- 1Centre National de Recherches Météorologiques, Météo France, Toulouse, France
- 2IFP Énergies Nouvelles, Rueil-Malmaison, France
- 3FCEyN, University of Buenos Aires, Buenos Aires, Argentina
- 4Computational Simulation Center, CSC - CONICET, Buenos Aires, Argentina
The offshore wind market is expected to see massive growth worldwide in the next decade. Recent research in this sector has led to a new generation of wind turbines characterized by larger rotors and higher capacity factors. Furthermore, the trend is to develop larger and denser wind farms which leads to potential deeper interactions with the troposphere. In order to provide an accurate modeling of the processes between the wind turbines, the surface and the atmospheric boundary layer, a new numerical tool has been developed. The ALM has been implemented into the opensource non-hydrostatic mesoscale atmospheric model Meso-NH used in the Large-Eddy Simulation (LES) framework. First, the tool is validated by comparing simulated results with data acquired during the New MEXICO experiments. In particular, good correlation are obtained for normal and tangential loads along the blades and the axial PIV traverse of instantaneous wind velocity components. Once the tool is validated, its ability to reproduce the Horns Rev 1 photo case is evaluated, since it is an emblematic case of wind farm-atmosphere interaction. Simulation output are post-processed using a render algorithm, resulting in synthetic images of cloud radiance. These images show great similarity with the photographs taken. All the results highlight the capacity of this new tool to represent interactions between wind farms and the lower atmosphere with a high level of details.
1. Introduction
Considerable deployments of offshore wind farms can be expected in the near future (IEA, 2019). After many years of development, the swept areas of modern, large rotors have increased substantially, resulting in wind plants influencing deeper portions of the atmospheric boundary layer. In fact, turbulent wakes produced by wind turbines can significantly affect the flow dynamics within wind farms and downstream areas. Operating offshore farms have already shown losses on electricity production (Barthelmie et al., 2009) and impacts on the local meteorology (Hasager et al., 2013).
To investigate these interactions, a better understanding of processes occurring between the atmospheric boundary layer, the surface and the wind turbines is necessary. This investigation can be done with numerical tools, among which Navier-Stokes based Computational Fluid Dynamics (CFD) models are frequently used. Several approaches can be implemented to solve the governing flow equations, such as the Reynolds averaged Navier Stokes (RANS), the Large-Eddy Simulation (LES), or the Direct Numerical Simulation (DNS). The RANS method computes mean flow and models an important part of turbulence aspects: it is not the proper tool to explore small scale wake eddies nor atmospheric boundary layer eddies. The DNS method is more precise but computationally too expensive as it resolves all turbulent scales. Because the LES approach consists in solving the large scales and modeling the smaller ones, it can be seen as an intermediate approximation and a good compromise.
In order to introduce wind turbines into a CFD software, different approaches can be used. One of those approaches is to simulate a fully-resolved blade, but this technique is highly resource-consuming for a whole wind farm for the moment. Introduced by Sørensen and Shen (2002), the Actuator Line Method (ALM) represents the three blades and their motion with a body force approach. Compared to the Actuator Disc Model from Rankine (1865) and Froude (1889), the ALM allows a better description of the wake behind the wind turbines, by reproducing the helical shape of the unsteady wake and capturing tip and root vortices as mentioned by Mikkelsen and Sørensen (2004), Ivanell (2009), and Troldborg (2009). This technique has already been coupled and validated with different LES solvers to simulate wind turbine and wind farms flows (Porté-Agel et al., 2011, Churchfield et al., 2012b). It has also been implemented into the Weather Research and Forecasting model (WRF) by Marjanovic et al. (2017).
The present paper introduces a new numerical tool allowing the study of wind farms impacts on local meteorology. This is achieved by coupling the ALM with the meteorological model Meso-NH (Lac et al., 2018). This model can simulate the atmospheric boundary layer using an LES framework, taking into account atmospheric phenomena such as buoyancy, thermal exchanges, humidity, and even cloud formation and precipitation.
The study is organized as follows: the numerical methods are described and validated in section 2. Section 3 introduces the Horns Rev 1 photo case, provides the simulation details and presents the results. Concluding remarks are provided in section 4.
2. Methods
2.1. Meso-NH Model
Meso-NH is a non-hydrostatic mesoscale atmospheric model (Lac et al., 2018) developed by the Centre National de Recherches Météorologiques (CNRM - Météo France/CNRS) and the Laboratories d'Aérologie (LA - UPS/CNRS). It can simulate atmospheric flows from meso to micro-scales. The governing equations are based on the conservation equations of mass, momentum, humidity and the thermodynamic equation derived from the conservation of entropy. A description of the dynamical core can be found in Lafore et al. (1998). The model is anelastic and based on the pseudo-compressible system of Durran (1989), filtering elastic effects from sound waves.
The numerical model can be used with a Large-Eddy Simulation framework. The second-order turbulent fluxes are computed through an 1.5-order closure proposed by Redelsperger and Sommeria (1981, 1986) and discussed in detail by Cuxart et al. (2000). The closure scheme is based on a prognostic equation of the subgrid turbulent kinetic energy and a diagnostic mixing length. The mixing length of Deardorff (1980) was chosen for this study, taking into account the mesh size and the stratification of the atmospheric boundary layer.
The approach is Eulerian and the domain is discretized using a staggered Arakawa C-grid (Mesinger and Arakawa, 1976). Wind advection is treated using a 4th-order centered spatial scheme combined with the explicit Runge Kutta scheme for time integration. Computations are horizontally parallelized (Jabouille et al., 1999), and the vertical coordinate can be stretched to increase the resolution in the region of interest.
2.2. Actuator Line Method
The ALM consists in modeling each blade by one line divided into blade element points (Sørensen and Shen, 2002). These points are applying aerodynamic forces into the flow. Each point carries a two-dimensional (2D) airfoil as shown in Figure 1, where c denotes the chord and the relative wind velocity. The relative velocity is a combination of the wind speed and the opposite of the tangential blade element speed , as seen by the airfoil. For a blade element point, the force is the sum of the drag force acting in the direction of (along the unit vector ), and the lift force , perpendicular to (along the unit vector ).
The aerodynamic forces are evaluated using the lift coefficient CL and the drag coefficient CD:
where ρ and dr are respectively, the air density and the length of the blade element. Both the lift and drag coefficients (CL and CD) depend on the airfoil characteristics, and are evaluated through a cubic spline interpolation of the tabulated data.
2.3. Coupled System
Meso-NH and the Actuator Line Method are exchanging information with each other. At each time step, the position, the velocity and the orientation matrix of blade element points are evaluated. Then, the ALM can use air properties (as ρ and ) from the three-dimensional (3D) fields of Meso-NH at the current time step in order to compute the aerodynamic force (Equation 1). The wind velocity is obtained using a tri-linear interpolation of the eight neighboring cell faces, surrounding the blade element point. Then, the aerodynamic force is projected in the global frame and added to the momentum equation of Meso-NH:
where is the advection term, the Coriolis term, the pressure gradient term, and the turbulence term.
To avoid numerical instabilities, a commonly used regularization kernel is used (Sørensen and Shen, 2002; Mikkelsen and Sørensen, 2004). Further studies also give guidelines for optimal volume force distribution regarding the kernel shape (Jha et al., 2014; Martínez-Tossas et al., 2016; Nathan et al., 2018). Nevertheless, in this study, the forces are distributed smoothly over nearby flux points by computing the spatially averaged force of the two closest mass points. It shows similar results, avoiding instabilities and the computational cost of a convolution product. Otherwise, the tip loss correction of Glauert (1935) is used to alleviate the over predicted loads at the blade tip region, as proposed by Mikkelsen and Sørensen (2004). One can note that this correction should only be used with the Actuator Disc Model to correct for finite number of blades: an improved load distribution will be investigated in future work, as advised by Churchfield et al. (2017).
To go further in computational cost savings, a time-splitting method was introduced, also known as the Actuator Sector Method (Storey et al., 2015). The Courant-Friedrichs-Lewy (CFL) criterion of Meso-NH imposes a time step ΔtMNH. Nevertheless, the ALM often requires a smaller time step in order to ensure that a blade element point will not skip a mesh cell during this time step (otherwise the information of the blade passage will be missed at this cell). The criterion for the Actuator Line Method time step ΔtALM can thus be expressed as:
where Δx, Δy, and Δz are the cell dimensions, Ω the angular speed of the rotor and rtip the tip radius of the blade. Because the meteorological model does not need such a small time step, a ΔtMNH respecting the CFL criterion -and not more- is preserved. In parallel, the ALM algorithm is called Nsplit times over this duration in order to respect the ALM time step criterion (Equation 3) and to reduce by Nsplit−1 the number of iterations from the LES solver. One can note that during a Meso-NH time step, the wind field is frozen: the same velocity vectors are sent to the blades at each sub-time step to compute the forces. In the end, for each blade element point, the applied force is the aerodynamic force evaluated by the ALM divided by Nsplit to respect the momentum budget over the time increment ΔtMNH.
2.4. Validation
2.4.1. The New MEXICO Experiments
To assess the accuracy of the results obtained with this coupled system, a validation study based on the New MEXICO (Model Experiments in Controlled Conditions) experiments is carried out (Boorsma and Schepers, 2014). These experiments have been performed in the German Dutch Wind Tunnel, using the 9.5 × 9.5 m open section of the Large Scale Low Speed Facility.
During these experiments, several flow cases were investigated (Boorsma and Schepers, 2014). The validation has been done for the three MexNext cases, but this study only focuses on the 10 m/s case corresponding to a high tip speed ratio (TSR ≈ 10). Indeed, in the work presented in section 3, the wind turbines were operating at a same TSR or higher.
The wind tunnel wind turbine is a three-bladed 4.5 m diameter rotor. The twisted and tapered blades are based on DU91-W2-250, RISØ-A1-21, and NACA64-418 airfoils from root to tip. The wind turbine is rotating at a constant rotational velocity Ω = 44.55 rad/s and a constant blade pitch angle β = –2.3°. The inflow is laminar and no initial turbulence is introduced. The normal and tangential loads are available at five positions thanks to the integration of pressure sensors measurements.
2.4.2. Numerical Set-Up
The dimension of the domain is about Lx = 40 m × Ly = 30 m × Lz = 30 m, with a Δx = Δy = Δz = 0.1 m resolution, leading to Nx = 400 × Ny = 300 × Nz = 300 uniformly spaced grid points. The discretization of 45 cells across the rotor diameter is thus consistent with the recommendations of Jha et al. (2014). For the Actuator Line Method, 42 elements are used to describe each blade. The hub is placed in the domain at (15, 15, 15 m). The wind turbine is placed at a high vertical level with the aim of avoiding the effect of the tiny boundary layer developing at the ground. The lateral and upper boundaries are open conditions (Bougeault et al., 1996). The upstream boundary generates a constant wind flow. The nacelle and the tower are not taken into account. As mentioned in section 2.3, the average method for the force distribution and the tip loss correction are used. A time step about Δt = 0.0005 s is chosen such that the blade tip does not pass through more than one grid cell. The time-splitting method will be investigated afterwards.
The wind speed, the potential temperature and the pressure are set as inputs according to the experimental data : respectively u = 10.05 m/s, θ = 293.65 K, p = 1013.98 hPa (Boorsma and Schepers, 2014). The density at the hub height, computed by Meso-NH regarding the aforementioned data, corresponds to the measured one (i.e., ρh = 1.197 kg/m3). The wind velocity profile is constant and the thermal conditions are neutral.
2.4.3. Validation Results
The numerical results are compared to the experimental data. The loads along the blade span (shown in Figure 2) and axial Particle Image Velocimetry (PIV) traverses (shown in Figure 3) are used. The obtained results are also compared to CASTOR and SOWFA simulations (Blondel et al., 2017). CASTOR is a free wake, vortex filament, lifting-line solver developed at IFPEN, and SOWFA is a library based on the CFD solver OpenFOAM developed at the National Renewable Energy Laboratory (Churchfield et al., 2012a).
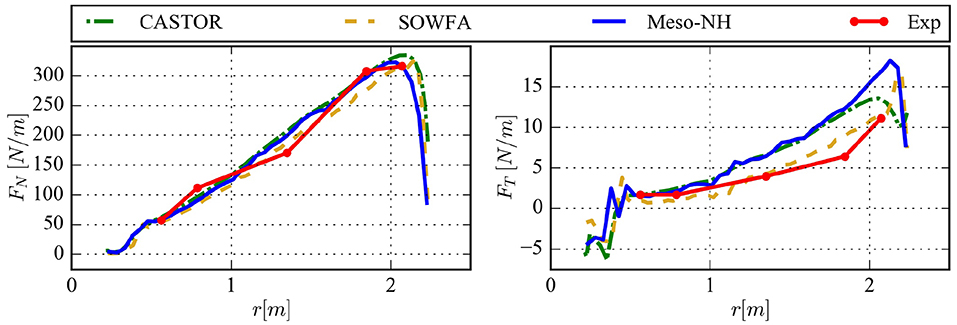
Figure 2. Comparison of computed normal FN and tangential FT forces per unit length along the blades for the 10 m/s case with the New MEXICO experiments (Boorsma and Schepers, 2014), CASTOR and SOWFA simulations (Blondel et al., 2017).
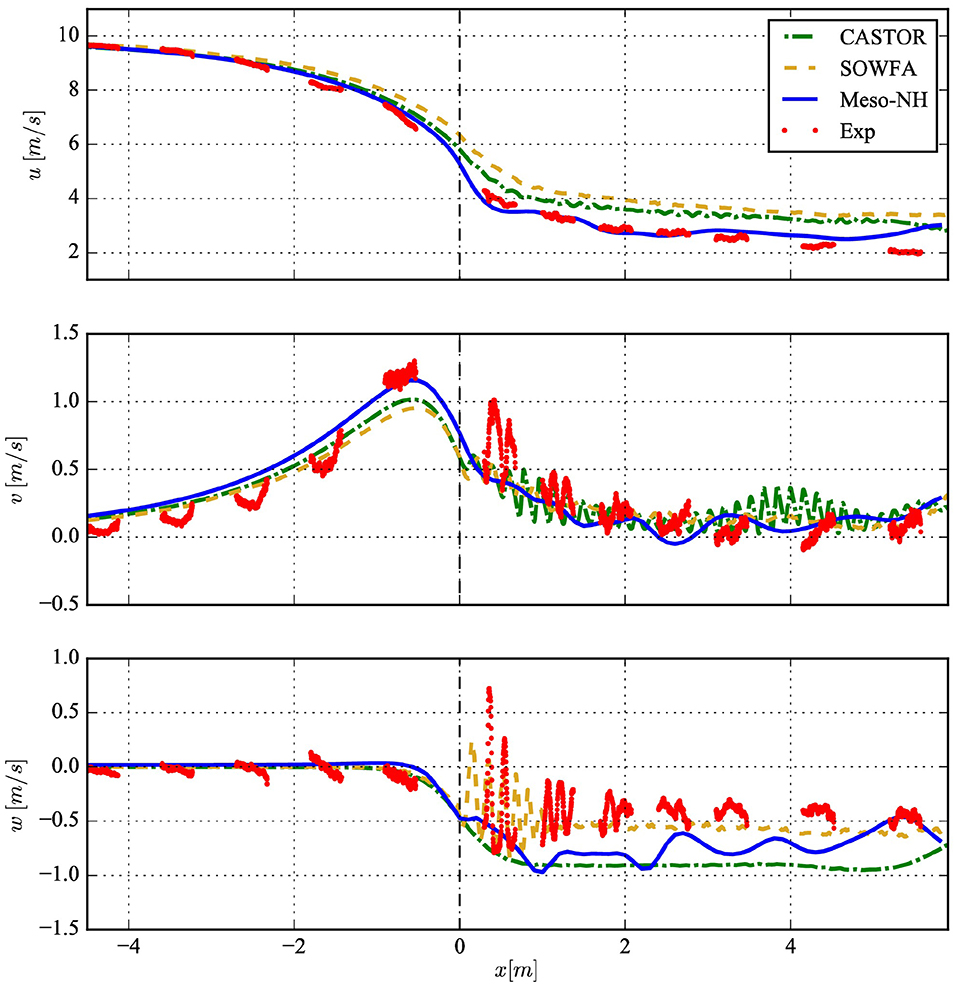
Figure 3. Comparison of axial PIV traverses for the case 10 m/s, with the New MEXICO experiments (Boorsma and Schepers, 2014), CASTOR and SOWFA simulations (Blondel et al., 2017).
Figure 2 shows the time-averaged normal and tangential loads along a blade after 7.552 s of simulation (i.e., more than 50 rotor rotations). The average loads are evaluated during the last rotation of the rotor (i.e., Δtaverage = 0.141 s). The computed normal load FN fits well with the comparative data. However, the computed tangential load FT shows an overestimation for the second half of the blade. As explained in Boorsma and Schepers (2014), the experimental tangential load is more difficult to obtain and is often overpredicted by numerical models. In fact, important uncertainties of tangential loads evaluation from pressure taps measurements are mentioned. The results can also be affected by the adopted assumptions, as the consideration of a 2D flow over airfoils, the use of non Reynolds-dependent airfoils, or the absence of the nacelle. Nevertheless, these results are in quite good agreement with both experimental and numerical data for the most part.
In Figure 3, the axial PIV traverse of instantaneous wind velocity components (u, v, and w) after 7.552 s of simulation are shown. These measurements are taken along an axial line ranging from x = −4.5 m to x = +5.9 m for a hub considered at position x = 0 m. This line crosses the rotor plane at radius r = 1.5 m in the 9 o'clock plane when looking from upstream, and when a blade is at azimuth 0° (upper vertical position). Numerically, it is not evident to reach this azimuth with a finite time step: at 7.552 s, the blade is at 2.1°.
The upper plot of Figure 3 shows the evolution of u component. A very good correlation between the Meso-NH results and the experiment can be observed. Below, both v and w components show good agreements in the induction area upstream the wind turbine, but do not fully capture the oscillating wake. It might be due to the mesh resolution of 10 cm, which is not enough to take into account smaller vortices. Furthermore, an underestimation of w can be noticed downstream the rotor, as for the others numerical results (Boorsma and Schepers, 2014). In any case, even if the nacelle and the tower are not modeled, and in spite of the quite low resolution, the results fit quite well with the experimental data.
2.4.4. Time-Splitting Results
In order to verify the conformity of the time-splitting method, another numerical study is carried out. The loads along the blades and the axial traverses are compared to the experimental data for different time-splitting factors Nsplit. The numerical set-up is the same as the one presented in 2.4.2, only the time step ΔtMNH is modified to obtain the desired time-splitting, as presented in Table 1. The duration about 7.552 s is conserved, but not for case D. In fact, this time is not reachable with a time step of 0.006 s. Because of that, an additional rotation is done for case D (i.e., 7.692 s), in order to reach an azimuth close to 0°.
Figure 4 shows a slight overestimation of the calculated loads when Nsplit increases. Nevertheless, the loads evaluation seems correct. The curve for case Dunsplit shows what would happen without the time-splitting method, and assess its functionality. A deterioration of the wake deficit can be observed in Figure 5 for the different cases. In fact, an important value of Nsplit leads to higher amplitude of oscillations. It might be due to the deformation of the numerical modeling of the blade. When the time-splitting is used, the modeled blade can be seen as a larger blade applying aerodynamic forces on a wider sector with less intensity.
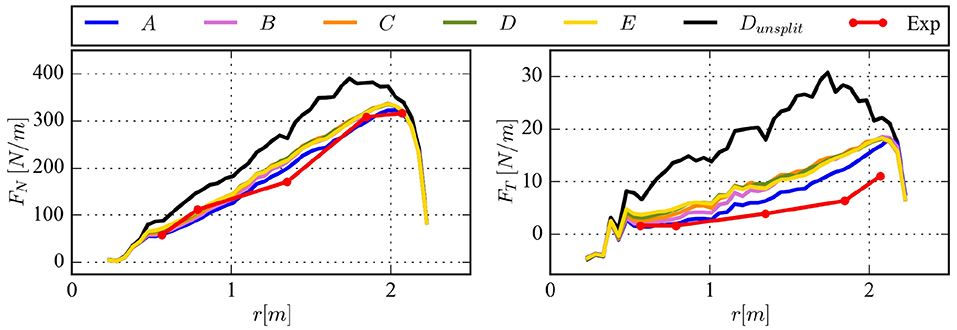
Figure 4. Comparison of predicted normal FN and tangential FT forces per unit length along the blades for the 10 m/s case with the New MEXICO experiment (Boorsma and Schepers, 2014) for different time-splitting factors.
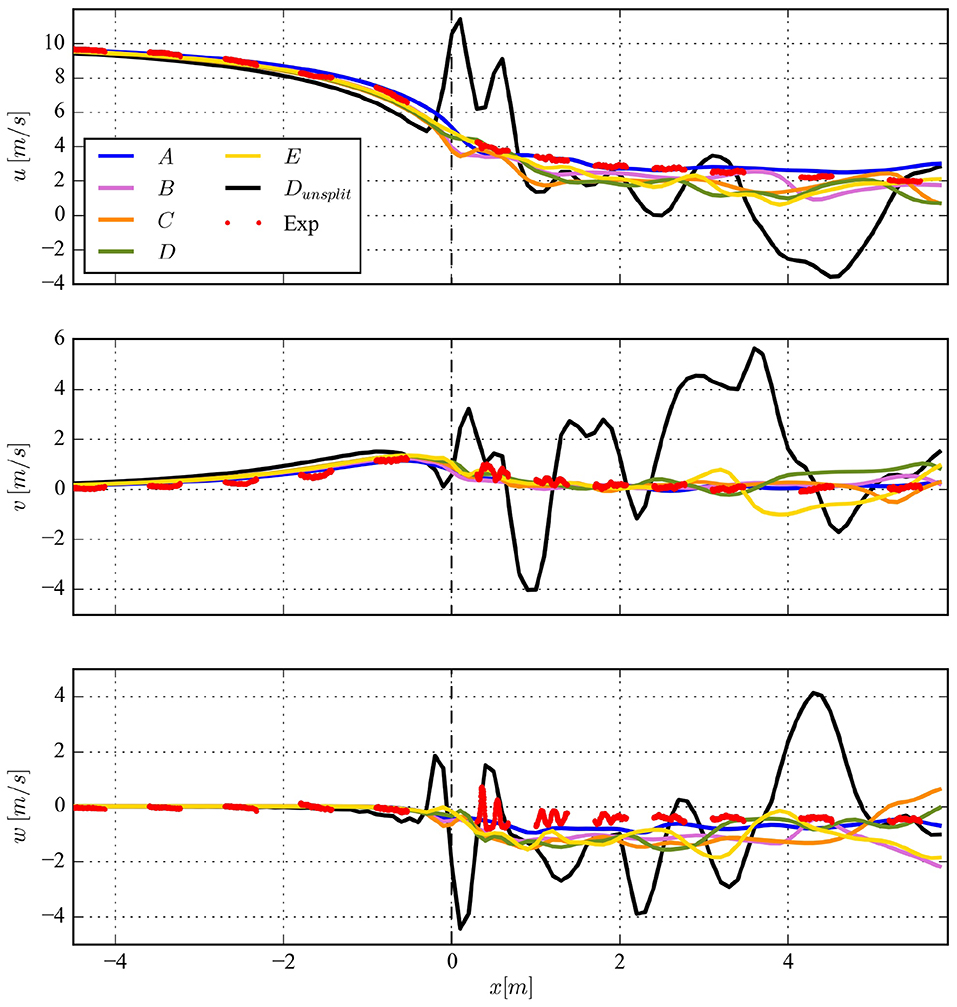
Figure 5. Comparison of axial PIV traverses for the case 10 m/s with the New MEXICO experiment (Boorsma and Schepers, 2014) for different time-splitting factors.
Table 1 also compares the computational time, evaluated during the last rotation of the wind turbine. It should be mentioned that the writing time of the output files is also taken into account. In any case, the gain obtained is directly linked to the meteorological solver, since unnecessary calculation steps have been avoided. The difference between D and Dunsplit about 3.6% is mainly due to the sub-calls of the ALM algorithm. According to the results, a factor of Nsplit = 7 seems to be a good compromise: it is enough to save computational cost and obtain a representative wake.
3. Application to the Horns Rev 1 Photo Case
3.1. Description
The Horns Rev 1 wind farm is well known for being the first large scale offshore wind farm in the world. It is located in the North Sea, west of Denmark and consists of 80 Vestas V80 wind turbines with 70 m hub height, 80 m rotor diameter and rated power of 2.0 MW. The parallelepiped shaped layout of the wind farm is arranged in 10 vertical and 8 horizontal rows, with a minimal distance of 7 diameters between the closest turbines.
In the morning of February 12, 2008 at around 10:10 UTC, the photographer Christian Steiness took two photos of the Horns Rev 1 wind farm from a helicopter (Figures 12A, 13A). These two photographs showed a physical evidence of the interaction between the wind farm and the lowest levels of the atmosphere, illustrating wind turbine-induced cloud formation downwind the wind farm.
A detailed description of the meteorological conditions at the time the photos were taken and the process that would have lead to the clouds formation can be found in Hasager et al. (2013). This rare phenomenon is a consequence of the combination of very specific conditions, involving a layer of cold and humid air above a warmer sea surface that re-condensates to fog in the turbines wakes. The torque exerted by the flow on the wind turbine blades induces rotation in the wake, causing upward movements of humid warm air from near the sea surface and downward movements of relatively cooler and dryer air. The rotor-induced updraft causes air parcel to rise, as the pressure decreases with height, the air parcel expands and its temperature decreases. Therefore, the relative humidity of the lifted parcel increases. At some height, the parcel becomes saturated and a subsequent condensation of water vapor leads to cloud formation. In summary, the large scale structure of the wake fog is produced by the rotational pattern of the spiraling bands.
Despite the fact that Horns Rev I is one of the most studied wind farms in the world, with three meteorological masts installed on-site, one of the main difficulties to simulate the photo case is the need of very precise information to reproduce the specific conditions that led to the wake fog formation. Hasager et al. (2013) conducted an exhaustive compilation of much of the available information, including satellite data, radiosounding profiles and surface observations. The mentioned on-site data come from the meteorological tower (M6) installed in the park which provides measurements of temperature at 16, 64, and –3 m (sea temperature). This met mast also gives measurements of wind velocity at three different heights (30, 40, and 70 m) and wind direction at 28 and 68 m. To have a more complete description of the vertical structure of the atmosphere for the region and moment of interest, the present work also used reanalysis data from the Modern-Era Retrospective analysis for Research and Applications (MERRA) (Rienecker et al., 2011).
The wake fog simulation is performed using Meso-NH through two steps. The objective of the first step is to establish the precursor meteorological conditions without the wind turbines. The second one includes the wind farm that leads to the wake clouds development.
3.2. Precursor Simulation
In order to start the spin-up simulation, various vertical profiles of the initial state of the atmosphere must be set. In Figure 6, the vertical profiles (horizontally and 10-min averaged) of wind speed, temperatures, and vapor mixing ratio, used to initialize the whole domain (blue dashed lines) are shown. The profiles after 2 h of simulation (blue solid lines) are also plotted. The potential temperature θ is defined as:
where P is the air pressure and P0 a reference pressure set to 1,000 hPa.
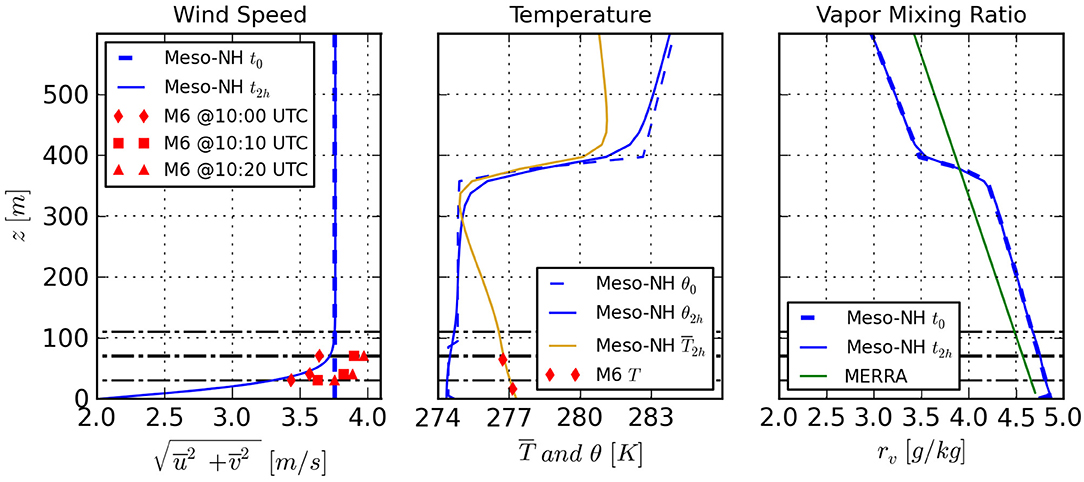
Figure 6. Wind speed, temperature and vapor mixing ration profiles. M6 Data from Hasager et al. (2013).
The initial conditions were deduced from the met mast (M6) and the reanalysis data. Because of its position, the met mast M6 is considered to be not affected by the wake, nor by the fog development. For the wind, several uniform conditions (i.e., intensity and direction) have been tested to initialize the atmospheric state. Indeed, during the simulation, the atmospheric flow will tend to an equilibrium between the geostrophic wind forcing, the surface friction and the Coriolis effect. The chosen value for the initial profile corresponds to the geostrophic wind and allow to obtain the measured intensity and direction provided in Hasager et al. (2013). As it can be seen in Figure 6, the 10 min averaged wind profile after 3 h of simulation shows good agreement with the met mast data at the moment before the photo, 10:00 UTC. At this time, by taking into account the reanalysis data, a boundary layer height of 400 m is determined. A strong inversion of potential temperature is established at this level. In the aim of maintaining the sea smoke under the rotor level and because it is the beginning of the day, a surface layer inside the boundary layer with a weaker inversion must be set. After a sensitivity analysis, a surface layer with a height of 90 m is introduced with a second and weaker inversion of Δθ = –0.4 K. It was shown that for any value greater than the selected one, no cloud would develop: the air mass would become too warm in the upper part of the rotor to condense. As it is shown in Figure 6, both surface and residual layers are neutral at the starting point of the simulation, and later, after 3 h of simulation, they become slightly stable but still distinguishable. It can also be noticed that the simulated temperature is in good agreement with the observed temperature from the mast near the surface. The initial condition for the sea temperature has been obtained from the met mast data at –3 m. The reported value at 10:00 UTC was 277.85 K with a ± 0.7 K precision. After a sensitivity study, this value was set to 277.35 K. A warmer sea would lead to a more unstable air layer, with greater associated thermal turbulence which would lead to a cloudy development that would not correspond to the layered sea smoke that can be observed in the photo (Figures 12A, 13A). The sea level pressure is about 103890 Pa. As there are no in-situ data for the vapor mixing ratio rv, the MERRA reanalysis data have been used to define its order of magnitude. In Figure 6, a typical shape of rv profile can be observed, with higher values in the atmospheric boundary layer, and lower ones in the free atmosphere.
The precursor simulation is configured with a domain of 7,500 × 25,000 × 837.5 m, a horizontal resolution of 5 m and a time step of 1 s. The vertical levels are defined with a vertical resolution of 5 m below 200 m and then a 20% stretching is applied until a resolution of 20 m is reached. The boundary conditions are cyclic. The long-wave and short-wave radiation are computed following (Fouquart and Bonnel, 1980; Mlawer et al., 1997), respectively. To deal with the micro-physical phenomena that enable clouds formation, the single moment 6-class scheme ICE3 is selected (see Pinty and Jabouille, 1998). Finally, the sea surface is represented by using the Sea Flux Scheme detailed in Belamari (2005).
3.3. Wind Farm Simulation
3.3.1. Set-Up
Once the precursor 3D state of the atmosphere is established, the Horns Rev 1 wind farm is placed in the domain and a second simulation is performed for a period of 32 min. The domain configuration is the same as the previous one with 1,500 points in the direction, 5,000 points in the direction and 75 levels in the vertical one with 16 levels within the rotor area. As shown in Figure 7, this configuration allows to have a distance of 5 km upstream the wind farm, and more than 15 km downstream to observe the evolution of the wake. On each side, a distance of 1,250 m (i.e., 15 rotor diameters) separates the wind farm from the lateral boundaries. The layout is based on the data from Hansen (2012).
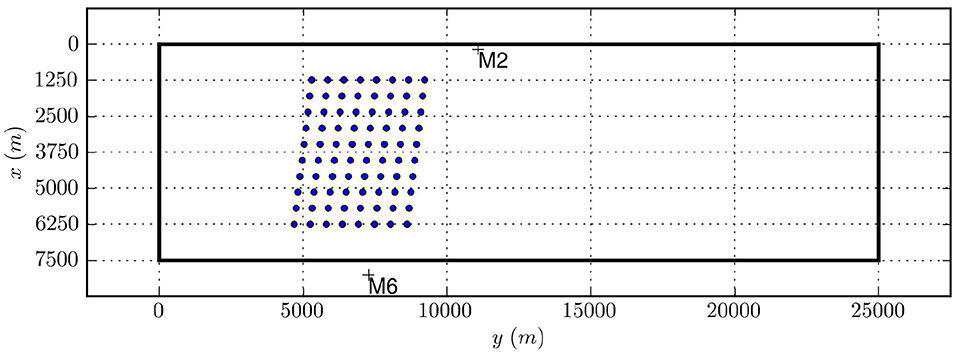
Figure 7. Layout of the Horns Rev 1 offshore wind farm. The blue bullets (•) indicate the location of wind turbines. The black crosses (+) indicate the met masts position. The thick black box denotes the domain boundaries.
The prevailing wind direction is 180° at the hub height (i.e., parallel to ) and the yaw angle of the wind turbines is set to zero. The Vestas V80 is a three-bladed rotor. The twisted and tapered blades are based on FFA-W3-301, FFA-W3-241, FFA-W3-221, NACA-63-221, and NACA-63-218 airfoils from root to tip, according to Hansen (2012). The different airfoil properties can be found in Fuglsang et al. (1998), Abbott and Von Doenhoff (1959) and Bertagnolio et al. (2001). The SCADA data of the whole Horns Rev 1 wind farm is reported in Hasager et al. (2013). The rotational velocities of the wind turbines recorded at 10:00 UTC are used. The pitch angle is set to 0° because the turbines operated near the cut-in speed. For the Actuator Line Method, the blades are divided into 42 element points and the tip loss correction is used Glauert (1935). The time step is set to 0.25 s, using a time-splitting factor of 7.
3.3.2. Results
After 32 min of simulation with the wind farm, several variables are studied to analyze the wind farm interaction with the local meteorology.
The relative humidity horizontal cross-sections, at 30 m and 110 m above the sea are presented in Figure 8. On the two figures, a quite high value of relative humidity (97–98%) can be observed around the wind farm. Differences can be noticed under and over the wind farm wake. At the 30 m level, the relative humidity values are mostly lower than the surroundings. An opposite behavior is shown at the 110 m level, with higher values of RH. This configuration is the consequence of the upward and downward displacements of air masses produced by the rotational pattern of the operating rotors, and can be explained by analyzing the vapor mixing ratio and the potential temperature. A moisture transport is clearly observable within the region of the wake, in Figure 9, where an area of lower moisture content in relation to its environment for low levels and higher moisture content for high levels can be observed. The wake of the wind turbines causes a vertical homogenization of the vapor mixing ratio. This homogenization is also noticeable in Figure 10, where the potential temperature cross-sections are plotted: the potentially cooler air mass under the farm is mixed with the potentially warmer air mass above it, in the wake of the turbines. The combination generates within the wake a potentially warmer air mass with less vapor below the farm and a potentially cooler air mass with more vapor above.
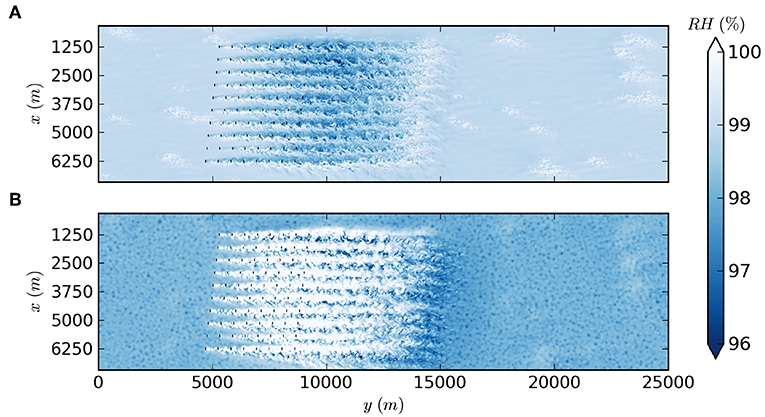
Figure 8. Horizontal cross-sections at 30 m (A) and 110 m (B) above the sea of the relative humidity RH. The black plain lines (–) delimits the locations of wind turbines.
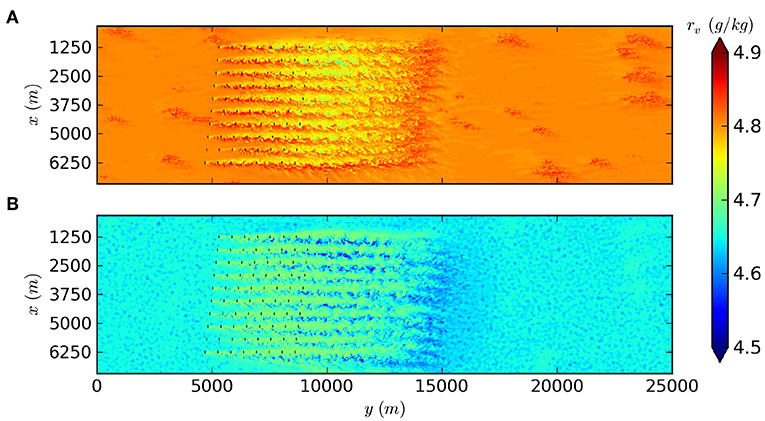
Figure 9. Horizontal cross-sections at 30 m (A) and 110 m (B) above the sea of the vapor mixing ratio rv. The black plain lines (–) indicate the locations of wind turbines.
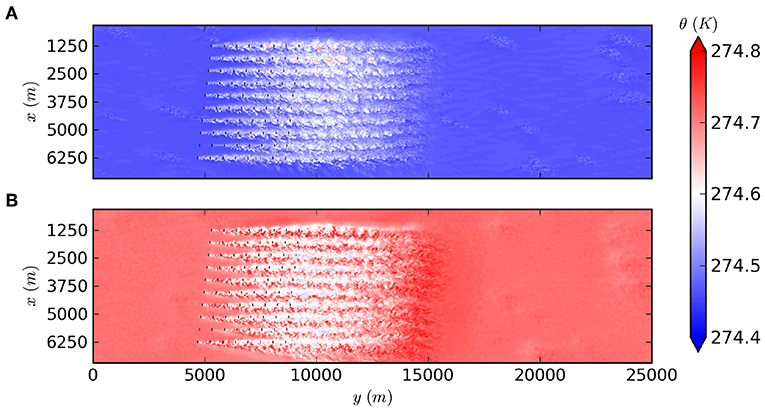
Figure 10. Horizontal cross-section at 30 m (A) and 110 m (B) above the sea of the potential temperature θ. The red plain lines (–) indicate the locations of wind turbines.
From these figures (Figures 8–10), it is also possible to identify the Ekman spiral effect as the wake is slightly tilted to the west in the 30 m plot and, on the contrary, deflected toward the east in the level of 110 m.
In Figure 11, the vertical cross-section of the cloud mixing ratio (rc) through the fourth row of the wind farm is shown. The development of the wake cloud above the hub height along the row is observed. As in the photo, behind each first wind turbines, a constant cloud about 2 or 3 diameters long can be observed at the top of the rotor. Downstream these clouds, at the tip, a vortex shedding is also distinguishable on the photos, and reproduced by the numerical model. Thereafter, eddies periodically interact with downstream wind turbines and become bigger. After a few downstream wind turbines, the chaotic structure of the cloud becomes more established. An underrepresentation of the clouds can be noticed: the sea smoke recognizable on the photo is not reproduced, even if high values of relative humidity are reached.
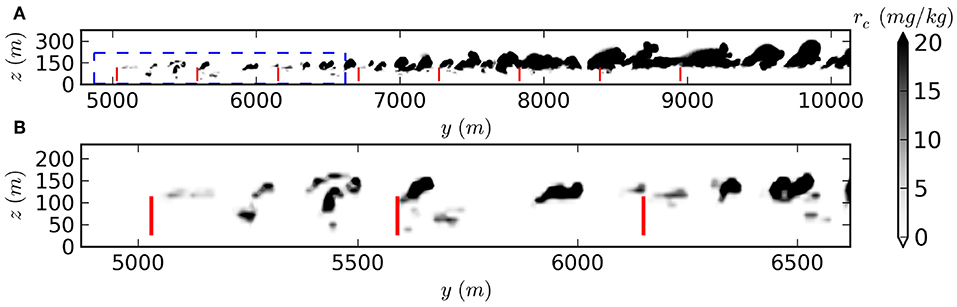
Figure 11. Vertical cross-section of the cloud mixing ratio through the whole fourth row of the wind farm (A) and the firsts wind turbines (B). The red plain lines (–) indicate the locations of wind turbines. The blue dashed line (- -) in A indicates the area of B.
Figures 12B, 13B are synthetic images of atmospheric fields (temperature, pressure, liquid and water vapor), simulated by the open source htrdr Monte Carlo code (Villefranque et al., 2019). To render these realistic cloud scenes, a virtual camera is placed approximatively at the positions of the camera of Christian Steiness when he took the photos (Figures 12A, 13A). From the comparison between Figures 12A,B, the capacity of the Meso-NH/ALM tool to reproduce the Horns Rev I photo case phenomenon can be observed. The cloud structures presented in the figures are very similar, with the wakes widening downstream and the spiral pattern noticeable in each row.
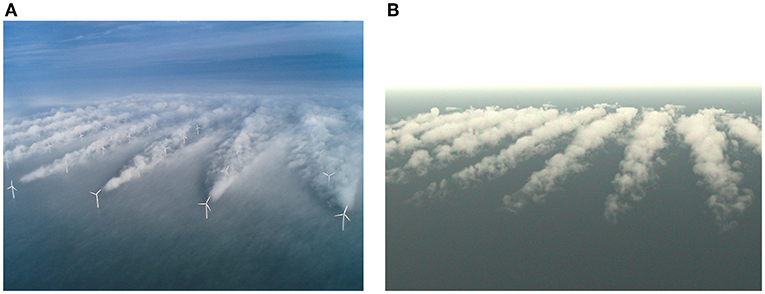
Figure 12. (A) Photograph of the Horns Rev 1 wind farm (12/02/2008 around 10:10 UTC) from south (Hasager et al., 2013). Courtesy Vattenfall. Photographer: Christian Steiness. (B) Post-processing (see Villefranque et al., 2019) of simulated cloud mixing ratio. Wind turbines are not represented.
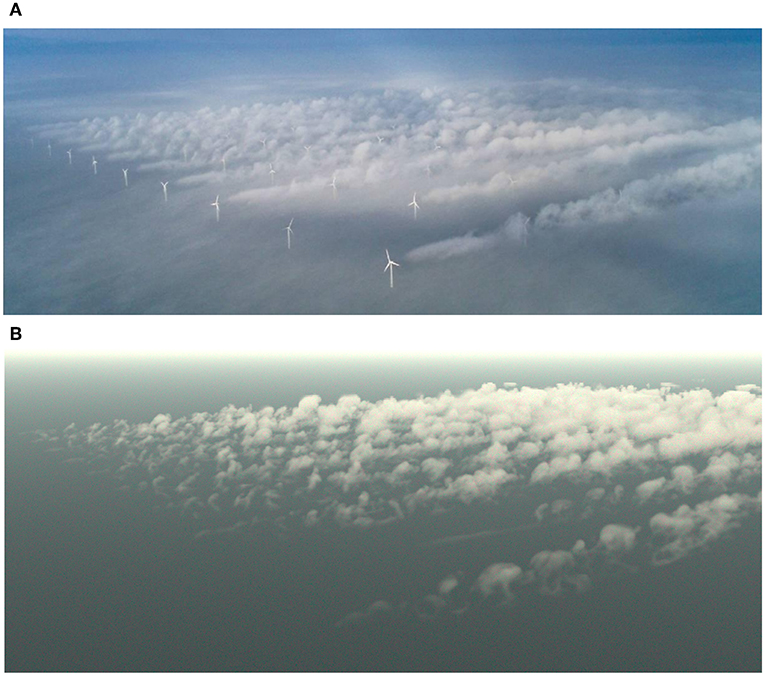
Figure 13. (A) Photograph of the Horns Rev 1 wind farm (12/02/2008 around 10:10 UTC) from southeast (Hasager et al., 2013). Courtesy Vattenfall. Photographer: Christian Steiness. (B) Post-processing (see Villefranque et al., 2019) of simulated cloud mixing ratio. Wind turbines are not represented.
Between the photo taken from the southeast (Figure 13A) and the post-processing render (Figure 13B) there is a great agreement. As the first turbine of the second row was not working at the time of the photo, it was also stopped in the simulation. This explains the absence of cloud wake downstream of that turbine in both the photo and the render.
4. Conclusion
As wind power production is increasing all over the world and the wind turbines are getting larger and taller over the years, the study of their interaction with the lowest levels of the atmosphere becomes relevant. To address this challenge, a new numerical tool was developed to explore the impacts of wind farms on the local meteorology. This new tool is a coupled model between the atmospheric mesoscale model Meso-NH used in the LES framework and the Actuator Line Method. In order to validate the tool, comparisons against New MEXICO measurements and other numerical models have been made. The predicted loads along the blades and the axial wind intensity at hub height showed good correlation with the measurements for the high tip speed ratio (TSR) value. The time-splitting method has also been validated, allowing to save computation time and obtain a representative wake.
With the goal of evaluating the capability of this new tool to reproduce a real case of interaction between a wind farm and the atmospheric boundary layer, a simulation of an idealized case of the Horns Rev I Photo case was performed. First, a spin-up simulation was carried out to reproduce the existing weather conditions before the photo, based on in situ met-mast measurements and MERRA reanalysis data. The resulting atmospheric 3D state showed good agreement with the measurements. This atmospheric field was then used as initial conditions for the second simulation where the full wind farm was introduced. With a post-processing of the cloud mixing ratio, two renders of the resulting cloud scenes were made. Their main feature was the great similarity with the photographs, presenting the well developed spiral cloud pattern in each row of the wind farm. It should be mentioned that the liquid water is slightly underestimated by the model, especially at the lower levels. It may be improved by taking into account aerosols such as the sea salts. However, the mechanism that gave rise to the wake cloud is well-described by the model, with rotating rotors carrying potentially cooler and wetter air from lower levels; and potentially warmer and drier air from higher ones. The condensation that occurred in the upward movement leads to the development of the cloud.
It should be underlined that the phenomenon showed by the Horns Rev 1 photo case can only appear under a specific set of atmospheric conditions. In fact, the sensitivity studies carried out without the wind farm (not presented) showed that small changes in temperatures would have led to a global fog formation or no cloud at all.
Based on the results of this study, the capability of this new tool to simulate the wake dynamics and to represent their impact and interaction with the atmospheric quantities was shown. Furthermore, this work demonstrates more generally the ability of coupled weather and wind plant simulation frameworks to capture their complex physical interactions.
Moreover, the atmospheric boundary layer is typically shallower over the sea, while offshore wind turbines are getting larger. It will then become possible for blades to cross the top of the boundary layer, which is often characterized by an abrupt temperature inversion and strong wind shear. At that point, simulations of new generations of wind farms will be conducted with the aim of investigating those interactions.
Author Contributions
P-AJ developed the numerical coupling, performed the numerical experiments, and led the analysis of the study. MM gathered all the meteorological data available for the Hors Rev 1 Photo case, launched the firsts Horns Rev simulations and participated to the redaction of the manuscript. VM, FB, QR, MC, and CL substantially contributed to the development and the manipulation of the different models. All authors contributed on the comprehension of the study, as well as on the revision and correction of the manuscript. Agreement to be accountable for all aspects of the work in ensuring that.
Funding
MM received funding from the Make Our Planet Great Again (MOPGA) initiative for a research stay at CNRM.
Conflict of Interest
The authors declare that the research was conducted in the absence of any commercial or financial relationships that could be construed as a potential conflict of interest.
Acknowledgments
New MEXICO data have been supplied by the consortium which carried out the EU FP5 project MEXICO: Model rotor Experiments In Controlled conditions. We wish to thank the IEA Wind Task 31 WakeBench which provided the description of the Horns Rev 1 wind farm and of the Vestas80 wind turbine model. We would like to acknowledge the Wake Conference 2019 for publishing our preliminary results under the IOP Proceedings Licence (https://iopscience.iop.org/article/10.1088/1742-6596/1256/1/012019). We also acknowledge Vattenfall to let us publish the photographs (https://creativecommons.org/licenses/by-nd/4.0/).
References
Abbott, I. H., and Von Doenhoff, A. E. (1959). Theory of Wing Sections, Including a Summary of Airfoil Data. New York, NY: Courier Corporation.
Barthelmie, R. J., Hansen, K., Frandsen, S. T., Rathmann, O., Schepers, J. G., Schlez, W., et al. (2009). Modelling and measuring flow and wind turbine wakes in large wind farms offshore. Wind Energy 12, 431–444. doi: 10.1002/we.348
Belamari, S. (2005). Report on uncertainty estimates of an optimal bulk formulation for surface turbulent fluxes. Marine EnviRonment and Security for the European Area–Integrated Project (MERSEA IP), Deliverable D, 4, France.
Bertagnolio, F., Sørensen, N. N., Johansen, J., and Fuglsang, P. (2001). Wind Turbine Airfoil Catalogue. Roskilde: Forskningscenter Risoe.
Blondel, F., Ferrer, G., Cathelain, M., and Teixeira, D. (2017). “Improving a bem yaw model based on newmexico experimental data and vortex/cfd simulations,” in Congrès Français de Mécanique (Lille: Association Française de Mécanique), 1–14.
Boorsma, K., and Schepers, J. (2014). New MEXICO experiment: Preliminary Overview With Initial Validation. Petten: ECN.
Bougeault, P., Mascart, P., and Chaboureau, J. (1996). The meso-nh Atmospheric Simulation System: Scientific Documentation. Touloouse: Météo-France and CNRS.
Churchfield, M., Lee, S., and Moriarty, P. (2012a). Overview of the Simulator for Wind Farm Application (sowfa). National Renewable Energy Laboratory.
Churchfield, M., Lee, S., Moriarty, P., Martinez, L., Leonardi, S., Vijayakumar, G., et al. (2012b). “A large-eddy simulation of wind-plant aerodynamics,” in 50th AIAA Aerospace Sciences Meeting including the New Horizons Forum and Aerospace Exposition, Nashville, TN, 537.
Churchfield, M. J., Schreck, S. J., Martinez, L. A., Meneveau, C., and Spalart, P. R. (2017). “An advanced actuator line method for wind energy applications and beyond,” in 35th Wind Energy Symposium, Grapevine, TX, 1998.
Cuxart, J., Bougeault, P., and Redelsperger, J.-L. (2000). A turbulence scheme allowing for mesoscale and large-eddy simulations. Quart. J. Roy. Meteorol. Soc. 126, 1–30. doi: 10.1002/qj.49712656202
Deardorff, J. W. (1980). Stratocumulus-capped mixed layers derived from a three-dimensional model. Bound. Layer Meteorol. 18, 495–527.
Fouquart, Y., and Bonnel, B. (1980). Computations of solar heating of the earth's atmosphere- a new parameterization. Beitraege zur Physik der Atmosphaere 53, 35–62.
Froude, R. E. (1889). On the part played in propulsion by differences of fluid pressure. Trans. Inst. Naval Architects 30:390.
Fuglsang, P., Antoniou, I., Dahl, K. S., and Madsen, H. A. (1998). Wind tunnel tests of the FFA-W3-241, FFA-W3-301 and NACA 63-430 airfoils. Roskilde: Forskningscenter Risoe.
Glauert, H. (1935). “Airplane propellers,” in Aerodynamic Theory, ed W. Durand (Berlin; Heidelberg: Springer), 169–360.
Hansen, K. (2012). Presentation of horns rev offshore wind farm and vestas v80 wind turbine. Lyngby: IEA WInd Task 31 Wakebench.
Hasager, C. B., Rasmussen, L., Peña, A., Jensen, L. E., and Réthoré, P.-E. (2013). Wind farm wake: the horns rev photo case. Energies 6, 696–716. doi: 10.3390/en6020696
Ivanell, S. S. A. (2009). Numerical computations of wind turbine wakes. Stockolm, Ph.D. thesis, KTH, Mechanics, Linné Flow Center, FLOW.
Jabouille, P., Guivarch, R., Kloos, P., Gazen, D., Gicquel, N., Giraud, L., et al. (1999). “Parallelization of the french meteorological mesoscale model mésonh,” in Euro-Par'99 Parallel Processing, eds P. Amestoy, P. Berger, M. Daydé, D. Ruiz, I. Duff, V. Frayssé (Berlin; Heidelberg. Springer Berlin Heidelberg), 1417–1422.
Jha, P. K., Churchfield, M. J., Moriarty, P. J., and Schmitz, S. (2014). Guidelines for volume force distributions within actuator line modeling of wind turbines on large-eddy simulation-type grids. J. Solar Energy Eng. 136:031003. doi: 10.1115/1.4026252
Lac, C., Chaboureau, J.-P., Masson, V., Pinty, J.-P., Tulet, P., Escobar, J., et al. (2018). Overview of the meso-nh model version 5.4 and its applications. Geoscient. Model Dev. 11, 1929–1969. doi: 10.5194/gmd-11-1929-2018
Lafore, J. P., Stein, J., Asencio, N., Bougeault, P., Ducrocq, V., Duron, J., et al. (1998). The meso-nh atmospheric simulation system. part i: adiabatic formulation and control simulations. Ann. Geophys. 16, 90–109.
Marjanovic, N., Mirocha, J. D., Kosoviç, B., Lundquist, J. K., and Chow, F. K. (2017). Implementation of a generalized actuator line model for wind turbine parameterization in the weather research and forecasting model. J. Renew. Sustain. Energy 9:063308. doi: 10.1063/1.4989443
Martínez-Tossas, L. A., Churchfield, M. J., and Meneveau, C. (2016). A highly resolved large-eddy simulation of a wind turbine using an actuator line model with optimal body force projection. J. Phys. Confer. Ser. 753:082014. doi: 10.1088/1742-6596/753/8/082014
Mesinger, F., and Arakawa, A. (1976). Numerical methods used in atmospheric models. Research report.
Mikkelsen, R. F., and Sørensen, J. N. (2004). Actuator Disc Methods Applied to Wind Turbines. Ph.D. thesis, Denmark.
Mlawer, E. J., Taubman, S. J., Brown, P. D., Iacono, M. J., and Clough, S. A. (1997). Radiative transfer for inhomogeneous atmospheres: Rrtm, a validated correlated-k model for the longwave. J. Geophys. Res. Atmosph. 102, 16663–16682.
Nathan, J., Masson, C., and Dufresne, L. (2018). Near-wake analysis of actuator line method immersed in turbulent flow using large-eddy simulations. Wind Energy Sci. 3, 905–917.
Pinty, J., and Jabouille, P. (1998). “A mixed-phase cloud parameterization for use in mesoscale non-hydrostatic model: simulations of a squall line and of orographic precipitations,” in Conference on Cloud Physics (Everett, WA: American Meteorological Society), 217–220.
Porté-Agel, F., Wu, Y.-T., Lu, H., and Conzemius, R. J. (2011). Large-eddy simulation of atmospheric boundary layer flow through wind turbines and wind farms. J. Wind Eng. Indust. Aerodynam. 99, 154–168. doi: 10.1016/j.jweia.2011.01.011
Rankine, W. J. M. (1865). On the mechanical principles of the action of propellers. Trans. Instit. Naval Archit. 6, 13–39.
Redelsperger, J. L., and Sommeria, G. (1981). Methode de representation de la turbulence d'echelle inferieure a la maille pour un modele tri-dimensionnel de convection nuageuse. Bound. Layer Meteorol. 21, 509–530.
Redelsperger, J. L., and Sommeria, G. (1986). Three-dimensional simulation of a convective storm: Sensitivity studies on subgrid parameterization and spatial resolution. J. Atmosph. Sci. 43, 2619–2635.
Rienecker, M. M., Suarez, M. J., Gelaro, R., Todling, R., Bacmeister, J., Liu, E., et al. (2011). Merra: Nasa's modern-era retrospective analysis for research and applications. J. Clim. 24, 3624–3648. doi: 10.1175/JCLI-D-11-00015.1
Sørensen, J. N., and Shen, W. Z. (2002). Numerical modeling of wind turbine wakes. J. Fluids Eng. 124, 393–399. doi: 10.1115/1.1471361
Storey, R. C., Norris, S. E., and Cater, J. E. (2015). An actuator sector method for efficient transient wind turbine simulation. Wind Energy 18, 699–711. doi: 10.1002/we.1722
Keywords: wind turbine wake, wind farm wake, meteorological interaction, actuator line method, large-eddy simulation, new MEXICO experiments, horns Rev
Citation: Joulin P-A, Mayol ML, Masson V, Blondel F, Rodier Q, Cathelain M and Lac C (2020) The Actuator Line Method in the Meteorological LES Model Meso-NH to Analyze the Horns Rev 1 Wind Farm Photo Case. Front. Earth Sci. 7:350. doi: 10.3389/feart.2019.00350
Received: 23 August 2019; Accepted: 17 December 2019;
Published: 10 January 2020.
Edited by:
Gert-Jan Steeneveld, Wageningen University & Research, NetherlandsReviewed by:
Jeffrey Mirocha, Lawrence Livermore National Laboratory, United States Department of Energy (DOE), United StatesChenghai Wang, Lanzhou University, China
Copyright © 2020 Joulin, Mayol, Masson, Blondel, Rodier, Cathelain and Lac. This is an open-access article distributed under the terms of the Creative Commons Attribution License (CC BY). The use, distribution or reproduction in other forums is permitted, provided the original author(s) and the copyright owner(s) are credited and that the original publication in this journal is cited, in accordance with accepted academic practice. No use, distribution or reproduction is permitted which does not comply with these terms.
*Correspondence: Pierre-Antoine Joulin, cGllcnJlLWFudG9pbmUuam91bGluQGlmcGVuLmZy