- National Satellite Meteorological Center, China Meteorological Administration, Beijing, China
Meteorological satellites have become an indispensable tool for weather and land observation. Traditionally, geostationary (GEO) satellites have been used in operational meteorological services due to their high temporal resolution, while polar-orbiting satellites, with their high spatial resolution, are applied more to monitor environmental change and natural disasters. The development of China’s next-generation geostationary meteorological satellites (the FY-4 series) represents an exciting expansion of Chinese non-meteorological remote sensing capabilities. The first satellite (FY-4A) of the FY-4 series was launched on 11 December 2016. The Advanced Geosynchronous Radiation Imager (AGRI) on board FY-4A has 14 spectral bands (increased from the 5 bands of FY-2) that are quantized with 12 bits per pixel (up from 10 bits for FY-2) and sampled at 1 km at nadir in the visible (VIS), 2 km in the near-infrared (NIR), and 4 km in the remaining IR spectral bands (compared with 1.25 km for VIS, no NIR, and 5 km for IR of FY-2). In later satellites in the FY-4A series, the AGRI channel number will be gradually increased from 14 to 18 with IR spatial resolution of 2 km, and the full-disk temporal resolution will be enhanced from 15 to 5 min. With their improved spectral, spatial, and temporal resolution properties, the FY-4 series will gradually approach low earth orbiting (LEO) sensors in spatial and spectral resolution, which will offer greater opportunity and capability for observing small objects and rapid changes in land, ocean, and atmosphere. This review paper provides an introduction to the Chinese FY-4 observation capabilities, a comparison of FY-4 with other new-generation GEO and LEO weather satellites, and associated non-meteorological applications. A series of typical examples based on recent and on-going operational work in National Satellite Meteorological Center of China Meteorological Administration (NSMC/CMA ) that use FY-4A data for non-meteorological applications are demonstrated and discussed, including (i) aerosol monitoring, (ii) dust monitoring, (iii) volcanic ash detection and aviation applications, (iv) fire detection and dynamical evaluation, (v) water body detection, and (vi) floating algae monitoring. The paper concludes with a synthesis of these application areas and the challenges that CMA has to address for future research, technological innovation, and in-depth applications.
Introduction
On 11 December 2016, China’s new-generation geostationary (GEO) meteorological satellite FY-4A was successfully launched at Xichang satellite launch center in the southwest of China. The successful launch of FY-4A and its applications will make it a key component of the international geostationary satellite constellation. FY-4, together with the Geostationary Operational Environmental Satellite (GOES-R, NOAA), Himawari-8/9 (JMA), and Meteosat Third Generation (EUMETSAT) satellites, will offer near-global observations and provide more accurate, more frequent, and more detailed remote sensing products for the monitoring, evaluation, and prediction of the ecological environment at a global scale.
FY-4A has four payload instruments: the Advanced Geosynchronous Radiation Imager (AGRI; the primary payload), the Geostationary Interferometric Infrared Sounder (GIIRS), the Lightning Mapping Imager (LMI), and the Space Environment Package (SEP). AGRI is designed mainly to image the land surface, atmosphere, and cloud targets with high spatial and temporal resolution (Yang et al., 2017). For a long time, the primary use of China’s geostationary meteorological satellites (FY-2 series) was to image the Earth’s weather due to their high temporal resolution. With significant improvement of FY-4A in calibration, geolocation, and observation pattern, the details of the images are clearer and the color is more realistic, demonstrating great potential in non-meteorological areas. Compared with China’s current operational geostationary satellites (FY-2 series), FY-4A has the following technical advances. (1) FY-4A uses three-axis stabilization technology and new image navigation and registration technology that can significantly increase observation efficiency compared with FY-2’s spin stabilization technology, and increase the accuracy of image navigation and registration. (2) FY-4A/AGRI has more spectral bands (increased from 5 in FY-2 to 14), which will greatly improve object detection (e.g., aerosol, vegetation, cloud, snow, fire and water bodies) and quantitative retrievals. (3) The temperature calibration accuracy of FY-4A/AGRI has increased from 1 K (FY-2 series) to 0.1–0.5 K, which helps improve the accuracy of quantitative retrieval of land surface and atmospheric parameters. (4) FY-4A/AGRI samples 1 km at nadir in the visible (VIS), 2 km in the near-infrared (NIR), and 4 km in the remaining IR spectral bands (compared with 1.25 km for VIS, no NIR, and 5 km for IR of FY-2). The highest spatial resolution of FY-4A/AGRI has been increased to 500 m in the red band (centered at 0.65 μm). The enhanced spatial resolution of FY-4A/AGRI will greatly increase detection and parameter retrieval capabilities. Applications that will benefit include the detection of small volcanic eruptions, regional aerosol and dust, small fires, and water body monitoring. (5) FY-4A/AGRI will also improve dynamic monitoring. FY-4A/AGRI’s regional observation mode can provide a regional scan each minute. Through multi-temporal synthesis, more cloud-free observations will be obtained, overcoming the limitations of traditional low Earth orbit (LEO) satellites such as low temporal resolution. FY-4A/AGRI, with its comprehensive improvements, will gradually change how the ecological environment is monitored by making more frequent observations.
Meteorological satellites are important components of Earth observation and their data are not limited to traditional weather applications, but can also be used in non-meteorological application areas. The World Meteorological Organization (WMO) and the Coordination Group for Meteorological Satellites (CGMS) have paid great attention to the development of non-meteorological applications for the new-generation meteorological satellites so as to maximize their usefulness (e.g., Ardanuy et al., 2015). In this study, the observation mode and channel characteristics of FY-4A/AGRI are first compared with other GEO and LEO meteorological satellites, and the potential and challenges of FY-4A/AGRI for non-meteorological applications are further discussed. FY-4A has finished in-orbit tests and data have been released to users worldwide. During the in-orbit tests, FY-4A/AGRI data have been used in a wide range of applications including monitoring of aerosol (e.g., dust) and volcanic ash, fire detection, water body monitoring, and floating algae detection. The related scientific research and operational work of these applications are reviewed and summarized in this paper. Finally, future work is discussed according to FY-4’s mission plan.
Comparison of Fy-4/Agri With Other Geo Instruments
Modes of Operation
FY-4A/AGRI has three flexible scanning modes: full disk (images of the whole Earth as seen from satellite) every 15 min, China area (3–55 °N, 70∼140 °E) every 5 min and target area (1000 km × 1000 km) every 1 min. GOES-R’s Advanced Baseline Imager (GOES-R /ABI) has similar scanning modes: full disk every 15 min and the continental U.S. every 5 min. When storm activity is present, GOES-R/ABI has two smaller scan areas every 1 min (or one every 30 s). In comparison, Himawari 8-9/AHI has more complicated scanning modes including full disk every 10 min, the Japan area every 2.5 min, target area every 2.5 min, and two landmark areas every 30 s (Bessho et al., 2016).
AGRI, ABI, and AHI have extremely flexible scanning modes. For full disk observation, AHI can scan up to every 10 min while AGRI and ABI scan every 15 min. For native continent observation, AGRI and ABI can scan every 5 min while AHI can scan every 2.5 min. In addition, AHI, ABI, and AGRI have similar scanning modes (from every 0.5 to every 1 min) for target area observation. However, at present only full disk AHI data are available to users worldwide, which limits their application in the Asia and Pacific area.
Comparison of Channels
Similarities
Compared with the last generation of geostationary satellites, AHI, AGRI, and ABI have more spectral channels and higher spatial resolution. The spatial resolution is 1 km for the 0.47 μm and 0.83 μm bands and 0.5 km for the 0.64 μm band. AHI, AGRI, and ABI have a band at 0.65 μm for cloud, snow, and volcanic ash detection. They all have bands close to 0.83 μm for aerosol detection and vegetation monitoring; 1.6 and 2.2 μm for snow, aerosol, and cloud particle size retrieval; 6.2 μm for upper troposphere water vapor detection; 8.4 μm for SO2 and cloud top phase detection; and 10.3, 12.3, and 13.3 μm for dust and volcanic ash detection, cloud particle size, and cloud height retrieval and aerosol detection (Table 1).
Differences
In the infrared bands, the spatial resolution of ABI and AHI is 2 km while that of FY-4A/AGRI is 4 km, indicating that FY-4A/AGRI still has a certain disadvantage in detecting fine-scale surface and cloud detail compared with ABI and AHI. Fortunately, this disadvantage will gradually be reduced in subsequent FY-4 satellites. Note also that the spatial resolution of ABI is 1 km at 1.6 μm while AHI and AGRI are still 2 km. This band is used for daytime cloud, snow, and ice discrimination, giving ABI an advantage in these applications over AHI and AGRI.
Besides the difference in spatial resolution, ABI and AHI have three additional bands in the thermal infrared, at 6.9, 9.6, and 11.2 μm. These three bands are used to enhance the capability to distinguish mid-level water vapor and ozone, and are also used in dust and volcanic ash parameter retrieval.
AHI, ABI, and AGRI have unique bands for specific applications (Table 1). AGRI has two middle infrared bands centered at 3.72 μm, “3.72 μm high,” and “3.72 μm low.” The “3.72 μm low” band is sensitive to objects with a low surface temperature. Therefore, it has the potential to detect low-temperature fires such as smoldering fires. AHI has an additional 0.51 μm band, which together with the red and blue bands can produce true-color composite images, whereas ABI and AGRI do not have this band. Only ABI has a 1.37 μm band located in the region where water vapor is strongly absorbed. Therefore, ABI will be more sensitive for thin cirrus detection in a water-vapor-rich area (Schmit et al., 2005). In addition, FY-4A/AGRI and ABI have a 1.37 μm band whereas AHI does not. This band is also located in a region of strong water-vapor absorption, which is helpful for upper-level cirrus. Therefore, AGRI will have an advantage in cirrus detection over AHI in the Asia–Pacific region.
Noise equivalent differential temperature (NEdT) is an important indicator of image quality. In general, the smaller the NEdT, the higher the quality of the image. In addition, due to limitations of hardware technology, NEdT will increase with decreasing spatial resolution. In 13 of the 14 AGRI spectral bands, the NEdT is less than 0.3 K, which is equivalent to the NEdT of AHI (Bessho et al., 2016; Yang et al., 2017). The exception is the 13.2 μm band (NEdT ≤ 0.5). In comparison, the NEdT of ABI in the IR window is less than 0.1, demonstrating the better radiometric performance of ABI.
Comparison of Fy-4/Agri With Leo Instruments
Modes of Operation
In this paper, FY-4A/AGRI is further compared with two LEO instruments: FY-3/MERSI and NPP/VIIRS. The Medium Resolution Spectral Imager (MERSI) is an important instrument on board the Chinese new-generation LEO satellite FY-3 series. The number of spectral bands, the spatial resolution and the radiometric accuracy of MERSI increase from FY-3A to FY-3D (for FY-3D: 25 channels; spatial resolution 250–1000 m; swath width 2916 km). The Visible Infrared Imaging Radiometer Suite (VIIRS) is the main instrument on Suomi NPP and JPSS (22 channels; spatial resolution 370–1000 m; swath width 3060 km; Cao et al., 2013). As the U.S. and China’s second-generation medium-resolution imagers, VIIRS and MERSI are responsible for monitoring the reflective and radiative properties of land, atmosphere, ice, and ocean. Their high spatial and multi-spectral properties give MERSI and VIIRS traditional advantages in detecting land-surface change such as fire dynamics, vegetation change, and ocean color.
As two typical LEO instruments, MERSI and VIIRS scan the Earth twice a day. In comparison, AGRI has more flexible scan modes. Therefore, AGRI has obvious advantages over MERSI and VIIRS for temporal resolution. Through further multi-temporal synthesis, clearer sky images can be acquired by AGRI, which are particularly suited to monitoring rapid changes in the land surface and atmosphere.
Comparison of Channels
We compare the spectral channels and spatial resolutions of AGRI, MERSI, and VIIRS so as to make best use of these different sensors in operational work (Table 2). FY-4A/AGRI has 11 spectral channels while FY-3D/MERSI has 25 and VIIRS has 22. AGRI has 3 spectral channels in the visible and near-infrared, whereas MERSI has up to 15 and VIIRS up to 10. MERSI and VIIRS are more suitable for ocean color monitoring due to the extra channels. The spatial resolution of the AGRI 0.65 μm channel is 500 m, approaching that of the similar channel of MERSI (250 m) and VIIRS (370 m). In the shortwave infrared region, AGRI has similar channels to MERSI and VIIRS, but MERSI and VIIRS have better spatial resolution (2 km for AGRI, 1 km for MERSI and VIIRS). MERSI and VIIRS, but not AGRI, have a special channel (1.24 μm) that is sensitive to cloud particle size. AGRI has two channels in the mid-infrared (“3.75 μm high” and “3.75 μm low”) whereas MERSI and VIIRS have only one channel (3.84 μm for MERSI and 3.74 μm for VIRRS). AGRI has two water-vapor channels in the 6–8 μm range, whereas MERSI and VIIRS do not, demonstrating that AGRI still has advantages in atmospheric monitoring. In the thermal infrared region, AGRI, MERIS, and VIIRS have an 8.5 μm channel and two “split-window” channels, which are helpful for dust, volcanic ash detection, and retrieving cloud top height as well as cloud micro-physical parameters. However, the spatial resolution of AGRI is only 4 km in the thermal infrared region, which is just one fourth that of MERSI and VIIRS. The spatial resolution in the thermal infrared region is the main limitation of AGRI for non-meteorological applications.
Non-Meteorological Applications of Agri
In this section, some non-meteorological applications of FY-4A/AGRI are demonstrated and the advantages and limitations of using FY-4A/AGRI data are discussed further.
Aerosol
Aerosol is an important component of the atmosphere that significantly influences global climate by affecting the radiative energy balance and hydrological cycle (e.g., IPCC, 2007). Aerosol is also the main component of smog and air pollution. The efficient removal of the contribution of the land surface is the first step in aerosol retrieval. The standard methods for this include the Dark Target (DT) algorithm, the multi-angle polarization algorithm (Kahn et al., 1998), and the Deep Blue (DB) algorithm (Remer et al., 2005). The DT algorithm uses the linear relationship between the blue and red channels to effectively remove the contribution of the land surface and has been used in developing the Moderate Resolution Imaging Spectroradiometer (MODIS) aerosol products (Levy et al., 2010). Since FY-4A/AGRI also has the corresponding channels for DT algorithm retrieval, an aerosol optical depth (AOD) product has been developed based on the DT algorithm and FY-4A/AGRI data (the FY-4 aerosol product). Under the assumption of a plane-parallel atmosphere and Lambertian land surface conditions, the FY-4 aerosol product uses the traditional DT algorithm to retrieve clear sky AOD over a dark land surface (e.g., vegetation).
Figure 1 shows a typical AOD retrieval result from FY-4A/AGRI. High AOD values are located mainly in central and eastern China, which agrees well with the distribution of haze on that day. This result is also consistent with the results derived from Himawari/AHI and MODIS with the same algorithm (not shown). The scatter plots in Figure 2 demonstrate that FY-4A/AGRI AOD and AHI AOD are closely correlated (r = 0.925). Similarly, the relationship between FY-4A/AGRI AOD and MODIS AOD is strong, with r up to 0.89. This case study shows that FY-4A/AGRI has comparable accuracy with the widely used GEO and LEO satellites. Compared with LEO instruments, FY-4A/AGRI has better temporal resolution; therefore, FY-4A/AGRI has great potential in detecting hourly variations in aerosol.
Dust
The FY-4A/AGRI dust detection product combines 12 widely used dust detection indexes with the radiative properties measured by FY-4A/AGRI (Zhang et al., 2006; Chen et al., 2016, 2017). The probability density function (PDF) and cumulative probability density function (CDF) are calculated for different backgrounds including cloud, dust, vegetation, and desert. A reliability index is attached to each dust index according to its threshold. Finally, the FY-4A/AGRI dust detection product is the combined result of the 12 dust detection indexes and a corresponding reliability index, so as to avoid missed detection and false detection. FY-4A/AGRI dust detection products include dust detection and the Infrared Difference Dust Index (IDDI). Figure 3 shows a dust event in north China on 4 May 2017. Ground-based dust observation data are used to validate the satellite retrieval of IDDI. The result shows that the FY-4A/AGRI dust product can detect 88% of the ground-based dust observations. This case study shows that the FY-4A/AGRI dust product can effectively detect dust areas, especially under cloud-free conditions. However, when the dust layer is under or mixed with meteorological cloud, the false detection rate is higher.
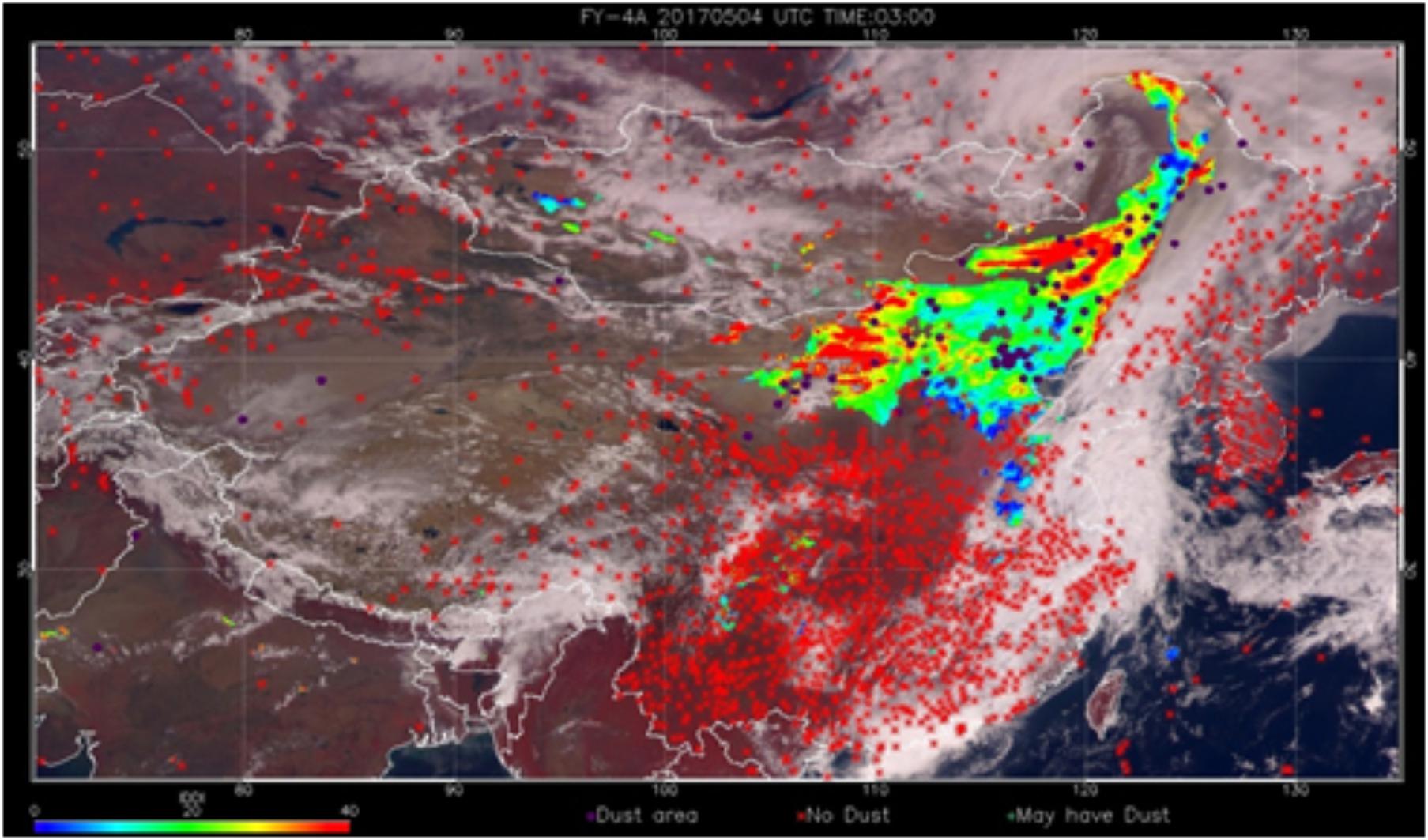
Figure 3. Multi-channel composite image using FY-4A/AGRI 0.83, 0.65, and 0.47 μm channels at 03:00 UTC 4 May 2017. Blue (red) dots represent ground observations of dust (no dust).
Volcanic Ash
Volcanic ash has serious impacts on aviation. As volcanic ash disperses, it also has a long-term influence on climate and the environment (Zhu et al., 2011). FY-4A/AGRI offers a dynamic method of observing visible to infrared signals of volcanic ash from space with high spectral and spatial resolution. A new algorithm for retrieving volcanic ash cloud height from FY-4A/AGRI thermal infrared channels has been developed from the simulation of volcanic ash microphysical properties and statistical optimal estimation theory (Zhu et al., 2017). Unlike previous methods, the new FY-4 ash height algorithm does not require simultaneous atmospheric profiles, providing a flexible way to estimate volcanic ash height using passive satellite infrared measurements (Zhu et al., 2017).
A case study was conducted during the eruption of Indonesia’s Agung volcano (8.34°S, 115.51°E) on 27 November 2017. Large amounts of volcanic ash were being released by Agung volcano at 00:00 UTC. The main body of ash was spreading to the east and had already affected the adjacent Lombok island, 110 km from the crater of the volcano. FY-4A/AGRI’s volcanic ash height retrieval result shows that the volcanic ash was at a height of 1.86–8.38 km, with 96% of the total pixels in the range 2–6 km (Figure 4). The FY-4 ash height algorithm was also applied to Himawari/AHI data, as shown in Figure 5. The height of volcanic ash derived from Himawari/AHI is in the range 2.52–8.40 km, with 81% of the total pixels in the range 2–6 km. The two results display similar spatial variations and have comparable accuracy, with a correlation coefficient of 0.58 and a standard deviation of 0.57 (Figures 4–6). Positive bias is observed for FY-4A/AGRI at lower heights, and FY-4A/AGRI tends to under-estimate the ash height at greater altitudes. One possible reason for the difference between the FY-4A/AGRI and Himawari/AHI ash heights is that we did not account for the spectral response differences between the two instruments. Another reason may be related to the spatial resolution in the thermal infrared channels, which is 2 km for Himawari/AHI and 4 km for FY-4A/AGRI. In addition, positioning deviation between FY-4A/AGRI and Himawari/AHI can give rise to differences in final retrievals, especially at the edge of the volcanic ash region.
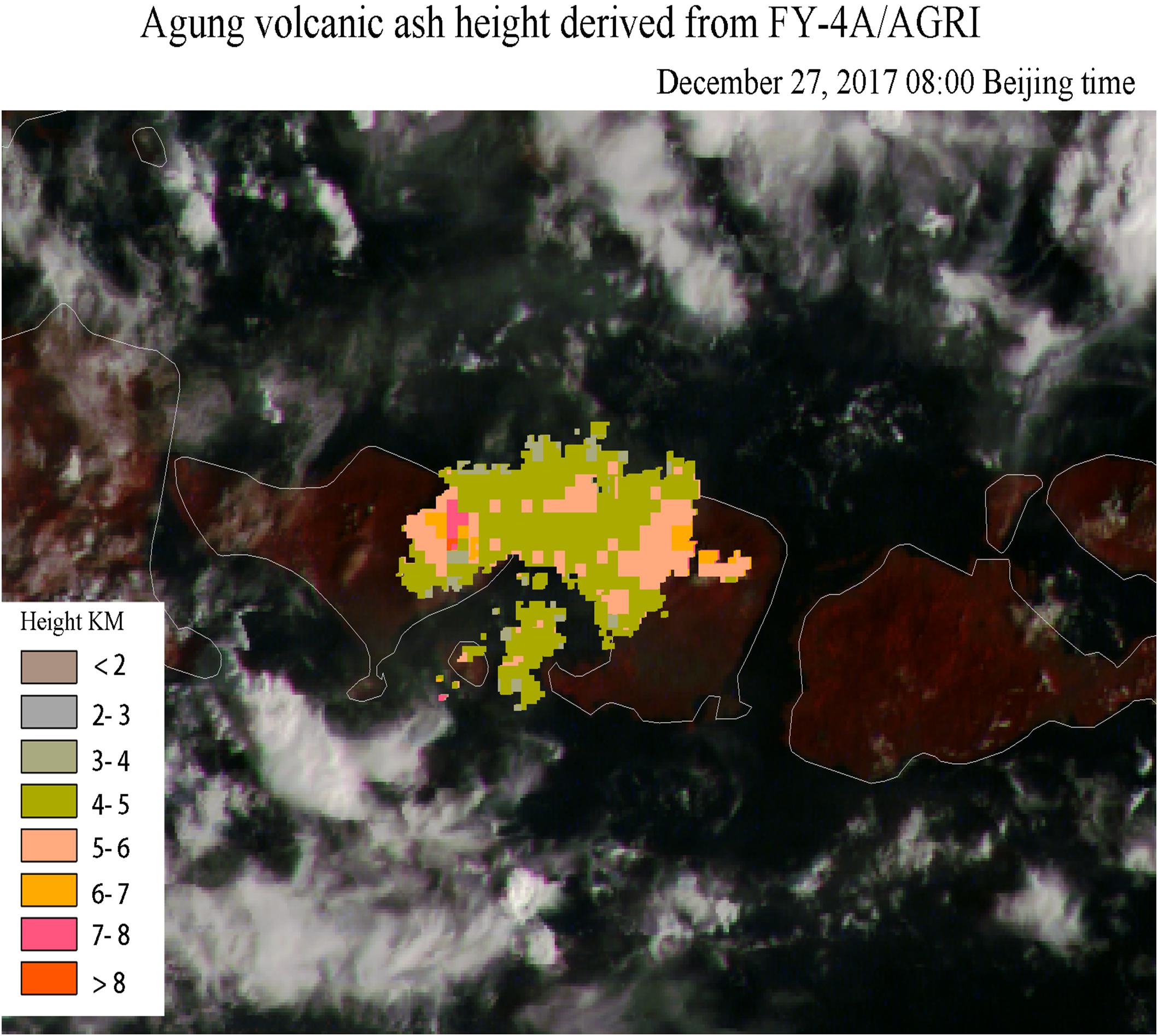
Figure 4. Volcanic ash height derived from FY-4A/AGRI (00:00 UTC 27 November 2017) (4 km resolution for ash detection and ash height retrieval).
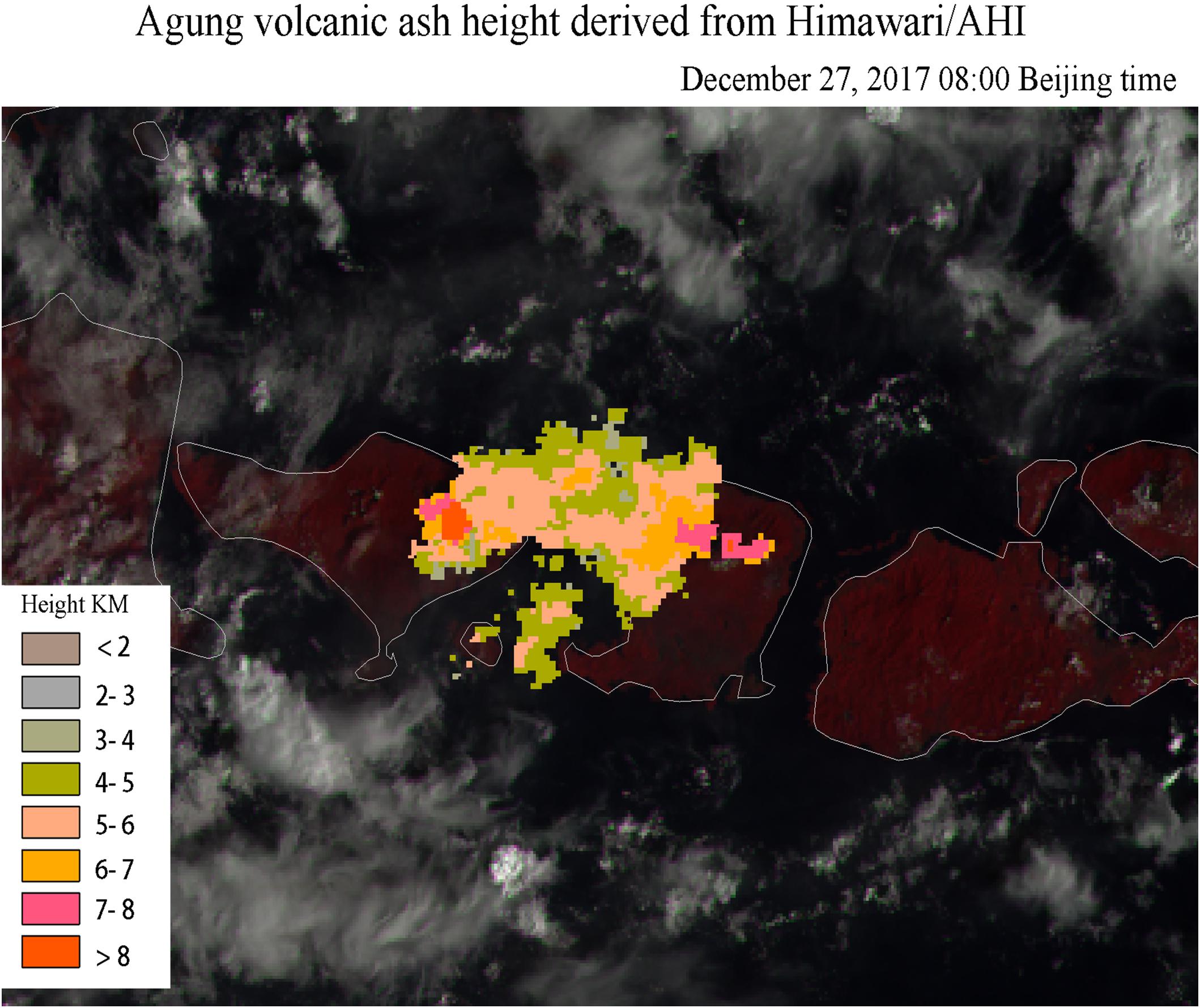
Figure 5. Volcanic ash height derived from Himawari/AHI (00:00 UTC 27 November 2017) (2 km resolution for ash detection and ash height retrieval).
According to weekly reports from both the Global Volcanism Program1 and the Darwin VAAC (Volcanic Ash Advisory Center), ash plumes rose over 6 km above the crater rim on 27 November. The ash height derived from FY-4A/AGRI generally agrees well with these reports.
Fire
For the mid-infrared bands, FY-4A/AGRI uses a linear conversion between Digital Number (DN) and a quantitative physical value (such as radiance), which cannot strike a balance for the detection of both high- and low-temperature objects (Lu et al., 2017). Therefore, two mid-infrared bands were designed for FY-4A/AGRI: channel 7 (3.72 μm, high) and channel 8 (3.72 μm, low). The spatial resolution of channel 7 is 2 km. Its dynamic range mainly covers the high-temperature range (>290 K) so as to enhance the ability to detect fire and strong reflection from the sun. In contrast, the spatial resolution of channel 8 is 4 km and its dynamic range covers low temperatures (Lu et al., 2017). Channel 8 is more suitable for relatively low-temperature detection such as fog, low cloud and fire with a very low temperature (<290 K).
According to Wien’s displacement law, there is an inverse relationship between the peak wavelength of black body emission and its temperature. FY-4A/AGRI’s mid-infrared sensors with a central wavelength at 3.72 μm can respond strongly to thermal emission from the surface at magmatic temperatures, even though those surface areas are very small. The FY-4A/AGRI fire product uses three main criteria to detect fire: (1) when there is fire, the radiation in the mid-infrared channel will increase and (2) the radiation in thermal infrared channels will also increase, but not as much as that in the mid-infrared channel (Dozier, 1981); and (3) the increase between thermal infrared channels will also differ. A weighted mean is therefore calculated for the radiation from the thermal and mid-infrared channels. When there is fire, the increase of the weighted mean for a fire pixel will be more significant than for a no-fire pixel. In this way, fires can be detected from FY-4A/AGRI multi-channel radiative information.
A case study was conducted for 25 September 2017, when an area of anomalously high land-surface temperature was detected east of Inner Mongolia (50.36°N, 129.56°E) at 15:00 Beijing time (07:00 UTC). At 15:30 Beijing time (07:30 UTC), the hot spot (red spot in Figure 7) was approaching the border. Using the sub-pixel area calculation method, it is estimated that the total area of the anomalously high temperature is 1.76 hm2. The FY-4A/AGRI fire detection result was further confirmed by the local fire prevention department as a grass fire at that location. Then the fire developed very quickly. At 16:00 Beijing time (08:00 UTC), the fire density reached its maximum with a total burned area of 2.32 hm2.
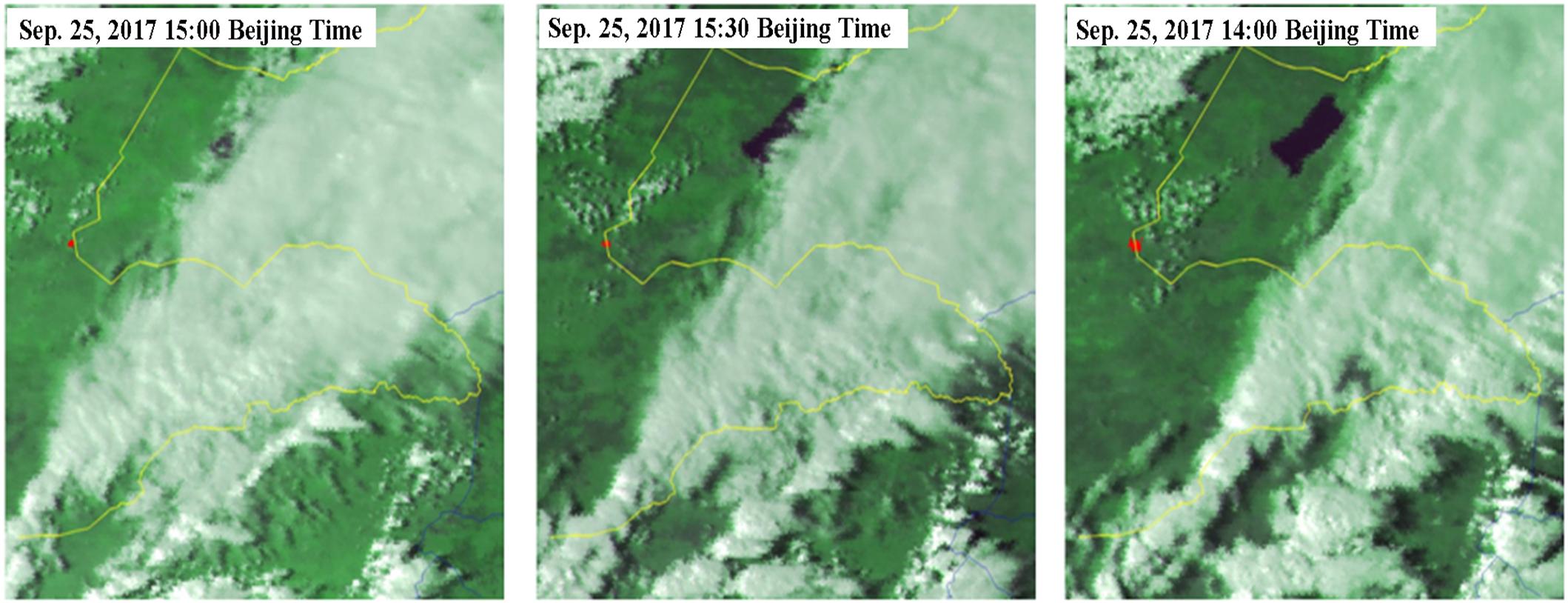
Figure 7. FY-4A/AGRI fire monitoring over Inner Mongolia, China, on 25 September 2017, 15:00–16:00 Beijing Time (2 km resolution).
The performance of AGRI for fire detection was compared with Himawari/AHI using the same fire detection algorithm and at the same time (Figure 8). The fire location derived from FY-4A/AGRI agrees well with that from Himawari/AHI, indicating FY-4A/AGRI’s great potential for automatically detecting the location of fire. However, there is still an obvious difference between the two instruments in the estimate of total burned area (Table 3), possibly caused by different dynamic response ranges between FY-4A/AGRI channel 7 and Himawari/AHI channel 7, and different satellite viewing angles.
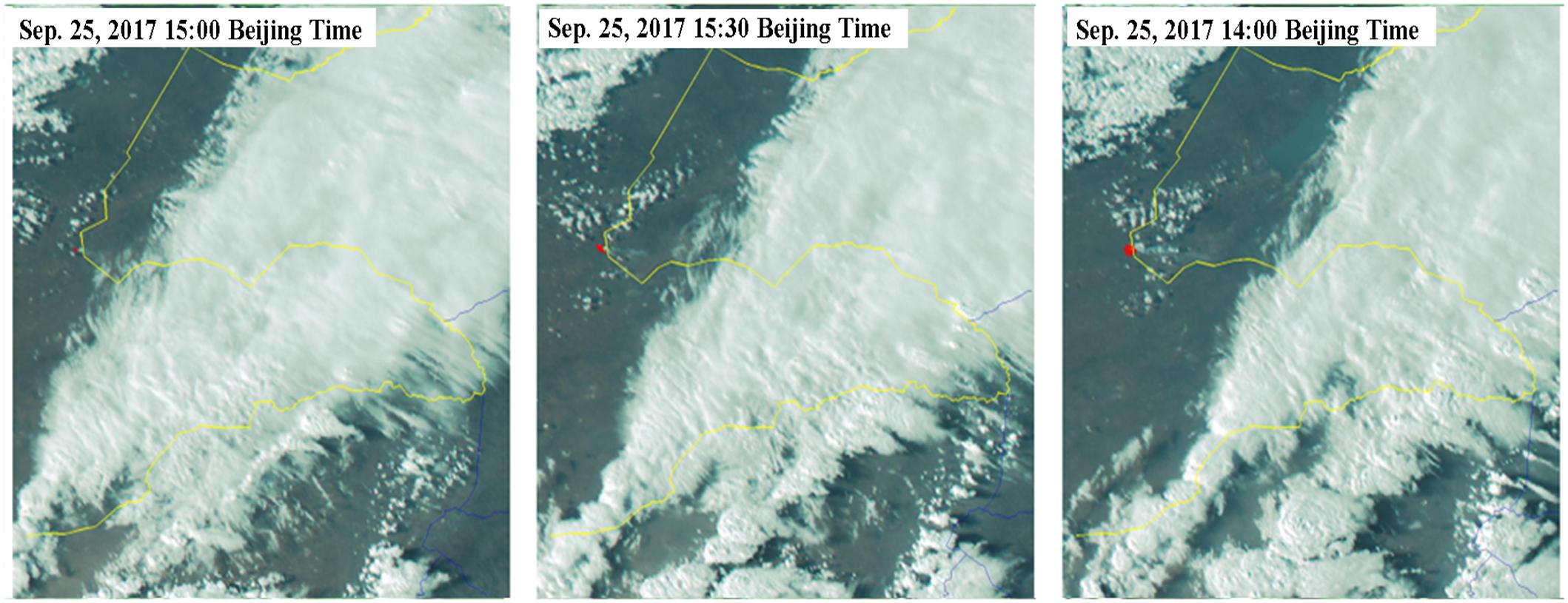
Figure 8. Himawari/AHI fire monitoring over Inner Mongolia, China, on 25 September 2017, 15:00–16:00 Beijing Time (2 km resolution).
Water Body
The reflectivity of water in the visible and near-infrared spectral region is very different from that of vegetation. The reflectivity of water is generally very low (4–5%) and decreases with increasing wavelength. In the red to near-infrared region, especially the latter, water absorbs nearly all the light (Sun et al., 2016). Relatively high reflectivity is mainly concentrated in the blue and green regions. FY-4A/AGRI uses the red and near-infrared bands to extract water information. Under clear sky conditions, an empirical threshold is set to detect a water area. When thin cloud is present, the ratio of the near-infrared to red bands (500 m resolution) is used to detect water (Sheng et al., 1998).
Figure 9 is a case study of the detection of Poyang and Dongting lakes in China on 30 October 2017 using both FY-4A/AGRI data and FY-3B/MERSI data. The spatial distribution of water area from FY-4A/AGRI (500 m resolution) agrees well with that from FY-3B/MERSI (250 m resolution), indicating that FY-4A/AGRI performs well in water detection. The area of Poyang Lake extracted from FY-4A/AGRI is 2626 km2, 3.9% less than that extracted from FY-3B/MERSI (Table 4). The Dongting Lake area is 1167 km2, 10% less than that extracted from FY-3B/MERSI. The mean deviation between the FY-4A/AGRI and FY-3B/MERSI detected water area is 6.9%, due to the different spatial resolutions of the two instruments.
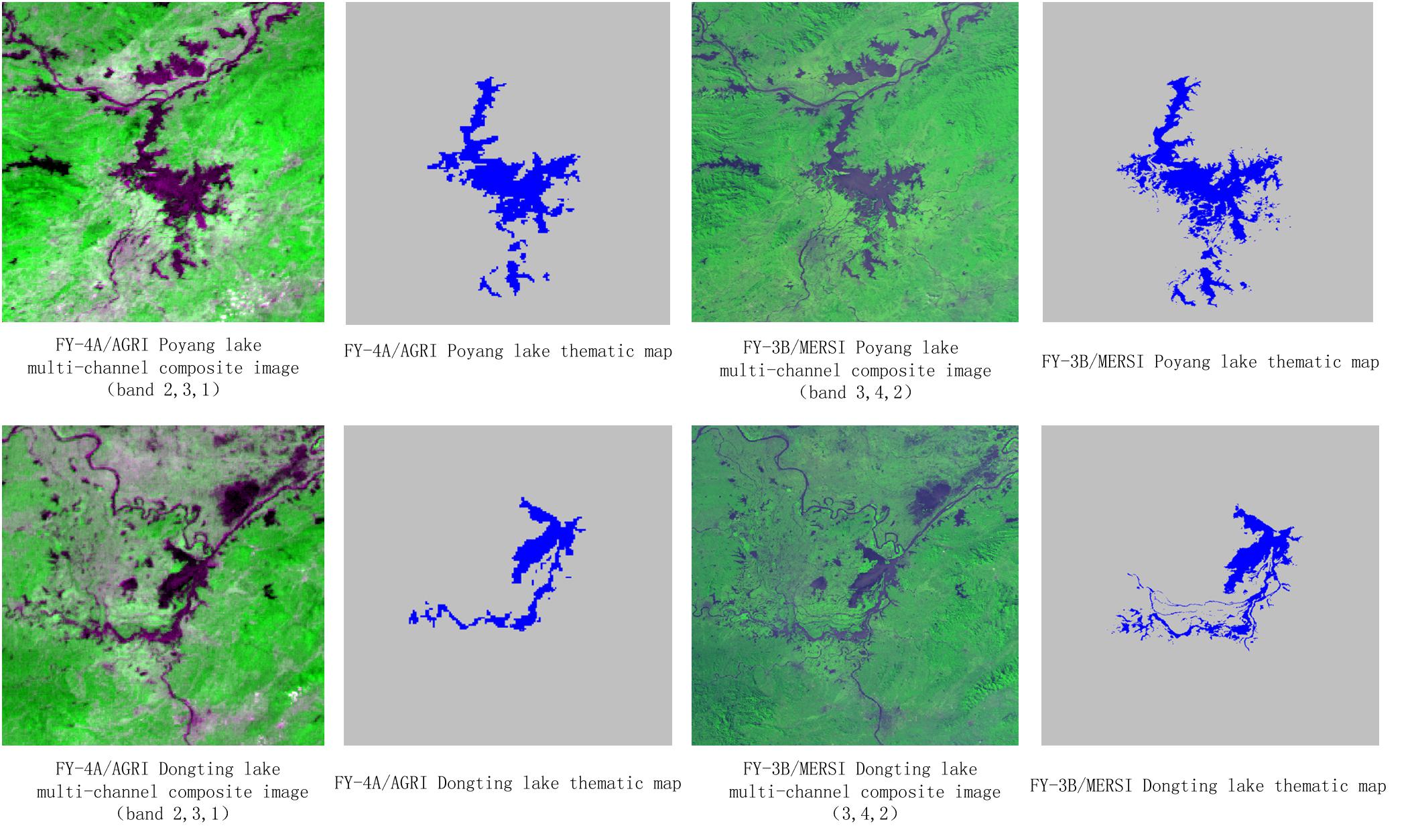
Figure 9. Monitoring of Poyang and Dongting lakes using FY-4A/AGRI and FY-3B/MERSI data, 30 October 2017.
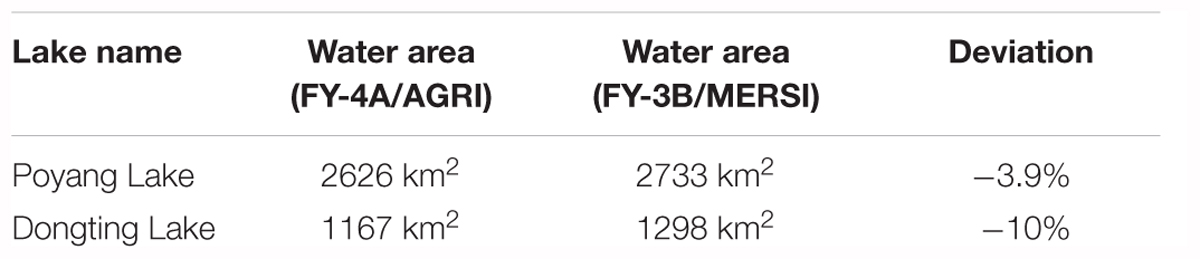
Table 4. Performance comparison between FY-4A/AGRI and FY-3B/MERSI for Dongting Lake and Poyang Lake detection.
Floating Algae
Cyanobacteria (also known as blue-green algae) are a type of primitive photosynthetic aquatic organism. Their toxicity and rapid growth properties, have made them a common ecological problem for shallow freshwater water bodies such as lakes. Cyanobacterial blooms have been reported frequently for Taihu, Caohu, and Dianchi lakes in China (Shi et al., 2017). Under low wind conditions intense cyanobacterial blooms often form floating mats at the water surface that cover large areas and can change rapidly, making it difficult to monitor the dynamic change from LEO satellites alone (Hu, 2009). FY-4A/AGRI, with its greater temporal resolution, offers a new data source for dynamic monitoring of floating algae.
Floating algae have similar spectral reflectance properties to vegetation when they accumulate on the water surface demonstrating high reflectivity in the near-infrared channel and relatively low reflectivity in the visible channels. Therefore, a vegetation index such as the Normalized Difference Vegetation Index (NDVI) can also be used in floating algae detection. The relationships between NDVI and floating algae are determined by fitting for pure water conditions and for a complicated background water condition. A self-adapted model is developed based on the fitted relationships using FY-4A/AGRI data to dynamically detect floating algae.
Figure 10 shows a case study of detecting the whole life cycle of a floating algal bloom on Taihu Lake based on FY-4A/AGRI 1 km spatial resolution and high temporal resolution data. The floating algae appeared at 08:00 (Beijing time) 06 May 2017 and developed very quickly. Four hours later, the density and area of floating algae reached their peak. After that, the area of floating algae gradually reduced and the density also weakened. The relationships between NDVI and floating algae developed from FY-4A/AGRI data were also applied to Himawari/AHI data for a preliminary comparison of the performance of FY-4A/AGRI and Himawari/AHI in floating algae detection. The location and area of floating algae detected by Himawari/AHI agree well with those detected by FY-4A/AHI (Figure 11). Area affected by floating algae estimated from FY-4A/AGRI and from Himawari/AHI display a similar trend (Table 5). Compared with Himawari/AHI, FY-4A/AGRI seems to overestimate the total area affected in the early stage of the floating algal bloom while it underestimates the area affected by floating algae during the weakening stage. At the peak of the floating algal bloom, there is only a very slight difference between Himawari/AHI and FY-4A/AGRI, indicating a good capability of FY-4A/AGRI for detecting floating algal blooms over a large area. Since the FY-4A/AGRI floating algae detection algorithm was directly applied to Himawari/AHI data, the differences in spectral response function and satellite view angle between the two instruments were not taken into account in the floating algae detection algorithm. This was probably the main cause of the final difference in floating algae location detection, area estimation, and density grading between the two instruments.
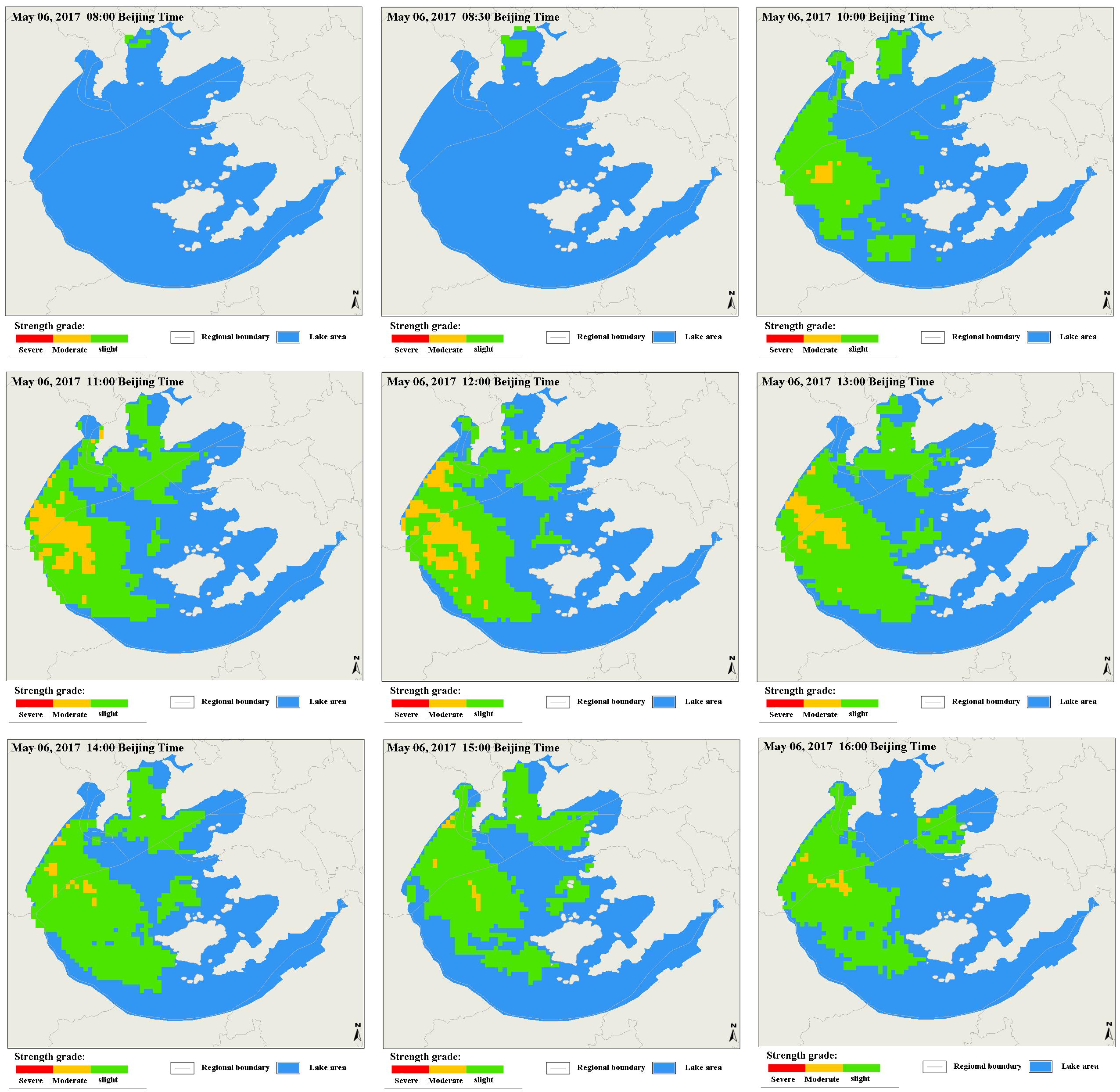
Figure 10. Floating algae detection using FY-4A/AGRI data for Taihu Lake, China, on 6 May 2017 (08:00–16:00 Beijing time).
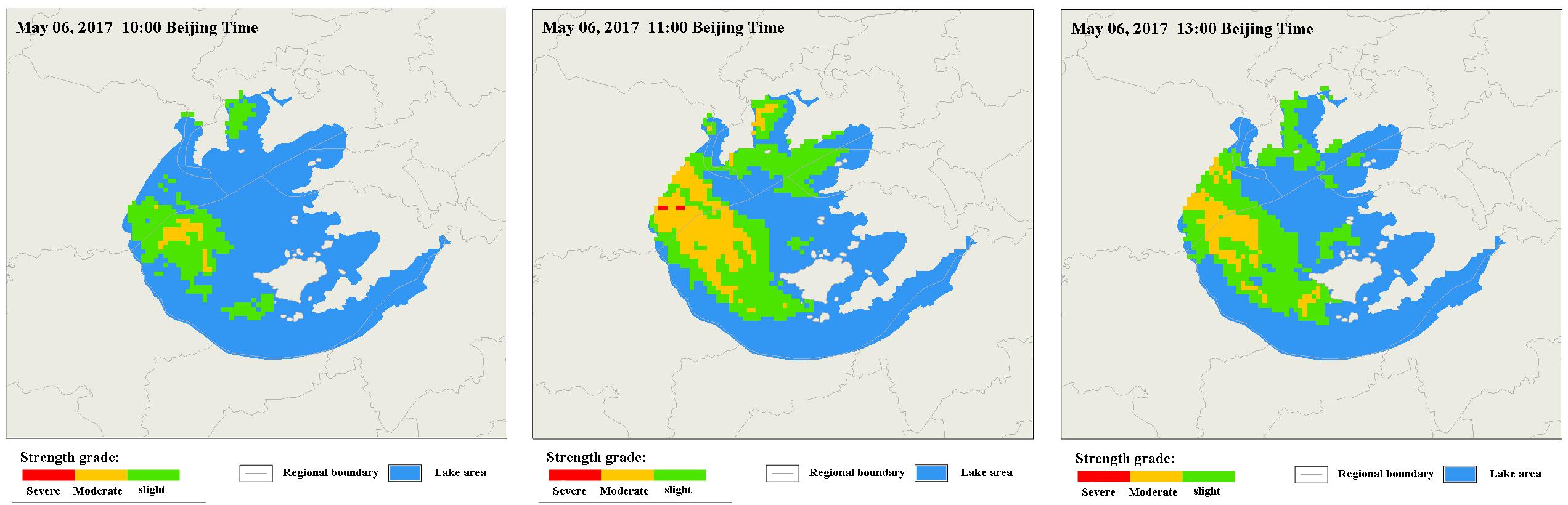
Figure 11. Floating algae detection using Himawari/AHI data for Taihu Lake, China, on 6 May 2017 (10:00–13:00 Beijing time).
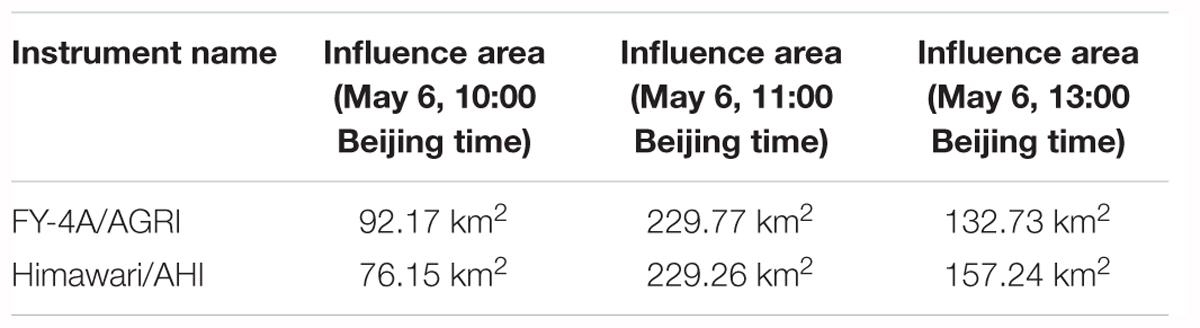
Table 5. Area of Taihu Lake affected by floating algae, as estimated from FY-4A/AGRI and Himawari/AHI data.
Challenges and Further Work
In the present paper, the characteristics of FY-4A/AGRI on board the Chinese new-generation satellites (FY-4A) are introduced and compared with other widely used LEO and GEO instruments. Some non-meteorological applications of FY-4A/AGRI that use these new characteristics are demonstrated, including the detection of aerosol, dust, volcanic ash, fire, water, and floating algae. This study shows the great potential of FY-4A/AGRI for non-meteorological applications. It is shown in section 4 that FY-4A/AGRI has the ability to acquire clearer sky images due to its flexible observation mode, thereby significantly improving the applications of FY-4A/AGRI to the land surface environment, atmospheric quality, and natural disaster. Compared with LEO satellite data, FY-4A/AGRI has higher observation frequency, which is helpful in detecting more rapidly changing targets, such as the occurrence, development, and decay of floating algae, fire monitoring, and the dynamics of dust and volcanic ash. In addition, with the improvement in spatial resolution, especially in the visible channels, the spatial resolution of FY-4A/AGRI is approaching that of current LEO satellites. This will greatly enhance the accuracy of detailed target monitoring and change detection (e.g., dynamic detection of water area and floating algae area).
Although the performance of FY-4A/AGRI is approaching that of similar American and Japanese instruments, some aspects of FY-4A/AGRI performance still fall behind. For example, the spatial resolution of the thermal channels of FY-4A/AGRI is 4 km whereas those of GOES-R/ABI and Himawari 8-9/AHI both reach up to 2 km, which will influence the detection of very detailed targets, such as volcanic ash detection. For retrieving volcanic ash or dust parameters, the leading algorithms still largely rely on different properties in different thermal channels. If the spatial resolution improves from 4 to 2 km, the mixed pixel effect will be further reduced and thus improve the accuracy of the algorithm. The relatively low spatial resolution of FY-4A/AGRI compared with Himawari/AHI and GOES-16/ABI will pose a new challenge for the combined use of FY-4A/AGRI with other GEO sensors.
This study was based on a small number of case studies, and limited field measurement data were used for product evaluation. Operational applications of FY-4A/AGRI to non-meteorological areas will require substantial validation over different areas and times. Furthermore, although the comparisons between FY-4A/AGRI and other sensors (e.g., Himawari/AHI, FY-3B) show comparable accuracy between FY-4A/AGRI derived parameters and those from other sensors, there are still considerable differences in the final results. Differences in positioning accuracy, spectral response function, and satellite view angle may all reduce the final agreement between products derived from FY-4A/AGRI and other sensors. We will investigate these considerations in future work. It is especially important to carry out cross-calibration between FY-4A/AGRI and GEO/LEO sensors, so as to make the algorithms more robust for different sensors. Only in this way can data from different sensors be used together for global monitoring of the atmosphere and the land surface.
Fortunately, the spatial resolution of AGRI will be significantly improved in the subsequent satellites of the FY-4 series. For FY-4B and FY-4C, the spatial resolution of AGRI will gradually improve from 4 to 2 km for the thermal channels, which will further improve the capability for fine target detection and reduce errors caused by mixed pixel effects in key parameter retrieval. Furthermore, the observation frequency of FY-4C will improve from every 15 min to every 5 min and the relative positioning accuracy and calibration accuracy will be enhanced overall. FY-4B and FY-4C are expected to be launched in 2018 and 2020, respectively. The FY-4 series will completely replace the FY-2 series step by step and offer high-quality observations and support operational work for disaster prevention and mitigation, climate change, and environmental change monitoring over the Asia–Pacific area and along the route of the Chinese “one belt and one road” plan. This will further enhance the international influence of China’s meteorological and aerospace industry.
Author Contributions
PZ and ST arranged the whole manuscript and also performed the comparisons of FY4A/AGRI with other LEO and GEO satellites. LZ carried out the volcanic ash detection and retrieving section and wrote the manuscript. LG developed the FY-4A/AGRI aerosol algorithm and carried out the related case study. LC developed dust detection algorithm and carried out the case study. WZ and JS carried out the land surface temperature retrieving case study and water monitoring study. JC carried out the fire detection study. XH carried out floating algae monitoring case study and land surface temperature study.
Funding
This work was funded by National Key Research and Development Program of China (2018YFA0605502) and National Natural Science Foundation of China (41871263).
Conflict of Interest Statement
The authors declare that the research was conducted in the absence of any commercial or financial relationships that could be construed as a potential conflict of interest.
Acknowledgments
The authors would like to thank the two reviewers and Dr. Thomas Schroeder for their valuable comments and suggestions, which helped the authors significantly improve the manuscript. The authors also would like to thank Weicheng Geng of the National Satellite Meteorological Center, China Meteorological Administration, who helped preprocess related remote sensing data.
Footnotes
References
Ardanuy, P. E., Gail, W., Hooke, W., Macauley, M., Marley, S., Puschell, J., et al. (2015). “Optimizing requirements for the next generation of satellite observing systems,” in Proceedings for the 2015 EUMETSAT Meteorological Satellite Conference, Toulouse.
Bessho, K., Date, K., Hayashi, M., Ikeda, A., Imai, T., Inoue, H., et al. (2016). An introduction to himawari-8/9—Japan’s new-generation geostationary meteorological satellites. J. Meteorol. Soc. Japan 94, 151–183. doi: 10.2151/jmsj.2016-009
Cao, C., Xiong, J., Blonski, S., Liu, Q., Uprety, S., Shao, X., et al. (2013). Suomi NPP VIIRS sensor data record verification, validation, and long-term performance monitoring. J. Geophys. Res. 118, 11664–11678. doi: 10.1002/2013JD020418
Chen, L., Jing, Y., Zhang, P., and Hu, X. (2016). “Analysis of aerosol properties derived from sun photometer and Lidar over Dunhuang radiometric calibration site,” in Proceedings of the SPIE 9876, Remote Sensing of the Atmosphere, Clouds, and Precipitation VI, 98763G, eds R. Kumar and S. Yang (Bellingham: SPIE).
Chen, L., Zhang, P., Lv, J., Xu, N., and Hu, X. (2017). Radiometric calibration evaluation for RSBs of suomi-NPP/VIIRS and aqua/MODIS based on the 2015 dunhuang chinese radiometric calibration site in situ measurements. Int. J. Remote Sens. 38, 5640–5656. doi: 10.1080/01431161.2017.1343514
Dozier, J. (1981). A method for satellite identification of surface temperature fields of sub-pixel resolution. Remote Sens. Environ. 11, 221–229. doi: 10.1016/0034-4257(81)90021-3
Hu, C. (2009). A novel ocean color index to detect floating algae in the global oceans. Remote Sens. Environ. 113, 2118–2129. doi: 10.1016/j.rse.2009.05.012
IPCC (2007). Climate Change 2007: The Physical Science Basis. Contribution of Working Group I to the Fourth Assessment Report of the Intergovernmental Panel on Climate Change. Cambridge: Cambridge University Press.
Kahn, R., Banerjee, P., McDonald, D., and Diner, D. J. (1998). Sensitivity of multiangle imaging to aerosol optical depth and to pure-particle size distribution and composition over ocean. J. Geophys. Res. 103, 32195–32213. doi: 10.1029/98JD01752
Levy, R. C., Remer, L. A., Kleidman, R. G., Mattoo, S., Ichoku, C., Kahn, R., et al. (2010). Global evaluation of the collection 5 MODIS dark-target aerosol products over land. Atmos. Chem. Phys. 10, 10399–10420. doi: 10.5194/acp-10-10399-2010
Lu, F., Zhang, X., Chen, B., Liu, H., Wu, R., and Han, Q. (2017). FY-4 geostationary meteorological satellite imaging characteristics and its application prospects. J. Mar. Meteorol. 37, 1–12.
Remer, L. A., Kaufman, Y., Tanré, D., Mattoo, S., Chu, D., Martins, J., et al. (2005). The MODIS aerosol algorithm, products, and validation. J. Atmos. Sci. 62, 947–973. doi: 10.1175/JAS3385.1
Schmit, T. J., Gunshor, M. M., Menzel, W. P., Gurka, J. J., Li, J., and Bachmeier, A. S. (2005). Introducing the next-generation advanced baseline imager on GOES-R. Bull. Am. Meteorol. Soc. 86, 1079–1096. doi: 10.1175/BAMS-86-8-1079
Sheng, Y. W., Su, Y. F., and Xiao, Q. G. (1998). Challenging the cloud-contamination problem in flood monitoring with NOAA/AVHRR imagery. Photogramm. Eng. Rem. Sens. 64, 191–198.
Shi, K., Zhang, Y., Zhou, Y., Liu, X., Zhu, G., Qin, B., et al. (2017). Long-term MODIS observations of cyanobacterial dynamics in lake taihu: responses to nutrient enrichment and meteorological factors. Sci. Rep. 7:40326. doi: 10.1038/srep40326
Sun, D., Li, S., Zheng, W., Croitoru, A., Stefanidis, A., and Goldberg, M. (2016). Mapping floods due to hurricane sandy using NPP VIIRS and ATMS data and geotagged flickr imagery. Int. J. Digit. Earth 9, 427–441. doi: 10.1080/17538947.2015.1040474
Yang, J., Zhang, Z., Wei, C., Feng, L. U., and Guo, Q. (2017). Introducing the new generation of chinese geostationary weather satellites – fengyun 4. Bull. Am. Meteorol. Soc. 98, 1637–1658. doi: 10.1175/BAMS-D-16-0065.1
Zhang, P., Lu, N. M., Hu, X. Q., and Dong, C. H. (2006). Identification and physical retrieval of dust storm using three MODIS thermal IR channels. Glob. Planet. Change 52, 197–206. doi: 10.1016/j.gloplacha.2006.02.014
Zhu, L., Li, J., Zhao, Y., Gong, H., and Li, W. (2017). Retrieval of volcanic ash height from satellite-based infrared measurements. J. Geophys. Res. Atmos. 122, 5364–5379. doi: 10.1002/2016JD026263
Keywords: FY-4A/AGRI, aerosol monitoring, dust monitoring, volcanic ash monitoring, fire monitoring, water body monitoring, floating algae monitoring
Citation: Zhang P, Zhu L, Tang S, Gao L, Chen L, Zheng W, Han X, Chen J and Shao J (2019) General Comparison of FY-4A/AGRI With Other GEO/LEO Instruments and Its Potential and Challenges in Non-meteorological Applications. Front. Earth Sci. 6:224. doi: 10.3389/feart.2018.00224
Received: 31 March 2018; Accepted: 21 November 2018;
Published: 11 January 2019.
Edited by:
Thomas Schroeder, CSIRO Oceans and Atmosphere (O&A), AustraliaReviewed by:
Bijoy Vengasseril Thampi, Science Systems and Applications, United StatesPaulina Wong, Lingnan University, China
Copyright © 2019 Zhang, Zhu, Tang, Gao, Chen, Zheng, Han, Chen and Shao. This is an open-access article distributed under the terms of the Creative Commons Attribution License (CC BY). The use, distribution or reproduction in other forums is permitted, provided the original author(s) and the copyright owner(s) are credited and that the original publication in this journal is cited, in accordance with accepted academic practice. No use, distribution or reproduction is permitted which does not comply with these terms.
*Correspondence: Lin Zhu, emh1bGluQGNtYS5nb3YuY24=