- 1Centre de Recerca en Economia i Desenvolupament Agroalimentari (CREDA-IRTA), Castelldefels, Spain
- 2Universitat Politecnica de Catalunya (UPC), Barcelona, Spain
- 3University of Barcelona, Barcelona, Spain
- 4National Institute of Agronomy of Tunisia (INAT) – University of Carthage, Carthage, Tunisia
- 5Rural Economy Laboratory (LER), National Institute of Agronomic Research of Tunisia, University of Carthage, Carthage, Tunisia
- 6Higher School of Agriculture of Mograne (ESAM) – University of Carthage, Carthage, Tunisia
- 7Department of Economics, Ünye Faculty of Economics and Administrative Sciences, Ordu University, Ordu, Türkiye
- 8Aragon Agrifood Research and Technology Center (CITA), Zaragoza, Spain
In 2022, the EU coordinated action “From the Hives” (Honey 2021–2022) revealed that 46% of imported honey in the EU did not comply with the “Honey Directive” and is suspected of adulteration. Nowadays the blockchain ecosystem is a digital technology that could be used to trace honey, assisting in fraud reduction and ensuring the authenticity of the botanical and geographical origin of the honey. Nevertheless, its implementation is limited by stakeholders’ acceptance, particularly beekeepers’ decisions. With the aim of identify principal factor affecting beekeepers blockchain adoption, this study applied two theoretical models at the farm level, related to technology adoption: The Theory of Planned Behavior (TPB) and the Technology Adoption Model (TAM). In both models, an additional construct identified as digital maturity was included, analysing their impact on the intention to adopt blockchain through the PLS-SEM method. General findings show that all constructs of both extended models influence the intention to adopt blockchain. Especially, the extended TAM model showed better results than extended TPB according to the explained variance (64.0 and 62.0% respectively). In these models, the perceived behavioral control of TPB and the usefulness perception of TAM were the major predictors of intention to adopt blockchain. Moreover, the digital maturity of farmers was a pivotal factor in enhancing the adoption of such innovations. The findings indicated that implementing blockchain necessitates the provision of training and resources to enhance beekeepers’ competencies, thereby augmenting their trust and proficiency in utilizing digital technologies. Furthermore, the creation of user-friendly interfaces is critical for acceptance, emphasizing the importance of a collaborative process between blockchain designers and beekeepers.
1 Introduction
Honey is widely recognized as a highly valuable and superior natural food produced and consumed globally (Khaoula et al., 2019; Edo et al., 2023). However, honey can be adulterated with cheap syrups, resins, and hive feeding, altering its color, origin, and composition (Marín et al., 2022). According to the Anti-Fraud Office from the European Commission within the EU coordinated action “From the Hives” (Honey 2021–2022), 46% of imported honey in the EU in 2022 was suspected of adulteration, making effective detection challenging. This indicates that honey adulteration is a widespread problem that affects the authenticity and quality of honey in Spain and other EU countries. Fraud can occur through incorrect labeling or intentional adulteration (Soares et al., 2017; Hall, 2023). Similarly, in Tunisia, honey adulteration is a growing concern. A 2022 study in Greater Tunis highlighted increasing consumer awareness of honey adulteration issues, which involve adding cheap sugars or syrups to inflate volume and reduce purity and quality (Jribi et al., 2022). Adulterating honey with sugar syrups offers a cost-effective advantage to fraudsters, leading to unfair competition. Most fraudulent honey entering the EU comes from countries like China and the United Kingdom (Ždiniaková et al., 2023). Ensuring honey authenticity is a significant challenge for Mediterranean beekeepers and stakeholders, impacting livelihoods and industry reputation. In order to confront this challenge, beekeepers should develop fraud mitigation strategies that combat honey adulteration and utilize technologies that enable them to guarantee their genuineness (García and Schwarzinger, 2021). Exist various technologies for food traceability, such as blockchain, internet of things (IoT), Radio Frequency Identification (RFID), sensors, wireless devices, and QR codes (Lukacs et al., 2025). The integration of digital technologies improves the food supply chain transparency and traceability management by tracking the product quality, environmental conditions and logistics enhancing efficiency (Verna et al., 2025). Traceability refers to the ability to connect all actors involved in the supply chain, enabling the flow of information to know, track and monitor the products effectively (Engelseth, 2009; Olsen and Borit, 2013). Traceability must encompass every stage of the honey supply chain, including production, processing, and distribution (Danieli and Lazzari, 2022). A track and trace system typically require methods for collecting and storing data, effective communication tools, and, if possible, a certification or trust mechanism to ensure the veracity of the information (Lukacs et al., 2025). Blockchain stands out for its immutable record, decentralization, and ability to guarantee information in real time, enabling transparency, efficiency, and improved customer satisfaction (Shukla et al., 2024). Blockchain strengthens traceability by securing the supply chain, minimizing fraud, and ensuring authenticity thereby enhancing transparency, resilience and food safety and quality standards (Dasaklis et al., 2022; Sri Vigna Hema and Manickavasagan, 2024). Blockchain is considered vital for fostering sustainable business models in the agri-food industry while also serving as a robust solution for enhancing traceability (Paunović and Alizadeh, 2022; Dal-Mas et al., 2023).
An additionally issue in Mediterranean (MED) countries such as Türkiye, Spain, and Tunisia, is the fragmented structure that characterize the beekeeping sector, where numerous small-scale beekeepers operate independently (Baytaroğlu, 2006). This fragmentation presents challenges for the widespread adoption of innovative technologies like blockchain, as the dispersed nature of the sector can make coordinated efforts and cohesive implementation difficult. However, blockchain technology offers a promising strategy to counter these challenges by enhancing competitiveness and reducing fragmentation. By providing a transparent, secure, and decentralized system for tracking honey production and supply chains, blockchain can foster greater collaboration among beekeepers, improve traceability, and increase trust in the authenticity and quality of honey products. Consequently, this can help unify the sector, making it more resilient and efficient, while boosting the overall market competitiveness of honey from these Mediterranean countries. Blockchain is defined as a distributed database shared among a peer-to-peer network, consisting of timestamped transactions secured by public-key cryptography (Sciarelli et al., 2022). Its adoption facilitates transaction monitoring and authentication during data processing and transfer and enables to access detailed product information generated along the value chain to all customers special through QR codes (AlShamsi et al., 2022; Do et al., 2025; Verna et al., 2025). In the agrifood sector, blockchain improves food safety, traceability, quality, transparency, and scalability (Bermeo-Almeida et al., 2018; Dal-Mas et al., 2023; Sharma et al., 2023). It is commonly used to verify the botanical and geographical origin of honey (Danieli and Lazzari, 2022). According to Dehghani et al. (2022) in the food sector one of the most important and crucial things of blockchain is consumer trust. Although this digital technology has inherent features such as decentralization, data integrity, security, and reliability, its rate of adoption is limited (AlShamsi et al., 2022; Danieli and Lazzari, 2022).
Understanding the factors affecting digital technology adoption is crucial for recognizing market opportunities and reducing fraud. Digital technologies enhance economic growth, productivity, and operational efficiency (Bravo et al., 2018). They are increasingly integrated into agriculture and the agrifood supply chain (Aras and Büyüközkan, 2023; Sharma et al., 2023). Blockchain, one of the most innovative technologies, improves transparency, traceability, and security in transactions (Liu and Ye, 2021).
The decision to adopt technology in agriculture is influenced by beliefs, perceptions, attitudes, social norms, skills, and abilities (Räisänen and Tuovinen, 2020; Concu et al., 2020). Studies have analyzed technological innovation adoption in various contexts based on different theoretical frameworks (Ullah, 2020; Räisänen and Tuovinen, 2020; Shang et al., 2021; Gandhi et al., 2023). Some of these theoretical models are the theory of reasoned action (TRA; Fishbein and Ajzen, 1975), the technology acceptance model (TAM; Davis et al., 1989), the theory of planned behavior (TPB; Ajzen, 1991), the unified theory of acceptance and use of technology (UTAUT; Venkatesh et al., 2003), the technology organization environment (TOE; Tornatzky et al., 1990) and the diffusion of innovations theory (DOI; Rogers, 1995). All of them provide empirical evidence on factors influencing the intention to adopt digital technologies as the blockchain (Palos-Sanchez et al., 2019). Two of the most important approaches influencing the digital innovations adoption are (TPB) and (TAM) and have been utilized to examine the adoption of blockchain technology, particularly in context where external factors like perceived benefits are significant (Sciarelli et al., 2022; Gandhi et al., 2023). TAM is better suited for analysing individual adoption behavior through constructs like perceived ease of use and usefulness, while TPB excels at integrating group dynamics and social norms. In contrast, UTAUT is a more complex theoretical model based on TRA, TAM, and TPB (Shukla et al., 2024), it focuses on constructs like performance expectancy, effort expectancy, social influence, and facilitating conditions. However, it is often applied in large-scale organizational context, which may not fully address the individual and small-group characteristics of beekeepers (Dwivedi et al., 2019).
Digital maturity influences the acceptance of digital advancements, making adoption easier (Bravo et al., 2018; Ladu et al., 2024). It involves indicators demonstrating an organization’s capacity to adapt and leverage digital tools. Agricultural companies with higher digital maturity experience enhanced efficiency and lower costs (Hansen et al., 2022). They are more prepared to adopt new technologies due to advanced capabilities and positive attitudes toward technology. Moreover, their robust and advanced technological infrastructure facilitates the integration of new tools and systems, and their data management focuses on effective information flow management to collect, process, and distribute data (Teichert, 2019).
The literature review highlights a scarcity of studies focused on investigating the adoption of blockchain in the agri-food sector, therefore, the main this study aims to identify the factors affecting the beekeeper’s intention to adopt the digital technology, specifically blockchain, as a traceability system for their honey in three Mediterranean countries (Spain, Türkiye, and Tunisia). This is achieved by extending the TAM and TPB theories using the Digital maturity construct. Further, this paper contributes to the literature by addressing current gaps on the use of theoretical models such as TPB and TAM to identify the factors influencing the adoption of emerging technologies in beekeeping. This can help to identify not only beekeepers’ attitudes and perceptions but also how these translate into actual behaviors. From an interdisciplinary perspective, this article can enrich the understanding of blockchain applications across different domains, combining knowledge from fields such as apiculture, technology, and supply chain management. Moreover, the inclusion of beekeepers’ digital maturity provides a more detailed vision of how the level of digitalization affects the willingness to adopt innovative technologies. This insight could be useful in designing strategies for the potential adoption of blockchain in beekeeping activities. Finally, the study of blockchain application in the agricultural sectors like the beekeeping niche provides new insights and broadens the scope of blockchain technology studies. The remainder of this paper is organized into six sections as follows. Section 2 presents the theorical framework and research hypotheses. Section 3 contains a broad exposition of the used methodology. In Section 4 is the exposition of the results. Section 5, corresponds to the discussion of the results, followed by the implications of the findings on Section 6, and finally in section 7 the conclusions of this study are presented.
2 Theoretical framework and research hypotheses
To comprehensively understand the factors influencing technology adoption, it is essential to explore three key theoretical frameworks: the Theory of Planned Behavior (TPB), the Technology Acceptance Model (TAM), and Digital Maturity. Each framework offers unique insights into the behavioral, and organizational dimensions of adopting new technologies. These theoretical frameworks provide a solid foundation for investigating and understanding the factors that affect beekeepers’ intention to adopt blockchain technology for honey traceability, offering detailed insight into the attitudes and perceptions that influence their behaviors.
2.1 Theory of planned behavior
The theory of planned behavior (TPB) is a model that predicts and explains how attitudes and social norms influence behavior. It consists of three underlying factors: attitude, subjective (social) norms, and perceived behavioral control (Figure 1). The TPB is commonly used to foresee the behavioral intentions of individuals (Mohr and Kühl, 2021), considering intention as the determinant of an action or the immediate behavior of a human (Tama et al., 2021). Attitude is defined as “the degree to which an individual evaluates the behavior as positive or negative and attitude can be based on instrumental beliefs and experiential or affective beliefs of performing the behavior” (Ajzen, 1991). It refers to the assessment of behavior as either positive or negative, representing an evaluated inclination toward either performing or not performing the behavior (Concu et al., 2020). The perceived behavioral control construct corresponds to the perception of their self-individual abilities, or self-efficacy toward the behavior. It is related to judgements about how well they can execute the necessary actions to deal with the situation, or the confidence in their ability to perform the behavior. Subject norms refer to the perceived social pressure to perform a behavior, it is related to the approval or disapproval of important others (friends, parents, family members) to perform the given behavior (Ajzen, 1991). TPB explores how attitudes, social norms, and perceived control influence the intention to accomplish an action. Building on this framework, Mahattanakhun and Suvittawat (2023) examined technology acceptance among Thai farmers, focusing on their ability to adapt to changes in agriculture. Their findings align with the TPB’ emphasis on attitudes, revealing that attitude is the most significant factor influencing the intention to use technology. This suggests that a positive attitude toward new technology, particularly those that are not yet widely adopted, greatly impacts the intention to utilize such technology. Regarding the digital technologies in the agrifood sector such as blockchain, the TPB constructs influence their acceptance or intention to adopt, as confirmed by a literature review (Gandhi et al., 2023; Rafiq and Malik, 2024). According to this the hypothesis for this study are:
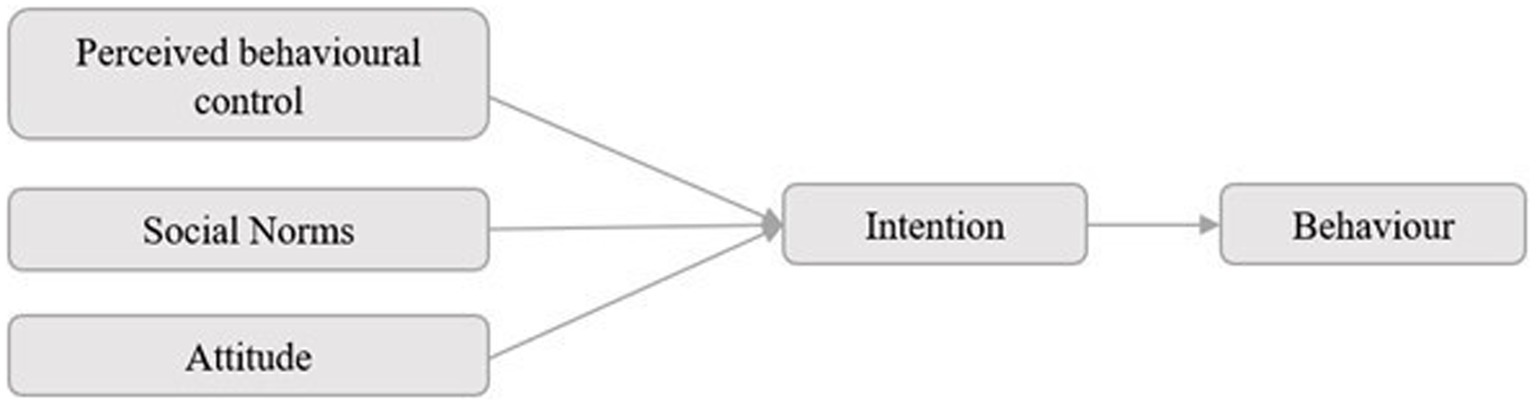
Figure 1. Theory of planned behavior (TPB-Ajzen, 1991).
Hypothesis 1A: Attitude has a positive significant influence (effect) on beekeepers’ intention to use BCT. A more positive attitude toward blockchain will increase the intention to adopt it by beekeepers.
Hypothesis 2A: Perceived behavioral control has a significant and positive impact (effect) on beekeepers’ intentions to use BCT. Beekeepers who feel more in control are more likely to adopt blockchain technology.
Hypothesis 3A: Social Norms has a positive significative influence (effect) on beekeepers’ intention to adopt the digital technology (BCT).
The hypotheses align with previous studies that have utilized TPB to understand the adoption of digital technologies in agriculture. For example, research conducted in Ontario, Canada, examined the adoption of digital technologies “Internet of Things (IoT), sensors, data analytics, artificial intelligence (AI)” in the aquaculture sector by rainbow trout producers (Chowdhury et al., 2024). Another study focused on the acceptance of AI in German agriculture (Mohr and Kühl, 2021). Similarly, studies on BCT adoption in European coffee supply chain (Kramer et al., 2021) and sustainable agricultural practices (Xiang and Guo, 2023; Rafiq and Malik, 2024) further validate the relevance of TPB in this context these examples illustrate the broad applicability of TPB in explaining the digital technologies adoption across diverse agricultural sectors.
2.2 Technology acceptance model (TAM)
The theoretical model of Technology Acceptance (TAM) proposed by Davis (1985) and Davis et al. (1989) explains the factors involve in the innovations’ adoption, and it is widely used to make predictions about the acceptance and use of innovative technologies as blockchain (AlShamsi et al., 2022). This theoretical framework is based on the theory of reasoned action proposed by Ajzen and Fishbein (1980), in which the determinants of conscious behavior are analysed (López-Bonilla and Bonilla, 2006; Rondan et al., 2015). In TAM, there are two fundamental constructs that focus on user perception: (i) perceived usefulness of the technology, and (ii) perceived ease of use, both of which directly influence the behavioral intention, as shown in Figure 2 (Venkatesh and Davis, 1996). The definition of the perceived usefulness corresponds to “the level to which a person believes the use of the specific technology will enhance their performance,” while perceived ease of use, is defined as “the degree to which a person believes that using a particular system would be free of effort” and it is related to the self-capacity to perform the behavior (Davis et al., 1989). Studies on factors influencing the intention to adopt digital technology across the agrifood supply chain using TAM model have demonstrated that perceived usefulness and perceived ease of use play a significant role (Pappa et al., 2018; Saurabh and Dey, 2021; Dong et al., 2022). Furthermore, Caffaro et al. (2020) investigated the factors influencing farmers’ intentions to adopt two categories of Smart Farming Technologies: drones, sensors for data acquisition, and agricultural apps, as well as agricultural robots and autonomous machines. Finding that perceived usefulness significantly influenced farmers’ intentions to adopt these technologies. Building on these findings, the following hypotheses were proposed for this study:
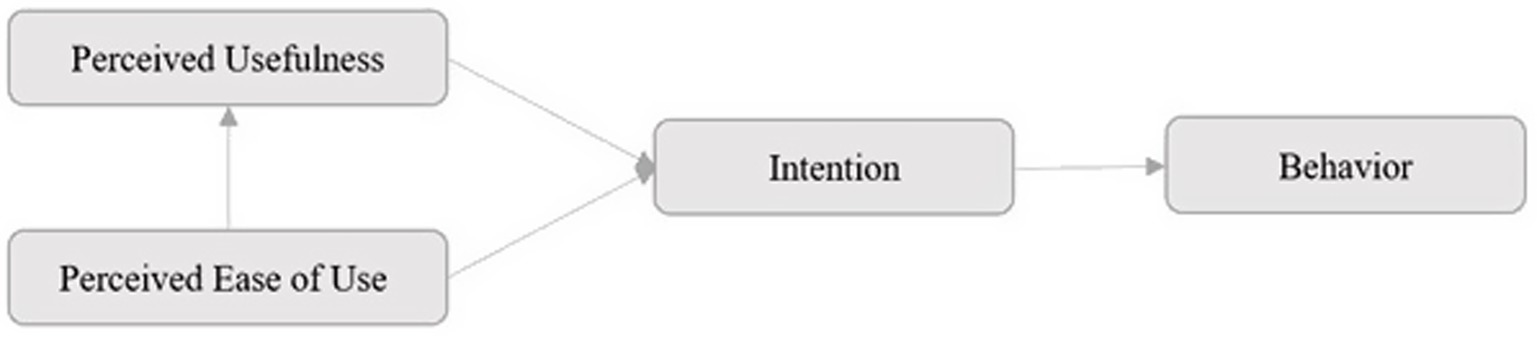
Figure 2. Technology acceptance model (TAM-Davis et al., 1989).
Hypothesis 1B: Perceived ease of use has a significant and positive impact on beekeepers’ intention to use BCT. Beekeepers are more likely to adopt blockchain technology if they perceive it to be easy to use.
Hypothesis 2B: Perceived usefulness has a positive and significant impact on beekeepers’ intentions to adopt BCT. Beekeepers’ willingness to adopt blockchain technology will increase as their perception of its utility grows.
Hypothesis 3B: Perceived ease of use has a significant positive impact on perceived blockchain utility. Beekeepers are more likely to adopt blockchain technology when they perceive it to be simple to use.
2.3 Digital maturity (DM)
Maturity refers to being in perfect condition to achieve a goal, prevent an issue or generate a solution (Nikkhou et al., 2016). A company’s digital maturity level reflects its current state in terms of digital transformation, technological adoption, and strategy (Haryanti et al., 2023). According to Aras and Büyüközkan (2023) digital maturity is closely related to the adoption of digital technology, to have a high level of digital maturity makes the successful adoption of digital technologies easier. Gökalp and Martinez (2021) argue that digital maturity is how integrated are the digital technology into the company activities, based on the capabilities and digital skills of its members, technological infrastructure, and the type of commercial relationships it develops. From Ladu et al. (2024) perspective, digital maturity corresponds to the status of digital transformation in the business, and comprises both technical and managerial aspects, and it involves capabilities, resources, and areas of action. MacPherson et al. (2022) highlight how digitalization, in association with certain institutional, social, and legal factors, enables the achievement of sustainability goals. According Safiullin et al. (2022) in agriculture the digital maturity involves all transformations driven by the integration of digital technologies into production management. Rogers (1995), in his DOI theory, explains how technological innovations are adopted by different groups based on factors such as knowledge, attitude toward innovation, and implementation capabilities. Digital maturity can facilitate this process by equipping individuals with the skills needed to recognize and adopt new technologies (Ostmeier and Strobel, 2022). In this context, both TAM (perceived ease of use and perceived usefulness) and TPB (perceived behavioral control) frameworks play crucial roles in technological adoption. Digital maturity underpins these constructs by enhancing trust and reducing technical barriers, those increase willingness to adopt new technologies. Over the years different digital maturity models have been developed from commercial, industry, and academic perspectives, employing diverse criteria and thematic aspects (Aslanova and Kulichkina, 2020). However there remains a lack of theoretical guidance on such models. To address this gap, in this study integrates the construct of digital maturity within both models and propose the following hypothesis:
Hypothesis 4A and Hypothesis 4B: Digital maturity has a positive significant influence (effect) on beekeepers’ intention to adopt the Blockchain technology (BCT).
Based on the literature review and the research objectives, the structure and the hypotheses for each model to be tested in this study are illustrated in Figure 3 (Extended TPB model) and Figure 4 (Extended TAM model).
3 Research methodology and data collection
To understand the intention of Mediterranean beekeepers to adopt blockchain technology, specifically from Spain, Türkiye, and Tunisia, was developed a survey instrument based on existing literature related to attitudinal and adoption theories. The survey was divided into three main parts: (i) demographic profile questions. (ii) Digital maturity questions and (iii) Specific section for questions related to the constructs, where attitudinal, behavioral, and perception questions were included based on the two theoretical frameworks used, TPB and TAM. Table 1 includes the TPB and TAM constructs, as well as the indicators used in this study to define each construct. Also, the indicators for the digital maturity construct were included in this table. Items from the constructs were measured using a five-point Likert scale (1. Strongly Disagree, 2. Disagree, 3. Neither agree nor disagree, 4. Agree, 5. Strongly agree). This scale was based on the operational definition of these variables as proposed by Ajzen and Fishbein (1980) and was also utilized and validated in the study by Yu et al. (2025) on farmers’ adoption of smart technologies.
Data collection for this study was carried out following a structured approach from November 2023 to March 2024, utilizing both face to face interactions and the Qualtrics platform. The target population consisted of beekeepers from three Mediterranean countries: Spain, Tunisia, and Türkiye. A list of all registered beekeepers the association registers of the regions studied (Zaragoza in Spain, northern and central regions of Tunisia and the Ordu Province in Türkiye) was generated to identify possible participants. The sample method employed was random, ensuring representativeness of the target population, with a recorded response rate of 78%, representing 159 valid responses out of the 203 questionnaires distributed. The survey originally written in English was translated into Spanish, French and Turkish to ensure accessibility for the participants. During the survey process, beekeepers were informed about the study’s purpose, the use, confidentiality, and anonymity of their responses. Specific exclusion criteria, such as beekeepers not dedicated to commercialization and incomplete responses, were applied to maintain the quality and relevance of the data. Furthermore, a pilot test was conducted with beekeepers participating in living labs established in each case study country. This test validated the survey instruments prior to data collection, ensuring their adequacy and reliability. Ethical approval was obtained from the ethics committee of the Center for Agri-food Economics and Development (2023–24).
3.1 Digital maturity
Measuring digital maturity involves evaluating various dimensions that determine the potential for digital transformation. Based on analyses of several digital maturity models, the most commonly examined dimensions include technological, organizational, and strategic factors (Safiullin et al., 2021; Piot-Lepetit et al., 2023). Additionally, Mendes et al. (2022) suggest that the digital maturity of agricultural organizations and their workforce is intrinsically tied to their ability to utilize digital technologies. While alternative measures, such as validated digital maturity indices, exist, the selection of the five indicators (people, data, organization, technology, and strategy) was based on their comprehensive representation of the critical dimensions observed in prior studies. The digital maturity structure for this study was designed using the five indicators that characterize the digital maturity model defined by Aslanova and Kulichkina (2020): “people, data, organization, technology, and management/strategy,” as well as those mentioned by Büyük et al. (2021). The indicators are then described specifically.
• “People” refers to the employee’s personal skills or digital capabilities required for the successful implementation of strategies in the company.
• “Data” refers to the use and management of data generated in the organization; proper data management is a prerequisite for the digitalization of activities.
• “Organization” is a key component of digital maturity, defining how business processes are structured and the organizational skills available.
• “Technology” indicator includes all of the equipment required for digitalization.
• “Strategy” focuses on the integration of specific actions to achieve digital transformation goals while considering the management and optimization of any information flux.
These selected indicators allow for a more specific and context-adjusted assessment of beekeeping activities and are aligned to the more accepted dimensions, which is not always covered in generic indices ensuring the conceptual validity of the approach adopted (Büyük et al., 2021).
Digital maturity indicators were assessed on a scale of 1–5 (1 = no affirmative answers, 2 = one affirmative answer, 3 = two affirmative answers, 4 = three affirmative answers, and 5 = all affirmative answers), based on the questions positively answered by the interviewed beekeepers (see Table 2). After completing the questionnaire, the score of each indicator is obtained, and the digital maturity level can be calculated using the average of all indicators, generating values between 1 and 5. Values from 1 to <=2 correspond to the “beginner” digital maturity level, indicating that the organization is in the first step toward digital maturity. Values (>2 and ≤3) indicate an “intermediate” level for companies with experience implementing digital practices. The values (>3 and =4) indicate “experienced” levels of digital maturity, indicating more professional practices in the digitalization data field within the organization. Values >4 and ≤5 indicate the “experts” level of maturity, where companies adapt their infrastructures and processes to focus on digital and smart solutions (Büyük et al., 2021). This methodology was selected because is a simple, understandable and transparent tool, applicable to different fields.
3.2 Data analysis
The Partial Least Squares-Structural Equation Models (PLS-SEM) were used as a widely used second-generation multivariate technique of analysis, due to their statistical efficiency and high level of confidence (Yaacob et al., 2021; Gandhi et al., 2023). Compared to Covariance-Based SEM (CB-SEM), the PLS-SEM is a more suitable method for evaluating research models, due to its lower requirements regarding measurement models (both reflective and formative), sample sizes, and residual distribution assumptions (Mustapa et al., 2024). SEM models allow us to simultaneously examine a series of dependency relationships between independent and dependent variables, with the aim of reducing data dimensionality and estimating a causality network (Al-Edrus et al., 2023). The PLS-SEM is a flexible Non-parametric model composed of 2 sub models, (i) the measurement model (also identified as outer model) where relations between the indicators and the constructs “latent variables” are analysed, and mathematically expressed as:
Where corresponds to the jth construct, is the path coefficient that relate the qth exogenous construct to the jth endogenous construct and the correspond to the error of the internal relation.
And (ii) the structural model (identified as inner model) where relation between and constructs are analysed, and in a reflexive model is given by the function
Where is the loading of the pth indicator from the qth construct and correspond to the error in the measurement process (González, 2018). The aim of the PLS-SEM is maximizing the amount of explained variance (R2). PLS-SEM has the advantage that it can be used with small samples in complex models (Martínez and Fierro, 2018). Hair et al. (2014) indicate that the minimum sample size should be estimated considering 10 times the number of arrows pointing at a variable.
However, being more conservative, to ensure that the results of the statistical method are solid and generalizable, in a more heterogeneous population the guidelines for a minimum sample size must be followed by using the inverse square root method (Hair et al., 2021). Next equation shows the needed sample size considering a significance level of 5%, and a minimum path coefficient equal to 0.2, with a power level of 80%.
To conduct data analysis in this study the software SmartPLS 4.0 was used (Ringle et al., 2024). In a first step, all indicators of each construct were included in the model, later the indicators with no significative loading were removed from the model. All constructs were structured by reflective indicators, because all of them act as causality from the latent variables according to the rational methodological background considerations (Hanafiah, 2020).
4 Results
4.1 Descriptive statistics
The descriptive analysis of the sample is summarized in Table 3. Demographic results revealed that most beekeepers interviewed were between the ages of 36 and 49, accounting for roughly half of the sample. The sample was dominated by men (87%). In terms of education level, more than one-third of respondents (40%) attended university, followed by beekeepers who completed a second stage of formal education (35%). The number of beekeepers surveyed was balanced by origin country, with each country accounting for approximately 33% of the total sample.
According to the established scale, the group of beekeepers surveyed in the Mediterranean countries (Spain, Türkiye, and Tunisia) for this study had an intermediate level of digital maturity (2.59). This maturity level indicates that beekeepers in this region have basic data analytics capabilities, as well as the ability to identify and understand basic digital tools and technologies; however, they must continue to work on full integration of digital practices across the organization, considering digital devises and innovations, to ensure the greatest impact on the apicultural organization’s development.
4.2 Assessment of the measurement model
The measurement models were analysed first, followed by the structural models. Table 4 presents empirical results from validation models (TPB extended, and TAM extended). All loadings of reflective constructs’ indicators (λ) in each model were higher than 0.70, confirming the individual reliability and indicating that all observable variables are positively correlated and measure correctly the composed theoretical concepts (Carmines and Zeller, 1979; Cardona et al., 2018). The VIF values for the indicators of each construct were verified, with all values found to be below 5, indicating low collinearity as recommended by Hair et al. (2014). Furthermore, correlations between the indicators were examined and found to be below 0.85, confirming that excessive correlation, which could hinder the interpretation of relationships between indicators and constructs, was not present. According to Cronbach’s alpha and the composite reliability coefficients of the constructs (rho_a and rho_c), all of them with values higher than 0.70, the composed reliability of the constructs was validated (González, 2018). The convergent validity test showed values >0.50 as observed through the average variance extracted (AVE) indicating that the constructs explain more than half of the components’ variation (Yaacob et al., 2021). The lowest values were for the digital maturity construct in the TPB model (0.668), and for ease-of-use perception on TAM model (0.647), both higher than the threshold.
The discriminant validity was tested with the Fornell-Larcker criteria, the Heterotrait-Monotrait Ratio, and cross-loadings (Yaacob et al., 2021; Dong et al., 2022). In both models, results proved the validity of the constructs as can be observed in Tables 5–7. In relation to the Fornell-Larcker criterion, results of the square root of AVE for each construct (black diagonal values of Table 5) exceed the correlation (r de Pearson) with the rest of the constructs of the model. As revealed by the matrix of the HTMT ratios (Table 6), all constructs had significative values (lower than 0.85), except for (Usefulness <− > Ease of use = 0.867) and (Usefulness <− > Intention = 0.867), however both were considered valid in a less conservative model, where the maximum level allowed is 0.90, considering that the constructs in the nomogram are not conceptually very different (Hair et al., 2019; Carrera et al., 2022). And for cross loadings (Table 7), all indicators’ loading of each construct were higher than the loadings of the indicators of its related variables.
4.3 Assessment of the structural model
Regarding the structural model, in both cases extended TPB and extended TAM, all the values of the variance inflation factor (VIF) were smaller than 3.33 (Table 8), allowing state that collinearity is not a major problem (Mustapa et al., 2024). Furthermore, based on the one-tailed hypothesis test, the results of the structural model analysis showed that all proposed hypotheses on the basis of the theory, including the extension of the digital maturity construct, were accepted. That means that all exogenous constructs (exogenous latent variables) have an influence on the beekeepers’ intention to adopt the digital technology of blockchain, and all p-values were significant. Specifically, in the extended TPB model, results showed that perceived behavioral control (H3A) had the greatest influence on BCT adoption intention (path coefficient = 0.391), followed by beekeepers’ personal attitude (H1A) (path coefficient = 0.352). While in extended TAM, the usefulness perception of the BCT (H4B) was the construct with more influence on the intention to adopt BCT (path coefficient = 0.506). Although the digital maturity construct demonstrated the lowest significant effect compared to other constructs in the models (as shown in Table 9), it remains a key indicator for assessing the ability to use digital technologies such as blockchain. Additionally, digital maturity may have an indirect influence on other critical relationships within the model related to technology usage. This inclusion is supported by Mendes et al. (2022), who emphasize the importance of digital maturity in determining the capacity to adopt and utilize digital technologies.
The structural model TPB explains the 60.2% of the total variance (R2 = 0.602) of the intention behavior to adopt the BCT (Figure 5). While the structural model TAM (Figure 6) explains the 64.0% of the total variance (R2 = 0.640) of the intention behavior and 51.8% for the usefulness perception (R2 = 0.518). Being the dependent construct enough explained by the independent variables. The results of the composite-based standardized root mean square residual (SRMR) analysis showed values lower than 0.08 in both estimated models and the normed fit index (NIF) values nearest to 1 (in TPB SRMR = 0.066 and NIF = 0.819 and in TAM SRMR = 0.062 and NIF = 0.802), confirming the robustness of the models (Henseler et al., 2015; Hair et al., 2019; Dong et al., 2022). Overall, the above results allow us to argue that extended TAM is a better model to predict the intention to adopt the blockchain by beekeepers in comparison to the extended TPB model. Additionally, contrasting the models with the quality criteria BIC, we confirmed that the TAM model generates a better value for intention than the TPB model (lower BIC), results can be observed in Table 10.
5 Discussion
Drawing on the theoretical frameworks of TPB and TAM, this study proposed and analysed two extended models with the digital maturity construct to identify the determinants of intention to adopt the blockchain traceability system in beekeeping. The research results highlight that beekeepers’ intention to adopt the blockchain is statistically significantly influenced by all constructs included in the models, confirming the initial hypothesis. The level of influence varied depending on the construct. On TPB, perceived control behavior had the greatest influence. This construct indicates that when beekeepers have self-confidence in their ability to manage the situation, they are more interested in adopting the proposed technology, as previously found (Mohr and Kühl, 2021; Mustapa et al., 2024). However, Sok et al. (2020) argue that in empirical studies the real measure about perceived control, related to the self-confidence, is often difficult to estimate, therefore it is frequently considered as an approximation. The second construct that had a greater impact on adoption intention was attitude toward blockchain, similar to the results obtained by Mohr and Kühl (2021) in their study about adoption of artificial intelligence (AI) in German agriculture. Findings made by Gandhi et al. (2023) also showed that attitudes toward blockchain positively affect the intention to adopt this digital technology. According to their findings, attitudes define the emotional aspects of intention, and appropriate emotions and beliefs have a significant positive impact on acceptance or intention to adopt blockchain throughout the agricultural supply chain. Influence of social norms came in third place. Perceived social pressure had a positive effect on individual decisions to adopt blockchain technology. This result was consistent with the study of Noor et al. (2013) and opposite to the findings of Kamble et al. (2018) and Abbas and Mehmood (2021) that indicated in their research that people did not make an effect on the decision to adopt the digital innovation proposed.
Regarding the TAM model, all constructs included in the structural equation had a positive effect on beekeepers’ intention to adopt the blockchain, and according to the magnitude of the constructs, perceived usefulness has the greatest effect on beekeepers’ intention. Previous research has shown that perceived usefulness is a stronger predictor of behavioral intention than perceived ease of use (Venkatesh, 2000; Kumari and Devi, 2023). Further, the findings are consistent with Shrestha and Vassileva (2019) and Gandhi et al. (2023) who reported that both perception of ease of use and perception of usefulness have a significant impact on the blockchain adoption intention in agricultural sector. As a result, the more simple, convenient, and adaptable blockchain is the more likely beekeepers will want to make use of it. As suggested by some studies (Kumari and Devi, 2023), it is critical to increase user training and assistance to improve their perception of the ease of use and usefulness of BCT. The digital maturity construct had the least influence on blockchain intention to adopt, but it was significantly positive in both models, with a stronger effect in the TAM model. The finding of this effect indicates that digital technology adoption, previous knowledge or familiarity with the digital technology, the digitalized management of the data in the organization, and a strategy focused on the optimized information management, increase the probability of adopting the blockchain. These findings highlight the multifaceted nature of technology adoption and emphasize the importance of addressing various psychological and contextual factors to encourage the use of blockchain in the agricultural sector. Compared to TAM, TPB’s focus on social norms and perceived behavioral control appears less applicable in the beekeeping context, where individual decision-making is primarily driven by the tangible benefits of technology rather than external social pressures. The findings of this study demonstrate that perceived usefulness, a central construct in TAM, significantly outweighs the influence of social norms in determining adoption behavior. This underscores the value of TAM’s practical, utility-driven approach, offering more actionable insights into fostering blockchain adoption through targeted interventions such as user training and support systems. These conclusions align with prior research (Venkatesh, 2000; Kumari and Devi, 2023) and are further validated by the study’s results, where perceived usefulness emerged as the strongest predictor of blockchain adoption intentions.
6 Implications
The findings of this study have significant implications for the apiculture industry and the adoption of emerging technologies such as blockchain. The implications relate to the need to provide training and resources that enable the development of beekeepers’ capabilities, increasing beekeepers’ confidence and skills in the use of digital technologies such as blockchain, thus increasing their level of digital maturity. Policymakers can leverage this training by promoting educational programs designed specifically for beekeepers, including practical workshops on the use of advanced technologies, simulations of blockchain-based traceability processes, and demonstrations of success stories. The results also highlight the importance of blockchain solution developers creating functional interfaces that can be perceived as useful and easy to use, as this will increase the intention to adopt such innovation. It is also essential to promote information campaigns that highlight the tangible benefits of blockchain adoption such as traceability, transparency, and trust in supply chains, in order to positively influence beekeepers’ attitudes. It should be noted that while positive attitudes toward blockchain have a significant impact on their adoption intention, there are some other specific barriers for beekeeper, such as high implementation costs, limited infrastructure, and a lack of technical expertise, that can still hinder its adoption. Finally, establishing collaborative networks policymakers can support the creation of knowledge-sharing platforms between beekeepers and experts in innovative technologies and solutions. This not only increases trust and reduce perceived barriers, but also creates a favorable environment for the adoption of digital innovations, ensuring that digital maturity training leads to a positive and tangible impact on the adoption rates of these innovations.
Some potential limitations of our study are related to: the sample size, which, although adequate for PLS/SEM analysis, its relatively small size limits the results generalization and does not allow differentiation between the regions analyzed (Spain, Türkiye, and Tunisia). Therefore, a larger sample size in future researches could provide a more representative view and a more in-depth analysis of the relationships between variables. Self-reported data, which can be subject to biases such as social desirability bias or inaccurate self-assessment. Future research, to reduce this bias, the survey could be applied complete anonym instead of a face to face and include control items to detect biased responses. Endogeneity, including self-selection and omitted variable bias, is a common challenge in behavioral studies. While VIF scores and correlation analyses showed no multicollinearity, unobserved heterogeneity cannot be entirely excluded. Future research could apply methods like the Gaussian copula or instrumental variables for more robust result. The cross-sectional design of our data, which reports a single point in time, limiting the ability to infer causality or changes presented over time. To address this, future studies could adopt longitudinal research designs to explore the dynamic nature of blockchain adoption, including shifts in attitudes, behaviors, and external influences over time. Constructs such as perceived control behavior and digital maturity are difficult to measure accurately because they are often approximations, which may affect the reliability of the results. External factors, such as economic conditions, regulatory changes, or technological advancements in other areas, are not accounted for in this study and may influence blockchain technology adoption. Including these variables in future models could provide a more comprehensive analysis of the factors influencing adoption.
Despite these limitations, this study provides a basis for understanding the principal factors related with the beekeepers’ intention to adopt a digital technology such as blockchain. And demonstrates the flexibility of these models which could be further expanded to incorporate other contextual factors such cultural norms or government regulations.
7 Conclusion
This study utilized and compared the Theory of Planned Behavior (TPB) and the Technology Acceptance Model (TAM), significantly contributing to existing research by integrating the Digital Maturity (DM) construct into the models. The aim was to investigate the factors influencing beekeepers’ intention to adopt blockchain technology for honey traceability to mitigate fraud in the Mediterranean and enhance consumer trust. The PLS SEM method was employed to empirically assess the measurement and structural model. This study empirically validates the theoretical models TPB and TAM, demonstrating that all constructs significantly affect beekeepers’ intention to adopt blockchain technology. The extended TAM model produced better results than the extended TPB. In these models, the perceived behavioral control of TPB and the perceived usefulness of TAM were the most important predictors of blockchain adoption intentions. Furthermore, farmers’ digital maturity was a crucial factor in increasing adoption of such innovation.
The findings indicated that the implementation of blockchain requires the provision of training and resources to enhance beekeepers’ capabilities, thereby enhancing their trust and proficiency in using digital technologies. Moreover, the development of intuitive interfaces is essential for acceptance, highlighting the necessity of a cooperative process between blockchain developers and beekeepers. These contributions not only advance academic understanding but also offer practical advice for increasing digital technology adoption in agriculture, specifically blockchain in beekeeping. In summary, this study expands the scope of the TAM and TPB, integrating a new construct and applying them to an innovative agricultural sector, offering both conceptual advances and practical recommendations for the adoption of digital technologies in beekeeping sector.
To encourage blockchain adoption among Mediterranean beekeepers and beyond, tailored training programs should enhance digital literacy and emphasize benefits like honey traceability and fraud prevention. Efforts should account for regional technological differences, foster trust through local partnerships, and align with regulatory frameworks. Cost-effective solutions, such as subsidies, and sharing success stories can further inspire confidence and promote adoption.
Data availability statement
The raw data supporting the conclusions of this article will be made available by the authors, without undue reservation.
Ethics statement
The studies involving humans were approved by Ethics Committee of the Polytechnical University of Catalunya CREDA-UPC (2023–24). The studies were conducted in accordance with the local legislation and institutional requirements. The participants provided their written informed consent to participate in this study. Written informed consent was obtained from the individual(s) for the publication of any potentially identifiable images or data included in this article.
Author contributions
SO: Conceptualization, Data curation, Formal analysis, Investigation, Methodology, Visualization, Writing – original draft, Writing – review & editing. YB: Conceptualization, Writing – review & editing. YE: Conceptualization, Methodology, Validation, Writing – review & editing. EO: Conceptualization, Writing – review & editing. LA: Conceptualization, Writing – review & editing. SC: Conceptualization, Writing – review & editing. TM: Funding acquisition, Project administration, Resources, Writing – review & editing. ZK: Conceptualization, Funding acquisition, Project administration, Resources, Supervision, Validation, Writing – review & editing.
Funding
The author(s) declare that financial support was received for the research and/or publication of this article. This research is part of the R&D project TECHONEY - “Development of a blockchain-based ecosystem that allows an improved positioning of small producers of honey on local and international markets” (CREDA grant reference PCI2022–132950 and CITA grant reference PCI2022–132917), funded by the Spanish Ministry of Science and Innovation (MICIN) and the Spanish State Research Agency (AEI) (DOI: 10.13039/501100011033), as well as by the European Union, Next Generation EU/PRTR within the framework of the Partnership for Research and Innovation in the Mediterranean Area (PRIMA Section 2 Call 2021 - Multi-topic). The content of this paper reflects only the authors’ view, and the European Union Agency is not responsible for any use that may be made of the information it contains.
Conflict of interest
The authors declare that the research was conducted in the absence of any commercial or financial relationships that could be construed as a potential conflict of interest.
Generative AI statement
The authors declare that no Gen AI was used in the creation of this manuscript.
Publisher’s note
All claims expressed in this article are solely those of the authors and do not necessarily represent those of their affiliated organizations, or those of the publisher, the editors and the reviewers. Any product that may be evaluated in this article, or claim that may be made by its manufacturer, is not guaranteed or endorsed by the publisher.
References
Abbas, A., and Mehmood, K. (2021). Understanding digital marketing adoption in India: integrated by technology acceptance model (TAM) and theory of planned behaviour (TPB) framework. J. Manage. Sci. 8, 70–87. doi: 10.20547/jms.2014.2108206
Ajzen, I. (1991). Theory of planned behavior. Organ. Behav. Hum. Decis. Process. 50, 179–211. doi: 10.1016/0749-5978(91)90020-T
Ajzen, I., and Fishbein, M. (1980). Understanding attitudes and predicting social behavior. Englewood Cliffs, NJ: Prentice-Hall.
Al-Edrus, S. A. S., Ahmad, I., and Hanafiah, M. H. (2023). Will you be a honey and help us raise money?: investigating online crowdfunding platforms acceptance, perceived trust and behavioural intention. Manage. Accounting Rev. 22, 79–108.
AlShamsi, M., Al-Emran, M., and Shaalan, K. (2022). A systematic review on blockchain adoption. Appl. Sci. 12:4245. doi: 10.3390/app12094245
Aras, A., and Büyüközkan, G. (2023). Digital transformation journey. A holistic digital maturity model based on a systematic literature review. Systems 11:213. doi: 10.3390/systems11040213
Aslanova, I. V., and Kulichkina, A. I. (2020). “Digital maturity: definition and model,” in 2nd International Scientific and Practical Conference “Modern Management Trends and the Digital Economy: From Regional Development to Global Economic Growth” (MTDE 2020), 443–449. Atlantis Press.
Baytaroğlu, A. (2006). Honey market in the context of Turkey-European Union relations: Transition to organic beekeeping (Master's thesis). Istanbul: Marmara Universitesi.
Bermeo-Almeida, O., Cardenas-Rodriguez, M., Samaniego-Cobo, T., Ferruzola-Gómez, E., Cabezas-Cabezas, R., and Bazán-Vera, W. (2018). “Blockchain in agriculture: a systematic literature review” in Technologies and innovation: 4th international conference, CITI 2018, Guayaquil, Ecuador, November 6–9, 2018, proceedings 4. eds. A. M. Rafael, D. C. M. Gema, and V. L. Javier (Cham: Springer International Publishing), 44–56.
Bravo, M. J., Terra, C., Alcalde, J. C., and Sánchez, F. (2018). Adopción de tecnologías digitales en emprendedores. España: OBER UDD.
Büyük, A. M., Ateş, G., Burghli, S., Yılmaz, D., Temur, G. T., and Sivri, Ç. (2021). “Digital maturity assessment model for smart agriculture” in Digital conversion on the way to industry 4.0: Selected papers from ISPR2020, September 24–26, 2020. eds. N. M. Durakbasa and M. G. Gençyılmaz (Cham: Springer International Publishing), 289–301.
Caffaro, F., Cremasco, M. M., Roccato, M., and Cavallo, E. (2020). Drivers of farmers’ intention to adopt technological innovations in Italy: the role of information sources, perceived usefulness, and perceived ease of use. J. Rural. Stud. 76, 264–271. doi: 10.1016/j.jrurstud.2020.04.028
Cardona, J. R., Bassi, D. Á., and Sánchez-Fernández, M. D. (2018). Principales causantes de la actitud de los residentes: Comparativa entre dos destinos de playa. RPER 47, 37–48. doi: 10.59072/rper.vi47.484
Carmines, E. G., and Zeller, R. A. (1979). Reliability and validity assessment. London: Sage University.
Carrera, C. A., Dakduk, S., and Mesa, J. C. C. (2022). Innovación tecnológica: Escala de medida para agronegocios. RVG 27, 787–805. doi: 10.52080/rvgluz.27.8.4
Chowdhury, A., Kabir, K. H., McQuire, M., and Burea, D. P. (2024). The dynamics of digital technology adoption in rainbow trout aquaculture: exploring multi-stakeholder perceptions in Ontario using Q methodology and the theory of planned behaviour. Aquaculture 594:741460. doi: 10.1016/j.aquaculture.2024.741460
Concu, G. B., Atzeni, G., Meleddu, M., and Vannini, M. (2020). Policy design for climate change mitigation and adaptation in sheep farming: insights from a study of the knowledge transfer chain. Environ Sci Policy 107, 99–113. doi: 10.1016/j.envsci.2020.02.014
Dal-Mas, F., Massaro, M., Ndou, V., and Raguseo, E. (2023). Blockchain technologies for sustainability in the agrifood sector: a literature review of academic research and business perspectives. Technol. Forecast. Soc. Chang. 187:122155. doi: 10.1016/j.techfore.2022.122155
Danieli, P. P., and Lazzari, F. (2022). Honey traceability and authenticity. Review of current methods Most used to face this problem. Journal of apicultural. Science 66, 101–119. doi: 10.2478/jas-2022-0012
Dasaklis, T. K., Voutsinas, T. G., Tsoulfas, G. T., and Casino, F. (2022). A systematic literature review of blockchain-enabled supply chain traceability implementations. Sustain. For. 14:2439. doi: 10.3390/su14042439
Davis, F. D. (1985). A technology acceptance model for empirically testing new end-user information systems: Theory and results. Ph.D. dissertation. Cambridge, MA: Massachusetts Institute of Technology.
Davis, F. D., Bagozzi, R. P., and Warshaw, P. R. (1989). User acceptance of computer technology: a comparison of two theoretical models. Manag. Sci. 35, 982–1003. doi: 10.1287/mnsc.35.8.982
Dehghani, M., Popova, A., and Gheitanchi, S. (2022). Factors impacting digital transformations of the food industry by adoption of blockchain technology. J. Bus. Ind. Marketing 37, 1818–1834. doi: 10.1108/JBIM-12-2020-0540
Do, M. H., Huang, Y. F., and Hoang, T. T. (2025). Blockchain adoption in green supply chains: analyzing key drivers, green innovation, and expected benefits. J. Theor. Appl. Electron. Commer. Res. 20:39. doi: 10.3390/jtaer20010039
Dong, H., Wang, H., and Han, J. (2022). Understanding ecological agricultural technology adoption in China using an integrated technology acceptance model—theory of planned behavior model. Front. Environ. Sci. 10:927668. doi: 10.3389/fenvs.2022.927668
Dwivedi, Y. K., Rana, N. P., Jeyaraj, A., Clement, M., and Williams, M. D. (2019). Re-examining the unified theory of acceptance and use of technology (UTAUT): towards a revised theoretical model. Inf. Syst. Front. 21, 719–734. doi: 10.1007/s10796-017-9774-y
Edo, G. I., Onoharigho, F. O., Akpoghelie, P. O., Akpoghelie, E. O., Agbo, J. J., Agoh, E., et al. (2023). Natural honey (raw honey): insights on quality, composition, economic and health effects: a comprehensive review. Food Sci. Eng. 22, 265–293. doi: 10.37256/fse.4220232713
Engelseth, P. (2009). Food product traceability and supply network integration. J. Bus. Ind. Market. 24, 421–430. doi: 10.1108/08858620910966291
Fishbein, M., and Ajzen, I. (1975). Belief, attitude, intention and behavior: An introduction to theory and research. Reading, MA: Addison-Wesley.
Gandhi, P. S., Prentice, C., Sassenberg, A. M., and Soar, J. (2023). Identifying an optimal model for Blockchain technology adoption in the agricultural sector. Logistics 7:59. doi: 10.3390/logistics7030059
García, N., and Schwarzinger, S. (2021). “Honey fraud” in Food Fraud. ed. L. Manning (New York, NY: Academic Press), 309–334.
Gökalp, E., and Martinez, V. (2021). Digital transformation capability maturity model enabling the assessment of industrial manufacturers. Comput. Ind. 132:103522. doi: 10.1016/j.compind.2021.103522
González, H. I. (2018). Modelos PLS-PM. (Trabajo Fin de Grado Inédito). Seville: Universidad de Sevilla.
Hair, J. F., Hult, G. T. M., Ringle, C. M., Sarstedt, M., Danks, N. P., Ray, S., et al. (2021). An introduction to structural equation modeling. Partial least squares structural equation modeling (PLS-SEM) using R: a workbook. PLS-SEM 2021, 1–29. doi: 10.1007/978-3-030-80519-7_1
Hair, J. F., Hult, M., Sarstedt, M., Castillo Apraiz, J., Cepeda Carrión, G. A., and Roldán, J. L. (2019). Manual de partial least squares structural equation modeling (PLS-SEM). OmniaScience. Cap 5:11. doi: 10.3926/OSS.37
Hair, J. F., Sarstedt, M., Hopkins, L., and Kuppelwieser, V. G. (2014). Partial least squares structural equation modeling (PLS-SEM) an emerging tool in business research. Eur. Bus. Rev. 26, 106–121. doi: 10.1108/EBR-10-2013-0128
Hall, D. C. (2023). Managing fraud in food supply chains: the case of honey laundering. Sustain. For. 15:14374. doi: 10.3390/su151914374
Hanafiah, M. H. (2020). Formative vs. reflective measurement model: guidelines for structural equation modeling research. Int. J. Anal. Appl. 18, 876–889. doi: 10.28924/2291-8639-18-2020-876
Hansen, B. D., Leonard, E., Mitchell, M. C., Easton, J., Shariati, N., Mortlock, M. Y., et al. (2022). Current status of and future opportunities for digital agriculture in Australia. Crop Pasture Sci. doi: 10.10.1071/CP21594
Haryanti, T., Rakhmawati, N. A., and Subriadi, A. P. (2023). The extended digital maturity model. Big data and cognitive computing, 7, 17. doi: 10.3390/bdcc7010017
Henseler, J., Ringle, C. M., and Sarstedt, M. (2015). A new criterion for assessing discriminant validity in variance-based structural equation modeling. J. Acad. Mark. Sci. 43, 115–135. doi: 10.1007/s11747-014-0403-8
Jribi, S., Ben, I. H., Hanafi, N. E. H., Ben, S. H., Jmal, S., and Debbabi, H. (2022). An exploratory study for measuring consumers’ perception toward honey adulteration: Evidence from Tunisia, conference paper, proceedings of 4th Euro-Mediterranean conference for environmental integration (EMCEI-4). Cham: Springer.
Kamble, S., Gunasekaran, A., and Arha, H. (2018). Understanding the Blockchain technology adoption in supply chains-Indian context. Int. J. Prod. Res. 57, 2009–2033. doi: 10.1080/00207543.2018.1518610
Khaoula, B., Zineb, N., Zakaria, A., Abdelmajid, S., Asmae, C., and Abderrazak, K. (2019). Consumption, preferences and habits of purchasing consumers of honey in Morocco. J. Hyg. Eng. Design 22, 61–65.
Kramer, M. P., Bitsch, L., and Hanf, J. H. (2021). The impact of instrumental stakeholder management on blockchain technology adoption behavior in Agri-food supply chains. J. Risk Financ. Manage. 14:598. doi: 10.3390/jrfm14120598
Kumari, A., and Devi, N. C. (2023). Blockchain technology acceptance by investment professionals: a decomposed TPB model. J. Financ. Rep. Account. 21, 45–59. doi: 10.1108/JFRA-12-2021-0466
Ladu, L., Koch, C., Ashari, P. A., Blind, K., and Castka, P. (2024). Technology adoption and digital maturity in the conformity assessment industry: empirical evidence from an international study. Technol. Soc. 77:102564. doi: 10.1016/j.techsoc.2024.102564
Liu, N., and Ye, Z. (2021). Empirical research on the blockchain adoption–based on TAM. Appl. Econ. 53, 4263–4275. doi: 10.1080/00036846.2021.1898535
López-Bonilla, L. M., and Bonilla, J. M. L. (2006). Estudio comparado de las estimaciones de dos versiones del modelo de aceptación de la tecnología (TAM) mediante los programas AMOS y PLS. Inv. Eur. Dirección Econ. Empresa 12, 95–110.
Lukacs, M., Toth, F., Horvath, R., Solymos, G., Alpár, B., Varga, P., et al. (2025). Advanced digital solutions for food traceability: enhancing origin, quality, and safety through NIRS, RFID, Blockchain, and IoT. J. Sens. Actuator Netw. 14:21. doi: 10.3390/jsan14010021
MacPherson, J., Voglhuber-Slavinsky, A., Olbrisch, M., Schöbel, P., Dönitz, E., Mouratiadou, I., et al. (2022). Future agricultural systems and the role of digitalization for achieving sustainability goals. A review. Agron. Sust. Dev. 42:70. doi: 10.1007/s13593-022-00792-6
Mahattanakhun, C., and Suvittawat, A. (2023). Perceived and attitudes influencing intention to adopt new Technology for Farm Production: a case study of farmers at Nakhon Ratchasima Province Thailand. Int. J. Prof. Bus. Rev. 8:e02111. doi: 10.26668/businessreview/2023.v8i5.2111
Marín, A., Stubrin, L., Palacín, R., and Mauro, L. M. (2022). Caso de estudio COOPSOL: un emprendimiento social con proyección mundial (No. 2270, p. 52). Washington, DC: Banco Interamericano de Desarrollo.
Martínez, Á. M., and Fierro, M. E. (2018). Aplicación de la técnica PLS-SEM en la gestión del conocimiento: un enfoque técnico práctico. RIDE 8, 130–164. doi: 10.23913/ride.v8i16.336
Mendes, J. A. J., Carvalho, N. G. P., Mourarias, M. N., Careta, C. B., Zuin, V. G., and Gerolamo, M. C. (2022). Dimensions of digital transformation in the context of modern agriculture. Sust. Prod. Consumpt. 34, 613–637. doi: 10.1016/j.spc.2022.09.027
Mohr, S., and Kühl, R. (2021). Acceptance of artificial intelligence in German agriculture: an application of the technology acceptance model and the theory of planned behavior. Precis. Agric. 22, 1816–1844. doi: 10.1007/s11119-021-09814-x
Mustapa, M. A. C., Kallas, Z., Silande, C., Gagnaire, V., Jan, G., López-Mas, L., et al. (2024). From taste to purchase: understanding the influence of sensory perceptions and informed tasting on plant-based product purchases-an extension of the theory of planned behavior. J. Agric. Food Res. 16:101188. doi: 10.1016/j.jafr.2024.101188
Nikkhou, S., Taghizadeh, K., and Hajiyakhchali, S. (2016). Designing a portfolio management maturity model (Elena). Procedia Soc. Behav. Sci. 226, 318–325. doi: 10.1016/j.sbspro.2016.06.194
Noor, M. N. M., Sreenivasan, J., and Ismail, H. (2013). Malaysian consumers attitude towards mobile advertising, the role of permission and its impact on purchase intention: a structural equation modeling approach. Asian Soc. Sci. 9, 135–153. doi: 10.5539/ass.v9n5p135
Olsen, P., and Borit, M. (2013). How to define traceability. Trends Food Sci. Technol. 29, 142–150. doi: 10.1016/j.tifs.2012.10.003
Ostmeier, E., and Strobel, M. (2022). Building skills in the context of digital transformation: how industry digital maturity drives proactive skill development. J. Bus. Res. 139, 718–730. doi: 10.1016/j.jbusres.2021.09.020
Palos-Sanchez, P., Reyes-Menendez, A., and Saura, J. R. (2019). Modelos de adopción de tecnologías de la información y cloud computing en las organizaciones. Inf. Tecnol. 30, 3–12. doi: 10.4067/S0718-07642019000300003
Pappa, I. C., Iliopoulos, C., and Massouras, T. (2018). What determines the acceptance and use of electronic traceability systems in Agri-food supply chains? J. Rural. Stud. 58, 123–135. doi: 10.1016/j.jrurstud.2018.01.001
Paunović, M., and Alizadeh, Z. (2022). “Conceptual Blockchain model for honey supply chain system” in Book of proceedings of 5th international scientific Conference Village and agriculture. eds. A. Inam and D. S. Sayogo (Bijeljina: Bijeljina University).
Piot-Lepetit, I., Florez, M., and Gauche, K. (2023). Digitalisation des exploitations agricoles—Déterminants et impacts de l’adoption des innovations numériques. TechInn 8, 1–15. doi: 10.21494/iste.op.2023.1007
Rafiq, A., and Malik, A. M. (2024). Exploring the Nexus between sustainable agricultural practices and Blockchain integration: unraveling the influencing factors. Pakistan Res. J. Soc. Sci. 3:2.
Räisänen, J., and Tuovinen, T. (2020). Digital innovations in rural micro-enterprises. J. Rural. Stud. 73, 56–67. doi: 10.1016/j.jrurstud.2019.09.010
Rondan, C., Arenas, G. J., and Ramírez, C. P. E. (2015). A comparison of the different versions of popular technology acceptance models: a non-linear perspective. Kybernetes 44, 788–805. doi: 10.1108/K-09-2014-0184
Safiullin, N. A., Kurakova, C. M., Valeeva, G. A., and Yachina, L. T. (2022). Evaluation of digital maturity for agricultural organizations based on DMPTSC analysis. BIO Web Conf. 52:00020. doi: 10.1051/bioconf/20225200020
Safiullin, N. A., Mironkina, A. Y., Kharitonov, S. S., Trofimenkova, E. V., and Shevtsova, T. P. (2021). Assessment of digital maturity of agricultural enterprises. BIO Web Conf. 37:00160. doi: 10.1051/bioconf/20213700160
Saurabh, S. , and Dey, K. (2021). Blockchain technology adoption, architecture, and sustainable agri-food supply chains. Journal of cleaner production, 284, 124731. doi: 10.1016/j.jclepro.2020.124731
Sciarelli, M., Prisco, A., Gheith, M. H., and Muto, V. (2022). Factors affecting the adoption of blockchain technology in innovative Italian companies: an extended TAM approach. J. Strateg. Manag. 15, 495–507. doi: 10.1108/JSMA-02-2021-0054
Shang, L., Heckelei, T., Gerullis, M. K., Börner, J., and Rasch, S. (2021). Adoption and diffusion of digital farming technologies-integrating farm-level evidence and system interaction. Agric. Syst. 190:103074. doi: 10.1016/j.agsy.2021.103074
Sharma, A., Sharma, A., Singh, R. K., and Bhatia, T. (2023). Blockchain adoption in Agri-food supply chain management: an empirical study of the main drivers using extended UTAUT. Bus. Process. Manag. J. 29, 737–756. doi: 10.1108/BPMJ-10-2022-0543
Shrestha, A. K., and Vassileva, J. (2019). “User acceptance of usable blockchain-based research data sharing system: an extended TAM-based study,” in First IEEE International Conference on Trust, Privacy and Security in Intelligent Systems and Applications (TPS-ISA), 203–208.
Shukla, V., Kumar, J., Rana, S., and Prashar, S. (2024). Navigating the adoption landscape of blockchain in food delivery: a UTAUT perspective. Br. Food J. 126, 3113–3133. doi: 10.1108/BFJ-08-2023-0681
Soares, S., Amaral, J. S., Oliveira, M. B. P., and Mafra, I. (2017). A comprehensive review on the main honey authentication issues: production and origin. Compr. Rev. Food Sci. Food Saf. 16, 1072–1100. doi: 10.1111/1541-4337.12278
Sok, J., Borges, J. R., Schmidt, P., and Ajzen, I. (2020). Farmer behaviour as reasoned action: a critical review of research with the theory of planned behaviour. J. Agric. Econ. 72, 388–412. doi: 10.1111/1477-9552.12408
Sri Vigna Hema, V., and Manickavasagan, A. (2024). Blockchain implementation for food safety in supply chain: a review. Compr. Rev. Food Sci. Food Saf. 23:e70002. doi: 10.1111/1541-4337.70002
Tama, R. A. Z., Ying, L., Yu, M., Hoque, M. M., Adnan, K. M., and Sarker, S. A. (2021). Assessing farmers’ intention towards conservation agriculture by using the extended theory of planned behavior. J. Environ. Manag. 280:111654. doi: 10.1016/j.jenvman.2020.111654
Teichert, R. (2019). Digital transformation maturity: a systematic review of literature. Acta Univ. Agric. Silviculturae Mendelianae Brunensis. 67, 1673–1687. doi: 10.11118/actaun201967061673
Tornatzky, L. G., Fleischer, M., and Chakrabarti, A. K. (1990). Processes of technological innovation. Lexington, MA: Lexington Books.
Ullah, N. (2020). “Integrating TAM/TRI/TPB frameworks and expanding their characteristic constructs for DLT adoption by service and manufacturing industries-Pakistan context,” in International Conference on Technology and Entrepreneurship (ICTE), 1–5. IEEE.
Venkatesh, V. (2000). Determinants of perceived ease of use: integrating control, intrinsic motivation, and emotion into the technology acceptance model. Inf. Syst. Res. 11, 342–365. doi: 10.1287/isre.11.4.342.11872
Venkatesh, V., and Davis, F. D. A. (1996). Model of the antecedents of perceived ease of use: development and test. Decis. Sci. 27, 451–481. doi: 10.1111/j.1540-5915.1996.tb00860.x
Venkatesh, V., Morris, M. G., Davis, G. B., and Davis, F. D. (2003). User acceptance of information technology: toward a unified view. MIS Q. 27:425. doi: 10.2307/30036540
Verna, E., Genta, G., and Galetto, M. (2025). Enhanced food quality by digital traceability in food processing industry. Food Eng. Rev. 15, 1–25. doi: 10.1007/s12393-024-09392-4
Xiang, P., and Guo, J. (2023). Understanding farmers’ intentions to adopt pest and disease green control techniques: comparison and integration based on multiple models. Sustain. For. 15:10822. doi: 10.3390/su151410822
Yaacob, N. A., Ab Latif, Z., Mutalib, A. A., and Ismail, Z. (2021). Farmers’ intention in applying food waste as fertilizer: reliability and validity using smart-PLS. Asian J. Voc. Educ. Hum. 2, 27–34. doi: 10.53797/ajvah.v2i2.5.2021
Yu, J., Li, J., Lo, K., Huang, S., Li, Y., and Zhao, Z. (2025). Farmers’ adoption of smart agricultural technologies for black soil conservation and utilization in China: the driving factors and its mechanism. Front. Sust. Food Syst. 9:1561633. doi: 10.3389/fsufs.2025.1561633
Keywords: blockchain (BCT), beekeepers’ adoption, technology acceptance model (TAM), theory of planned behavior (TPB), digital maturity (DM)
Citation: Ornelas Herrera SI, Baba Y, Erraach Y, Ouertani E, Arfa L, Çamoğlu SM, de-Magistris T and Kallas Z (2025) Analysing blockchain adoption in beekeeping: application of theoretical models and their effectiveness. Front. Sustain. Food Syst. 9:1566341. doi: 10.3389/fsufs.2025.1566341
Edited by:
Xiao-Li Yin, Yangtze University, ChinaReviewed by:
Abid Yahya, Botswana International University of Science and Technology, BotswanaVishal Shukla, Auro University, India
Copyright © 2025 Ornelas Herrera, Baba, Erraach, Ouertani, Arfa, Çamoğlu, de-Magistris and Kallas. This is an open-access article distributed under the terms of the Creative Commons Attribution License (CC BY). The use, distribution or reproduction in other forums is permitted, provided the original author(s) and the copyright owner(s) are credited and that the original publication in this journal is cited, in accordance with accepted academic practice. No use, distribution or reproduction is permitted which does not comply with these terms.
*Correspondence: Selene Ivette Ornelas Herrera, c2VsZW5lLml2ZXR0ZS5vcm5lbGFzQHVwYy5lZHU=