- 1Department of Anthropology, University of Delhi, New Delhi, India
- 2Public Health Foundation of India, Gurugram, India
The increased prevalence of non-communicable chronic diseases (NCDs) is reflected in the rising economic burden of health conditions. Observational studies conducted in health economics research are detecting associations of NCDs or related risk factors with economic measures like health insurance, economic inequalities, accessibility of jobs, education, annual income, health expenditure, etc. The inferences of such relationships do not prove causation and are limited to associations which are many times influenced by confounding factors and reverse causation. Mendelian randomization (MR) approach is a useful method for exploring causal relations between modifiable risk factors and measures of health economics. The application of MR in economic assessment of health conditions has been started and is producing fruitful results.
Introduction
The world is facing epidemiological transition that has been attributed the rise of non-communicable chronic diseases (NCDs) and shifted the goal-post from fatal communicable diseases (1). United Nations' Sustainable Development Goals (SDG, 2015) has specified NCDs as one of their important health related targets (Target-3.4) for improving overall wellbeing of human populations (2). NCDs include chronic diseases like cardiovascular disease (CVDs), cancer, diabetes, and chronic respiratory diseases, etc. Global Burden of Disease (GBD) has observed rise in proportion of deaths attributable to NCDs from 57.6% in 1990 to 71.3% in 2015 (3). India spends only 4.5% of the GDP on health (4) and was ranked 143 in SDG index under which the indicator of NCDs (i.e., age-standardized death rate due to cardiovascular disease, cancer, diabetes, and chronic respiratory disease in populations aged 30–70 years, per 100,000 population) was scored low (i.e., 46 less than the median score of 50; scale used: 0 to 100) (2).
Among the South Asian countries, India and Pakistan, had received the bulk of development assistance for health (DAH) which is a financial (or in-kind) resource transferred from development agencies (like UNICEF, WHO, etc.) to low- and middle-income countries (LMICs) primarily for maintaining or improving health (4, 5).
Over 60% of deaths in India are due to NCDs (6) and it was ranked second in the burden of NCDs in 2015 vs. average DAH allocated for 2012–14 (4). NCDs may cost about $4.58 trillion between 2012 and 2030 to India, of which, $2.17 trillion and $1.03 trillion will be due to CVDs and mental health conditions, respectively (7). CVD patients in India spend higher out-of-pocket health expenditure and rely more on borrowing and household asset sales (8). The rising economic costs are due to increased burden of NCDs and their risk factors. For example, alcohol is a known risk factor for several types of cancers and cardiovascular conditions, like hypertension, stroke, etc. (9). Lifestyle based risk factors, like physical inactivity, alcohol abuse, tobacco use, and high body mass index, etc., jointly accounts for 61% of cardiovascular deaths (10).
Health economists are interested in studying the role of socio-economic patterning of health inequalities related to NCDs and their risk factors (11). A study on NCD multi-morbidity and its impact on health care utilization and out-of-pocket expenditure (OOPE) found that medicines constitute the largest proportion of OOPE (12). The scope of research in health economics is gradually widening with the availability of large resources containing variety of data on biological and economic factors. It is allowing the health economists to evaluate variety of economic factors in relation to risk factors of NCDs (13, 14). Epidemiologists in order to balance the distribution of confounders between the analytical sub-groups, usually adjust the statistical models for confounding while evaluating exposure-outcome associations in observational studies. However, it is difficult to authenticate whether statistical adjustment has truly nullified the effect of confounders in reported associations. Testing of such reported associations fail in RCTs. The analytical approach of Mendelian randomization (MR) can be very helpful in preventing failures of RCTs by providing strong causal evidence between exposure and outcomes in comparison to typical observational studies. The objective of this paper is to review the use of Mendelian randomization (MR) approach in studying health economic aspects of NCDs.
Causality in Epidemiology
The interpretation of association between two factors in an observational study is limited to the plausible relationship and it does not prove causality (15). Epidemiologically, observational studies like cross-sectional population based or hospital based designs are also prone to biases like reverse causation and confounding (16, 17). The science of etiological epidemiology provides triangulation strategies that help in obtaining reliable evidence for translating it into the policy statement. The causal research question can be addressed by triangulation approach which allows the integration of results from different approaches each having unrelated and different sources of potential biases (18). Appropriately designed randomized controlled trials (RCTs), where study participants are randomly assigned to the intervention and control groups, are considered as gold standard in detecting the causal evidence (19). However, the evaluation of long term effects of social behaviors (e.g., health care expenditure, academic performance, supplementary nutrition to the child, alcohol consumption and smoking) on human health may not be feasible to test with RCTs (20). Moreover, high cost of RCTs and feasibility problems due to ethical considerations may pose further challenges in their implementation. Therefore, we need alternative approaches for testing causal relationships in research related to health economics that can overcome the potential biases of observational designs and also provide reliable evidence for causal associations. Mendelian randomization (MR) is one of such approaches whose reliability has been established in epidemiology and is gaining popularity among health economists in testing causal research statements and obtaining consistent evidence in a cost effective manner.
Mendelian Randomization
MR utilizes genes as an instrument for modifiable exposures in order to test their causal relationship with health outcomes or risk factors (21). The random allocation of groups in MR is based on Mendelian law of independent assortment, i.e., distribution of genetic variants inherited randomly from parental to offspring generation. Thus, the differences between individuals due to genetic variation in MR will not be subject to the confounding or reverse-causation bias which generally distorts the results of observational studies (22).
The random distribution of known and unknown confounders in MR approach is natural in nature which is achieved by genotypes that are known to be associated with specific health condition. Like intention-to-treat in RCTs where study participants are analyzed irrespective of their compliance with the intervention, MR investigates people irrespective of their genotypes that may lead to differences in outcomes (23). Therefore, MR designs are generally considered similar to RCTs. Many times randomization in RCTs is likely to be biased due to the reason of non-compliance. For example, randomization allocates the study participants in RCTs only on the basis of specific treatment chosen. People exercise their choice to reduce high body mass index, through selective lifestyle changes, and that influences their long and short term social outcomes like educational attainment and career achievements. The element of exercising choice while treatment selection by the participants is usually influenced by their socio-economic position, dietary habits, caste and religion, thus, has effects on their level and duration of compliance (24). Unlike observational studies, the genetic variants used in MR are largely unrelated to social and behavioral traits, and avoid systematic biases in randomization process. The strength of MR lies in the use of standard instrumental variable (IV) approach commonly applied in econometrics where exogenous or independent variables are used to detect the effects of endogenous or dependent variables.
The potential genetic variant for IV analysis must comply with three main assumptions of MR (25, 26): (1) the genetic variant must be truly associated with the health condition or its measure, so that it can be used as a proxy for exposure of interest (2) it should not be associated with measured/unmeasured confounders of the exposure-outcome relation, and (3) exclusion restriction criteria, i.e., the instrument/genetic variant should not be directly associated with the outcome and its effect must be mediated only through the exposure, implying that no alternative causal pathway exists between instrument and outcome except via exposure (27). These assumptions are highly credible when the effects of an exposure are directly due to the genetic variant (Figure 1).
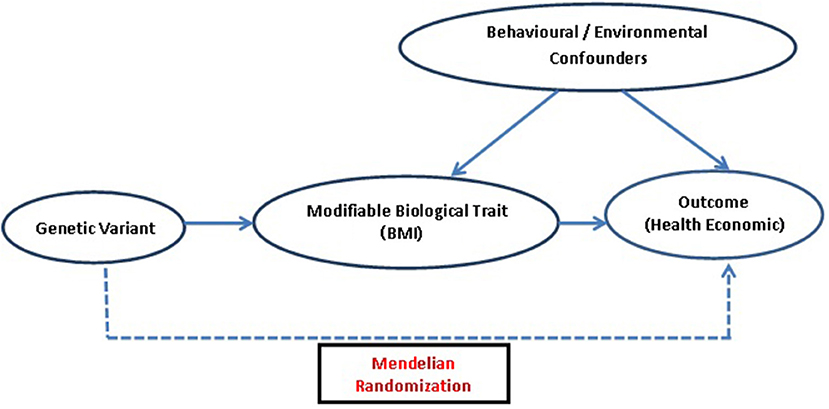
Figure 1. Diagrammatic representation of MR approach: assessing causal association of genetic variant which acts as an IV, i.e., a proxy for modifiable biological traits, with outcomes related to health economics.
MR assumptions lose credibility to some extent when exposures are polygenic traits i.e., multiple genetic variants may be responsible for the exposure. The multiple genetic variants used for polygenetic traits may lead to pleiotropy i.e., a single gene having multiple expressions, by being involved in multiple pathways (27, 28). This limitation of MR can be statistically resolved using MR-Egger method (27). MR approach has been widely utilized in the last decade and various methods have been proposed to improve the inferences given the potential limitations (29).
One of the important step of the MR is to rely on a single genetic instrument which is used as a proxy for the modifiable exposure. This can be partially addressed by using multiple variants and using weighted allelic score as an instrument (25). It increases the proportion of variance explained by the instrument, thus increasing power of the study (30), apart from addressing the other issue like pleiotropy or being in linkage disequilibrium (LD) or in close proximity with a variant that affects the outcome (23). If a genetic variant has a pleiotropic in nature (i.e., multiple effects) or is in LD with another gene, it cannot represent a valid instrument for the exposure of interest, as it may produce biased findings. Over the years, MR has methodologically moved forward from the use of simple IVs based on single variant to the composite IVs based on multiple genetic variants without the knowledge of their functions (26). Recently, method for conducting pleiotropy robust MR has been proposed which first estimates the amount of pleiotropy in the model and then corrects it (31). Bi-directional MR approach has also been suggested to be useful in understanding causal pathways where genetic instruments for both exposure and outcome are applied in both directions in MR analysis to establish the direction of causal pathway (23).
Guide for MR
The identification of genetic instrument variable (IV) to be used as proxies for exposures in MR analyses should satisfy the underlying assumptions of an IV method.
IV Is Associated With the Exposure (Relevance Assumption)
Relevance means the genetic variant(s) that can be used as an instrument that robustly predicts the exposure. In order to identify genetic variants associated with the exposure, we need to conduct a comprehensive literature search (using PubMeD and MEDLINE) for articles based on genome-wide association studies (GWASs) directly related to the exposure. Nowadays, there is an online GWAS catalog of published literature available (https://www.ebi.ac.uk/gwas/) for identifying genetic variants associated with exposure of interest. “PhenoScanner” is another online resource developed by University of Cambridge, which can be used for genotypic-phenotypic associations (32). These resources are helpful in developing a list of potential genetic variants associated with the exposure (for using them as instruments). One can extract the gene names, single nucleotide polymorphisms (SNPs), their effect sizes (along with their direction and standard errors), biological pathways and significance level (p-values < 10−8) from the original GWAS articles. This list further guides the second round of literature search for identifying the primarily identified variants that have been replicated and validated in other populations to prepare a list of well-established genetic variants. Researcher should give more weightage to those genetic variants which are well replicated and validated in the target population of interest, having strong association with the exposure, and have relatively large effect sizes (explaining the maximum variation in the exposure). In case of unavailability of reliable instruments, one can also use multiple SNPs to generate a “risk score” (a polygenic score) which can be used as an instrument in MR studies instead of using one single SNP.
However, it is possible that one may not be able to find a GWAS nor a validation study related to the exposure in their target population during literature search. Therefore, the last step would be to genotype the potential genetic variants in a statistically powered sub-sample to ensure the genetic association with the exposure in the desired population. It should be noted that the instruments should not be internally derived using single sample to avoid bias (i.e., Winner's curse: the overestimation of association between SNP and exposure) (33). Therefore, it is advised that the identification of an instrument and MR analyses should be performed in different set of samples from the same population.
For MR analyses one can use R package (i.e., TwoSampleMR) available at “MR-Base” website which is also a database of genotypic-phenotypic associations based on GWAS studies (34). MRrobust is a STATA package for MR analyses (35).
IV Is Not Associated With Any Confounder of the Exposure–Outcome Association (Independence or Exchangeability Assumption)
The MR designs are considered similar to RCTs and the key property of RCTs is that the two randomized subgroups are exchangeable in nature which means the expected distribution of confounders is balanced for both the subgroups. Similarly, in MR the division of population into genetic subgroups based on IV (i.e., genetic variant) leads to exchangeable subgroups, independent of all observed and unobserved confounders, having similar distribution of confounders like RCTs. However, this assumption cannot be tested empirically. Nevertheless, the genetic variant to be used as instrument should not be directly associated with any of the possible confounders affecting the relationship between exposure and outcome of interest. Moreover, one can assess the biological mechanisms (given in Table 1) that can make a genetic variant unsuitable for IV.
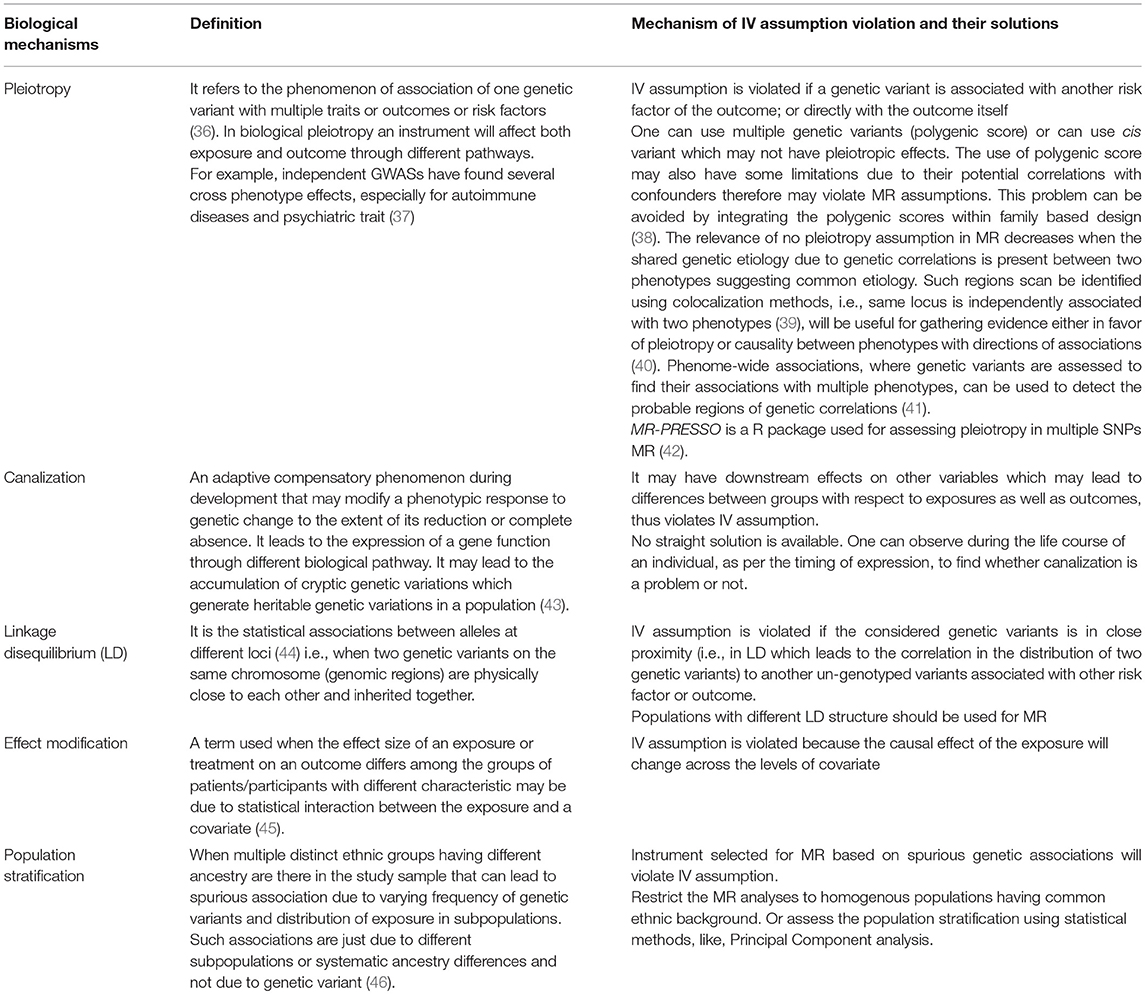
Table 1. Types of biological mechanisms that can violate IV assumptions and their possible solutions.
IV Does Not Affect the Outcome, Except Possibly via Its Association With the Exposure (Exclusion Restriction Assumption)
The genetic instrument should be associated with the outcome only through the route of exposure, and not directly. The conditional assumption of exclusion restriction can be evaluated through the knowledge of biological pathway of the genetic instrument used (47). Since the specific functions of the genetic variants and their exact biological mechanisms are generally unknown, the direct assessment of exclusion restriction assumption is not possible, thus, validity of this “exclusion restriction” assumption cannot be measured. The biological mechanisms like canalization and pleiotropy can also violate exclusion restriction (48). One can conduct gene by environment interaction analyses that could provide evidence for canalization (48). A pleiotropy robust Mendelian randomization (PRMR) method has been suggested which corrects for pleiotropy after estimating the magnitude of pleiotropy (31). Moreover, if it is difficult to estimate the degree of pleiotropy even then PRMR can be applied for the sensitivity analyses. Recently, researchers have suggested an alternative approach, i.e., genetic instrumental variable regression, which controls for pleiotropic effects using polygenic scores (49). Furthermore, a new way to estimate IV (i.e., MR G-Estimation under No Interaction with Unmeasured Selection) which is robust to additive unmeasured confounding and exclusion restriction violations has also been suggested (50).
MR in Health Economics and Insurance
The estimation of healthcare cost expenditures of medical conditions are important for planning public health programmes. Many times confounding factors, like socio-economic status, natural histories of diseases, ascertainment bias and measurement error make the economic assessment of health outcomes difficult in observational studies (48). Moreover, exposing patients to interventions just for collecting information on cost consequences of health in RCTs is also not practical and ethical (51). Since genetic variants associated with health conditions are generally unrelated to known and unknown confounders (i.e., environmental and behavioral factors), therefore, MR designs offer the reliable detection of causal effects of health conditions and thereby the cost of healthcare.
Case Study
Scholder et al. (57) examined causal association between child's/adolescent's height and cognitive, mental health and behavioral outcomes like academic performance, IQ, self-esteem, depression symptoms and behavioral problems, using genetic determinants of height utilizing Instrument variable analyses using available data from Avon Longitudinal Study of Parents and Children (ALSPAC).
Authors used a set of nine genetic variants associated with height among Europeans: HMGA2 (rs1042725), ZBTB38 (rs6440003), GDF5 (rs6060373), LOC387103 (rs4549631), EFEMP1 (rs3791675), SCMH1 (rs6686842), ADAMTSL3 (rs10906982), DYM (rs8099594), and C6orf106 (rs2814993). Firstly, authors tested the validity of genetic variants to be used as instruments for height by testing all the MR assumptions. The genetic instruments were found to be uncorrelated with a large set of family background variables which my confound the relationship between height and outcomes of interest. Authors also ran two falsification checks. They first examined the effect of height on maternal education for which they did not assumed any effect. Thereafter, the effect of height on body weight was examined for which they assumed a strong effect. After confirming the validity of instruments they examined the causal relationship between height and set of cognitive, behavioral and mental health outcomes.
The IV results showed that there is causal relationship between taller height and cognitive function among girls with respect to national exam taken at age of 14 years (β = 0.54, SE = 0.24) and IQ test at the age of 8 years (β = 0.67, SE = 0.28). They did not find any evidence of height effecting scholastic self-esteem, self worthself-worth, or depression. Authors suggested that height presents disadvantage rather than advantage as it was fund to increase hyperactive behaviour among girls and emotional and peer problems among boys.
Advantages of MR
• Highly useful in strengthening the evidence from purely observational to causality which can effectively paves a path for RCTs.
• MR also useful in detecting the direction of causality of association between the exposure and outcome.
• Use of genetic variants as proxy for exposure helps in the random allocation of confounders between exposed and unexposed that also eliminates selection bias. Moreover, the genetic data can be robustly generated without errors at affordable cost that tend to decline more in future.
• Methodology for conducting MR analysis is well established due to the continuous efforts made by the researchers, and it has the potential to grow further in future.
• Identification of genetic instruments from available databases is useful and manageable even for researchers, having no background in genetics, with little training.
Limitations of MR
• The inferences based on modeling driven MR approach are heavily dependent upon the credibility of the assumptions underlying MR.
• The execution of MR is contingent upon the identification of genetic proxy for the exposure of our interest.
• Large sample sizes are required for MR analysis, due to small effect sizes of genetic variants to meet the optimum statistical power, which is difficult to obtain in low and middle income countries.
• Genetic instruments of MR are selected from hypothesis free genome-wide association studies which lacks in-depth understanding of the mechanisms of associations between genetic variants and traits/diseases.
The health economists are increasingly interested in studying the causal effects of modifiable non-genetic factors on the outcomes of their interest (Table 2). For example, a study on the effect of prenatal alcohol exposure on child's educational outcome in terms of academic achievements has been reported (52). Since the feasibility of studying fetal blood alcohol levels is very low and may pose some ethical challenges, they examined the data on maternal alcohol exposure during pregnancy as a proxy for alcohol exposure in utero; the results were ambiguous due to confounding of socio-economic position. Therefore, MR approach was applied by using ADH1B gene as an instrument variable for maternal alcohol exposure and found long term negative effects on child's educational achievements (52). Another study on longevity and frailty (i.e., a state of high vulnerability to trivial stressors) did not find any causal association of triglyceride levels with these two complimentary aspects of aging. This study also rejected the previous observational associations of triglyceride levels with longevity and frailty (53). Such studies can be attempted again using MR approach with the help of established genetic markers of triglyceride levels.
Observational studies have shown that taller stature and low body mass index (BMI) are associated with high SES in developed countries (58, 59). The relationship between child's height and economic outcomes is confounded by a variety of detrimental environmental experiences during childhood, like, unobserved family income and variation in their nutrition. With the help of MR approach a study assessed the causal effects of height on human capital accumulation (in terms of academic performance, IQ, self-esteem, depression symptoms and behavioral problems) (54). They used genetic variants as proxy of height un-confounded by above environmental experiences and found an important role of height in child's cognitive performance, handling emotional and peer pressures. Recently, a large MR study (119,000 participants of UK Biobank) was conducted to examine the causal relationship between stature, BMI and SES using genetic variants as unconfounded proxies for height and BMI (55). It was reported that taller stature (of 6.3 cm) leads to higher educational attainment that further leads to odds of 1.12 for higher status of jobs and higher annual household income (of £1130). Their analyses also showed a causal association of higher BMI (of 4.6 kg/m2) with lower annual household income (~ £2940) (55).
Physical traits and economic success are inextricably entwined through body size, beauty and height (59–61). A long term empirically tested belief suggests that taller people have better cognitive and non-cognitive skills, thus, possess more positive qualities which helps them to earn more than relatively shorter people (59, 61). The causal role of height on earnings was evaluated in a study using twins to attenuate the influence of genetic factors and family background (62). They reported no association may be due to the inability of twin design to eliminate the confounding from early life conditions and differential parental investments (62). A recent study applied MR using gene score of previously associated genetic variants of height as a proxy or IV to examine its relationship with administrative information related to long term labor market outcomes i.e., earnings and labor market attachment (56). Their MR analysis did not find any association of gene score with the earnings, may be due to low power to conduct MR with sample size of 2000 individuals, and confirmed the findings of the above mentioned twin study (which are generally difficult to conduct). In their opinion, the previously reported associations may not be causal as they have observed (56) but it is difficult to conclude unless proved with the help large sample size to get more precise estimates.
Further, education is one of the three components of a regular instrument for assessing socio-economic position. In the above study, protective causal effects of education on obesity in Finnish population using MR design was reported (56). It was suggested that low birth weight is associated with lower educational attainment and earnings (63). MR based economic evaluations in future can assess the short and long term causal relationships between birth weight and educational attainment and earnings. The medical reimbursement is a central aspect of insurance for the health insurance companies especially for medical technologies which rely heavily upon their clinical efficacy (64). MR can be used as a cost and time effective strategy for testing the clinical efficacy of medical technologies instead of costly RCTs (65).
Conclusion
MR has high potential in research related to health economics and health insurance consequences, and will be very useful in making informed decisions with respect to policy coverage on latest medical technologies. The utility of MR is even higher in countries with limited resources for health care financing and moving toward universal health coverage as an important public health policy.
Author Contributions
GW, VG, and MS conceptualized the idea of the manuscript. VG and GW completed the literature search. GW and VG wrote the manuscript. MS critically reviewed and improved the manuscript.
Conflict of Interest Statement
The authors declare that the research was conducted in the absence of any commercial or financial relationships that could be construed as a potential conflict of interest.
Acknowledgments
We would like to acknowledge Wellcome/DBT India Alliance for funding Early Career Fellowship on mendelian randomization to Gagandeep Kaur Walia (Grant number: IA/CPHE/16/1/502649).
References
1. Murray CJ, Barber RM, Foreman KJ, Abbasoglu Ozgoren A, Abd-Allah F, Abera SF, et al. Global, regional, and national disability-adjusted life years (DALYs) for 306 diseases and injuries and healthy life expectancy (HALE) for 188 countries, 1990-2013: quantifying the epidemiological transition. Lancet (2015) 386:2145–91. doi: 10.1016/S0140-6736(15)61340-X
2. Lim SS, Allen K, Bhutta ZA, Dandona L, Forouzanfar MH, Fullman N, et al. Measuring the health-related Sustainable Development Goals in 188 countries: a baseline analysis from the Global Burden of Disease Study 2015. Lancet (2016) 388:1813–50. doi: 10.1016/S0140-6736(16)31467-2
3. Wang H, Naghavi M, Allen C, Barber RM, Bhutta ZA, Carter A, et al. Global, regional, and national life expectancy, all-cause mortality, and cause-specific mortality for 249 causes of death, 1980-2015: a systematic analysis for the Global Burden of Disease Study 2015. Lancet (2016) 388:1459–544. doi: 10.1016/S0140-6736(16)31012-1
4. Institutefor Health Metrics and Evaluation Seattle (IHME). Financing Global Health 2016: Development Assistance, Public and Private Health Spending for the Pursuit of Universal Health Coverage. Seattle, WA: IHME (2017).
5. Van de Maele N, Evans DB, Tan-Torres T. Development assistance for health in Africa: are we telling the right story? Bull World Health Organ. (2013) 91:483–90. doi: 10.2471/BLT.12.115410
6. World Health Organization (WHO). Non Communicable Diseases Country Profiles: 2014. Geneva: WHO (2014).
7. Bloom DE, Cafiero-Fonseca ET, Candeias V, Adashi E, Bloom L, Gurfein L, et al. Economics of Non-Communicable Diseases in India: The Costs and Returns on Investment of Interventions to Promote Healthy Living and Prevent, Treat, and Manage NCDs. Geneva: World Economic Forum, Harvard School of Public Health (2014).
8. Karan A, Engelgau M, Mahal A. The household-level economic burden of heart disease in India. Trop Med Int Health (2014) 19:581–91. doi: 10.1111/tmi.12281
9. Parry CD, Patra J, Rehm J. Alcohol consumption and non-communicable diseases: epidemiology and policy implications. Addiction (2011) 106:1718–24. doi: 10.1111/j.1360-0443.2011.03605.x
10. WHO. Global Health Risks: Mortality and Burden of Disease Attributable to Selected Major Risks. Geneva: WHO (2009).
11. Vellakkal S, Subramanian SV, Millett C, Basu S, Stuckler D, Ebrahim S. Socioeconomic inequalities in non-communicable diseases prevalence in India: disparities between self-reported diagnosis and standardized measures. PLoS ONE (2013) 8:e68219. doi: 10.1371/journal.pone.0068219
12. Pati S, Agrawal S, Swain S, Lee JT, Vellakkal S, Hussain MA, et al. Non Communicable diseases multimorbidity and associated health care utilization and expenditures in India: cross-sectional study. BMC Health Serv Res. (2014) 14:451. doi: 10.1186/1472-6963-14-451
13. Kien VD, Minh HV, Ngoc NB, Phuong TB, Ngan TT, Quam MB. Inequalities in household catastrophic health expenditure and impoverishment associated with noncommunicable diseases in Chi Linh, Hai Duong, Vietnam. Asia Pac J Public Health (2017) 29:35S−44S. doi: 10.1177/1010539517712919
14. Blaga OM, Vasilescu L, Chereches RM. Use and effectiveness of behavioural economics in interventions for lifestyle risk factors of non-communicable diseases: a systematic review with policy implications. Perspect Public Health (2018) 138:100–110. doi: 10.1177/1757913917720233
15. Altman N, Krzywinski M. Association, correlation and causation. Nat Methods (2015) 12:899–900. doi: 10.1038/nmeth.3587
16. Keavney B. Commentary: Katan's remarkable foresight: genes and causality 18 years on. Int J Epidemiol. (2004) 33:11–4. doi: 10.1093/ije/dyh056
17. Sattar N, Preiss D. Reverse causality in cardiovascular epidemiological research: more common than imagined? Circulation (2017) 135:2369–72. doi: 10.1161/CIRCULATIONAHA.117.028307
18. Lawlor DA, Tilling K, Davey Smith G. Triangulation in aetiological epidemiology. Int J Epidemiol. (2016) 45:1866–86. doi: 10.1093/ije/dyw314
19. Bothwell LE, Greene JA, Podolsky SH, Jones DS. Assessing the gold standard–lessons from the history of RCTs. N Engl J Med. (2016) 374:2175–81. doi: 10.1056/NEJMms1604593
20. Wolff N. Using randomized controlled trials to evaluate socially complex services: problems, challenges and recommendations. J Ment Health Policy Econ. (2000) 3:97–109. doi: 10.1002/1099-176X(200006)3:2<97::AID-MHP77>3.0.CO;2-S
21. Smith GD, Ebrahim S. ‘Mendelian randomization’: can genetic epidemiology contribute to understanding environmental determinants of disease? Int J Epidemiol. (2003) 32:1–22. doi: 10.1093/ije/dyg070
22. Davey Smith G, Paternoster L, Relton C. When will Mendelian randomization become relevant for clinical practice and public health? JAMA (2017) 317:589–91. doi: 10.1001/jama.2016.21189
23. Davey Smith G, Hemani G. Mendelian randomization: genetic anchors for causal inference in epidemiological studies. Hum Mol Genet. (2014) 23:R89–98. doi: 10.1093/hmg/ddu328
24. von Hinke S, Davey Smith G, Lawlor DA, Propper C, Windmeijer F. Genetic markers as instrumental variables. J Health Econ. (2016) 45:131–48. doi: 10.1016/j.jhealeco.2015.10.007
25. Burgess S, Thompson SG. Use of allele scores as instrumental variables for Mendelian randomization. Int J Epidemiol. (2013) 42:1134–44. doi: 10.1093/ije/dyt093
26. Burgess S, Davies NM, Thompson SG. Bias due to participant overlap in two-sample Mendelian randomization. Genet Epidemiol. (2016) 40:597–608. doi: 10.1002/gepi.21998
27. Burgess S, Thompson SG. Interpreting findings from Mendelian randomization using the MR-Egger method. Eur J Epidemiol. (2017) 32:377–89. doi: 10.1007/s10654-017-0255-x
28. VanderWeele TJ, Tchetgen Tchetgen EJ, Cornelis M, Kraft P. Methodological challenges in Mendelian randomization. Epidemiology (2014) 25:427–35. doi: 10.1097/EDE.0000000000000081
29. Evans DM, Brion MJ, Paternoster L, Kemp JP, McMahon G, Munafò M, et al. Mining the human phenome using allelic scores that index biological intermediates. PLoS Genet. (2013) 9:e1003919. doi: 10.1371/journal.pgen.1003919
30. Brion MJ, Shakhbazov K, Visscher PM. Calculating statistical power in Mendelian randomization studies. Int J Epidemiol. (2013) 42:1497–501. doi: 10.1093/ije/dyt179
31. van Kippersluis H, Rietveld CA. Pleiotropy-robust Mendelian randomization. Int J Epidemiol. (2018) 47:1279–88. doi: 10.1093/ije/dyx002
32. Staley JR, Blackshaw J, Kamat MA, Ellis S, Surendran P, Sun BB, et al. PhenoScanner: a database of human genotype-phenotype associations. Bioinformatics (2016) 32:3207–9. doi: 10.1093/bioinformatics/btw373
33. Burgess S, Butterworth AS, Thompson JR. Beyond Mendelian randomization: how to interpret evidence of shared genetic predictors. J Clin Epidemiol. (2016) 69:208–16. doi: 10.1016/j.jclinepi.2015.08.001
34. Hemani G, Zheng J, Elsworth B, Wade KH, Haberland V, Baird D, et al. The MR-Base platform supports systematic causal inference across the human phenome. Elife (2018) 7:e34408. doi: 10.7554/eLife.34408
35. Spiller W, Davies NM, Palmer TM. Software Application Profile: mrrobust - a tool for performing two-sample summary Mendelian randomization analyses. Int J Epidemiol. (2018). dyy195. doi: 10.1093/ije/dyy195
36. Wagner GP, Zhang J. The pleiotropic structure of the genotype-phenotype map: the evolvability of complex organisms. Nat Rev Genet. (2011) 12:204–13. doi: 10.1038/nrg2949
37. Solovieff N, Cotsapas C, Lee PH, Purcell SM, Smoller JW. Pleiotropy in complex traits: challenges and strategies. Nat Rev Genet. (2013) 14:483–95. doi: 10.1038/nrg3461
38. Minică CC, Dolan CV, Boomsma DI, de Geus E, Neale MC. Extending causality tests with genetic instruments: an integration of Mendelian randomization and the classical twin design. Behav Genet. (2018) 48:337–49. doi: 10.1007/s10519-018-9904-4
39. Giambartolomei C, Vukcevic D, Schadt EE, Franke L, Hingorani AD, Wallace C, et al. Bayesian test for colocalisation between pairs of genetic association studies using summary statistics. PLoS Genet. (2014) 10:e1004383. doi: 10.1371/journal.pgen.1004383
40. Pingault JB, O'Reilly PF, Schoeler T, Ploubidis GB, Rijsdijk F, Dudbridge F. Using genetic data to strengthen causal inference in observational research. Nat Rev Genet. (2018) 19:566–80. doi: 10.1038/s41576-018-0020-3
41. Pickrell JK, Berisa T, Liu JZ, Ségurel L, Tung JY, Hinds DA. Detection and interpretation of shared genetic influences on 42 human traits. Nat Genet. (2016) 48:709–17. doi: 10.1038/ng.3570
42. Verbanck M, Chen CY, Neale B, Do R. Widespread pleiotropy confounds causal relationships between complex traits and diseases inferred from Mendelian randomization. bioRxiv (2017). bioRxiv:157552. doi: 10.1101/157552
43. Paaby AB, Rockman MV. Cryptic genetic variation: evolution's hidden substrate. Nat Rev Genet. (2014) 15:247–58. doi: 10.1038/nrg3688
44. de Leeuw CA, Neale BM, Heskes T, Posthuma D. The statistical properties of gene-set analysis. Nat Rev Genet. (2016) 17:353–64. doi: 10.1038/nrg.2016.29
45. Corraini P, Olsen M, Pedersen L, Dekkers OM, Vandenbroucke JP. Effect modification, interaction and mediation: an overview of theoretical insights for clinical investigators. Clin Epidemiol. (2017) 9:331–8. doi: 10.2147/CLEP.S129728
46. Price AL, Zaitlen NA, Reich D, Patterson N. New approaches to population stratification in genome-wide association studies. Nat Rev Genet. (2010) 11:459–63. doi: 10.1038/nrg2813
47. Davies NM, Holmes MV, Davey Smith G. Reading Mendelian randomisation studies: a guide, glossary, and checklist for clinicians. BMJ (2018) 362:k601. doi: 10.1136/bmj.k601
48. Dixon P, Davey Smith G, von Hinke S, Davies NM, Hollingworth W. Estimating marginal healthcare costs using genetic variants as instrumental variables: mendelian randomization in economic evaluation. Pharmacoeconomics (2016) 34:1075–86. doi: 10.1007/s40273-016-0432-x
49. DiPrete TA, Burik CAP, Koellinger PD. Genetic instrumental variable regression: Explaining socioeconomic and health outcomes in nonexperimental data. Proc Natl Acad Sci USA (2018) 115:E4970–79. doi: 10.1073/pnas.1707388115
50. Tchetgen Tchetgen EJ, Suny B, Walter S. The GENIUS Approach to Robust Mendelian Randomization Inference. arXiv. (2017). arXiv:1709.07779.
51. Petrou S, Gray A. Economic evaluation alongside randomised controlled trials: design, conduct, analysis, and reporting. BMJ (2011) 342:d1548. doi: 10.1136/bmj.d1548
52. von Hinke Kessler Scholder S, Wehby GL, Lewis S, Zuccolo L. Alcohol exposure in utero and child academic achievement. Econ J. (2014) 124:634–67. doi: 10.1111/ecoj.12144
53. Liu Z, Burgess S, Wang Z, Deng W, Chu X, Cai J, et al. Associations of triglyceride levels with longevity and frailty: a Mendelian randomization analysis. Sci Rep. (2017) 7:41579. doi: 10.1038/srep41579
54. von Hinke Kessler Scholder S, Davey Smith G, Lawlor DA, Propper C, Windmeijer F. Child height, health and human capital: Evidence using genetic markers. Eur Econ Rev. (2013) 57:1–22. doi: 10.1016/j.euroecorev.2012.09.009
55. Tyrrell J, Jones SE, Beaumont R, Astley CM, Lovell R, Yaghootkar H, et al. Height, Body mass index, and socioeconomic status: mendelian randomisation study in UKBiobank. BMJ (2016) 352:i582. doi: 10.1136/bmj.i582
56. Böckerman P, Viinikainen J, Vainiomäki J, Hintsanen M, Pitkänen N, Lehtimäki T, et al. Stature and long-term labor market outcomes: Evidence using Mendelian randomization. Econ Hum Biol. (2017) 24:18–29. doi: 10.1016/j.ehb.2016.10.009
57. von Hinke Kessler Scholder S, Davey Smith G, Lawlor DA, Propper C, Windmeijer F. The effect of fat mass on educational attainment: examining the sensitivity to different identification strategies. Econ Hum Biol. (2012) 10:405–18. doi: 10.1016/j.ehb.2012.04.015
58. Magnusson PK, Rasmussen F, Gyllensten UB. Height at age 18 years is a strong predictor of attained education later in life: cohort study of over 950,000 Swedish men. Int J Epidemiol. (2006) 35:658–63. doi: 10.1093/ije/dyl011
59. Case A, Paxson C. Stature and status: Height, ability, and labor market outcomes. J Polit Econ. (2008) 116:499–532. doi: 10.1086/589524
60. Cawley J, Spiess CK. Obesity and skill attainment in early childhood. Econ Hum Biol. (2008) 6:388–97. doi: 10.1016/j.ehb.2008.06.003
61. Persico N, Postlewaite A, Silverman D. The effect of adolescent experience on labor market outcomes: the case of height. J Polit Econ. (2004) 116:1019–53. doi: 10.1086/422566
62. Böckerman P, Vainiomäki J. Stature and life-time labor market outcomes: accounting for unobserved differences. Labour Econ. (2013) 24:86–96. doi: 10.1016/j.labeco.2013.06.003
63. Black SE, Devereux PJ, Salvanes KG. From the cradle to the labor market? The effect of birth weight. Q. J. Econ. (2007) 122:409–39. doi: 10.1162/qjec.122.1.409
64. Endrei D, Molics B, Agoston I. Multicriteria decision analysis in the reimbursement of new medical technologies: real-world experiences from Hungary. Value Health (2014) 17:487–9. doi: 10.1016/j.jval.2014.01.011
Keywords: health economics, Mendelian randomization, genes, epidemiology, public health
Citation: Gupta V, Sachdeva MP and Walia GK (2019) “Mendelian Randomization” Approach in Economic Assessment of Health Conditions. Front. Public Health 7:2. doi: 10.3389/fpubh.2019.00002
Received: 31 October 2018; Accepted: 04 January 2019;
Published: 04 February 2019.
Edited by:
Jimmy Thomas Efird, University of Newcastle, AustraliaCopyright © 2019 Gupta, Sachdeva and Walia. This is an open-access article distributed under the terms of the Creative Commons Attribution License (CC BY). The use, distribution or reproduction in other forums is permitted, provided the original author(s) and the copyright owner(s) are credited and that the original publication in this journal is cited, in accordance with accepted academic practice. No use, distribution or reproduction is permitted which does not comply with these terms.
*Correspondence: Vipin Gupta, dWRhaWlnQGdtYWlsLmNvbQ==
Gagandeep Kaur Walia, Z2thdXJ3QGdtYWlsLmNvbQ==