Downward Counterfactual Search for Extreme Events
- Risk Management Solutions, London, United Kingdom
An event catalog is a foundation of the risk analysis for any natural hazard. Especially if the catalog is comparatively brief relative to the return periods of possible events, it may well be deficient in extreme events that are of special importance to risk stakeholders. It is common practice for quantitative risk analysts to construct ensembles of future scenarios that include extreme events that are not in the event catalog. But past poor experience for many hazards shows that these ensembles are still liable to be missing crucial unknown events. An explicit systematic procedure is proposed here for searching for these key missing events. This procedure starts with the historical catalog events, and explores alternative realizations of them where things turned for the worse. These are termed downward counterfactuals. By repeatedly exploring ways in which the event loss might have been incrementally worse, missing events can be discovered that may take risk analysts, and risk stakeholders, by surprise. The downward counterfactual search for extreme events is illustrated with examples drawn from a variety of natural hazards. Attention is drawn to the problem of overfitting to the historical record, and the value of stochastic modeling of the past.
Definition of Downward Counterfactual
Counterfactual thinking considers other ways in which events might have evolved than they actually did. There is a large literature on counterfactual thinking, spanning many different disciplines, including history (e.g., Roberts, 2004; Ferguson, 2011; Evans, 2014; Gallagher, 2018); literature (e.g., Roth, 2004; Leiber, 2010); and the philosophy of causation (Pearl, 2000; Collins et al., 2004; Pearl and Mackenzie, 2018). This is a risk analyst’s contribution to counterfactual thinking about extreme events.
A downward counterfactual is a thought about the past where the outcome was worse than what actually happened (Roese, 1997). This contrasts with an upward counterfactual where the outcome was better. This terminology is commonly used in cognitive psychology (e.g., Gambetti et al., 2017), and is invoked by historians (Prendergast, 2019), but has only recently been introduced into risk analysis (Woo, 2016). This is reflected in the previous lack of usage of the apt downward counterfactual terminology in the literature on extreme risks (Woo et al., 2017). But whenever a major hazard event occurs, risk stakeholders should enquire not just about what happened, but also about the downward counterfactuals. Such enquiry is discouraged by outcome bias, where special weight is attached to the actual outcome (Kahneman, 2011), and the additional effort involved. But if risk stakeholders made this effort, their reward would be less surprise from extreme events. The exposition of downward counterfactuals in the above 2017 Lloyd’s report (Woo et al., 2017) for the insurance industry has since encouraged the use of this terminology in infrastructure risk assessment (Oughton et al., 2019).
The State Space of Extreme Events
Risk stakeholders around the world are tasked with the risk management of extreme events. Governments, civic authorities, corporations and insurers all need to identify, assess, and control extreme losses. However, even the first step of identifying potential extreme losses can be very challenging. This is often addressed in an ad hoc manner, or through some form of statistical extreme value analysis, but the rarity of extreme events relative to the length of the historical record tends to undermine quantitative efforts at robust estimation of potential extreme losses.
For a given peril, the loss potential in a specific region can be expressed generically as a multivariate function L(X1,X2,..XN) of the underlying dynamic variables {Xk(t)} which include human behavioral factors as well as physical and environmental hazard and vulnerability factors. A challenge for a quantitative risk analyst is that the state space of extreme events is obscure and not clearly delineated, and data are sparse. Apart from the significant known historical events, it is uncertain which postulated extreme scenarios are actually possible within the state space. Furthermore, the loss function, including both direct and indirect losses, is often poorly defined and inadequately understood, and not expressible in a succinct compact form conducive to mathematical analysis to facilitate the discovery of maximum loss values.
Quantitative risk analysis advanced enormously in the final quarter of the twentieth century, yet too often in the twenty-first century, extreme events have occurred that were not on the risk horizon, and completely missing from risk analysis, either deterministic or probabilistic. Inevitably, their occurrence was a cause of universal surprise, professional disappointment, and public consternation about the deficiencies of risk analysis. A passive response to criticism about wrong models is for risk analysts to invoke the “unknown unknowns.”
The word “catastrophe” means a down-turning in the original Greek. Down-turnings can take many different forms, and are not easy to find serendipitously or anticipate. In order to avoid surprise at future catastrophes, a systematic active procedure needs to be developed for searching for these down-turnings, rather than accept these surprises as and when they occur. Such a procedure is constructed here to look for these down-turnings, which might not be discovered otherwise.
A notorious and tragic example of a surprising event is the March 11, 2011, magnitude 9.0 Tohoku, Japan, earthquake, which resulted in the deaths of about 20,000 people. No earthquake hazard model for the region countenanced such a giant earthquake. This was largely due to the high confidence placed in the maximum magnitude of 8.2 prescribed by Prof. Hiroo Kanomori, the doyen of Japanese seismology, who made fundamental contributions to understanding the physics of earthquakes. His geophysical theory that the weakness of older tectonic plates is not conducive to the generation of great earthquakes (Kanamori, 1986) was highly credible. However, there was circumstantial historical information suggesting that magnitude 8.2 might already have been exceeded. A regional earthquake occurred in 869 which left expansive tsunami deposits covering as many as three prefectures in northeast Japan.
From an academic perspective, the substantial uncertainty over the size of the 869 Jogan earthquake obviated the need for scrutiny of the 8.2 maximum magnitude figure. However, from a practical seismic hazard perspective, sufficient evidence existed to allow for the 869 earthquake exceeding 8.2 in magnitude (Sugawara et al., 2012). Indeed, at a meeting of the Japanese Society of Engineering Geology in 2007, it was conjectured that this historical earthquake might even have been around magnitude 9 (Muir-Wood, 2016).
Back in 2007, probabilistic seismic hazard analysis was under development in Japan. But it would have been possible for seismic hazard analysts to construct a logic-tree for different values of regional maximum magnitude, with one branch associated with a magnitude 9 assignment. Just the possibility alone of this magnitude estimate would have been grounds for major risk stakeholders, such as nuclear utilities, to consider reviewing their safety and preparedness protocols. Such a review might have instigated further historical studies of the 869 earthquake. The latest tsunami deposit research (Namegaya and Satake, 2014) indicates that the 869 Jogan earthquake had a magnitude of at least 8.6, and it might have been close to 9.0.
Stochastic Simulation of Hazard Systems
Even if the magnitude of the 869 Jogan earthquake actually had been somewhat smaller than 9.0, given the stochastic process of earthquake generation, it is possible that this rupture might have been greater, and the magnitude might have attained 9.0. Random aleatory variability is an intrinsic characteristic of all Earth hazard systems. Shortly before an earthquake fault rupture begins, there is large uncertainty over the final extent of the rupture. This uncertainty in rupture dynamics is a fundamental constraint on earthquake predictability and early warning (Minson et al., 2019).
Most hazard systems have non-linear dynamic characteristics, which make them very sensitive to initial conditions. So if the dynamics of a hazard system were replicated a large number of times, there would be a diverse array of outcomes, some of which might correspond to dangerous system states. Examples of dangerous outcomes include a tropical cyclone making landfall with hurricane intensity; earthquake rupture propagation jumping from one fault to another and releasing much greater energy; a volcanic eruption tapping additional mush zones and increasing in magnitude.
The intrinsic dynamical complexity of geohazards results in history being only one of a number of ways events may have unfolded. The possibility of reimagining history is routinely considered by meteorologists who conduct historical reanalysis of past weather events all around the world. One global 3-D atmospheric reanalysis dataset reaching back to 1851 provides ensembles of 56 members based on the assimilation of surface and sea-level pressure observations (Brönnimann, 2017).
Reimagining history can provide valuable practical insight into hazard events worse than any actually observed to date. The risk forecasting value of conducting stochastic simulations of the past has been demonstrated explicitly by Thompson et al. (2017) in the important societal context of flooding in southeast England. In the winter of 2013–2014, a succession of storms led to record rainfall and flooding there, with no historical precedent. A large ensemble climate simulation analysis was conducted for the period from 1981 to 2015, using the Hadley Centre global climate model. This showed that this extreme flooding event could have been anticipated. Indeed, in southeast England, there is a 7% chance of exceeding the current rainfall record in at least 1 month in any given winter. This answers the enquiry from the UK Department of the Environment as to whether the severe flooding of 2013–2014 might have been worse.
Downward Counterfactual Search
The downward counterfactual search process involves explicit identification of ways in the loss outcome from a historical event might have been progressively worse. Even for the community of catastrophe risk modelers, who are professional experts in extremes, it is very uncommon for a systematic search of downward counterfactuals to be undertaken for historical events. Catastrophe risk modeling emerged as a discipline in the late 1980s with the arrival of affordable desktop computing (Woo, 1999). At that time, desktop computers would only accommodate a basic hazard model, one parsimonious in the number of extreme scenarios. In subsequent decades, computer power has escalated, and catastrophe risk models have correspondingly grown in size and sophistication. Yet, there has never been a systematic methodology for capturing extreme events in a catastrophe risk model. Economy of effort, and aversion to superfluous work and unnecessary cost, restrict the amount of research into extreme scenarios beyond the historical catalog.
The tape of history is only run once, so there is only one actual event catalog. However, history is only one realization of what might have happened, and a stochastic simulation of the past would generate alternative events, some of which might be larger. Such a simulation provides a means of exploring the state space of possible events for potential surprises. Extreme events can be tracked in an exploratory manner through the kind of systematic numerical crawl algorithms familiar from computer science and operations research.
A methodical algorithm for searching for extreme events within the state space is to begin with those specific events known to lie within the state space, i.e., notable historical events, and consider a sequence of exploratory stochastic variations which lead to progressively larger loss values. Pathways from historical events are therefore constructed that result ultimately in extreme events in a disaster domain, that may as yet be unknown (Figure 1).
As perceptively noted by Roth (2004), what is unexpected in its own time is typically chronicled as if the past were somehow inevitable. Catastrophe modelers tend to chronicle the historical record in this way. But this ignores the common observation that even slight nudges can make major change happen (Sunstein, 2019). A catalog of extreme events can be highly sensitive to these nudges.
For risk analysts, event catalogs are routinely treated as a fixed valuable data source, albeit recognizing uncertainty in the observation and measurement of events. And even if the effort and resources required to treat catalogs otherwise were allocated for risk analysis, there is an inherent human psychological bias favoring consideration of upward counterfactuals, where things turned for the better, over downward counterfactuals, where things turned for the worse. When disaster strikes, thoughts naturally turn positively to how the loss situation might have been ameliorated rather than deteriorated. A new conceptual paradigm is needed for thinking about downward counterfactuals.
The exploratory search for extreme events can be reframed as a simple counterfactual thought experiment. Starting with a notable historical event, a perturbation is considered where the loss is increased by X%. This might be of the order of 10%. Other perturbations are then considered with higher loss increments, until no plausible pathways of further loss increase can be reasonably conjectured, and the downward counterfactuals are exhausted.
Amongst a group of risk analysts, this search exercise might be conducted sequentially around a table, rather as in a Victorian parlor game. All such games were imitations of real life situations (Beaver, 1997), reflecting the struggle to live in the world of reality. Each player in turn is called to suggest a perturbation causing an incremental additional loss. Anyone unable to come up with such a suggestion is passed over in favor of the next player who can. This could also be a virtual lateral thinking exercise for an individual risk analyst. However, as with the elicitation of expert judgment, the ability to think creatively about plausible disaster scenarios may vary considerably from one risk analyst to another (Cooke, 1991). Hence a group exercise is favored.
Such a group exercise was carried out for the first time at a workshop convened at the Earth Observatory of Singapore (EOS), August 26–27, 2019, sponsored by the Singapore National Research Foundation. The workshop theme was the use of counterfactual analysis to track so-called Black Swans: rare events which have no historical precedent. The Black Swan metaphor originated in the philosophical discussion of the principle of induction; no amount of observations of white swans can disprove the existence of a Black Swan.
The workshop venue was particularly appropriate, because EOS was established in the aftermath of the catastrophic 2004 Indian Ocean tsunami, which was so devastating for Singapore’s Asian neighbors. This will be shown later to be a prime example of a counterfactual Black Swan, a surprising event that might have been anticipated through counterfactual analysis. Twenty eight participants from six countries, and a variety of risk backgrounds, attended the EOS workshop. This paper is based on a presentation by the author at this workshop.
One major event that was explored counterfactually was the devastating April 16, 2016, M7.8 Muisne earthquake which struck along the central coast of Ecuador (Franco et al., 2017). The leader of the EEFIT Earthquake Engineering Field Investigation Team, Guillermo Franco, also led the counterfactual exploration of this event. The author was also involved in the round-table discussion of this earthquake.
The two key downward counterfactuals pertained to the earthquake rescue and evacuation process. First, there was an earthquake engineering discussion about the seismic integrity of bridges, which were critical elements of the transport infrastructure. The second downward counterfactual involved a crucial post-event government decision: the military was called upon for assistance. This was quite controversial for political reasons; Ecuador has been ruled by a military junta in the past. In many countries, including democracies, the presence of 10,000 members of the armed forces on city streets would be an absolute red line. Furthermore, there is a view within the disasters community that emergency response operations should remain in the hands of civil authorities. However, had the military not been called upon, with all its logistical resources such as air ambulances, the rescue and evacuation process would have been far less efficient, and a major aftershock could have resulted in more citizen casualties.
Any natural disaster has myriad alternative ways of unfolding. The outcome depends not just on the hazard event itself and the vulnerability of the built environment, but also on the human response, particularly decisions made by civil protection authorities. The downward counterfactual search process can help identify decision pathways that might have aggravated the disaster, and help develop lessons for promoting future resilience. A downward counterfactual can consider both the addition and subtraction of salient factors. In this case, the subtraction of the military assistance would have been a turn for the worse, despite apprehension over the political wisdom of invoking this intervention.
One of the advantages of counterfactual analysis is the capability to address fundamental issues of causation, when statistical methods based on correlation are inadequate (Pearl and Mackenzie, 2018): correlation is not causation. This type of analysis is central to attribution analysis for climate change, where the enhanced risk of adverse weather due to climate change is assessed. A review of the Ecuador government response to the 2016 Muisne earthquake disaster allows the inference to be drawn that, but for the military intervention, the death toll would have been higher. This is important to understand for future crisis management.
The 9/11 Black Swan
The political dimension to the earthquake crisis in Ecuador in 2016 leads to consideration of the epitome of a most surprising act of political violence: the Al Qaeda terrorist attack of September 11, 2001. This is a classic Black Swan: just because an event has never happened before does not preclude it from happening in the future. According to Taleb (2007), Black Swan logic makes what you don’t know more relevant than what you do know; many Black Swans can be caused and exacerbated by their being unexpected. He cites 9/11: “Had the risk been reasonably conceivable on September 10, it would not have happened.”
This is a man-made rather than natural disaster, but is specifically included here to illustrate the universal applicability of the downward counterfactual search procedure for all extreme events. Risk analysis methodologies are all the more powerful for covering the full range of both natural and man-made perils (Woo et al., 2017). Furthermore, all natural disasters have a human dimension. Indeed, according to the UN Office for Natural Disaster Reduction, natural disasters do not exist, but natural hazards do, and the term natural disaster should be replaced by disaster from natural hazard events.
Of all the questions that were asked in the aftermath of 9/11, one of the most insightful and challenging for risk analysis is also one of the most simple and curious: Why didn’t this happen before? It might well have done. This most unforeseeable of events might actually have been arrived at via the following four step downward counterfactual search process.
(a) Less than 2 years before 9/11, on October 31, 1999, an EgyptAir pilot, Gameel al Batouti, flying out of JFK airport, New York, to Cairo, took deliberate actions that resulted in flight 990 crashing into the Atlantic, killing everyone on board (NTSB, 2002).
(b) The loss outcome would have been worse if the plane had crashed near the Atlantic coast, also killing some local fishermen and sailors.
(c) The loss would have been worse still, if the plane had crashed soon after take-off in the Queens borough of New York City, and killed people on the ground. Such an event actually occurred on November 12, 2001, several months after 9/11, when flight AA587 crashed through rudder failure.
(d) A further downward counterfactual would have had the pilot decide to turn back to New York City and fly the plane into an iconic Manhattan skyscraper. There was an international precedent for such suicidal action: on Christmas Eve 1994, Algerian terrorists planned to bring down a hijacked Air France plane over the Eiffel Tower.
Contrary to Taleb’s assertion (2007), not only was 9/11 conceivable – it was actually conceived by the Al Qaeda leader himself on Halloween 1999 through counterfactual thinking. According to his aide-de-camp, Osama bin Laden had this counterfactual thought when informed of the October 31, 1999 EgyptAir crash (Soufan, 2017).
Indeed, many so-called Black Swan events can be found from a downward counterfactual search for extreme events. Most catastrophes have either happened before; nearly happened before; or might have happened before. Each notable historical event generates its own accompanying set of downward counterfactuals. The larger the number of notable historical events in the recorded past, the more extensive is the combined coverage of extreme events spanned by the space of downward counterfactuals.
The 2004 Indian Ocean Tsunami Black Swan
The other most notable example of an eponymous Black Swan in the first decade of the twenty-first century was the great Indian Ocean earthquake and tsunami of December 26, 2004, which killed 230,000 people. This example was explicitly cited by Taleb along with 9/11 in the prolog to his book, The Black Swan: “Had it been expected, an early warning system would have been put in place.” This quote comes from a section on “what you do not know.” Following the M9.5 Chile earthquake and tsunami of May 22, 1960, and the M9.2 Alaska earthquake and tsunami of March 22, 1964, a tsunami warning center for the Pacific was established in 1965. The Intergovernmental Oceanographic Commission did also recommend the setting up of an Indian Ocean tsunami warning system, but all too often it takes a major event to convert a hazard mitigation suggestion to an actual implementation. As commented above, near-misses, such as moderate Indian Ocean earthquakes, are discounted through outcome bias.
It is a tough and demanding challenge for a Black Swan tracking procedure to be capable of finding extreme events as disparate as the terrorist attack on 9/11 and the 2004 Indian Ocean tsunami. The power and utility of the downward counterfactual search procedure over other approaches is that it can actually do this. The millennium-eve year 1999 was especially noteworthy for catastrophe risk analysts scanning the risk horizon for extreme events. This was the year of the EgyptAir crash on Halloween, and also the year of publication of a seminal scientific paper (Zachariasen et al., 1999) on a great historical earthquake in Sumatra, Indonesia, on November 25, 1833. Whereas the evidence from observations of ground shaking and tsunami extent suggested a lesser magnitude of 8.7 or 8.8, further evidence emerged from the growth of coral microatolls. These are massive solitary coral heads that record sea level fluctuations, such as from earthquake displacements, and which can be precisely dated. This new palaeoseismic evidence suggested a larger magnitude of 8.8 up to 9.2, which is the magnitude of the great 2004 Indian Ocean earthquake, although the fault rupture length was shorter.
The 1833 earthquake ruptured a southern section of the Sumatra subduction zone (Figure 2) about 1000 km southeast of the rupture area of the 2004 earthquake. Whilst the event was destructive in Sumatra, it was fortunate that most of the energy of the 1833 tsunami was dissipated comparatively harmlessly in the open Indian Ocean (Cummins and Leonard, 2005), well south of India and Sri Lanka. Because of this good fortune, this great earthquake is little known internationally, even to seismologists. It was a seismological near-miss, rather than a massive earthquake disaster. But if the tsunami source had been further north, southeast India and Sri Lanka would have been very much in harm’s way.
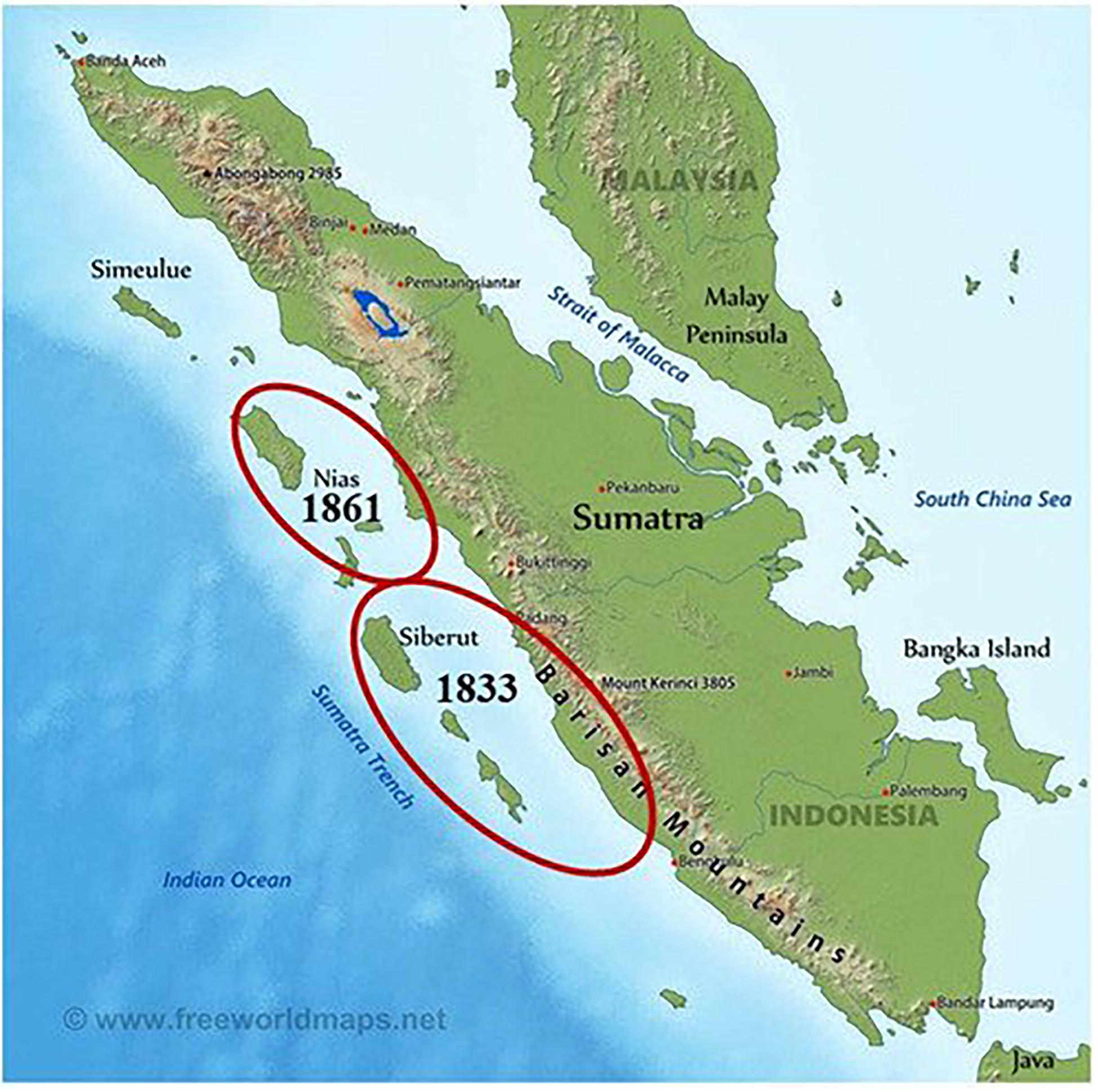
Figure 2. Map of Sumatra showing the earlier 1833 and 1861 earthquake ruptures. Aceh, the site of prehistoric tsunami deposits, is at the northernmost tip of Sumatra.
A downward counterfactual thought experiment undertaken in 1999 would have considered stochastic variations of the 1833 earthquake that would have generated ever larger incremental losses, leading ultimately to the great tsunami disaster, less than 6 years later. Subsequent palaeoseismic research into the stratigraphic sequence of prehistoric tsunami deposits from a coastal cave in Aceh, at the northernmost tip of Sumatra (Figure 2), has revealed at least 11 prehistoric tsunamis that struck the Aceh coast between 7,400 and 2,900 years ago. The average time period between tsunamis is about 450 years with intervals ranging from over 2,000 years to multiple tsunamis within the span of a century (Rubin et al., 2017).
A downward counterfactual search process undertaken in 1999 could have progressed in the following five stages:
(a) Whatever the actual magnitude of the 1833 earthquake, the fault rupture might well have been large enough for a magnitude assignment of 9.2.
(b) As evidenced by the rupture geometry of the 1861 earthquake (Figure 2), which had magnitude 8.5, the 1833 earthquake might potentially have ruptured further to the northwest of the Sumatra subduction zone.
(c) Extending the rupture geometry 1000 km to the northwest, the 1833 earthquake might potentially have occurred where the 2004 event did. Indeed, in the US Geological Survey seismic hazard model for the region, published just 3 months before the 2004 earthquake (Petersen et al., 2004), the Sumatra subduction zone is modeled as a single seismic source with a maximum magnitude of 9.2.
(d) A tsunami spreading out from this more menacing geographical location would have a highly destructive impact in southeast India, Sri Lanka as well as Thailand.
(e) In the absence of any tsunami warning system in the Indian Ocean, the regional death toll would have been catastrophic.
This counterfactual thought experiment might have been undertaken as a desk exercise in 1999, upon the publication of the paper by Zachariasen et al. on the 1833 Sumatra earthquake. Far from being a mere academic exercise, it could have made a highly significant and crucial practical contribution to risk mitigation in the Indian Ocean.
The September 2004 seismic hazard paper by Petersen et al. was focused on ground motion hazard with no consideration of tsunami hazard. So even though the December 26, 2004 magnitude 9.2 earthquake rupture was anticipated as a scenario in this probabilistic seismic hazard model, the serious tsunami risk implications were not addressed. This was not through any oversight of the authors, but due to the traditional narrow scope of seismic hazard analysis, which addresses ground shaking but does not routinely include secondary perils like tsunamis or landslides.
Downward counterfactual thinking was required to appreciate the catastrophic tsunami hazard potential, and to recognize the 1833 tsunami as a near-miss. However, adequate attention is rarely accorded to near-misses in the geohazards and georisks community. If there were more attention, the vocabulary of downward counterfactuals would be in professional risk parlance. Regrettably, catalogs of near-misses are not routinely compiled, unlike catalogs of actual events. This reflects the finding of psychologists (Roese, 1997) that nine out of ten counterfactual thoughts are upward. Human beings are inherently optimistic. But optimism has never been a prudent basis for disaster risk management.
After 1999, there was scientific hazard knowledge of the potential for a devastating tsunami to travel across the Indian Ocean and strike the southeast coast of India and Sri Lanka. Even without commitment of any financial resources on an Indian Ocean tsunami warning system, the 2 h travel time from Sumatra to southern India would have allowed for a basic rudimentary risk-informed tsunami warning system to have been improvised (Woo, 2017).
Such a system might have operated as follows. Once it was confirmed by seismologists that a great earthquake had occurred in the Sumatra subduction zone, a pre-compiled library of tsunami paths would be scanned to identify the areas across the Indian Ocean at risk, and warning messages would be disseminated to principal risk stakeholders in southeast India and Sri Lanka. Among these stakeholders was the Madras atomic power plant at Kalpakkam, near Chennai, which had a regional public evacuation plan for a release of radioactivity (Vijayan et al., 2013).
Almost 6 years of time were available for scientific knowledge of the 1833 earthquake and tsunami to be disseminated before December 26, 2004. Such knowledge could have reduced the 50,000 toll of lives lost a thousand miles away in southeast India and Sri Lanka. Even though tsunami hazard in the Indian Ocean was not within the geographical domain of responsibility of the Pacific Tsunami Warning Centre in Hawaii which recorded the great 2004 earthquake, real-time decision-making there potentially might have been influenced by the 1833 downward counterfactual. There was of course a lack of sufficient instrumentation for tsunami detection in the Indian Ocean, but also there was a lack of counterfactual thinking. Training in reimagining history can save lives.
The Gorkha, Nepal, Earthquake of April 25, 2015
About one-third of the victims of the 2004 Indian Ocean tsunami were children. In the Seychelles, where there were only a few fatalities, children were lucky that schools were closed over the weekend, when the first tsunami wave arrived on a high tide. For similar fortuitous reasons, another tragic death toll of children from a geological hazard was averted a decade later in North Asia.
At 11.56 a.m. local time on Saturday April 25, 2015, a large earthquake M7.8 struck central Nepal, with a hypocenter located in the Gorkha region about 80 km north–west of Kathmandu. Approximately three quarters of a million buildings were damaged, and about 9,000 people were killed. The earthquake rupture propagated from west to east and from deep to shallow parts of the shallowly dipping fault plane, and consequently, strong shaking was experienced in Kathmandu and the surrounding municipalities (Goda et al., 2015). But counterfactually, the loss would have been greater had the rupture extended further toward Kathmandu. The location of the Gorkha earthquake meant that the most proximate and worst-hit districts were primarily rural with low population densities. Indeed, this was not the expected great Kathmandu Valley earthquake. The Gorkha earthquake should thus be considered a near-miss (Petal et al., 2017). The ground accelerations were only about 1/3 of the design code accelerations.
This is a spatial counterfactual, which is an alternative event realization with a different geometry. Severe as this is, it is eclipsed by a temporal counterfactual, which is an alternative event realization with a different occurrence time. The lethality rate associated with the Gorkha earthquake was rather modest: 0.019 per heavily damaged or collapsed building (Petal et al., 2017). However, a large part of the rural population was outdoors at noon on a Saturday, so that the building occupancy rate was quite low at the time. In rural areas, this time of day generally has the lowest occupancy rate of under 20% (Coburn and Spence, 1992). But had the earthquake struck at night, with most people being indoors, the casualty rate might have been about five times higher.
A downward counterfactual search for a worse temporal counterfactual posits the earthquake occurring during a week day, rather than on a Saturday. With this timing, children would have been indoors in poorly constructed schools. According to the Ministry of Education, the majority of schools were more than 50 years old and built of mud and mortar. The casualty count amongst school children would then have been catastrophic. Out of 8242 public schools damaged during the earthquake, 25134 classrooms were fully damaged, 22097 classrooms were partially damaged and 15990 classrooms were slightly damaged (Neupane et al., 2018). Furthermore, 956 classrooms in private schools were fully damaged, and 3983 were partly damaged.
Taking a student-teacher ratio of 20:1 or more (NIRT, 2016), the occupancy of the 26,000 wrecked classrooms might potentially have reached the level of half a million children. Five thousand school buildings were completely destroyed. Had these buildings been occupied, even with just a handful of deaths per destroyed school building, or just one per wrecked classroom, the death toll might well have exceeded 25,000.
In 1998–1999, the National Society for Earthquake Technology-Nepal (NSET) evaluated the risk to schools in Kathmandu Valley, as part of KVERMP: The Kathmandu Valley Earthquake Risk Management Project (Dixit et al., 2000). Notwithstanding the high risk of earthquakes, school construction in Nepal had largely ignored issues of structural safety, and they were built very informally. NSET warned that in a severe earthquake 66% of the schools in Kathmandu Valley would be likely to collapse, another 11% would partially collapse and the rest would be damaged. If an earthquake happened during school hours, NSET predicted that 29,000 students, teachers and staff would be killed and 43,000 seriously injured. Such an alarming death toll might well have been realized if the Gorkha earthquake had not occurred on a Saturday.
Irrespective of the final death count, the grim toll of casualties in so many classrooms would make this the worst ever counterfactual Black Swan for education. The shooting of children in a single U.S. school classroom can traumatize a whole nation; this would be multiplied by a factor of 26,000. Indeed, allowing for the international trauma associated with so many child casualties at school, this might have been one of the most appalling earthquake disasters. The catastrophe could have blighted long-term school attendance in Nepal and other developing countries: high risk exposure is an unacceptable price to pay for education (World Bank, 2017).
Earthquakes are not generated deterministically: the Gorkha earthquake could have occurred randomly at any hour and day of the week. Thankfully, it occurred on the optimal day of the week, and at a time when most people were outdoors. Saturday is the only day when schools and offices are universally closed. Counterfactually, the chance of the Gorkha earthquake occurring when the children were at class was as high as about 20%.
Disaster catalogs traditionally list events in a quasi-deterministic manner. The uncertainty in data measurement is included, but not the inherent stochastic variability in the underlying data. Thus a catalog of earthquake casualties might include an estimate of the uncertainty in the reporting of casualties, but not recognize the significant aleatory uncertainty in the counts. In particular, disaster catalogs do not record near-misses, so it is not known if there has ever been a closer earthquake near-miss for mass school mortality, anywhere in the world, than the Gorkha earthquake.
Downward Counterfactual Factors
In searching for downward counterfactuals, a temporal counterfactual is an obvious place to start. An event time shift is just one mechanism for worsening the loss outcome beyond what happened historically. A degree of randomness in the time of occurrence of events is an intrinsic feature of a dynamic chaotic world. As evident from the Gorkha earthquake, even a modest change in event time can leverage a massive impact on the loss outcome. The coincidence of a coastal storm surge or tsunami with a high tide can greatly amplify the level of coastal flooding. Prolonged stalling of a meteorological depression system can result in sustained rainfall and exacerbate the severity of river flooding. More generally, anomalous precipitation levels can be produced from the coincidence of several atmospheric dynamic movements (Wang et al., 2019).
A basic principle of physics holds that if a system is invariant under a shift in time, then its energy is conserved. Accordingly, just as a time shift is an important downward counterfactual factor for system change, so also is a perturbation in system energy, which is the basic fuel of catastrophe. An energy counterfactual is an alternative event realization with a different energy release. The rate of energy release is a stochastic risk factor for many natural hazard systems. Loss potential can be exacerbated by the accelerated release of energy over a short time period. For example, an increment in seismic energy release can raise the hazard severity level above an engineering design basis, and trigger structural damage. Meteorologically, the warming of sea surface temperature, such as associated with El Niňo every few years, can lead to extreme weather.
A seismological example of an energy counterfactual is a runaway earthquake which releases seismic energy along a major fault in a single great event, rather than in several smaller events at different times. The Kocaeli, northern Turkey, earthquake of August 17, 1999 killed 17,000, but the death toll might have tripled if the rupture had run away westwards past Istanbul (Woo and Mignan, 2018).
Volcanic eruptions may occur episodically with a moderate amount of explosive energy. Counterfactually, the energy release may be concentrated in a single much larger, and potentially far more dangerous, explosive eruption. Such a significant downward counterfactual was mooted by the noted volcanologist Frank Perret in the context of 2 years of volcanic unrest in Montserrat in the 1930s, and has been studied by Aspinall and Woo (2019) for the 1997 volcanic crisis on Montserrat.
With time change being a significant downward counterfactual factor, so also is spatial change. The spatial coincidence of intense hazard with high exposure is a common recipe for catastrophe. It is just as well that hazard footprints are often misaligned with urban exposure maps. However, a modest geographical shift in a severe hazard footprint, such as associated with an intense tornado, may increase the loss enormously.
The loss sensitivity to a shift in storm geography is graphically best visualized in hurricane tracks that narrowly miss striking a major city. This happened with Hurricane Matthew in early October 2016, which skirted Florida, but had tracked toward Palm Beach at Category 4. The following year on September 7, 2017, Hurricane Irma was headed directly for Miami at Category 5, but veered westwards and weakened to make landfall on the west coast of Florida. At the end of August 2019, Hurricane Dorian threatened to strike Florida at Category 4, but veered north to skirt the coast. The final track of Hurricane Dorian belies the high dimensionality of alternative tortuous Atlantic tracks which Dorian might have followed.
All natural hazard crises involve human decision-making to mitigate casualties and financial loss. Human intervention is always a potential downward counterfactual factor: misjudgments and errors can turn a crisis into a disaster. A natural hazard event may be a necessary but not sufficient cause of a disaster. In 2011, the rainfall in the Chao Phraya river basin in central Thailand was similar to that in 1995. In contrast with 1995, extensive flooding in 2011 arose because of a failure to release enough water from upstream dams to accommodate the monsoon. A downward counterfactual search in 1995 would have identified this kind of misjudgment as a potential source of extreme flood loss. Past crises provide a valuable training laboratory to explore the realm of downward counterfactuals. For decision-makers, they offer the opportunity of re-living alternative realizations and evolutions of past crises, including identifying possible sources of error, so gaining important practical experience in dealing with future chaotic challenging situations. Lessons learned have international application: mismanagement of the reservoir dams was a contributory cause to flooding in Kerala, India, in August 2018.
Given a large number of possible parameterizations of individual downward counterfactual factors (temporal, energy, spatial, human etc.), the number of potential combinations increases exponentially into the many thousands. This combinatorial complexity is a measure of the opportunity and scale of a downward counterfactual search to discover surprising unknown events. For practical purposes, interest is focused on a fairly compact subset of the most plausible alternative realizations, which correspond to near-misses. There is no catalog of near-misses, so these can easily be overlooked. Take the 1833 Sumatra earthquake, for example. This was a near-miss disaster because it was large enough for the tsunami to have wrought havoc in Sri Lanka, if the epicenter had been further northwest along the Sumatra subduction zone. However, if the 1833 Sumatra had been only magnitude 7, it would have not have impacted Sri Lanka, even with this displaced epicenter.
Hindsight and Foresight
Taleb (2007) summarizes Black Swan attributes in the following triplet: rarity, extreme impact and retrospective (though not prospective) predictability. According to Kahneman (2011), when an unpredicted event occurs, we immediately adjust our view of the world to accommodate the surprise. This is hindsight bias. Kahneman adds that the illusion that we understand the past fosters overconfidence in our ability to predict the future. Using the rise of Google as an example, he notes that luck can play a more important role in an actual event than in the telling of it.
Given the intrinsic randomness in the way that events evolve, the past cannot be adequately understood without considering what else nearly happened, or might have happened. Kahneman notes that the unfolding of the Google story is thrilling because of the constant risk of disaster. It is not the function of a risk analyst to predict disasters, but rather to assess the risk of disaster occurrence. Whatever the type of risk, this risk assessment cannot be based solely on what happened, but should explicitly recognize the stochastic nature of history. This recognition is manifest in a downward counterfactual search for extreme events.
When an extreme event happens which does have a known historical precedent, it should not come as a surprise, and the event should have been duly represented within a risk assessment. Hindsight is defined as perception after an event, and is the opposite of foresight. The historical occurrence of an extreme event provides us with clear foresight as to its potential recurrence in the future – no hindsight is involved.
Over time, an event catalog builds up to include many if not most events that might happen. However, rare events may still be absent from a historical catalog. But given the stochastic nature of event generation, some of these rare events might potentially have happened. Provided a downward counterfactual search is undertaken for significant historical events, the space of events that almost happened, which might be counted as near-misses, can be charted. This downward counterfactual search thus is capable of providing additional foresight into the future, provided that diligent effort is made to recognize and identify near-misses. If then one of these near-misses were actually to materialize in the future, it would not be so surprising.
To illustrate this, consider again the December 26, 2004, Indian Ocean tsunami. A downward counterfactual search of extreme tsunami events in the Indian Ocean would have identified the 1833 Sumatra earthquake as providing foresight into the December 26, 2004, event. The foresight offered by a downward counterfactual search is inherently limited by the extent of the known historical record. Had there been no knowledge of the 1833 event, such foresight into the December 26, 2004 would not have been possible.
Each historical event serves as a radar detector on the risk horizon. The power and effectiveness of such a radar detector increases with the size and significance of the historical event. Also, the longer and larger the historical catalog, the more extensive will be the overall coverage of the risk horizon. Of all the innumerable alternative realizations of a historical event, only those that are physically plausible are useful for scanning the risk horizon. The plausible alternative realizations of the k’th historical event constitute a set R(k).
The union of these historical event sets R(k) spans a subset of all risk scenarios, and provides valuable foresight into future extreme events that might be missing from existing stochastic event sets of catastrophe risk models used for quantitative risk assessment. Of these plausible alternative realizations, the downward counterfactual search process aims to identify the most plausible scenarios, especially those that might be counted as near-misses, or otherwise quite likely. All catastrophe risk models are constrained in size by computer hardware and budget limitations and run-time tolerance, as well as a degree of human aversion to perceived unnecessary labor.
There is no formalism or criteria for checking the completeness of the underlying stochastic event sets. The downward counterfactual search process is capable of identifying supplementary scenarios that should be represented in these stochastic event sets. As demonstrated with site-specific seismic hazard studies for critical industrial installations, as many scenarios for an entire region might be narrowly focused on a high-resolution study for just a single site, such as a nuclear installation. As shown by the damage to the Fukushima nuclear plant, and subsequent release of radioactivity following the March 11, 2011, Tohoku earthquake and tsunami, even for a site-specific hazard study, a surprising event may occur that is not represented within a stochastic event set.
It has taken decades of intensive historical research for reliable event catalogs to be compiled for natural hazards occurring around the world. But there are no comparable catalogs of near-misses. In Leiber’s (2010) counterfactual short story, reference is made to a masterwork named either “If things had gone wrong” or “If things had turned for the worse.” Regrettably for risk analysts and risk stakeholders, no such book exists. Material for compiling such a masterwork would require the patient accumulation of information from numerous downward counterfactual searches.
To illustrate the future foresight that can be gained from the downward counterfactual search process, some salient examples are given for China, which is heavily exposed to both earthquake and flood risk. Northern China is an intraplate tectonic region of far greater complexity for seismic hazard analysis than plate boundaries. The July 28, 1976, Tangshan earthquake, which killed a quarter of a million people, occurred on a previously unknown fault. Even assignment of maximum magnitude to specific active faults is a challenge; the Tangshan earthquake had a magnitude of 7.8. For the Hetao Rift, situated west of Beijing, earthquakes from magnitude 6 to 7 have occurred since 1900, but from slip-rate analysis, there is an unreleased seismic moment equivalent to a M7.9 event (Huang et al., 2018). A standard Gutenberg-Richter magnitude-frequency analysis for the Hetao Rift based on the observed local evidence of past earthquake occurrence might underestimate the maximum magnitude. An energy counterfactual perspective would recognize that one or more of the twentieth century earthquakes on the Hetao Rift might have been considerably larger, and that the maximum magnitude on the Hetao Rift might approach M7.9.
Another Chinese hazard example of future foresight from counterfactual analysis is winter flooding on the Yellow River. In December 2003, ice blocks appeared in the upper reaches of the Yellow River. Sustained rainfall since August had resulted in Autumn rains persisting into December. In the middle reaches, the volume of water flows reached 1300 cu.m./s, which is about five times the typical December volume. A major water conservation and irrigation facility, the Xiaolangdi reservoir, stored 8.2 billion cu.m. of water, and could only accommodate one billion cu.m. for the potential flood caused by the ice. But this was only half what was estimated might have been needed; fortunately, there was no flood disaster. Counterfactually, this could be counted as a near-miss. Should there be a future Yellow River flood induced by ice blockage, this would not come as a surprise. Indeed, flood risk mitigation measures have since been instigated. One draconian measure taken in 2014 was the aerial bombing of river ice to reduce blockage. This was a pragmatic exercise in counterfactual thinking; unless the ice blockage was removed, there was a high risk of flooding. Other than ice blockage, landslide blockage of Chinese rivers also provides practical examples of the foresight achievable through counterfactual analysis.
A salient U.S. example of future flood foresight comes from another of the world’s great rivers, the Mississippi. On July 13, 2019, Hurricane Barry made landfall in Louisiana whilst the Mississippi River was in flood stage. This was the first time this had happened for a landfalling tropical storm. Fortunately, Barry turned westward and the rainfall was much lower than forecast, so river levels never reached the levels which might have overtopped the levees and flooded New Orleans. However, this near-miss provides future warning of the dire consequences of a compound event coupling river flood and an early season landfalling hurricane. Climate change makes such an event increasingly likely. Indeed, future foresight into climate-induced compound events would be significantly advanced through this type of counterfactual analysis. If New Orleans were to be flooded in future through the combination of a late flood stage of the Mississippi River and an early Gulf hurricane, it would now not be surprising.
The Pitfalls of Over-Fitting Limited Historical Data
In the field of artificial intelligence, there is a well-known overfit effect, where a mathematical model is so good at matching the data that it is poor at predicting what data might come next. The human brain has evolved to avoid the overfit effect through the transience of memory (Gravitz, 2019). Memories can be viewed as models of the past. They are simplified representations that capture the essence, but not necessarily the detail, of past events. Transience of memory prevents overfitting to specific past events, thereby promoting generalization, which is optimal for decision-making (Richards and Frankland, 2017).
The manner in which the past is recollected is of practical safety importance in hazardous situations. Imagine the life-threatening experience of being confronted by a dangerous wild animal. Remembering every detail of such a harrowing experience is detrimental to preventing being attacked in the future. A detailed memory may make it harder to generalize for a future dangerous encounter (Gravitz, 2019). The size and color of animal, the exact location and environment of a past encounter may be remembered in detail. However, the next encounter may be with a wild animal of a different size and color, in another location and environment. If swans were aggressive, such as when protecting their young, there would be safety benefit in not presuming that all swans are necessarily of similar appearance, e.g., white. Cognitive dissonance might otherwise set in when confronted with a swan of an unexpected and surprising color.
To learn most, and make the best decisions from past hazardous experience, one needs to be able to think laterally beyond relying on a photographic memory of the past. Such reliance evokes an idealized deterministic world of detailed but artificial inevitability. The overfit effect is a challenge for risk analysts dealing with rare events. There is a natural tendency for sparse historical catalogs of severe hazard events to be overfitted. To overcome the overfit effect, there needs to be some reimagination of the past, capturing the essence but not all the fine detail of past severe events. Downward counterfactual thought experiments, as implemented in the stochastic modeling of the past, provide a systematic practical methodology for avoiding the overfitting effect. Artificial intelligence has yet to advance to encompass counterfactual thinking (Pearl and Mackenzie, 2018), so it is not coincidental that counterfactual thinking can be used to tackle the overfitting problem.
Regarding Indian Ocean tsunami risk, the 1833 Sumatra subduction zone tsunami was a highly salient event. Fortunately, the propagation path of the large tsunami was south of India and Sri Lanka, and it had a negligible impact there (Dominey-Howes et al., 2007). But this aspect of this large historical tsunami, i.e., the lack of significant impact in India and Sri Lanka, was imprinted in the tsunami catalog, and may have disincentivized disaster preparedness through human outcome bias. The finite attention span of civil protection authorities is concentrated on the exact catalog.
The possibility of a similar large rupture occurring further north on the Sumatra subduction zone was recognized before 2004, and was indeed explicitly incorporated within a probabilistic seismic hazard model (Petersen et al., 2004) published online before the December 26, 2004 tsunami. If the historical spread of the 1833 tsunami had been reimagined to be further north from where it was recollected as being, this would have provided an alarming downward counterfactual risk lesson for Indian Ocean disaster preparedness.
Taleb has cited human nature as making us concoct explanations after the fact, making a Black Swan explainable and predictable. This may be true if the historical record is very poor. But downward counterfactual exploration of historical events can increase disaster preparedness before the fact, after a near-miss has occurred. Learning from the example of the 1833 Sumatra tsunami, a downward counterfactual search for extreme events could be undertaken for numerous historical major earthquakes and tsunamis. Potentially significant cliff-edge phenomena may be discovered in this systematic search process.
As an example, had the M6.6 February 9, 1971, San Fernando, California, earthquake been rather more intense, or the aftershocks more severe, the badly damaged Lower San Fernando dam might have collapsed, which would have had disastrous consequences for the population downstream, killing more people than have died from earthquakes in the whole of recorded California history. This downward counterfactual is a forceful argument for prioritizing enhanced state dam seismic safety. Similarly, downward counterfactuals of historical wildfires in California might have encouraged upgrading the electricity distribution system to reduce the risk of wildfire ignition during windstorms.
Conclusion
Whenever a major hazard event occurs, reconnaissance missions report on what happened, and analyze the hazard, vulnerability and loss outcomes in considerable detail. However, with extensive resources committed to elucidating the events themselves, the systematic focused exploration of increasingly worse outcomes from historical events has rarely been undertaken, and constitutes a substantial innovative research agenda for the future. This is a very broad expansive agenda; as much effort and resources could be directed toward a downward counterfactual search following an event, as to investigating the primary event itself. Specifically, the counterfactual possibility of different modes of infrastructure and supply chain disruption (bridge or power plant failure, dam collapse etc.) can be investigated for individual past events.
Retrospective counterfactual risk analysis is a logical supplement to prospective catastrophe risk analysis. Much more information can be extracted from a finite historical record. This type of extended historical analysis provides a valuable independent additional tool for regulatory stress testing, sense-making of the results of catastrophe risk models, and helping all stakeholders to form their own view of risk. This tool is applicable to any type of risk: a generic universal problem requires a generic universal solution.
In keeping with the fractal geometry of nature, the number of hazard model scenarios generated for a given region can be generated for a region half the size, and so on recursively. The enormous complexity of possible evolutions of any historical scenario is the underlying rationale for a downward counterfactual search for extreme events. Because so little of this complexity jungle has ever been charted or explored, there is a clear opportunity for significant discovery. Out of all the sheer complexity may emerge potential alternative event evolutions that may be surprising and unexpected, and correspondingly insightful for disaster awareness and preparedness. Such insight would be foresight into the future, and not hindsight about the past.
Data Availability Statement
All datasets generated for this study are included in the article/supplementary material.
Author Contributions
GW was the sole contributor and accountable for all the content.
Funding
Singapore travel was funded by the Singapore National Research Foundation.
Conflict of Interest
GW was employed by the company RMS.
Acknowledgments
GW is grateful for collaborative support from colleagues at RMS and NTU, Singapore, and discussions with Willy Aspinall and Kathy Cashman.
References
Aspinall, W. P., and Woo, G. (2019). Counterfactual analysis of runaway volcanic explosions. Front. Earth Sci. 7:222. doi: 10.3389/feart.2019.00222
Brönnimann, S. (2017). “Weather extremes in an ensemble of historical reanalyses,” in Historical Weather Extremes in Reanalyses, ed. S. Brönnimann, (Switzerland: Geographica Bernensia), 7–22. doi: 10.4480/GB2017.G92.01
Cummins, P., and Leonard, M. (2005). The Boxing Day 2004 Tsunami – a Repeat of the 1833 Tsunami? Karachi: AusGeo News, 77.
Dixit, A. M., Dwelley-Samant, L. R., Nakarmi, M., Pradhanang, S. B., and Tucker, B. E. (2000). “The Kathmandu Valley earthquake risk management project: an evaluation,” in Proceedings of the 12 World. Conference Earthquake, Auckland, 788.
Dominey-Howes, D., Cummins, P., and Burbidge, D. (2007). Historic records of teletsunami in the Indian Ocean and insights from numerical modelling. Nat. Hazards 42, 1–17. doi: 10.1007/s11069-006-9042-9
Franco, G., Stone, H., Ahmed, B., Chian, S. C., Hughes, F., Jirouskova, N., et al. (2017). “The April 16, 2016, Mw 7.8 Muisne earthquake in Ecuador – preliminary observations from the EEFIT reconnaissance mission of May 24 – June 7,” in Proceedings of the 16 World Conference Earthquake, Santiago.
Gambetti, E., Nori, R., Marinello, F., Zucchelli, M. M., and Giusberti, F. (2017). Decisions about a crime: downward and upward counterfactuals. J. Cogn. Psychol. 29, 352–363. doi: 10.1080/20445911.2016.1278378
Goda, K., Kiyota, T., Pokhrei, R. M., Chiaro, G., Katagiri, T., Sharma, K., et al. (2015). The 2015 Gorkha Nepal earthquake: insights from earthquake damage survey. Front. Built Environ. 1:8. doi: 10.3389/fbuil.2015.00008
Huang, Y., Wang, Q., Hao, M., and Zhou, S. (2018). Fault slip rates and seismic moment deficits on major faults in Ordos constrained by GPS observation. Nat. Sci. Rep. 8:16092. doi: 10.1038/s41598-018-345
Kanamori, H. (1986). Rupture process of subduction-zone earthquakes. Ann. Rev. Earth Planet. Sci. 14, 293–322. doi: 10.1146/annurev.earth.14.1.293
Leiber, F. (2010). “Catch that zeppelin!,” in Alternate Histories, eds I. Watson, and I. Whates, (London: Robinson).
Minson, S. E., Baltay, A. S., Cochran, E. S., Hanks, T. C., Page, M. T., McBride, S. K., et al. (2019). The limits of earthquake early warning accuracy and best alerting strategy. Sci. Rep. 9:2478. doi: 10.1038/s41598-019-39384-y
Namegaya, Y., and Satake, K. (2014). Reexamination of the A.D. 869 Jogan earthquake size from tsunami deposit distribution, simulated flow depth, and velocity. Geophys. Res. Lett. 41, 2297–2303. doi: 10.1002/2013/GL058678
Neupane, C. C., Anwar, N., and Adhikari, S. (2018). “Performance of school buildings in Gorkha earthquake of 2015,” in Proceedings of the 7th Asia Conference on Earthquake, Bangkok, 22–25.
NTSB, (2002). Aircraft Accident Brief: EgyptAir Flight 990 Boeing 767-366ER, SU-GAP 60 miles South of Nantucket, October 31, 1999. National Transportation Safety Board Incident Report: NTSB/AAB-02/01 PB2002-B910401. National Transportation Safety Board, Washington, DC.
Oughton, E. J., Ralph, D., Pant, R., Leverett, E., Copic, J., Thacker, S., et al. (2019). Stochastic counterfactual risk analysis for the vulnerability assessment of cyber physical attacks on electricity distribution infrastructure networks. Risk Anal. 39, 2012–2031. doi: 10.1111/risa.13291
Petal, M., Barat, S., Giri, S., Rajbanshi, S., Gajurel, S., Green, R. P., et al. (2017). Causes of Deaths and Injuries in the 2015 Gorkha (Nepal) Earthquake. Kathmandu: Save the Children.
Petersen, M. D., Dewey, J., Hartzell, S., Mueller, C., Harmsen, S., Frankel, A. D., et al. (2004). Probabilistic seismic hazard analysis for Sumatra, Indonesia and across the Southern Malaysian Peninsula. Tectonophysics 390, 141–158. doi: 10.1016/j.tecto.2004.03.026
Richards, B. A., and Frankland, P. W. (2017). The persistence and transience of memory. Neuron 94, 1071–1084. doi: 10.1016/j.neuron.2017.04.037
Rubin, C. M., Horton, B. P., Sieh, K., Pilarczyk, J. E., Daly, P., Ismail, M., et al. (2017). Highly variable occurrence of tsunamis in the 7,400 years before the 2004 Indian Ocean tsunami. Nat. Commun. 8:16019.
Soufan, A. (2017). Anatomy of Terror: From the Death of Bin Laden to the Rise of Islamic State. London: W.W. Norton.
Sugawara, D., Goto, K., Imamura, F., Matsumoto, H., and Minoura, K. (2012). Assessing the magnitude of the 869 Jogan tsunami using sedimentary deposits: prediction and consequence of the 2011 Tohoku-oki tsunami. Sedimen. Geol. 282, 14–26. doi: 10.1016/j.sedgeo.2012.08.001
Thompson, V., Dunstone, N. J., Scaife, A. A., Smith, D. M., Slingo, J. M., Brown, S., et al. (2017). High risk of unprecedented UK rainfall in the current climate. Nat. Commun. 8:107. doi: 10.1038/s41467-017-00275-3
Vijayan, P. J., Kamble, M. T., Nayak, A. K., Vaze, K. K., and Sinha, R. K. (2013). Safety features in nuclear power plants to eliminate the need of emergency planning in public domain. Sâdhanâ 38, 925–943. doi: 10.1007/s12046-013-0178-5
Wang, L., Wang, L., Liu, Y., Gu, W., Xu, P., and Chen, W. (2019). The southwest China flood of July 2018 and its causes. Atmosphere 10:247. doi: 10.3390/atmos10050247
Woo, G. (2016). Counterfactual disaster risk analysis. Variance 10, 279–291. doi: 10.1016/S2542-5196(18)30209-2
Woo, G. (2017). Risk-Informed Tsunami Warning, Vol. 456. London: Geological Society, Special Publications, 191–197. doi: 10.1144/SP456.3
Woo, G., Maynard, T., and Seria, J. (2017). Counterfactual Risk Analysis. London: Lloyd’s of LONDON Report.
Woo, G., and Mignan, A. (2018). Counterfactual analysis of runaway earthquakes. Seism. Res. Lett. 89, 2266–2273. doi: 10.1785/0220180138
World Bank (2017). Nepal education sector analysis. National Institute for Research and Training and American Institute of Research report. Washington, DC: World Bank. doi: 10.1785/0220180138
Keywords: extreme event, downward counterfactual, Black Swan, stochastic modeling, over-fitting data
Citation: Woo G (2019) Downward Counterfactual Search for Extreme Events. Front. Earth Sci. 7:340. doi: 10.3389/feart.2019.00340
Received: 16 September 2019; Accepted: 04 December 2019;
Published: 19 December 2019.
Edited by:
Mark Bebbington, Massey University, New ZealandReviewed by:
Robin L. Dillon-Merrill, Georgetown University, United StatesDavid Johnston, Massey University, New Zealand
Copyright © 2019 Woo. This is an open-access article distributed under the terms of the Creative Commons Attribution License (CC BY). The use, distribution or reproduction in other forums is permitted, provided the original author(s) and the copyright owner(s) are credited and that the original publication in this journal is cited, in accordance with accepted academic practice. No use, distribution or reproduction is permitted which does not comply with these terms.
*Correspondence: Gordon Woo, Gordon.Woo@rms.com