attributed to their initial disadvantages in accessing and utilizing digital tools due to technological barriers. However, once equipped with digital skills, these farmers can overcome challenges such as limited access to market information and narrow sales channels, leading to improved economic outcomes and consumption capacity. Additionally, digital skills help older farmers adopt modern consumption practices, further reducing inequality. The application of digital skills effectively mitigates consumption inequality across all age groups while narrowing the disparity in consumption inequality between younger and older farmers.
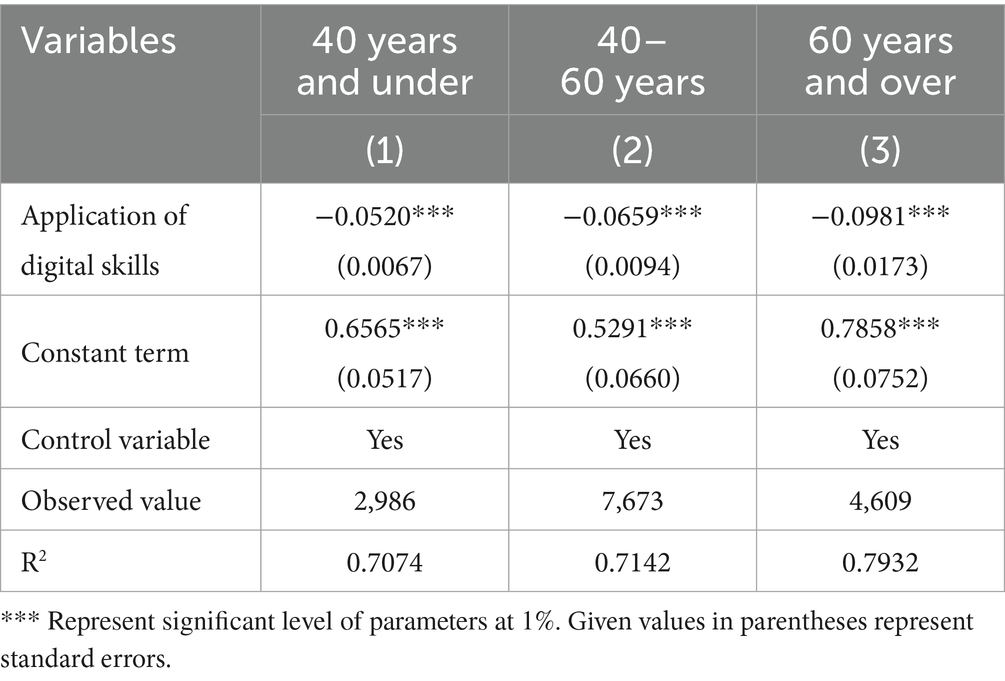
Table 8. Age heterogeneity analysis of the impact of digital skills application on farmers’ consumption inequality.
6 Conclusion and policy implications
Achieving sustainable food systems requires addressing social and economic disparities that hinder equitable development, particularly in rural areas where access to digital technologies remains uneven. Digital skills, as a critical component of technological transformation, can play a significant role in enhancing consumption equity and promoting sustainable livelihoods among rural populations. This study provides valuable insights into how digital skills application influences consumption inequality among rural farmers in China, emphasizing the potential of digital transformation to enhance inclusive economic growth and social equity. The findings indicate that digital skills significantly reduce consumption inequality, particularly in developmental and enjoyment-related categories, through mechanisms such as alleviating mobility constraints, enhancing access to non-farm employment opportunities, and improving information accessibility. Notably, the effects are more pronounced among older farmers and those residing in the central, western, northeastern, and northern regions, suggesting regional and demographic disparities that warrant targeted interventions. These results align with existing evidence from other developing economies, where digital adoption has been shown to improve financial access, expenditure efficiency, and employment transitions (Sant’Ana et al., 2021; Vyas and Jain, 2021).
Despite these positive findings, several challenges persist, including inadequate digital infrastructure, low literacy levels, financial barriers, and limited access to technological resources. Addressing these issues requires tailored training programs, simplified tools, and strengthened institutional support to enhance digital literacy and promote equitable access to digital resources. Expanding digital infrastructure, particularly in underserved regions, and integrating digital skills training into agricultural extension programs can further contribute to narrowing consumption disparities.
Future research should explore broader social and cultural factors influencing digital adoption and assess the long-term impacts of digital skills on intergenerational inequality. Comparative studies across different countries would also offer valuable insights into how digital transformation can be leveraged to promote inclusive development and enhance consumption equity on a global scale.
Data availability statement
The raw data supporting the conclusions of this article will be made available by the authors, without undue reservation.
Author contributions
XL: Writing – original draft, Data curation, Formal analysis, Software. UN: Validation, Writing – original draft. ZK: Writing – review & editing, Writing – original draft, Conceptualization. XZ: Data curation, Methodology, Writing – review & editing. MG: Formal analysis, Resources, Visualization, Writing – review & editing. MS: Formal analysis, Methodology, Resources, Writing – review & editing. MA: Conceptualization, Software, Supervision, Writing – original draft, Writing – review & editing.
Funding
The author(s) declare that no financial support was received for the research and/or publication of this article.
Conflict of interest
The authors declare that the research was conducted in the absence of any commercial or financial relationships that could be construed as a potential conflict of interest.
Generative AI statement
The authors declare that Gen AI was used in the creation of this manuscript. The AI was only used to correct the language of the manuscript.
Publisher’s note
All claims expressed in this article are solely those of the authors and do not necessarily represent those of their affiliated organizations, or those of the publisher, the editors and the reviewers. Any product that may be evaluated in this article, or claim that may be made by its manufacturer, is not guaranteed or endorsed by the publisher.
References
Atalay, K., and Edwards, R. (2022). House prices, housing wealth and financial well-being. J. Urban Econ. 129:103438. doi: 10.1016/j.jue.2022.103438
Attanasio, O., and Pistaferri, L. (2014). Consumption inequality over the last half century: some evidence using the new PSID consumption measure. Am. Econ. Rev. 104, 122–126. doi: 10.1257/aer.104.5.122
Aziz, A., and Naima, U. (2021). Rethinking digital financial inclusion: evidence from Bangladesh. Technol. Soc. 64:101509. doi: 10.1016/j.techsoc.2020.101509
Beheshti, M., ZareRavasan, A., Mahdiraji, H. A., Jafari-Sadeghi, V., and Sakka, G. (2024). An overview of the consumer-centric disruptive technology research: insights from topic modelling and literature review. J. Consum. Behav. 23, 372–388. doi: 10.1002/cb.2204
Chen, Z., Li, X., Zhang, J., and Xia, X. (2024). Does digital finance alleviate household consumption inequality? Evidence from China. Finance Res. Lett. 60:104844. doi: 10.1016/j.frl.2023.104844
Cheng, C., Gao, Q., Ju, K., and Ma, Y. (2024). How digital skills affect farmers’ agricultural entrepreneurship? An explanation from factor availability. J. Innov. Knowl. 9:100477. doi: 10.1016/j.jik.2024.100477
Cheng, P., Wu, S., and Xiao, J. (2025). Exploring the impact of entrepreneurial orientation and market orientation on entrepreneurial performance in the context of environmental uncertainty. Sci. Rep. 15:1913. doi: 10.1038/s41598-025-86344-w
Christelis, D., Georgarakos, D., Jappelli, T., Pistaferri, L., and Van Rooij, M. (2019). Asymmetric consumption effects of transitory income shocks. Econ. J. 129, 2322–2341. doi: 10.1093/ej/uez013
Correa, T. (2016). Digital skills and social media use: how internet skills are related to different types of Facebook use among ‘digital natives’. Inf. Commun. Soc. 19, 1095–1107. doi: 10.1080/1369118X.2015.1084023
Courtois, P., and Subervie, J. (2015). Farmer bargaining power and market information services. Am. J. Agric. Econ. 97, 953–977. doi: 10.1093/ajae/aau051
Cruz-Jesus, F., Oliveira, T., Bacao, F., and Irani, Z. (2017). Assessing the pattern between economic and digital development of countries. Inf. Syst. Front. 19, 835–854. doi: 10.1007/s10796-016-9634-1
Ertz, M., Durif, F., and Arcand, M. (2019). A conceptual perspective on collaborative consumption. AMS Rev. 9, 27–41. doi: 10.1007/s13162-018-0121-3
Espinosa Zárate, Z., Camilli Trujillo, C., and Plaza-de-la-Hoz, J. (2023). Digitalization in vulnerable populations: a systematic review in Latin America. Soc. Indic. Res. 170, 1183–1207. doi: 10.1007/s11205-023-03239-x
Fabregas, R., Kremer, M., and Schilbach, F. (2019). Realizing the potential of digital development: the case of agricultural advice. Science 366:eaay3038. doi: 10.1126/science.aay3038
French, D., McKillop, D., and Stewart, E. (2021). “The effectiveness of smartphone apps in improving financial capability” in Financial literacy and responsible finance in the FinTech era (London: Routledge), 6–22.
Gamede, N. P. (2022). Impact of digitalisation on socio-economic development in emerging economies. South Africa: University of Johannesburg.
Guo, D., Li, L., Qiao, L., and Qi, F. (2023). Digital economy and consumption upgrading: scale effect or structure effect? Econ. Chang. Restruct. 56, 4713–4744. doi: 10.1007/s10644-023-09571-z
Hamari, J., Sjöklint, M., and Ukkonen, A. (2016). The sharing economy: why people participate in collaborative consumption. J. Assoc. Inf. Sci. Technol. 67, 2047–2059. doi: 10.1002/asi.23552
Han, J. H., and Kim, H.-M. (2019). The role of information technology use for increasing consumer informedness in cross-border electronic commerce: An empirical study. Electron. Commer. Res. Appl. 34:100826. doi: 10.1016/j.elerap.2019.100826
Holm, M. B. (2018). Consumption with liquidity constraints: An analytical characterization. Econ. Lett. 167, 40–42. doi: 10.1016/j.econlet.2018.03.004
Huang, Q., Zheng, X., and Wang, R. (2022). The impact of the accessibility of transportation infrastructure on the non-farm employment choices of rural laborers: empirical analysis based on China’s micro data. Land 11:896. doi: 10.3390/land11060896
Hussain, S., Gupta, S., and Bhardwaj, S. (2024). Determinants inhibiting digital payment system adoption: an Indian perspective. Qualit. Res. Finan. Mark. [Ahead of Print]. doi: 10.1108/QRFM-09-2023-0223
Imamov, M., and Semenikhina, N. (2021). The impact of the digital revolution on the global economy. Linguist. Culture Rev. 5, 968–987. doi: 10.21744/lingcure.v5nS4.1775
Ji, C., Chen, Q., and Zhuo, N. (2020). Enhancing consumer trust in short food supply chains: the case evidence from three agricultural e-commerce companies in China. J. Agribus. Dev. Emerg. Econ. 10, 103–116. doi: 10.1108/JADEE-12-2018-0180
Jiao, L., Lu, S., Chen, C., and Feng, Y. (2024). Social security, relative deprivation, digital capacity, and household financial investment behavior. Int. Rev. Financ. Anal. 96:103771. doi: 10.1016/j.irfa.2024.103771
Kong, T., Sun, R., Sun, G., and Song, Y. (2022). Effects of digital finance on green innovation considering information asymmetry: An empirical study based on Chinese listed firms. Emerg. Mark. Financ. Trade 58, 4399–4411. doi: 10.1080/1540496X.2022.2083953
Kumar, P., Pillai, R., Kumar, N., and Tabash, M. I. (2023). The interplay of skills, digital financial literacy, capability, and autonomy in financial decision making and well-being. Borsa Istanbul Rev. 23, 169–183. doi: 10.1016/j.bir.2022.09.012
Li, C., and Chen, F. (2024). Impacts of ICT-related factors on students’ digital reading literacy: evidence from high-performing Asian countries and regions. Educ. Inf. Technol. 29, 16717–16747. doi: 10.1007/s10639-024-12501-9
Li, G., and Qin, J. (2022). Income effect of rural E-commerce: empirical evidence from Taobao villages in China. J. Rural. Stud. 96, 129–140. doi: 10.1016/j.jrurstud.2022.10.019
Li, G., Zhang, R., Feng, S., and Wang, Y. (2022). Digital finance and sustainable development: evidence from environmental inequality in China. Bus. Strateg. Environ. 31, 3574–3594. doi: 10.1002/bse.3105
Lin, B., and Ma, R. (2022). How does digital finance influence green technology innovation in China? Evidence from the financing constraints perspective. J. Environ. Manag. 320:115833. doi: 10.1016/j.jenvman.2022.115833
Liu, Y., and Zhou, Y. (2021). Reflections on China's food security and land use policy under rapid urbanization. Land Use Policy 109:105699. doi: 10.1016/j.landusepol.2021.105699
Liu, Y., Zhou, M., and Li, Q. (2024). How penetration of e-commerce in rural areas drives consumption in China. China Agric. Econ. Rev. 17, 339–357. doi: 10.1108/CAER-01-2024-0016
Luo, J., and Li, B.-Z. (2022). Impact of digital financial inclusion on consumption inequality in China. Soc. Indic. Res. 163, 529–553. doi: 10.1007/s11205-022-02909-6
Luo, J., Zhao, C., Chen, Q., and Li, G. (2022). Using deep belief network to construct the agricultural information system based on internet of things. J. Supercomput. 78, 379–405. doi: 10.1007/s11227-021-03898-y
Meyer, B. D., and Sullivan, J. X. (2011). Further results on measuring the well-being of the poor using income and consumption. Canad. J. Econ. 44, 52–87. doi: 10.1111/j.1540-5982.2010.01623.x
Nguyen, D. H., de Leeuw, S., and Dullaert, W. E. (2018). Consumer behaviour and order fulfilment in online retailing: a systematic review. Int. J. Manag. Rev. 20, 255–276. doi: 10.1111/ijmr.12129
Nie, P., Ma, W., and Sousa-Poza, A. (2021). The relationship between smartphone use and subjective well-being in rural China. Electron. Commer. Res. 21, 983–1009. doi: 10.1007/s10660-020-09397-1
Njuguna, E., Daum, T., Birner, R., and Mburu, J. (2025). Silicon Savannah and smallholder farming: how can digitalization contribute to sustainable agricultural transformation in Africa? Agric. Syst. 222:104180. doi: 10.1016/j.agsy.2024.104180
Palese, B., and Usai, A. (2018). The relative importance of service quality dimensions in E-commerce experiences. Int. J. Inf. Manag. 40, 132–140. doi: 10.1016/j.ijinfomgt.2018.02.001
Purcărea, T., Ioan-Franc, V., Ionescu, Ş.-A., Purcărea, I. M., Purcărea, V. L., Purcărea, I., et al. (2022). Major shifts in sustainable consumer behavior in Romania and retailers’ priorities in agilely adapting to it. Sustain. For. 14:1627. doi: 10.3390/su14031627
Reardon, T., Taylor, J. E., Stamoulis, K., Lanjouw, P., and Balisacan, A. (2000). Effects of non-farm employment on rural income inequality in developing countries: an investment perspective. J. Agric. Econ. 51, 266–288. doi: 10.1111/j.1477-9552.2000.tb01228.x
Reichlin, P. (2020). Social welfare, parental altruism, and inequality. J. Public Econ. Theory 22, 1391–1419. doi: 10.1111/jpet.12429
Rosário, A., and Raimundo, R. (2021). Consumer marketing strategy and E-commerce in the last decade: a literature review. J. Theor. Appl. Electron. Commer. Res. 16, 3003–3024. doi: 10.3390/jtaer16070164
Sant’Ana, D. A., Pache, M. C. B., Borges, P. P., and Dias, J. L. E. (2021). Accessibility and digital inclusion in Brazil and South Korea: a comparison between micro and macro territorial approach. Sustain. Cities Soc. 64:102524. doi: 10.1016/j.scs.2020.102524
Tan, Y., and Li, X. (2022). The impact of internet on entrepreneurship. Int. Rev. Econ. Finance 77, 135–142. doi: 10.1016/j.iref.2021.09.016
Tim, Y., Cui, L., and Sheng, Z. (2021). Digital resilience: how rural communities leapfrogged into sustainable development. Inf. Syst. J. 31, 323–345. doi: 10.1111/isj.12312
Vatsa, P., Li, J., Luu, P. Q., and Botero-R, J. C. (2023). Internet use and consumption diversity: evidence from rural China. Rev. Dev. Econ. 27, 1287–1308. doi: 10.1111/rode.12935
Vyas, V., and Jain, P. (2021). Role of digital economy and technology adoption for financial inclusion in India. Indian Growth Dev. Rev. 14, 302–324. doi: 10.1108/IGDR-01-2020-0009
Walker, C. (2019). The white-collar hustle: Academic writing & the Kenyan digital labour economy. United Kingdom: University of Oxford.
Wang, S., Li, C., Wang, Z., and Sun, G. (2023). Digital skills and household financial asset allocation. Financ. Res. Lett. 58:104566. doi: 10.1016/j.frl.2023.104566
Wang, J., Xu, H., and Wang, M. (2025). Technology diffusion considering technological progress and multiple policy combinations based on evolutionary game theory. Technol. Soc. 81:102799. doi: 10.1016/j.techsoc.2024.102799
Wilmers, N. (2017). Does consumer demand reproduce inequality? High-income consumers, vertical differentiation, and the wage structure. Am. J. Sociol. 123, 178–231. doi: 10.1086/692063
Yang, H., Zheng, Z., and Sun, C. (2022). E-commerce marketing optimization of agricultural products based on deep learning and data mining. Comput. Intell. Neurosci. 2022:6564014. doi: 10.1155/2022/6564014
Yi, S., Qi, Y., Ya, Y., Shi, J., and Cui, Y. (2024). The impact of China’s digital inclusive financial development gap on the optimization of rural consumption structure. PLoS One 19:e0308412. doi: 10.1371/journal.pone.0308412
Yu, M., Chen, Y., Yi, Z., Wang, Q., and Zhang, Z. (2022). Benefits of market information and professional advice in a vertical agricultural supply chain: the role of government provision. Int. J. Prod. Res. 60, 3461–3475. doi: 10.1080/00207543.2021.1924409
Yu, C., Jia, N., Li, W., and Wu, R. (2022). Digital inclusive finance and rural consumption structure–evidence from Peking University digital inclusive financial index and China household finance survey. China Agric. Econ. Rev. 14, 165–183. doi: 10.1108/CAER-10-2020-0255
Zhan, Y., and Yang, S. (2024). Does internet use improve employment? Empirical evidence from China. PLoS One 19:e0301465. doi: 10.1371/journal.pone.0301465
Zhang, S., Li, X., Zhang, C., Luo, J., Cheng, C., and Ge, W. (2023a). Measurement of factor mismatch in industrial enterprises with labor skills heterogeneity. J. Bus. Res. 158:113643.
Zhang, Y., Ma, G., Tian, Y., and Dong, Q. (2023). Nonlinear effect of digital economy on urban–rural consumption gap: evidence from a dynamic panel threshold analysis. Sustain. For. 15:6880. doi: 10.3390/su15086880
Zhang, S., Zhang, C., Su, Z., Zhu, M., and Ren, H. (2023b). New structural economic growth model and labor income share. J. Bus. Res. 160:113644. doi: 10.1016/j.jbusres.2023.113644
Zhao, T., Chen, K., Wang, Q., and Luo, C. (2023). Financial literacy, liquidity constraits and household risk asset allocation. Financ. Res. Lett. 58:104555. doi: 10.1016/j.frl.2023.104555
Zhao, S., Guan, Y., Zhou, H., and Hu, F. (2024a). Making digital technology innovation happen: the role of the CEO's information technology backgrounds. Econ. Model. 140:106866. doi: 10.1016/j.econmod.2024.106866
Zhao, S., Zhang, L., An, H., Peng, L., Zhou, H., and Hu, F. (2023). Has China's low-carbon strategy pushed forward the digital transformation of manufacturing enterprises? Evidence from the low-carbon city pilot policy. Environ. Impact Assess. Rev. 102:107184. doi: 10.1016/j.eiar.2023.107184
Zhao, S., Zhang, L., Peng, L., Zhou, H., and Hu, F. (2024b). Enterprise pollution reduction through digital transformation? Evidence from Chinese manufacturing enterprises. Technol. Soc. 77:102520. doi: 10.1016/j.techsoc.2024.102520
Keywords: digital skills, food systems, farmer consumption inequality, rural development, China
Citation: Liu X, Nisar U, Khalid Z, Zhang X, Guo M, She M and Ahmad M (2025) Digital skills and farmers’ consumption inequality: mechanisms, regional insights, and policy implications. Front. Sustain. Food Syst. 9:1562816. doi: 10.3389/fsufs.2025.1562816
Edited by:
Amar Razzaq, Huanggang Normal University, ChinaReviewed by:
Nand Kumar, Delhi Technological University, IndiaMuhammad Azmat, National University of Sciences and Technology (NUST), Pakistan
Copyright © 2025 Liu, Nisar, Khalid, Zhang, Guo, She and Ahmad. This is an open-access article distributed under the terms of the Creative Commons Attribution License (CC BY). The use, distribution or reproduction in other forums is permitted, provided the original author(s) and the copyright owner(s) are credited and that the original publication in this journal is cited, in accordance with accepted academic practice. No use, distribution or reproduction is permitted which does not comply with these terms.
*Correspondence: Ubair Nisar, dWJhaXIubmlzYXJAZ21haWwuY29t; Zainab Khalid, emFpbmFia2hhbGlkQHNldS5lZHUuY24=