- 1Department of Experimental, Diagnostic and Specialty Medicine, University of Bologna, Bologna, Italy
- 2IRCCS Istituto delle Scienze Neurologiche di Bologna, Bologna, Italy
- 3Department of Physics and Astronomy, University of Bologna, Bologna, Italy
- 4Department of Neurology, Pain Center, LIM 62, University of São Paulo, São Paulo, Brazil
- 5Pain Center, Instituto do Câncer do Estado de São Paulo, São Paulo, Brazil
- 6U. O. Neurologia, Ospedale Bufalini, Cesena, Italy
- 7Department of Biomedical and Neuromotor Sciences, University of Bologna, Bologna, Italy
- 8Department of Laboratory Medicine, Clinical Chemistry, Karolinska Institutet, Karolinska University Hospital, Stockholm, Sweden
- 9Applied Biomedical Research Center (CRBA), Policlinico S.Orsola-Malpighi Polyclinic, Bologna, Italy
- 10Unit of Bologna, CNR Institute of Molecular Genetics Luigi Luca Cavalli-Sforza, Bologna, Italy
Chronic pain prevalence is high worldwide and increases at older ages. Signs of premature aging have been associated with chronic pain, but few studies have investigated aging biomarkers in pain-related conditions. A set of DNA methylation (DNAm)-based estimates of age, called “epigenetic clocks,” has been proposed as biological measures of age-related adverse processes, morbidity, and mortality. The aim of this study is to assess if different pain-related phenotypes show alterations in DNAm age. In our analysis, we considered three cohorts for which whole-blood DNAm data were available: heat pain sensitivity (HPS), including 20 monozygotic twin pairs discordant for heat pain temperature threshold; fibromyalgia (FM), including 24 cases and 20 controls; and headache, including 22 chronic migraine and medication overuse headache patients (MOH), 18 episodic migraineurs (EM), and 13 healthy subjects. We used the Horvath's epigenetic age calculator to obtain DNAm-based estimates of epigenetic age, telomere length, levels of 7 proteins in plasma, number of smoked packs of cigarettes per year, and blood cell counts. We did not find differences in epigenetic age acceleration, calculated using five different epigenetic clocks, between subjects discordant for pain-related phenotypes. Twins with high HPS had increased CD8+ T cell counts (nominal p = 0.028). HPS thresholds were negatively associated with estimated levels of GDF15 (nominal p = 0.008). FM patients showed decreased naive CD4+ T cell counts compared with controls (nominal p = 0.015). The severity of FM manifestations expressed through various evaluation tests was associated with decreased levels of leptin, shorter length of telomeres, and reduced CD8+ T and natural killer cell counts (nominal p < 0.05), while the duration of painful symptoms was positively associated with telomere length (nominal p = 0.034). No differences in DNAm-based estimates were detected for MOH or EM compared with controls. In summary, our study suggests that HPS, FM, and MOH/EM do not show signs of epigenetic age acceleration in whole blood, while HPS and FM are associated with DNAm-based estimates of immunological parameters, plasma proteins, and telomere length. Future studies should extend these observations in larger cohorts.
Introduction
Chronic pain is defined as a “pain which has persisted beyond normal tissue healing time” (1), a process that, in the absence of additional unfavorable factors, is expected to not exceed a period of 3 months. Chronic pain is common in both developed and developing countries (2, 3). In 2006, a large computer-assisted telephone survey reported that in European countries, the prevalence of chronic pain varied from 12 to 30%, with Spain, Ireland, and UK among the countries with the lowest prevalence, and Italy, Poland, and Norway among those with the highest prevalence (4). These country-dependent differences are probably triggered by multiple factors, including differences in pain perception and treatment, lifestyle, and age of the participants.
Accordingly, the etiology of chronic pain is multifactorial and embraces a broad range of factors that can be grouped in demographic, clinical, psychological, and lifestyle domains. Risk factors for chronic pain may not only trigger the onset of a persistent syndrome, but may also influence its eventual manifestation, having impact on different chronic pain dimensions like duration, localization, intensity, interference in daily life activities, or influence on emotional state. Advanced chronological age, female biological sex, feminine gender identity, deprived socio-economic status, unemployment, and adverse and unsatisfactory occupational situation are among the demographic positive risk factors for chronic pain (5–8). Although the reported prevalence rates tend to be higher in developing countries, the correlation between ethnicity and chronic pain is complex and the driving mechanisms are not clearly determined yet (9). In addition, cultural heritage and tradition with its practices and rituals are additional risk agents that modulate the attitudes toward the painful experience influencing the manifestation and/or perception of chronic condition (10). Among the clinical risk factors, the most pronounced one is the coexistence of another acute or chronic pain (11). Co-morbid physical and mental disorders, surgical, and medical interventions that have been undergone, increased BMI, and sleep disorders are the risk agents favoring persistent painful phenotypes (12–15). Also, several DNA variants that may be responsible for the genetic pre-disposition to develop pain have been identified (16). More than 150 genes have been already associated with pain-related conditions, among which are COMT, OPRM, SNC9A, IL6, or TNFA. The personal attitude and beliefs, concerns, and fears stimulate the development of the chronic pain conditions and can restrain or totally impede the recovery, as in the case of fear-avoidance model behavior in musculoskeletal pain disorders (17). Finally, the risk factors connected to lifestyle are smoking, alcohol use disorders, limited physical activity, and painogenic modern urban environment with, for example, low sun exposure or high air pollution (18–21). Additionally, the individual alimentary habits plausibly contribute to development and prevention of long-lasting pain disorders but the mechanism remains unclear (22).
As mentioned above, advanced age is a risk factor for chronic pain and often phenotypes of pre-mature aging are observed in patients. These manifestations of accelerated aging involve not only structural changes in the brain, like a total and regional decrease of gray matter (23–25), but also more systemic changes like a decrease in peripheral blood leukocyte telomere length (26) and increased inflammation (27–29).
Advances in recent research have led to the identification of a limited set of biomarkers that are considered potential biological age predictors (30), i.e., that are informative of the discrepancy between chronological age and biological age in conditions associated with successful (biological age deceleration) or unsuccessful (biological age acceleration) conditions. Potential markers of biological age include the analysis of telomere length, a brain age predictor based on structural neuroimaging [T1-weighted magnetic resonance imaging (MRI)], and different types of epigenetic clocks based on the DNA methylation (DNAm) values of specific CpG sites. In particular, epigenetic clocks have been extensively analyzed in physiological and pathological conditions (31) and an increase in predicted epigenetic age compared with chronological age has been associated to multiple conditions including neurological diseases (32, 33), progeroid syndromes (34–36) and, although in a less straightforward way, morbidity, and mortality (37, 38). Epigenetic clock measurements in whole blood have been associated with socio-cultural aspects, including education, lifestyle, and socio-economic status (38–41) and with exposure to stress and trauma (42, 43).
The “first generation” epigenetic clocks were developed on the basis of the association between DNAm and chronological age. The most used predictors were built using different training sets, which included large datasets of multiple tissues (44), whole blood (45), or human cell types used in ex vivo studies (35). Recently, more sophisticated epigenetic clocks have been built using not only chronological age but also clinical biomarkers that are informative of the quality of aging or associated with mortality more than age itself. The PhenoAge clock includes 10 variables (albumin, creatinine, serum glucose, C-reactive protein, lymphocyte percent, mean cell volume, red cell distribution width, alkaline phosphatase, white blood cell count, and age) (38), while the GrimAge is a composite biomarker based on the DNAm surrogates of seven plasma proteins and of smoking pack-years (40). Both PhenoAge and GrimAge outperformed previous epigenetic clocks in their associations with age-related conditions and mortality.
To the best of our knowledge, only one study investigated epigenetic age acceleration in chronic pain (46). The authors analyzed 20 individuals with chronic pain between 60 and 83 years and 9 age-matched controls and evaluated biological age acceleration by calculating the difference between Horvath's DNAm age and chronological age. A younger epigenome was observed in subjects that did not experience chronic pain in the past 3 months. Individuals characterized as emotionally stable, conscientious, and extrovert demonstrated lower epigenetic age. Epigenetic age acceleration was shown to be positively associated with higher experimental pain sensitivity and negatively associated with fluid cognition and memory, globally supporting an association between epigenetic age and chronic pain.
The aim of the present work is to further explore the association between epigenetic age and chronic pain, by investigating first- and second-generation epigenetic clocks and DNAm surrogates of plasma proteins, blood cell counts, and telomere length in different pain-related conditions for which methylation data are available.
Materials and Methods
Datasets
Our work involves DNAm data from three epigenome-wide studies investigating methylation patterns in pain-related phenotypes: heat pain sensitivity (HPS), fibromyalgia (FM), and headache syndromes comprising medication-overuse headache and episodic migraine. The characteristics of the datasets are provided in Table S1 and are summarized in the following paragraphs.
Heat Pain Sensitivity
The HPS dataset was acquired through Gene Expression Omnibus (GEO) NCBI repository (http://www.ncbi.nlm.nih.gov/geo/) under accession number GSE53128 (47). It includes DNAm data generated using the Infinium Human Methylation 450K BeadChip on whole blood from female monozygotic twins discordant for HPS, belonging to the British TwinsUK collection (48). Methylation data were available for 43 whole-blood samples. Three subjects were not considered in the analysis due to missing data and unfeasibility to assign them unequivocally to one of the phenotypic classes, thus leaving 20 twin pairs. The individuals ranged in age between 47 and 76 years old. The heat pain suprathreshold (HPST) scores were obtained with quantitative sensory testing (QST) and discordance was defined as a minimum difference of 2°C within the twin pairs. On the basis of HPST values, we assigned each participant to one of two phenotypic groups: high pain sensitivity (H), i.e., siblings with lower HPST values compared with their co-twin; low pain sensitivity (L), i.e., siblings with higher HPST values compared with their co-twin. The analysis of the HPS dataset was performed considering the entire cohort or dividing it into two subsets, including subjects younger than 60 years old (8 twin pairs) or older than 60 years old (12 twin pairs).
Fimbromyalgia
The FM dataset was retrieved from GEO NCBI repository under accession number GSE85506 (49). This pilot study assessed whole-blood DNAm in female patients with FM using the Infinium Human Methylation 450K BeadChip. It includes 24 cases and 23 age- and sex-matched controls recruited from the Brazilian population. The age range of the cohort was 19–80 years old. One healthy subject was not included in the analysis due to missing information on chronological age. Patients were classified as cases after neurological and psychiatric evaluation, verifying differential diagnosis according to current gold standard guidelines. In addition, FM-positive individuals were clinically characterized with a battery of tests and questionnaires: McGill Pain Questionnaire assessing sensory, affective, evaluative dimension of pain (MPQ_sensory, MPQ_affective, and MPQ_evaluative); Visual Analog Scale (VAS) reflecting the pain intensity; Brief Pain Inventory (BPI) evaluating the interference of painful experience with daily activities (total score) and registering the dosage and efficacy of pharmaceutical treatment (7th item of BPI questionnaire); FM Impact Questionnaire (FIQ) examining the impact of pain on different health domains; Pain Catastrophizing Scale (PCS) measuring a tendency to exaggerated negative attitudes in response to noxious stimuli. Three cases had missing values for the duration time of painful symptoms.
Headache
The Headache dataset is part of an exploratory GWAS longitudinal study on Italian subjects with painful cephalic phenotypes (50). According to the criteria defined by the International Headache Society 3rd edition (beta version), during the clinical examination, all participants were assigned to one of the following phenotypic groups: (i) chronic migraine and medication overuse headache patients (MOH), (ii) episodic migraine patients (EM), and (iii) healthy controls (HC). In this work, we focused on DNAm data collected at baseline time point (T0), which included 22 MOH (20 females, 2 males), 18 EM (17 females, 1 male), and 13 HC (8 females, 5 males). The age range of the subjects was between 24 and 69 years old. Whole-blood DNAm patterns were assessed by the Illumina Infinium Human Methylation EPIC BeadChip.
Data Pre-processing
Raw data files (.idat format) from the three studies were downloaded and separately pre-processed using minfi package within Rstudio software (version 3.5.1) in Linux environment. minfi package provides tools for the analysis of Infinium DNA Methylation microarrays and can handle both 450k and EPIC arrays (51, 52). The pre-processing, quality control, and normalization steps were implemented as recommended by Maksimovic et al. (53). Probes with a detection p-value higher than 0.05 were recognized as failed. Only samples with at least 95% of successfully assessed probes were retained and probes that did not reach significant detection p-values in at least 99% of samples were filtered out. According to these filtering criteria, all the samples from the three cohorts were retained, while 3,493, 2,034, and 4,773 probes were removed in HPS, FM, and MOH/EM datasets, respectively.
Calculation of DNAm Estimates
DNAm estimates were calculated using the New DNA Methylation Age Calculator, an open access tool available at https://dnamage.genetics.ucla.edu/ (44). Pre-processed methylation data were first normalized by the preprocessQuantile function implemented in minfi R package, as suggested in the Horvath's tutorial. Then, beta values matrixes were uploaded in the online tool, selecting the options “Advanced Analysis” and “Normalize Data,” as recommended in the software tutorial. Horvath's epigenetic age calculator returned as output a set of variables including different measures of biological age in blood and of epigenetic age acceleration in blood, DNAm-based surrogate biomarkers of seven plasma proteins, an estimate of smoking cigarette pack per year (these eight measures are components of GrimAge prediction), and an estimate of telomere length and predictions of blood cell counts. Table 1 provides a detailed list and description of DNAm-based measures that were used for statistical analysis in our work. Two subjects were filtered out in FM dataset as they had outlier values for DNAmAge estimate (values below Q1 – 1.5IQR or above Q3 + 1.5IQR, where Q1 and Q3 are first and third quartile, respectively, and IQR refers to interquartile range), reducing the total number of analyzed samples to 44 (24 cases and 20 controls). No outlier was found in the case of HPS and MOH/EM cohorts and all samples were retained.
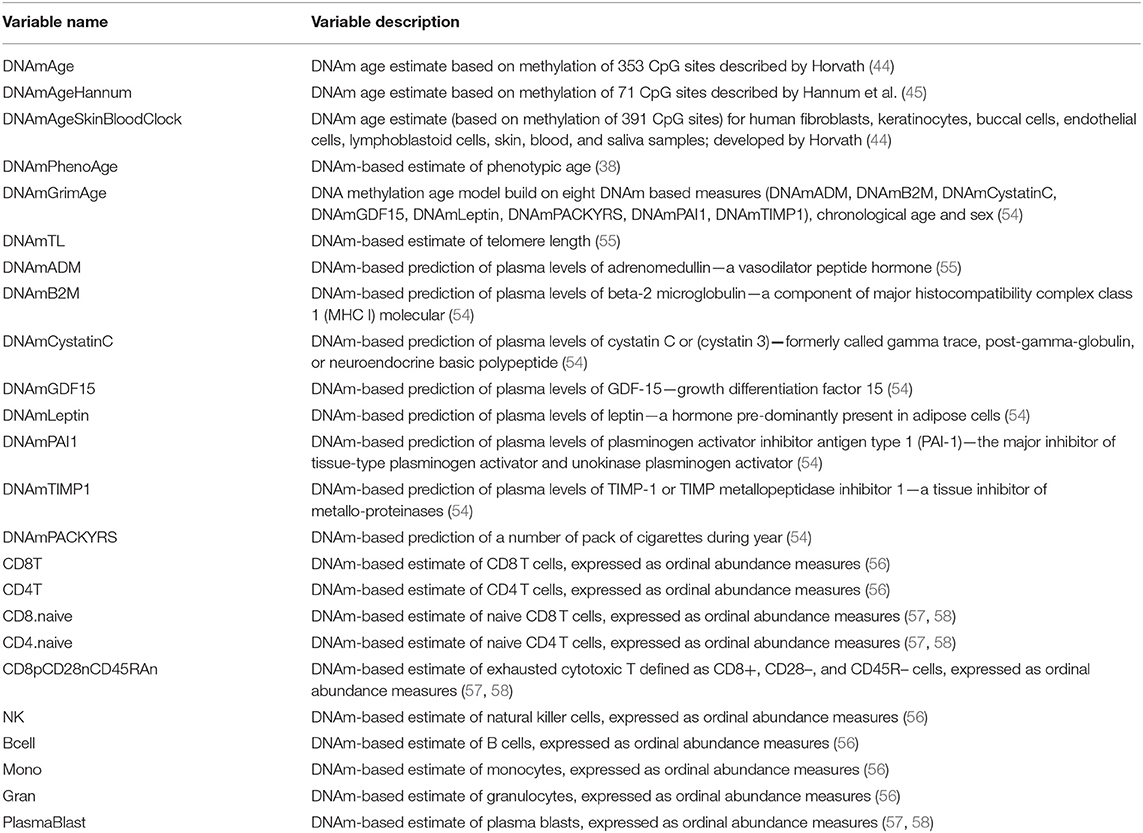
Table 1. List of variables calculated by the new DNA methylation age calculator available online at https://dnamage.genetics.ucla.edu/.
Statistical Analysis
Different methods of calculating biological age acceleration have been applied so far (59). Multiple linear regression (MLR) has been used to examine the influence of the disease status on DNAm age, correcting for chronological age and additional potential confounders. Alternatively, comparison of residuals of DNAm age regressed on chronological age (two-stage residual-outcome regression analysis, 2SR) has been largely used, although in genetic association studies, it has been shown that this method can lead to bias (60), and this could be true also in the case of epigenetics. To achieve consistent results, in this work, we have conducted parallel analyses and, for each of the epigenetic estimates listed in Table 1, we have compared the phenotypic groups using MLR or 2SR.
More specifically, in the first approach (MLR), the differences in each epigenetic variable among the phenotypic groups were examined building a linear regression model correcting for chronological age: lm(Epigenetic_variable ~ Group + Age). For HPS twin cohort, the lmer function from the lmerTest R package was used to build a linear mixed model, including family as a random effect: lmer(Epigenetic_variable ~ Group + Age + (1/Family)).
In the second approach (2SR), each of the variables was adjusted for chronological age by building a linear regression model on the control group (healthy subjects in FM and MOH/EM cohorts, siblings with lower HPS in the HPS cohort)): lm(Epigenetic_variable[control_group] ~ Age[control_group]. This regression model was then applied to both cases and controls to predict the epigenetic variable under investigation and calculate the chronological age-corrected residuals. Finally, residuals were compared among the phenotypic groups using parametric Student's t-test, or paired Student's t-test in the case of HPS twin cohort.
Prior to hypothesis testing, the distribution of epigenetic variables was tested using the ggqqplot function in the ggpubr R package. According to visual inspection of the plots (data not shown), none of the variables violated the assumption of normality.
Power calculation for MLR and 2SR approaches was performed using the pwr.t.test function from the pwr R package (the powerSim function from simr R package was used for linear mixed models in the HPS cohort). As expected, given the small size of the cohorts, power tended to be low for most of the epigenetic variables; this was true especially for the 2SR approach, as previously reported (61).
Finally, we calculated the association between DNAm-based estimates and continuous clinical variables related to painful phenotypes, correcting for chronological age. In the HPS cohort, HPST values were considered and a linear mixed model was built, including family as a random effect: lmer(Epigenetic_variable ~ HPST + Age + (1/Family)). In the FM cohort, several clinical variables (duration of painful symptoms, MPQ, VAS, BPI FIQ, and PCS scores) were considered as follows: lm(Epigenetic_variable ~ Clinical_variable + Age).
The results from all the analyses described above were corrected with Benjamini–Hochberg procedure for multiple tests: “locally”—within a single cohort and “globally”—within all the cohorts included in the study. The statistical significance level in all hypothesis tests was defined as α = 0.05.
All the analyses were conducted using R software (version 3.6.0 in Linux environment).
Results
In our analysis, we considered three datasets of pain-related conditions: HPS, FM, and headache (MOH/EM). The characteristics of each dataset are summarized in Table S1. In each dataset, we analyzed a series of variables returned by the Horvath's epigenetic age calculator, including (1) different measures of epigenetic age (DNAmAge, DNAmAgeHannum, DNAmAgeSkinBloodClock, DNAmPhenoAge, GrimAge); (2) a DNAm-based estimate of telomere length (DNAmTL); (3) DNAm surrogates of components that contribute to GrimAge (abundance of adrenomedullin, DNAmADM; abundance of beta-2 microglobulin, DNAmB2M; abundance of cystatin C, DNAmCystatinC; abundance of growth differentiation factor 15, DNAmGDF15; abundance of leptin, DNAmLeptin; abundance of plasminogen activator inhibitor antigen type 1, DNAmPAI1; abundance of metallopeptidase inhibitor 1, DNAmTIMP1; predicted number of pack of cigarettes during year, DNAmPACKYRS); and (4) DNAm-based predictions of blood cell counts (CD8 T cells, CD8T; CD4 T cells, CD4T; naive CD8 T cells, CD8.naive; naive CD4 T cells, CD4.naive; joined estimation of CD8+, CD28–, and CD45RA– T cells, CD8pCD28nCD45RAn; natural killer cells, NK; B cells, Bcell; monocytes, Mono; granulocytes, Gran; plasma blasts, PlasmaBlast).
As described in the section Materials and Methods, we used two different approaches to compare the epigenetic variables listed above among the phenotypic groups within each dataset. In the first approach (MLR), we performed a MLR analysis correcting for chronological age. In the second approach (2SR), we compared the residuals of the epigenetic variable regressed on chronological age in control subjects within each dataset. Although the latter method has been largely used in the analysis of Horvath's clocks results, it has been associated to bias and loss of power in genetic association studies (60, 61). Accordingly, also in our datasets, the power was higher for the MLR approach compared with 2SR. For this reason, we provide the results of MLR in the main text and report those of the 2SR in Supplementary Materials.
Heat Pain Sensitivity
Twenty monozygotic female twin pairs discordant for HPST were analyzed. The scatterplots of epigenetic estimates of age, DNAmGrimAge components, and blood cell counts against chronological age are reported respectively in Figures S1–S3. The results of the comparison between the twins with lower and higher HPST (using the MLR approach and including family as a random effect, see Materials and Methods) are reported in Table 2. No differences in epigenetic age acceleration were found between discordant twins (Figure 1A and Table 2). When considering nominal p-values, we found significant differences in estimates of CD8+ T blood cell counts (nominal p = 0.028; Table 2; Figure S3A): high pain sensitivity siblings showed decreased levels of CD8+ T cells compared with their co-twin. After correction for multiple tests, the difference in CD8+ T cells did not remain significant.
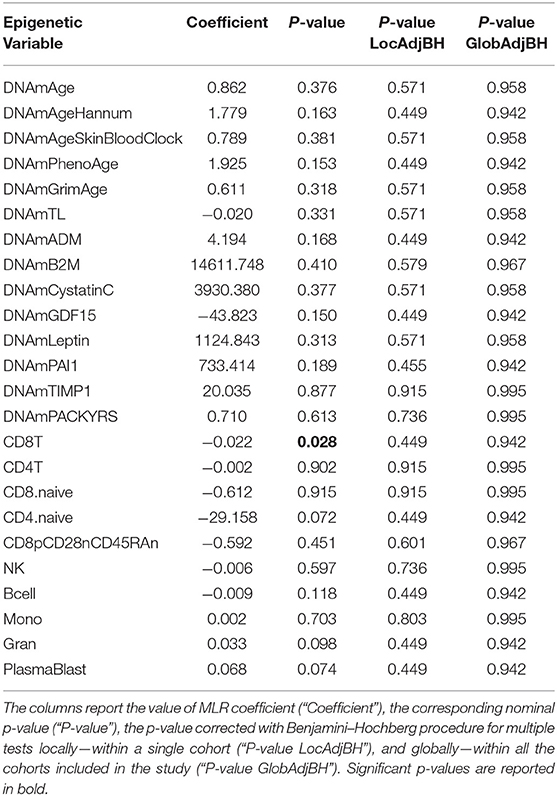
Table 2. Results of statistical hypothesis testing comparing discordant MZ twins with high and low heat pain sensitivity, using the MLR approach correcting for chronological age, and including family as a random effect.
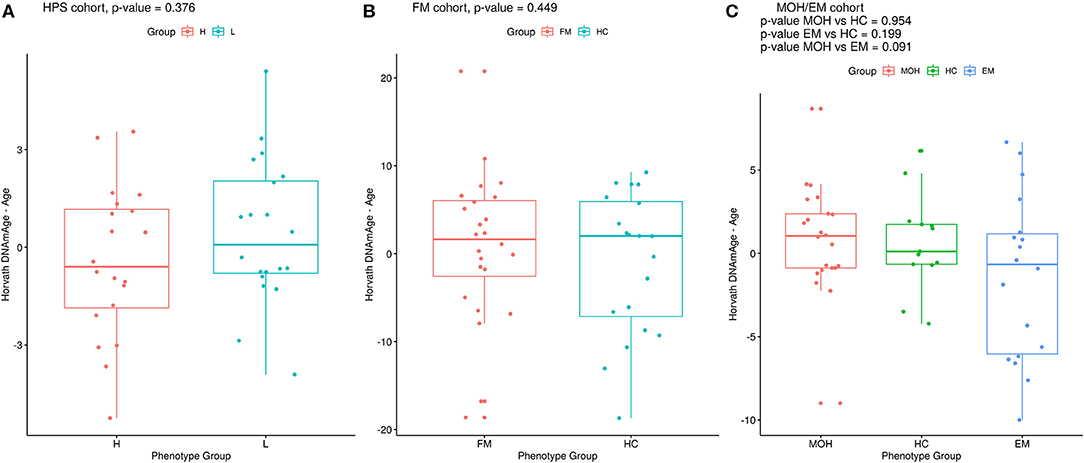
Figure 1. Epigenetic age difference (Horvath's DNAmAge – chronological age) adjusted for chronological age in the phenotypic groups in (A) HPS, (B) FM, and (C) MOH/EM cohorts. Reported p-values are from MLR analysis, as described in the Materials and Methods section.
We next considered the cohort as a whole, without dividing the twins according to HPST, and calculated the associations between HPST and epigenetic estimates using mixed model adjusted on age and including family as a random effect (Table 3). HPSTs were negatively associated with DNAmGDF15 (nominal p = 0.007; Table 3; Figure 2). After correction for multiple tests, this association was no longer significant.
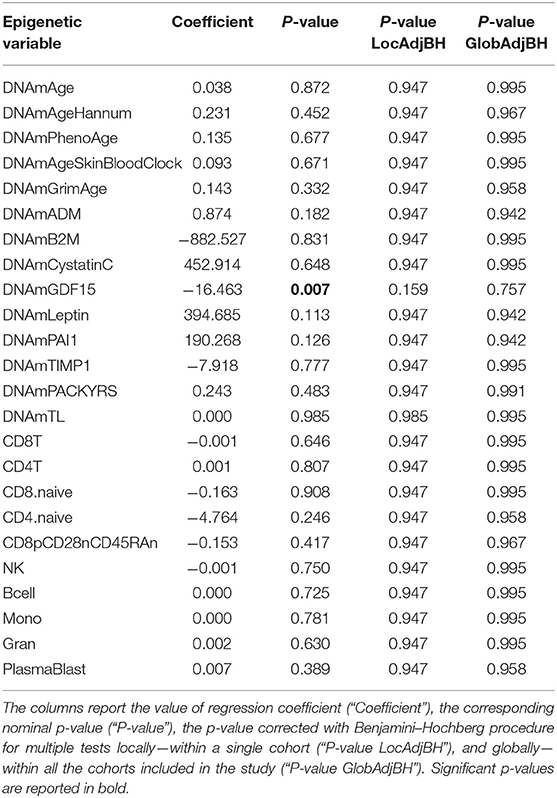
Table 3. Results of association analysis between epigenetic measurements and HPST values in the HPS cohort, correcting for chronological age, and including family as a random effect.
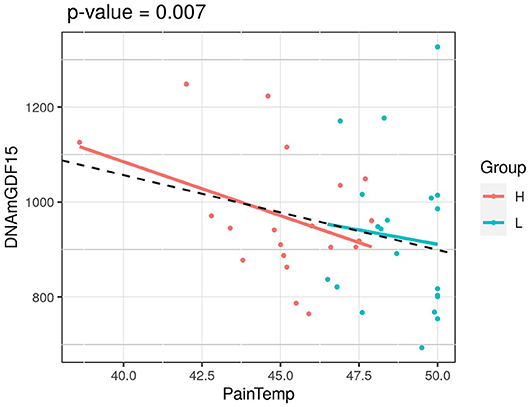
Figure 2. Association of HPST value and DNAmGDF15 in HPS cohort (L, twins with lower heat pain sensitivity; H, with higher heat pain sensitivity). The p-value of a mixed linear model correcting for age and using family as a random effect is reported.
The subjects analyzed in the study by Cruz-Almeida et al. were older than 60 years. Thus, in order to make our results more comparable to those already published, we divided the HPS cohort in two subsets: twin pairs younger and older than 60 years old.
Twin pairs with age above 60 years old (12 couples) presented significant differences in DNAmAgeHannum age estimates (nominal p = 0.021; Table S2), and subjects with higher HPS were found to be epigenetically younger compared with their siblings. In the same subset, discordant twins differed in predicted CD8+ T and B cell counts (nominal p = 0.001 and 0.044, respectively; Table S2), with both estimates increased in more sensitive individuals. Only the difference in predicted CD8+ T cell counts was significant after correction for multiple tests (BH adjusted p = 0.033). No significant associations between epigenetic variables and HPST values were found in this subset (Table S3).
In the subset with subjects younger than 60 years old, DNAmGDF15 estimates were found to be significantly higher among siblings with lower HPST (nominal p = 0.026; Table S2). Association analysis confirmed negative relationship between HPST and DNAmGDF15 in this data subset (rnominal p = 0.002; Table S3). The latter association remained significant after multiple tests correction (BH adjusted p = 0.040).
The results obtained using 2SR approach were comparable to those presented above and are reported in Tables S4, S5.
The power analysis outcomes for MLR and 2SR approaches are reported in Tables S6, S7, respectively.
Fibromyalgia
Twenty-four FM female cases and 20 sex- and age-matched controls that passed the quality control steps were analyzed. The scatterplots of epigenetic estimates of age, DNAmGrimAge components, and blood cell counts against chronological age are presented, respectively, in Figures S4–S6. In MLR analysis, we did not find differences in epigenetic age acceleration comparing FM patients and healthy subjects (Figure 1B and Table 4). The two phenotypic groups differed, however, in the ordinal abundance measure of naive CD4+ T cells adjusted by age, which was significantly lower, at the nominal level, in the affected individuals (nominal p = 0.025; Table 4; Figure S6D). The results obtained with 2SR approach were comparable to those of the MLR approach and are reported in Table S8.
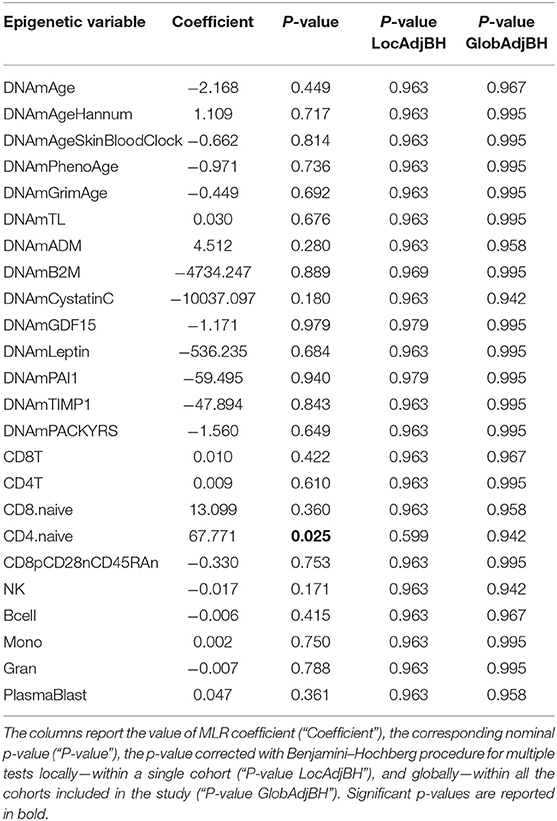
Table 4. Results of statistical hypothesis testing comparing FM patients and healthy individuals (HC), using the MLR approach correcting for chronological age.
Investigation of associations between a set of clinical data and the epigenetic estimates, correcting for chronological age, revealed significant negative association of BPI_interference with DNAmLeptin (nominal p = 0.006; Table 5; Figure 3A) and with predicted CD8+ T cell counts (nominal p = 0.016; Table 5; Figure 3B). The VAS score was also negatively associated with DNAmLeptin (nominal p = 0.013; Table 5; Figure 3C). MPQ_evaluative score was negatively associated with DNAmTL (nominal p = 0.013; Table 5; Figure 3D). Duration of painful symptoms expressed in years and DNAmTL were found to be positively associated (nominal p = 0.034; Table 5; Figure 3E). Finally, a negative association was found between PCS and abundance in NK cells (nominal p = 0.048; Table 5; Figure 3F). None of these associations remained significant after correction for multiple tests.
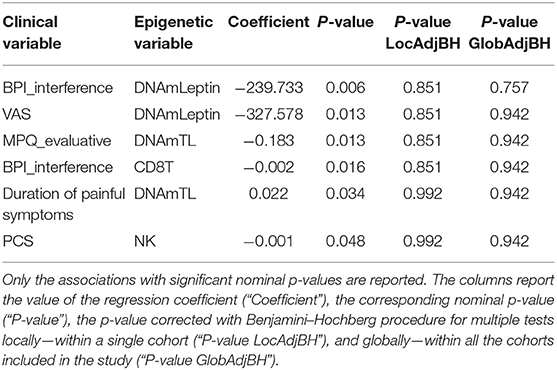
Table 5. Results of association analysis between epigenetic measurements and continuous clinical data related to phenotypes in FM cohort, correcting for chronological age.
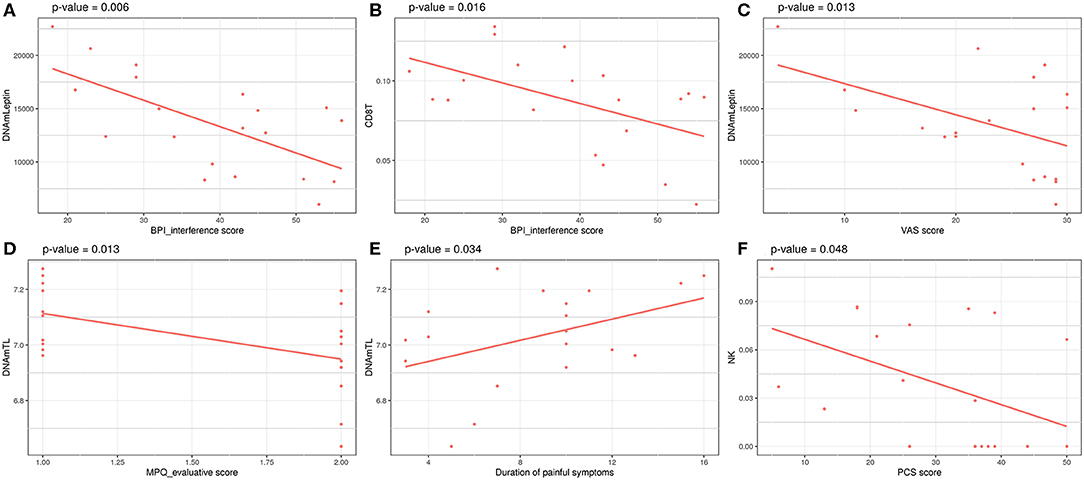
Figure 3. Significant associations between clinical data related to painful phenotype and epigenetic measurements in FM cohort: (A) BPI_interference score vs. DNAmLeptin estimates, (B) VAS score vs. DNAmLeptin estimates, (C) MPQ_evaluative score vs. DNAmTL, (D) BPI_interference score vs. CD8T estimates, (E) Duration of painful symptoms vs. DNAmTL estimates, (F) PCS score vs. NK cells estimates. p-values of a linear model correcting for age are reported.
The outcomes of power calculation for FM dataset are reported in Tables S9, S10.
Headache
Twenty-two MOH patients, 18 EM cases, and 13 HC controls were analyzed. The scatterplots of epigenetic estimates of age, DNAmGrimAge components, and blood cell counts against chronological age are reported, respectively, in Figures S7–S9. MLR did not reveal any significant difference in epigenetic age acceleration, DNAm surrogates comprised in GrimAg, and estimates of telomere length and blood cell counts, between MOH and HC cases or between EM and HC cases (Figure 1C and Table 6). 2SR provided comparable results (Table S11).
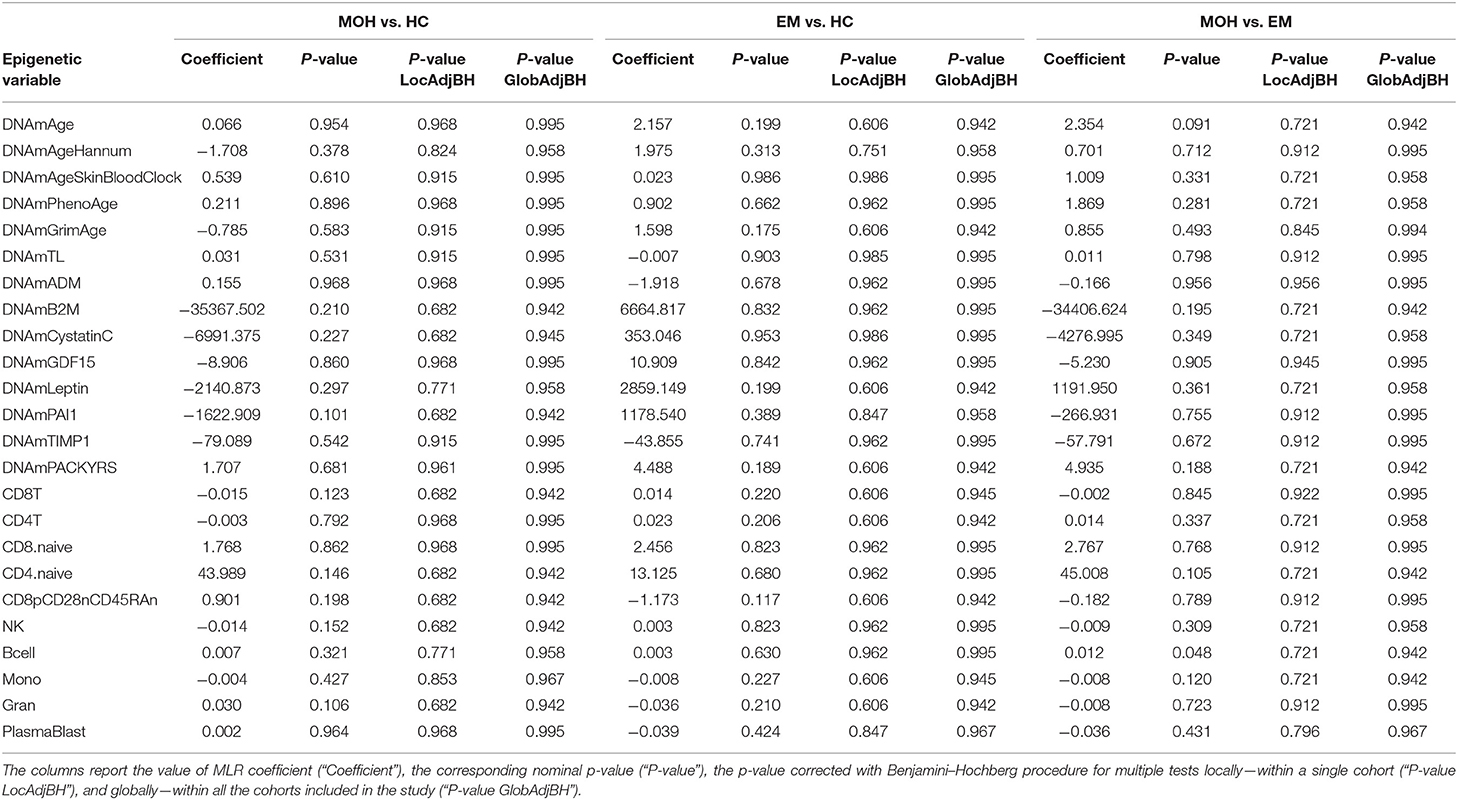
Table 6. Results of statistical hypothesis testing comparing MOH patients, EM patients, and healthy individuals (HC), using the MLR approach correcting for chronological age.
The outcomes of power calculation for MOH/EM dataset are reported in Tables S12, S13.
Discussion
In this study, we analyzed methylation-based estimates of biological aging in three pain-related conditions, for which genome-wide DNAm data were available: HPS, FM, and medication overuse headache/episodic migraine (MOH/EM). In none of the three cohorts did we find evidences of epigenetic age acceleration associated to pain.
So far, only Cruz-Almeida et al. investigated the association between Horvath's epigenetic clock and chronic pain (46). The authors reported higher epigenetic age acceleration, expressed as difference between DNAmAge and chronological age, among 20 participants (age range: 60–83 years old) with persistent painful symptoms during the past 3 months compared with healthy age-matched controls. The study also showed significant negative partial correlations, accounting for age, sex, and race, between heat pain thresholds and epigenetic age. In a subsequent study, authors reported in the same cohort an association between brain age acceleration, predicted by structural neuroimaging, and chronic pain, but not with heat pain thresholds (62). It is worth to be noted that brain age acceleration was not observed in a similar group of chronic pain patients using any kind of pain remedies (63). This result suggests that the association between biomarkers of biological age and pain-related conditions is not obvious and that it can be modulated by several factors, including, for example, the use of medications (64). Thus, the differences between our results and those reported by Cruz-Almeida et al. (46) could be at least in part due to the different pain-related conditions evaluated. Furthermore, it should be noted that most of the subjects included in the FM and in the MOH/EM cohorts were younger than 60 years old, the lowest age in the cohort assessed by Cruz-Almeida et al. The HPS study included a larger number of subjects older than 60 years, but also when we considered this subset of twins, no age acceleration was observed in high pain sensitivity subjects.
It is worth to be noted that the HPS cohort does not involve a pathological phenotype, but is rather representative of differences naturally occurring within a population of individuals of different ages. Nevertheless, this cohort has been successfully used to identify epigenetic changes in the pain gene TRPA1 [(47), p. 1], which have been confirmed in independent studies involving chronic pain patients (65). Several studies investigated if and how heat pain perception changes throughout the life (66–73), but they did not converge to consistent conclusions (67, 74, 75). In our analysis of HPS cohort, we observed a non-significant trend toward lower epigenetic ages in the high pain sensitivity twins. This trend was more marked after 60 years old, when age acceleration calculated by the DNAmAgeHannum predictor was significantly lower in the high pain sensitivity group. At the same time, when considering the cohort as a whole, we observed a non-significant trend toward an inverse association between epigenetic age acceleration (concordantly for all the five clocks) and HPST, similarly to what was observed by Cruz-Almeida et al. (62).
The second cohort that we considered includes female patients suffering from FM, one of the best-studied centralized pain conditions. As firstly proposed and summarized by Hassett et al. (76), FM patients show signs of premature aging, including a decrease in cognitive (77) and physical (78) condition, gray matter atrophy (79, 80) and a trend toward telomere shortening in leukocytes (81). In the latter study, subjects with higher pain intensities and more severe depression had shorter telomeres compared to milder phenotypes. In our cohort, on the contrary, no differences in DNAmTL were found between FM patients and healthy controls, and on the contrary, the duration of painful symptoms was positively associated with DNAmTL. One explanation for this observation is that patients experiencing painful symptoms for a longer time have also a longer history of medication use that can have attenuated age-associated telomere shortening, as previously suggested (82).
Finally, the third cohort that we considered in our study includes patients with MOH and EM. Also in this case, evidences in literature suggest the presence of age-related biological manifestations in the disease. Migraine patients tend to display thinner brain cortex compared with control subjects and this abnormal process seems to become more prominent with advanced chronological age (83, 84). Ren and colleagues reported significantly reduced telomere length among patients suffering from migraine compared with healthy controls (85), while a relationship between migraine and mitochondrial dysfunction has been largely described (86, 87).
Although our results do not provide evidence on acceleration of biological age expressed by epigenetic clocks, we identified a number of additional DNAm-based measures that are associated (mainly at the nominal level of significance) with pain-related phenotypes and that could reflect other alterations that are not captured by the clocks.
In the HPS dataset, we found higher age-adjusted estimates of blood CD8+ T cells counts in twins with high HPS compared with their siblings. This difference was more marked in the subgroup of subjects older than 60 years, where an increase in B cells was also observed. The reasons for this observation are unclear, but possibly related to a different inflammatory status of the co-twins. Changes in predicted blood cell counts were also found in the FM dataset, in which we observed a decrease in predicted CD4+ naive cells in patients and an inverse association between CD8+ T cells and NK cells and the severity of the disease symptoms, assessed as BPI_interference and PCS. Collectively, these results sustain the role of the immune system in pain-related conditions (88).
In the HPS cohort, HPST was negatively associated with DNAmGDF15 levels. Multiple reports showed that plasma levels of GDF15 increase with age (89, 90). Interestingly, GDF15 expression increased in dorsal spinal cord of rats with neuropathic pain (91) and higher serum levels of this protein were detected among myalgic encephalomyelitis/chronic fatigue syndrome patients when compared with healthy subjects (92). In the same study, GDF15 levels were shown to be positively associated with severity of disorder symptoms including fatigue and pain. Thus, our results support the hypothesis that increased levels of GDF15 could contribute to pain sensitivity.
Finally, increased DNAmLeptin levels were associated with less severe FM symptoms. Current data on leptin levels in pain-related conditions are controversial, possible due to high fluctuations in day-to-day leptin measurements (93). One study demonstrated that women with FM serum leptin levels are positively associated with the experience of pain (93). On the contrary, an independent study reported significantly reduced leptin levels in serum of Egyptian FM women compared with controls (94) and researches on animal models of nephropathies suggested that leptin may exert neuroprotective activity and bring pain relief (95–97).
In conclusion, in this paper, we investigated a set of DNAm estimates informative of biological age and of age-related parameters in different pain-related conditions. We did not find evidences of pain-related acceleration in epigenetic age, while we reported some changes in predicted blood cell counts and plasma protein levels. The main strength of our work is that it addresses a research question—the relationship between aging and chronic pain—which has been poorly investigated so far. We implemented a comprehensive approach to analyze age-related DNAm variables in various types of pain-related conditions. However, we are aware that our study has some limitations. The analyzed cohorts had small sample sizes and the statistical power tended to be low, possibly preventing to reach statistically significant results. Furthermore, the study missed replication datasets for each pain-related condition, on which the observed outcomes could be validated. Therefore, additional studies in independent cohorts are required to better characterize chronic pain conditions by epigenetic biomarkers of age.
Data Availability Statement
The datasets analyzed for this study can be found in Gene Expression Omnibus (GEO) NCBI repository (http://www.ncbi.nlm.nih.gov/geo/) under accession numbers GSE53128 and GSE85506.
Author Contributions
KK, PG, and CP contributed to the conception and design of the study. HK, DA, RT, GG, SC, GP, and PC organized the databases. KK, MB, and CS performed the statistical analysis. KK, MB, PG, and CP wrote the manuscript. All authors contributed to manuscript revision, read, and approved the submitted version.
Funding
This project has received funding from the European Union's Horizon 2020 research and innovation programme under the Marie Skłodowska-Curie Grant Agreement No. 721841 PainNET.
Conflict of Interest
The authors declare that the research was conducted in the absence of any commercial or financial relationships that could be construed as a potential conflict of interest.
Supplementary Material
The Supplementary Material for this article can be found online at: https://www.frontiersin.org/articles/10.3389/fpubh.2020.00172/full#supplementary-material
Figure S1. Associations between chronological age and DNAm-based biological age estimates in MZ twins discordant on heat pain sensitivity (L, twins with lower; H, with higher heat pain sensitivity): (A) DNAmAgeHorvath, (B) DNAmAgeHannum, (C) DNAmPhenoAge, (D) DNAmAgeSkinBloodClock, (E) DNAmGrimAge, (F) DNAmTL. P-values of linear regressions are reported for H and L twins separately.
Figure S2. Associations between chronological age and DNAm surrogates of components contributing to DNAmGrimAge in MZ twins discordant on heat pain sensitivity (L, twins with lower; H, with higher heat pain sensitivity): (A) DNAmADM, (B) DNAmB2M, (C) DNAmCystatinC, (D) DNAmGDF15, (E) DNAmLeptin, (F) DNAmPAI1, (G) DNAmTIMP1, (H) DNAmPACKYRS. P-values of linear regressions are reported for H and L twins separately.
Figure S3. Associations between chronological age and DNAm-based predictions of blood cell counts in MZ twins discordant on heat pain sensitivity (L, twins with lower; H with higher heat pain sensitivity): (A) CD8T, (B) CD4T, (C) CD8.naive, (D) CD4.naive, (E) CD8pCD28nCD45RAn, (F) NK, (G) Bcell, (H) Mono, (I) Gran, (J) PlasmaBlast. P-values of linear regressions are reported for H and L twins separately.
Figure S4. Associations between chronological age and DNAm-based biological age estimates in FM and HC samples: (A) DNAmAgeHorvath, (B) DNAmAgeHannum, (C) DNAmPhenoAge, (D) DNAmAgeSkinBloodClock, (E) DNAmGrimAge, (F) DNAmTL. P-values of linear regressions are reported for FM and HC samples.
Figure S5. Associations between chronological age and DNAm surrogates of components contributing to DNAmGrimAge in FM and HC samples: (A) DNAmADM, (B) DNAmB2M, (C) DNAmCystatinC, (D) DNAmGDF15, (E) DNAmLeptin, (F) DNAmPAI1, (G) DNAmTIMP1, (H) DNAmPACKYRS. P-values of linear regressions are reported for FM and HC samples.
Figure S6. Associations between chronological age and DNAm-based predictions of blood cell counts in FM and HC samples: (A) CD8T, (B) CD4T, (C) CD8.naive, (D) CD4.naive, (E) CD8pCD28nCD45RAn, (F) NK, (G) Bcell, (H) Mono, (I) Gran, (J) PlasmaBlast in FM cohort. P-values of linear regressions are reported for FM and HC samples.
Figure S7. Associations between chronological age and DNAm-based biological age estimates in MOH, EM and HC samples: (A) DNAmAgeHorvath, (B) DNAmAgeHannum, (C) DNAmPhenoAge, (D) DNAmAgeSkinBloodClock, (E) DNAmGrimAge, (F) DNAmTL. P-values of linear regressions are reported for MOH, EM and HC samples.
Figure S8. Associations between chronological age and DNAm surrogates of components contributing to DNAmGrimAge in MOH, EM and HC samples: (A) DNAmADM, (B) DNAmB2M, (C) DNAmCystatinC, (D) DNAmGDF15, (E) DNAmLeptin, (F) DNAmPAI1, (G) DNAmTIMP1, (H) DNAmPACKYRS in MOH/EM cohort. P-values of linear regressions are reported for MOH, EM and HC samples.
Figure S9. Associations between chronological age and DNAm-based predictions of blood cell counts in MOH, EM and HC samples: (A) CD8T, (B) CD4T, (C) CD8.naive, (D) CD4.naive, (E) CD8pCD28nCD45RAn, (F) NK, (G) Bcell, (H) Mono, (I) Gran, (J) PlasmaBlast in MOH/EM cohort. P-values of linear regressions are reported for MOH, EM and HC samples.
References
1. Merskey H, Bogduk N (Eds.). IASP Task Force on Taxonomy, Part III: Pain Terms, A Current List with Definitions and Notes on Usage. Seattle, WA: IASP Press (1994). p. 209–14.
2. Sá KN, Moreira L, Baptista AF, Yeng LT, Teixeira MJ, Galhardoni R, et al. Prevalence of chronic pain in developing countries: systematic review and meta-analysis. Pain Rep. (2019) 4:e779. doi: 10.1097/PR9.0000000000000779
3. Tsang A, Von Korff M, Lee S, Alonso J, Karam E, Angermeyer MC, et al. Common chronic pain conditions in developed and developing countries: gender and age differences and comorbidity with depression-anxiety disorders. J Pain. (2008) 9:883–91. doi: 10.1016/j.jpain.2008.05.005
4. Breivik H, Collett B, Ventafridda V, Cohen R, Gallacher D. Survey of chronic pain in Europe: prevalence, impact on daily life, and treatment. Eur J Pain. (2006) 10:287–333. doi: 10.1016/j.ejpain.2005.06.009
5. Brekke M, Hjortdahl P, Kvien TK. Severity of musculoskeletal pain: relations to socioeconomic inequality. Soc Sci Med. (2002) 54:221–8. doi: 10.1016/S0277-9536(01)00018-1
6. Fayaz A, Croft P, Langford RM, Donaldson LJ, Jones GT. Prevalence of chronic pain in the UK: a systematic review and meta-analysis of population studies. BMJ Open. (2016) 6:e010364. doi: 10.1136/bmjopen-2015-010364
7. Greenspan JD, Craft RM, LeResche L, Arendt-Nielsen L, Berkley KJ, Fillingim RB, et al. Studying sex and gender differences in pain and analgesia: a consensus report. Pain. (2007) 132:S26–45. doi: 10.1016/j.pain.2007.10.014
8. Teasell RW, Bombardier C. Employment-related factors in chronic pain and chronic pain disability. Clin J Pain. (2001) 17:S39–45. doi: 10.1097/00002508-200112001-00010
9. Campbell CM, Edwards RR. Ethnic differences in pain and pain management. Pain Manag. (2012) 2:219–30. doi: 10.2217/pmt.12.7
10. Free MM. Cross-cultural conceptions of pain and pain control. Proceedings. (2002) 15:143–5. doi: 10.1080/08998280.2002.11927832
11. Elliott AM, Smith BH, Hannaford PC, Smith WC, Chambers WA. The course of chronic pain in the community: results of a 4-year follow-up study. Pain. (2002) 99:299–307. doi: 10.1016/S0304-3959(02)00138-0
12. Dominick CH, Blyth FM, Nicholas MK. Unpacking the burden: understanding the relationships between chronic pain and comorbidity in the general population. Pain. (2012) 153:293–304. doi: 10.1016/j.pain.2011.09.018
13. Gan TJ. Poorly controlled postoperative pain: prevalence, consequences, and prevention. J Pain Res. (2017) 10:2287–98. doi: 10.2147/JPR.S144066
14. Hitt HC, McMillen RC, Thornton-Neaves T, Koch K, Cosby AG. Comorbidity of obesity and pain in a general population: results from the southern pain prevalence study. J Pain. (2007) 8:430–6. doi: 10.1016/j.jpain.2006.12.003
15. Jank R, Gallee A, Boeckle M, Fiegl S, Pieh C. Chronic pain and sleep disorders in primary care. Pain Res Treat. (2017) 2017:9081802. doi: 10.1155/2017/9081802
16. Veluchamy A, Hébert HL, Meng W, Palmer CNA, Smith BH. Systematic review and meta-analysis of genetic risk factors for neuropathic pain. Pain. (2018) 159:825–48. doi: 10.1097/j.pain.0000000000001164
17. Rainville J, Smeets RJEM, Bendix T, Tveito TH, Poiraudeau S, Indahl AJ. Fear-avoidance beliefs and pain avoidance in low back pain–translating research into clinical practice. Spine J. (2011) 11:895–903. doi: 10.1016/j.spinee.2011.08.006
18. Johnson MI. The landscape of chronic pain: broader perspectives. Medicina. (2019) 55:182. doi: 10.3390/medicina55050182
19. Maleki N, Tahaney K, Thompson BL, Oscar-Berman M. At the intersection of alcohol use disorder and chronic pain. Neuropsychology. (2019) 33:795–807. doi: 10.1037/neu0000558
20. Mior S. Exercise in the treatment of chronic pain. Clin J Pain. (2001) 17:S77–85. doi: 10.1097/00002508-200112001-00016
21. Weingarten TN, Moeschler SM, Ptaszynski AE, Hooten WM, Beebe TJ, Warner DO. An assessment of the association between smoking status, pain intensity, and functional interference in patients with chronic pain. Pain Physician. (2008) 11:643–53.
22. Mills SEE, Nicolson KP, Smith BH. Chronic pain: a review of its epidemiology and associated factors in population-based studies. BJA: Br J Anaesth. (2019) 123:e273–83. doi: 10.1016/j.bja.2019.03.023
23. Cauda F, Palermo S, Costa T, Torta R, Duca S, Vercelli U, et al. Gray matter alterations in chronic pain: a network-oriented meta-analytic approach. NeuroImage Clin. (2014) 4:676–86. doi: 10.1016/j.nicl.2014.04.007
24. Fjell AM, Walhovd KB. Structural brain changes in aging: courses, causes and cognitive consequences. Rev Neurosci. (2010) 21:187–221. doi: 10.1515/REVNEURO.2010.21.3.187
25. Smallwood RF, Laird AR, Ramage AE, Parkinson AL, Lewis J, Clauw DJ, et al. Structural brain anomalies and chronic pain: a quantitative meta-analysis of gray matter volume. J Pain. (2013) 14:663–75. doi: 10.1016/j.jpain.2013.03.001
26. Sibille KT, Chen H, Bartley EJ, Riley JI, Glover TL, King CD, et al. Accelerated aging in adults with knee osteoarthritis pain: consideration for frequency, intensity, time, and total pain sites. Pain Rep. (2017) 2:e591. doi: 10.1097/PR9.0000000000000591
27. Groven N, Fors EA, Reitan SK. Patients with fibromyalgia and chronic fatigue syndrome show increased hsCRP compared to healthy controls. Brain Behav Immun. (2019) 81:172–7. doi: 10.1016/j.bbi.2019.06.010
28. Lasselin J, Kemani MK, Kanstrup M, Olsson GL, Axelsson J, Andreasson A, et al. Low-grade inflammation may moderate the effect of behavioral treatment for chronic pain in adults. J Behav Med. (2016) 39:916–24. doi: 10.1007/s10865-016-9769-z
29. Teodorczyk-Injeyan JA, Triano JJ, Injeyan HS. Nonspecific low back pain: inflammatory profiles of patients with acute and chronic pain. Clin J Pain. (2019) 35:818–25. doi: 10.1097/AJP.0000000000000745
30. Jylhävä J, Pedersen NL, Hägg S. Biological age predictors. EBioMedicine. (2017) 21:29–36. doi: 10.1016/j.ebiom.2017.03.046
31. Horvath S, Raj K. DNA methylation-based biomarkers and the epigenetic clock theory of ageing. Nat Rev Genet. (2018) 19:371–84. doi: 10.1038/s41576-018-0004-3
32. Horvath S, Ritz BR. Increased epigenetic age and granulocyte counts in the blood of Parkinson's disease patients. Aging. (2015) 7:1130–42. doi: 10.18632/aging.100859
33. Levine ME, Lu AT, Bennett DA, Horvath S. Epigenetic age of the pre-frontal cortex is associated with neuritic plaques, amyloid load, and Alzheimer's disease related cognitive functioning. Aging. (2015) 7:1198–211. doi: 10.18632/aging.100864
34. Horvath S, Garagnani P, Bacalini MG, Pirazzini C, Salvioli S, Gentilini D, et al. Accelerated epigenetic aging in down syndrome. Aging Cell. (2015) 14:491–5. doi: 10.1111/acel.12325
35. Horvath S, Oshima J, Martin GM, Lu AT, Quach A, Cohen H, et al. Epigenetic clock for skin and blood cells applied to hutchinson gilford progeria syndrome and ex vivo studies. Aging. (2018) 10:1758–75. doi: 10.18632/aging.101508
36. Maierhofer A, Flunkert J, Oshima J, Martin GM, Haaf T, Horvath S. Accelerated epigenetic aging in werner syndrome. Aging. (2017) 9:1143–52. doi: 10.18632/aging.101217
37. Fransquet PD, Wrigglesworth J, Woods RL, Ernst ME, Ryan J. The epigenetic clock as a predictor of disease and mortality risk: a systematic review and meta-analysis. Clin Epigenetics. (2019) 11:62. doi: 10.1186/s13148-019-0656-7
38. Levine ME, Lu AT, Quach A, Chen BH, Assimes TL, Bandinelli S, et al. An epigenetic biomarker of aging for lifespan and healthspan. Aging. (2018) 10:573. doi: 10.18632/aging.101414
39. Fiorito G, Polidoro S, Dugué PA, Kivimaki M, Ponzi E, Matullo G, et al. Social adversity and epigenetic aging: a multi-cohort study on socioeconomic differences in peripheral blood DNA methylation. Sci Rep. (2017) 7:16266. doi: 10.1038/s41598-017-16391-5
40. Quach A, Levine ME, Tanaka T, Lu AT, Chen BH, Ferrucci L, et al. Epigenetic clock analysis of diet, exercise, education, and lifestyle factors. Aging. (2017) 9:419–46. doi: 10.18632/aging.101168
41. Zhao W, Ammous F, Ratliff S, Liu J, Yu M, Mosley TH, et al. Education and lifestyle factors are associated with DNA methylation clocks in older african americans. Int J Environ Res Public Health. (2019) 16:E3141. doi: 10.3390/ijerph16173141
42. Wolf EJ, Maniates H, Nugent N, Maihofer AX, Armstrong D, Ratanatharathorn A, et al. Traumatic stress and accelerated DNA methylation age: a meta-analysis. Psychoneuroendocrinology. (2018) 92:123–34. doi: 10.1016/j.psyneuen.2017.12.007
43. Wolf EJ, Logue MW, Morrison FG, Wilcox ES, Stone A, Schichman SA, et al. Posttraumatic psychopathology and the pace of the epigenetic clock: a longitudinal investigation. Psychol Med. (2019) 49:791–800. doi: 10.1017/S0033291718001411
44. Horvath S. DNA methylation age of human tissues and cell types. Genome Biol. (2013) 14:R115. doi: 10.1186/gb-2013-14-10-r115
45. Hannum G, Guinney J, Zhao L, Zhang L, Hughes G, Sadda S, et al. Genome-wide methylation profiles reveal quantitative views of human aging rates. Mol Cell. (2013) 49:359–67. doi: 10.1016/j.molcel.2012.10.016
46. Cruz-Almeida Y, Sinha P, Rani A, Huo Z, Fillingim RB, Foster T. Epigenetic aging is associated with clinical and experimental pain in community-dwelling older adults. Mol Pain. (2019) 15:1744806919871819. doi: 10.1177/1744806919871819
47. Bell JT, Loomis AK, Butcher LM, Gao F, Zhang B, Hyde CL, et al. Differential methylation of the TRPA1 promoter in pain sensitivity. Nat Commun. (2014) 5:2978. doi: 10.1038/ncomms3978
48. Moayyeri A, Hammond CJ, Valdes AM, Spector TD. Cohort profile: twinsUK and healthy ageing twin study. Int J Epidemiol. (2013) 42:76–85. doi: 10.1093/ije/dyr207
49. Ciampi de Andrade D, Maschietto M, Galhardoni R, Gouveia G, Chile T, Victorino Krepischi AC, et al. Epigenetics insights into chronic pain: DNA hypomethylation in fibromyalgia—a controlled pilot-study. Pain. (2017) 158:1473. doi: 10.1097/j.pain.0000000000000932
50. Terlizzi R, Bacalini MG, Pirazzini C, Giannini G, Pierangeli G, Garagnani P, et al. Epigenetic DNA methylation changes in episodic and chronic migraine. Neurol Sci. (2018) 39 (Suppl. 1):67–8. doi: 10.1007/s10072-018-3348-8
51. Aryee MJ, Jaffe AE, Corrada-Bravo H, Ladd-Acosta C, Feinberg AP, Hansen KD, et al. Minfi: a flexible and comprehensive Bioconductor package for the analysis of Infinium DNA methylation microarrays. Bioinformatics. (2014) 30:1363–9. doi: 10.1093/bioinformatics/btu049
52. Fortin J-P, Triche TJ, Hansen KD. Preprocessing, normalization and integration of the Illumina HumanMethylationEPIC array with minfi. Bioinformatics. (2017) 33:558–60. doi: 10.1093/bioinformatics/btw691
53. Maksimovic J, Phipson B, Oshlack A. A cross-package bioconductor workflow for analysing methylation array data. F1000Research. (2016) 5:1281. doi: 10.12688/f1000research.8839.2
54. Lu AT, Quach A, Wilson JG, Reiner AP, Aviv A, Raj K, et al. DNA methylation GrimAge strongly predicts lifespan and healthspan. Aging. (2019) 11:303–27. doi: 10.18632/aging.101684
55. Lu AT, Seeboth A, Tsai P-C, Sun D, Quach A, Reiner AP, et al. DNA methylation-based estimator of telomere length. Aging. (2019) 11:5895–923. doi: 10.18632/aging.102173
56. Houseman EA, Accomando WP, Koestler DC, Christensen BC, Marsit CJ, Nelson HH, et al. DNA methylation arrays as surrogate measures of cell mixture distribution. BMC Bioinformatics. (2012) 13:86. doi: 10.1186/1471-2105-13-86
57. Horvath S, Gurven M, Levine ME, Trumble BC, Kaplan H, Allayee H, et al. An epigenetic clock analysis of race/ethnicity, sex, and coronary heart disease. Genome Biol. (2016) 17:171. doi: 10.1186/s13059-016-1030-0
58. Horvath S, Levine AJ. HIV-1 infection accelerates age according to the epigenetic clock. J Infect Dis. (2015) 212:1563–73. doi: 10.1093/infdis/jiv277
59. El Khoury LY, Gorrie-Stone T, Smart M, Hughes A, Bao Y, Andrayas A, et al. Systematic underestimation of the epigenetic clock and age acceleration in older subjects. Genome Biol. (2019) 20:283. doi: 10.1186/s13059-019-1810-4
60. Demissie S, Cupples LA. Bias due to two-stage residual-outcome regression analysis in genetic association studies. Genet Epidemiol. (2011) 35:592–6. doi: 10.1002/gepi.20607
61. Che R, Motsinger-Reif AA, Brown CC. Loss of power in two-stage residual-outcome regression analysis in genetic association studies. Genet Epidemiol. (2012) 36:890–4. doi: 10.1002/gepi.21671
62. Cruz-Almeida Y, Fillingim RB, Riley JL, Woods AJ, Porges E, Cohen R, et al. Chronic pain is associated with a brain aging biomarker in community-dwelling older adults. Pain. (2019) 160:1119–30. doi: 10.1097/j.pain.0000000000001491
63. Sörös P, Bantel C. Chronic non-cancer pain is not associated with accelerated brain aging as assessed by structural MRI in patients treated in specialized outpatient clinics. Pain. (2019) 161:641–50. doi: 10.1097/j.pain.0000000000001756
64. Cruz-Almeida Y, Cole J. Pain aging, and the brain: new pieces to a complex puzzle. Pain. (2019) 161:461–3. doi: 10.1097/j.pain.0000000000001757
65. Sukenaga N, Ikeda-Miyagawa Y, Tanada D, Tunetoh T, Nakano S, Inui T, et al. Correlation between DNA methylation of TRPA1 and chronic pain states in human whole blood cells. Pain Med. (2016) 17:1906–10. doi: 10.1093/pm/pnv088
66. Edwards RR, Fillingim RB, Ness TJ. Age-related differences in endogenous pain modulation: a comparison of diffuse noxious inhibitory controls in healthy older and younger adults. Pain. (2003) 101:155–65. doi: 10.1016/S0304-3959(02)00324-X
67. El-Tumi H, Johnson MI, Tashani OA. An experimental investigation of the effect of age and sex/gender on pain sensitivity in healthy human participants. Open Pain J. (2018) 11:41–51. doi: 10.2174/1876386301811010041
68. Heft MW, Robinson ME. Age Differences in Orofacial Sensory Thresholds. J Dent Res. (2010) 89:1102–5. doi: 10.1177/0022034510375287
69. Helme RD, Meliala A, Gibson SJ. Methodologic factors which contribute to variations in experimental pain threshold reported for older people. Neurosci Lett. (2004) 361:144–6. doi: 10.1016/j.neulet.2003.12.014
70. Lue Y-J, Wang H-H, Cheng K-I, Chen C-H, Lu Y-M. Thermal pain tolerance and pain rating in normal subjects: gender and age effects. Eur J Pain. (2018) 22:1035–42. doi: 10.1002/ejp.1188
71. Magerl W, Krumova EK, Baron R, Tölle T, Treede R-D, Maier C. Reference data for quantitative sensory testing (QST): refined stratification for age and a novel method for statistical comparison of group data. PAIN®. (2010) 151:598–605. doi: 10.1016/j.pain.2010.07.026
72. Petrini L, Matthiesen ST, Arendt-Nielsen L. The effect of age and gender on pressure pain thresholds and suprathreshold stimuli. Perception. (2015) 44:587–96. doi: 10.1068/p7847
73. Silva L, da Lin SM, Teixeira MJ, Siqueira J, de Filho WJ, Siqueira S, et al. Sensorial differences according to sex and ages. Oral Dis. (2014) 20:e103–10. doi: 10.1111/odi.12145
74. Lautenbacher S. Experimental approaches in the study of pain in the elderly. Pain Med. (2012) 13 (Suppl 2):S44–50. doi: 10.1111/j.1526-4637.2012.01326.x
75. Lautenbacher S, Peters JH, Heesen M, Scheel J, Kunz M. Age changes in pain perception: a systematic-review and meta-analysis of age effects on pain and tolerance thresholds. Neurosci Biobehav Rev. (2017) 75:104–13. doi: 10.1016/j.neubiorev.2017.01.039
76. Hassett AL, Clauw DJ, Williams DA. Premature aging in fibromyalgia. Curr Aging Sci. (2015) 8:178–85. doi: 10.2174/1874609808666150727112214
77. Rodríguez-Andreu J, Ibáñez-Bosch R, Portero-Vázquez A, Masramon X, Rejas J, Gálvez R. Cognitive impairment in patients with fibromyalgia syndrome as assessed by the mini-mental state examination. BMC Musculoskelet Disord. (2009) 10:162. doi: 10.1186/1471-2474-10-162
78. Jones J, Rakovski C, Rutledge D, Gutierrez A. A comparison of women with fibromyalgia syndrome to criterion fitness standards: a pilot study. J Aging Phys Act. (2015) 23:103–11. doi: 10.1123/JAPA.2013-0159
79. Kuchinad A, Schweinhardt P, Seminowicz DA, Wood PB, Chizh BA, Bushnell MC. Accelerated brain gray matter loss in fibromyalgia patients: premature aging of the brain? J Neurosci. (2007) 27:4004–7. doi: 10.1523/JNEUROSCI.0098-07.2007
80. Robinson ME, Craggs JG, Price DD, Perlstein WM, Staud R. Gray matter volumes of pain related brain areas are decreased in fibromyalgia syndrome. J Pain. (2011) 12:436–43. doi: 10.1016/j.jpain.2010.10.003
81. Hassett AL, Epel E, Clauw DJ, Harris RE, Harte SE, Kairys A, et al. Pain is associated with short leukocyte telomere length in women with fibromyalgia. J Pain. (2012) 13:959–69. doi: 10.1016/j.jpain.2012.07.003
82. Prasad KN, Wu M, Bondy SC. Telomere shortening during aging: attenuation by antioxidants and anti-inflammatory agents. Mech Ageing Dev. (2017) 164:61–6. doi: 10.1016/j.mad.2017.04.004
83. Chong CD, Dodick DW, Schlaggar BL, Schwedt TJ. Atypical age-related cortical thinning in episodic migraine. Cephalalgia. (2014) 34:1115–24. doi: 10.1177/0333102414531157
84. Magon S, May A, Stankewitz A, Goadsby PJ, Schankin C, Ashina M, et al. Cortical abnormalities in episodic migraine: a multi-center 3T MRI study. Cephalalgia. (2019) 39:665–73. doi: 10.1177/0333102418795163
85. Ren H, Collins V, Fernandez-Enright F, Quinlan S, Griffiths L, Choo K. Shorter telomere length in peripheral blood cells associated with migraine in women. Headache. (2010) 50:965–72. doi: 10.1111/j.1526-4610.2010.01693.x
86. Dong X, Guan X, Chen K, Jin S, Wang C, Yan L, et al. Abnormal mitochondrial dynamics and impaired mitochondrial biogenesis in trigeminal ganglion neurons in a rat model of migraine. Neurosci Lett. (2017) 636:127–33. doi: 10.1016/j.neulet.2016.10.054
87. Yorns WR, Hardison HH. Mitochondrial dysfunction in migraine. Semin Pediatr Neurol. (2013) 20:188–93. doi: 10.1016/j.spen.2013.09.002
88. Laumet G, Ma J, Robison AJ, Kumari S, Heijnen CJ, Kavelaars A. T cells as an emerging target for chronic pain therapy. Front Mol Neurosci. (2019) 12:216. doi: 10.3389/fnmol.2019.00216
89. Conte M, Ostan R, Fabbri C, Santoro A, Guidarelli G, Vitale G, et al. Human aging and longevity are characterized by high levels of mitokines. J Gerontol A Biol Sci Med Sci. (2019) 74:600–7. doi: 10.1093/gerona/gly153
90. Lehallier B, Gate D, Schaum N, Nanasi T, Lee SE, Yousef H, et al. Undulating changes in human plasma proteome profiles across the lifespan. Nat Med. (2019) 25:1843–50. doi: 10.1038/s41591-019-0673-2
91. Cao S, Yuan J, Zhang D, Wen S, Wang J, Li Y, et al. Transcriptome changes in dorsal spinal cord of rats with neuropathic pain. J Pain Res. (2019) 12:3013–23. doi: 10.2147/JPR.S219084
92. Melvin A, Lacerda E, Dockrell HM, O'Rahilly S, Nacul L. Circulating levels of GDF15 in patients with myalgic encephalomyelitis/chronic fatigue syndrome. J Transl Med. (2019) 17:409. doi: 10.1186/s12967-019-02153-6
93. Younger J, Kapphahn K, Brennan K, Sullivan SD, Stefanick ML. Association of leptin with body pain in women. J Women's Health. (2016) 25:752–60. doi: 10.1089/jwh.2015.5509
94. Olama SM, Elsaid TO, El-Arman M. Serum leptin in Egyptian patients with fibromyalgia syndrome: relation to disease severity. Int J Rheum Dis. (2013) 16:583–9. doi: 10.1111/1756-185X.12155
95. Fernández-Martos CM, González P, Rodriguez FJ. Acute leptin treatment enhances functional recovery after spinal cord injury. PLoS ONE. (2012) 7:e35594. doi: 10.1371/journal.pone.0035594
96. Li X, Kang L, Li G, Zeng H, Zhang L, Ling X, et al. Intrathecal leptin inhibits expression of the P2X2/3 receptors and alleviates neuropathic pain induced by chronic constriction sciatic nerve injury. Mol Pain. (2013) 9:65. doi: 10.1186/1744-8069-9-65
Keywords: epigenetic aging, aging biomarker, epigenetic clock, chronic pain, pain sensitivity, fibromyalgia, headache, DNA methylation
Citation: Kwiatkowska KM, Bacalini MG, Sala C, Kaziyama H, de Andrade DC, Terlizzi R, Giannini G, Cevoli S, Pierangeli G, Cortelli P, Garagnani P and Pirazzini C (2020) Analysis of Epigenetic Age Predictors in Pain-Related Conditions. Front. Public Health 8:172. doi: 10.3389/fpubh.2020.00172
Received: 17 January 2020; Accepted: 20 April 2020;
Published: 09 June 2020.
Edited by:
Silvia Polidoro, Italian Institute for Genomic Medicine (IIGM), ItalyReviewed by:
Karin van Veldhoven, University of London, United KingdomChristiana A. Demetriou, University of Nicosia, Cyprus
Copyright © 2020 Kwiatkowska, Bacalini, Sala, Kaziyama, de Andrade, Terlizzi, Giannini, Cevoli, Pierangeli, Cortelli, Garagnani and Pirazzini. This is an open-access article distributed under the terms of the Creative Commons Attribution License (CC BY). The use, distribution or reproduction in other forums is permitted, provided the original author(s) and the copyright owner(s) are credited and that the original publication in this journal is cited, in accordance with accepted academic practice. No use, distribution or reproduction is permitted which does not comply with these terms.
*Correspondence: Paolo Garagnani, cGFvbG8uZ2FyYWduYW5pMkB1bmliby5pdA==
†These authors share first authorship