- 1Institute for Radiological Protection and Nuclear Safety, Fontenay-aux-Roses, France
- 2CEA, DAM, DIF, Arpajon, France
This paper illustrates how an emulator (or meta-model) of a tsunami code can be a useful tool to evaluate or qualify tsunami hazard levels associated with both specific and unknown tsunamigenic seismic sources. The meta-models are statistical tools permitting to drastically reduce the computational time necessary for tsunami simulations. As a consequence they can be used to explore the tsunamigenic potential of a seismic zone, by taking into account an extended set of tsunami scenarios. We illustrate these concepts by studying the tsunamis generated by the Azores-Gibraltar Plate Boundary (AGPB) and potentially impacting the French Atlantic Coast. We first analyze the impact of two realistic scenarios corresponding to potential sources of the 1755-Lisbon tsunami (when uncertainty on seismic parameters is considered). We then show how meta-models could permit to qualify the tsunamis generated by this seismic area. All the results are finally discussed in light of tsunami hazard issued by the TSUMAPS-NEAM research project available online (http://ai2lab.org/tsumapsneam/interactive-hazard-curve-tool/). From this methodological study, it appears that tsunami hazard issued by TSUMAPS-NEAM research project is envelop, even when compared to all the likely and unlikely tsunami scenarios generated in the AGPB area.
Introduction
The evaluation of tsunami impact requires accurate simulation results for planning and risk assessment purposes because of the severe consequences which could be associated to this kind of event. Considering that tsunami phenomena involve a large span of parameters at different spatial and temporal scales (Behrens and Dias, 2015), even a single run of a tsunami numerical model can be prohibitively long, in the order of minutes to days, according to the study area characteristics and to the resolution of the numerical model. Hence, a common practice when the computational code is time-consuming is the use of meta-models (also denoted surrogate-models or emulators). A meta-model is a mathematical model of approximation of the numerical model, built on a learning basis (Razavi et al., 2012a). Meta-models have been applied, for example, in hydraulic fields to model physical variables such as flows (Wolfs et al., 2015; Machac et al., 2016), flood damages (Yazdi and Salehi Neyshabouri, 2014), or in the field of the design for civil flood defenses (Richet and Bacchi, 2019). A comprehensive review of the use of meta-models in environmental research was proposed by Razavi et al. (2012a) for the interested reader.
Meta-models have also already been used in the field of tsunamis. For instance, Sraj et al. (2014) investigate the uncertainties in the resulting wave elevation predictions due to the uncertainty in the Manning’s friction coefficient, using polynomial chaos expansion to build a surrogate model that is a computationally cheap approximation of the computer model. Sarri et al. (2012) used in a similar way a statistical emulator of the analytical landslide-generated tsunami model developed by Sammarco and Renzi (2008). More recently, Rohmer et al. (2018) studied the uncertainty related to the source parameters through a Bayesian procedure to infer (i.e., learn) the probability distribution of the source parameters of the earthquake. However, to our knowledge, meta-modeling has never been used for tsunami hazard analysis.
In this paper, we propose to apply meta-modeling techniques in the framework of deterministic tsunami hazard assessment (DTHA) and evaluate how it can be useful in seismic areas with no (or poor) seismotectonic knowledge. In such cases, when seismotectonic parameters are uncertain, it may be of interest to provide a first order idea of the tsunami hazard potential through DTHA, the implementation of DTHA being simpler than the probabilistic method (PTHA). The scenario-based (or DTHA) approach classically relies on the study of “maximum credible scenarios” (MCS). In particular, DTHA tries to explore the potential of the largest scenarios, by selecting some of the extreme ones (i.e., a recorded/reconstructed historical event) and simulating them for the target area through numerical modeling (JSCE, 2002; Lynett et al., 2004), without addressing the likelihood of occurrence of such a big event (Omira et al., 2016). With this approach, MCS is assessed through an expert opinion. The outputs of the deterministic analysis are, in general, tsunami travel time, wave height, flow depth, run-up, and current velocity maps corresponding to the chosen scenario (Omira et al., 2016).
As mentioned above, DTHA relies on a refined knowledge of the seismic sources generating the tsunamis. As a consequence, it could be hampered by the use of specific values of input parameters which may be subjective depending on the person or group carrying out the analysis (Roshan et al., 2016). A good example is the 1755 Lisbon tsunami, generated by an earthquake in the Azores Gibraltar Plate Boundary (AGPB). The Great 1755 Lisbon earthquake generated the most historically destructive tsunami near the Portugal coasts (Santos and Koshimura, 2015). Source location and contemporary effects of such tsunami are not precisely identified and several earthquake scenarios have already been published in the literature in the last decades (Johnston, 1996; Baptista et al., 1998, 2003; Zitellini et al., 1999; Gracia et al., 2003; Terrinha et al., 2003; Gutscher et al., 2006; Grandin et al., 2007; Horsburgh et al., 2008; Barkan et al., 2009; Cunha et al., 2010). All of these studies show how variable the parameters of the seismic source can be and the importance to take into account their uncertainty.
In DTHA, the classical approach to deal with uncertainties consists in performing a limited number of deterministic simulations with conservative values of the seismic sources (e.g., JSCE, 2002; Lynett et al., 2004; Allgeyer et al., 2013). However, the great M 9.0 Tohoku-Oki subduction earthquake of 2011, the largest ever recorded in Japan (Saito et al., 2011), has clearly shown the limitations of the classical approach focused on the identification of known maximum tsunamigenic sources (MCS approach). Recently, Roshan et al. (2016) improved the DTHA procedure detailed in Yanagisawa et al. (2007) in order to better evaluate the effects of the seismic source uncertainties through Monte-Carlo simulations of a limited number of seismic source parameters (the dip angle, the strike and the source location), leading to around 300 tsunami scenarios. The authors presented an improvement of the classical MCS approach by introducing uncertainties on seismic source parameters.
In this context, the objective of this work is to propose a new methodology to evaluate or qualify tsunami hazard levels associated to both specific and unknown tsunamigenic seismic sources by integrating the uncertainty related to the seismic parameters in the DTHA procedure. The main idea is to develop a meta-model, or emulator, of the tsunami numerical model, that makes it possible to perform a large number of tsunami scenarios with reduced computational time and, consequently, to intensively explore a tsunamigenic area for which geological and geophysical datasets may be limited. The constructed meta-models, when exploited with the statistical criteria classically employed in uncertainty quantification (UQ) studies (Saltelli et al., 2000, 2008; Saltelli, 2002; Iooss and Lemaître, 2015), can permit to go beyond the classical approach for DTHA and perform a better quantification of uncertainties of a large set of seismic source parameters.
In the next sections we will first present the methodology used in this study (see section “Methodology”), and then develop and validate the meta-models for AGPB related tsunamis impacting the French Atlantic Coast (see section “Application of the methodology to the French Coast”). In section “Potential application of meta-models for tsunami hazard analysis,” we (1) evaluate the impact of two realistic scenarios corresponding to potential sources of the 1755-Lisbon tsunami (when uncertainty on seismic parameters is considered), and (2) present the analysis of the tsunamigenic potential of the AGPB zone, considered for the purpose of the exercise as a poorly known tsunamigenic area. Finally, we show how the numerical results obtained with this method can be discussed in light of tsunami hazard issued by the TSUMAPS-NEAM research project available on line1.
Methodology
The proposed approach relies on three main steps, as reported in Figure 1. STEP 1 consists in the construction of a numerical model able to reproduce the tsunami heights generated by a given seismic area and impacting a target area. In STEP 2, the numerical model simulates a regular set of physical tsunamigenic scenarios (so called design data-base) that are used for the construction and the validation of an emulator (the meta-model) able to reproduce the original model results in the target zone. In STEP 3, the validated meta-models may be used for DTHA assessment and/or qualification of other THA results. The UQ performed using meta-models instead of the original model permits to assess the uncertainty related to a given tsunami scenario (see section “In the case of expert opinion: uncertainty quantification in DTHA”) and to explore intensively the tsunamigenic area with nearly zero computational time (see section “Without expert opinion: exploration of a large tsunamigenic area”).
Step 1: Tsunami Simulations
The first step consists in the construction of a tsunami numerical model of the area to explore. In this study, the tsunami numerical simulations were performed by using the tsunami code reported in Allgeyer et al. (2013) (or CEA-code), which exploits two models, one for tsunami initialization and the other one for tsunami propagation. The initial seabed deformation caused by an earthquake is generated with the Okada model (Okada, 1985) and is transmitted instantaneously to the surface of the water. This analytical model uses simplistic planar fault parameters with uniform slip, satisfying the expression of the seismic moment Mo:
where μ denotes the shear modulus, D [m] the average slip along the rupture of length L [m] and width W [m]. Then, the seismic moment magnitude “Mw” is directly computed through equation 2 (Hanks and Kanamori, 1979), as follow:
The following parameters are also required for tsunami initiation: longitude, latitude, and depth [km] of the center of the source, strike [degrees], dip [degrees], and rake [degrees]. A conceptual scheme of the input parameters for the tsunami-code and the numerical domain used in this study are reported in Figure 2. Then, the computation of the tsunami propagation is based on hydrodynamic equations, under the non-linear shallow water approximation (the Boussinesq equations as reported in Allgeyer et al., 2013). Shallow water equations are discretized using a finite-differences method in space and time (FDTD). Pressure and velocity fields are evaluated on uniform separate grids according to Arakawa’s C-grid (Arakawa, 1972). Partial derivatives are approximated using upwind finite-differences (Mader, 2004). Time integration is performed using the iterated Crank-Nicholson scheme. No viscosity terms are taken into account in our simulations. The only parameters of this model are the bathymetry (space step and depth resolution) and the time step.
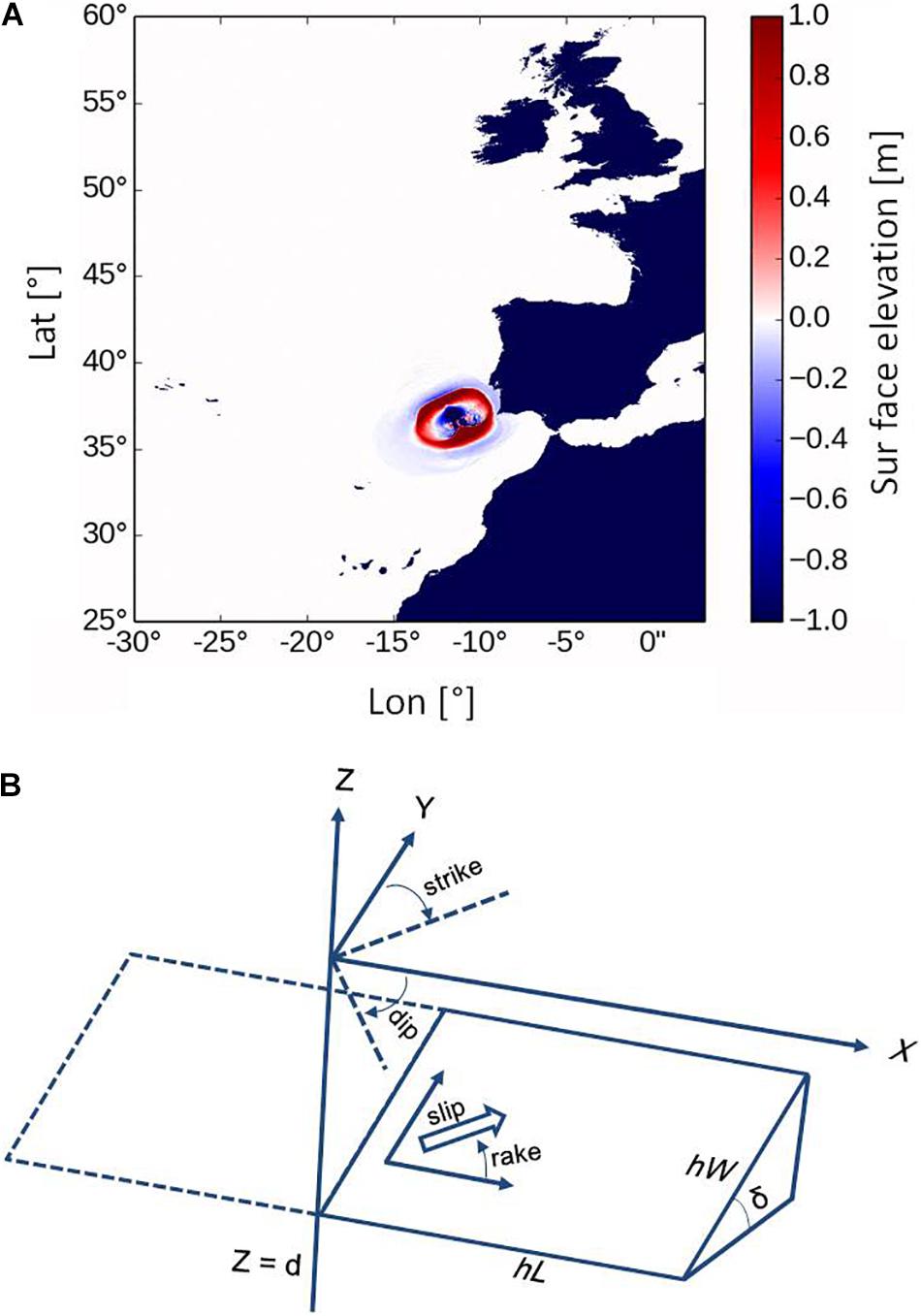
Figure 2. (A) Numerical domain used for the deterministic simulations performed in this study and (B) geometrical input parameters of the tsunami-code employed in the study. d [km] is the depth of the seismic source which is assumed to be at the middle of the fault, hL [km] is the half fault length, W [km] is the half fault width, x-y-z are three-dimensional axes.
Step 2: Meta-Model Design and Validation
Meta-Models Design
A variety of metamodels have been applied in the water resources literature (Santana-Quintero et al., 2010; Razavi et al., 2012b). Moreover, some examples of applications have already been published in the context of flood management (e.g., Yazdi and Salehi Neyshabouri, 2014; Löwe et al., 2018) and in the field of tsunamis (e.g., Sarri et al., 2012; Sraj et al., 2014; Rohmer et al., 2018). The classical steps for meta-models construction and validation are reported in various studies (i.e., Saltelli, 2002; Saltelli et al., 2008; Faivre et al., 2013), and are shortly summarized in Table 1.
For the study presented here, we rely on conditional Gaussian processes (also known as kriging (Roustant et al., 2012), derived from Danie Krige’s pioneering work in mining (Krige, 1951), later formalized within the geostatistical framework by Matheron (1963). Kriging meta-model has already shown good predictive capacities in many practical applications (see Marrel et al., 2008, for example), it became a standard meta-modeling method in operational research (Santner et al., 2003; Kleijnen, 2005) and it has performed robustly in previous water resource applications (Razavi et al., 2012b; Villa-Vialaneix et al., 2012; Löwe et al., 2018).
A general kriging model “M(x)” (which later provides an estimation of the maximum tsunami height in a given location) can be defined for x = (x1,…,xd) ∈ D ∈ Rd as the following Gaussian process “N(.)”:
Where, for simple kriging:
- m(x) = C(x)TC(X)−1Y is the conditional mean;
- s2(x) = c(x)−C(x)TC(X)−1C(x) is the conditional variance;
- C:(u,v) ∈ D2→C(u,v) ∈ R is the covariance Kernel;
- c(x) is the vector of covariance between the kriging predictions and the original model evaluations.
It must be noted that {X,Y} are the coordinates of the design simulations used for kriging parameters evaluation:
- X is a matrix containing for each design simulation (for a total of N simulations) the value of the seismic source model parameters defined in section “Step 1: tsunami simulations” (for a total of 9 inputs parameters):
- Y is a vector containing, for each gauge, the maximum water depth associated to a simulation:
More than a commonplace deterministic interpolation method (like splines of any order) this model is much more informative owing to its predicted expectation and uncertainty. The fitting procedure of this model includes the choice of a covariance model [here a tensor product of the “Matern52” function (Roustant et al., 2012)], and then the covariance parameters (e.g., range of covariance for each input variable, variance of the random process, nugget effect), could be estimated using Maximum Likelihood Estimation (standard choice we made) or Leave-One-Out minimization [known to mitigate the arbitrary covariance function choice (see Bachoc, 2013)].
It must be noted that the kriging interpolation technique requires computing and inverting the n × n covariance matrix C(X, X) between the observed values Y(X), which leads to a O(n2) complexity in space and O(n3) in time (Rullière et al., 2018). In practice, this computational burden makes Gaussian process regression difficult to use when the number of observation points is in the range [103,104] or greater, as in this study. As a consequence, we used in this article the procedure for estimating the parameters of kriging reported in Rullière et al. (2018), by using an adapted R-tool available on line2. The full details of this methodology are reported in the abovementioned paper. This approach is proven to have better theoretical properties than other aggregation methods that can be found in the literature, and permitted us to drastically reduce the computational time necessary for meta-models construction and validation.
Meta-Models Validation
A general method for meta-models validation is the K-fold cross-validation method (Friedman et al., 2001). The principle of cross-validation is to split the data into K folds of approximately equal size A1A1,…,AKAK. For k = 1 to K, a model is fitted from the data Uj≠kAk (all the data except the Ak fold) and this model is validated on the fold Ak. Given a criterion of quality L as the Mean Square Error:
the quantity used for the “evaluation” of the model is computed as follow:
where and yi are, respectively, the meta-model and the model response and n is the number of simulations in the kth sample. When K is equal to the number of simulations of the training set, the cross-validation method corresponds to the leave-one-out technique not performed in this study. The methodology employed is described in the DiceEval R-package reference-manual (Dupuy et al., 2015). In our application case, we considered K = 10.
In this study, the accuracy of the meta-model is evaluated through several statistical metrics permitting to quantify the overall quality of regression models. This includes:
- R-squared (R2), representing the squared correlation between the observed outcome values and the values predicted by the model. The higher the adjusted R2 is, the better the model is;
- Root Mean Squared Error (RMSE), which measures the average prediction error made by the model in predicting the outcome for an observation. It corresponds to the average difference between the observed known outcomes and the values predicted by the model. The lower the RMSE is, the better the model is.
Step 3: Uncertainty Quantification and Global Sensitivity Analysis
Considering the variety and the complexity of the geophysical mechanisms involved in tsunami generation, tsunami hazard assessment is generally associated with strong uncertainties (aleatory and epistemic). In PTHA, uncertainties are classically integrated in a rigorous way (Sørensen et al., 2012; Horspool et al., 2014; Selva et al., 2016) and quantified using the logic-tree approach (Horspool et al., 2014) and/or random simulations performed using the Monte-Carlo sampling of probability density functions of geological parameters (Sørensen et al., 2012; Horspool et al., 2014). An alternative and interesting approach was recently proposed by Selva et al. (2016), consisting in the use of an event tree approach and ensemble modeling (Marzocchi et al., 2015). Moreover, a new procedure was recently proposed by Molinari et al. (2016) for the quantification of uncertainties related to the construction of a tsunami data-base based on the quantification of elementary effects.
In this work we propose a classical methodology that could also be adapted to analyze tsunamigenic regions with poor (or no) information on crustal characteristics and based on the classical uncertainty study steps (Saltelli et al., 2004, 2008; Faivre et al., 2013; Iooss and Lemaître, 2015). This methodology, which was already tested in other hydraulic context in recent years (Nguyen et al., 2015; Abily et al., 2016), relies on Monte Carlo simulations for UQ steps and on GSA (Global Sensitivity Analysis) approaches for the analysis of the AGPB tsunamigenic potential, by computing Sobol indices (Sobol, 1993, 2001). These methods rely on sampling based strategies for uncertainty propagation, willing to fully map the space of possible model predictions from the various model uncertain input parameters and then, allowing to rank the significance of the input parameter uncertainty contribution to the model output variability (Baroni and Tarantola, 2014). The objectives with this approach are mostly to identify the parameter or set of parameters which significantly impact model outputs (Iooss et al., 2008; Volkova et al., 2008). GSA approaches are robust, have a wide range of applicability, and provide accurate sensitivity information for most models (Adetula and Bokov, 2012). Moreover, even if they are theoretically defined for linear mathematical systems, it was demonstrated that they are well suited to be applied with models having non-linear behavior and when interactions among parameters occur (Saint-Geours, 2012), as in the present study. For these reasons, these indices were already adopted for the analysis of bi-dimensional hydrodynamic simulations in urban areas (Abily et al., 2016) or of complex coastal models including interactions between waves, current and vegetation (Kalra et al., 2018) and they seem well suited for the present work.
For the computation of Sobol’ indices, a large variety of methodologies are available, as the so-called “extended-FAST” method (Saltelli et al., 1999), already used in previous studies by IRSN (Nguyen et al., 2015). In this study, we used the methodology proposed by Jansen et al. (1994) already implemented in the open source sensitivity-package R (Pujol et al., 2017). This method estimates first order and total Sobol’ indices for all the factors “v” at higher total cost of “v × (p + 2)” simulations (Faivre et al., 2013).
Application of the Methodology to the French Coast
The French Atlantic coast is subjected to two main seismogenic sources that could generate tsunamis, one in the lesser Indies, and a second one from the AGPB. In this application we only consider the AGPB and we only compute water heights offshore for four locations (Figure 3), ignoring the necessary refinements for propagation to the coast. Because in this study case the sources are far from the considered gauges, we propose a very simplified approach to characterize the source region. In the following we first present how the meta-model was constructed and validated through a series of statistical tests in comparison with published data from Allgeyer et al. (2013).
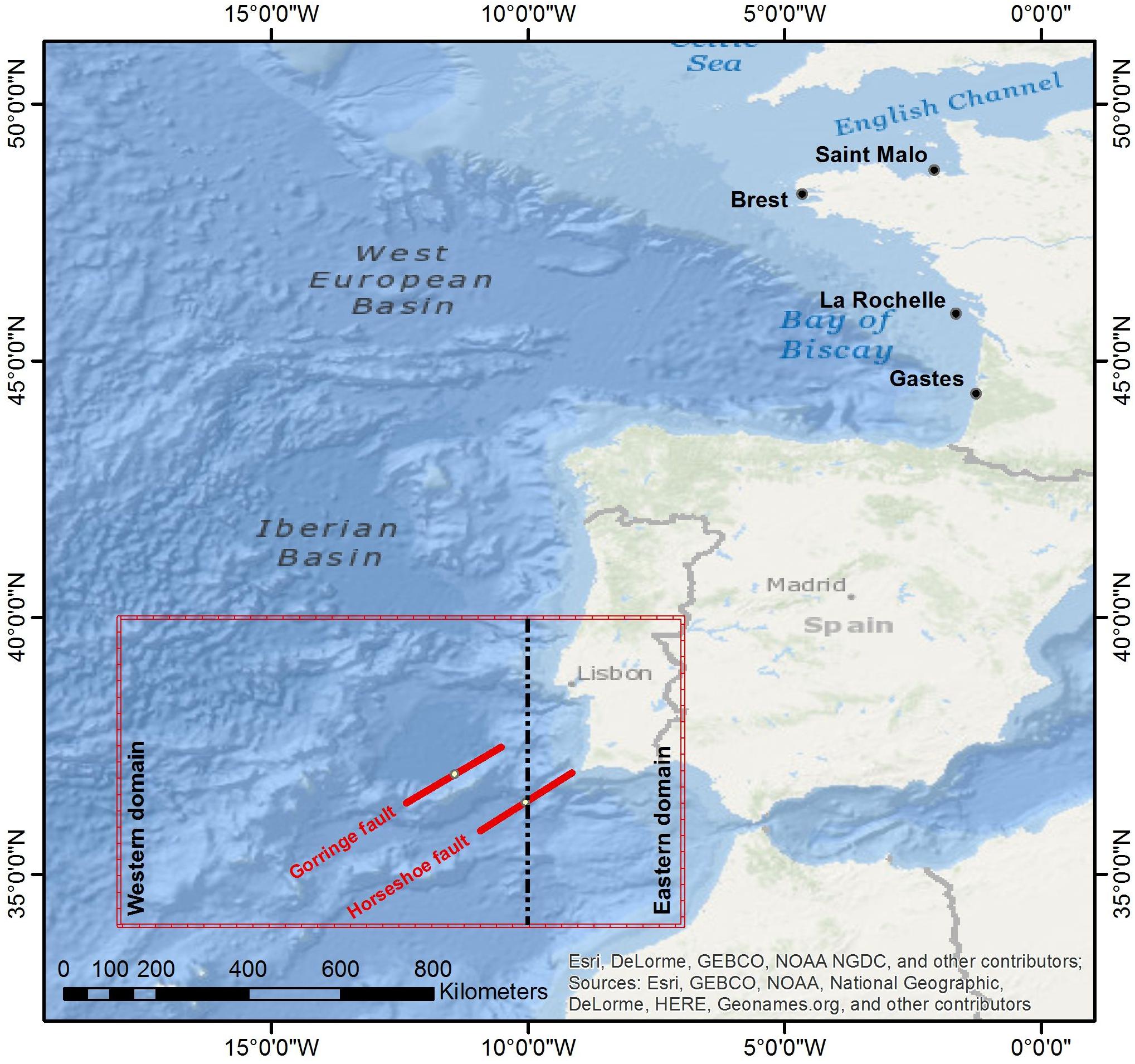
Figure 3. Bathymetry covering the computational domain. Red cross hatching area shows location of tsunamigenic sources used for meta-models construction. The points represent the gauge locations selected for tsunami database construction along the French Atlantic Coast. Gorringe fault and Horseshoe fault are special structures at the boundary between the Eastern domain (on the right) and the Western domain (on the left).
Numerical Tool and Design Data-Base
All the simulations were performed on the same bathymetric grid with a space resolution of 2’ (∼3.6 km). The numerical model was not directly validated by the comparison with similar simulations from literature. In fact, considering the rough bathymetrical grid resolution, the developed numerical model is not adapted to the estimation of the tsunamis run-up and the inundation areas and it can’t be used for a real assessment of the tsunami hazard along the French Atlantic Coast. However, this work being methodological, we consider that the numerical results are consistent with the objectives of the study. Moreover, the tsunami-code was largely validated through extensive benchmarks in the framework of the TANDEM research project (Violeau et al., 2016) by ensuring its ability to reproduce tsunamis generation and propagation. As a consequence, the order of magnitude of the tsunami heights computed in this study should be realistic and adapted to the test of the methodology.
In order to perform the numerical simulations needed for the meta-model construction and validation (see section “Step 2: meta-model design and validation”), the CEA-code was coupled with the IRSN Promethee bench. Promethee is an environment for parametric computation that allows carrying out UQ studies, when coupled (or warped) to a code. This software is freely distributed by IRSN3 and allows the parameterization with any numerical code and is optimized for intensive computing. Promethee was first linked to the numerical code by means of a set of software links (similar to bash scripts). In this way, numerical simulations were directly lunched by the IRSN environment. Then, the statistical analysis, such as the Monte-Carlo simulations used for the meta-model construction (see section “Meta-models design”) and UQ (see section “Analysis of the Impact of Tsunamis on a Target Area through UQ”) or the Sobol indices computation (see section “Global Analysis of Seismic Source Influence on the Target Area”) were also driven by Promethee, which integrates R statistical computing environment by permitting this kind of analysis (R Core Team, 2016).
In this methodological work, we considered a widened AGPB tsunamigenic area and we chose to explore as largely as possible the potential tsunami height along the French Atlantic Coast generated by earthquakes from 34° to 40°N and from 18 to 7°W, encompassing to the East the southern part of Portugal down to Morocco, and reaching the oceanic sea-floor west of the Madeira-Tore rise (as reported in Figure 3). Because the design database is a learning base for meta-modeling, the range of variation of the input parameters (column “Design database” in Table 2) need to be large in order to cover a wide range of earthquake scenarios. Thus, if correctly estimated, meta-models will be able to reproduce the model behavior for a large range of variations of the seismic inputs parameters, including physical scenarios from geological studies of the zone.
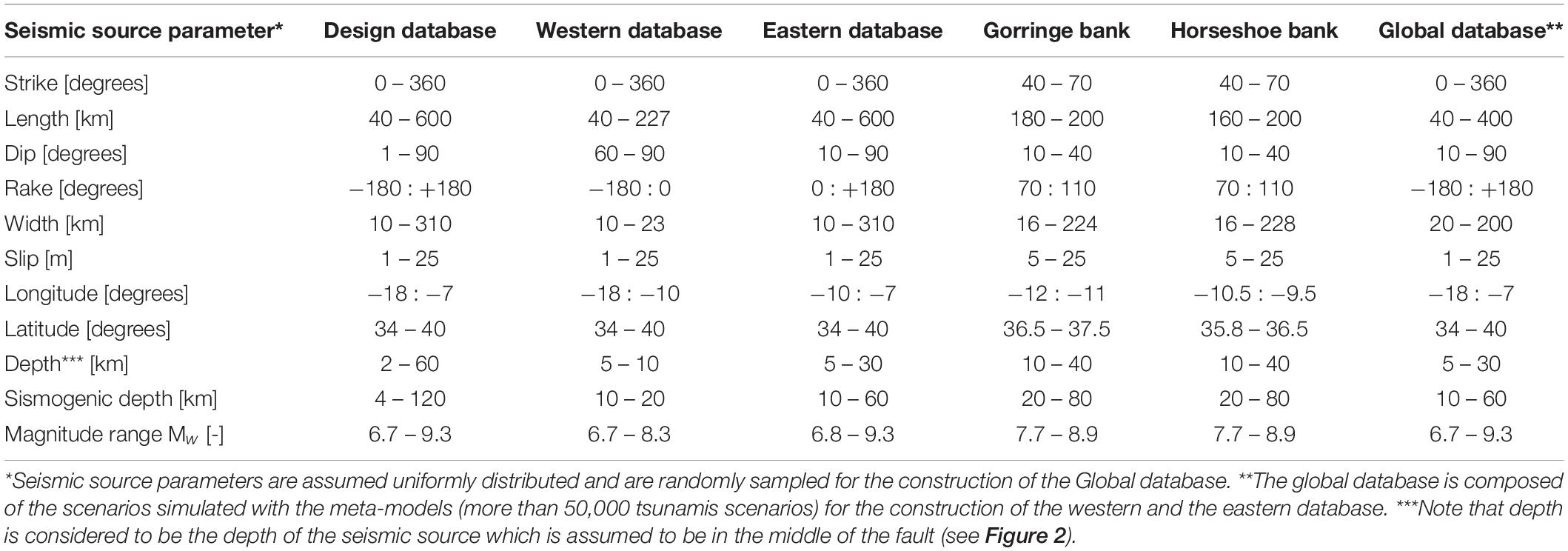
Table 2. Summary of the variation range of the seismic source input parameters for the design, the western, the eastern database and for the tsunami scenarios associated to the Gorringe bank and the Horseshoe bank (hypothesis from Duarte et al., 2013; Grevemeyer et al., 2017).
In order to build the design database, fault parameters as defined in section “Step 1: tsunami simulations” and Table 2 were sampled randomly and independently with the Monte-Carlo method and supposing uniform distributions. The uniform distribution was chosen in order to build meta-models able to reproduce tsunami heights generated by various tsunamigenic sources with the same accuracy. The resulting earthquake magnitudes are computed using the sampled parameters with equation 2. The shear modulus chosen for the magnitude estimation is a constant value assumed to be equal to 30 GPA. This design database is a matrix which associates to a given combination of fault parameters estimates the maximum simulated water height at each point of the numerical grid and also at four selected locations along the French Atlantic Coast called gauges (Table 3 and Figure 3), namely, from North to South, “Saint-Malo,” “Brest,” “La Rochelle” and “Gastes.” The maximum tsunami water height is the relevant parameter when estimating tsunami hazard.
Meta-Models Construction and Validation
Meta-models were constructed using the NestedKriging procedure described in Rullière et al. (2018), as reported in section “Step 2: meta-model design and validation.” The design database contains 5839 scenarios used for the meta-model construction and validation. The water height characteristics associated to these scenarios are reported in Table 4. Each meta-model is a function able to compute the maximum tsunami water height at the gauge location for a given set of seismic source parameters (strike, length, dip, rake, width, slip, longitude, latitude and depth). Obviously, the input parameters should be included in the parameter range used for the meta-model construction and reported in Table 2.
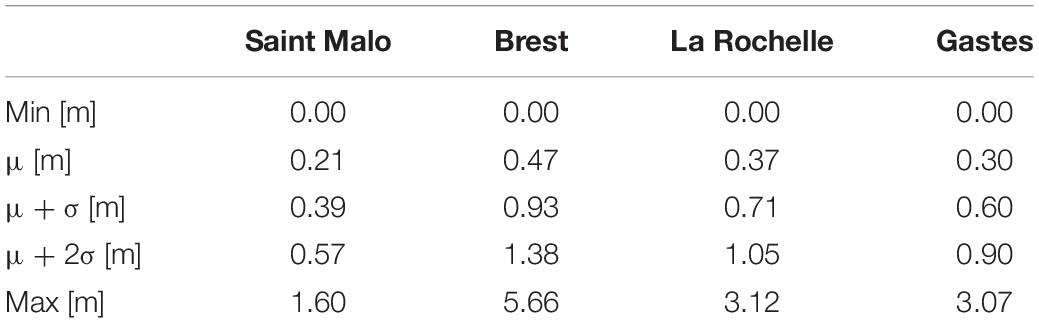
Table 4. Maximum water height associated with the design database; μ, σ and Max correspond to the mean, standard-deviation and maximum modeled values.
For meta-model validation, the design data-base is split into K folds (K = 10 in this study, for a total of 584 simulations) of approximately equal size and a model is fitted from the data and validated on the fold Ak. K-fold cross validation is used for two main purposes: (i) to tune hyper parameters of the meta-model and (ii) to better evaluate the prediction accuracy of the meta-model. In both of these cases, the choice of k should permit to ensure that the training and testing sets are drawn from the same distribution. Especially, both sets should contain sufficient variation such that the underlining distribution is represented. From a practical point of view, the value of K is typically chosen as a good compromise between the computational times needed for the analysis and its reliability (Hastie et al., 2009). Indeed, there is not, to our knowledge, a well-established methodology allowing identifying the optimum number of folds necessary for cross-validation. In this methodological work, we considered K = 10 as a robust value with regards to the objectives of the study.
Results in terms of the criteria of quality L are reported in Table 5 and Figure 4. It appears that the mean computed values from cross validation are satisfying when considering the large range of parameters variations of the design data-base and the methodological purpose of the study. Indeed, except for the “Gastes” gauge, the mean R2 is higher than 80%, which is satisfying according to Marrel et al. (2009) and Storlie et al. (2009), and the mean RMSE of few centimeters, indicating that the kriging meta-model is a good emulator choice for reproducing the CEA-tsunami code behavior.
However, it must be underlined that, out of four gauges, results obtained for “Gastes” gauge are not satisfying, at least in terms of statistical performance. In fact, the large variation of the RMSE parameter (from 0.03 to 0.25 m) and the low values of R2 (varying from 0.6 to 0.9) reported in Figure 4 suggest that further numerical runs should be necessary to improve the accuracy of kriging.
It must be noted that the methodology used for the meta-model validation is a “state of the art” methodology permitting to focus on the ability of the meta-model to reproduce the mean model response and to estimate the model variability (represented by the variance). Even if this is common in literature, for hazard studies it would also be of interest to focus in the future on other criteria that account for the behavior in the tails of the distributions of the simulated values (extreme values).
Validation With Results From Allgeyer et al. (2013)
We perform an additional test in order to evaluate the ability of our meta-models to reproduce state of the art tsunami scenarios generated by the AGPB and impacting the French coast. With this aim, we compare our meta-models results with tsunami height simulated at the same location by Allgeyer et al. (2013). This comparison is of interest in order to confirm the ability of the constructed meta-models to reproduce the order of magnitude of the modeled tsunami height at a given location.
In this study, the authors analyzed the impact of a Lisbon-like tsunami on the French Atlantic Coast through numerical modeling. The authors focused on the simulated maximum tsunami water height in the North Atlantic associated to three different sources for the 1755 events derived from Johnston (1996), Baptista et al. (2003), and Gutscher et al. (2006), for a total of five tsunami scenarios (see Table 6). The same scenarios were simulated with the constructed meta-models for the four French Atlantic Gauges and with the CEA-tsunami model with a more refined grid, spacing of 1’. Even if meta-models prediction slightly overestimate the modeled tsunami height (see Figure 5 and Table 7), these results indicate a good agreement between the meta-modeled and the modeled water height, for the “Saint Malo,” “Brest” and “La Rochelle” gauges. On the contrary, these results confirm that further numerical runs should be necessary to improve the accuracy of kriging for “Gastes” gauge, which largely overestimate the tsunami heights of the original model (Figure 5).

Table 6. Seismic sources simulated in Allgeyer et al. (2013) for the 1755 Lisbon-tsunami.
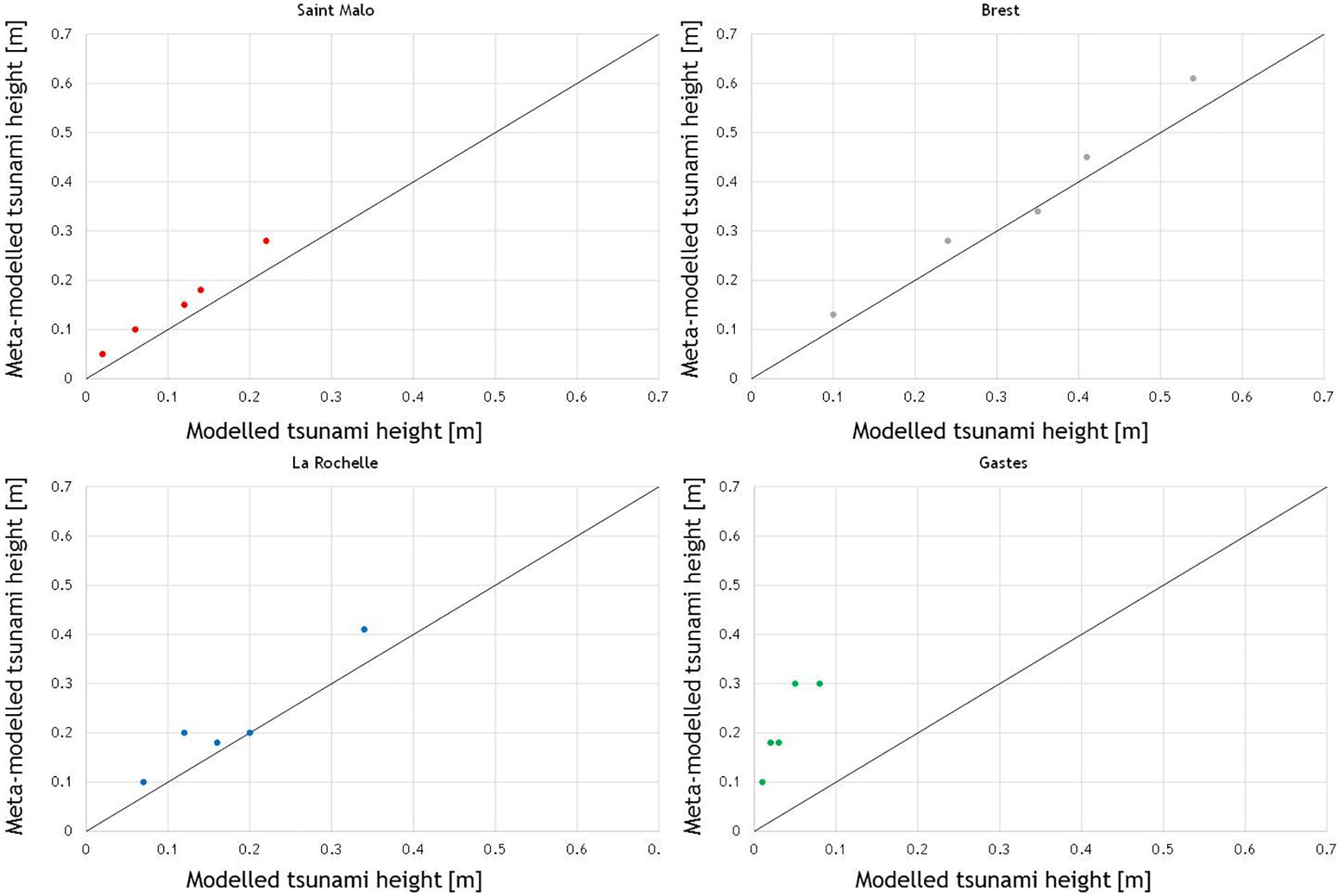
Figure 5. Comparison between the meta-modeled ant the modeled tsunami height issued by the tsunami scenarios reported in Allgeyer et al. (2013). The black line indicates the perfect match (y = y–).

Table 7. Maximum tsunamis height simulated in Allgeyer et al. (2013) for the 1755 Lisbon-tsunami and computed with meta-models.
Considering the methodological purpose of the study, these results are satisfying. However, for a practical application, a more extended set of physical scenarios should be of interest for the validation of the tsunami height predicted by meta-models.
Potential Application of Meta-Models for Tsunami Hazard Analysis
The objective of this section is to present how meta-models can be employed for (i) the integration of uncertainties of “known” tsunami scenarios (see section “In the case of expert opinion: uncertainty quantification in DTHA”) and (ii) the analysis of the tsunamigenic potential of a poorly known tsunamigenic area (see section “Without expert opinion: exploration of a large tsunamigenic area”). Finally, the obtained results are discussed in light of the tsunami hazard issued by a probabilistic analysis (see section “Qualifying these approaches with respect to Probabilistic Tsunami Hazard Assessment”).
We recall here that this is not an operational tsunami hazard assessment and that all the presented results are purely methodological.
In the Case of Expert Opinion: Uncertainty Quantification in DTHA
DTHA is classically assessed by means of considering particular source scenarios (usually maximum credible scenario) and the associated maximum tsunami height is generally retained as hazard level (MCS). A more sophisticated method was recently proposed by Roshan et al. (2016). The authors tested around 300 tsunamis scenarios in a [8 – 9.5] magnitude range associated with various faults potentially impacting the Indian coast. Finally, the authors suggested that an appropriate water level for hazard assessment (e.g., mean value or mean plus sigma value) should be retained. They proposed the mean value of the simulated water heights, as test, by considering that this value may need to be revisited in the future.
In the case of the tsunamis hazard associated with the AGPB, the 1755 Lisbon tsunami is the classical reference scenario. In order to illustrate how to integrate the evaluation of uncertainties in a MCS approach, we focus on two specific and nearly deterministic scenarios considered as likely sources generating the Lisbon 1755 tsunami, namely the Gorringe and Horseshoe structures (Buforn et al., 1988; Stich et al., 2007; Cunha et al., 2012; Duarte et al., 2013; Grevemeyer et al., 2017). Both structures were modeled taking into account available maps (Cunha et al., 2012; Duarte et al., 2013) and fault parameters (Stich et al., 2007; Grevemeyer et al., 2017) summarized in Table 2. For the computation of the tsunami heights associated with these scenarios, fault parameters are considered uniformly distributed and are randomly sampled in their range of variation (Table 2), for a total of nearly 10,000 tsunami scenarios. The magnitude range associated with the sources of these tsunami scenarios varies from 7.7 to 8.9, which is coherent with the range of estimated magnitudes for the 1755 earthquake (Johnston, 1996; Gutscher et al., 2006). The convergence of statistics (defined as the evolution of the mean modeled tsunami height and of the mobile standard deviation) is largely achieved, indicating that the number of tsunami scenarios is sufficient to represent the expected variability of tsunamis height.
The distribution of tsunami heights resulting from the two Gorringe and Horseshoe sources are reported in Figure 6 for each gauge and a summary of the numerical values in Table 8. It can be observed that the tsunami heights generated by the Horseshoe sources are globally lower than those generated by the Gorringe sources. At first glance, this result does not seem surprising considering the closer proximity of the French gauges to the Gorringe bank. It can also be observed that both scenarios are affected by strong uncertainties. Indeed, the ratio between the maximum and the mean tsunami height is very large and it can vary from 2 to 5, according to the chosen gauges. Moreover, the variability of the modeled values around the mean value can be higher than 1.0 m for the Gorringe scenarios and it is always higher than 0.24 m (for Saint Malo gauge).
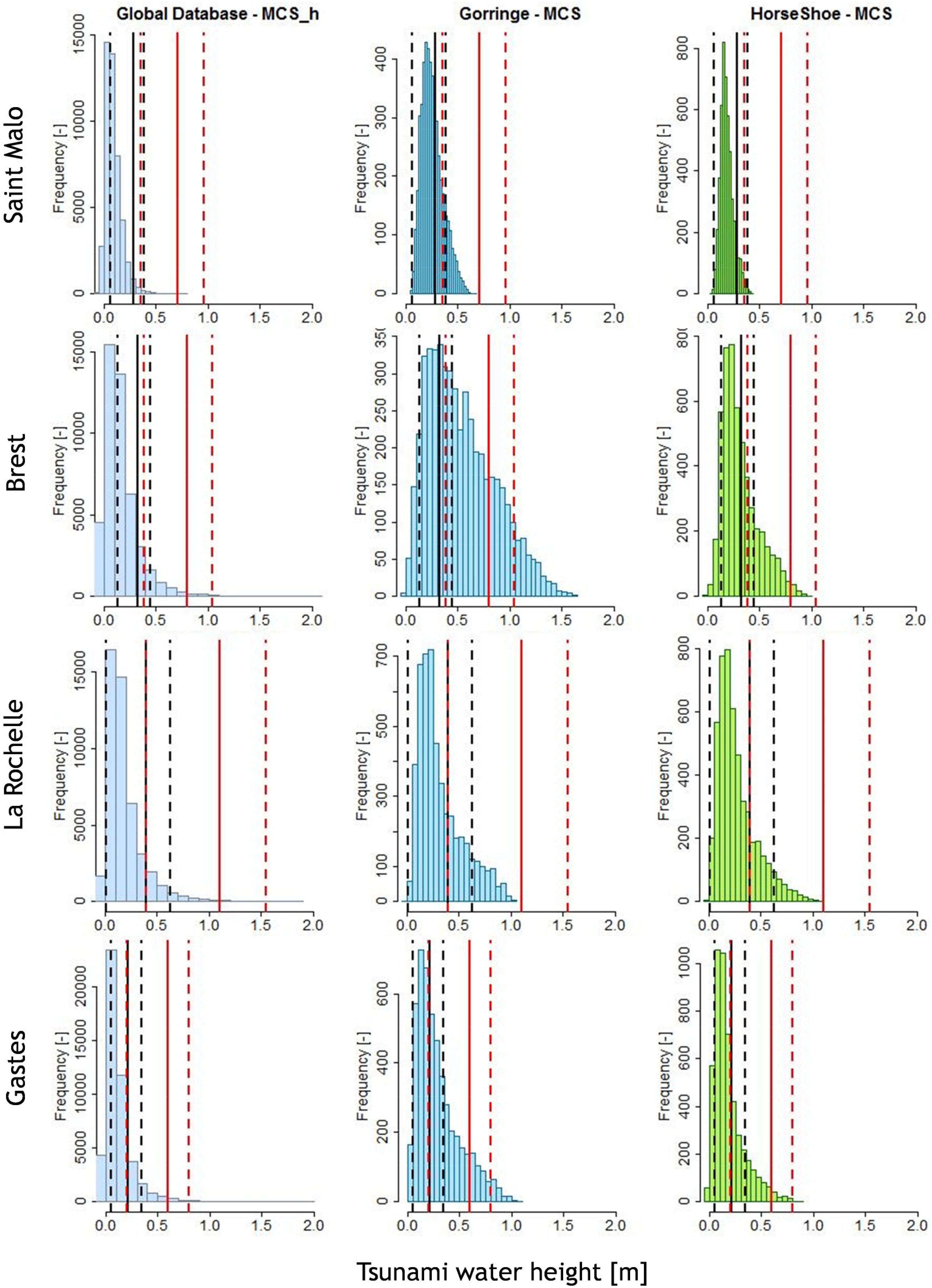
Figure 6. Tsunami height frequency distribution associated to the global data base (gray-blue), the Gorringe bank (blue) and the Horseshoe bank (green). The black and red lines correspond, respectively, to the mean tsunami height associated to a return period of 1 000 and 10 000 years issued by TSUMAPS-NEAM research project. Dotted lines represent the 2th and 98th percentile of the hazard curves.

Table 8. Maximum tsunami height distributions associated with the global database (GD), HorseShoe (HS), and Gorringe (GR) scenarios; μm, σm and Maxm correspond to the mean, standard-deviation and maximum meta-modeled values.
If we assume that the MCS approach is to take into account the worst possible scenarios, a hazard level corresponding to the maximum modeled water height could be retained for each gauge. However, if we consider the excursion of our numerical results this hazard level could be too high. This is probably why, as previously mentioned, other authors proposed to set a mean level as representative of the tsunami hazard (Roshan et al., 2016). In general, a first methodological conclusion is that the impact of the uncertainty on the source parameters on water height can be high and it should be taken into account by decision makers.
Without Expert Opinion: Exploration of a Large Tsunamigenic Area
Let’s now assume, for a methodological purpose, that AGPB is a poorly known seismic area and that the objective of the study are to (i) evaluate the “possible” impact of tsunamis generated by this area on a target location (in this example, the French gauges) and (ii) to better understand the relative influence of the source parameters on these tsunamis. This latter analysis should permit to better guide the geological investigations in the area.
With this objective, UQ and GSA appear as two useful and complementary tools, respectively to answer to objectives (i) and (ii).
Analysis of the Impact of Tsunamis on a Target Area Through UQ
We propose to evaluate the tsunami hazard level, called here MCS_h, by exploring meta-models results based on a very wide range of tsunamigenic scenarios, beyond those proposed so far in the literature as we suppose that this tsunamigenic area is not well known. As a consequence, we accept that through this approach we explore the effects of both likely and very unlikely scenarios relative to the MCS (the “MCS_h” scenarios) that could potentially arise in a context of poor seismotectonic knowledge. As in the previous case, depending on the specific hazard target (civil or industrial facilities), and its location with respect to the source zone, the end-user of this methodology needs to decide which level of water height to choose from the obtained distribution. In this exercise, we will consider the maximum simulated water height for MCS_h.
We build a database (called global database) of tsunami scenario generated by the considered AGPB area at four French Atlantic Gauges, with the aim to cover a wider range of tsunamigenic scenarios (Table 2). For the purpose of this methodological paper, the modeled area, which encompasses different seismotectonic domains, was split in a very simplistic way into two main seismic source zones (Figure 3): a western domain where normal to transtensive earthquakes occur within a thin crust and an eastern domain where reverse to transpressive earthquakes mainly occur on a thicker crust. We considered the Western domain west of the 10°W meridian and the Eastern domain east of this meridian, coinciding roughly with the base of the continental slope facing the Portuguese coastline. The main fault characteristics considered to build the tsunami data-base in both eastern and western domains are reported in Table 2, following data contained in Buforn et al. (1988), Molinari and Morelli (2011), Cunha et al. (2012).
The considered seismogenic thickness takes into account the depth of the observed seismicity as well as the fact that part of the upper mantle can potentially be mobilized during major earthquakes: the western domain seismogenic crust is considered to be up to 20 km deep (after Baptista et al., 2017), and up to 60 km for the eastern domain (after Silva et al., 2017). The fault parameters are considered uniformly distributed and are randomly sampled in their range of variation. We finally filtered the resulting database according to an aspect-ratio criterion, allowing the ratio between the length and the width of the faults not to exceed the value of 10, which corresponds to an upper bound of what is observed in nature (Mc Calpin, 2009). The final global database contains nearly 50,000 tsunami scenarios, resulting in earthquake magnitudes varying from 6.7 to 9.3 (Table 2), depending on the explored earthquake source characteristics and calculated from Eq 1. This range of magnitudes is consistent with the magnitude range of the design database.
The tsunami water heights distributions associated to the four gauges are reported in Table 8 and Figure 6. As for the previous paragraph, these results show a very large variability in tsunamis height, which is not surprising considering the large range of variation of the source parameters. However, if we compare these results with those obtained in the previous section, we can also observe that Gorringe and Horseshoe banks are among the major contributors to tsunami hazard along the French Atlantic Coast, generated by seismic sources in the AGPB. Indeed, Figure 6 clearly shows that even if some isolated tsunami scenarios can generate a hazard level higher than the Gorringe Scenarios, globally, these sources are representative of the higher tsunamis from the global data-base.
Global Analysis of Seismic Source Influence on the Target Area
A sensitivity analysis is hereafter performed in order to decipher the relative influence of the seismic source parameters. Homma and Saltelli (1996) introduced the total sensitivity index which measures the influence of a variable jointly with all its interactions. If the total sensitivity index of a variable is zero, this variable can be removed because neither the variable nor its interactions at any order have an influence on the results. This statistical index (called Sobol index “ST” in this paper) is here of particular interest in order to highlight the earthquake source parameters that mostly control the tsunamis height at each tested gauge. In Figure 7, we reported the total Sobol index for the four meta-models of the French Atlantic Gauges computed with the methodology proposed by Jansen et al. (1994) using the sensitivity-package R (Pujol et al., 2017). The accuracy of Sobol indices performed with Jansen’s method depends on the number of model evaluations. For instance, in this study, we performed nearly 20 000 simulations using meta-models. Results show that the slip parameter is globally the most-influencing parameter for all the French Atlantic Gauges meta-models. Concretely, nearly 50% of the variance of the tsunami water height (the uncertainty) could be reduced by a better knowledge of this parameter. This result is quite obvious considering that the fault-slip directly conditions the ocean floor deformation and hence the tsunami amplitude.
However, this analysis also suggests that the most influencing parameters for the four gauges are slightly different, depending on their location. One can differentiate results obtained for the southern gauges (i.e., La Rochelle and Gastes) from those obtained at northern gauges (i.e., Saint Malo and Brest):
• For the southern gauges, width is the second most relevant parameter, especially at La Rochelle where it is almost as important as the slip parameter. Other important parameters are length and rake, suggesting that for these gauges, fault source parameters in terms of magnitudes (depending on width, slip and length according to equations 1 and 2) and kinematics are the most important in generating hazard;
• For the northern gauges, apart from slip, strike, width and rake are also important, but slightly less than the longitude parameter. This means that the location of the source is here of major importance. A possible physical reason could be associated to the lack of the natural barrier composed by the north of Spain and Portugal which protects southern gauges from AGPB related tsunamis compared to northern gauges. As a consequence, the northern gauges should be more exposed to hazard in comparison to southern gauges, located in the shadow of Portugal and Spain.
Qualifying These Approaches With Respect to Probabilistic Tsunami Hazard Assessment
Let’s imagine now that for the target area a reference hazard level is provided through a probabilistic study and that we aim to qualify the robustness of this hazard level with regards to possible tsunamis height issued by expert scenarios (MCS, see section “In the case of expert opinion: uncertainty quantification in DTHA”) or by the exploration of poorly known tsunamigenic area (MCS_h, see section “Without expert opinion: exploration of a large tsunamigenic area”). The idea is here to compare the results from a probabilistic analysis with the deterministic approach proposed in this study, which should permit to completely cover the uncertainty related to seismic source parameters.
With this aim, we compare our results to the tsunami hazard level (Figure 6) issued from TSUMAPS-NEAM research project4 in four points located near to the gauges used in this study. TSUMAPS-NEAM results are provided in terms of Maximum Inundation Height (MIH), which is the estimated maximum flow depth from the envelope of the tsunami wave at all times, as reported in the NEAMTHM18 documentation (Basili et al., 2018, 2019). TSUMAPS-NEAM has developed a long-term PTHA for earthquake-induced tsunamis for the coastlines of the NEAM region (NE Atlantic, the Mediterranean, and connected seas). TSUMAPS-NEAM results largely relied on inputs from the EU FP7 project ASTARTE, of the GAR15 (global risk quantification under the HFA), and national PTHAs like those of USA and Italy. One of the major results of the project is the Interactive Hazard Curve Tool5 which represents online hazard maps for different hazard probability/average return periods (mean, median, 2nd, 16th, 84th, and 98th percentiles hazard curves). In TSUMAPS-NEAM, tsunamis are computed using an approach which relies on the use of unit sources to reproduce tsunami scenarios (e.g., Molinari et al., 2016; Baptista et al., 2017). The main differences with our methodology are that these methods rely on a decomposition of the tsunami waves form recorded in a target area with simplified methods (e.g., the Green’s law), and on the main assumption that the non-linear terms of tsunami propagation are negligible. The scope of these studies is to develop a fast emulator, permitting to replace a tsunami model at some selected locations, along the same philosophy of the meta-models developed here. As a consequence, according to the author’s purpose, MIH is suitable for a regional, initial screening assessment type such as the objective of TSUMAPS-NEAM. These results must be considered only as input reference study for further site-specific assessment. Considering the methodological objective of this work, it is of interest to compare our results with this previous work.
For this exercise, we selected two targets return periods of 1,000 and 10,000 years from the hazard curves of TSUMAPS-NEAM. To take into account the uncertainty related to these results, we consider the mean, the 2th and the 98th percentile hazard curves. These targets, and the associated uncertainty, are typical of classical risk analysis and permit to compare the distributions issued by PTHA with our results. For instance, after the Fukushima accident, a return period of 10,000 years is considered as the target for hazard assessment in the field of nuclear safety (WENRA, 2015).
Figure 6 illustrates the comparison of our results (MCS and MCS_h) with those selected from the TSUMAPS-NEAM project. One can notice that:
• The tsunami intensity associated to a target mean return period of 1,000 years does not cover the tsunamis intensities related to expert scenarios (Gorringe and Horseshoe banks) or to MCS_h scenarios (all the likely and unlikely scenarios from AGPB);
• The tsunami intensity associated to a target mean return period of 10,000 years covers all the tsunami intensities related to seismic sources from the AGPB, including very unlikely tsunami scenarios (MCS_h);
• A target hazard level of 10,000 years covers the tsunamis related to Horseshoe banks;
• A target hazard level of 10,000 years covers the tsunamis related to Gorringe bank, with the exception of “Brest” gauge and to some extent that of “Gastes”.
Even if these results are purely methodological, it appears that a target hazard level of 10,000 years issued from TSUMAP-NEAM project covers all the variability related to seismic sources from the AGPB, even by exploring very unlikely tsunami scenarios (MCS_h) and most of the variability related to Gorringe and Horseshoe scenarios. However, concerning the “Brest” gauge, the methodology indicates a high sensitivity of this coastal area to the characteristics of the Gorringe seismic sources.
Discussion and Conclusion
The research work presented in this paper was performed in order to test the interest of UQ for the analysis and the qualification of the DTHA generated by earthquakes. We propose a new methodology, permitting the assessment of the uncertainty related to tsunami hazard through the analysis of a wide range of tsunami scenarios at a given location. This concept should permit to define a hazard level which goes beyond the definition of the Maximum Credible Scenario (MCS) classically reported in the literature (JSCE, 2002; Lynett et al., 2004) and employed for DTHA and permit to integrate uncertainty in hazard quantification. Moreover, tsunami hazard evaluated through UQ can also permit the exploration of tsunamigenic potential of a poorly known seismic zone, as well as a qualification of PTHA.
From a methodological point of view, meta-models appear as a very efficient and viable solution to the problem of generating many computationally expensive tsunamis simulations. As reported in Behrens and Dias (2015), the statistical emulator gives perfect predictions at the input points that are used in its generation process (it interpolates). Statistical emulation does not accelerate the model itself. The significant advantage of using the emulator is that it is much less computationally demanding to be evaluated and, therefore, it can be employed to carry out fast predictions and inexpensive analyses, such as sensitivity and uncertainty analyses reported in this study. Even if it is the first time, to our knowledge, that meta-models are proposed for THA, they have been already employed for tsunami modeling (Sarri et al., 2012; Sraj et al., 2014; Rohmer et al., 2018). As reported in section “Qualifying these approaches with respect to Probabilistic Tsunami Hazard Assessment,” in the field of tsunami hazard, an alternative approach relies on the use of unit sources to reproduce tsunami scenarios (e.g., Molinari et al., 2016; Baptista et al., 2017). Results from these studies are satisfactory for most of the practical applications such as probabilistic tsunami hazard analysis, tsunami source inversion and tsunami warning systems. However, we consider that our methodology can be proposed as an alternative to these studies as it does not rely on any assumption on tsunami propagation and can be applied everywhere in the model domain, without any limitation. For instance, meta-models could be constructed and validated from detailed simulations in a given target area, including complex physical phenomena as overtopping, run-up, and breaking. However, we want to stress that meta-model construction is time consuming and needs an appropriate design data-base, which requires a good compromise between the range of variations of the inputs and the number of simulations.
Concerning our application at four selected location, with the exception of “Gastes” gauge, both the statistical tests we performed and the comparison with result from Allgeyer et al. (2013) suggest that the meta-models are able to reproduce the tsunamis generated by the AGPB. Thus, the constructed meta-models could be employed in a further study to roughly evaluate the impact of other seismic scenarios from the AGPB and impacting the French Atlantic Coast offshore. In order to increase the accuracy of “Gastes” meta-model, the design data-base should be however enriched.
Results from GSA suggests that beyond earthquake magnitudes, the position and the orientation of the faults are influent parameters, at least for the sites considered along the northern French Atlantic Coast. Indeed, sensitivity analysis can be a useful tool not only to parametrize the design data-base but also to orient future geological surveys in a specific area.
In conclusion, MCS_h in the AGPB study region as defined in our study could be implicitly associated to a mean return period of 10,000, when considering the strong hypothesis we did on source characteristics. In this sense, results from UQ shows that a hazard level of 10,000 years issued from TSUMAP-NEAM project covers a very wide range of uncertainties related to characterization of seismic sources from the AGPB, even by exploring very unlikely tsunami scenarios (MCS_h) and most of the uncertainties related to Gorringe and Horseshoe banks expert opinion scenarios. However, concerning the “Brest” gauge, even a target hazard level of 10,000 years does not seem appropriate to cover all the uncertainties related to the Gorringe source, indicating a high sensitivity of this coastal area to the characteristics and the kinematics of the Gorringe seismic source.
Perspectives
For an operational far-field DTHA, it should be necessary, at first, to improve the actual numerical model in order to better represent the tsunamis run-up and the inundated areas, with a more accurate bathymetric grid. For an operational application to locations closer to the source zones (i.e., Portugal or Spain, Morocco), it may be challenging to gather the necessary details for a proper establishment of both models and meta-models. However this point deserves further attention because uncertainties will always remain. Thus even in such cases, where more refined databases need to be established, the proposed approach should be of interest to at least efficiently explore in a more exhaustive way the uncertainties (e.g., different probability distribution of inputs parameters, non-uniform slip distribution, fault geometries) in the source parameters linked to the MCS approach. Moreover, it must be noted that for this methodological study we chose a very simple but widely used source description (planar faults with homogeneous slip). However, more robust simulations should take into account a more complex source representation (e.g., 3D geometry, heterogeneous slip distribution), as recently suggested by Davies and Griffin (2019). Indeed, meta-models may also be useful to account for these parameters, allowing for many simulations.
From a methodological point of view, it could be of interest to compare our methodology with the alternative approaches using unit sources to reproduce tsunami scenarios (e.g., Molinari et al., 2016; Baptista et al., 2017), in terms of simulations needed, computational time, accuracy, for instance.
Finally, from a numerical point of view, it would be of interest to (1) introduce recurrence models for each tsunamigenic source to go toward PTHA calculations, and (2 introduce meta-models in systems developed for tsunami early warning, considering the low computational time inherent to this statistical tool.
Data Availability Statement
The datasets generated for this study are available on request to the Institute for Radiological Protection and Nuclear Safety (IRSN).
Author Contributions
VB: development of the methodology, meta-models construction and validation, and sensitivity and hazard analysis. HJ and OS: seismic sources definition, and seismological and hazard analysis. EA: tsunamis modeling (model construction and simulations of the design data-base). LB and C-MD: technical discussions. HH: tsunamis modeling and technical discussions. VB, HJ, and OS wrote the manuscript.
Funding
This research was funded by the French National Agency for Research (ANR).
Conflict of Interest
The authors declare that the research was conducted in the absence of any commercial or financial relationships that could be construed as a potential conflict of interest.
Acknowledgments
This study was performed through a cooperative research between the Institute for Radiological Protection and Nuclear Safety (IRSN) and the CEA, in the framework of the French ANR-TANDEM research project. We want to thank ENS Paris and particularly the Geology Laboratory for their technical support on this project. We acknowledge especially Eric Calais for the availability of a part of the team’s cluster and Pierpaolo Dubernet for the help he offered to install Promethee. The present work also benefited from the inputs of Msc. Hend Jebali and Dr. Audrey Gailler and from the valuable technical assistance of Dr. Maria Lancieri, Dr. Yann Richet, and Miss Ludmila Provost.
Abbreviations
μ, shear modulus; [N(m(x),s2(x))], Gaussian process of mean “;m(x) ” and variance ” s2(x) ”[ M(x) ], kriging surrogate {X,Y}, are the coordinates of the design simulations used for kriging parameters evaluation; C(.), covariance kernel; CEA, commissariat à l’énergie atomique et aux énergies alternatives; D [m], average slip along the rupture surface; DTHA, deterministic tsunami hazard assessment; GSA, global sensitivity analysis; L [m], length of the rupture surface; MCS, maximum credible scenario; MCS_h, tsunami hazard level issued by an exploration of a very wide range of tsunamigenic scenarios; Mo, seismic moment; Mw, seismic moment magnitude; MSE, mean squared error; PTHA, probabilistic tsunami hazard assessment; R2, squared correlation coefficient; RMSE, root mean squared error; UQ, uncertainty quantifications; W [m], width of the rupture surface.
Footnotes
- ^ http://ai2lab.org/tsumapsneam/interactive-hazard-curve-tool/
- ^ https://github.com/drulliere/nestedKriging
- ^ http://promethee.irsn.org/doku.php
- ^ http://www.tsumaps-neam.eu/
- ^ http://ai2lab.org/tsumapsneam/interactive-hazard-curve-tool/
References
Abily, M., Bertrand, N., Delestre, O., Gourbesville, P., and Duluc, C.-M. (2016). Spatial Global sensitivity analysis of high resolution classified topographic data use in 2d urban flood modelling. Environ. Model. Softw. 77, 183–195. doi: 10.1016/j.envsoft.2015.12.002
Adetula, B. A., and Bokov, P. M. (2012). Computational method for global sensitivity analysis of reactor neutronic parameters. Sci. Technol. Nucl. Installations 2012:11. doi: 10.1155/2012/109614
Allgeyer, S., Daubord, C., Hébert, H., Loevenbruck, A., Schindelé, F., and Madariaga, R. (2013). Could a 1755-like tsunami reach the french atlantic coastline? Constraints from twentieth century observations and numerical modeling. Pure Appl. Geophys. 170, 1415–1431. doi: 10.1007/s00024-012-0513-5
Arakawa, A. (1972). Design of the UCLA General Circulation model. In Numerical Simulation of Weather and Climate. Tech. Rep. No. 7. Los Angeles, CA.
Bachoc, F. (2013). Cross validation and maximum likelihood estimations of hyper-parameters of gaussian processes with model misspecification. Computa. Stati. Data Anal. 66, 55–69. doi: 10.1016/j.csda.2013.03.016
Baptista, M., Miranda, P., Miranda, J., and Victor, L. M. (1998). Constrains on the source of the 1755 Lisbon tsunami inferred from numerical modelling of historical data on the source of the 1755 Lisbon tsunami. J. Geodyn. 25, 159–174. doi: 10.1016/s0264-3707(97)00020-3
Baptista, M. A., Miranda, J. M., Chierici, F., and Zitellini, N. (2003). New study of the 1755 earthquake source based on multi-channel seismic survey data and tsunami modeling. Nat. Hazards Earth Syst, Sci. 3, 333–340. doi: 10.5194/nhess-3-333-2003
Baptista, M. A., Miranda, J. M., Matias, L., and Omira, R. (2017). Synthetic tsunami waveform catalogs with kinematic constraints. Nat. Hazards Earth Syst. Sci. 17, 1253–1265. doi: 10.5194/nhess-17-1253-2017
Barkan, R., Uri, S., and Lin, J. (2009). Far field tsunami simulations of the 1755 Lisbon earthquakeImplications for tsunami hazard to the US East Coast and the Caribbean. Mar.Geol. 264, 109–122. doi: 10.1016/j.margeo.2008.10.010
Baroni, G., and Tarantola, S. (2014). A General probabilistic framework for uncertainty and global sensitivity analysis of deterministic models A hydrological case study. Environ. Model. Softw. 51, 26–34. doi: 10.1016/j.envsoft.2013.09.022
Basili, R., Brizuela, B., Herrero, A., Iqbal, S., Lorito, S., Maesano, F. E., et al. (2018). NEAM Tsunami Hazard Model 2018 (NEAMTHM18)Online Data of the Probabilistic Tsunami Hazard Model for the NEAM Region from the TSUMAPS-NEAM project, Chap. Rome. Istituto Nazionale di Geofisica e Vulcanologia (INGV).
Basili, R., Brizuela, B., Herrero, A., Iqbal, S., Lorito, S., Maesano, F. E., et al. (2019). NEAMTHM18 Documentationthe Making of the TSUMAPS-NEAM Tsunami Hazard Model 2018.Rome:Istituto Nazionale di Geofisica e Vulcanologia (INGV).
Behrens, J., and Dias, F. (2015). New computational methods in tsunami science, philosophical transactions of the royal society a mathematical. Phys. Eng. Sci. 373:20140382. doi: 10.1098/rsta.2014.0382
Breiman, L., and Spector, P. (1992). Submodel selection and evaluation in regression. The X-random case. Int. Stat. Rev. Int. Stat. 60, 291–319. doi: 10.2307/1403680
Buforn, E., Udias, A., and Mezcua, J. (1988). Seismicity and focal mechanisms in south Spain. Bull.Seismol.Soc.Am. 78:2024.
Cunha, T., Matias, L., Terrinha, P., Negredo, A., Rosas, F., Fernandes, R., et al. (2012). Neotectonics of the SW Iberia margin, gulf of cadiz and alboran seaa reassessment including recent structural, seismic and geodetic data. Geophys. J. Int. 188, 850–872. doi: 10.1111/j.1365-246x.2011.05328.x
Cunha, T., Watts, A., Pinheiro, L., and Myklebust, R. (2010). Seismic and gravity anomaly evidence of large-scale compressional deformation off SW Portugal. Earth Planet. Sci. Lett. 293, 171–179. doi: 10.1016/j.epsl.2010.01.047
Davies, G., and Griffin, J. (2019). Sensitivity of probabilistic tsunami hazard assessment to far-field earthquake slip complexity and rigidity depth-dependenceCase study of australia. Pure Appl. Geophys. 1–28. doi: 10.1007/s00024-019-02299-w
Duarte, J. C., Rosas, F. M., Terrinha, P., Schellart, W. P., Boutelier, D., Gutscher, M.-A., et al. (2013). Are subduction zones invading the Atlantic? Evidence from the southwest Iberia margin. Geology 41, 839–842. doi: 10.1130/g34100.1
Dupuy, D., Helbert, C., and Franco, J. (2015). Dice design and dicee valtwo R packages for design and analysis of computer experiments. J. Stat. Softw. 65, 1–38.
Faivre, R., Iooss, B., Mahévas, S., Makowski, D., and Monod, É (2013). Analyse de sensibilité et Exploration de Modèles. Application aux Sciences de la Nature et de L’environnement.Bengaluru:HAL.
Friedman, J., Hastie, T., and Tibshirani, R. (2001). The Elements of Statistical Learning, Vol. 1. New York, NY.:Springer series in statistics.
Gracia, E., Danobeitia, J., and Vergés, J. Parsifal Team. (2003). Mapping active faults offshore Portugal (36 N–38 N)implications for seismic hazard assessment along the southwest Iberian margin. Geology 31, 83–86.
Grandin, R., Borges, J. F., Bezzeghoud, M., Caldeira, B., and Carrilho, F. (2007). Simulations of strong ground motion in SW Iberia for the 1969 February 28 (M s= 8.0) and the 1755 November 1 (M∼ 8.5) earthquakes-II. Strong ground motion simulations. Geophys. J. Int. 171, 807–822. doi: 10.1111/j.1365-246x.2007.03571.x
Grevemeyer, I., Lange, D., Villinger, H., Custodio, S., and Matias, L. (2017). Seismotectonics of the horseshoe abyssal plain and gorringe bank, eastern atlantic ocean constraints from ocean bottom seismometer data. J. Geophys. Res. Solid Earth. 122, 63–78. doi: 10.1002/2016jb013586
Gutscher, M.-A., Baptista, M., and Miranda, J. (2006). The Gibraltar Arc seismogenic zone (part 2)constraints on a shallow east dipping fault plane source for the 1755 Lisbon earthquake provided by tsunami modeling and seismic intensity. Tectonophysics 426, 153–166. doi: 10.1016/j.tecto.2006.02.025
Hanks, T. C., and Kanamori, H. (1979). A moment magnitude scale. J. Geophys. Res. Solid Earth 84, 2348–2350.
Hastie, T., Tibshirani, R., and Friedman, J. (2009). The Elements of Statistical Learning: Data Mining, Inference, and Prediction.Springer Science & Business Media. doi: 10.1007/b94608
Homma, T., and Saltelli, A. (1996). Importance measures in global sensitivity analysis of nonlinear models. Reliabi. Eng. Syst. Saf. 52, 1–17. doi: 10.1016/0951-8320(96)00002-6
Horsburgh, K., Wilson, C., Baptie, B., Cooper, A., Cresswell, D., Musson, R., et al. (2008). Impact of a Lisbon−type tsunami on the UK coastline and the implications for tsunami propagation over broad continental shelves. J. Geophys. Res. Oceans 113. doi: 10.1029/2007JC004425
Horspool, N., Pranantyo, I., Griffin, J., Latief, H., Natawidjaja, D., Kongko, W., et al. (2014). A probabilistic tsunami hazard assessment for Indonesia. Nat. Hazards Earth Syst. Sci. 14, 3105–3122.
Iooss, B., and Lemaître, P. (2015). A Review on Global Sensitivity Analysis Methods, in Uncertainty Management in Simulation-Optimization of Complex Systems.Springer, 101–122.
Iooss, B., Ribatet, M., and Marrel, A. (2008). Global sensitivity analysis of stochastic computer models with joint metamodels. arXiv [preprint],Google Scholar
Jansen, M. J., Rossing, W. A., and Daamen, R. A. (1994). Monte carlo estimation of uncertainty contributions from several independent multivariate sources. In: Predictability and nonlinear modelling in natural sciences and economics / Grasman, ed J. G. van Straaten (Dordrecht:Kluwer Academic Publishers), 334–343. doi: 10.1007/978-94-011-0962-8_28
Johnston, A. C. (1996). Seismic moment assessment of earthquakes in stable continental regions—III. New Madrid 1811–1812, Charleston 1886 and Lisbon 1755. Geophys. J. Int. 126, 314–344. doi: 10.1111/j.1365-246x.1996.tb05294.x
Kalra, T. S., Aretxabaleta, A., Seshadri, P., Ganju, N. K., and Beudin, A. (2018). Sensitivity analysis of a coupled hydrodynamic-vegetation model using the effectively subsampled quadratures method. Geosci. Model. Dev. 10, 4511–4523.
Kleijnen, J. P. (2005). An overview of the design and analysis of simulation experiments for sensitivity analysis. Eur. J. Operati. Res. 164, 287–300. doi: 10.1016/j.ejor.2004.02.005
Kohavi, R. (1995). A study of cross-validation and bootstrap for accuracy estimation and model selection. Ijcai 1137–1143. Available online at: https://www.researchgate.net/profile/Ron_Kohavi/publication/2352264_A_Study_of_Cross-Validation_and_Bootstrap_for_Accuracy_Estimation_and_Model_Selection/links/02e7e51bcc14c5e91c000000.pdf
Krige, D. G. (1951). A statistical approach to some basic mine valuation problems on the Witwatersrand. J. South, Afr. Inst. Min. Metall. 52, 119–139.
Löwe, R., Urich, C., Kulahci, M., Radhakrishnan, M., Deletic, A., and Arnbjerg-Nielsen, K. (2018). Simulating flood risk under non-stationary climate and urban development conditions–experimental setup for multiple hazards and a variety of scenarios. Environ. Model. Softw. 102, 155–171. doi: 10.1016/j.envsoft.2018.01.008
Lynett, P., Wei, Y., and Arcas, D. (2004). “Tsunami hazard assessmentBest modelling practices and state-of-the-art technology (nureg/cr-7223),” in Numerical Modeling of Water Waves. ed C. L. Mader. (Boca Raton, FL:CRC press).
Machac, D., Reichert, P., Rieckermann, J., and Albert, C. (2016). Fast mechanism-based emulator of a slow urban hydrodynamic drainage simulator. Environ. Model. Softw. 78, e54–e67. doi: 10.1016/j.envsoft.2015.12.007
Marrel, A., Iooss, B., Laurent, B., and Roustant, O. (2009). Calculations of the sobol indices for the gaussian processes metamodel. Reliabil. Engi. Syst. Saf. 94, 742–751. doi: 10.1016/j.ress.2008.07.008
Marrel, A., Iooss, B., Van Dorpe, F., and Volkova, E. (2008). An efficient methodology for modeling complex computer codes with Gaussian processes. Comput. Stat. Data Anal. 52, 4731–4744. doi: 10.1016/j.csda.2008.03.026
Marzocchi, W., Taroni, M., and Selva, J. (2015). Accounting for epistemic uncertainty in PSHALogic tree and ensemble modeling. Bull. Seismol. Soc. Am. 105, 2151–2159. doi: 10.1785/0120140131
Matheron, G. (1963). Principles of geostatistics. Econ. Geol. 58, 1246–1266. doi: 10.2113/gsecongeo.58.8.1246
Molinari, I., and Morelli, A. (2011). EPcrusta reference crustal model for the European Plate. Geophys. J. Int. 185, 352–364. doi: 10.1111/j.1365-246x.2011.04940.x
Molinari, I., Tonini, R., Lorito, S., Piatanesi, A., Romano, F., Melini, D., et al. (2016). Fast evaluation of tsunami scenariosuncertainty assessment for a Mediterranean Sea database. Nat. Hazards Earth Syst. Sci. 16, 2593–2602. doi: 10.5194/nhess-16-2593-2016
Nguyen, T.-M., Richet, Y., Balayn, P., and Bardet, L. (2015). Propagation des incertitudes dans les modeles hydrauliques 1D. La Houille Blanche 55–62. doi: 10.1051/lhb/20150055
Okada, Y. (1985). Surface deformation due to shear and tensile faults in a half-space. Bull. Seismol. Soc. Am. 75, 1135–1154.
Omira, R., Matias, L., and Baptista, M. A. (2016). Developing an event-tree probabilistic tsunami inundation model for NE atlantic coastsapplication to a case study. Pure Appl. Geophys. 173, 3775–3794. doi: 10.1007/s00024-016-1367-z
Pujol, G., Iooss, B., and Janon, A. (2017). Sensitivity: Global Sensitivity Analysis of Model Outputs. Available online at: https://CRAN.R-project.org/package=sensitivity
R Core Team (2016). A Langage and Environment for Statistical Computing, in, R Foundation for Statistical Computing.Vienne:R core Team.
Razavi, S., Tolson, B. A., and Burn, D. H. (2012a). Review of surrogate modeling in water resources. Water Resour. Res. 48, doi: 10.1029/2011WR011527
Razavi, S., Tolson, B. A., and Burn, D. H. (2012b). Numerical assessment of metamodeling strategies in computationally intensive optimization. Environ. Model. Softw. 34, 67–86. doi: 10.1016/j.envsoft.2011.09.010
Richet, Y., and Bacchi, V. (2019). Inversion algorithm for civil flood defense optimizationApplication to two-dimensional numerical model of the garonne river in france. Front. Environ. Sci. 7:160. doi: 10.3389/fenvs.2019.00160
Rohmer, J., Rousseau, M., Lemoine, A., Pedreros, R., Lambert, J., and Benki, A. (2018). Source characterisation by mixing long-running tsunami wave numerical simulations and historical observations within a metamodel-aided ABC setting. Stoch. Environ. Res. Risk Assess. 32, 967–984. doi: 10.1007/s00477-017-1423-y
Roshan, A. D., Basu, P. C., and Jangid, R. S. (2016). Tsunami hazard assessment of Indian coast. Nat. Hazards 82, 733–762. doi: 10.1007/s11069-016-2216-1
Roustant, O., Ginsbourger, D., and Deville, Y. (2012). DiceKriging, dice optim two R packages for(the)analysis of computer experiments by kriging-based metamodeling and optimization. J. Stat. Softw. 51, 1–55.
Rullière, D., Durrande, N., Bachoc, F., and Chevalier, C. (2018). Nested kriging predictions for datasets with a large number of observations. Stat. Comput. 28, 849–867. doi: 10.1007/s11222-017-9766-2
Saint-Geours, N. (2012). Sensitivity Analysis of Spatial Modelsapplication to Cost-Benefit Analysis of. (flood)Risk Management Plans.Montpellier:Université Montpellier II-Sciences et Techniques du Languedoc.
Saito, T., Ito, Y., Inazu, D., and Hino, R. (2011). Tsunami source of the 2011 Tohoku−Oki earthquake, JapanInversion analysis based on dispersive tsunami simulations. Geophys. Res. Lett. 38. doi: 10.1029/2011GL049089
Saltelli, A. (2002). Making best use of model evaluations to compute sensitivity indices. Comput. Phys. Commun. 145, 280–297. doi: 10.1016/s0010-4655(02)00280-1
Saltelli, A., Ratto, M., Andres, T., Campolongo, F., Cariboni, J., Gatelli, D., et al. (2008). Global Sensitivity Analysisthe Primer.New York, NY:John Wiley & Sons.
Saltelli, A., Tarantola, S., Campolongo, F., and Ratto, M. (2004). Sensitivity Analysis in Practicea Guide to Assessing Scientific Models.New York, NY:John Wiley & Sons.
Saltelli, A., Tarantola, S., and Chan, K.-S. (1999). A quantitative model-independent method for global sensitivity analysis of model output. Technometrics 41, 39–56. doi: 10.1080/00401706.1999.10485594
Sammarco, P., and Renzi, E. (2008). Landslide tsunamis propagating along a plane beach. J. Fluid Mech. 598, 107–119. doi: 10.1017/S0022112007009731
Santana-Quintero, L. V., Montano, A. A., and Coello, C. A. (2010). “A review of techniques for handling expensive functions in evolutionary multi-objective optimization,” in Computational Intelligence in Expensive Optimization Problems, edsM. H. Lim and Y. S. Ong (Springer Berlin Heidelberg), 29e59.
Santner, T. J., Williams, B. J., and Notz, W. I. (2003). The Design and Analysis of Computer Experiments. 1st edn. New York, NY:Springer-Verlag.
Santos, A., and Koshimura, S. (2015). The historical review of the 1755 Lisbon Tsunami. J. Geodesy Geomat. Eng. 1, 38–52.
Sarri, A., Guillas, S., and Dias, F. (2012). Statistical emulation of a tsunami model for sensitivity analysis and uncertainty quantification. arXiv 205, 1780–1803.
Selva, J., Tonini, R., Molinari, I., Tiberti, M. M., Romano, F., Grezio, A., et al. (2016). Quantification of source uncertainties in Seismic Probabilistic Tsunami Hazard Analysis (SPTHA). Geophys. J. Int. 205, 1780–1803. doi: 10.1093/gji/ggw107
Silva, S., Terrinha, P., Matias, L., Duarte, J. C., Roque, C., Ranero, C. R., et al. (2017). Micro-seismicity in the Gulf of CadizIs there a link between micro-seismicity, high magnitude earthquakes and active faults? Tectonophysics. 717, 226–241. doi: 10.1016/j.tecto.2017.07.026
Sobol, I. M. (1993). Sensitivity estimates for non linear mathematical models. Math. Model. Comput. Exp. 1, 407–414.
Sobol, I. M. (2001). Global sensitivity indices for nonlinear mathematical models and their Monte Carlo estimates. Math. Comput. Simul. 55, 271–280. doi: 10.1016/s0378-4754(00)00270-6
Sørensen, M. B., Spada, M., Babeyko, A., Wiemer, S., and Grünthal, G. (2012). Probabilistic tsunami hazard in the mediterranean Sea. J. Geophys. Res. Solid Earth 117. doi: 10.1029/2010JB008169
Sraj, I., Mandli, K. T., Knio, O. M., Dawson, C. N., and Hoteit, I. (2014). Uncertainty quantification and inference of Manning’s friction coefficients using dart buoy data during the Tôhoku Tsunami. Ocean Model. 83, 82–97. doi: 10.1016/j.ocemod.2014.09.001
Stich, D., Mancilla, F. D. L., Pondrelli, S., and Morales, J. (2007). Source analysis of the February 12th 2007, Mw 6.0 Horseshoe earthquakeImplications for the 1755 Lisbon earthquake. Geophys. Res. Lett. 34. doi: 10.1029/2007GL030012
Storlie, C. B., Swiler, L. P., Helton, J. C., and Sallaberry, C. J. (2009). Implementation and evaluation of nonparametric regression procedures for sensitivity analysis of computationally demanding models. Reliab. Eng. Syst. Saf. 94, 1735–1763. doi: 10.1016/j.ress.2009.05.007
Terrinha, P., Pinheiro, L. M., Henriet, J.-P., Matias, L., Ivanov, M., Monteiro, J. H., et al. (2003). Tsunamigenic-seismogenic structures, neotectonics, sedimentary processes and slope instability on the southwest Portuguese Margin. Mar. Geol. 195, 55–73. doi: 10.1016/s0025-3227(02)00682-5
Villa-Vialaneix, N., Follador, M., Ratto, M., and Leip, A. (2012). A comparison of eight metamodeling techniques for the simulation of N 2O fluxes and N leaching from corn crops. Environ. Model. Softw. 34, e51–e66. doi: 10.1016/j.envsoft.2011.05.003
Violeau, D., Ata, R., Benoit, M., Joly, A., Abadie, S., Clous, L., et al. (2016). “A database of validation cases for tsunami numerical modelling, Sustainable Hydraulics in the Era of Global Change,” in Proceedings of the 4th IAHR Europe Congress (Liege), 377.
Volkova, E., Iooss, B., and Van Dorpe, F. (2008). Global sensitivity analysis for a numerical model of radionuclide migration from the RRC “Kurchatov Institute” radwaste disposal site. Stoch. Environ. Res. Risk Assess. 22, 17–31. doi: 10.1007/s00477-006-0093-y
WENRA (2015). Guidance document issue tNatural hazards head document, Guideline for the WENRARHWG Safety Reference Levels for Natural hazards introduced as lesson learned from TEPCO Fukushima Daiichi accident. Available online at: http://www.wenra.org/media/filer_public/2016/11/04/wenra_guidance_on_extreme_weather_conditions_-_2016-10-11.pdf
Wolfs, V., Meert, P., and Willems, P. (2015). Modular conceptual modelling approach and software for river hydraulic simulations. Environ. Model. Softw. 71, 60e77. doi: 10.1016/j.envsoft.2015.05.010
Yanagisawa, K., Imamura, F., Sakakiyama, T., Annaka, T., Takeda, T., and Shuto, N. (2007). Tsunami assessment for risk management at nuclear power facilities in Japan. Pure Appl.Geophys. 164, 565–576. doi: 10.1007/978-3-7643-8364-0_16
Yazdi, J., and Salehi Neyshabouri, S. A. A. (2014). Adaptive surrogate modeling for optimization of flood control detention dams. Environ. Model. Softw. 61, 106–120. doi: 10.1016/j.envsoft.2014.07.007
Keywords: kriging surrogate, uncertainty quantification, tsunami modeling, hazard analysis, sensitivity analysis
Citation: Bacchi V, Jomard H, Scotti O, Antoshchenkova E, Bardet L, Duluc C-M and Hebert H (2020) Using Meta-Models for Tsunami Hazard Analysis: An Example of Application for the French Atlantic Coast. Front. Earth Sci. 8:41. doi: 10.3389/feart.2020.00041
Received: 08 May 2019; Accepted: 04 February 2020;
Published: 03 March 2020.
Edited by:
Philippe Renard, Université de Neuchâtel, SwitzerlandReviewed by:
Jacopo Selva, Istituto Nazionale di Geofisica e Vulcanologia (INGV), ItalyTipaluck Krityakierne, Mahidol University, Thailand
Copyright © 2020 Bacchi, Jomard, Scotti, Antoshchenkova, Bardet, Duluc and Hebert. This is an open-access article distributed under the terms of the Creative Commons Attribution License (CC BY). The use, distribution or reproduction in other forums is permitted, provided the original author(s) and the copyright owner(s) are credited and that the original publication in this journal is cited, in accordance with accepted academic practice. No use, distribution or reproduction is permitted which does not comply with these terms.
*Correspondence: Vito Bacchi, dml0by5iYWNjaGlAZ21haWwuY29t