- 1GFZ German Research Centre for Geosciences, Potsdam, Germany
- 2Central Asian Institute for Applied Geosciences, Bishkek, Kyrgyzstan
The first real-time digital strong-motion network in Central Asia has been installed in the Kyrgyz Republic since 2014. Although this network consists of only 19 strong-motion stations, they are located in near-optimal locations for earthquake early warning and rapid response purposes. In fact, it is expected that this network, which utilizes the GFZ-Sentry software, allowing decentralized event assessment calculations, not only will provide useful strong motion data useful for improving future seismic hazard and risk assessment, but will serve as the backbone for regional and on-site earthquake early warning operations. Based on the location of these stations, and travel-time estimates for P- and S-waves, we have determined potential lead times for several major urban areas in Kyrgyzstan (i.e., Bishkek, Osh, and Karakol) and Kazakhstan (Almaty), where we find the implementation of an efficient earthquake early warning system would provide lead times outside the blind zone ranging from several seconds up to several tens of seconds. This was confirmed by the simulation of the possible shaking (and intensity) that would arise considering a series of scenarios based on historical and expected events, and how they affect the major urban centers. Such lead times would allow the instigation of automatic mitigation procedures, while the system as a whole would support prompt and efficient actions to be undertaken over large areas.
Introduction
The rapid growth of urban areas, especially in developing countries where seismic hazard is significant, calls for improved seismic risk assessment, preparedness and mitigation, in particular in real or near real-time. For this reason, the development of Earthquake Early Warning Systems (EEWS) has been a focus of seismologists and engineers who have shown that the effectiveness of these systems could significantly contribute to seismic risk mitigation (Wenzel and Zschau, 2014; Clinton et al., 2016; Strauss and Allen, 2016; Wu et al., 2016).
However, the implementation of EEWS so far has taken place in high seismic hazard zones where seismic monitoring is realized through dense, high-quality seismic and/or strong-motion networks (Allen et al., 2009). In particular, EEWS have been implemented, either operationally or in the testing phase, in Europe (Italy, Romania), the USA, Japan, Mexico, Turkey, and Taiwan (e.g., Espinosa-Aranda et al., 1995; Böse et al., 2007; Hoshiba et al., 2008; Alcik et al., 2009; Allen et al., 2009; Hsiao et al., 2009; Satriano et al., 2011; Wu et al., 2013).
Furthermore, most of these systems are designed to mainly undertake the rapid assessment of the potential shaking arising from an event, providing decision makers with timely access to information related to the potential losses (and their distribution) that the event could generate (e.g., Pittore et al., 2014; Bindi et al., 2016; Parolai et al., 2016). In the cases where such information would not be able to trigger an alarm within a certain target area (i.e., within the event's blind zone), the resulting real-time information about the amount and distribution of losses would still be of major importance to disaster managers and decision makers (e.g., Pittore et al., 2014).
Although, Central Asia is one of the regions in the world with very high levels of seismic hazard and risk, it is not served by an adequate seismic monitoring network, especially in terms of strong-motion stations. This lack of instruments, and consequently of data, not only inhibits the rapid accurate estimation of loss just after the occurrence of a seismic event, but it also hampers the robust assessment of seismic hazard and risk over time.
For this reason, within the framework of the Advanced Remote Sensing—Ground Truth Demo and Test Facilities (ACROSS1) initiative, the first real-time strong-motion network has been installed in one of the Central Asian countries, namely the Kyrgyz Republic. This network is complemented by the installation of low-cost sensors in eight buildings and a vertical downhole array of accelerometers in the Kyrgyz capital, Bishkek (Parolai et al., 2013; Bindi et al., 2015), in synergy with the Global Change Observatory Central Asia GCO2 initiative of the German Research Centre for Geosciences GFZ. In this paper, we will first describe the newly installed ACROSS network and illustrate the possibilities that the collected data offers for improving seismic hazard and risk assessment in this region. Second, we will describe the potential such a real-time network offers in terms of earthquake early warning, currently in the testing phase, combining both so-called regional and on-site approaches. Regional approaches in this work refer to those involving a network of seismic stations distributed over a region (as is the case for ACROSS), not necessarily in the vicinity of a target site. By contrast, on-site approaches, as the name suggests, involves a station located at the target site itself. In this work, we will demonstrate how combining these approaches can enhance the capacity of an EEWS, even when dealing with a limited number of stations.
In particular, we will show how, even with a small number of stations with nearly optimal locations as defined by previous theoretical studies (Stankiewicz et al., 2013) and limited logistical constraints, an alarm with sufficient lead time could be issued for areas that might suffer serious structural damage, for example, in the case of the repetition of strong historical events like the M = 7.8 Kemin 1911 earthquake (Arrowsmith et al., 2017).
Materials and Methods
The ACROSS Network
In 2017, the ACROSS network was made up of 19 strong-motion stations (Figure 1) distributed throughout the whole of the Kyrgyz Republic. One station located in Bishkek is equipped with a Guralp CMG-5TC3 sensor and a CMG-DM24S12EAM4 digitizer recording continuously at 500 samples per second (sps). The other 18 stations are made up of Nanometrics Centaur Digitisers5 and Titan strong motion sensors6 that are recording in continuous mode at 100 sps. The sensor used in Bishkek has a dynamic range of 145 dB at 1 Hz, while those used at the other stations are slightly more sensitive with a range of 166 dB. The data are transmitted to the Central Asian Institute for Applied Geosciences (CAIAG) in Bishkek in real-time via UMTS and DSL communication protocols.
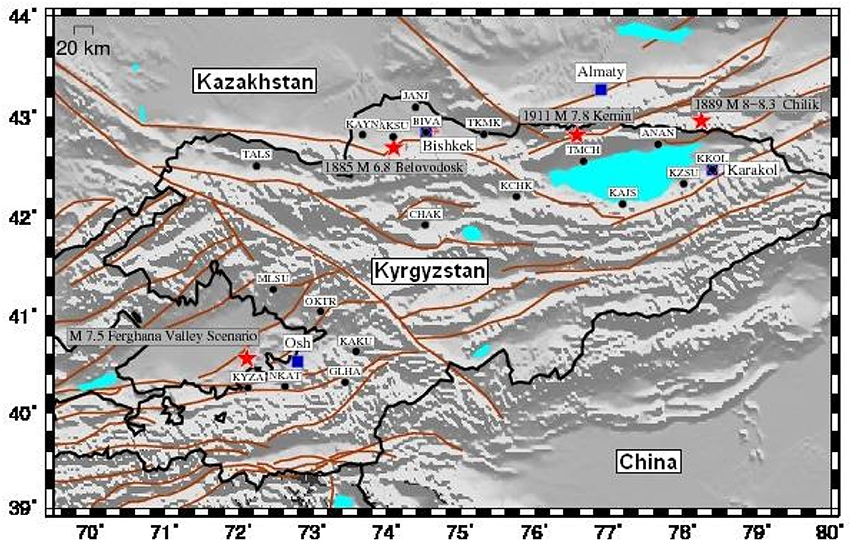
Figure 1. The location of the current stations of the ACROSS network (black circles). Also shown are the main cities (blue squares) and the epicenters of a selection of historical or hypothetical earthquakes (see the text for explanation) considered in this study (red stars). The brown lines indicate the main tectonic alignments and faults identified in this region.
The locations of the stations were chosen, given their limited number, so as to cover areas with high seismicity and the highest population density. However, logistical issues such as accessibility to potential sites (high altitudes or too-remote locations were not considered) and the proximity of infrastructure for real-time data communication were also considered. For choosing an optimal network design, several steps were taken.
First, ad-hoc optimization of the topology of the network was undertaken by Stankiewicz et al. (2015), who demonstrated that regional warning systems are most efficient when they are designed to protect a single, clearly defined target, such as a particular city. A large-scale system to protect multiple targets should thus ideally consist of individual single-target subsystems. Second, starting from the identified optimal station locations, a search for suitable sites nearby (following the logistical considerations mentioned above) was carried out in cooperation with the Ministry of Emergency Situations (MES7) of the Kyrgyz Republic.
This close cooperation with a possible end user of the system allowed the identification of the fire stations of MES (located in proximity of the proposed sites) as safe locations for permanent installations, with nearly continuous power supply and facilitated data communication.
Data
During the period over which the network has operated (which started as non-permanent installations in May 2015), 464 events at local and regional distances have been recorded. Of these events, a total of 2,286 recordings were obtained, covering hypocentral distances ranging from 20 (local) to nearly 1,000 (regional) km, with magnitudes spanning between 3.6 and 7.5 (Figure 2). Information (location, magnitude) about these events was dependent upon the network that provided it: for example, different magnitude scales are used by different networks (i.e., of those used in this work, 94% were in Mb,5% MW and 1% ML). Most of this data are relevant to regional distances and for magnitudes ranging between 4 and 5. The majority of the events were located to the south of the ACROSS network in the Pamir region (36°–39° N, 69°–75° E), as well as around the Ferghana Valley (40°–41° N, 70°–74 E; Figure 2).
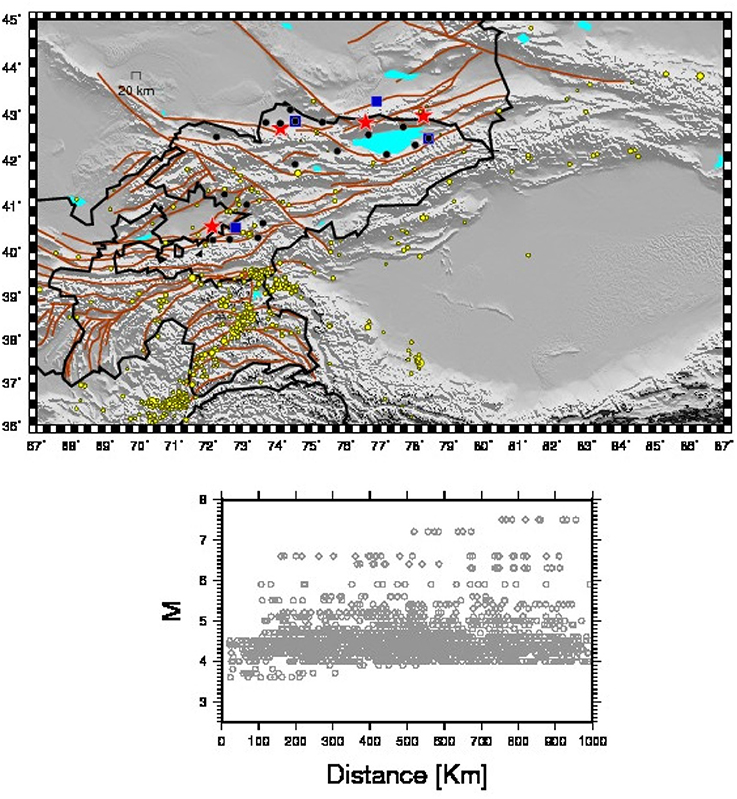
Figure 2. (Top) The locations of the earthquakes recorded by the ACROSS network (yellow circles) since its establishment (May 2015). The red stars and blue squares indicate the positions of a selection of historical events and the main cities of the country, respectively. Black circles indicate the positions of the ACROSS stations. The brown lines indicate some of the main tectonic alignments and faults that have been identified in the region. (Bottom) The distribution of the recorded data in terms of hypocentral distance vs. magnitude.
Results
Decentralized Earthquake Early Warning Performance
During the operation of the network, the signals generated by the recorded earthquakes have been analyzed in real-time by the GFZ-Sentry software (Parolai et al., 2015, 2017). This software, following the concept of Decentralized Earthquake Early Warning System (i.e., the computations are carried out on-site), detects a possible seismic event and, based on the first few seconds (with a maximum of 3 s) of recordings after the triggering of the event, forecasts the expected peak ground velocity (PGV) at the recording site (Zollo et al., 2010; Parolai et al., 2015, 2017). The software considers a combination of the expected median plus/minus the standard deviation of the PGV values with respect to pre-selected thresholds for declaring a certain alarm status (Parolai et al., 2015). The alarm status is defined as being either RED (i.e., the expected PGV is likely to be >8.1 cm/s), meaning an Alarm will be issued since at least light damage is to be expected, i.e., an intensity ≥VI, ORANGE (i.e., the expected PGV is likely to lie between 8.1 cm/s and 3.4 cm/s), when only very light damage is expected, i.e., an intensity equal to V, and GREEN (i.e., the expected PGV is likely to be <3.4 cm/s), when no damage is expected, i.e., an intensity ≤ IV (see Figure 4 in Parolai et al., 2015, 2017).
Figure 3 shows the results obtained during the analyzed period, where the software carried out the analysis correctly for 99.8% of the events. Only one event saw false alarms being issued. This happened when a large (M = 6.6) regional event was recorded at hypocentral distances of around 750–800 km. In this case, large impulsive P-waves were registered on the vertical component, leading to the declaration of a false alarm by three stations (i.e., an ORANGE alert was issued, whereas a GREEN alert was the proper response) Therefore, although the performance of the followed procedure may be considered to be extremely satisfactory, it needs to be noted that none of the analyzed recordings were characterized by an observed PGV value >8.1 cm/s (a factor simply of no events able to induce such ground motion having occurred during the relatively short operational time of the network). Hence, additional analysis would be required to see how the software performs given the occurrence of larger events.
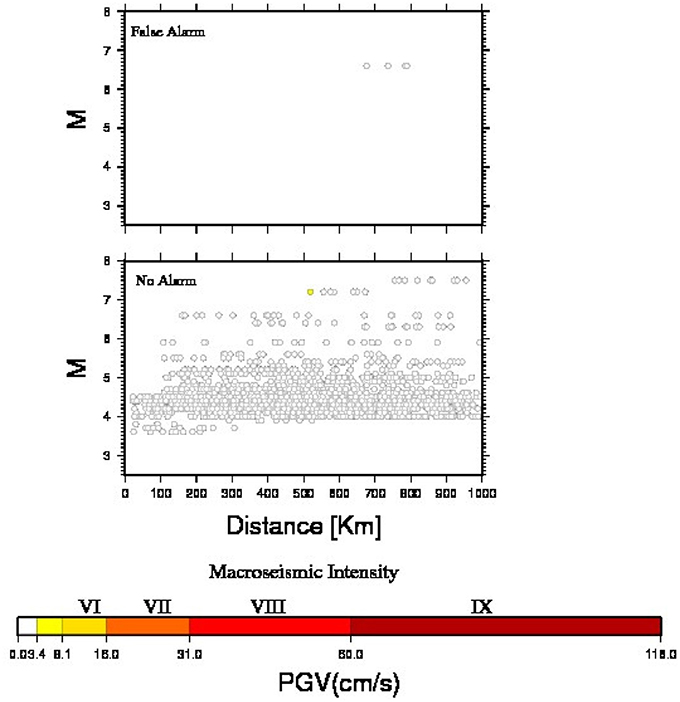
Figure 3. The classification of each analyzed recording as a function of hypocentral distance and magnitude. The top plot represents the recordings for which a false alarm was issued. The bottom plot shows the events for which no alarm was sent out. Note that the observed PGA is always <8.1 cm/s, although for one recording (the lone yellow point in the lower panel), this was above the 3.4 cm/s threshold, and hence should have been denoted with an ORANGE status.
Figure 4 shows the observed PGV values vs. the measured peak amplitude of vertical ground displacement (Pd) found within the analyzed 3-s signal window after triggering. The figure also depicts the relationship between PGV and Pd developed by Zollo et al. (2010) which has been utilized in the system. The relationship seems to perform reasonably well in the region lying within the range of Pd and PGV values for which it was calibrated (gray-shaded area), although when it is applied outside of the range of PGV and Pd values for which it was calibrated, it tends to overestimate the PGV for larger events, which were recorded at regional distances (i.e., for Pd values ranging between 0.05 and 0.1 cm in Figure 4), and underestimate them (the majority) for smaller magnitude events. However, within the range of low Pd values relevant to this paper, the estimated PGV values are still well below the thresholds proposed in Parolai et al. (2015) for a declaration of an ORANGE (3.4 cm/s) or RED (8.1 cm/s) status. Only one recording was observed to overstep the values that would have led to an ORANGE status (note this was the yellow point in the lower panel in Figure 3), but it was classified incorrectly as a no Alarm. Please note that this is due to the fact that both the median and 16th percentile of the expected PGV for a Pd at this level are below the 3.4 cm/s threshold chosen by Parolai et al. (2015), hence a GREEN (No Alarm) status was declared, although the subsequently observed was above this threshold.
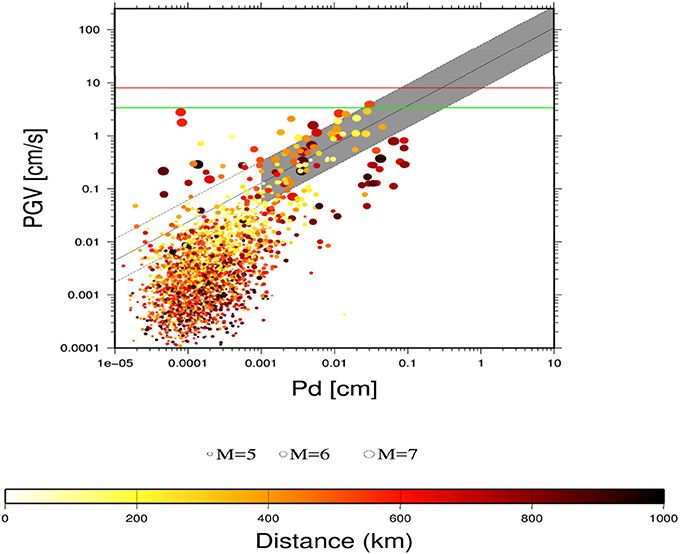
Figure 4. The observed PGV of an event vs. the observed peak amplitude of vertical displacement Pd (circles) derived from the first 3 s of recordings after the event trigger. The size of the symbols indicates the magnitude, while the color shows the hypocentral distance. The continuous and dashed gray lines denote the mean and the standard deviation of the Zollo et al. (2010) relationship. The gray shaded area indicates the range of PGV/Pd over which the relationship was calibrated. The horizontal green line indicates the threshold of PGV = 3.4 cm/s for which a GREEN status is expected (no Alarm), while the red line shows the values above which an Alarm (RED status) should be issued.
Finally, note that during the network testing period, although the GFZ-Sentry software tries to avoid as much as possible false triggering that may be generated by local sources of disturbance (Parolai et al., 2015), even in the absence of a seismic event, the stations were triggered several times. For example, considering the 5 months between January 2017 and May 2017, when the network started working in its full configuration, nearly 140,000 triggers on non-earthquake events were declared. This is due to the installation of the stations in urban areas and inside operational buildings of the MES. However, these triggers never lead to a declaration of a RED status (False Alarm), confirming the appropriate separation of the triggering and the alarming procedure in the software.
Estimation of Lead Times from Regional, Decentralized and Combined Early Warning Schemes
The distribution of the ACROSS network stations can be useful for implementing a national EEWS which combined regional and decentralized on-site procedures. In order to evaluate the potential benefit coming from the implementation of such a EEWS procedure, we calculated the possible lead-time for three different localities in Kyrgyzstan (Bishkek, Karakol, Osh) and one in Kazakhstan (Almaty) by considering the occurrence of earthquakes distributed over a 0.1° (longitude and latitude) grid. A similar study on information-dependent lead-times for the Campania (Southern Italy) region has been carried out by Iervolino et al. (2009).
The lead-times when simulating the performance of a regional approach are calculated after having estimated the P-wave and the S-wave travel times between the hypocenter (i.e., each grid point represents an epicenter) and the target location, and between the hypocenter and the stations' locations (assuming a depth of 10 km, see Adamova et al., 2006). Under the assumption that a reasonable location and magnitude estimate of the earthquake can be obtained when at least three stations are reached by the P-waves, the lead-time is then calculated by subtracting from the S-waves travel time at the target site the P-waves travel time at the last triggered station, further taking into account another 4 s for data analysis and transmission. When determining lead times for a Decentralized Earthquake Early Warning System, the lead-time is calculated as the difference between the S-waves and the P-waves travel times at the site, while again taking into account four extra seconds for data analysis. Note that the GFZ-Sentry software carrying out the decentralized on-site early warning (DOSEW) task uses a maximum signal window length of 3 s for the analysis; hence a window of 4 s as adopted here may be considered to be rather conservative. The resulting times, however, need to be considered the maximum that is available for mitigating actions to be undertaken, although one would expect these to be very rapid (e.g., closing gas lines, school children getting under desks).
In case a combination of the two systems (regional and on-site) is possible (as is the case in Karakol and Bishkek), the final lead-time is chosen for each earthquake location as the larger one. In the following for sake of simplicity, we will show separately the results of the regional, on-site, and combined applications only for the case of Bishkek. For the other considered cities, only either the regional (if there is not a station in the target city) or combined approach results are shown.
Bishkek
Figure 5 shows the resulting lead time isochrones for the gridded earthquake locations obtained following a regional early warning approach. Note that for Bishkek, due to the distribution of the stations of the network, an irregular blind zone area with a dimension of nearly 40 km is expected which could still guarantee sufficient lead-time for earthquakes occurring on most of the major faults indicated in the figure.
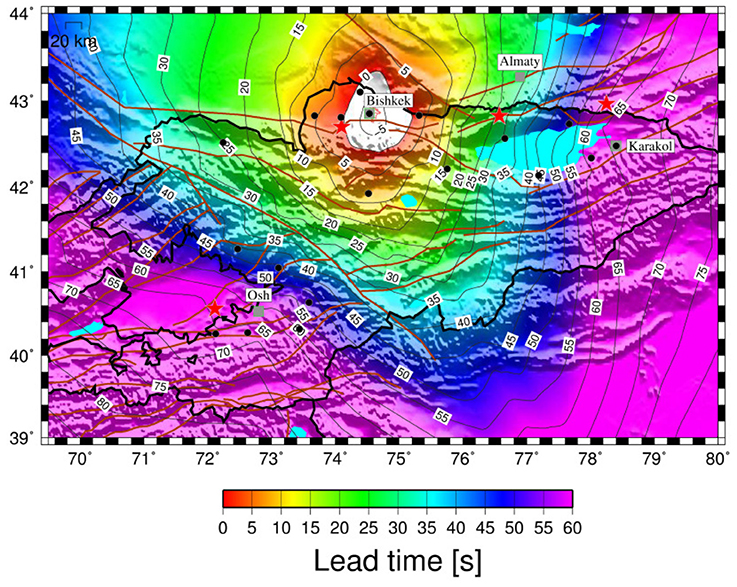
Figure 5. The lead-times for Bishkek when using a regional approach as a function of the location of possible seismic events (i.e., a 0.1 by 0.1° grid). Also shown are the main cities (gray squares) and a selection of historical or hypothetical earthquakes (red stars, see Figure 1 and text for explanation). The brown lines indicate the main tectonic alignments and faults existing in the area.
Figure 6 shows the lead-time results derived based on the on-site approach for station locations in Bishkek. In this case, there is a clear narrowing of the blind zone, in particular in the northern, eastern and southern quadrants. However, for distances larger than around 60–70 km, the advantages of the regional approach appear obvious, with much smaller lead times resulting from the on-site scheme as opposed to those found following the regional approach (Figure 5).
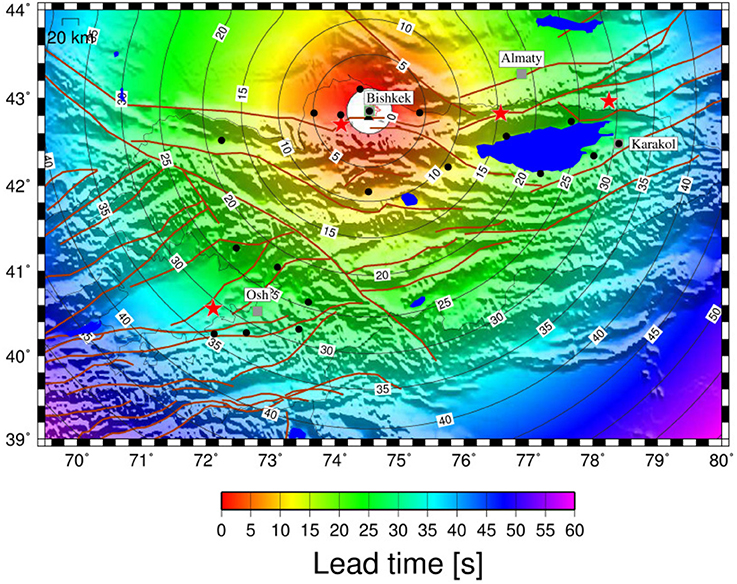
Figure 6. As in Figure 5, but for an on-site approach.
In Figure 7, the results of combining the regional and DOSEW approaches are shown. The figure emphasizes that the use of the DOSEW allows a reduction in the extent of the blind zone compared to the regional approach, while for more distant events, the greater effectiveness of the regional system dominates when compared to the on-site scheme. Interestingly, while Bishkek would be in the blind zone for the case of a repetition of the M = 6.8 1885 Belovodosk earthquake (see Figure 1), nearly 25 s of lead-time would be possible given a repetition of the M = 7.8 1911 Kemin earthquake (Figure 1).
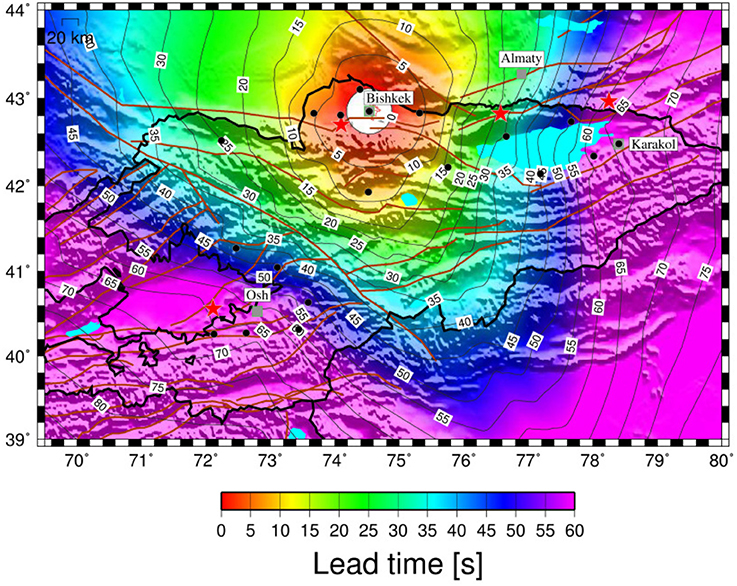
Figure 7. As in Figure 5, but using a combined regional and on-site approach.
Karakol
Figure 8 shows the results obtained for the expected lead times for the city of Karakol when using a combined regional and DOSEW approach. Similarly to what is observed for Bishkek, Figure 8 emphasizes how the use of the DOSEW allows a reduction in the size of the blind zone (resulting in a more circular lead-time isoline) while for more distant events, the effectiveness of the regional system dominates. In particular, due to the station distribution (which sees most stations located west of Karakol), the effectiveness of the regional system is obviously more evident for earthquakes occurring in the western quadrants of Karakol. For example, in the case of the repetition of the M = 7.8 1911 Kemin earthquake, Karakol could benefit from about 25 s of lead-time, while even for a repetition of the strong M = 8–8.3 1889 Chilik earthquake, a few seconds could still be available for starting automatic mitigation procedures (e.g., closure of gas lines).
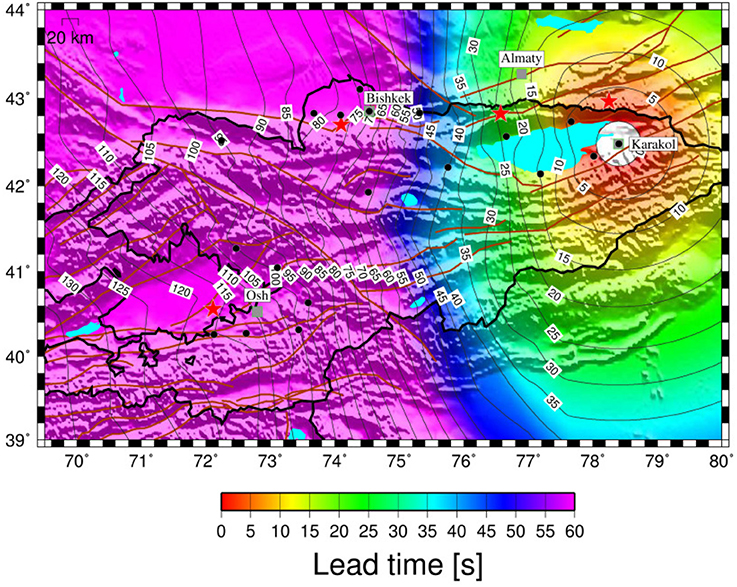
Figure 8. The lead-times for Karakol, using a combined regional and on-site approach, as a function of the location of possible seismic events (i.e., a 0.1 by 0.1° grid). Also shown are the main cities (gray squares) and a selection of historical or hypothetical earthquakes (red stars, see Figure 1 and text for explanation). The brown lines indicate the main tectonic alignments and faults existing in the area.
Osh
As currently no ACROSS station is installed within the city of Osh, only the results derived by applying the regional approach are reported in Figure 9. Although the lead-times for seismic events occurring on the fault structures around the Ferghana Valley (and which could cause damage to the city) range between 5 and 15 s, the blind zone is rather extended, particularly toward the south-west. Nonetheless, tests that have been carried out (not reported here) that indicate that installing a station in the city of Osh would dramatically decrease the size of the blind zone to a radius of around, 30 km, rather than a value of around 60 km which is the case now.
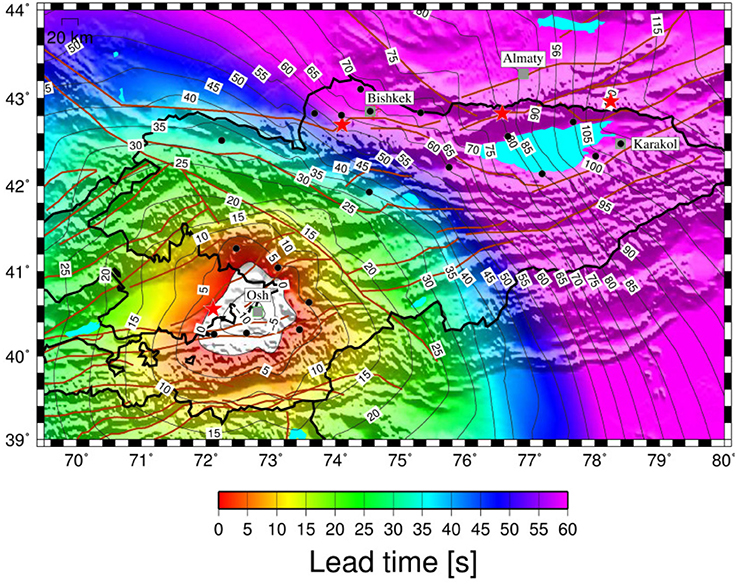
Figure 9. The lead-times for Osh, using a regional approach, as a function of the location of possible seismic events (i.e., a 0.1 by 0.1° grid). Also shown are the main cities considered in this study (gray squares) and a selection of historical or hypothetical earthquakes (red stars, see Figure 1 and text for explanation). The brown lines indicate the main tectonic alignments and faults existing in the area.
Almaty
Although located in Kazakhstan, we show the lead-time results for Almaty from following a regional early warning approach, since this city is of major interest, both in terms of its large population (1.7 million inhabitants) and the fact that it was destroyed or suffered serious damage as a result of events that occurred during historical time (M = 7.3 1887 Verny earthquake, M = 8-8.3 1889 Chilik earthquake, M = 7.8 1911 Kemin earthquake).
The results indicate (Figure 10) that Almaty would benefit from alarms issued in the event of earthquakes occurring on many of the tectonic lineament surrounding the city, including the one that generated the M 8-8.3 Chilik earthquake, with lead-times of the order of 15 s, although not for the 1911 Kemin event which is on the boundary of the blind zone. We see in fact that the blind zone when using only the ACROSS network is rather large (around 60 km). Additional tests carried out showed that by simply including one station in Almaty itself and by following the DOSEEW system would allow a reduction in the blind zone to around 30 km. For example, a few seconds of lead-time would be available even in the case of the repetition of the M = 7.8 1911 Kemin earthquake that destroyed the town. Remarkably, up to 20 s of lead-time would be available in the case of a repetition of the 1889 Chilik earthquake, which resulted in macroseismic intensity values of up to VII on the MSK-64 scale in the city (Mushketov, 1891; Korjenkov et al., 2003).
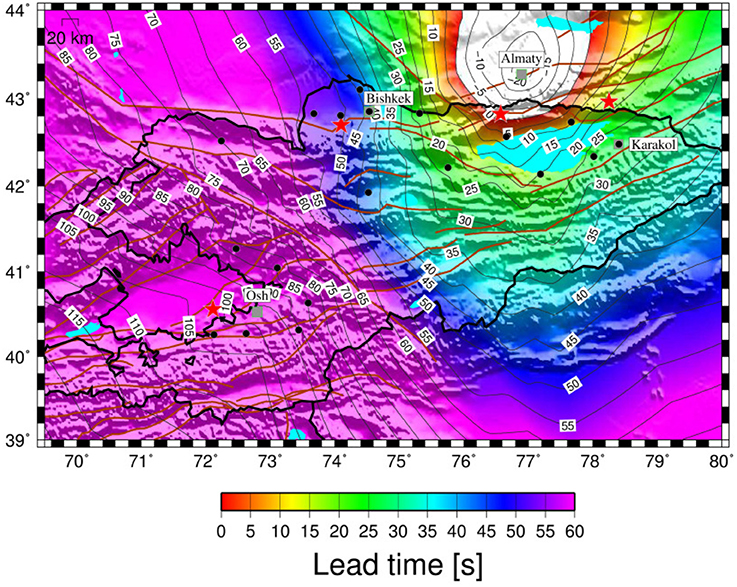
Figure 10. The lead-time for Almaty, using a regional approach, as a function of the location of possible seismic events i.e., a 0.1 by 0.1° grid. Also shown are the main cities considered in this study (gray squares) and a selection of historical or hypothetical earthquakes (red stars, see Figure 1 and text for explanation). The brown lines indicate the main tectonic alignments and faults existing in the area.
Modeling Historical Events and the Resulting Lead Times
In this section, we will present the resulting lead times that would be expected when considering the early warning schemes presented above and a selection of historical and expected events (see Table 1). The modeling is carried out using the OpenQuake (Pagani et al., 2014; Silva et al., 2014) suite of software tools. OpenQuake is a product of the Global Earthquake Model8, a public/private initiative that is setting out to develop tools and standards for earthquake hazard, vulnerability and risk assessment. OpenQuake is a modular program written in the python language, which allows the user not only to define the parameters of the seismic event they wish to model, but to also choose the ground motion prediction equations (GMPEs) and site parameters. For this work, we have undertaken the calculations to obtain peak ground acceleration (PGA), which are then converted to intensity using the relationship of Wald et al. (1999). As there are no GMPEs specifically for Central Asia, we have made use of three GMPEs derived from recordings in similar tectonic environments, namely Akkar et al. (2014), Boore et al. (2014), and Cauzzi et al. (2015). We generated 1,000 random realizations of the ground motion field for each GMPE (leading to a total of 3,000 calculations), then sorted each locations values. The results we present in the following are the median (50th percentile) values. We have assumed bedrock conditions (Vs30 = 760 m/s) for the site response. However, while this would affect the actual ground shaking, it would only very marginally influence the lead times.

Table 1. The historical (Chilik, Kemin) and expected (Ferghana Valley) events considered in this work and modeled using the OpenQuake suite of software tools (Pagani et al., 2014, Silva et al., 2014).
M 7.8 1911 Kemin Earthquake Scenario
The M 7.8 1911 Kemin earthquake (Figure 1, Table 1) occurred close to the border between Kyrgyzstan and Kazakhstan. The city of Almaty, which at that time was populated by nearly 30.000 inhabitants, was mostly destroyed and around 300 persons were reported dead (Bogdanovic, 1911; Velitzky, 1911; Bogdanovich et al., 1914). At the time of the event, the town was mainly made up of adobe and stone buildings that were severely damaged or collapsed, and wooden houses that survived the shaking. Historical sources (Abdrakhmatov et al., 2003) also mention devastation in Bishkek.
The distribution of ground shaking in terms of PGA and macroseismic intensities (Wald et al., 1999) is shown in Figure 11, together with the lead-times, which have been calculated for each grid point (0.1° by 0.1°) into which the area has been subdivided. This lead-time is calculated by subtracting from the S-wave arrival at each considered point the P-wave arrival time (augmented by 4 s) at the third station (in order of time) of the ACROSS network triggered by the event.
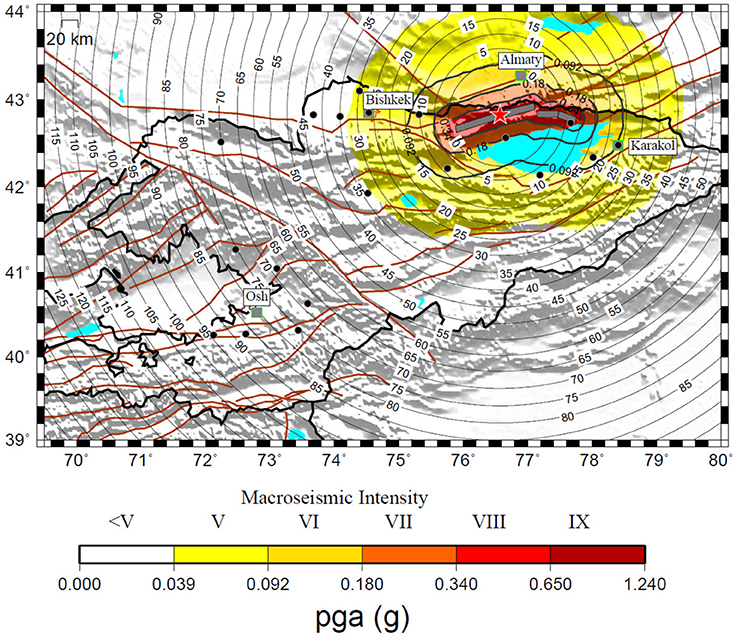
Figure 11. The lead-times available across the Kyrgyz Republic and surrounding area for a repetition of the M 7.8 1911 Kemin earthquake (red star, Table 1), using the regional approach (black contour lines). The distribution of the calculated PGA (macroseismic intensity is found using the relationships of Wald et al., 1999) is shown by the color scale. Also shown are the main cities (gray squares) of the country (see Figure 1). The brown lines indicate the main tectonic alignments and faults existing in the region.
Figure 11 shows that not only a large area (including the cities of Bishkek and Karakol) would suffer light damage (Intensities V–VI), but it would benefit from more than 10 s of lead-time, although, particularly toward the east of the epicenter, there are areas that could be affected by moderate/heavy (Intensities VII–VIII) potential damage. Amongst these there are the localities on the shore line of the Issyk-Kul which are of major touristic interest and where there may be a large concentration of people during the holiday season.
M 8-8.3 1889 Chilik Earthquake Scenario
The M 8-8.3 1889 Chilik earthquake (Figure 1, Table 1) destroyed or damaged about 3,000 buildings near the epicentral area, as well as in the city of Karakol. Although the city of Almaty, which had been destroyed by the M 7.3 1887 Verny (Almaty) earthquake just 2 years before and which had been reconstructed following new restrictive seismic rules that prohibited the construction of adobe buildings, was also heavily damaged, no human losses were reported.
Figure 12 highlights that in case of a repetition of this earthquake, Almaty (estimated intensity VI–VII) could benefit from more than 20 s of lead-time, while Karakol (estimated intensity VI) would lie just at the border of the blind zone. However, as shown in Figure 8, adopting a DOSEEW approach would increase the lead-time of Karakol to a few seconds, which might be sufficient to activate automatic procedures or to adopt simple actions (e.g., taking shelter under a desk, or keeping away from glass windows, Japan Meteorological Association, 2016) that can reduce casualties.
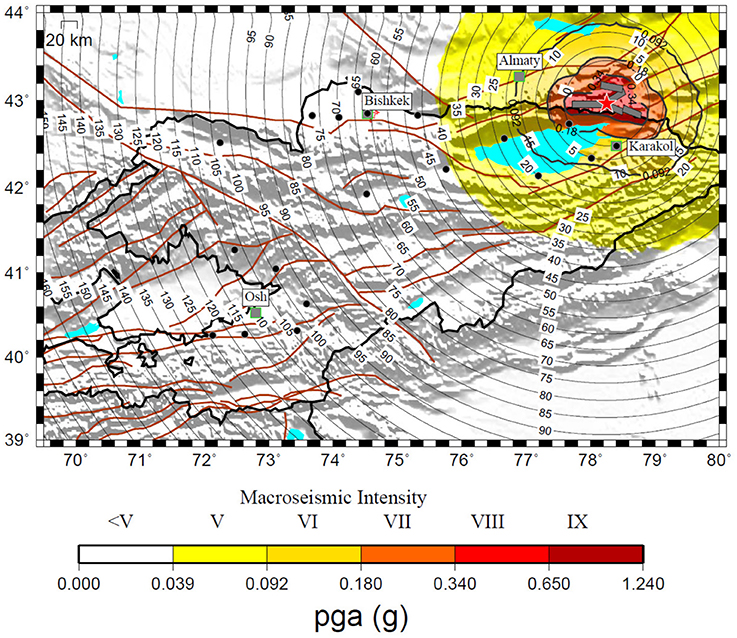
Figure 12. As in Figure 11 but for the M 8-8.3 1889 Chilik earthquake (red star).
M 7.5 Ferghana Valley Earthquake Scenario
As a final example, we calculated the possible scenario for an earthquake occurring in the Ferghana Valley (see Table 1). While there are no major earthquake events we can draw upon as a scenario, the example we present here is considered a worst-case scenario. This example is of major importance since the occurrence of such an event would generate damage not only in Kyrgyzstan, but also in nearby Tajikistan and Uzbekistan, therefore requiring close cross-border cooperation for earthquake risk management.
Figure 13 shows that for large areas in Kyrgyzstan that might be affected by moderate to heavy damage (Intensity VII-VIII), the lead-time could be up to 20 s. Also, areas affected by slight/moderate damage (Intensity V-VI) in Uzbekistan and Tajikistan, if integrated into a transboundary alarm system, could also benefit from several seconds of lead-time.
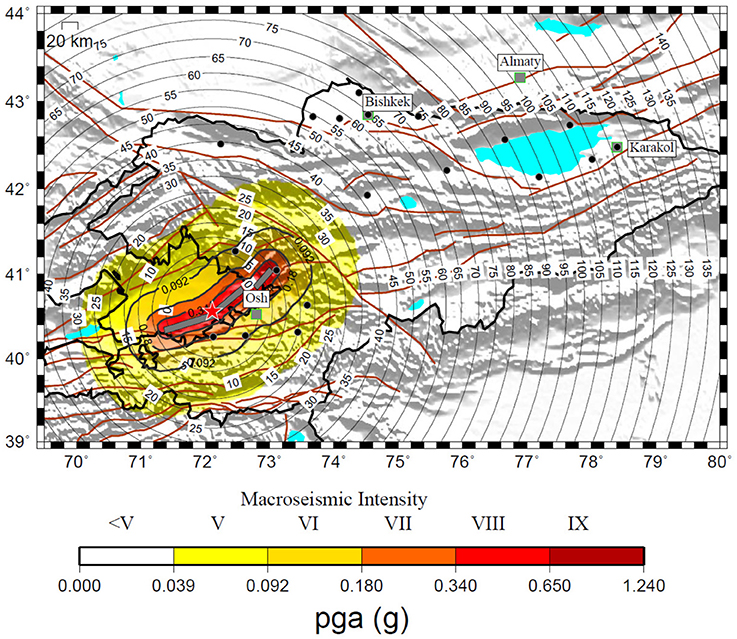
Figure 13. As in Figure 11, but for a scenario involving the Ferghana Valley fault system (M 7.5, red star).
Discussion and Conclusions
In this study we report on the installation of the first real-time digital strong-motion network in Central Asia (ACROSS), namely in the Kyrgyz Republic. The network, which is comprised of 19 stations, has already recorded several minor to major earthquakes at local and regional distances.
The real-time application of the GFZ-Sentry software which allows a decentralized earthquake early warning procedure was shown to perform very well in avoiding (at least for the data tested until now) false alarms. In particular, despite noise signals due to the installation of the strong-motion stations in fire stations of the MES triggering the system, none of these has led to an alarm, confirming the reliability of the procedure in the GFZ-Sentry software in separating the event detection and alarming procedures. However, further testing is required and the performance of the software and the procedures followed should be carefully evaluated when larger local events occur. The tests have been carried out using the relationship proposed by Zollo et al. (2010) based on a global data set. However, in the future new local relationships will be derived making use of the recordings collected by the ACROSS network.
We further carried out scenario simulations to evaluate the possible performance of the network when used for carrying out regional or on-site early warning operations. The tests showed a clear benefit for the major cities in Kyrgyzstan (Bishkek, Osh, and Karakol) if an EEW system would be calibrated and made available for mitigation purposes. The calculations of possible shaking (and intensity) scenarios relevant to historical earthquakes that affected the area highlight that the introduction of an EEWS, provided that proper actions are taken, would allow prompt and efficient response in areas where damage could be moderate, as well as in those that may expect moderate to heavy damages. A similar test for a possible seismic event occurring in the Ferghana Valley outlined that, based on the existing coverage, neighboring countries (Uzbekistan and Tajikistan) could benefit from the provided EEW information. It is therefore recommended that cooperation between these countries be strengthened and the real-time data exchange of seismological networks is foreseen in future.
Associated therewith, the software system CARAVAN (Central Asia Risk and Vulnerability Analysis Tool, Parolai et al., 2016) allows the estimation of the consequences of a seismic event in a probabilistic sense (in the form of human losses) almost in real-time as the strong ground motion parameters are recorded. In this sense, the integration of the real-time data of the ACROSS network into CARAVAN will allow the development of a loss-based earthquake early warning system for the region.
Obviously, while our study emphasizes that there is a potential significant benefit in introducing an earthquake early warning system to the region, without any appropriate initiatives in terms of raising the awareness of the general population as to the value of such a system, and the organizing of training for improving the required response, any action would ineffective. To this regard, we believe that having started the installation of the network in close cooperation with local authorities, who have already introduced educational projects into schools, represents an important step toward a culture of earthquake awareness and sustainable risk mitigation for the Kyrgyz Republic, and potentially the wider Central Asian region. Similarly, the need for enforcing appropriate building codes and sensible land planning schemes are essential for any comprehensive seismic mitigation outlook.
Finally, we want to remark that the data of the ACROSS network are automatically stored in the ITACA data base (Pacor et al., 2011) at the CAIAG institute. The data are automatically processed in order to immediately provide after an event not only the waveforms, but also the parameters of engineering interest. The data base will be publicly available when the project finishes at the end of 2018.
Author Contributions
SP conceptualisation of the work and writing of the manuscript, calculation of lead time calculations and wrote the GFZ-sentry software, TB carried out installations of the network, MarP carried out calculations and data interpretation and helped in installation of the network, KF wrote parts of the manuscript and helped in data interpretation, MH referencing and compilation of literature sources, wrote parts of the manuscript, MasP helped in links to risk assessment, BP helped in data interpretation, BM helped in network location and installation, AZ studied network sites, JL: conceptualisation of the work and final overview.
Conflict of Interest Statement
The authors declare that the research was conducted in the absence of any commercial or financial relationships that could be construed as a potential conflict of interest.
Acknowledgments
The installation of the strong motion network has been financed by the ACROSS initiative of the Helmholtz association. The network installations have been supported by the Global Change Observatory Central Asia of GFZ. We thank the Ministry of Emergency Situations of the Kyrgyz Republic for their permission to install stations on their territory. We acknowledge Azamat Sharshebaev and Ruslan Bolotov for their support in the field activities.
Footnotes
1. ^http://www.gfz-potsdam.de/en/section/ews/infrastructure/
2. ^http://www.gfz-potsdam.de/en/scientific-infrastructure/observatories/regional-observatories/gco-ca/
3. ^http://www.guralp.com/documents/DAS-050-0004.pdf
4. ^http://labotecfz.com/services/digitisers/
5. ^http://www.nanometrics.ca/seismology/products/digitizers/centaur
References
Abdrakhmatov, K. E., Thompson, S., and Weldon, R. (2007). Active Tectonics of the Tien Shan (in Russian). Bishkek: ILIM.
Abdrakhmatov, K., Havenith, H.-B., Delvaux, D., Jongmans, D., and Trefois, P. (2003). Probabilistic PGA and arias intensity maps of Kyrgyzstan (Central Asia). J. Seismol. 7, 203–220. doi: 10.1023/A:1023559932255
Adamova, A. A., Sabitova, T. M., Mirkin, E. L., and Bagmanova, N. H. (2006). “Approximation of distribution of seismic velocities,” in Crustal Parameters of the Tien Shan in Connection with Geodynamics ad Seismicity, ed A. B. Bakirov (Bishkek: ILIM), 9–18.
Akkar, S., Sandıkkaya, M. A., and Bommer, J. J. (2014). Empirical ground-motion models for point- and extended-source crustal earthquake scenarios in Europe and the Middle East. Bull. Earthq. Eng. 12, 359–387. doi: 10.1007/s10518-013-9461-4
Alcik, H., Ozel, O., Apaydin, N., and Erdik, M. (2009). A study on warning algorithms for Istanbul earthquake early warning system. Geophys. Res. Lett. 36. doi: 10.1029/2008GL036659
Allen, R. M., Gasparini, P., Kamigaichi, O., and Böse, M. (2009). The status of earthquake early warning around the world: an introductory overview. Seismol. Res. Lett. 80, 682–693. doi: 10.1785/gssrl.80.5.682
Arrowsmith, J. R., Crosby, C. J., Korzhenkov, A. M., Mamyrov, E., Povolotskaya, I., Guralnik, B., et al. (2017). Surface rupture of the 1911 Kebin (Chon-Kemin) earthquake, Northern Tien Shan, Kyrgyzstan. Geol. Soc. Lond. Special Publ. 432, 233–253. doi: 10.1144/SP432.10
Bindi, D., Boxberger, T., Orunbaev, S., Pilz, M., Stankiewicz, J., Pittore, M., et al. (2015). On-site early-warning system for Bishkek (Kyrgyzstan). Ann. Geophys. 58. doi: 10.4401/ag-6664
Bindi, D., Iervolino, I., and Parolai, S. (2016). On-site structure-specific real-time risk assessment: perspectives from the REAKT project. Bull. Earthq. Eng. 14, 2471–2493. doi: 10.1007/s10518-016-9889-4
Bogdanovic, K. I. (1911). An earthquake of December 22, 1910 (January 4, 1911) in northern chains of the Tien Shan between Verny and Issyk-Kul. Proc. Geol. Comm. 30, 329–419.
Bogdanovich, K. I., Kark, I. M., Korolkov, B. Y., and Muchketov, I. V. (1914). Earthquake of the 4th January 1911 in the northern districts of the Tien Shan. Trans. Geol. Comm. Ser. 89:270.
Boore, D. M., Stewart, J. P., Seyhan, E., and Atkinson, G. M. (2014). NGA-West 2 equations for predicting PGA, PGV, and 5%-damped PSA for shallow crustal earthquakes. Earthq. Spectra 30, 1057–1085. doi: 10.1193/070113EQS184M
Böse, M., Ionescu, C., and Wenzel, F. (2007). Earthquake early warning for Bucharest, Romania: novel and revised scaling relations. Geophys. Res. Lett. 34. doi: 10.1029/2007GL029396
Cauzzi, C., Faccioli, E., Vanini, M., and Bianchini, A. (2015). Updated predictive equations for broadband (0.01–10 s) horizontal response spectra and peak ground motions, based on a global dataset of digital acceleration records. Bull. Earthq. Eng. 13, 1587–1612. doi: 10.1007/s10518-014-9685-y
Clinton, J., Zollo, A., Marmureanu, A., Zulfikar, C., and Parolai, S. (2016). State-of-the art and future of earthquake early warning in the European region. Bull. Earthq. Eng. 14, 2441–2458. doi: 10.1007/s10518-016-9922-7
Delvaux, D., Abdrakhmatov, K., Lemzin, N., and Strom, A. (2001). Landslide and surface breaks of the 1911 M 8.2 Kemin Earthquake. Landslides 42, 1583–1592.
Espinosa-Aranda, J., Jimenez, A., Ibarrola, G., Alcantar, F., Aguilar, A., Inostroza, M., et al. (1995). Mexico City seismic alert system. Seismol. Res. Letters 66, 42–53. doi: 10.1785/gssrl.66.6.42
Hoshiba, M., Kamigaichi, O., Saito, M., Tsukada, S., and Hamada, N. (2008). Earthquake early warning starts nationwide in Japan. EOS Trans. Am. Geophys. Union 89, 73–74. doi: 10.1029/2008EO080001
Hsiao, N.-C., Wu, Y.-M., Shin, T.-C., Zhao, L., and Teng, T.-L. (2009). Development of earthquake early warning system in Taiwan. Geophys. Res. Lett. 36. doi: 10.1029/2008GL036596
Iervolino, I., Giorgio, M., Galasso, C., and Manfredi, G. (2009). Uncertainty in early warning predictions of engineering ground motion parameters: what really matters? Geophys. Rese. Lett. 36, L00B06. doi: 10.1029/2008GL036644
Japan Meteorological Association, P. (2016). Earthquakes and Tsunamis – Disaster Prevention and Mitigation Efforts, Chiyoda-ku. Tokyo.
Korjenkov, A., Baipakov, K., Chang, C., Peshkov, Y., and Savelieva, T. (2003). Traces of ancient earthquakes in medieval cities along the Silk Road, northern Tien Shan and Dzhungaria. Turk. J. Earth Sci. 12, 241–261.
Mushketov, I. V. (1891). “Materials for investigation of earthquakes in Russia,” in Annex to the 27th Volume of Tidings of the Imperial Russian Geographical Society (St. Petersburg, FL).
Pacor, F., Paolucci, R., Luzi, L., Sabetta, F., Spinelli, A., Gorini, A., et al. (2011). Overview of the Italian strong motion database ITACA 1.0. Bull. Earthq. Eng. 9, 1723–1739. doi: 10.1007/s10518-011-9327-6
Pagani, M., Monelli, D., Weatherill, G., Danciu, L., Crowley, H., Silva, V., et al. (2014). OpenQuake Engine: an open hazard (and risk) software for the global earthquake model. Seismol. Res. Lett. 85. doi: 10.1785/0220130087
Parolai, S., Bindi, D., Boxberger, T., Milkereit, C., Fleming, K., and Pittore, M. (2015). On-Site early warning and rapid damage forecasting using single stations: Outcomes from the REAKT project. Seismol. Res. Lett. 86, 1393–1404. doi: 10.1785/0220140205
Parolai, S., Bindi, D., Ullah, S., Orunbaev, S., Usupaev, S., Moldobekov, B., et al. (2013). The Bishkek vertical array (BIVA): acquiring strong motion data in Kyrgyzstan and first results. J. Seismol. 17, 707–719. doi: 10.1007/s10950-012-9347-y
Parolai, S., Boxberger, T., Pilz, M., Bindi, D., Pittore, M., Wieland, M., et al. (2016). Auf dem Weg zur Schadensabschätzung in Echtzeit. Syst. Erde 6, 32–37. doi: 10.2312/GFZ.syserde.06.01.5
Parolai, S., Oth, A., and Boxberger, T. (2017). Performance of the GFZ decentralized on site earthquake Early warning software (GFZ-Sentry): application to K-NET and KiK-net recordings, Japan. Seismol. Res. Lett. doi: 10.1785/0220170048
Pittore, M., Bindi, D., Stankiewicz, J., Oth, A., Wieland, M., Boxberger, T., et al. (2014). Toward a loss-driven earthquake early warning and rapid response system for Kyrgyzstan (Central Asia). Seismol. Res. Lett. 85, 1328–1340. doi: 10.1785/0220140106
Satriano, C., Elia, L., Martino, C., Lancieri, M., Zollo, A., and Iannaccone, G. (2011). PRESTo, the earthquake early warning system for Southern Italy: concepts, capabilities and future perspectives. Soil Dyn. Earthq. Eng. 31, 137–153. doi: 10.1016/j.soildyn.2010.06.008
Silva, V., Crowley, H., Pagani, M., Monelli, D., and Pinho, R. (2014). Development of the OpenQuake engine, the Global Earthquake Model's open-source software for seismic risk assessment. Nat. Hazards 72, 1409–1427. doi: 10.1007/s11069-013-0618-x
Stankiewicz, J., Bindi, D., Oth, A., and Parolai, S. (2013). Designing efficient earthquake early warning systems: case study of Almaty, Kazakhstan. J. Seismol. 17, 1125–1137. doi: 10.1007/s10950-013-9381-4
Stankiewicz, J., Bindi, D., Oth, A., and Parolai, S. (2015). Toward a cross-border early-warning system for Central Asia. Ann. Geophy. 58. doi: 10.4401/ag-6667
Strauss, J. A., and Allen, R. M. (2016). Benefits and costs of earthquake early warning. Seismol. Res. Lett. 87, 765–772. doi: 10.1785/0220150149
Tibaldi, A., Graziooto, E., Forcella, F., and Gapich, V. H. (1997). Morphotectonic indicators of Holocene faulting in central Tien Shan, Kazakstan, and geodynamic implications. J. Geodyn. 23, 23–45. doi: 10.1016/S0264-3707(96)00021-X
Velitzky, S. N. (1911). “An earthquake in Verny town and Semirech'e Oblast (Region) of December 22, 1910 and January 1, (1911),” in Proceedings of Emperor Russian Geographical Society, 47(I/IV) (St. Petersburg, FL), 113–163.
Wald, D. J., Quitoriano, V., Heaton, T. H., and Kanamori, H. (1999). Relationships between Peak Ground Acceleration, Peak Ground Velocity, and Modified Mercalli Intensity in California. Earthq. Spectra 15, 557–564. doi: 10.1193/1.1586058
Walker, R. T., Abdrakhmatov, K., Campbell, G. E., and Mukambayev, A. (2015). “Historic and Prehistoric earthquake ruptures of central Asia,” in Miscellanea INGV, 27, Abstracts Volume of the 6th International INQUA Meeting on Palaeoseismology, Active Tectonics and Archaeoseimology, 19-24 April 2015, Pescina.
Wenzel, F., and Zschau, J. (ed.). (2014). Early Warning for Geological Disasters: Scientific Methods and Current Practice. Berlin: Springer.
Wu, Y., Liang, W., Mittal, H., Chao, W., Lin, C., Huang, B., et al. (2016). Performance of a Low? Cost Earthquake Early Warning System (P-Alert) during the 2016 M L = 6.4 Meinong (Taiwan) Earthquake. Seismol. Res. Lett. 87, 1050–1059. doi: 10.1785/0220160058
Wu, Y.-M., Chen, D.-Y., Lin, T.-L., Hsieh, C.-Y., Chin, T.-L., Chang, W.-Y., et al. (2013). A high-density seismic network for earthquake early warning in taiwan based on low cost sensors. Seismol. Res. Lett. 84, 1048–1054. doi: 10.1785/0220130085
Keywords: earthquake, early warning system, lead time, seismic risk mitigation, Central Asia
Citation: Parolai S, Boxberger T, Pilz M, Fleming K, Haas M, Pittore M, Petrovic B, Moldobekov B, Zubovich A and Lauterjung J (2017) Assessing Earthquake Early Warning Using Sparse Networks in Developing Countries: Case Study of the Kyrgyz Republic. Front. Earth Sci. 5:74. doi: 10.3389/feart.2017.00074
Received: 07 July 2017; Accepted: 04 September 2017;
Published: 20 September 2017.
Edited by:
Mark Naylor, University of Edinburgh, United KingdomReviewed by:
David Jay Wald, United States Geological Survey, United StatesCarmine Galasso, University College London, United Kingdom
Copyright © 2017 Parolai, Boxberger, Pilz, Fleming, Haas, Pittore, Petrovic, Moldobekov, Zubovich and Lauterjung. This is an open-access article distributed under the terms of the Creative Commons Attribution License (CC BY). The use, distribution or reproduction in other forums is permitted, provided the original author(s) or licensor are credited and that the original publication in this journal is cited, in accordance with accepted academic practice. No use, distribution or reproduction is permitted which does not comply with these terms.
*Correspondence: Marco Pilz, cGlsekBnZnotcG90c2RhbS5kZQ==