- 1Department of Environmental Science, The University of Arizona, Tucson, AZ, United States
- 2Earth, Ocean and Atmospheric Science, Florida State University, Tallahassee, FL, United States
- 3Department of Microbiology, The Ohio State University, Columbus, OH, United States
- 4Bruker Daltonics Inc., Billercia, MA, United States
- 5Ecology and Evolutionary Biology, The University of Arizona, Tucson, AZ, United States
- 6Environmental Molecular Sciences Laboratory, Pacific Northwest National Laboratory, Richland, WA, United States
Warming-induced permafrost thaw could enhance microbial decomposition of previously stored soil organic matter (SOM) to carbon dioxide (CO2) and methane (CH4), one of the most significant potential feedbacks from terrestrial ecosystems to the atmosphere in a changing climate. The environmental parameters regulating microbe-organic matter interactions and greenhouse gas (GHG) emissions in northern permafrost peatlands are however still largely unknown. The objective of this work is to understand controls on SOM degradation and its impact on porewater GHG concentrations across the Stordalen Mire, a thawing peat plateau in Northern Sweden. Here, we applied high-resolution mass spectrometry to characterize SOM molecular composition in peat soil samples from the active layers of a Sphagnum-dominated bog and rich fen sites in the Mire. Microbe-organic matter interactions and porewater GHG concentrations across the thaw gradient were controlled by aboveground vegetation and soil pH. An increasingly high abundance of reduced organic compounds experiencing greater humification rates due to enhanced microbial activity were observed with increasing thaw, in parallel with higher CH4 and CO2 porewater concentrations. Bog SOM however contained more Sphagnum-derived phenolics, simple carbohydrates, and organic- acids. The low degradation of bog SOM by microbial communities, the enhanced SOM transformation by potentially abiotic mechanisms, and the accumulation of simple carbohydrates in the bog sites could be attributed in part to the low pH conditions of the system associated with Sphagnum mosses. We show that Gibbs free energy of C half reactions based on C oxidation state for OM can be used as a quantifiable measure for OM decomposability and quality to enhance current biogeochemical models to predict C decomposition rates. We found a direct association between OM chemical diversity and δ13C-CH4 in peat porewater; where higher substrate diversity was positively correlated with enriched δ13C-CH4 in fen sites. Oxidized sulfur-containing compounds, produced by Sphagnum, were further hypothesized to control GHG emissions by acting as electron acceptors for a sulfate-reducing electron transport chain, inhibiting methanogenesis in peat bogs. These results suggest that warming-induced permafrost thaw might increase organic matter lability, in subset of sites that become wetlands, and shift biogeochemical processes toward faster decomposition with an increasing proportion of carbon released as CH4.
Introduction
Permafrost peatlands, characterized by their cold and wet conditions that promote the accumulation of soil organic matter (SOM), contain an estimated 277 Pg of carbon (within the 3 m depth) (Schuur et al., 2008), which represents over one-third of the carbon stock in the atmosphere (ca. 800 Pg) (Schuur et al., 2008; McGuire et al., 2009). In the past 100 years, the mean annual Arctic temperatures have increased at almost twice the rate of the global average (IPCC, 2007; Solomon, 2007), leaving the fate of the previously frozen landscape uncertain. Due to these increasing temperatures coupled with seasonal thawing and freezing, permafrost thaw is changing the thickness of the soil active layer (Fenner et al., 2007), surface vegetation composition (Limpens et al., 2008), carbon accumulation rates in peat profiles (McGuire et al., 2009; Frolking et al., 2011), and microbial communities that govern degradation and transformation of organic C, with direct implications on greenhouse gas (GHG) emissions (de Graaff et al., 2010; Deng et al., 2014).
Absence of oxygen, created by water-logged conditions in thawing permafrost can promote anaerobic oxidation of organic matter (OM) (McLatchey and Reddy, 1998) by microbial communities via multiple anaerobic pathways (e.g., hydrolysis, fermentation, and methanogenesis) (Keller and Bridgham, 2007; Sutton-Grier et al., 2011; Wilson et al., 2017). However accurate prediction of GHG emissions from these systems due to changing environmental conditions is limited by our understanding of substrate quality, heterogeneity, and composition (Bölscher et al., 2017), microbial-organic matter interactions and biotic and abiotic OM degradation pathways (Mikutta et al., 2019). Recently, Zhou et al. (2020) found a positive correlation between the richness and diversity of the microbial communities and the concentration and bioavailability of the dissolved organic matter in pit, headstream, and river samples collected from the northern Qinghai-Tibetan Plateau, as well as physiochemical parameters such as pH and DOC and soluble ion concentrations. While some organisms are affected mainly by the concentration of specific substrates, it was found that the overall diversity of organisms may be affected by substrate quality and heterogeneity (Allison, 2005; Hättenschwiler and Jørgensen, 2010), and these effects in turn depend on the prevalence of resource generalists or resource specialists that shapes the microbial communities (Monard et al., 2016; Muscarella et al., 2019). Recently, (Woodcroft et al., 2018) investigated microbial community composition and function in the active layer of three sites across a thaw gradient at the Stordalen Mire (an intact palsa, a partially thawed bog and a fully thawed fen) and were able to link the Stordalen genome and functionality to the changing habitat. Metagenomics revealed distinct differences in diversity and overall composition of the microbial communities, with fen having the widest range of genes belonging to different phyla and thus higher microbial diversity. Metatranscriptomic data further confirmed the genomic trends, suggesting that in fen, wider range of microorganisms encode more diverse pathways in which organic substrates such as cellulose and xylan are rapidly degraded, providing substrates needed for methanogenesis and a more heterogenous organic matter composition. On the contrary, microbial communities in bog displayed less diverse functionalities in general with the lowest percentage of cellulase- and xylanase-encoding microorganisms. These results were consistent with previous studies at the same site where Hodgkins et al. (2014) and Hodgkins et al. (2016) showed that dissolved organic matter (DOM) becomes more labile with thaw in the Mire as the pH increases from bog to fen, with bog DOM being more recalcitrant, less diverse and contains more oxygen-rich compounds such as phenols and tannin-like compounds with higher oxidation state and overall lower decomposition rate due to the presence of sphagnan and other acidic inhibitors (Stalheim et al., 2009; Hájek et al., 2011). Conversely, fen DOM was more diverse and contained more low molecular weight compounds, more abundant nitrogen-containing compounds (interpreted as evidence of increased microbial activity), and less organic acids (Hodgkins et al., 2014, 2016). Moreover, differences in microbial community composition and function and OM quality were found to alter pathways by which CO2 and CH4 are produced. Studies by Svensson and Rosswall (1984), Galand et al. (2005), Lupascu et al. (2012), McCalley et al. (2014), and Singleton et al. (2018) suggested a shift from hydrogenotrophic to acetoclastic methanogenesis along the permafrost thaw gradient from bog to fen in the Stordalen Mire.
Although the ecological roles and properties of resource generalists and specialists in many discontinuous permafrost areas have been examined via extensive metagenomic and metatranscriptomics analysis (Liao et al., 2016; Sriswasdi et al., 2017; Singleton et al., 2018; Woodcroft et al., 2018), highly resolved molecular characterization of the OM is an essential foundation upon which we can understand ecosystem C cycling by both plants and microbes in peatlands for predicting future changes in CO2 and CH4 production potential (Qualls and Richardson, 2003). Moreover, current models for estimating permafrost soil carbon loss rates are lacking representations of key microbial processes that control OM transformations, leading to enormous uncertainty in model simulations (Burke et al., 2012; Koven et al., 2015; Chang et al., 2019). At most, such models only include a quantitative measure of OM concentration (Lucchese et al., 2010) but neglect the fact that the rate of OM degradation is dependent on the abundance and diversity of active microbes and OM quality and diversity. As such, factors accounting for OM transformation (biotically and abiotically) are poorly constrained in most models and are an important area for model improvement. To address this knowledge gap, we used gas chromatography (GC) and Fourier transform ion cyclotron resonance (FT-ICR) mass spectrometry (MS) to characterize SOM chemical composition in bog and fen soil samples collected from the Stordalen Mire. First, we hypothesized that SOM composition and heterogeneity can be used to capture shifts in the functional diversity of microbial communities with thaw gradient. Second, we hypothesized that NOSC and Gibbs free energy of C oxidation can be used to capture C decomposability and changes to the redox conditions of the surroundings (LaRowe and van Cappellen, 2011). Third, we hypothesized that sulfate reduction pathways by sulfate-reducing microorganisms (SRMs) are more active in the bog and are in competition with methanogens, rapidly changing the SOM composition, stimulating CO2 and suppressing CH4 emission potentials. Understanding OM energetics and metabolic transformation pathways along the thaw gradient can provide insights on resource heterogeneity and microbial diversity which can then be used to indirectly incorporate microbial processes in different biogeochemistry models and to predict how this ecosystem-level shift will alter GHG emission under a continually changing climate.
Materials and Methods
Site Information and Soil Sampling and Preparation
Stordalen Mire (68.35°N, 19.05°E) is a peat plateau in subarctic Sweden underlain by discontinuous permafrost, which is thawing as the region warms. The Mire has three dominant vegetation community types (Johansson et al., 2006): (1) Palsa (intact permafrost) with woody herbaceous vegetation; (2) Ombrotrophic peatland or bog (intermediate thaw) features with Sphagnum spp., sedges, and shrubs as the dominant vegetations; and (3) Minerotrophic peatland or fen (fully thawed) dominated by sedges, mainly Eriophorum spp. (Bäckstrand et al., 2010). Generally, bogs are fed only by precipitation, have acidic (pH ~ 4) surface waters and Sphagnum-dominated vegetation. These Sphagnum mosses, are known to inhibit alternate plant growth by acidifying water and creating an environment that is harsh to other plant species (van Breemen, 1995). Fens, on the other hand, are characterized by more mineral-rich water because they are fed by surface water and groundwater, in addition to rainfall. They are usually dominated by grasses and sedges and maintain slightly acidic to alkaline pH. Due to continued warming in the Arctic, the areal extent of intact palsa (Malmer et al., 2005) and Sphagnum community (Norby et al., 2019) across the Stordalen Mire has declined significantly since 1970 (Malmer et al., 2005) while at the same time, wetter fen habitats have expanded (Kokfelt et al., 2009; Bäckstrand et al., 2010). The ground's thaw state and consequent relationship to the water table determine the plant community, which in turn determines organic matter composition. In areas where the permafrost is degraded, land subsidence leads to varying degrees of water inundation. In this study, we focused on these inundated sites, and specifically a fen and a bog site with an extensive set of historical gas flux, microbial, and geochemical measurements (previously described by Hodgkins et al., 2014, 2016; McCalley et al., 2014; Woodcroft et al., 2018). In June 2012, peat was collected from both sites with an 11-cm-diameter homemade circular push corer. Both sites were cored in triplicate to capture spatial heterogeneity. Cores were divided into three sections, and the sections were placed into plastic bags and stored frozen until analysis. Eighteen soil samples were collected in total, including nine samples (3 cores × 3 depths per core) collected from a Sphagnum-derived ombrotrophic bog and nine samples collected from a sedge-dominated fen (Table 1). Porewater for dissolved organic carbon (DOC) analysis was gathered from the same depths by syringe suction through a 1-m long, 0.5-cm-diameter stainless steel tube with holes drilled along the bottom 3 cm, then filtered through 0.7-μm Whatman GF/F glass microfiber filters into 120-mL brown borosilicate bottles. The pH was measured on-site with an Oakton Waterproof pHTestr 10. The bottles were frozen within 8 hours of collection and stored frozen until analysis. DOC concentrations were measured by high-temperature catalytic oxidation on a Shimadzu Total Organic Carbon analyzer with a non-dispersive infrared detector. Each sample was analyzed in triplicate measurements, which constantly had a coefficient of variance of <2%. The concentration was reported as millimolar (mM). Sulfate and nitrate concentrations were measured by ion chromatography on a Dionex ICS-1100 with a 4-mm IonPac AS22 column, with a 4.5 mM carbonate/1.4 mM bicarbonate eluent at a flow rate of 1.2 mL/min. The concentration was reported as micromolar (μM). In this study, we will focus on the overall SOM molecular composition differences observed in the bog vs. the fen and not the depth effect.
Porewater Gas Measurements
Porewater samples for dissolved gas analysis were collected similarly to the DOC samples, with porewater drawn up by syringe suction through perforated stainless-steel tubes that were inserted into the peat at the desired depth. Porewater was immediately filtered in the field using 2.7-μm Whatman GF/D glass-fiber filters, then stored in pre-evacuated glass vials sealed with butyl stoppers. Samples were then processed according to the method described by (Hodgkins et al., 2015). Phosphoric acid (1 mL of 20%) was added to each sample, to convert all dissolved inorganic carbon to CO2 (thus removing differences in /CO2 ratios due to differing pH) and to preserve for shipment to Florida State University. Helium was then added to the sample headspaces to bring them to a constant pressure of 1 atm. Samples were analyzed for CH4 and CO2 concentrations and stable isotopic composition (δ13C) on a Thermo Finnigan Delta-V Isotope Ratio Mass Spectrometer. As described in (Corbett et al., 2013, 2015) and (Hodgkins et al., 2014), headspace gas concentrations were then converted to dissolved concentrations (mM) in the original unacidified porewater based on previously-determined gas extraction efficiencies (defined as the proportion of formerly-dissolved gas in the headspace after acidification and equilibration between the water and headspace) (Supplementary Table 1). Each sample was analyzed twice and the average results for each sample were recorded. Analytical precision of replicate δ13C measurements was 0.2%0.
Gas Chromatography—Mass Spectrometry (GC-MS)
Organic Matter Extraction
Water was used to extract the labile fraction of SOM from the collected peat (n = 18, 9 fen and 9 bog samples) according to the protocol described by Tfaily et al. (2015) and Tfaily et al. (2017). Briefly, extracts were prepared by adding 1 mL of H2O to air dried and ground 100 mg bulk soil and shaking in 2 mL capped glass vials for 2 h on an Eppendorf Thermomixer. Samples were then removed from the shaker and left to stand before spinning down and pulling off the supernatant to stop the extraction. All samples were stored at −80°C until analysis. Water extractable organic matter (WEOM; Corvasce et al., 2006) is described as a bioavailable fraction of organic matter that contains mostly polar compounds. Changes in the molecular weight and elemental composition of the WEOM fraction can provide important information regarding ecologically relevant processes in soil.
Around 0.5 ml of water extracted organic matter from nine bog samples and only three fen samples (due to sample limitations) were first freeze dried using a Labconco™ FreeZone Freeze Dryer and then derivatized as described previously (Kim et al., 2015). Briefly, 20 μl of 30 mg/ml methoxyamine hydrochloride in pyridine (Sigma-Aldrich, Saint Louis, MO) was added to each freeze-dried sample for the protection of carbonyl groups. Samples were vortexed for 20s, sonicated for 60s, and were incubated at 37°C for 90 min with generous shaking (1,000 rpm). After the incubation, 80 μL of N-methyl-N-(trimethylsilyl) trifluoroacetamide (MSTFA) (Sigma-Aldrich) with 1% trimethylchlorosilane (TMCS) (Sigma-Aldrich) was added to each sample. The samples were vortexed for 20s, sonicated for 60s, and incubated for 30 min at 37°C with shaking (1000 rpm). After derivatization, the samples were allowed to cool to room temperature.
GC-MS Analysis
Samples were transferred to autosampler vials and analyzed by GC-MS in random order. Blanks and fatty acid methyl ester (FAME) samples (C8-28) were also included in the analysis for the background referencing and retention time calibration, respectively. Metabolites were separated using a HP-5MS column (30 m × 0.25 mm × 0.25 μm; Agilent Technologies, Santa Clara, CA) and analyzed in an Agilent GC 7890A coupled with a single quadrupole MSD 5975C (Agilent Technologies, Santa Clara, CA). For each analysis, 1 μl of sample was injected in splitless mode. The injection port temperature was kept constant at 250°C for the duration of the analysis. The GC analysis started with an initial oven temperature fixed at 60°C for 1 min after injection, and then at a steady rate of 10°C/min, the temperature was increased to 325°C with the analyses finishing with a 5 min hold at 325°C. The GC-MS raw data files were processed with MetaboliteDetector as previously reported by Hiller et al. (2009). Briefly, retention indices (RI) of the detected polar metabolites were calibrated based on the mixture of FAME internal standards, followed by deconvolution, and subsequent chromatographic alignment of the detected features across all analyses. Identification of the metabolites was carried out by first matching GC-MS spectra and retention indices against a Pacific Northwest National laboratory (PNNL) augmented version (>900 metabolites) of FiehnLib (PMID: 19928838) library containing validated retention indices and spectral information (Kind et al., 2009). Subsequently, unidentified metabolites were screened against the NIST14 GC-MS library by spectral matching alone. To eliminate false identification and reduce the deconvolution errors created during the automated data-processing, all metabolite identifications were individually validated.
Row-wise normalization (by sum) was then performed on the metabolite data using MetaboAnalyst 4.0 to allow for general-purpose adjustment for differences among samples. Data transformation [Generalized log transformation (log2)] and data scaling [Auto scaling (mean-centered and divided by standard deviation of each variable)] were further performed to make features more comparable using the same platform.
Fourier-Transform Ion Cyclotron Resonance Mass Spectrometry (FT-ICR-MS)
Direct Inject FT-ICR-MS Analysis
A 12 Tesla Bruker FT-ICR mass spectrometer located at PNNL was used to collect high resolution mass spectra of the WEOM. A Suwannee River Fulvic Acid standard (SRFA), obtained from the International Humic Substance Society (IHCC), was used as a control to monitor potential carry over from one sample to another and ensure instrument stability in day to day operation. The instrument was flushed between samples using a mixture of Milli-Q water and HPLC grade methanol. The ion accumulation time (IAT) was varied between 0.03 and 0.05 s to account for variations in C concentrations in different samples. For each sample, 144 individual scans were averaged, and an organic matter homologous series separated by 14 Da was used as an internal calibration. The mass measurement accuracy across 100–1,200 m/z range was <1 ppm (accounted for singly charged ions) and the mass resolution at 341 m/z was ~240 K and the transient was 0.8 s. BrukerDaltonik version 4.2 data analysis software was used to convert raw spectra (obtained from each sample) to a list of m/z values applying FT-MS peak picker module with a signal-to-noise ratio (S/N) threshold of 7 and absolute intensity threshold of 100. Formularity (Tolić et al., 2017) software was used to assign chemical formulae based on the criteria described in Tfaily et al. (2018).
Chemical Compounds Classification and Data Processing
The molecular formulae in each sample were evaluated on van Krevelen diagrams (Van Krevelen, 1950), based on their H:C ratios (y-axis) and O:C ratios (x-axis) (Kim et al., 2003), assigning them to the major biochemical classes (e.g., lipid, protein, lignin, carbohydrate etc.). The H:C and O:C ranges for biochemical classification are provided in Table 2.
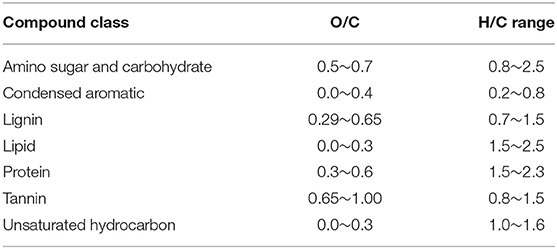
Table 2. O/C and H/C ranges used to classify the compounds detected via FT-ICR-MS in this study (Adopted from Tfaily et al., 2015, 2017).
Oxidation state is related to the bioenergetic potential of a specific compound based on its atomic makeup. Oxidation state indicates how a compound may be transformed in biochemical processes without necessarily knowing its structure (LaRowe and van Cappellen, 2011). For each individual compound, the average nominal oxidation state of carbon (NOSC), which represents the number of electrons transferred in C oxidation half reactions, was calculated based on Equation 1 (where C, H, N, O, P, and S represent the numbers of each element, and number coefficients represent typical oxidation states). More negative values for NOSC indicates the presence of more reduced carbon atoms.
ΔG°C-ox (kJ/mol C) indicates the thermodynamic favorability of the compound, with lower values of ΔG°C-ox for a compound indicating greater likelihood of degradation (LaRowe and van Cappellen, 2011). ΔG°C-ox was estimated from NOSC following Equation (2):
Positive values for ΔG°C-ox indicate that the oxidation of the C must be coupled to the reduction of a terminal electron acceptor, which in the TEA-depleted mire can include the soil organic matter itself (Keller and Takagi, 2013; Wilson et al., 2017). Organic matter degradation may become thermodynamically limited depending on the availability of terminal electron acceptors (Boye et al., 2017). Under oxic conditions the availability of high energy yielding O2 as a terminal electron acceptor couples the decomposition of organic matter of all naturally occurring NOSC values (Keiluweit et al., 2016). However, under the inundated conditions of subsurface peat deposits, oxygen and other terminal electron acceptors become depleted thereby slowing or completely inhibiting the decomposition of organic matter with low NOSC values (Boye et al., 2017). In peat, oxygen is rapidly depleted to below detection limits (20 ppb) within 2–3 cm below the water surface (Lin et al., 2014). At Stordalen Mire, the average annual water table is typically near the peat surface in the fen (Hodgkins et al., 2014) and was 3.5–7 cm above the peat surface during our sampling. Thus, even the shallow fen peat is consistently under anoxic conditions and subject to depleted TEA availability. In the bog, the mean annual water table depth is around 17 cm (Hodgkins et al., 2014), but was only 2 cm below the peat surface during sampling for this project. Thus, the bog peat is subjected to fluctuating water (and subsequently oxygen) levels throughout the growing season. This could have the effect of enhancing decomposition rates during dry periods and re-oxidizing previously reduced TEAs to support decomposition following subsequent inundation. Relative energy yields associated with the terminal electron acceptors in anoxic conditions also provide insight on the sequences in which organic matter gets decomposed (Grandy and Neff, 2008). Lower ΔG°C-ox is an indication of compounds more thermodynamically favored to undergo metabolic and chemical reactions (Wilson and Tfaily, 2018) (i.e., available for microbial communities), whereas higher ΔG°C-ox reflects microbially degraded and transformed (i.e., recalcitrant) OM.
For each elemental formula, double-bond equivalent (DBE) minus oxygen (DBE-O) was calculated using Equation (3):
While NOSC and ΔG°C-ox provide information on overall quality of the OM, DBE-O provides more structural information, mostly of carbon-to-carbon double bonds (i.e., lack of hydrogen saturation) without considering the oxygen atoms that mostly exist as carboxyl groups in OM (Bae et al., 2011). Using this formulation, more oxygenated molecules have lower (negative) values for DBE-O while higher values for DBE-O indicate highly unsaturated compounds such as condensed aromatic structures (Herzsprung et al., 2014).
Identification of Metabolic Transformations in Bog and Fen Samples Using Network Analysis
The rich database generated by high resolution mass spectrometry provides accurate mass information, enabling us to infer metabolite composition in complex matrices. We used network analysis to explore metabolic transformations in the bog and fen samples to further understand how two compounds are related to one another and how they might be involved in the same metabolic transformation (via a mass difference between two m/z values demonstrated as an edge in the network, and the initial and final compounds as nodes). Here, we used molecular transformation analysis to identify potential microbial transformation or decomposition pathways using FT-ICR-MS data. For a reaction to be considered and hence counted by this method, both the reactant and the product should be present, hence this approach can provide some information about SOM decomposition, uptake and accumulation (Burgess et al., 2017). We used Cytoscape (Shannon et al., 2003; Kohl et al., 2011) with the MetaNetter plug-in to generate molecular interaction networks. For each sample, the list of m/z values generated by the FT-ICR-MS analysis along with a list of 86 pre-defined m/z values and their subsequent molecular formula and IUPAC names [adopted from (Breitling et al., 2006) (Supplementary Table 2)] were imported into Cytoscape. The molecular interaction networks are consisting of nodes (m/z values) and edges (mass difference between two m/z values). Each m/z value inferred from the FT-ICR-MS analysis was defined as a node (described previously in Longnecker and Kujawinski, 2016) connected to other node (s) through the pre-defined m/z values defined as edges, representing a metabolic transformation. For example, gain or loss of CH2 (Δm = 14.01565007) between two compounds in the molecular interaction networks is depicted by two nodes and an edge. Nodes represent the two compounds with mass difference of 14.01565007 Da and edge is the mass difference (named CH2).
In total, 86 metabolic transformations were grouped into four different categories: amino acid-associated transformation (loss/gain of an amino acid and/or involvement of nucleoside or nucleotide), sugar -associated transformation (loss/gain of monomeric or polymeric carbohydrates), carbon transformation (reactions that can occur both biotically or abiotically depending on the environmental conditions), and other transformation that includes heteroatoms (such as loss/gain of a phosphate or an amine group). For this study, we have only focused on the first three categories since similar trends for the bog and fen samples (regardless of depth) were observed in the ‘other transformation’ category. The output of the Cytoscape is a list with the number of times (count) each transformation occurred between any two peaks in a particular sample as shown in Supplementary Figure S5. Normalized values (to the total number of transformations observed in each sample) and subsequently, normalized percentages are then calculated and used for comparison between samples and across sites.
Furthermore, a comprehensive set of topological parameters calculated from these undirected network plots (Assenov et al., 2008) based on transformation data generated by the Cytoscape with the MetaNetter plug-in was used to compare SOM metabolic transformation pathways between the bog and fen samples. For this study, two different parameters were chosen: clustering coefficient and average number of neighbors, all of which can be used to represent the level of population of the transformation network (Barabási and Albert, 1999; Ravasz et al., 2002). The clustering coefficient, Cn, in undirected networks is a ratio of the number of edges (N) between the neighbors of a node n, and the maximum number of edges (M) between the neighbors of n (Barabási and Oltvai, 2004). Cn is calculated as Cn = 2en/(kn(kn-1)), where kn is the number (size) of neighbors of n and en is the number of connected pairs between all neighbors of n (Watts and Strogatz, 1998). The clustering coefficient of a node is always between 0 and 1(Barabási and Oltvai, 2004). A high clustering coefficient indicates a high number of neighbors for each node and hence represents low selectivity and high diversity since a compound could be produced by multiple reactions. The average number of neighbors therefore is an average of kn of all nodes in the undirected network (Dong and Horvath, 2007). High number of neighbors indicates low degradation and high selectivity. Values for clustering coefficient and average number of neighbors for each sample are provided in Supplementary Table 3. Moreover, the network analysis parameters (clustering coefficient and average number of neighbors) were used to correlate the overall number and/or density of the metabolic reactions happening in each sample to measured biogeochemical properties in pore water such as pH, δ13C and DOC.
Statistical Analyses and Data Visualization
GC-MS Data
Univariate (Pearson correlation coefficient, t-test significance), multivariate (principal component analysis, PCA) and clustering analysis (heatmap, t-test significance) were performed on normalized values to visualize differences between treatments and among samples using MetaboAnalyst 4.0 and plotted using JMP 14.2.0 and R (R Core Team, 2019) package ggplot2 version 3.3.1 (Wickham, 2016).
Porewater Gas, FT-ICR-MS, and Network Analysis Data
Analysis of variance (ANOVA), unpaired t-test, and multivariate (principal component analysis, PCA) analysis were performed using the computing environment R (R Core Team, 2019). Figures were produced using the package ggplot2 version 3.3.1 (Wickham, 2016). Additional software packages (ggpubr, factoextra, ggnewscale, ggrepel, scales, hrbrthemes, and RColorBrewer) were taken from the Bioconductor project (Gentleman et al., 2004).
Results
Changes in SOM Molecular Composition Associated With Permafrost Thaw
Principal component analysis of the relative abundance of different SOM compound classes (as inferred by FT-ICR-MS), biogeochemical properties of peat porewater (TN concentration, DOC, sulfate, nitrate, and ammonia concentration and pH) and peat porewater CO2 and CH4 concentrations revealed clear separation between the bog and fen sites along PC1 (which explained 41% of the variance), with higher PC1 scores in rich fens (Figure 1). SOM in the Sphagnum-dominated bog sites had a higher relative abundance of tannins, lignins, unsaturated, and condensed hydrocarbons (UnSatHC and CondHC, respectively), and lipids compared to the fen sites. In contrast, fen samples were separated from bog samples along PC1 which correlated positively with the abundance of peptide-, carbohydrates (cellulose-)-, and aminosugar-like (AS) compounds. PC2 explained a small proportion of the variance (16%). Higher concentrations (measured in mM) of TN and DOC were observed in the bog porewaters compared to the fen porewater. In contrast, sulfate, nitrate and ammonia concentrations were higher in fen porewater and correlated positively with peat porewater pH. Porewater CO2 and CH4 concentrations were higher in the fen sites. Although some depth trends were observed, these trends were generally weak, as they were not consistently observed over the entire depth range and none of the principal components showed significant correlations with depth (Supplementary Figure 1).
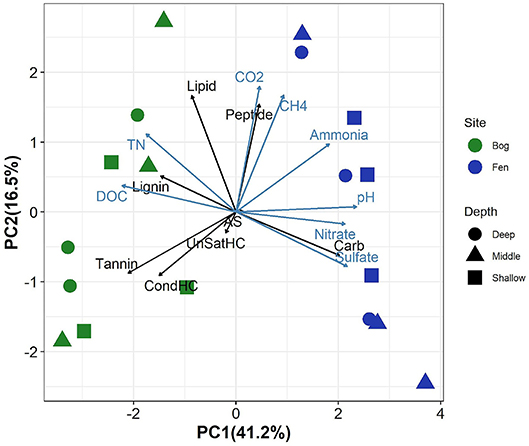
Figure 1. Principal components analysis on elemental composition classes from FT-ICR-MS data (black arrows)and biogeochemical properties including the concentrations of total nitrogen(TN), dissolved organic carbon (DOC), sulfate, nitrate, ammonia, and pH (measured in porewater, indicated by blue arrows) and CO2 and CH4 (values for each sample is described in Supplementary Table 1). Fen samples are clearly separated from bog along PC2 (which positively correlates with peptides, CO2, CH4, sulfate, pH, and nitrate) and bog samples show positive and high correlations with carbohydrates, tannins and condensed hydrocarbon and DOC.
To better visualize the differences in the bog and fen SOM molecular composition, unique peaks present in each group (bog vs. fen, regardless of the depth) were reported separately on van Krevelen diagrams (Figures 2A,B). SOM in bog samples (Figure 2A) appeared to be more rich in lignin-like (0.29 < O/C < 0.65 and 0.7 < H/C < 1.5), tannin-like (0.65 < O/C < 1 and 0.8 < H/C < 1.5), and lipid-like (0 < O/C < 0.3 and 1.5 < H/C < 2.5) compounds and fen SOM (Figure 2B) was more rich in peptide-like (0.3 < O/C < 0.6 and 1.5 < H/C < 2.3) compounds and amino sugars (0.5 < O/C < 0.7 and 0.8 < H/C < 2.5). Out of 6,906 peaks detected in the bog samples in total, 24% were unique to bog, while only 8% of the peaks (out of 5,973 in total) detected in the fen were unique to fen, suggesting a higher number of unique compounds in bog (potentially not yet degraded) compared to fen samples (Venn diagram in panel B).
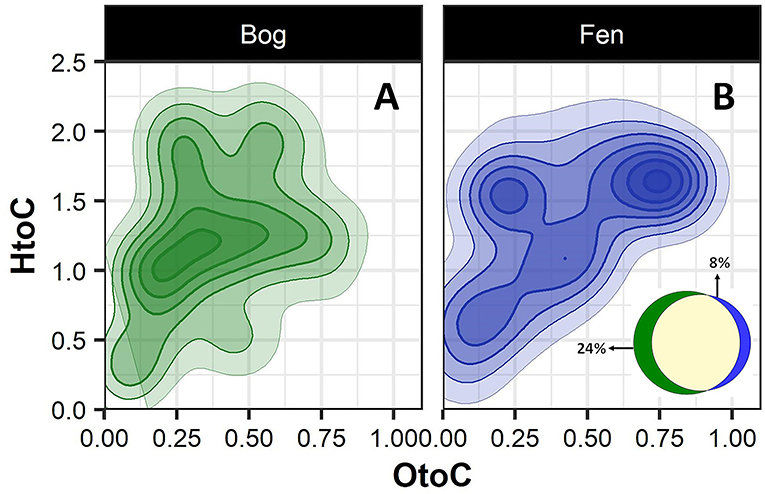
Figure 2. Van Krevelen diagram generated from FT-ICR-MS data comparing the compositional differences in the bog (A) and fen (B) samples (regardless of depth). Oxygen to carbon (O/C) and hydrogen to carbon (H/C) ratios were calculated for unique peaks in bog (green) and fen (blue) sites. Ven diagram (B) indicates that 24% of the peaks (out of 6,906 peaks in total) detected in bog samples are unique to bog and 8% of the peaks (out of 5,973 peaks in total) detected in fen are unique to fen.
Shifts in Thermodynamics of Carbon Oxidation With Permafrost Thaw
Figure 3A shows ΔG°C-ox calculated for 6906 assigned molecular formula in nine bog samples and 5973 assigned molecular formulas in nine fen samples. Lower ΔG°C-ox and high NOSC represent thermodynamically more labile organic compounds and vise-versa. For each sample, ΔG°C-ox values (Figure 3A; Table 3) and NOSC values (Table 3) were averaged across all formulas and unpaired t-test was performed on the averaged values obtained from nine bog samples and nine fen samples. Average ΔG°C-ox values in bog samples were lower than fen samples (Figure 3A; Table 3) and subsequently, average NOSC values were higher in bog samples compared to fen samples (Table 3). Interestingly, different ΔG°C-ox values were obtained for bog and fen samples within the same class of chemical compounds (Figure 3B). While amino sugars were more abundant in the fen sites compared to bog sites, they were characterized with higher average ΔG°C-ox compared to those in the bog sites. On the other hand, while bog sites had an abundance of lignin-like and tannin-like compounds compared to fen, however, they were of lower average ΔG°C-ox compared to those in the fen sites (more oxidized). The lower overall NOSC values of the bog SOM relative to the fen SOM could reflect the influence of oxygen at the bog site. As noted in section Chemical Compounds Classification and Data Processing, the fen is more consistently inundated (hence anoxic), while the bog habitat experiences alternating periods of oxic and anoxic conditions. These periods of exposure to oxygenation and the potential re-oxidation of organic and inorganic TEAs may facilitate decomposition at the bog site resulting in SOM with an overall lower NOSC value relative to the fen. However, the overall lower NOSC in the bog site may also reflect different starting substrates.
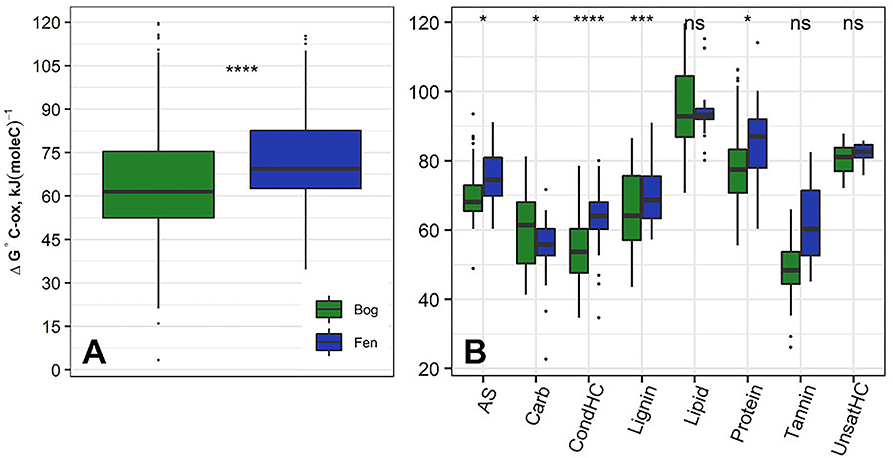
Figure 3. (A) Average ΔG°C-ox (Gibbs free energy of C half reactions based on C oxidation; kJ/mol C) values based on FT-ICR-MS data calculated for the bog (green) and fen (blue) samples (regardless of depth). Low ΔG°C-ox represents thermodynamically favorable organic compounds for microbial degradation. Unpaired t-test was performed on the calculated values to determine the significant differences and P-values were reported. Additionally, (B) ΔG°C-ox values were graphed based on compound classifications for bog and fen samples. Statistical significances were determined by unpaired t-test. The “ns” symbol indicates P > 0.05. All P < 0.05, 0.001, and 0.0001 are summarized with one, three and four asterisks, respectively, indicating ΔG°C-ox values for those classes were significantly different between the bog and fen samples.
Sulfur and Nitrogen Dynamics With Permafrost Thaw
To further investigate the sulfur and nitrogen dynamics in the Mire, composition of S-containing SOM (regardless of depth) was compared in the bog (A) and fen (B) samples and unique peaks were reported on a van Krevelen diagram (Figures 4A,B). Sulfur containing compounds in bog fell in the lipid-like (0 < O/C < 0.3 and 1.5 < H/C < 2.5), peptide-like (0.3 < O/C < 0.6 and 1.5 < H/C < 2.3), and amino sugar-like (0.5 < O/C < 0.7 and 0.8 < H/C < 2.5) regions of the van Krevelen diagram. In contrast, S-containing compounds in fen fell in the condensed hydrocarbon-like (0 < O/C < 0.4 and 0.2 < H/C < 0.8) and lignin-like (0.29 < O/C < 0.65 and 0.7 < H/C < 1.5) regions of the van Krevelen diagram. Overall, a higher abundance of unique S-containing organic compounds was observed in the bog compared to the fen system (~ 2.6 to ~1.3% unique S containing compounds, respectively, depicted on Venn diagram in panel A) coincident with lower sulfate concentrations in bog porewater (Table 1). Furthermore, molecular weights and nitrogen to carbon ratios (N/C) for unique compounds were calculated, and both were overlaid on the van Krevelen diagram (Figures 4C,D). Higher abundance of N-containing compounds (~5% −345 unique compounds out of 6,906 peaks in total) with molecular weight >400 m/z (>400 Da) was observed in bog compared to fen samples. Conversely, fen SOM appeared to have a lower abundance of N-containing compounds (1% −70 unique compounds out of 5,973 peaks in total) and the N-containing compounds in the fen tended to be of lower mass (Supplementary Figure 2).
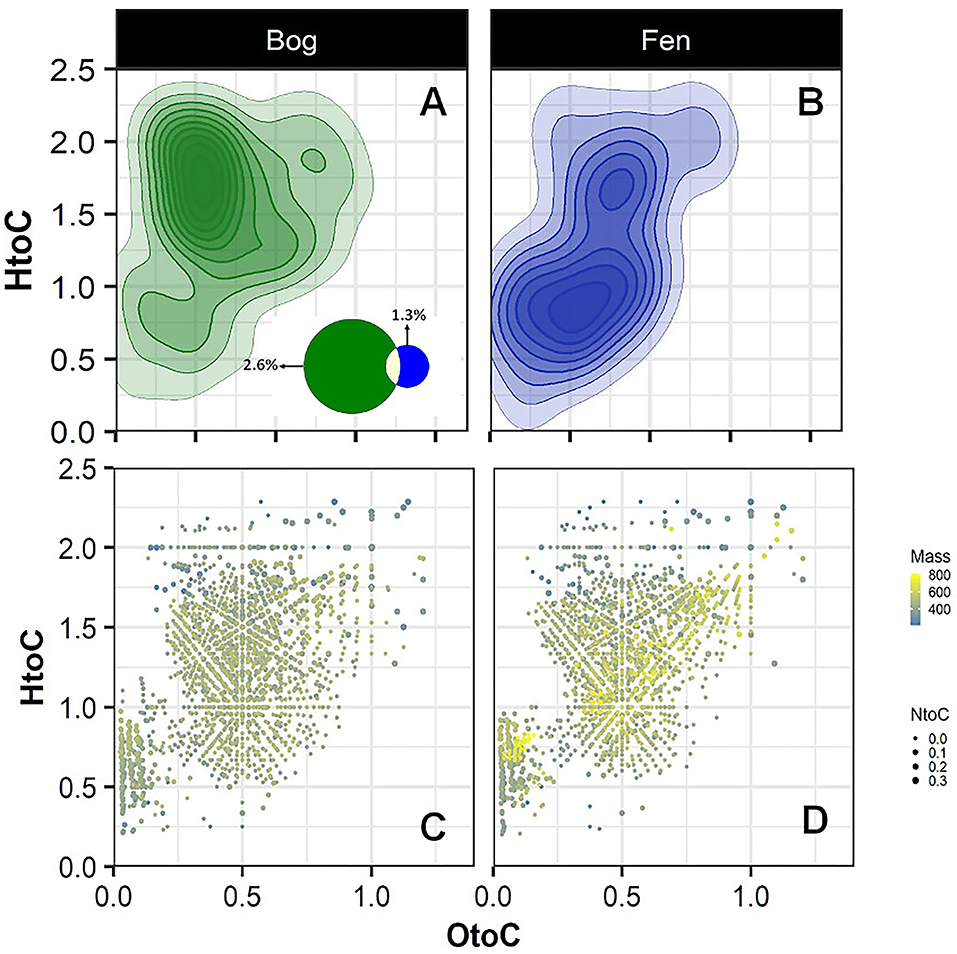
Figure 4. Van Krevelen diagram generated from FT-ICR-MS unique peaks comparing the compositional differences in the bog (A) and fen (B) samples for sulfur containing compounds (regardless of depth). Venn diagram (A) indicates that 177 peaks (out of 6,906 peaks in total) detected in bog samples were unique to bog and 79 peaks of the peaks (out of 5,973 peaks in total) detected in fen were unique to fen. (C,D) graphs were generated from graphs (A,B) where mass of the unique peaks and nitrogen to carbon (N/C) ratios from unique peaks were overlaid on the van Krevelen diagram separately for the bog and fen. Steel blue to yellow color gradient indicates the mass of the compounds and the size of the points indicates the N/C ratios.
DBE-O was calculated for all unique fen and bog S- and N-containing compounds to better characterize the OM in the bog and fen samples (Supplementary Figure 3). Interestingly, for S-containing compounds, average DBE-O value for bogs and fens were significantly different (p < 0.01). Average bog DBE-O was 1.36 (±7.03) compared to the fen DBE-O of 4.18 (±8.05), suggesting that S-containing compounds in bog are more oxygenated with potentially higher number of carboxyl groups and fewer C=C double bonds. Average DBE-O values calculated for N-containing compounds were not significantly different between the bog and fen samples.
Shifts in Primary Metabolite Metabolism
We used multiple statistical tests to look at changes in SOM primary (central) metabolite composition between bog and fen samples (Figure 5; Table 4) where we observed statistically significant differences in bog and fen primary metabolite abundance. Principal component analysis (Figure 5A) revealed distinct differences in metabolite abundance (explained by PC1, 47.1%) between bog and fen samples. Effect of depth was also explained by PC2 (19.9%). Correlation coefficients of 25 primary metabolite abundances between bog and fen samples (regardless of depth) using Pearson r distance measure were determined at p < 0.05 (Figure 5B). Sugar acids such as D-galacturonic acid and simple sugars such as D-glucose, melibiose, sucrose, fructose, and D-mannose were significantly upregulated (more abundant) in bog samples (regardless of depth) whereas upregulated metabolites in fen samples were mainly classified as acids such as Erythronic acid, glycolic acid, pyruvic acid and amino acids such as glutamine and isoleucine. Another way to view this data is through the use of heatmaps. A heatmap of the same 25 identified metabolites (t-test significance) showed a similar separation (unpregulated sugar acids and simple sugars in bog and organic acid and amino acids in fen) between metabolites identified in different bog and fen samples (Figure 5D). Figure 5C features the top four upregulated metabolites in bog and fen samples created via fold change (FC) analysis and t-test on absolute value changes between metabolites identified in bog and fen samples.
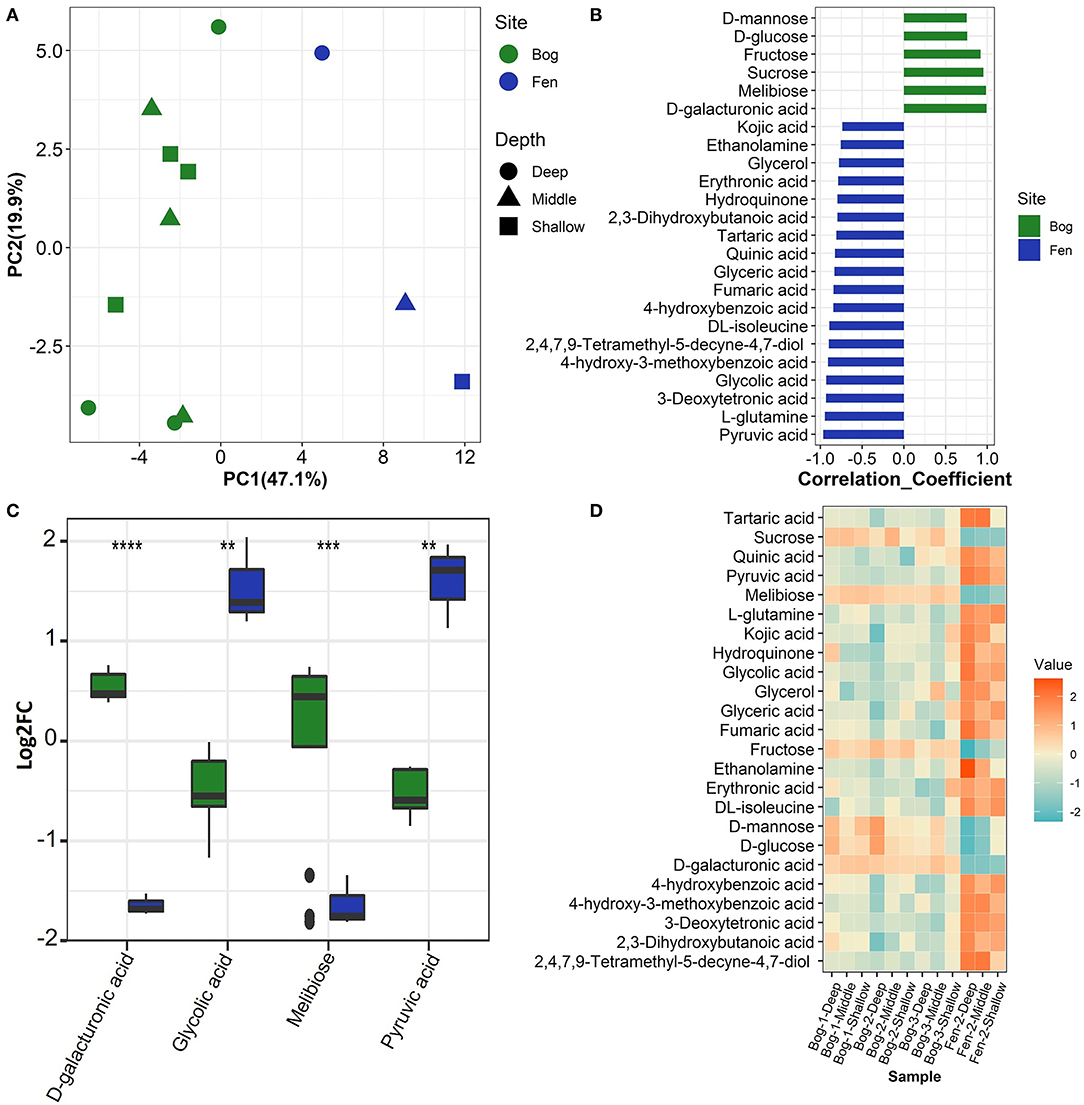
Figure 5. (A) Principal component analysis (PCA) plot derived from GC-MS primary metabolite abundance variation between the bog (green) and fen (blue) groups. (B) Correlation analysis performed against Fen-Bog showing the 25 most significant features correlated with the pattern with p < 0.05. Distance measure used: Pearson r. (C) Box plots visualizing distribution of a subset of metabolites that are significantly changing (pairwise t-test, p < 0.05) between the two treatments. All P values less than 0.01, 0.001, and 0.0001 are summarized with two, three and four asterisks, respectively. (D) Heatmap of the significant metabolites (pairwise t-test) upregulated (orange) and downregulated (blue) between the bog and fen groups at different time points.
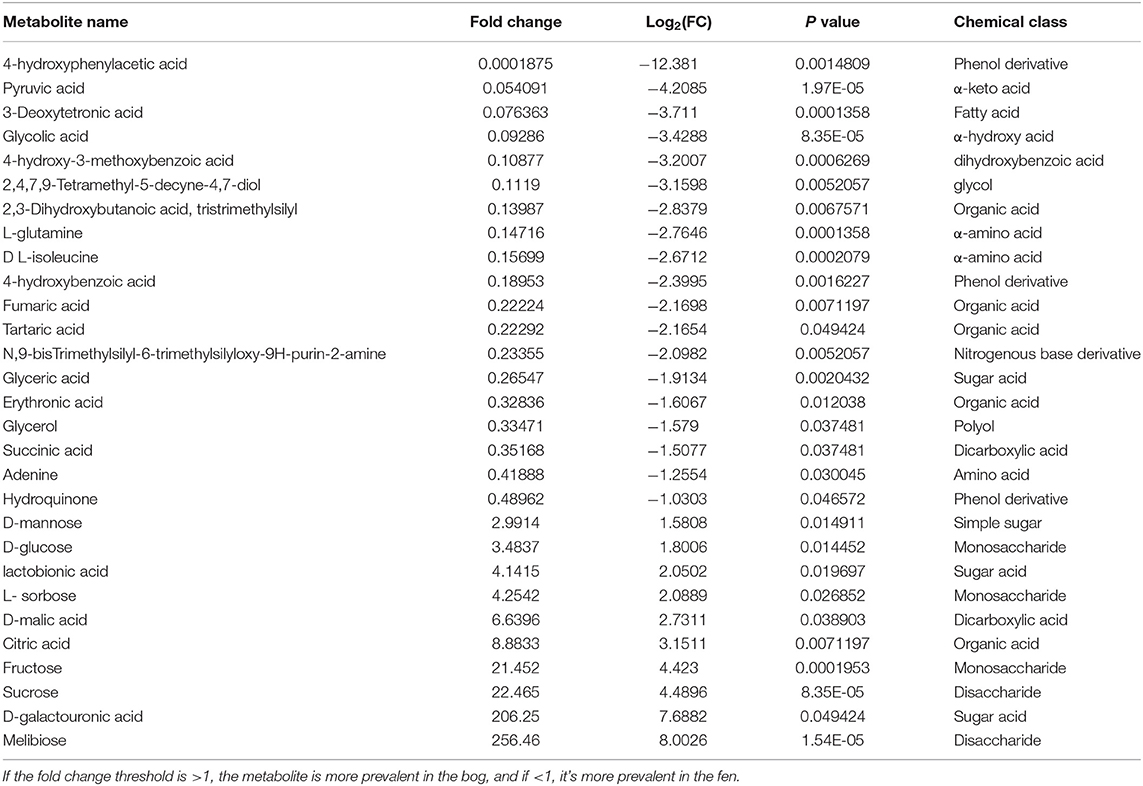
Table 4. Metabolites that display both large magnitude fold-changes (fold change threshold >2 and p < 0.05); comparison type” Bog/Fen) and high statistical significance (P-value threshold = 0.05).
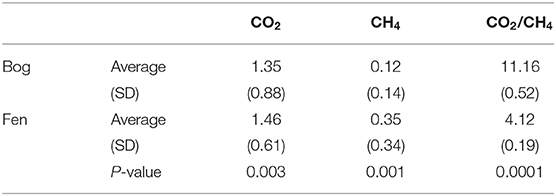
Table 5. Concentrations of porewater CO2 and CH4 (reported in mM) and CO2/CH4 ratio measured in site for bog and fen (averaged).
Shifts in C Transformations With Thaw
Consistent with changes in SOM and primary metabolite composition, transformation analysis indicated that the biochemical processes associated with the SOM degradation were significantly different between the bog and fen sites (Figure 6A). We observed higher abundances of sugar-associated transformations for the bog sites compared to the fen sites, which generally correlated with carbon transformations (Figure 6A). These data are consistent with Figures 2, 4B where bog sites had a lower abundance of carbohydrate molecules with mass >400 Da but a higher abundance of mono and disaccharides, reflective of decomposition of complex carbohydrates into mono, and disaccharides. However, based on their higher relative abundance in the porewater (Figure 5), these sugars are likely being slowly utilized by microbial communities and/or undergoing slow decomposition. In contrast, fen samples had a higher abundance of complex carbohydrate molecules, but lower abundance of simple, mono, and disaccharides. Despite the higher abundance of complex carbohydrate compounds in the fen site and the higher abundance of sugar - associated transformations in the bog compared to the fen, principal component analysis on specific sugar -associated metabolic transformations on all elemental formulas detected in the bog and fen samples (Figure 6B) indicated similar sugar transformations, suggesting that microbes at both sites have the capability of degrading simple and complex sugar molecules. These data suggest that simple sugars are being utilized at a higher rate in the fen sites compared to the bog sites. The slow decomposition at the bog sites and/or slow uptake of glucose by microbial communities for fermentation or anaerobic cellular respiration suggest additional controls (e.g., thermodynamic, abiotic processes) on SOM decomposition in the bog sites. The high amino acid-associated transformations in the fen samples (Figure 6A) is consistent with a higher abundance of amino acid compounds at the fen sites, reflective of protein degradation and /or release of amino acids by fen vegetation through roots.
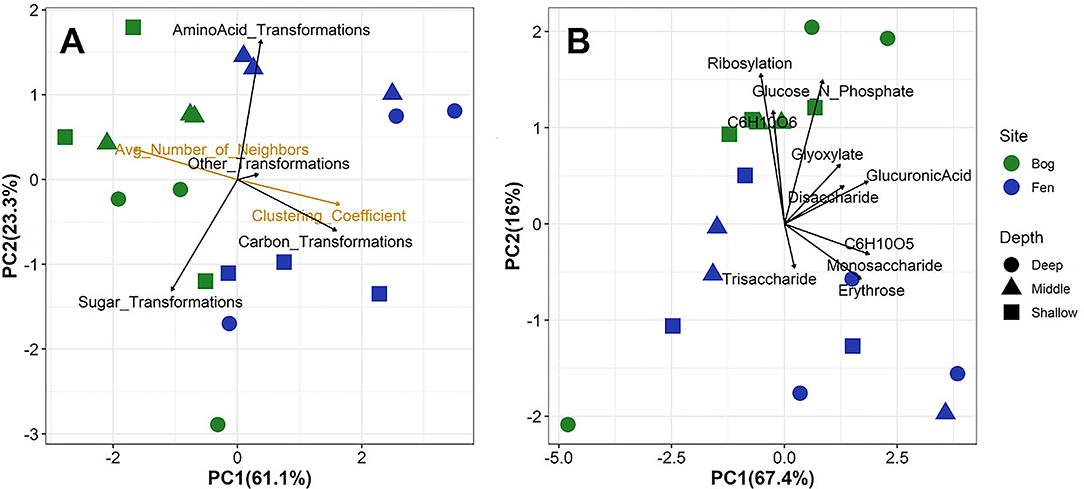
Figure 6. Principal component analysis of (A) overall metabolic transformations and network analysis parameters and (B) specific sugar-associated metabolic transformation for all formulas detected in the bog and fen samples. Fen samples are separated by higher correlation to clustering coefficient whereas bog samples are highly correlated to average number of neighbors. Higher correlation between bog samples and sugar-associated transformation indicates preservation of these compounds in the bog. However, (B) indicates similar specific sugar-associated metabolic transformation in both the bog and fen samples.
CO2 and CH4 Emissions Associated With SOM Chemical Composition
Figure 7 and Supplementary Figure 4 show the correlation between network analysis parameters to pH, DOC (mM), and δ13C -CH4 of bog and fen porewater samples. Significant correlations were observed between clustering coefficient and average number of neighbors for SOM molecules to porewater pH, DOC (mM) and δ13C -CH4 values. SOM clustering coefficient increased with increasing pH (Figure 7A), decreasing DOC (Figure 7B), and enriched δ13C -CH4 (Figure 7C) values as in the case of fen and decreased with decreasing pH (Figure 7A) and depleted δ13C -CH4 values (Figure 7C) as in the case of bog. On the other hand, SOM average number of neighbors had an opposite trend to the clustering coefficient (Supplementary Figure 4). Significant correlations (p < 0.05) were also observed between different biogeochemical properties including concentrations of DOC, TN, DIC (CO2), CH4, ammonia, nitrate, sulfate, δ13Cpw-DIC, δ13Cpw-CH4 (Supplementary Table 4), revealing clear coupling between different biogeochemical properties and GHG emissions.
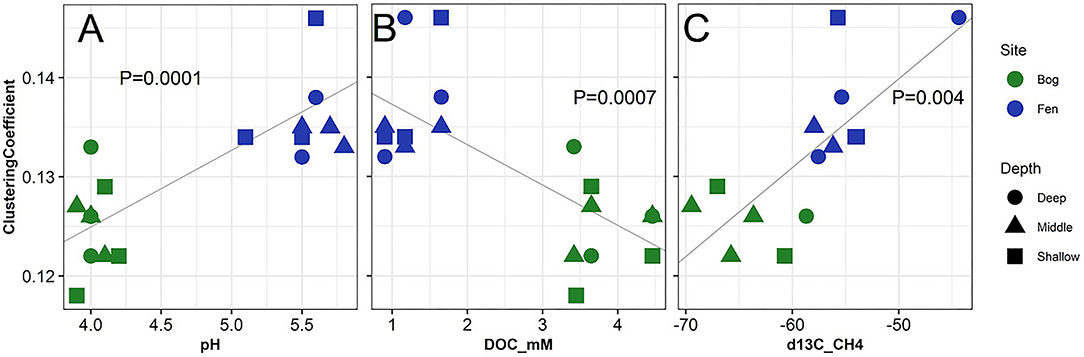
Figure 7. Correlation of network analysis parameter (clustering coefficient) with (A) pH, (B) dissolved organic carbon and (C) δ13C for CH4 measured from the porewater of the bog (green) and fen (blue) samples. One-Way ANOVA (at p < 0.05) was performed on the calculated values to determine the significant differences and P-values were reported to be 0.0001, 0.0007, and 0.004, respectively.
Discussion
Based on our advanced analytical characterization of peat SOM along a permafrost thaw gradient, organic matter compositional differences were clear between the bog and fen sites. Fen SOM appeared to be more degraded (regardless of the depth), transformed, and reduced as evident by the more negative NOSC and higher ΔG°C-ox values. This data is consistent with previous studies comparing bog and rich fen samples from different locations across the Stordalen Mire from different years (Hodgkins et al., 2014, 2016). Phenol oxidase is an important enzyme in peatlands, as it is one of the few that can decompose recalcitrant phenolic compounds generally produced by Sphagnum spp. (McLatchey and Reddy, 1998; Freeman et al., 2004). Degradation of these phenolic compounds by microbes without the aid of phenol oxidase is challenging due to the high resonance energy that stabilizes the C-C bonds in aromatic rings (Harwood and Parales, 1996). Our data indicated that the bog SOM was more oxidized, dominated by tannin-like (polyphenolic) and organic acids, all with large number of carboxyl, aromatic, and methoxylated phenol groups (higher MWs). Previous reports have shown inhibition of microorganisms and the extracellular enzymes by these large polymers via crossbridged hydrogen bonds with proteins (enzymes) as well as penetration to the sensitive sites within microorganisms, hence altering their physiology (Wang et al., 1991; Field and Lettinga, 1992). These findings suggest that the high abundance of phenolic compounds in peat bogs could have a toxic effect on microorganisms in addition to lower decomposition rates by extracellular enzymes (Freeman et al., 2004). The activity of phenol oxidase is also dependent on various factors such as bimolecular oxygen availability, extent of waterlogging, and plant communities, which in the peat bog are factors controlled by Sphagnum spp. (Freeman et al., 2004). Sphagnum spp. creates waterlogged conditions, acidifies its environment using high cation exchange rates (Rudolph and Samland, 1985; Rasmussen et al., 1995; Thormann, 2006), produces lignin-like polymeric phenols that inhibit enzymatic activity (such as 5-keto-D-mannuronic acid, p-hydroxy-b[carboxymethyl]-cinnamic acid) (Stalheim et al., 2009; Fudyma et al., 2019), and has highly recalcitrant cell walls (Stalheim et al., 2009; Hájek et al., 2011). Sphagnum moss is also known to decay more slowly than vascular plants (Hodgkins et al., 2014; Tfaily et al., 2014). The preservation of labile compounds in the peat bog observed in our data is likely due to the anoxic, acidic, and nutrient sequestration traits intrinsic to Sphagnum spp. and likely impedes phenol oxidase activity and subsequent organic matter decay by microbial communities (Williams et al., 2000; Freeman et al., 2004).
Diversity in microbial communities is important to the function and stability of many microbial ecosystems, such as animal- and plant-associated microbiomes (Worm et al., 2002; Muscarella et al., 2019). Uncovering mechanisms to maintain microbial diversity in these environments is important in understanding belowground metabolic transformations and subsequent GHG emissions (Muscarella et al., 2019). Substrate generalists vs. specialists can interact with different available substrates and depending upon the substrates that are used, we can partly understand microbial preferences and predict gas flux changes (Chen et al., 2020; Zhou et al., 2020). Based on our data, bog SOM exhibited higher DOC porewater concentrations, lower microbial diversity (inferred from Shannon diversity; Singleton et al., 2018; Woodcroft et al., 2018), and activity (inferred from the high average number of neighbors and lower clustering coefficient in the bog metabolic transformations analysis—Supplementary Table 3), suggesting that bog microbial communities are comprised of substrate specialists that are only capable of performing a limited set of specific functions (Monard et al., 2016; Muscarella et al., 2019) under anoxic and acidic conditions mainly created by Sphagnum spp. (Williams et al., 2000; Freeman et al., 2004). On the other hand, fen SOM was characterized by increased substrate heterogeneity (higher clustering coefficient), suggesting fen microbial communities are substrate generalists and are able to use and transform same substrates through different metabolic pathways. This observation is again consistent with Woodcroft et al. (2018) that showed microbial diversity (Shannon index) in the bog to be significantly lower than that in the fen. GC-MS data showed that the majority of upregulated metabolites in the bog were mono and disaccharides and sugar acids, in particular galacturonic acid, while the fen had higher abundances of amino acids and polyphenols (Figures 5, 6B), suggesting that in the fen, mono and disaccharides are consumed by microbial communities as soon as they are degraded by various biotic and abiotic metabolic pathways and the high abundances of amino acids and polyphenols reflect microbial byproducts and thus an active microbial community (Whiting and Chanton, 1992; Chanton et al., 1995, 2008; Hodgkins et al., 2014).
Recently, Fudyma et al. (2019) showed that Sphagum fallax metabolite profile is composed of flavonoid glycoside compounds (a compound formed from a simple sugar and a phenol), that could be acting as potent antimicrobial compounds. Thus, the quality of bog SOM (i.e., high abundance of lignin-like and polyphenolics and simple sugars, recalcitrant dead Sphagnum litter, and the low phenol oxidase enzymes activity) all suggest limited OM degradation by biotic factors. Here we hypothesize that the accumulation of simple sugars is either due to low rates of biotic decomposition and consumption, leading to its accumulation or that the release of simple sugars is occurring abiotically through acid catalyzed hydrolysis of lignocellulose and cellulose (BeMiller, 1967; Timell, 1967; Hopkinson, 1969; Girisuta et al., 2007; Ahmad and Pant, 2018) (Figures 2A, 5B–D). Therefore, we further postulate that low pH could be aiding the acid catalyzed hydrolysis of carbohydrates and sugar acids (glycosides) in bog systems (Ahmad and Pant, 2018) leading to the release of mono and disaccharides with lower average ΔG°C-ox. However, accumulation of these compounds in the peat with time probably due to low pH and high abundance of galacturonic acids produced by Sphagnum, further shed light on the limited microbial activities in respiring these compounds (Figures 1, 3, 5) (Rasmussen et al., 1995; Thormann, 2006). Metabolic transformation analysis and FT-ICR-MS data confirm this trend, with higher abundance of sugar transformations present in bog samples. Because both reactant and product of the metabolic transformation are present in the bog samples, we believe in the bog biotic degradation of organic matter happens more slowly than at fen sites, and that possible byproducts of Sphagnum OM degradation in the bog (i.e., upregulated galacturonic acid—which is a by-product of pectin degradation known to have antimicrobial effects—Hájek et al., 2011) could be limiting the biotic complex carbohydrate degradation pathways, resulting in the accumulation of both complex and labile sugars. Observed correlations between the pH and the SOM clustering coefficient (positive correlation; p < 0.0001) and the average number of neighbors (negative correlation; p = 0.0002) potentially indicates the impact of pH on substrate quality and concentration. Lower pH values promote carbon accumulation and low substrate diversity whereas higher pH values are correlated with higher substrate quality and diversity. The bog porewater DOC concentration correlated positively (p = 0.0008) with the average number of neighbors, reflecting accumulation of OM in the bog sites consistent with lower decomposition rates (Supplementary Figure 3) and acidic conditions (low pH). Moreover, in fens, vascular plant roots may transport oxygen into the soil, where it activates phenol oxidase and thereby decreases the concentration of decomposition-inhibiting phenolics and organic acids (Joabsson and Christensen, 2001; Estop-Aragonés et al., 2012). However, further experiments are required to validate these assumptions.
Depleted δ13C -CH4 in the bog porewater samples suggest that hydrogenotrophic rather than acetcolastic methanogenesis is likely the preferred pathway for methane production. This is consistent with low degradation potential of the bog SOM and higher DOC concentrations (Hodgkins et al., 2014; McCalley et al., 2014; Tfaily et al., 2014). More enriched δ13C -CH4 in fen porewater, on the other hand, suggests that acetoclastic methanogenesis is the dominant pathway for CH4 production and that methanogenisis substrates are produced by OM fermentation leading to low DOC concentration (Chanton et al., 1995; Chasar et al., 2000). Observed correlation between the clustering coefficients and the δ13C -CH4 for the bog and fen samples potentially suggests that the network analysis parameters indicating the substrate diversity and heterogeneity can be used as an indication of pathways by which microbial communities are utilizing the OM and ultimately producing GHG and they indirectly provide information on the microbial diversity. However, further studies should aim to replicate these findings in a larger experimental setup.
Pathways underlying CO2 and CH4 productions in peatlands are also dependent on S and N cycling and the microorganisms that interact with these compounds (Granberg et al., 2001; Limpens et al., 2008; Pester et al., 2012). Sulfate (SO4 2−) and nitrate () can act as terminal electron acceptors in anoxic conditions, allowing microorganisms to couple the oxidization of substrates (e.g., H2, organosulfur and organonitrogen compounds) and reduction of TEAs to produce CO2 and CH4 (Muyzer and Stams, 2008). Hence sulfate reducing microorganisms (SRM) and methanogens can co-exist or compete for substrates within these oxygen-limited environments (Raskin et al., 1996; Schink, 1997). Recently, Woodcroft et al. (2018) identified the presence and activation of high diversity deep-branching lineages of the dsrAB genes (dissimilatory sulfate reduction genes) belonging to sulfate-reducing microorganisms (SRMs) in Sphagnum-dominated bog in the Mire. SRMs utilize sulfur containing compounds and produce CO2. In peatlands, H2 is generated from fermentation of carbohydrates (especially the ones with aromatic rings), while oxidized S-containing compounds come from plant matter (Rawat et al., 2012). Peat bogs generally have larger amounts of oxidized organosulfur compounds due to high S uptake potential by Sphagnum and therefore dead Sphagnum is rich in organic S compounds, which can drive sulfate reduction (Urban et al., 1989; Novák and Wieder, 1992; Fritz et al., 2014). Our data suggests that hydrogenotrophic reduction by SRM and nitrate reduction is occurring in the peat bog due to the following factors: (1) increased organosulfur compounds, potentially acting as TEAs for SRMs and suggesting that sulfate reduction is not limited by S availability (Baena et al., 1998); (2) low porewater mM concentrations of SO4 2− and , suggesting rapid turnover of TEAs; (3) low pH, which is likely inhibiting methanogen activities (Tang et al., 2018); and (4) high abundance of mono and disaccharides, suggesting degradation of large carbohydrates by fermentative bacteria in addition to acid-catalyzed dissociations. Fermentation byproducts such as CO2, and H2, are then further consumed in hydrogenotrophic reduction (Conrad et al., 1987; Goodwin et al., 1991). CO2 and CH4 porewater concentrations measured in the bog samples were lower compared to the fen samples (Table 4), however, CO2:CH4 production ratios (Table 4) were higher in the bog compared to the fen, suggesting likely consumption of fermentation and acid-catalyzed dissociation byproducts by SRMs, leading to suppressed methanogenesis potentials by methanogens in the bog (Ward et al., 2009; Rawat et al., 2012; Hausmann et al., 2018). Similarly, SRMs can oxidize CH4 coupled with sulfate reduction, which is also likely driving the lower CH4 production rates in bogs (Cord-Ruwisch and Ollivier, 1986; Ward et al., 2009; Rawat et al., 2012). Higher CO2 and CH4 porewater concentrations in the fen are likely due to a decrease in Sphagnum-derived inhibitory organic acids (Figure 5) driven by changing plant inputs and increasing pH (Table 1 and Supplementary Table 1) from bog to fen systems. Lower C/N ratios in the fen, driven by lower C/N of plant inputs from sedges compared to Sphagnum, may also lead to greater organic matter decomposition in the fen by alleviating N limitation for decomposers (Hodgkins et al., 2014; Khadka et al., 2016).
Conclusion
Due to the contribution from aboveground vegetation and belowground metabolic transformations under various redox conditions, microbial processes both affect and are being affected by the chemical composition and quality of SOM. In order to gain better insight on the overall metabolic transformations in bog and fen and to link substrate diversity and quality with microbial activity and GHG production, we calculated the Gibbs free energy of C half reactions based on C oxidation state for OM, a quantifiable measure for OM decomposability and quality, which can be incorporated into current biogeochemical models to represent OM lability and microbial diversity. We further correlated clustering coefficients and average number of neighbors, two network analysis parameters, with different biogeochemical properties of bog and fen samples. Our data revealed that pH is a main driver of SOM decomposition. We hypothesized that the low pH of the bog, driven by Sphagnum-derived organic acids, along with phenolics, could potentially impact substrate quality and concentration and microbial diversity thereby promoting carbon accumulation and low substrate diversity. We further hypothesized that abiotic degradations such as acid catalyzed hydrolysis reactions (i.e., hydrolysis of Lignocellulose, complex carbohydrates, and sugar acids) are happening at a greater rate in bog, freeing bioavailable compounds for microbial utilization and further biotic metabolic pathways. In the fen, the presence of lower molecular weight compounds with higher Gibbs free energy, low DOC, more populated and diverse metabolic transformations, and higher net CH4 and CO2 fluxes and higher pH is indicative of more diverse substrate composition and higher microbial diversity than the bog, suggesting a more active system where biotic pathways are largely upregulated. The correlation observed between the clustering coefficient and the average number of neighbors with δ13C -CH4 values further reflects that organic matter quality and composition has an impact on microbial activity (as revealed by FT-ICR-MS and GC-MS data) measured indirectly through CH4 fluxes.
While many microbial pathways can be inhibited by acidic and anoxic conditions, as observed in the bog, we found evidence of activity of sulfur reducing bacteria. Lower concentrations of sulfate in the bog porewater suggest a rapid turnover of organosulfur compounds acting as TEAs. This is also confirmed by lower CH4 production rates and lower pH in the bog, with low pH likely inhibiting methanogenesis, as well as SRM either consuming H2 and producing CO2 or consuming CH4 and producing CO2 (CH4 + → + HS− + H2O). Due to these results, fen ecosystems that are composed of more diverse and active microbial communities should be more stable with changing environmental conditions (e.g., similar GHG fluxes) whereas bog microbial populations may be more easily affected by a changing climate. It is expected that thaw progression in northern peatlands will rapidly change the water table level, plant communities and nutrient input sources, all contributing to a decline of Sphagnum moss (Norby et al., 2019). This could result in shifts in decomposition, as well as altered contributions from biotic and abiotic pathways of OC toward higher CH4 and CO2 emission which ultimately creates positive climate feedbacks. Therefore, insights into how metabolite diversity and resource heterogeneity relate to the taxonomic and functional diversity of microbial communities allows for aspects of ecosystem stability and functional redundancy to be studied and provides a unique opportunity to understand how microbial communities will respond to climate perturbations.
Data Availability Statement
The original contributions generated for the study are included in the article/Supplementary Materials. The data sets generated from this study are currently available online at https://doi.org/10.17605/OSF.IO/GS2UT.
Author Contributions
RW, SH, and MT designed and performed the experiment and data acquisition. RA and MT did the analysis and interpretation of data for the work. RA, MT, and RW drafted and revised the manuscript with contribution from SH, JF, JC, VR, and SS. All authors contributed to the article and approved the submitted version.
Funding
This study was funded by the Genomic Science Program of the United States Department of Energy (DOE) Office of Biological and Environmental Research (BER), grants DE-SC0004632, DE-SC0010580, and DE-SC0016440. A portion of the research was performed using Environmental Molecular Sciences Laboratory (EMSL), a DOE Office of Science User Facility under proposal number 48467.
Conflict of Interest
HH was employed by the company Bruker Daltonics Inc. after the data was collected and while the manuscript was written.
The remaining authors declare that the research was conducted in the absence of any commercial or financial relationships that could be construed as a potential conflict of interest.
Acknowledgments
We thank the Abisko Scientific Research Station for providing infrastructure for sampling. We thank the IsoGenie 1, 2, and 3 Project Teams (https://isogenie.osu.edu) and the 2012 IsoGenie sampling team for sample collection, particularly Carrie McCalley, and Tyler Logan, as well as the Abisko Scientific Research Station for sampling infrastructure and support.
Supplementary Material
The Supplementary Material for this article can be found online at: https://www.frontiersin.org/articles/10.3389/feart.2020.557961/full#supplementary-material
References
Ahmad, E., and Pant, K. K. (2018). “Lignin conversion: a key to the concept of lignocellulosic biomass-based integrated biorefinery,” in Waste Biorefinery (Elsevier), 409–444. doi: 10.1016/B978-0-444-63992-9.00014-8
Allison, S. D. (2005). Cheaters, diffusion and nutrients constrain decomposition by microbial enzymes in spatially structured environments. Ecol. Lett. 8, 626–635. doi: 10.1111/j.1461-0248.2005.00756.x
Assenov, Y., Ramírez, F., Schelhorn, S.-E., Lengauer, T., and Albrecht, M. (2008). Computing topological parameters of biological networks. Bioinformatics 24, 282–284. doi: 10.1093/bioinformatics/btm554
Bäckstrand, K., Crill, P., Jackowicz-Korczyński, M., Mastepanov, M., Christensen, T., and Bastviken, D. (2010). Annual carbon gas budget for a subarctic peatland, northern Sweden. Biogeosciences 7, 95–108. doi: 10.5194/bg-7-95-2010
Bae, E., Yeo, I. J., Jeong, B., Shin, Y., Shin, K.-H., and Kim, S. (2011). Study of double bond equivalents and the numbers of carbon and oxygen atom distribution of dissolved organic matter with negative-mode FT-ICR MS. Anal. Chem. 83, 4193–4199. doi: 10.1021/ac200464q
Baena, S., Fardeau, M.-L., Labat, M., Ollivier, B., Garcia, J.-L., and Patel, B. K. C. (1998). Desulfovibrio aminophilus sp. nov., a novel amino acid degrading and sulfate reducing bacterium from an anaerobic dairy wastewater lagoon. Syst. Appl. Microbiol. 21, 498–504. doi: 10.1016/S0723-2020(98)80061-1
Barabási, A.-L., and Albert, R. (1999). Emergence of scaling in random networks. Science 286, 509–512. doi: 10.1126/science.286.5439.509
Barabási, A.-L., and Oltvai, Z. N. (2004). Network biology: understanding the cell's functional organization. Nat. Rev. Genet. 5, 101–113. doi: 10.1038/nrg1272
BeMiller, J. N. (1967). “Acid-catalyzed hydrolysis of glycosides,” in Advances in Carbohydrate Chemistry, Vol. 22 (Academic Press), 22, 25–108. doi: 10.1016/S0096-5332(08)60151-4
Bölscher, T., Paterson, E., Freitag, T., Thornton, B., and Herrmann, A. M. (2017). Temperature sensitivity of substrate-use efficiency can result from altered microbial physiology without change to community composition. Soil Biol. Biochem. 109, 59–69. doi: 10.1016/j.soilbio.2017.02.005
Boye, K., Noël, V., Tfaily, M. M., Bone, S. E., Williams, K. H., Bargar, J. R., and Fendorf, S. (2017). Thermodynamically controlled preservation of organic carbon in floodplains. Nat. Geosci. 10, 415–419. doi: 10.1038/ngeo2940
Breitling, R., Ritchie, S., Goodenowe, D., Stewart, M. L., and Barrett, M. P. (2006). Ab initio prediction of metabolic networks using fourier transform mass spectrometry data. Metabolomics 2, 155–164. doi: 10.1007/s11306-006-0029-z
Burgess, K. E. V., Borutzki, Y., Rankin, N., Daly, R., and Jourdan, F. (2017). MetaNetter 2: a Cytoscape plugin for ab initio network analysis and metabolite feature classification. J. Chromatogr. B, 1071, 68–74. doi: 10.1016/j.jchromb.2017.08.015
Burke, E. J., Jones, C. D., and Koven, C. D. (2012). Estimating the permafrost-carbon climate response in the CMIP5 climate models using a simplified approach. J. Clim., 26, 4897–4909. doi: 10.1175/JCLI-D-12-00550.1
Chang, K.-Y., Riley, W. J., Brodie, E. L., McCalley, C. K., Crill, P. M., and Grant, R. F. (2019). Methane production pathway regulated proximally by substrate availability and distally by temperature in a high-latitude mire complex. J. Geophys. Res. Biogeosci. 124, 3057–3074. doi: 10.1029/2019JG005355
Chanton, J. P., Bauer, J. E., Glaser, P. A., Siegel, D. I., Kelley, C. A., Tyler, S. C., et al. (1995). Radiocarbon evidence for the substrates supporting methane formation within northern Minnesota peatlands. Geochim. Cosmochim. Acta 59, 3663–3668. doi: 10.1016/0016-7037(95)00240-Z
Chanton, J. P., Glaser, P. H., Chasar, L. S., Burdige, D. J., Hines, M. E., Siegel, D. I., et al. (2008). Radiocarbon evidence for the importance of surface vegetation on fermentation and methanogenesis in contrasting types of boreal peatlands. Glob. Biogeochem. Cycles 22, 1–11. doi: 10.1029/2008GB003274
Chasar, L. S., Chanton, J. P., Glaser, P. H., Siegel, D. I., and Rivers, J. S. (2000). Radiocarbon and stable carbon isotopic evidence for transport and transformation of dissolved organic carbon, dissolved inorganic carbon, and CH4 in a northern Minnesota peatland. Glob. Biogeochem. Cycles 14, 1095–1108. doi: 10.1029/1999GB001221
Chen, J., Elsgaard, L., van Groenigen, K. J., Olesen, J. E., Liang, Z., Jiang, Y., et al. (2020). Soil carbon loss with warming: new evidence from carbon-degrading enzymes. Glob. Chang. Biol. 26, 1944–1952. doi: 10.1111/gcb.14986
Conrad, R., Schütz, H., and Babbel, M. (1987). Temperature limitation of hydrogen turnover and methanogenesis in anoxic paddy soil. FEMS Microbiol. Ecol. 3, 281–289. doi: 10.1111/j.1574-6968.1987.tb02378.x
Corbett, J. E., Burdige, D. J., Tfaily, M. M., Dial, A. R., Cooper, W. T., Glaser, P. H., et al. (2013). Surface production fuels deep heterotrophic respiration in northern peatlands. Glob. Biogeochem. Cycles 27, 1163–1174. doi: 10.1002/2013GB004677
Corbett, J. E., Tfaily, M. M., Burdige, D. J., Glaser, P. H., and Chanton, J. P. (2015). The relative importance of methanogenesis in the decomposition of organic matter in northern peatlands. J. Geophys. Res. Biogeosci. 120, 280–293. doi: 10.1002/2014JG002797
Cord-Ruwisch, R., and Ollivier, B. (1986). Interspecific hydrogen transfer during methanol degradation by Sporomusa acidovorans and hydrogenophilic anaerobes. Arch. Microbiol. 144, 163–165. doi: 10.1007/BF00414728
Corvasce, M., Zsolnay, A., D'Orazio, V., Lopez, R., and Miano, T. M. (2006). Characterization of water extractable organic matter in a deep soil profile. Chemosphere 62, 1583–1590. doi: 10.1016/j.chemosphere.2005.07.065
de Graaff, M.-A., Classen, A. T., Castro, H. F., and Schadt, C. W. (2010). Labile soil carbon inputs mediate the soil microbial community composition and plant residue decomposition rates. New Phytol. 188, 1055–1064. doi: 10.1111/j.1469-8137.2010.03427.x
Deng, J., Li, C., Frolking, S., Zhang, Y., Backstrand, K., and Crill, P. (2014). Assessing effects of permafrost thaw on C fluxes based on multiyear modeling across a permafrost thaw gradient at Stordalen, Sweden. Biogeosciences 4753–4770. doi: 10.5194/bg-11-4753-2014
Dong, J., and Horvath, S. (2007). Understanding network concepts in modules. BMC Syst. Biol. 1:24. doi: 10.1186/1752-0509-1-24
Estop-Aragonés, C., Knorr, K.-H., and Blodau, C. (2012). Controls on in situ oxygen and dissolved inorganic carbon dynamics in peats of a temperate fen. J. Geophys. Res. Biogeosci. 117:1–14. doi: 10.1029/2011JG001888
Fenner, N., Freeman, C., Lock, M. A., Harmens, H., Reynolds, B., and Sparks, T. (2007). Interactions between elevated CO2 and warming could amplify DOC exports from peatland catchments. Environ. Sci. Technol. 41, 3146–3152. doi: 10.1021/es061765v
Field, J. A., and Lettinga, G. (1992). “Toxicity of tannic compounds to microorganisms,” in Plant Polyphenols. Basic Life Sciences, Vol. 59, eds R. W. Hemingway and P. E. Laks (Boston, MA: Springer). doi: 10.1007/978-1-4615-3476-1_39
Freeman, C., Ostle, N. J., Fenner, N., and Kang, H. (2004). A regulatory role for phenol oxidase during decomposition in peatlands. Soil Biol. Biochem. 36, 1663–1667. doi: 10.1016/j.soilbio.2004.07.012
Fritz, C., Lamers, L. P. M., Riaz, M., van den Berg, L. J. L., and Elzenga, T. J. T. M. (2014). Sphagnum mosses—masters of efficient N-uptake while avoiding intoxication. PLoS One 9:e79991. doi: 10.1371/journal.pone.0079991
Frolking, S., Talbot, J., Jones, M. C., Treat, C. C., Kauffman, J. B., Tuittila, E.-S., et al. (2011). Peatlands in the Earth's 21st century climate system. Environ. Rev. 19, 371–396. doi: 10.1139/a11-014
Fudyma, J., Lyon, J., AminiTabrizi, R., Gieschen, H., Chu, R. K., Hoyt, D. W., et al. (2019). Untargeted Metabolic Profiling of Sphagnum fallax from Boreal Peatlands Identifies Antimicrobial Compounds and Novel Metabolites. AGU Fall Meeting Abstracts 13. Available online at: http://adsabs.harvard.edu/abs/2019AGUFM.B13G2584F
Galand, P. E., Fritze, H., Conrad, R., and Yrjälä, K. (2005). Pathways for methanogenesis and diversity of methanogenic archaea in three boreal peatland ecosystems. Appl. Environ. Microbiol. 71, 2195–2198. doi: 10.1128/AEM.71.4.2195-2198.2005
Gentleman, R. C., Carey, V. J., Bates, D. M., Bolstad, B., Dudoit, S., Ellis, B. C., et al. (2004). Bioconductor: open software development for computational biology and bioinformatics. Genome Biol. 5:R80. doi: 10.1186/gb-2004-5-10-r80
Girisuta, B., Janssen, L. P. B. M., and Heeres, H. J. (2007). Kinetic study on the acid-catalyzed hydrolysis of cellulose to levulinic acid. Ind. Eng. Chem. Res. 46, 1696–1708. doi: 10.1021/ie061186z
Goodwin, S., Giraldo-Gomez, E., Mobarry, B., and Switzenbaum, M. S. (1991). Comparison of diffusion and reaction rates in anaerobic microbial aggregates. Microb. Ecol. 22, 161–174. doi: 10.1007/BF02540221
Granberg, G., Sundh, I., Svensson, B. H., and Nilsson, M. (2001). Effects of temperature, and nitrogen and sulfur deposition, on methane emission from a boreal mire. Ecology, 82, 1982–1998. doi: 10.1890/0012-9658(2001)082[1982:EOTANA]2.0.CO;2
Grandy, A. S., and Neff, J. C. (2008). Molecular C dynamics downstream: the biochemical decomposition sequence and its impact on soil organic matter structure and function. Sci. Total Environ. 404, 297–307. doi: 10.1016/j.scitotenv.2007.11.013
Hájek, T., Ballance, S., Limpens, J., Zijlstra, M., and Verhoeven, J. T. A. (2011). Cell-wall polysaccharides play an important role in decay resistance of Sphagnum and actively depressed decomposition in vitro. Biogeochemistry 103, 45–57. doi: 10.1007/s10533-010-9444-3
Harwood, C. S., and Parales, R. E. (1996). The β-ketoadipate pathway and the biology of self-identity. Annu. Rev. Microbiol. 50, 553–590. doi: 10.1146/annurev.micro.50.1.553
Hättenschwiler, S., and Jørgensen, H. B. (2010). Carbon quality rather than stoichiometry controls litter decomposition in a tropical rain forest. J. Ecol. 98, 754–763. doi: 10.1111/j.1365-2745.2010.01671.x
Hausmann, B., Pelikan, C., Herbold, C. W., Köstlbacher, S., Albertsen, M., Eichorst, S. A., et al. (2018). Peatland Acidobacteria with a dissimilatory sulfur metabolism. ISME J. 12, 1729–1742. doi: 10.1038/s41396-018-0077-1
Herzsprung, P., Hertkorn, N., von Tümpling, W., Harir, M., Friese, K., and Schmitt-Kopplin, P. (2014). Understanding molecular formula assignment of fourier transform ion cyclotron resonance mass spectrometry data of natural organic matter from a chemical point of view. Anal. Bioanal. Chem. 406, 7977–7987. doi: 10.1007/s00216-014-8249-y
Hiller, K., Hangebrauk, J., Jäger, C., Spura, J., Schreiber, K., and Schomburg, D. (2009). Metabolite detector: comprehensive analysis tool for targeted and nontargeted GC/MS based metabolome analysis. Anal. Chem. 81, 3429–3439. doi: 10.1021/ac802689c
Hodgkins, S. B., Chanton, J. P., Langford, L. C., McCalley, C. K., Saleska, S. R., Rich, V. I., et al. (2015). Soil incubations reproduce field methane dynamics in a subarctic wetland. Biogeochemistry 126, 241–249. doi: 10.1007/s10533-015-0142-z
Hodgkins, S. B., Tfaily, M. M., McCalley, C. K., Logan, T. A., Crill, P. M., Saleska, S. R., et al. (2014). Changes in peat chemistry associated with permafrost thaw increase greenhouse gas production. Proc. Natl. Acad. Sci. 111, 5819–5824. doi: 10.1073/pnas.1314641111
Hodgkins, S. B., Tfaily, M. M., Podgorski, D. C., McCalley, C. K., Saleska, S. R., Crill, P. M., et al. (2016). Elemental composition and optical properties reveal changes in dissolved organic matter along a permafrost thaw chronosequence in a subarctic peatland. Geochim. Cosmochim. Acta 187, 123–140. doi: 10.1016/j.gca.2016.05.015
Hopkinson, A. C. (1969). Acid-catalysed hydrolysis of alkyl benzoates. J. Chem. Soc. B Phys. Organic 203–205. doi: 10.1039/j29690000203
IPCC (2007). “Climate change 2007: the physical science basis,” in Contribution of Working Group I to the Fourth Assessment Report of the Intergovernmental Panel on Climate Change, eds S. Solomon, D. Qin, M. Manning, Z. Chen, M. Marquis, K. B. Averyt, M. Tignor and H. L. Miller (Cambridge, New York, NY: Cambridge University Press).
Joabsson, A., and Christensen, T. R. (2001). Methane emissions from wetlands and their relationship with vascular plants: an arctic example. Glob. Chang. Biol. 7, 919–932. doi: 10.1046/j.1354-1013.2001.00044.x
Johansson, T., Malmer, N., Crill, P. M., Friborg, T., Åkerman, J. H., Mastepanov, M., et al. (2006). Decadal vegetation changes in a northern peatland, greenhouse gas fluxes and net radiative forcing. Glob. Chang. Biol. 12, 2352–2369. doi: 10.1111/j.1365-2486.2006.01267.x
Keiluweit, M., Nico, P. S., Kleber, M., and Fendorf, S. (2016). Are oxygen limitations under recognized regulators of organic carbon turnover in upland soils?. Biogeochemistry 127, 157–171. doi: 10.1007/s10533-015-0180-6
Keller, J. K., and Bridgham, S. D. (2007). Pathways of anaerobic carbon cycling across an ombrotrophic-minerotrophic peatland gradient. Limnol. Oceanogr. 52, 96–107. doi: 10.4319/lo.2007.52.1.0096
Keller, J. K., and Takagi, K. K. (2013). Solid-phase organic matter reduction regulates anaerobic decomposition in bog soil. Ecosphere 4:art54. doi: 10.1890/ES12-00382.1
Khadka, B., Munir, T. M., and Strack, M. (2016). Dissolved organic carbon in a constructed and natural fens in the Athabasca oil sands region, Alberta, Canada. Sci. Total Environ. 557–558, 579–589. doi: 10.1016/j.scitotenv.2016.03.081
Kim, S., Kramer, R. W., and Hatcher, P. G. (2003). Graphical method for analysis of ultrahigh-resolution broadband mass spectra of natural organic matter, the van krevelen diagram. Anal. Chem. 75, 5336–5344. doi: 10.1021/ac034415p
Kim, Y.-M., Nowack, S., Olsen, M. T., Becraft, E. D., Wood, J. M., Thiel, V., et al. (2015). Diel metabolomics analysis of a hot spring chlorophototrophic microbial mat leads to new hypotheses of community member metabolisms. Front. Microbiol. 6:209. doi: 10.3389/fmicb.2015.00209
Kind, T., Wohlgemuth, G., Lee, D. Y., Lu, Y., Palazoglu, M., Shahbaz, S., et al. (2009). FiehnLib: Mass spectral and retention index libraries for metabolomics based on quadrupole and time-of-flight gas chromatography/mass spectrometry. Anal. Chem. 81, 10038–10048. doi: 10.1021/ac9019522
Kohl, M., Wiese, S., and Warscheid, B. (2011). “Cytoscape: software for visualization and analysis of biological networks,” in Data Mining in Proteomics. Methods in Molecular Biology (Methods and Protocols),. Vol. 696, eds M. Hamacher, M. Eisenacher, and C. Stephan (Humana Press). doi: 10.1007/978-1-60761-987-1_18
Kokfelt, U., Rosén, P., Schoning, K., Christensen, T. R., Förster, J., Karlsson, J., et al. (2009). Ecosystem responses to increased precipitation and permafrost decay in subarctic Sweden inferred from peat and lake sediments. Glob. Chang. Biol. 15, 1652–1663. doi: 10.1111/j.1365-2486.2009.01880.x
Koven, C. D., Schuur, E. A. G., Schädel, C., Bohn, T. J., Burke, E. J., Chen, X., et al. (2015). A simplified, data-constrained approach to estimate the permafrost carbon–climate feedback. Philos. Trans. R Soc. A Math. Phys. Eng. Sci. 373:20140423. doi: 10.1098/rsta.2014.0423
LaRowe, D. E., and van Cappellen, P. (2011). Degradation of natural organic matter: a thermodynamic analysis. Geochim. Cosmochim. Acta 75, 2030–2042. doi: 10.1016/j.gca.2011.01.020
Liao, J., Cao, X., Zhao, L., Wang, J., Gao, Z., Wang, M. C., et al. (2016). The importance of neutral and niche processes for bacterial community assembly differs between habitat generalists and specialists. FEMS Microbiol. Ecol. 92:fiw174. doi: 10.1093/femsec/fiw174
Limpens, J., Berendse, F., Blodau, C., Canadell, J. G., Freeman, C., Holden, J., et al. (2008). Peatlands and the carbon cycle: From local processes to global implications? A synthesis. Biogeosci. Discussions 5, 1379–1419. doi: 10.5194/bgd-5-1379-2008
Lin, X., Tfaily, M. M., Steinweg, J. M., Chanton, P., Esson, K., Yang, Z. K., et al. (2014). Microbial community stratification linked to utilization of carbohydrates and phosphorus limitation in a boreal peatland at Marcell experimental forest, Minnesota, USA. Appl. Environ. Microbiol. 80, 3518–3530. doi: 10.1128/AEM.00205-14
Longnecker, K., and Kujawinski, E. B. (2016). Using network analysis to discern compositional patterns in ultrahigh-resolution mass spectrometry data of dissolved organic matter. Rapid Commun. Mass Spectrometry 30, 2388–2394. doi: 10.1002/rcm.7719
Lucchese, M., Waddington, J. M., Poulin, M., Pouliot, R., Rochefort, L., and Strack, M. (2010). Organic matter accumulation in a restored peatland: evaluating restoration success. Ecol. Eng. 36, 482–488. doi: 10.1016/j.ecoleng.2009.11.017
Lupascu, M., Wadham, J. L., Hornibrook, E. R. C., and Pancost, R. D. (2012). Temperature sensitivity of methane production in the permafrost active layer at stordalen, Sweden: a comparison with non-permafrost Northern Wetlands. Arct. Antarct. Alp. Res. 44, 469–482. doi: 10.1657/1938-4246-44.4.469
Malmer, N., Johansson, T., Olsrud, M., and Christensen, T. R. (2005). Vegetation, climatic changes and net carbon sequestration in a North-Scandinavian subarctic mire over 30 years. Glob. Chang. Biol. 11, 1895–1909. doi: 10.1111/j.1365-2486.2005.01042.x
McCalley, C. K., Woodcroft, B. J., Hodgkins, S. B., Wehr, R. A., Kim, E.-H., Mondav, R., et al. (2014). Methane dynamics regulated by microbial community response to permafrost thaw. Nature 514, 478–481. doi: 10.1038/nature13798
McGuire, A. D., Anderson, L. G., Christensen, T. R., Dallimore, S., Guo, L., Hayes, D. J., et al. (2009). Sensitivity of the carbon cycle in the Arctic to climate change. Ecol. Monogr. 79, 523–555. doi: 10.1890/08-2025.1
McLatchey, G. P., and Reddy, K. R. (1998). Regulation of organic matter decomposition and nutrient release in a wetland soil. J. Environ. Qual. 27, 1268–1274. doi: 10.2134/jeq1998.00472425002700050036x
Mikutta, R., Turner, S., Schippers, A., Gentsch, N., Meyer-Stüve, S., Condron, L. M., et al. (2019). Microbial and abiotic controls on mineral-associated organic matter in soil profiles along an ecosystem gradient. Sci. Rep. 9:10294. doi: 10.1038/s41598-019-46501-4
Monard, C., Gantner, S., Bertilsson, S., Hallin, S., and Stenlid, J. (2016). Habitat generalists and specialists in microbial communities across a terrestrial-freshwater gradient. Sci. Rep. 6:37719. doi: 10.1038/srep37719
Muscarella, M. E., Boot, C. M., Broeckling, C. D., and Lennon, J. T. (2019). Resource heterogeneity structures aquatic bacterial communities. ISME J. 13, 2183–2195. doi: 10.1038/s41396-019-0427-7
Muyzer, G., and Stams, A. J. M. (2008). The ecology and biotechnology of sulphate-reducing bacteria. Nat. Rev. Microbiol. 6, 441–454. doi: 10.1038/nrmicro1892
Norby, R. J., Childs, J., Hanson, P. J., and Warren, J. M. (2019). Rapid loss of an ecosystem engineer: sphagnum decline in an experimentally warmed bog. Ecol. Evol. 9, 12571–12585. doi: 10.1002/ece3.5722
Novák, M., and Wieder, R. K. (1992). Inorganic and organic sulfur profiles in nine Sphagnum peat bogs in the United States and Czechoslovakia. Water Air Soil Pollut. 65, 353–369. doi: 10.1007/BF00479898
Pester, M., Knorr, K.-H., Friedrich, M. W., Wagner, M., and Loy, A. (2012). Sulfate-reducing microorganisms in wetlands – fameless actors in carbon cycling and climate change. Front. Microbiol. 3:72. doi: 10.3389/fmicb.2012.00072
Qualls, R. G., and Richardson, C. J. (2003). Factors controlling concentration, export, and decomposition of dissolved organic nutrients in the Everglades of Florida. Biogeochemistry 62, 197–229. doi: 10.1023/A:1021150503664
R Core Team (2019). R: A Language and Environment for Statistical Computing. Vienna: R Foundation for Statistical Computing. Available online at: http://www.R-project.org/
Raskin, L., Rittmann, B. E., and Stahl, D. A. (1996). Competition and coexistence of sulfate-reducing and methanogenic populations in anaerobic biofilms. Appl. Environ. Microbiol. 62, 3847–3857. doi: 10.1128/AEM.62.10.3847-3857.1996
Rasmussen, S., Wolff, C., and Rudolph, H. (1995). Compartmentalization of phenolic constituents in sphagnum. Phytochemistry 38, 35–39. doi: 10.1016/0031-9422(94)00650-I
Ravasz, E., Somera, A. L., Mongru, D. A., Oltvai, Z. N., and Barabási, A.-L. (2002). Hierarchical organization of modularity in metabolic networks. Science 297, 1551–1555. doi: 10.1126/science.1073374
Rawat, S. R., Männist,ö, M. K., Bromberg, Y., and Häggblom, M. M. (2012). Comparative genomic and physiological analysis provides insights into the role of Acidobacteria in organic carbon utilization in Arctic tundra soils. FEMS Microbiol. Ecol. 82, 341–355. doi: 10.1111/j.1574-6941.2012.01381.x
Rudolph, H., and Samland, J. (1985). Occurrence and metabolism of sphagnum acid in the cell walls of bryophytes. Phytochemistry 24, 745–749. doi: 10.1016/S0031-9422(00)84888-8
Schink, B. (1997). Energetics of syntrophic cooperation in methanogenic degradation. Microbiol. Mol. Biol. Rev. 61, 262–280. doi: 10.1128/.61.2.262-280.1997
Schuur, E. A. G., Bockheim, J., Canadell, J. G., Euskirchen, E., Field, C. B., Goryachkin, S. V., et al. (2008). Vulnerability of permafrost carbon to climate change: implications for the global carbon cycle. Bioscience 58, 701–714. doi: 10.1641/B580807
Shannon, P., Markiel, A., Ozier, O., Baliga, N. S., Wang, J. T., Ramage, D., et al. (2003). Cytoscape: a software environment for integrated models of biomolecular interaction networks. Genome Res. 13, 2498–2504. doi: 10.1101/gr.1239303
Singleton, C. M., McCalley, C. K., Woodcroft, B. J., Boyd, J. A., Evans, P. N., Hodgkins, S. B., et al. (2018). Methanotrophy across a natural permafrost thaw environment. ISME J. 12, 2544–2558. doi: 10.1038/s41396-018-0065-5
Solomon, S. (2007). Intergovernmental Panel on Climate Change and Intergovernmental Panel on Climate Change. Climate Change 2007: The Physical Science Basis: Contribution of Working Group I To The Fourth Assessment Report of the Intergovernmental Panel on Climate Change. Cambridge University Press.
Sriswasdi, S., Yang, C., and Iwasaki, W. (2017). Generalist species drive microbial dispersion and evolution. Nat. Commun., 8, 1–8. doi: 10.1038/s41467-017-01265-1
Stalheim, T., Ballance, S., Christensen, B. E., and Granum, P. E. (2009). Sphagnan – a pectin-like polymer isolated from Sphagnum moss can inhibit the growth of some typical food spoilage and food poisoning bacteria by lowering the pH. J. Appl. Microbiol. 106, 967–976. doi: 10.1111/j.1365-2672.2008.04057.x
Sutton-Grier, A. E., Keller, J. K., Koch, R., Gilmour, C., and Megonigal, J. P. (2011). Electron donors and acceptors influence anaerobic soil organic matter mineralization in tidal marshes. Soil Biol. Biochem. 43, 1576–1583. doi: 10.1016/j.soilbio.2011.04.008
Svensson, B. H., and Rosswall, T. (1984). In situ methane production from acid peat in plant communities with different moisture regimes in a subarctic mire. Oikos 43, 341–350. doi: 10.2307/3544151
Tang, J., Yurova, A. Y., Schurgers, G., Miller, P. A., Olin, S., Smith, B., et al. (2018). Drivers of dissolved organic carbon export in a subarctic catchment: importance of microbial decomposition, sorption-desorption, peatland and lateral flow. Sci. Total Environ. 622–623, 260–274. doi: 10.1016/j.scitotenv.2017.11.252
Tfaily, M. M., Chu, R. K., Tolić, N., Roscioli, K. M., Anderton, C. R., Paša-Tolić, L., et al. (2015). Advanced solvent based methods for molecular characterization of soil organic matter by high-resolution mass spectrometry. Anal. Chem. 87, 5206–5215. doi: 10.1021/acs.analchem.5b00116
Tfaily, M. M., Chu, R. K., Toyoda, J., Tolić, N., Robinson, E. W., Paša-Tolić, L., et al. (2017). Sequential extraction protocol for organic matter from soils and sediments using high resolution mass spectrometry. Anal. Chim. Acta 972, 54–61. doi: 10.1016/j.aca.2017.03.031
Tfaily, M. M., Cooper, W. T., Kostka, J. E., Chanton, P. R., Schadt, C. W., Hanson, P. J., et al. (2014). Organic matter transformation in the peat column at Marcell experimental forest: humification and vertical stratification. J. Geophys. Res. Biogeosci. 119, 661–675. doi: 10.1002/2013JG002492
Tfaily, M. M., Wilson, R. M., Cooper, W. T., Kostka, J. E., Hanson, P., and Chanton, J. P. (2018). Vertical stratification of peat pore water dissolved organic matter composition in a peat bog in Northern Minnesota. J. Geophys. Res. Biogeosci. 123, 479–494. doi: 10.1002/2017JG004007
Thormann, M. N. (2006). Diversity and function of fungi in peatlands: a carbon cycling perspective. Can. J. Soil Sci. 86, 281–293. doi: 10.4141/S05-082
Timell, T. E. (1967). Recent progress in the chemistry of wood hemicelluloses. Wood Sci. Technol. 1, 45–70. doi: 10.1007/BF00592255
Tolić, N., Liu, Y., Liyu, A., Shen, Y., Tfaily, M. M., Kujawinski, E. B., et al. (2017). Formularity: software for automated formula assignment of natural and other organic matter from ultrahigh-resolution mass spectra. Anal. Chem. 89, 12659–12665. doi: 10.1021/acs.analchem.7b03318
Urban, N. R., Eisenreich, S. J., and Grigal, D. F. (1989). Sulfur cycling in a forested Sphagnum bog in northern Minnesota. Biogeochemistry 7, 81–109. doi: 10.1007/BF00004123
van Breemen, N. (1995). How Sphagnum bogs down other plants. Trends Ecol. Evol. (Amst). 10, 270–275. doi: 10.1016/0169-5347(95)90007-1
Van Krevelen, D. W. (1950). Graphical-statistical method for the study of structure and reaction processes of coal. Fuel 29, 269–284.
Wang, Y., Gabbard, H. D., and Pai, P. (1991). Inhibition of acetate methanogenesis by phenols. J. Environ. Eng. 117. doi: 10.1061/(ASCE)0733-9372(1991)117:4(487)
Ward, S. E., Bardgett, R. D., McNamara, N. P., and Ostle, N. J. (2009). Plant functional group identity influences short-term peatland ecosystem carbon flux: Evidence from a plant removal experiment. Funct. Ecol. 23, 454–462. doi: 10.1111/j.1365-2435.2008.01521.x
Watts, D. J., and Strogatz, S. H. (1998). Collective dynamics of ‘small-world’ networks. Nature 393, 440–442. doi: 10.1038/30918
Whiting Chanton (1992). Plant-Dependent CH4 Emission In A Subarctic Canadian Fen—Whiting−1992—Global Biogeochemical Cycles—Wiley Online Library. (n.d.). Available online at: https://agupubs.onlinelibrary.wiley.com/doi/abs/10.1029/92GB00710 (accessed April 30, 2020).
Wickham, H. (2016). ggplot2: Elegant Graphics for Data Analysis. New York, NY: Springer-Verlag. doi: 10.1007/978-3-319-24277-4
Williams, C. J., Shingara, E. A., and Yavitt, J. B. (2000). Phenol oxidase activity in peatlands in New York state: response to summer drought and peat type. Wetlands 20, 416–421. doi: 10.1672/0277-5212(2000)020[0416:POAIPI]2.0.CO;2
Wilson, R. M., and Tfaily, M. M. (2018). Advanced molecular techniques provide new rigorous tools for characterizing organic matter quality in complex systems. Rev. Geophys. 1790–1795. doi: 10.1029/2018JG004525
Wilson, R. M., Tfaily, M. M., Rich, V. I., Keller, J. K., Bridgham, S. D., Zalman, C. M., et al. (2017). Hydrogenation of organic matter as a terminal electron sink sustains high CO2:CH4 production ratios during anaerobic decomposition. Org. Geochem. 112, 22–32. doi: 10.1016/j.orggeochem.2017.06.011
Woodcroft, B. J., Singleton, C. M., Boyd, J. A., Evans, P. N., Emerson, J. B., Zayed, A. A. F., et al. (2018). Genome-centric view of carbon processing in thawing permafrost. Nature 560, 49–54. doi: 10.1038/s41586-018-0338-1
Worm, B., Lotze, H. K., Hillebrand, H., and Sommer, U. (2002). Consumer versus resource control of species diversity and ecosystem functioning. Nature 417, 848–851. doi: 10.1038/nature00830
Keywords: permafrost, peatlands, soil organic matter, fen, bog, mass spectrometry, sphagnum
Citation: AminiTabrizi R, Wilson RM, Fudyma JD, Hodgkins SB, Heyman HM, Rich VI, Saleska SR, Chanton JP and Tfaily MM (2020) Controls on Soil Organic Matter Degradation and Subsequent Greenhouse Gas Emissions Across a Permafrost Thaw Gradient in Northern Sweden. Front. Earth Sci. 8:557961. doi: 10.3389/feart.2020.557961
Received: 01 May 2020; Accepted: 13 August 2020;
Published: 28 September 2020.
Edited by:
Matthias Peichl, Swedish University of Agricultural Sciences, SwedenReviewed by:
Jinyun Tang, Lawrence Berkeley National Laboratory, United StatesBirgit Wild, Stockholm University, Sweden
Copyright © 2020 AminiTabrizi, Wilson, Fudyma, Hodgkins, Heyman, Rich, Saleska, Chanton and Tfaily. This is an open-access article distributed under the terms of the Creative Commons Attribution License (CC BY). The use, distribution or reproduction in other forums is permitted, provided the original author(s) and the copyright owner(s) are credited and that the original publication in this journal is cited, in accordance with accepted academic practice. No use, distribution or reproduction is permitted which does not comply with these terms.
*Correspondence: Malak M. Tfaily, dGZhaWx5QGFyaXpvbmEuZWR1