- 1Department of Environmental Systems Science, Swiss Federal Institute of Technology, ETH Zürich, Zurich, Switzerland
- 2Department of Plant and Environmental Sciences, Faculty of Science, University of Copenhagen, Taastrup, Denmark
- 3European Commission, Joint Research Centre, Ispra, Italy
- 4Department of Knowledge Technologies, Jozef Stefan Institute, Ljubljana, Slovenia
- 5Department for Soil Health and Plant Nutrition, Austrian Agency for Health and Food Safety, Vienna, Austria
- 6Natural Resources Management & Environmental Sciences, California State University, San Luis Obispo, CA, United States
- 7Department of Soil Quality, Wageningen University & Research, Wageningen, Netherlands
Soils perform many functions that are vital to societies, among which their capability to regulate global climate has received much attention over the past decades. An assessment of the extent to which soils perform a specific function is not only important to appropriately value their current capacity, but also to make well-informed decisions about how and where to change soil management to align the delivered soil functions with societal demands. To obtain an overview of the capacity of soils to perform different functions, accurate and easy-to-use models are necessary. A problem with most currently-available models is that data requirements often exceed data availability, while generally a high level of expert knowledge is necessary to apply these models. Therefore, we developed a qualitative model to assess how agricultural soils function with respect to climate regulation. The model is driven by inputs about agricultural management practices, soil properties and environmental conditions. To reduce data requirements on stakeholders, the 17 input variables are classified into either (1) three classes: low, medium and high or (2) the presence or absence of a management practice. These inputs are combined using a decision tree with internal integration rules to obtain an estimate of the magnitude of N2O emissions and carbon sequestration. These two variables are subsequently combined into an estimate of the capacity of a soil to perform the climate regulation function. The model was tested using data from long-term field experiments across Europe. This showed that the model is generally able to adequately assess this soil function across a range of environments under different management practices. In a next step, this model will be combined with models to assess other soil functions (soil biodiversity, primary productivity, nutrient cycling and water regulation and purification). This will allow the assessment of trade-offs between these soil functions for agricultural land across Europe.
Introduction
Soils in agroecosystems play an important role regulating the global climate as they have contributed substantially to the increase in atmospheric greenhouse gases such as carbon dioxide (CO2) and nitrous oxide (N2O) during the past centuries (Ciais et al., 2013; Le Quéré et al., 2018). The conversion of soil organic carbon (SOC) to CO2 in agroecosystems mainly occurs as a consequence of the conversion of native vegetation to arable land. This process results in an average loss of topsoil organic carbon (OC) of ca. 32 ± 20 % in temperate regions (Poeplau et al., 2011). N2O is mainly emitted as a consequence of microbial transformations of fertilizer containing reactive nitrogen (N) that is applied on agricultural land. N2O emissions occur both directly after application on the field or indirectly, after reactive N has been transferred to other ecosystems, as nitrate () losses or ammonia (NH3) emissions (Galloway et al., 2003; Zhou et al., 2017). These emissions are not trivial, as greenhouse gas emissions from agroecosystems have constituted ca. 11.2 % of total emissions (mainly as N2O and CH4), while the share resulting from land use changes associated with food production was ca. 10.0 % in 2010 (mainly as CO2) (Tubiello et al., 2015).
The regulation of the global climate is thus an important ecosystem function that soils perform through carbon storage and a reduction of greenhouse gas emissions, referred to as the climate regulation soil function. This soil function is defined here as the capacity of a soil to reduce the negative impact of increased greenhouse gas emissions on climate, among which its capacity to store carbon (C) and to minimize N2O emissions. In line with the recognition of the importance of the climate regulating function of soils (Schulte et al., 2014), the 4 per mille Initiative: Soils for Food Security and Climate has been proposed (https://www.4p1000.org/). This initiative aims to increase the amount of OC in soils around the world, not only to reduce atmospheric CO2 concentrations, but also to improve food production and mitigate soil degradation. The 4 per mille initiative has received many criticisms (Minasny et al., 2018), mainly related to the magnitude of achievable gains in SOC over the coming decades and social and economic constraints, despite the many benefits associated with increasing SOC stocks (Paustian et al., 2016; Chabbi et al., 2017; Soussana et al., 2017).
Although uncertainties about the achievable magnitude of future soil C sequestration exist, many long-term experiments (LTEs) have shown that the consistent application of certain management practices does increase the OC content of agricultural soils (Paustian et al., 1997; Ogle et al., 2005; Minasny et al., 2017; Chenu et al., 2018). An increase in SOC stocks is achievable through management practices that increase C inputs to the soil, such as the addition of organic fertilizers (Haynes and Naidu, 1998; Sandén et al., 2018), the incorporation of crop residues in the soil after harvest (Lehtinen et al., 2014) or the cultivation of cover crops (Poeplau and Don, 2015). In contrast, practices that aim to reduce SOC losses, such as no-till, generally lead to a mere redistribution of OC along the soil profile while not significantly increasing total SOC stocks (Luo et al., 2010; Powlson et al., 2014). The application of no-till combined with an increase in C inputs to the soils has, however, been shown to be an effective strategy to increase the SOC content (Luo et al., 2010; Virto et al., 2012). When discussing changes in SOC stocks through changes in management practices, two important considerations have to be taken into account (Minasny et al., 2017; Chenu et al., 2018). First, the efficiency with which SOC stocks are increased is negatively correlated to the initial SOC stock. Second, the rate of the increase in SOC stocks is highest in the first years after the initiation of improved management practices and decreases substantially in the following years or decades. Both effects are a consequence of the maximum amount of OC that can be stored in mineral soils, as a function of the applied management (Six et al., 2002; Stewart et al., 2007; Castellano et al., 2015).
Although increasing the OC content of soils can lead to a net removal of CO2 from the atmosphere, trade-offs with N2O emissions should be taken into account, as these can reduce or completely offset the climate mitigation effect of certain management practices (Gao et al., 2018). For example, while the application of farmyard manure (FYM) can significantly increase topsoil OC stocks (Bai et al., 2018; Sandén et al., 2018, 2019b), an accompanying increase in N2O emissions can offset this benefit (Zhou et al., 2017). Similar observations have been made for crop rotations including cover crops, which can increase topsoil OC stocks significantly (Poeplau and Don, 2015), while N2O emissions can increase substantially when their biomass is decomposed (Basche et al., 2014). The same pattern has been observed in a modeling study at the European scale by Lugato et al. (2018), who found that the climate mitigation obtained by increasing SOC stocks can be canceled out by increased N2O emissions due to changing management practices in the long term. Also the practice of crop residue incorporation has been shown to increase losses of reactive N in the form of N2O and NH3, despite its positive effect on the SOC content of upland soils (Xia et al., 2018). In contrast, also positive interactions are possible. For example, the potential of cover crops to uptake nitrate () can substantially decrease indirect N2O emissions and therefore increase their climate mitigation potential (Tonitto et al., 2006; Basche et al., 2014). These trade-offs thus show that the climate regulation potential of soils in agroecosystems depends on both C sequestration and losses of reactive N species, such as N2O, NH3, and .
A thorough evaluation of the climate regulation function of soils in agroecosystems therefore requires a holistic assessment of the effect of different management practices on this soil function (Vogel et al., 2018). In addition, interactions between different management practices, the effect of local environmental conditions and trade-offs between C sequestration and losses of reactive N need to be taken into account. As a consequence, evaluating the climate regulation function of soils is not straightforward, with models generally being used to achieve this goal. Ideally, these models should assess different aspects of this soil function for a given combination of environmental conditions and management practices based on knowledge of the relevant processes. In addition, these models should be simple enough to be applicable by non-expert users, while providing reliable simulations based on the limited amount of data that is generally at hand.
A problem associated with existing models is that data requirements often exceed available data, which limits their application to a regional or national scale. For example, the 1D-ICZ (one-dimensional Integrated Critical Zone) model quantifies four different soil functions (biomass production, C and nutrient sequestration, water filtration and biodiversity) using process-based simulations at the soil profile scale (Giannakis et al., 2017), with a focus on the simulation of temporal changes in soil structure and aggregate dynamics. Although these types of models greatly improve our ability to use process understanding to quantify different soil functions, the simulation of these processes requires a large amount of data, while the range of management practices on which this model has been tested is currently still limited (Kotronakis et al., 2017). Other existing tools that have been developed to quantify different soil functions have been calibrated for North America [Fieldprint calculator (https://calculator.fieldtomarket.org), Comet-Farm (http://cometfarm.nrel.colostate.edu/) and HOLOS (Little et al., 2008)] or the global scale (Coolfarm (https://coolfarmtool.org/), which may limit their applicability to European agroecosystems.
To overcome these problems, we developed a relatively simple, qualitative model to assess the climate regulation potential of agricultural soils that can be coupled to similarly structured models assessing other soil functions. This qualitative model aims to inform different stakeholders, such as farmers or farm advisors, about the directional effects of combinations of different agricultural practices on the climate regulation capacity of mineral, non-peatland, agricultural soils. The aim of this tool is not to provide a detailed quantitative assessment of different fluxes of greenhouse gases from agricultural soils, as other tools are available to achieve this [e.g., DayCent; (Parton et al., 1998) or DNDC; (Li et al., 1992)]. Rather, this tool provides the user with qualitative information regarding the capacity of an agricultural soil to perform the climate regulation function. In addition, the aim of this tool is to increase awareness among model users about the multifunctionality of agricultural soils, and the existence of important trade-offs between the performance of these soil functions as a consequence of the applied management. The model has been developed in the framework of the Horizon 2020 Landmark project, which aims to quantify the current and potential supply of different soil functions from farm scale application to the scale of Europe. These are (i) primary productivity, (ii) water regulation and purification, (iii) soil biodiversity and habitat provision, (iv) nutrient cycling and provision and (v) climate regulation. To achieve this goal, decision support tools for every soil function have been developed. These tools have been brought together to assess the trade-offs between different soil functions for a given set of management practices across Europe (Debeljak et al., 2019). The main aims of this paper are (1) to present the model developed to assess the climate regulation function of agricultural soils and (2) to test this model based on available data from long-term field experiments across Europe.
Materials and Methods
Model Description
The model has been developed based on the rationale that it should make a reliable assessment of the climate regulation function of agricultural soils based on data that is readily available. It is built using multi-criteria decision analyses, in particular the DEX (Decision Expert) integrative methodology for qualitative decision modeling (Bohanec and Rajkovič, 1990; Bohanec et al., 2013; Bohanec, 2017). Using this methodology, the main decision problem (assessing the climate regulation soil function) is broken down into smaller, less complex sub-problems in a hierarchical way. The main concept (the climate regulation soil function) is at the top of the hierarchy and is related to lower-level attributes on which it depends. These attributes represent the characteristics of the system, which are environmental variables, soil properties and management practices. The attributes on the lowest level of the hierarchy are the basic attributes. The intermediate attributes are obtained using integration rules, which also determine how the attributes are combined into the final climate regulation function.
The developed model has two distinct parts that separately simulate (i) C sequestration and (ii) N2O emissions, both direct (from soils) and indirect (originated from NH3 volatilization/deposition or leaching) (Figure 1), as presented in more detail in the following sections. Although the term ‘C sequestration’ is generally used to describe changes in the SOC stock that result from a net transfer of C from the atmosphere to the soil (Powlson et al., 2011; Chenu et al., 2018), this term is used here in the broad sense of the capacity of a soil to store C. The assessments are made for the upper 0.3 m of agricultural soils. If one aims to evaluate the current climate regulation function of a soil, input data should represent the average environmental conditions and management practices for the past 5 years. This time span was chosen to account for previous management practices, while avoiding problems with providing the average management for a longer period of time, which might be characterized by multiple changes in management practices. If the aim is to evaluate the effect of potential future management practices, input data should represent the current conditions with the desired change in management practices adjusted accordingly, with predictions being made for the medium term (<10 years). It is noted that the model does not account for potential legacy effects from a previous land use. Consequently, the model only provides information about the effect of the applied management practices on the climate regulation soil function. In the model, all attributes are classified into the categorical variables “low,” “medium,” and “high,” or “yes” and “no.” Thresholds to classify quantitative variables into these categories were agreed upon by the members of the Landmark project, as shown in Table 1. The model result, i.e., how well a soil performs the climate regulation function, is similarly expressed as “low,” “medium,” or “high.” The latter categories are not explicitly coupled to a quantitative value, due to the lack of a quantitative definition of this soil function. The model has been developed to be applicable to agroecosystems throughout Europe, regardless of crop type. More information about how different management practices are translated into model input variables is provided in Table 1.
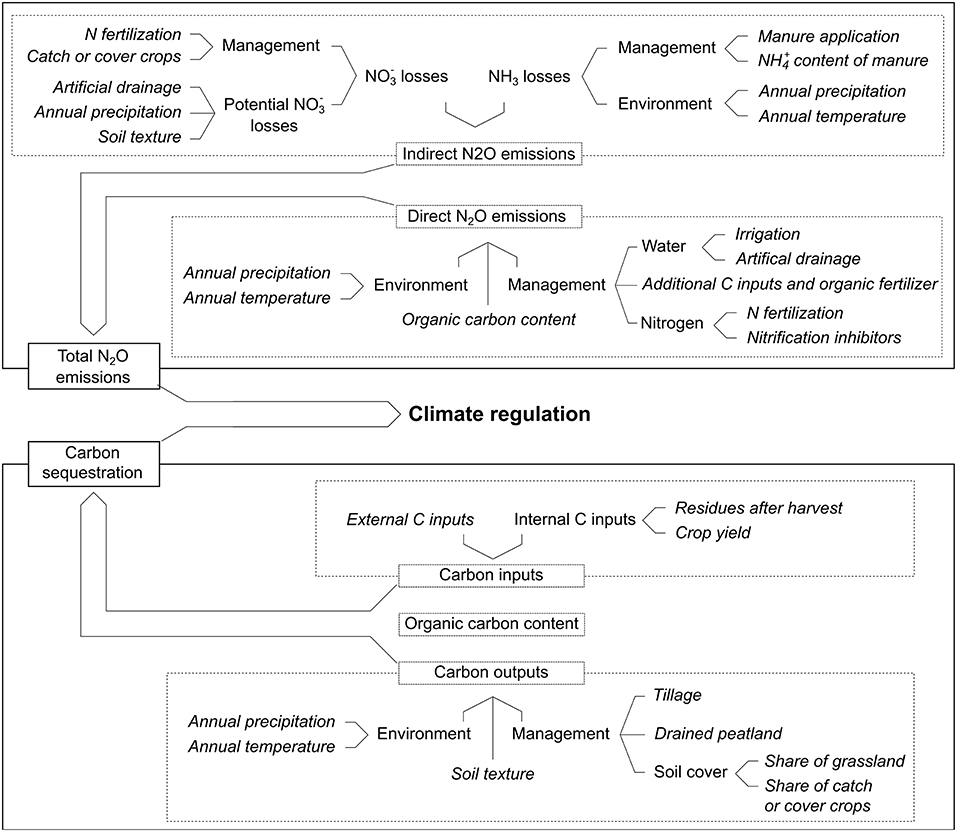
Figure 1. Structure of the model assessing the climate regulation soil function. Input attributes (shown in italic) are classified into “low,” “medium,” and “high,” or “yes” and “no,” as shown in Table 1. Internal integration rules determine how input attributes are combined into the final climate regulation function, which is similarly classified into “low,” “medium,” and “high”.
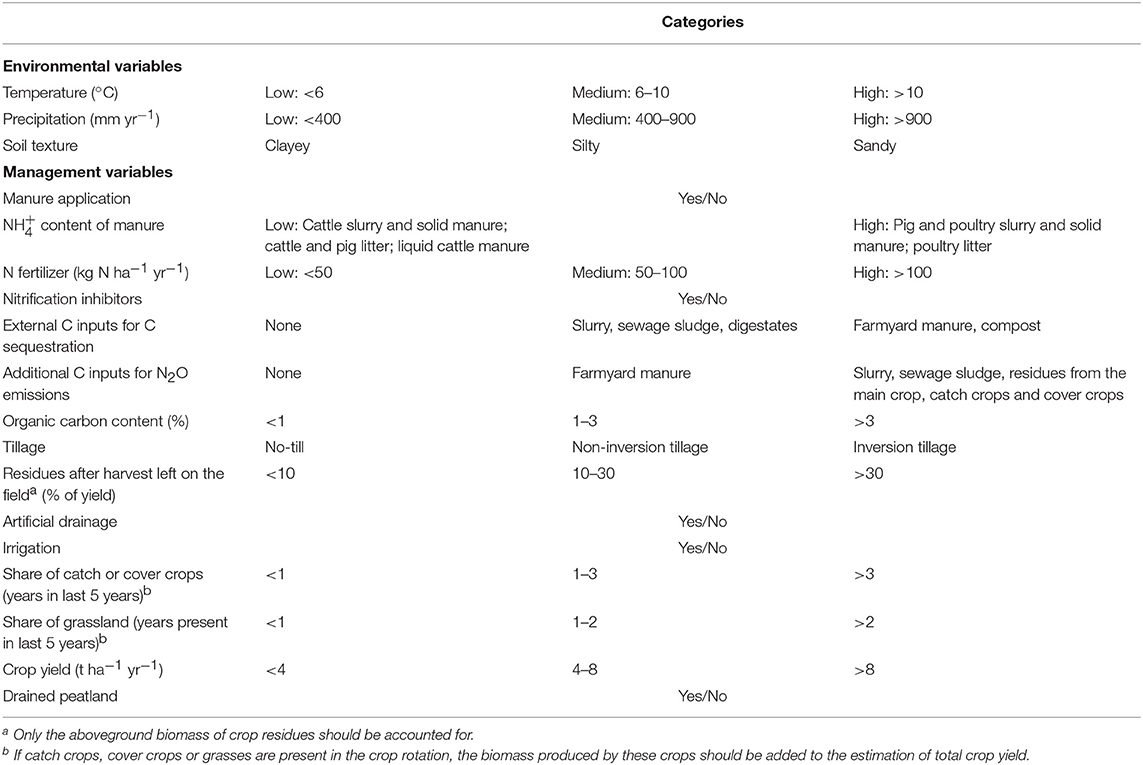
Table 1. Thresholds used to categorize input variables into “low,” “medium,” and “high,” or “yes” or “no”. Input variable should reflect the average management practices for the past 5 years, while temperature and precipitation inputs should be based on climatic data (30-year average).
Nitrous Oxide Emissions
The model simulates direct and indirect N2O emissions separately. Direct emissions are considered as emissions occurring in-situ in the field, as a result of the nitrification and denitrification of mineral N derived from applied fertilizer or mineralized organic N. The two sources of reactive N in the model are (i) mineral N fertilizer and (ii) additional C inputs and organic fertilizers (e.g., farmyard manure, slurry and plow-in crop residues). Together with attributes influencing the moisture content of the soil (irrigation and artificial drainage), the magnitude of N inputs determines how management practices influence direct N2O emissions. The integration rules that determine how the rate of N inputs affects total N2O emissions are chosen so that the N2O emissions increase with increasing rates of N application. This is in line with empirical observations, showing that once the amount of applied N exceeds the crop demand, N2O emissions increase exponentially with every additional unit of applied N (Bouwman et al., 2002; Hoben et al., 2011; Shcherbak et al., 2014). The calculated magnitude of N2O emissions is further constrained by climatic conditions [N2O emissions are enhanced by high values of average annual temperature and precipitation, with a higher weight assigned to precipitation (Groffman and Tiedje, 1991; Barnard et al., 2006; Butterbach-Bahl et al., 2013)] and the SOC concentration (high OC concentrations increase N2O production by providing a substrate for denitrifying bacteria).
Indirect N2O emissions are the result of the management applied on the field, but occur at downstream locations due to cascading effects (Syakila and Kroeze, 2011; Butterbach-Bahl et al., 2013). The two simulated sources of indirect N2O emissions occur after leaching of nitrate () to groundwater, or after NH3 emissions that are deposited back on the soil surface (Sommer and Hutchings, 2001; Galloway et al., 2003). losses are enhanced by the application of N fertilizer and reduced by the presence of catch or cover crops (Hansen and Djurhuus, 1997; Di and Cameron, 2002; Kirchmann et al., 2002). The potential for losses to actually occur is further determined by the presence or absence of artificial drainage, the rate of precipitation and soil texture (Di and Cameron, 2002). The calculation of NH3 losses is driven by whether or not manure is applied and its content (Sommer and Hutchings, 2001), while being enhanced by high average annual temperature and precipitation. Attributes that are known to influence N2O emissions but are not present in the model include the effect of soil pH and different types of (i) compost, (ii) cover crops, (iii) tillage and (iv) irrigation, as discussed in section Discussion.
Carbon Sequestration
The model evaluates the extent to which a soil sequesters C based on (i) C inputs, (ii) C losses, and (iii) the OC concentration of the soil. This soil function is assessed based on the following integration rules: (i) a soil that loses C (outputs > inputs) receives a low value, while (ii) a soil with an increasing C content (inputs > outputs) receives a high value. When (iii) the C stock is in equilibrium (inputs = outputs), the assigned value equals the value of the C concentration. The rationale behind this last rule is that a soil with a high OC content performs the climate regulation soil function better than a soil with a low OC content. It is noted that the model is not designed to make predictions of the OC concentration of the soil. Instead, it evaluates the capacity of a soil to perform the climate regulation soil function while using the SOC concentration as a model input. In addition, the model has not been designed to account for legacy effects on the current SOC concentration, e.g., caused by a recent previous land use. Therefore, the model assumes that the soil has been under cultivation for a timespan of multiple decades. In the model, inputs of C are divided into external and internal inputs (Table 1). The former can consist of e.g., farmyard manure or slurry, while the latter consist of the amount of crop residues (as a percentage of the total yield) that is left on the field after harvest and the mean annual crop yield. C outputs are evaluated based on a combination of soil texture, environmental conditions (mean annual precipitation and temperature) and management practices. These include tillage intensity, the share of grasslands and cover crops in the crop rotation and whether or not the soil is a drained peatland. To correctly represent the effect of cover crops or grass in the model, in addition to indicating the number of years a cover crop or grass was present in the past 5 years, an estimate of its biomass has to be added to the mean annual net primary productivity. Attributes that are known to affect C sequestration but are not present in the model include the effect of N fertilizer application, biochar application and soil pH, as discussed in section Discussion.
The Climate Regulation Soil Function
The final climate regulation soil function is determined based on the combination of the magnitude of N2O emissions and C sequestration. The integration rules that define the magnitude of the climate regulation soil function are shown in Table 2. These are a logical combination of the simulated values for N2O emissions and C sequestration, while a higher weight is given to N2O emissions because N2O is a much more potent greenhouse gas than CO2 and often dominates the GHG balance of agroecosystems. For example, a medium value for C sequestration and a high value for N2O emissions lead to a low overall climate regulation value.
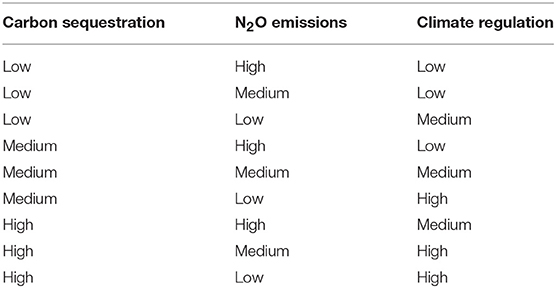
Table 2. Integration rules used to classify the climate regulation soil function as “low,” “medium,” or “high” based on the determined magnitude of N2O emissions and carbon sequestration.
Sensitivity Analysis
A sensitivity analysis was performed to assess the extent to which the results of the model are influenced by changes in management practices. This was done separately for the simulated magnitude of N2O emissions and C sequestration, as the combinations of both attributes are straightforward to interpret (Table 2). The assessment of how the magnitude of C sequestration is affected by changes in management practices was done relative to a reference management. This reference management was chosen not to favor C sequestration, resulting in low C inputs, high C outputs and therefore low C sequestration (Figure 3). The model sensitivity was assessed by gradually changing different management practices which are expected to increase C sequestration. The sensitivity of the N2O component of the model was assessed using a similar procedure. Here, the reference management was chosen to favor N2O emissions. Gradually, one, two or three management practices were changed to reduce N2O emissions. In addition, the effect of average temperature and precipitation on C sequestration and N2O emissions was assessed for soils with different textures.
Model Testing Using Long-Term Field Experiments
An assessment of the accuracy of the model was made by simulating agricultural soils in long-term experiments (LTEs) and comparing the model outcomes to reported changes in N2O emissions or C sequestration. LTEs were chosen because they facilitate the assessment of a range of different management practices on the component parts of the climate regulation function on a decadal timescale. The geographical location of the LTEs was limited to Europe, in line with the intended geographical extent of model application. For this purpose, the database constructed by Sandén et al. (2018) was used. This database contains publications on 251 European LTEs in which the effect of alternative management practices on soil quality were assessed. From these, 78 LTEs reported on changes in SOC stocks and 40 reported on changes in N2O emission or leaching. A large portion of these LTEs studied the effect of tillage (n = 18 for N2O, n = 33 for C stocks). As the effect of tillage on these soil properties has been summarized in multiple meta-studies, it was chosen not to run all these studies separately by the model, but instead, model performance was assessed based on these meta-analyses (Luo et al., 2010; Powlson et al., 2014; Meurer et al., 2018). After excluding studies on the effect of tillage and studies using parameters that are not simulated by the model, the number of studies that was retained to test the model was 6 for N2O emissions, 2 for leaching and 12 for changes in SOC stocks. This includes one additional study on leaching (Hansen and Djurhuus, 1997) and one on C sequestration (Spiegel et al., 2018) that were added to the dataset.
The aim of this exercise was to test if the model is able to correctly predict the climate regulation function of (i) a soil with a constant management through time and (ii) a soil which experiences a change in management practices. For the first purpose, the climate regulation function of the control treatments of the LTEs were predicted and compared to reported values. As it was assumed that the OC concentration of the control treatments was constant through time (C inputs equal C outputs), the simulation of the control treatments was used to test if this equilibrium was predicted correctly by the model. Therefore, the classified value of C sequestration by the control treatments was equal to the SOC concentration of these treatments (see section carbon sequestration). For the second purpose, the treatment studied in the LTEs was simulated and compared to the reported change in the soil function. To this end, the results of the LTEs had to be classified into low, medium and high. This was done based on the results reported in the articles presenting the outcomes of the LTEs. The outcomes of the LTEs that were used to validate the C sequestration part of the model were classified based on differences in the SOC concentration between the controls and treatments, as reported in Table 3. The outcomes of the LTEs used to validate the N2O part of the model were classified based on the reported differences in N2O emissions and leaching between controls and treatments, as reported in Table S1. For N2O, the intensity of emissions for the control situation and the change in management practices was classified (i.e., into low, medium or high) based on the data provided. The classification of the magnitude of N2O emissions for the treatment was based on the relative change in N2O emissions. For example, if the control management (without N fertilizer application) led to low N2O emissions, a low value was assigned. If the treatment (which included N fertilizer application) resulted in a substantial increase in N2O emissions, a value higher than low was assigned. Thus, if the model predicted N2O emissions for the treatment to be medium or high, this was assumed to be a correct model outcome. Also for C sequestration, the classification of the outcomes of the LTEs was based on the data provided in the articles. This information was used to derive the direction of change in SOC concentration as a consequence of the change in management practices, according to the rules outlined in section carbon sequestration. It is noted that term “control treatment” is used to refer to the treatments in the LTEs to which changes in management practices are compared, while the term “reference management” is used to refer to the management practices to which the outcomes of the sensitivity analyses are compared.
Results
Sensitivity Analysis
Carbon Sequestration
The environmental variables (precipitation and temperature) and the soil texture have a substantial effect on the predicted magnitude of C sequestration (Figure 2). On average, higher C sequestration is predicted for clayey soils, while this decreases for soils with a coarser texture. Furthermore, the predicted C sequestration is highest for environments with a low temperature and precipitation and decreases when temperature and precipitation increase simultaneously.
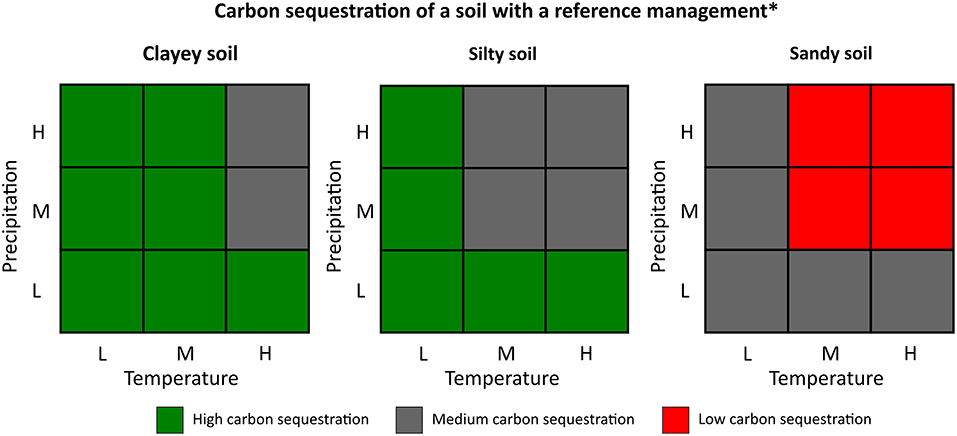
Figure 2. Example of the effect of temperature and precipitation on the predicted C sequestration function of soils with different textures. *Reference management: a mineral soil with medium values for yield, OC content, share of cover crops, external C inputs and residues after harvest; low share of grassland and non-inversion tillage.
The results of the sensitivity analysis of the C sequestration part of the model are shown in Figure 3. Increasing the amount of C inputs to the soil (through crop residue incorporation or the addition of external C inputs, not through an increase in yield) from low to medium does not improve the predicted value for C sequestration. When high values for these C inputs are chosen, the predicted value for C sequestration increases from low to medium. When crop residue incorporation and the addition of external C inputs are both at high levels, similar outcomes are obtained. Consequently, for the considered combination of environmental conditions and management practices, only increasing the amount of C inputs while C losses remain high increases the magnitude of C sequestration from low to medium, but not to high. Similarly, the adoption of minimum tillage or no-till does not lead to an increased prediction of C sequestration. As a consequence, only reducing C outputs, while C inputs remain low, does not lead to high predictions of C sequestration. When a management practice that increases C inputs and one that decreases C outputs are jointly applied, medium or high values for C sequestration are predicted. Improving multiple management practices together thus consistently leads to a high predicted value for C sequestration.
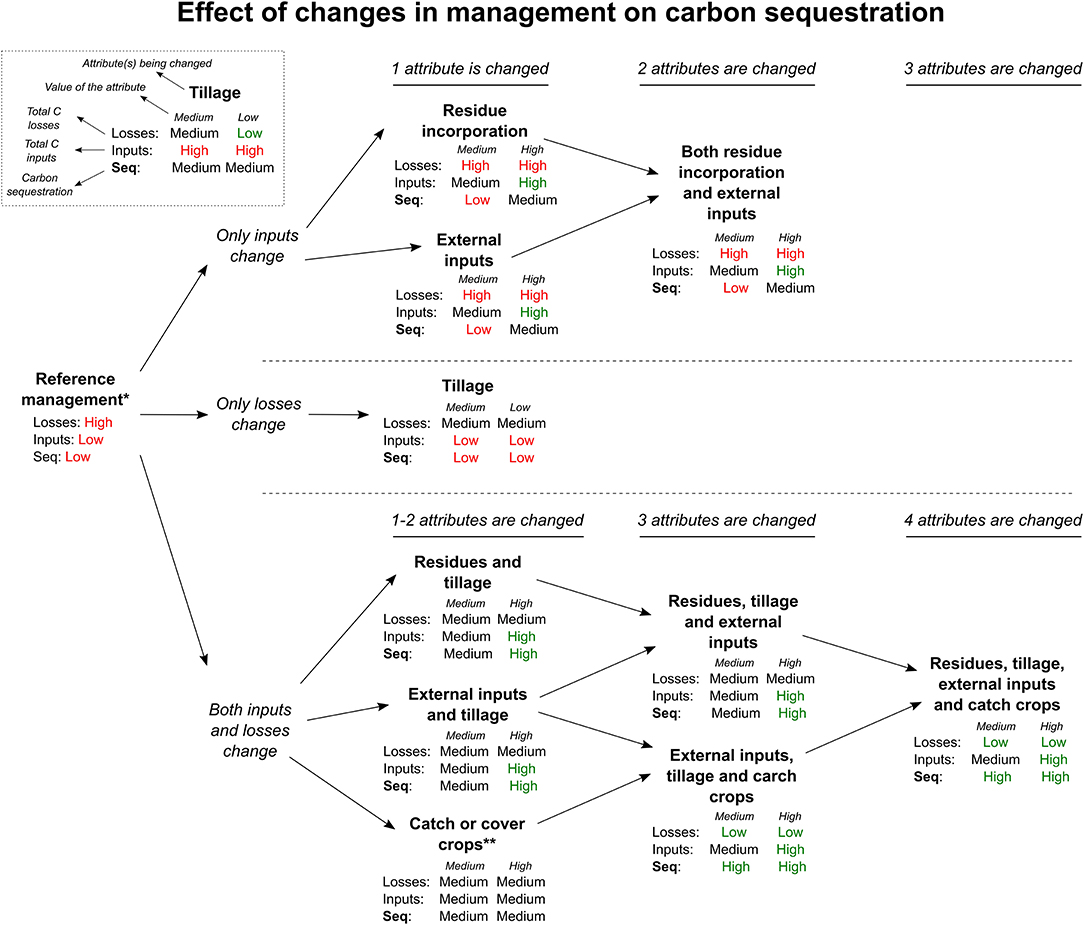
Figure 3. Sensitivity analysis of the C sequestration part of the model (Figure 1), based on variations in management practices. The model outcomes are compared to a *reference management: a silty mineral soil with a low yield and medium values for temperature, precipitation and C content, no drainage and management practices that do not favor C sequestration, leading to low C inputs and high C losses. The inset in the upper left corner shows the legend. ** The effect of catch or cover crops is simulated by a reduction of C losses, while an increase in NPP accounts for increased C inputs.
N2O Emissions
The effect of precipitation and soil texture on predicted indirect N2O losses via and NH3 losses is shown in Figure 4. For the particular combination of management practices chosen (see Figure 4), low values of precipitation do always lead to medium predicted values for losses, while medium and high precipitation rates lead to higher losses. On a clayey soil, only high values of precipitation lead to high losses, while coarser soils result in high losses for medium and high precipitation values. This is in line with studies showing that higher losses occur in sandy vs. clayey soils (Gaines and Gaines, 1994; Vinten et al., 1994). Predictions of low NH3 losses are only obtained at low rates of precipitation, while high losses are consistently predicted for medium and high rates of precipitation. In contrast, temperature and precipitation do not have a marked effect on direct N2O emissions (data not shown). This is a consequence of the fact that a higher weight is given to the rate of N fertilizer application in the internal decision rules of the model.
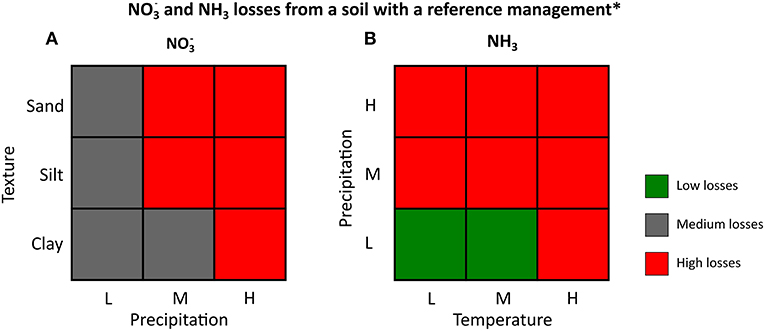
Figure 4. (A) Example of the effect of combinations of texture and precipitation and (B) temperature and precipitation on and NH3 losses respectively. *Reference management for losses: mineral soil without artificial drainage, medium N fertilizer application and no catch or cover crops. Reference management for NH3 emissions: mineral soil with application of manure with a high content.
The results of the sensitivity analysis of the N2O emissions part of the model are shown in Figure 5. High values for direct and total N2O emissions are consistently predicted when high rates of N fertilizer are applied. The model predicts decreased N2O emission when N fertilization is improved (i.e., lower application rates of N fertilizer). Predictions of low total N2O emissions are not obtained as a consequence of high predicted indirect N2O emissions in the reference management. Predictions of low direct N2O emissions are obtained consistently when low rates of N fertilizer are applied.
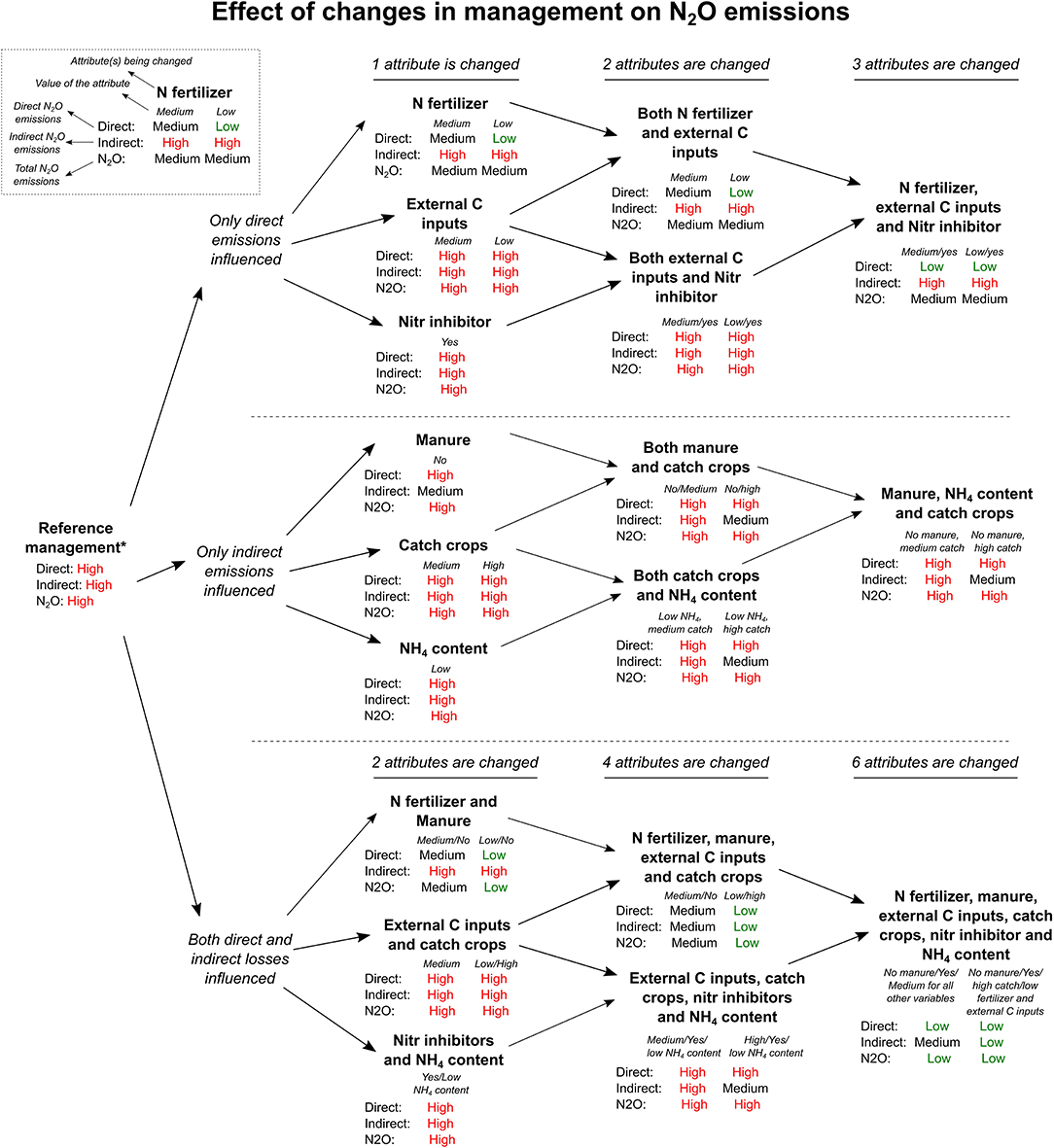
Figure 5. Sensitivity analysis of the N2O emissions part of the model (Figure 1), based on variations in management practices. The model outcomes are compared to a *reference management: a silty mineral soil with medium values for temperature, precipitation, OC content, no irrigation or artificial drainage and management practices that favor N2O emissions.
When management practices that influence indirect N2O emissions are improved (e.g., no manure application, reduced content of manure or planting catch crops instead of a fallow period), reduced indirect emissions are only predicted when no manure is applied, or when two or more of these management practices are applied together. However, this does not lead to a decrease in total N2O emissions in each of these cases, since the reference management leads to high direct N2O emissions. Optimizing one management practice that reduces direct N2O emissions and one management practice that reduces indirect emissions only leads to lower predictions of total N2O emissions when the amount of applied N fertilizer is reduced. Similarly, improving four or six management practices only leads to lower predictions of total N2O emissions when the amount of N fertilizer applied is reduced.
Model Testing Using Long-Term Field Experiments
For C sequestration, 11 of the 14 control treatments were predicted correctly by the model (Table 3, see Table S1 for additional information), indicating that the model is able to correctly predict the C sequestration function of agricultural soils when no change in OC concentration occurs over time. The model thus correctly simulates that C outputs were equal to C inputs in the control treatments of the LTEs. For treatments that included a change in management practice, 7 out of 14 experiments were predicted correctly by the model. The management practice that was varied most often was the addition of external C inputs (e.g., manure). The outcomes of the LTEs differed, with some experiments reporting no substantial increase in SOC stocks (Jäger et al., 2011; Kismányoky and Tóth, 2013), while others did report an increase (e.g., Blair et al., 2006; Monaco et al., 2008). In most cases, however, the model did not predict an increase in C sequestration following the addition of manure, in line with the results of the sensitivity analysis. This is a result of the prediction of high C losses for the majority of these experiments, because of the combination of inversion tillage and the absence of cover crops applied in most of these LTEs. As a result, modeled C losses were higher than C inputs in most cases, resulting in low predictions of C sequestration. It should be noted that since most LTEs only report changes in C concentration without reporting changes in bulk density, this may overestimate the amount of sequestered C.
The three LTEs that assessed the effect of crop residue incorporation reported relatively small absolute increases in OC concentrations (0.03–0.17% OC) after multiple decades (Perucci et al., 1997; Spiegel et al., 2018). This was correctly predicted by the model, with no modeled increase in C sequestration for two experiments (with a SOC concentration below 1%) and an increase for one experiment (with ca. 2% SOC). For all these experiments, the incorporation of crop residues led to a high predicted value for C inputs, which was balanced by high predicted OC losses, as a consequence of the application of a combination of inversion tillage and the absence of cover crops in these LTEs. Since modeled C inputs and outputs had an equal magnitude (high), the modeled increase in C sequestration in the experiment with higher SOC (ca. 2 %) in Spiegel et al. (2018) from low to medium was a consequence of the medium OC concentration of this soil.
The only experiment that resulted in high modeled values for C sequestration included both manure application and the presence of forage crops in the rotation (Bolinder et al., 2010), thereby increasing C inputs while reducing C outputs. This was in line with the results from the sensitivity analysis, which showed that the predicted magnitude of C sequestration generally increases when C inputs are increased and C losses are reduced.
The model was successful in predicting the magnitude of direct N2O emissions for all control (no N fertilizer application) (6/6) and management treatments (8/8) of the LTEs. In most of the LTEs, the control treatment resulted in low N2O emissions, while most of the treatments included the application of high rates of mineral N fertilizer, leading to higher N2O emissions. This was predicted well by the model. As shown in the sensitivity analysis, the model predicts high rates of N2O emissions when high rates of N fertilizer (i.e., > 100 kg N ha−1) are applied, regardless of other mitigation practices. This is in line with studies that have shown that rates of N2O emission increase exponentially once the amount of N fertilizer exceeds the N requirements of the crops (Bouwman et al., 2002; Hoben et al., 2011; Shcherbak et al., 2014). The addition of crop residues, included as “additional C inputs” in the model, without the application of mineral N fertilizer did not result in higher modeled N2O emissions, in line with observations (Abalos et al., 2013).
The three LTEs that reported on leaching assessed the effect of the incorporation of catch crops in the crop rotation cycle. The experiments by Hansen and Djurhuus (1997) were performed under similar environmental conditions and management practices, while soil texture differed between the experiments. Although the authors reported a decrease in leaching following the growth of catch crops, the model predicted a decrease in leaching only for the silty soil, while no decrease for the sandy soil was predicted because of the high precipitation rate. Also the model predictions for another experiment, which measured the effect of catch crops on leaching in a silty soil, were in line with observations (Constantin et al., 2010).
The effect of different tillage practices on SOC sequestration and N2O emissions has been studied in numerous long-term field experiments. Although it was initially assumed that the adoption of no-till or minimum tillage increases the SOC content, multiple studies and meta-analyses have shown that these practices generally only lead to a mere redistribution of OC in the topsoil, while not increasing the OC stock at the soil profile scale (Luo et al., 2010; Powlson et al., 2014; Haddaway et al., 2017). This effect is also simulated by the model, as shown in the sensitivity analysis (Figure 3). When reduced tillage or no-till is the only management practice that is changed, C outputs are reduced but C sequestration remains low when C inputs are low. When reduced tillage or no-till is combined with increased C inputs, e.g., the incorporation of crop residues, the model does predict a higher C sequestration. This is in line with field observations, which have shown that reduced tillage or no-till only lead to increased SOC stocks when combined with increased C inputs (Luo et al., 2010; Virto et al., 2012; Chowdhury et al., 2015).
Another management practice that has been studied intensively with respect to its effect on SOC stocks is the presence of cover crops between the main crops. Based on data from 37 different sites, Poeplau and Don (2015) calculated that the inclusion of cover crops in the crop rotation cycle leads to a significant increase in topsoil OC stocks on a multi-decadal timescale. In the model, the effect of cover crops is represented in two different ways: (1) through an increase in crop yield, which increases C inputs, and (2) through a reduction in the magnitude of C losses during the time no cash crops are present. When both variables are improved compared to the reference management, similar to the sensitivity analyses (Figure 3), the predicted C sequestration increases from low to medium. This modeled increase in C sequestration after the inclusion of cover crops in the crop rotation cycle is thus in line with the results from Poeplau and Don (2015).
Another measure that has been proposed to increase the OC content of agricultural soils is the incorporation of crop residues in the soil (Paustian et al., 2016; Chenu et al., 2018). In an extensive review, Lehtinen et al. (2014) found that the incorporation of crop residues did not lead to a significant increase in the topsoil OC concentration when this practice was applied for <10 years, although the effect became apparent after > 10 years. When high rates of crop residue incorporation were considered in the sensitivity analysis (i.e., > 30 % of the yield), the predicted C sequestration increased from low to medium. This model outcome is thus in line with the results from Lehtinen et al. (2014), given that this management practice is maintained over a long period of time.
Discussion
Designing a strategy to manage soils to mitigate climate change is not straightforward, as a thorough understanding of relevant processes at play is necessary. A change in soil management that increases the climate regulation function of soils can cause a decrease in another soil function, such as primary productivity (O'Sullivan et al., 2015; Schulte et al., 2015). Given the complexity of soil systems, models are often used to qualify or quantify the extent to which they perform different functions (Vogel et al., 2018), but the analysis of the multifunctional role of soils in society is still in its infancy. In this study, we presented a qualitative model to assess the climate regulation function of soils. This model has been coupled to similar models simulating other soil functions, in order to assess the trade-offs between these soil functions as a consequence of changes in management practices (Debeljak et al., 2019).
A sensitivity analysis has confirmed that changes in agricultural management practices have an effect on the predicted magnitude of C sequestration and N2O emissions by the developed model. The predicted magnitude of C sequestration generally only increases from low to high when C inputs are increased while C losses are reduced. If only C inputs are increased, while C outputs remain high, a low predicted magnitude of C sequestration only increases to medium. When only C outputs are decreased, while C inputs remain low, no increase in the magnitude of C sequestration is predicted. This is in line with studies showing that only reducing C outputs, e.g., through the adoption of no-till, only leads to increases in SOC storage when being accompanied by increases in C inputs (e.g., Virto et al., 2012). In addition, the small predicted increase in C sequestration when C inputs are increased, while C outputs remain high, is in line with studies showing that only increasing C inputs can increase SOC concentrations (Lehtinen et al., 2014). Given the fact that the model has been developed for a timescale of several years, while increases in SOC stocks are generally a slow process [in the order of tens of g C m−2 yr−1 (Paustian et al., 2016; Minasny et al., 2017)], this model outcome is in line with current knowledge, and avoids an overestimation of the C sequestration potential of agricultural soils. With respect to N2O emissions, the sensitivity analysis showed that the major factor determining the magnitude of predicted N2O emissions is the rate at which N fertilizer (either mineral or organic) is applied. This is in line with multiple studies that have shown that the magnitude of direct N2O emissions increases substantially when the plant demand for N is exceeded (Bouwman et al., 2002; Shcherbak et al., 2014).
The verification of the model performance has shown that the model is capable of correctly predicting the C sequestration function of soils that have received a constant management over the past decades (the control treatments). However, the model efficiency was lower for predictions of the alternative management practices that were applied in the LTEs. This was related to the fact that most LTEs assessed the effect of the addition of external C inputs on changes in SOC concentrations. While the model predicts that this has no effect, the majority of the LTEs reported an increase in SOC concentrations. The inability of the model to predict this change can be related to the combination of (i) the classification of inputs data and (ii) the small increase in SOC stocks that is generally observed when improved management practices are applied (10–100 g m−2 yr−1; Paustian et al., 2016; Minasny et al., 2017). The effect of other management practices on changes in SOC concentrations (e.g., tillage and cover crops) was predicted adequately by the model. The evaluation of the model performance has thus shown that, in general, the model was able to satisfactorily predict the direction of the change in C sequestration for most of the assessed management practices. However, the effect of the addition of external C inputs on SOC stocks was generally underestimated. Also the effect of the application of N fertilizer on N2O emissions was adequately assessed by the model in all cases. The magnitude of leaching was correctly predicted in 2 out of 3 cases, indicating that also this management practice is adequately simulated by the model.
The extent to which the model performance could be verified depended on the availability of data from LTEs. With respect to C, the majority of European LTEs evaluated the effect of tillage and the addition of different organic amendments on changes in SOC storage. Other management practices (e.g., the effect of grass in the crop rotation) were assessed in only a few experiments. The main focus of the assessment of the model performance was therefore on the former management practices, while also the effect of cover crops could be assessed based on a meta-analysis (Poeplau and Don, 2015). The performance of the C sequestration part of the model could thus be assessed fairly well. For N2O emissions, the variation in available LTEs was substantially lower, as they mostly focused on the effect of the rate of N fertilizer application on direct N2O emissions and the effect of catch crops on leaching. With respect to direct N2O emissions, management practices that could not be assessed are the addition of external C inputs, irrigation, and artificial drainage. However, while the high rate of N fertilizer application generally has the greatest effect on direct N2O emissions (Shcherbak et al., 2014), the uncertainties on the effect of the other variables will likely have a limited effect on the overall model uncertainty. The number of experiments used to test the part of the model was limited, but confirmed that the model correctly predicted lower losses as a consequence of the presence of catch crops. Furthermore, the structure of the part of the model is in line with evidence that the rate of losses is enhanced when high rates of N fertilizer are applied (Kirchmann et al., 2002) combined with a downward flux of water at high precipitation rates (Di and Cameron, 2002). In addition, losses were being reduced when catch crops were planted (Hansen and Djurhuus, 1997). In contrast, no experiments that assessed the effect of manure application on NH3 losses were present. Therefore, this part of the model was constructed based on evidence that high rates of NH3 emissions are enhanced by high rates of manure addition and a high content of manure (Sommer and Hutchings, 2001). This part of the model is thus in line with knowledge of the main variables affecting NH3 losses.
The available dataset allowed to evaluate the model for locations in all three temperature classes, but only for medium and high precipitation classes and silty and sandy soils. As a consequence, the model performance could not be assessed for clayey soils and environments with a mean annual precipitation below 400 mm. Future model evaluations should therefore focus on these environments in order to reduce uncertainties. The crops for which treatment effect were studied in the LTEs included mainly maize, winter wheat, barley and sugar beet, among other less-represented crops (Table S1). Some cropping systems, such as orchards, were thus not present in the validation dataset.
The effect of C sequestration and N2O emissions on the overall climate regulation soil function could not be evaluated, as this soil function is difficult to quantify. In addition, this soil function as such is generally not evaluated in field experiments, but evaluated based on measurements of C sequestration or N2O emissions separately. The combinations of both C sequestration and N2O emissions into the climate regulation soil function (Table 2) are therefore an attempt to provide the user with an indication of this soil function. As this is an expert-based interpretation, model users are encouraged to look at the modeled magnitude of C sequestration and N2O emissions to assess how management practices can be changed to improve the overall climate regulation soil function.
Although most of the generally applied management practices are represented in the model, some management practices are currently not included. For example, the effect of different types of compost on N2O emissions is currently not represented in the model, although it has been shown that this has an important effect on N2O emissions from agricultural soils (Zhou et al., 2017). However, it was chosen not to include this variable in the model since the effect is highly variable and greatly depends on soil type and climate (Zhou et al., 2017). Another treatment that has been the subject of multiple LTEs is the effect of mineral N fertilizer on C sequestration. Although generally no effect is observed (e.g., Nardi et al., 2004; Triberti et al., 2008; Poeplau et al., 2017), some authors report a small increase in SOC stocks after mineral N fertilization (Dersch and Böhm, 2001; Ladha et al., 2011). However, a potential increase in SOC stocks can be offset by the greenhouse gases produced during the manufacturing of mineral N fertilizer (Gao et al., 2018). Therefore, this has been omitted from the model. However, since the application of mineral N fertilizer generally leads to an increase in crop yields (Jiang et al., 2018), this effect can be included by increasing the NPP model input. Also the effect of cover crops on N2O emissions was not included as an independent variable in the model. This is because it has been shown that the effect of cover crops on N2O emissions greatly depends on factors other than the mere presence of cover crops, such as the rate of fertilizer application, the type of cover crop and the potential incorporation of the cover crop in the soil (Basche et al., 2014). Including all these interactions in the model would greatly increase model complexity and, as a consequence, model uncertainty. The addition of an additional C source, such as plowed-in residues of covers crops is, however, included in the model as a variable that stimulates direct N2O emission. Also the effect of tillage on N2O emissions was omitted from the model, as it has been shown that there is no significant difference in N2O emissions under different magnitudes of tillage (no-till, reduced tillage, or inversion tillage) when a timescale more than 10 years is considered (Six et al., 2004; van Kessel et al., 2013). A last set of management practices that is not evaluated by the model to minimize model complexity include the application of different types of irrigation, e.g., drip-irrigation vs. furrow irrigation (Kennedy et al., 2013), biochar application, agroforestry and subsoil management. In addition to considering additional management practices, potential future model improvements may involve adapting the model to simulate (i) managed grassland systems, (ii) managed peatlands, including resulting methane emissions, (iii) the effect of soil pH on C sequestration and N2O emissions and (iv) methane oxidation.
The model has been developed in the framework of the Horizon 2020 Landmark project, which aims to improve knowledge of the functions performed by European agricultural soils, while developing tools to assess the trade-offs between different soil functions. To achieve this, similar models have been developed for other soil functions: primary productivity (Sandén et al., 2019a), soil biodiversity and habitat provision (van Leeuwen et al., 2019), water regulation (Delgado et al., submitted) and nutrient recycling (Schröder et al., 2016). These separate models are brought together into a tool that assesses the extent to which agricultural soils perform these different soil functions (Debeljak et al., 2019). This allows to assess the win-wins and trade-offs between different soil functions as a consequence of management practices, and represents an important step forward in the quantification of different soil functions in agroecosystems across Europe in order to contribute to the understanding and management of soils to fulfill societal needs.
Conclusion
A qualitative decision support tool to assess the climate regulation soil function in European agroecosystems has been developed. This tool has been constructed based on the rationale that it should provide a reliable estimate of the magnitude of C sequestration and N2O emissions of arable soils using data that is generally available. A sensitivity analysis and an assessment of the model performance based on European LTEs have shown that the model is generally able to correctly assess the effect of different management practices on C sequestration and N2O emissions. However, the lack of validation data for agroecosystems in dry climates and on clayey soils prevented the model to be validated in these environments. This tool will be combined with similar models to assess trade-offs between different soil functions, in order to inform key stakeholders about the effect of different agricultural management practices on trade-offs between soil functions.
Author Contributions
This article resulted from cooperation within the climate regulation task group in the LANDMARK H2020 project. CD, JS, MV, CH, EL, BG, AT, VK, and MD contributed to the development of the model. The validation dataset was constructed by MV, while the validation and interpretation of the results was done by MV, JS, CD, TS, and HS. MV did most of the writing, with major inputs from CD, AT, JS, TS, EL, HS, RC, and BG.
Funding
This work was supported by the Swiss State Secretariat for Education, Research and Innovation (SERI) under contract number 635201. The opinions expressed and arguments employed herein do not necessarily reflect the official views of the Swiss Government. The Landmark project, leading to this application, has received funding from the European Union's Horizon 2020 research and innovation programme under grant agreement No. 635201.
Conflict of Interest Statement
The authors declare that the research was conducted in the absence of any commercial or financial relationships that could be construed as a potential conflict of interest.
Acknowledgments
This study was conducted as part of the LANDMARK (LAND Management: Assessment, Research and Knowledge base) project.
Supplementary Material
The Supplementary Material for this article can be found online at: https://www.frontiersin.org/articles/10.3389/fenvs.2019.00131/full#supplementary-material
Table S1. Detailed information about the input data used to validate the model and model outputs.
References
Abalos, D., Sanz-Cobena, A., Garcia-Torres, L., van Groenigen, J. W., and Vallejo, A. (2013). Role of maize stover incorporation on nitrogen oxide emissions in a non-irrigated Mediterranean barley field. Plant Soil 364, 357–371. doi: 10.1007/s11104-012-1367-4
Abdalla, M., Rueangritsarakul, K., Jones, M., Osborne, B., Helmy, M., Roth, B., et al. (2012). How effective is reduced tillage–cover crop management in reducing N2O fluxes from arable crop soils? Water Air Soil Pollut. 223, 5155–5174. doi: 10.1007/s11270-012-1268-4
Baggs, E. M., Rees, R. M., Smith, K. A., and Vinten, A. J. A. (2006). Nitrous oxide emission from soils after incorporating crop residues. Soil Use Manag. 16, 82–87. doi: 10.1111/j.1475-2743.2000.tb00179.x
Bai, Z., Caspari, T., Gonzalez, M. R., Batjes, N. H., Mäder, P., Bünemann, E. K., et al. (2018). Effects of agricultural management practices on soil quality: a review of long-term experiments for Europe and China. Agric. Ecosyst. Environ. 265, 1–7. doi: 10.1016/j.agee.2018.05.028
Barnard, R., Le Roux, X., Hungate, B. A., Cleland, E. E., Blankinship, J. C., Barthes, L., et al. (2006). Several components of global change alter nitrifying and denitrifying activities in an annual grassland. Funct. Ecol. 20, 557–564. doi: 10.1111/j.1365-2435.2006.01146.x
Basche, A. D., Miguez, F. E., Kaspar, T. C., and Castellano, M. J. (2014). Do cover crops increase or decrease nitrous oxide emissions? A meta-analysis. J. Soil Water Conserv. 69, 471–482. doi: 10.2489/jswc.69.6.471
Bertora, C., Zavattaro, L., Sacco, D., Monaco, S., and Grignani, C. (2009). Soil organic matter dynamics and losses in manured maize-based forage systems. Eur. J. Agron. 30, 177–186. doi: 10.1016/j.eja.2008.09.006
Blair, N., Faulkner, R. D., Till, A. R., and Poulton, P. R. (2006). Long-term management impacts on soil C, N and physical fertility. Soil Tillage Res. 91, 30–38. doi: 10.1016/j.still.2005.11.002
Bohanec, M. (2017). “Multi-criteria dex models: an overview and analysis,” in The 14th international symposium on operational research in Slovenia, eds. L. Z. Stirn, M. K. Borštar, J. Žerovnik and S. Drobne (Ljubljana: Slovenian Society Informatika–Section for Operational Research, 155–160.
Bohanec, M., and Rajkovič, V. (1990). DEX : an expert system shell for decision support. Sistemica 1, 145–157.
Bohanec, M., Žnidaršič, M., Rajkovic, V., Bratko, I., and Zupan, B. (2013). DEX methodology: three decades of qualitative multi-attribute modeling. Informatica 37, 49–54.
Bolinder, M. A., Kätterer, T., Andrén, O., Ericson, L., Parent, L.-E., and Kirchmann, H. (2010). Long-term soil organic carbon and nitrogen dynamics in forage-based crop rotations in Northern Sweden (63–64°N). Agric. Ecosyst. Environ. 138, 335–342. doi: 10.1016/j.agee.2010.06.009
Bouwman, A. F., Boumans, L. J. M., and Batjes, N. H. (2002). Emissions of N2O and NO from fertilized fields: Summary of available measurement data. Global Biogeochem. Cycles 16, 6-1-6–13. doi: 10.1029/2001GB001811
Butterbach-Bahl, K., Baggs, E. M., Dannenmann, M., Kiese, R., and Zechmeister-Boltenstern, S. (2013). Nitrous oxide emissions from soils: how well do we understand the processes and their controls? Philos. Trans. R. Soc. Lond. B. Biol. Sci. 368:20130122. doi: 10.1098/rstb.2013.0122
Castellano, M. J., Mueller, K. E., Olk, D. C., Sawyer, J. E., and Six, J. (2015). Integrating plant litter quality, soil organic matter stabilization, and the carbon saturation concept. Glob. Chang. Biol. 21, 3200–3209. doi: 10.1111/gcb.12982
Chabbi, A., Lehmann, J., Ciais, P., Loescher, H. W., Cotrufo, M. F., Don, A., et al. (2017). Aligning agriculture and climate policy. Nat. Clim. Chang. 7, 307–309. doi: 10.1038/nclimate3286
Chenu, C., Angers, D. A., Barré, P., Derrien, D., Arrouays, D., and Balesdent, J. (2018). Increasing organic stocks in agricultural soils: knowledge gaps and potential innovations. Soil Tillage Res. 188, 41–52. doi: 10.1016/j.still.2018.04.011
Chowdhury, S., Farrell, M., Butler, G., and Bolan, N. (2015). Assessing the effect of crop residue removal on soil organic carbon storage and microbial activity in a no-till cropping system. Soil Use Manag. 31, 450–460. doi: 10.1111/sum.12215
Ciais, P., Sabine, C., Bala, L., Bopp, L., Brovkin, V., Canadell, J., et al. (2013). “Carbon and Other Biogeochemical Cycles,” in Climate Change 2013: The Physical Science Basis. Contribution of Working Group I to the Fifth Assessment Report of the Intergovernmental Panel on Climate Change, eds. T. F. Stocker, D. Qin, G.-K. Plattner, M. Tigmor, S. K. Allen, J. Boschung, et al. (Carmbridge; New York, NY: Cambridge University Press), 465–570.
Constantin, J., Mary, B., Laurent, F., Aubrion, G., Fontaine, A., Kerveillant, P., et al. (2010). Effects of catch crops, no till and reduced nitrogen fertilization on nitrogen leaching and balance in three long-term experiments. Agric. Ecosyst. Environ. 135, 268–278. doi: 10.1016/j.agee.2009.10.005
Debeljak, M., Trajanov, A., Kuzmanovski, V., Schröder, J., Sandén, T., Spiegel, H., et al. (2019). A field-scale decision support system for assessment and management of soil functions. Front. Environ. Sci. 7:115. doi: 10.3389/fenvs.2019.00115
Dersch, G., and Böhm, K. (2001). Effects of agronomic practices on the soil carbon storage potential in arable farming in Austria. Nutr. Cycl. Agroecosystems 60, 49–55. doi: 10.1023/A:1012607112247
Di, H. J., and Cameron, K. C. (2002). Nitrate leaching in temperate agroecosystems: sources, factors and mitigating strategies. Nutr. Cycl. Agroecosystems 46, 237–256. doi: 10.1023/A:1021471531188
Gaines, T. P., and Gaines, S. T. (1994). Soil texture effect on nitrate leaching in soil percolates. Commun. Soil Sci. Plant Anal. 25, 2561–2570. doi: 10.1080/00103629409369207
Galloway, J. N., Aber, J. D., Erisman, J. W., Seitzinger, S. P., Howarth, R. W., Cowling, E. B., et al. (2003). The nitrogen cascade. Bioscience 53, 341–356. doi: 10.1641/0006-3568(2003)053[0341:TNC]2.0.CO;2
Gao, B., Huang, T., Ju, X., Gu, B., Huang, W., Xu, L., et al. (2018). Chinese cropping systems are a net source of greenhouse gases despite soil carbon sequestration. Glob. Chang. Biol. 24, 5590–5606. doi: 10.1111/gcb.14425
Giannakis, G. V., Nikolaidis, N. P., Valstar, J., Rowe, E. C., Moirogiorgou, K., Kotronakis, M., et al. (2017). Integrated critical zone model (1D-ICZ): a tool for dynamic simulation of soil functions and soil structure. Adv. Agron. 142, 277–314. doi: 10.1016/bs.agron.2016.10.009
Groffman, P. M., and Tiedje, J. M. (1991). Relationships between denitrification, CO2 production and air-filled porosity in soils of different texture and drainage. Soil Biol. Biochem. 23, 299–302. doi: 10.1016/0038-0717(91)90067-T
Haddaway, N. R., Hedlund, K., Jackson, L. E., Kätterer, T., Lugato, E., Thomsen, I. K., et al. (2017). How does tillage intensity affect soil organic carbon? A systematic review. Environ. Evid. 6, 1–48. doi: 10.1186/s13750-016-0079-2
Hansen, E. M., and Djurhuus, J. (1997). Nitrate leaching as influenced by soil tillage and catch crop. Soil Tillage Res. 41, 203–219. doi: 10.1016/S0167-1987(96)01097-5
Haynes, R. J., and Naidu, R. (1998). Influence of lime, fertilizer and manure applications on soil organic matter content and soil physical conditions: a review. Nutr. Cycl. Agroecosystems 51, 123–137. doi: 10.1023/A:1009738307837
Hoben, J. P., Gehl, R. J., Millar, N., Grace, P. R., and Robertson, G. P. (2011). Nonlinear nitrous oxide (N2O) response to nitrogen fertilizer in on-farm corn crops of the US Midwest. Glob. Chang. Biol. 17, 1140–1152. doi: 10.1111/j.1365-2486.2010.02349.x
Jäger, N., Stange, C. F., Ludwig, B., and Flessa, H. (2011). Emission rates of N2O and CO2 from soils with different organic matter content from three long-term fertilization experiments—a laboratory study. Biol. Fertil. Soils 47, 483–494. doi: 10.1007/s00374-011-0553-5
Jeuffroy, M. H., Baranger, E., Carrouée, B., de Chezelles, E., Gosme, M., Hénault, C., et al. (2013). Nitrous oxide emissions from crop rotations including wheat, oilseed rape and dry peas. Biogeosciences 10, 1787–1797. doi: 10.5194/bg-10-1787-2013
Jiang, G., Zhang, W., Xu, M., Kuzyakov, Y., Zhang, X., Wang, J., et al. (2018). Manure and mineral fertilizer effects on crop yield and soil carbon sequestration: a meta-analysis and modeling across china. Global Biogeochem. Cycles 32, 1659–1672. doi: 10.1029/2018GB005960
Kennedy, T. L., Suddick, E. C., and Six, J. (2013). Reduced nitrous oxide emissions and increased yields in California tomato cropping systems under drip irrigation and fertigation. Agric. Ecosyst. Environ. 170, 16–27. doi: 10.1016/j.agee.2013.02.002
Kirchmann, H., Johnston, A. E., and Bergström, L. F. (2002). Possibilities for reducing nitrate leaching from agricultural land. AMBIO 31, 404–408. doi: 10.1579/0044-7447-31.5.404
Kismányoky, T., and Tóth, Z. (2013). Effect of mineral and organic fertilization on soil organic carbon content as well as on grain production of cereals in the IOSDV (ILTE) long-term field experiment, Keszthely, Hungary. Arch. Agron. Soil Sci. 59, 1121–1131. doi: 10.1080/03650340.2012.712208
Kotronakis, M., Giannakis, G. V., Nikolaidis, N. P., Rowe, E. C., Valstar, J., Paranychianakis, N. V., et al. (2017). Modeling the impact of carbon amendments on soil ecosystem functions using the 1D-ICZ model. Adv. Agron. 142, 315–352. doi: 10.1016/bs.agron.2016.10.010
Ladha, J. K., Reddy, C. K., Padre, A. T., and van Kessel, C. (2011). Role of nitrogen fertilization in sustaining organic matter in cultivated soils. J. Environ. Qual. 40, 1756–1766. doi: 10.2134/jeq2011.0064
Le Quéré, C., Andrew, R. M., Friedlingstein, P., Sitch, S., Pongratz, J., Manning, A. C., et al. (2018). Global Carbon Budget 2017. Earth Syst. Sci. Data 10, 405–448. doi: 10.5194/essd-10-405-2018
Lehtinen, T., Schlatter, N., Baumgarten, A., Bechini, L., Krüger, J., Grignani, C., et al. (2014). Effect of crop residue incorporation on soil organic carbon and greenhouse gas emissions in European agricultural soils. Soil Use Manag. 30, 524–538. doi: 10.1111/sum.12151
Li, C., Frolking, S., and Frolking, T. A. (1992). A model of nitrous oxide evolution from soil driven by rainfall events: 1. Model structure and sensitivity. J. Geophys. Res. Atmos. 97, 9759–9776. doi: 10.1029/92JD00509
Little, S., Lindeman, J., Maclean, K., and Janzen, H. (2008). Holos - A tool to estimate and reduce GHGs from farms. Methodology and algorithms for Version 1.1.x. Ottawa, ON: Agriculture & Agri-Food Canada.
Lugato, E., Leip, A., and Jones, A. (2018). Mitigation potential of soil carbon management overestimated by neglecting N2O emissions. Nat. Clim. Chang. 8, 219–223. doi: 10.1038/s41558-018-0087-z
Luo, Z., Wang, E., and Sun, O. J. (2010). Can no-tillage stimulate carbon sequestration in agricultural soils? A meta-analysis of paired experiments. Agric. Ecosyst. Environ. 139, 224–231. doi: 10.1016/j.agee.2010.08.006
Meurer, K. H. E., Haddaway, N. R., Bolinder, M. A., and Kätterer, T. (2018). Tillage intensity affects total SOC stocks in boreo-temperate regions only in the topsoil — A systematic review using an ESM approach. Earth Sci. Rev. 177, 613–622. doi: 10.1016/j.earscirev.2017.12.015
Minasny, B., Arrouays, D., McBratney, A. B., Angers, D. A., Chambers, A., Chaplot, V., et al. (2018). Rejoinder to comments on Minasny et al., 2017 Soil carbon 4 per mille Geoderma 292, 59–86. Geoderma 309, 124–129. doi: 10.1016/j.geoderma.2017.05.026
Minasny, B., Malone, B. P., McBratney, A. B., Angers, D. A., Arrouays, D., Chambers, A., et al. (2017). Soil carbon 4 per mille. Geoderma 292, 59–86. doi: 10.1016/j.geoderma.2017.01.002
Moeskops, B., Buchan, D., Van Beneden, S., Fievez, V., Sleutel, S., Gasper, M. S., et al. (2012). The impact of exogenous organic matter on SOM contents and microbial soil quality. Pedobiologia. 55, 175–184. doi: 10.1016/j.pedobi.2012.03.001
Monaco, S., Hatch, D. J., Sacco, D., Bertora, C., and Grignani, C. (2008). Changes in chemical and biochemical soil properties induced by 11-yr repeated additions of different organic materials in maize-based forage systems. Soil Biol. Biochem. 40, 608–615. doi: 10.1016/j.soilbio.2007.09.015
Nardi, S., Morari, F., Berti, A., Tosoni, M., and Giardini, L. (2004). Soil organic matter properties after 40 years of different use of organic and mineral fertilisers. Eur. J. Agron. 21, 357–367. doi: 10.1016/j.eja.2003.10.006
Ogle, S. M., Breidt, F. J., and Paustian, K. (2005). Agricultural management impacts on soil organic carbon storage under moist and dry climatic conditions of temperate and tropical regions. Biogeochemistry 72, 87–121. doi: 10.1007/s10533-004-0360-2
O'Sullivan, L., Creamer, R. E., Fealy, R., Lanigan, G., Simo, I., Fenton, O., et al. (2015). Functional land management for managing soil functions: a case-study of the trade-off between primary productivity and carbon storage in response to the intervention of drainage systems in Ireland. Land Use Policy 47, 42–54. doi: 10.1016/j.landusepol.2015.03.007
Parton, W. J., Hartman, M., Ojima, D., and Schimel, D. (1998). DAYCENT and its land surface submodel: description and testing. Glob. Planet. Change 19, 35–48. doi: 10.1016/S0921-8181(98)00040-X
Paustian, K., Andrén, O., Janzen, H. H., Lal, R., Smith, P., Tian, G., et al. (1997). Agricultural soils as a sink to mitigate CO 2 emissions. Soil Use Manag. 13, 230–244. doi: 10.1111/j.1475-2743.1997.tb00594.x
Paustian, K., Lehmann, J., Ogle, S., Reay, D., Robertson, G. P., and Smith, P. (2016). Climate-smart soils. Nature 532, 49–57. doi: 10.1038/nature17174
Perucci, P., Bonciarelli, U., Santilocchi, R., and Bianchi, A. A. (1997). Effect of rotation, nitrogen fertilization and management of crop residues on some chemical, microbiological and biochemical properties of soil. Biol. Fertil. Soils 24, 311–316. doi: 10.1007/s003740050249
Poeplau, C., and Don, A. (2015). Carbon sequestration in agricultural soils via cultivation of cover crops – A meta-analysis. Agric. Ecosyst. Environ. 200, 33–41. doi: 10.1016/j.agee.2014.10.024
Poeplau, C., Don, A., Vesterdal, L., Le, J., van Wesemael, B., Schumacher, J., et al. (2011). Temporal dynamics of soil organic carbon after land-use change in the temperate zone - carbon response functions as a model approach. Glob. Chang. Biol. 17, 2415–2427. doi: 10.1111/j.1365-2486.2011.02408.x
Poeplau, C., Reiter, L., Berti, A., and Kätterer, T. (2017). Qualitative and quantitative response of soil organic carbon to 40 years of crop residue incorporation under contrasting nitrogen fertilisation regimes. Soil Res. 55, 1–9. doi: 10.1071/SR15377
Powlson, D. S., Stirling, C. M., Jat, M. L., Gerard, B. G., Palm, C. A., Sanchez, P. A., et al. (2014). Limited potential of no-till agriculture for climate change mitigation. Nat. Clim. Chang. 4, 678–683. doi: 10.1038/nclimate2292
Powlson, D. S., Whitmore, A. P., and Goulding, K. W. T. (2011). Soil carbon sequestration to mitigate climate change: a critical re-examination to identify the true and the false. Eur. J. Soil Sci. 62, 42–55. doi: 10.1111/j.1365-2389.2010.01342.x
Sanchez-Martín, L., Meijide, A., Garcia-Torres, L., and Vallejo, A. (2010). Combination of drip irrigation and organic fertilizer for mitigating emissions of nitrogen oxides in semiarid climate. Agric. Ecosyst. Environ. 137, 99–107. doi: 10.1016/j.agee.2010.01.006
Sandén, T., Spiegel, H., Stüger, H.-P., Schlatter, N., Haslmayr, H.-P., Zavattaro, L., et al. (2018). European long-term field experiments: knowledge gained about alternative management practices. Soil Use Manag. 34, 167–176. doi: 10.1111/sum.12421
Sandén, T., Trajanov, A., Spiegel, H., Kuzmanovski, V., Saby, N. P. A., Picaud, C., et al. (2019a). Development of an agricultural primary productivity decision support model: a case study in France. Front. Environ. Sci. 7:58. doi: 10.3389/fenvs.2019.00058
Sandén, T., Zavattaro, L., Spiegel, H., Grignani, C., Sandén, H., Baumgarten, A., et al. (2019b). Out of sight: profiling soil characteristics, nutrients and bacterial communities affected by organic amendments down to one meter in a long-term maize experiment. Appl. Soil Ecol. 134, 54–63. doi: 10.1016/j.apsoil.2018.10.017
Sanz-Cobena, A., Sánchez-Martín, L., García-Torres, L., and Vallejo, A. (2012). Gaseous emissions of N2O and NO and NO3– leaching from urea applied with urease and nitrification inhibitors to a maize (Zea mays) crop. Agric. Ecosyst. Environ. 149, 64–73. doi: 10.1016/j.agee.2011.12.016
Schröder, J. J., Schulte, R. P. O., Creamer, R. E., Delgado, A., van Leeuwen, J., Lehtinen, T., et al. (2016). The elusive role of soil quality in nutrient cycling: a review. Soil Use Manag. 32, 476–486. doi: 10.1111/sum.12288
Schulte, R. P. O., Bampa, F., Bardy, M., Coyle, C., Creamer, R. E., Fealy, R., et al. (2015). Making the most of our land: managing soil functions from local to continental scale. Front. Environ. Sci. 3:81. doi: 10.3389/fenvs.2015.00081
Schulte, R. P. O., Creamer, R. E., Donnellan, T., Farrelly, N., Fealy, R., O'Donoghue, C., et al. (2014). Functional land management: a framework for managing soil-based ecosystem services for the sustainable intensification of agriculture. Environ. Sci. Policy 38, 45–58. doi: 10.1016/j.envsci.2013.10.002
Shcherbak, I., Millar, N., and Robertson, G. P. (2014). Global metaanalysis of the nonlinear response of soil nitrous oxide (N2O) emissions to fertilizer nitrogen. Proc. Natl. Acad. Sci. USA. 111, 9199–9204. doi: 10.1073/pnas.1322434111
Šimon, T., Mikanová, O., and Cerhanová, D. (2013). Long-term effect of straw and farmyard manure on soil organic matter in field experiment in the Czech Republic. Arch. Agron. Soil Sci. 59, 1193–1205. doi: 10.1080/03650340.2012.706871
Six, J., Conant, R. T., Paul, E. A., and Paustian, K. (2002). Stabilization mechanisms of soil organic matter: implications for C-saturation of soils. Plant Soil 241, 155–176. doi: 10.1023/A:1016125726789
Six, J., Ogle, S. M., Jay breidt, F., Conant, R. T., Mosier, A. R., and Paustian, K. (2004). The potential to mitigate global warming with no-tillage management is only realized when practised in the long term. Glob. Chang. Biol. 10, 155–160. doi: 10.1111/j.1529-8817.2003.00730.x
Sommer, S., and Hutchings, N. (2001). Ammonia emission from field applied manure and its reduction—invited paper. Eur. J. Agron. 15, 1–15. doi: 10.1016/S1161-0301(01)00112-5
Soussana, J.-F., Lutfalla, S., Ehrhardt, F., Rosenstock, T., Lamanna, C., Havlík, P., et al. (2017). Matching policy and science: Rationale for the ‘4 per 1000 - soils for food security and climate’ initiative. Soil Tillage Res. 188, 3–15. doi: 10.1016/j.still.2017.12.002
Spiegel, H., Sandén, T., Dersch, G., Baumgarten, A., Gründling, R., and Franko, U. (2018). “Soil organic matter and nutrient dynamics following different management of crop residues at two sites in Austria,” in Soil Management and Climate Change, eds M. Á. Muñoz and R. Zornoza (London: Elsevier), 253–265. doi: 10.1016/B978-0-12-812128-3.00017-3
Stewart, C. E., Paustian, K., Conant, R. T., Plante, A. F., and Six, J. (2007). Soil carbon saturation: concept, evidence and evaluation. Biogeochemistry 86, 19–31. doi: 10.1007/s10533-007-9140-0
Syakila, A., and Kroeze, C. (2011). The global nitrous oxide budget revisited. Greenh. Gas Meas. Manag. 1, 17–26. doi: 10.3763/ghgmm.2010.0007
Tonitto, C., David, M. B., and Drinkwater, L. E. (2006). Replacing bare fallows with cover crops in fertilizer-intensive cropping systems: a meta-analysis of crop yield and N dynamics. Agric. Ecosyst. Environ. 112, 58–72. doi: 10.1016/j.agee.2005.07.003
Triberti, L., Nastri, A., Giordani, G., Comellini, F., Baldoni, G., and Toderi, G. (2008). Can mineral and organic fertilization help sequestrate carbon dioxide in cropland? Eur. J. Agron. 29, 13–20. doi: 10.1016/j.eja.2008.01.009
Tubiello, F. N., Salvatore, M., Ferrara, A. F., House, J., Federici, S., Rossi, S., et al. (2015). The contribution of agriculture, forestry and other land use activities to global warming, 1990-2012. Glob. Chang. Biol. 21, 2655–2660. doi: 10.1111/gcb.12865
van Eekeren, N., Bommelé, L., Bloem, J., Schouten, T., Rutgers, M., de Goede, R., et al. (2008). Soil biological quality after 36 years of ley-arable cropping, permanent grassland and permanent arable cropping. Appl. Soil Ecol. 40, 432–446. doi: 10.1016/j.apsoil.2008.06.010
van Kessel, C., Venterea, R., Six, J., Adviento-Borbe, M. A., Linquist, B., and van Groenigen, K. J. (2013). Climate, duration, and N placement determine N 2 O emissions in reduced tillage systems: a meta-analysis. Glob. Chang. Biol. 19, 33–44. doi: 10.1111/j.1365-2486.2012.02779.x
van Leeuwen, J. P., Creamer, R. E., Cluzeau, D., Debeljak, M., Gatti, F., Henriksen, C. B., et al. (2019). Modeling of soil functions for assessing soil quality: soil biodiversity and habitat provisioning. Front. Environ. Sci. 7:113. doi: 10.3389/fenvs.2019.00113
Vinten, A. J. A., Vivian, B. J., Wright, F., and Howard, R. S. (1994). A comparative study of nitrate leaching from soils of differing textures under similar climatic and cropping conditions. J. Hydrol. 159, 197–213. doi: 10.1016/0022-1694(94)90256-9
Virto, I., Barré, P., Burlot, A., and Chenu, C. (2012). Carbon input differences as the main factor explaining the variability in soil organic C storage in no-tilled compared to inversion tilled agrosystems. Biogeochemistry 108, 17–26. doi: 10.1007/s10533-011-9600-4
Vogel, H.-J., Bartke, S., Daedlow, K., Helming, K., Kögel-Knabner, I., Lang, B., et al. (2018). A systemic approach for modeling soil functions. SOIL 4, 83–92. doi: 10.5194/soil-4-83-2018
Xia, L., Lam, S. K., Wolf, B., Kiese, R., Chen, D., and Butterbach-Bahl, K. (2018). Trade-offs between soil carbon sequestration and reactive nitrogen losses under straw return in global agroecosystems. Glob. Chang. Biol. 24, 5919–5932. doi: 10.1111/gcb.14466
Keywords: soil functions, climate regulation, carbon sequestration, N2O emissions, agroecosystems, qualitative decision modeling
Citation: Van de Broek M, Henriksen CB, Ghaley BB, Lugato E, Kuzmanovski V, Trajanov A, Debeljak M, Sandén T, Spiegel H, Decock C, Creamer R and Six J (2019) Assessing the Climate Regulation Potential of Agricultural Soils Using a Decision Support Tool Adapted to Stakeholders' Needs and Possibilities. Front. Environ. Sci. 7:131. doi: 10.3389/fenvs.2019.00131
Received: 01 February 2019; Accepted: 22 August 2019;
Published: 11 September 2019.
Edited by:
Hans-Joerg Vogel, Helmholtz Centre for Environmental Research (UFZ), GermanyReviewed by:
Martin Wiesmeier, Technical University of Munich, GermanyPeter Dörsch, Norwegian University of Life Sciences, Norway
Copyright © 2019 Van de Broek, Henriksen, Ghaley, Lugato, Kuzmanovski, Trajanov, Debeljak, Sandén, Spiegel, Decock, Creamer and Six. This is an open-access article distributed under the terms of the Creative Commons Attribution License (CC BY). The use, distribution or reproduction in other forums is permitted, provided the original author(s) and the copyright owner(s) are credited and that the original publication in this journal is cited, in accordance with accepted academic practice. No use, distribution or reproduction is permitted which does not comply with these terms.
*Correspondence: Marijn Van de Broek, bWFyaWpuLnZhbmRlYnJvZWtAdXN5cy5ldGh6LmNo