- 1Department of Earth Sciences, National Taiwan Normal University, Taipei, Taiwan
- 2Institute of Earth Sciences, Academia Sinica, Nankang, Taiwan
- 3Graduate School of Education, Chung Yuan Christian University, Taoyuan, Taiwan
Citizen seismology encourages the public involvement in data collection, analysis, and reporting, and has the potential to greatly improve the emergency response to seismic hazard. We believe in the value of citizen seismology and started with distributing Quake-Catcher Network (QCN) sensors at schools in Taiwan. Unlike most of western countries, Earth Sciences is a required course in junior and senior high schools of Taiwan (Ministry of Education, 2014). Low-cost seismometer is potentially a powerful tool in classrooms to teach earthquake science, which makes school teachers our targeted users. We work closely with school teachers and students to understand how a citizen seismology project can help them to encourage successful engagement. In this study, we establish the achievements gained and problems encountered in different phases of the project since 2013. The main tasks carried out here include (1) refinement of Citizen Seismology Literacy (CSL) into three dimensions: Awareness, and Willingness, and Technology fluency (2) development of an interactive competition platform called the Near-Real Time Earthquake Game Competition (EGCnrt) that allows citizen seismologists to report earthquake information by processing P- and S-wave arrivals, peak ground motion, and first motion of P-waves for every inland ML≥4 earthquake in Taiwan; (3) development of the formative assessment for the 1.5 h long, non-lecture-based game activities. Based on 565 student surveys completed after our 2016 summer outreach activity, we found that all three CSL dimensions have an influence on students’ score in the training activity. The final score in EGCnrt is also found to significantly correlate with the performance in the training sections. We therefore, propose that the CSL can act as a powerful indicator for the performance and engagement in earthquake learning activities. The game-based, non-lecture-based learning approach can be effective in promoting citizen seismology in the future.
Highlight
– How to motivate the long-term engagement in citizen seismology remains a global challenge. In this paper we review different phases of citizen seismology in Taiwan since 2013. The highlight of the project is the development of a near-real time earthquake game competition. This competition platform allows citizen seismologist to report earthquake information by processing P- and S-wave arrivals (Finding Earthquakes game), peak ground motion (Measuring Earthquake Shaking and Sizing Up Earthquakes games), and first motion of P waves (Measuring How a Fault Moves game). The users are allowed to process the real data, to estimate the location, seismic intensity, magnitude, and focal mechanism of any magnitude greater than 4 earthquake in Taiwan. Through the formative assessment during the outreach activities, we propose a 1.5 h long, game-based, non-lecture-based learning approach that can be effective in promoting citizen seismology in the future.
Project Background
In the past decade, citizen seismology has become more popular due to the rise of internet, the development of social media, and the low-cost sensor technologies that allow for building networks of non-scientists for collecting and analyzing seismic data. The successful projects for rapid earthquake information include DYFI (Did You Feel It) developed by U.S. Geological Survey (Wald et al., 2011), multichannel rapid information system comprising websites developed by European Mediterranean Seismological Centre (EMSC) (e.g., Bossu et al., 2008, 2015, 2018), and other social networking tools (e.g., Twitter, facebook, Instagram, LinkedIn) that allow the citizens to provide the first-hand accounts of ground conditions for situational awareness and emergence response (Earle et al., 2010; Guy et al., 2010). For places where the seismic station coverage is sparse, crowdsourcing approaches also play a crucial role in understanding the shaking level of an earthquake. There exist some projects of citizen seismology that aim at distributing low-cost sensors for early warning or data sharing purposes. With a collection of seismic signals from a denser seismic network, they provide accurate and useful seismic signals for scientific investigation and hazard awareness. For example, QCN and Community Seismic Network provide citizens with micro-electro-mechanical systems (MEMS) accelerometers that are installed at home and school, connected to the computer, and sending continuous data to the server (Cochran et al., 2009). MyShake makes use of the data from phone motion sensors, to provide early warning of earthquakes (Kong et al., 2016; Allen et al., 2019). P-Alert distributes the MEMS sensors at schools that are equipped with P wave alarm technology (Wu et al., 2013). These sensor-based citizen networks provide precise and real-time information, which allow the science community to collect and analyze data. However, the participation of citizens has been minimal due to several reasons: (1) the devices are black-boxes to the users, (2) the recorded signals are difficult for non-seismologists to read and understand, (3) application does not encourage the instant feedback from users, (4) no routine exercises or activities to attract users’ attention. How to motivate citizens to participate is a big challenge.
Taiwan is situated at a complicated plate boundary zone between the Eurasian plate (EP) and the Philippine Sea Plates (PSP), which exhibits a unique interaction between the EP and PSP. In northeast Taiwan, the PSP subducts beneath the rifted Eurasian plate margin along the Ryukyu Trench at a rate of 8 cm/yr to the north-west (Seno, 1977; Yu et al., 1977; Seno et al., 1993), whereas in southwest Taiwan, the Eurasian plate subducts underneath PSP along the Malina trench. As a result, approximately 21,000 earthquakes strike the island with an average rate of 39 ML ≥ 5 events [equivalent to Mw ≥ 4.48 using Mw – ML relation derived from local seismicity by Huang et al. (2000)] every year (in the past 29 years since 1991). In the next 30 years, the probability of Mw ≥ 6.5 earthquakes is predicted to be higher than 87% based on probabilistic seismic hazard analysis (PSHA) (Wang et al., 2016). Note that the moment magnitude is used in PSHA to avoid the ML saturation at a large magnitude. To better prepare Taiwanese citizens for future impacts of seismic hazard, it is important that they understand why earthquakes happen, how they occur, and how to best prepare for an earthquake. Citizen seismology in Taiwan was motivated by the need for volunteers to host QCN sensors (Liang et al., 2016). Unlike most western countries, Earth Sciences is a required course in junior and senior high schools of Taiwan (Ministry of Education, 2014). Can QCN be potentially a powerful tool in classrooms for teaching earthquake science? Given that very dense seismic networks in Taiwan (e.g., 5 km spacing for P-Alert network) already existed, our main goal was not to increase the number of sensors. Instead, we work closely with school teachers and students to understand how a citizen seismology project can help them to encourage successful engagement. The key questions are: how to motivate the teachers and their students to interact with the seismograms, how to transform their contribution to useful information, and how to plan a series of activities for a long-term engagement of citizens? In this paper we detail the scopes and tasks since 2013, the result of formative assessment, and the problems encountered in different phases of the project.
Phase I: Qcn Network
Motivated by the collaboration with QCN project (Cochran et al., 2009) for promoting citizen seismology in Asia, the Citizen Seismologists in Taiwan Project (CSTaiwan) was initiated in 2012. The purpose is to build a cloud-based computing service incorporating an earthquake school where the volunteers (teachers and students) can contribute to QCN data collection, analysis, and reporting with the potential of improving the emergency response to earthquakes. Up to 2019, 149 volunteers in total installed QCN sensors with Internet-enabled computers at schools. The population is highest at the places where we had intensive Professional Teacher Development workshops held by Taiwan Rotary club. Figure 1 shows the current QCN site distribution in Taiwan. Most of the stations are located in southwestern Taiwan (Tainan and Chiayi counties). The time evolution from 2012 January to 2016 October of active QCN sensors is shown in Figure 2. System logs indicate that the sensors are on average active ∼50–60% of the time. Note that to keep the QCN project active (i.e., seismic data can be continuously sent to the servers), the local host needs to check “project manager window” of the BOINC server software from time to time. It is a difficult mission for the hosts who are not familiar with the software in the English interface. Another problem is the switching role of local QCN host. In schools, the QCN sensor is usually hosted by the computer/information system manager, which is a temporary position with only a few years in service. Thus from time to time, the QCN hosts lost contact with the administration server, leading to the low “active” rate.
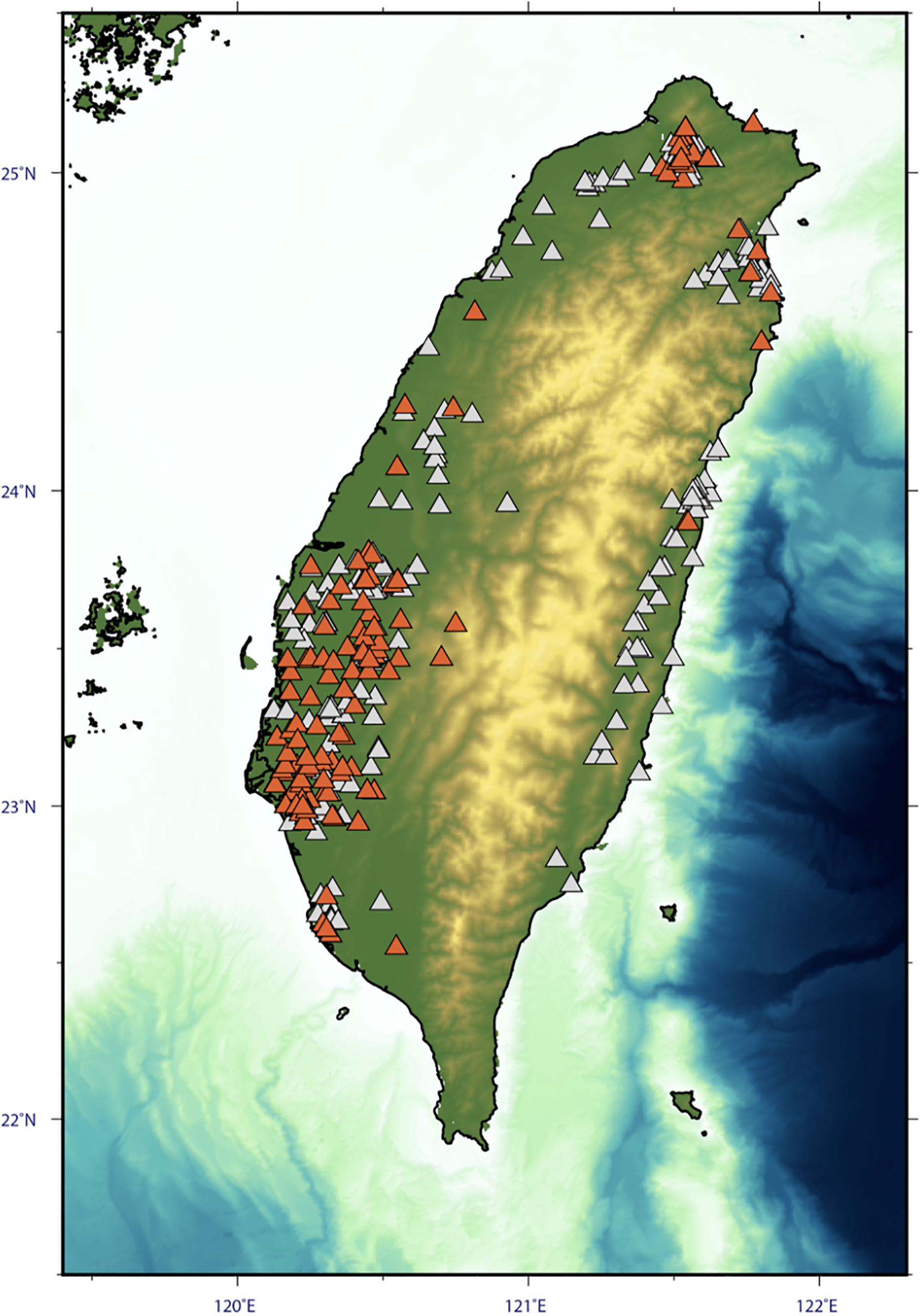
Figure 1. The distribution of QCN in Taiwan. The active stations are indicated by orange, while the inactive stations are shown by white.
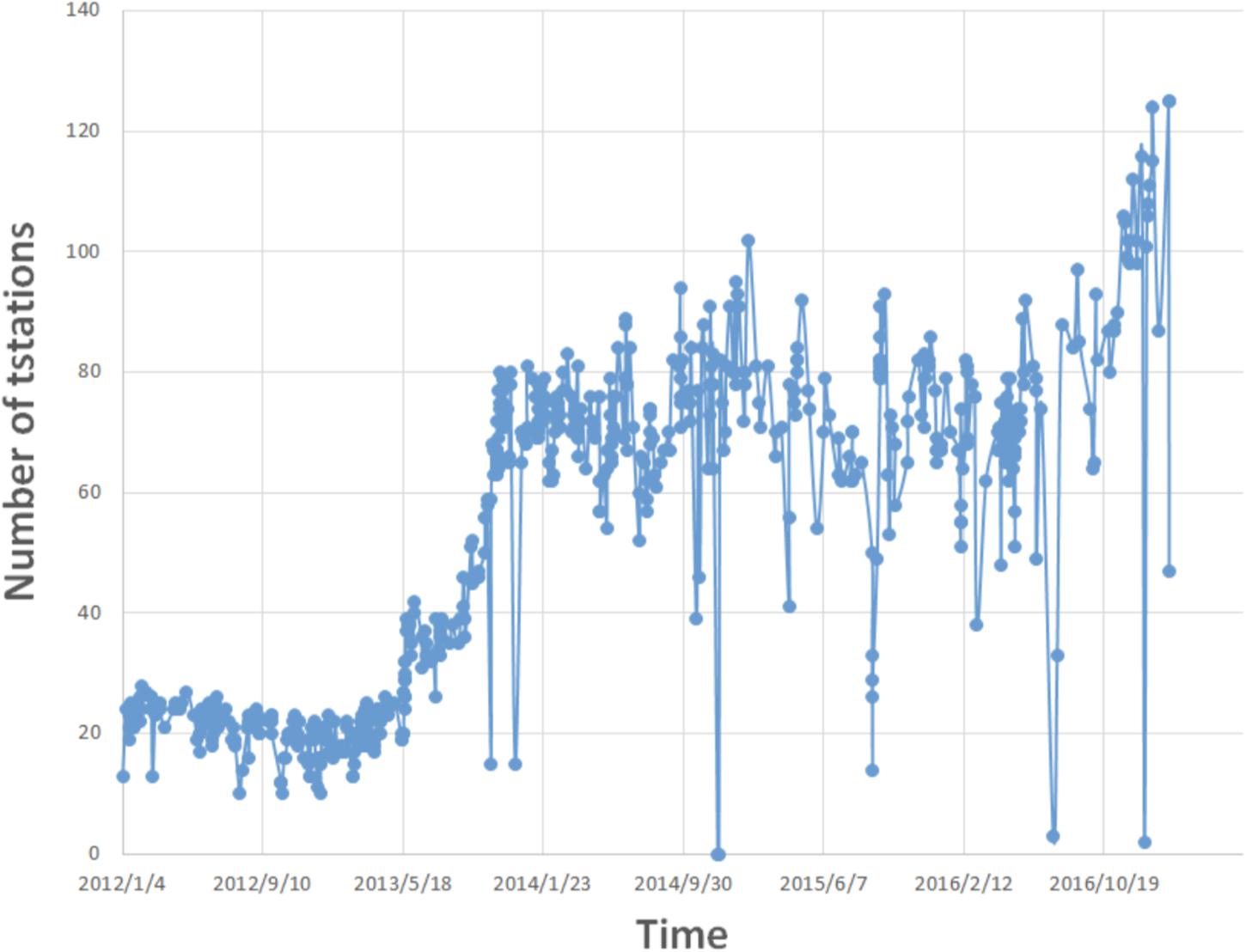
Figure 2. Time history of active QCN in Taiwan since 2012 January to 2016 November. After then the QCN server had encountered an unknown problem leading to an acquisition gap.
The recorded waveforms for magnitude greater than 4 earthquakes in Taiwan are shared in the platform of School QCN network (Figure 3). The volunteers can view and download the waveforms with Seismic Analysis Code (SAC) format (Goldstein et al., 2003) and further, analyze the seismic signal through the program of Seisgram2K. Continuous signals collected at homes/schools are streamed to Academia Sinica of Taiwan for data archiving. The QCN hosts who make effort to maintain the “active QCN,” often request teachable materials on “what the recorded signal means” and “what can be learned from the signals.” That’s where the next phase began.
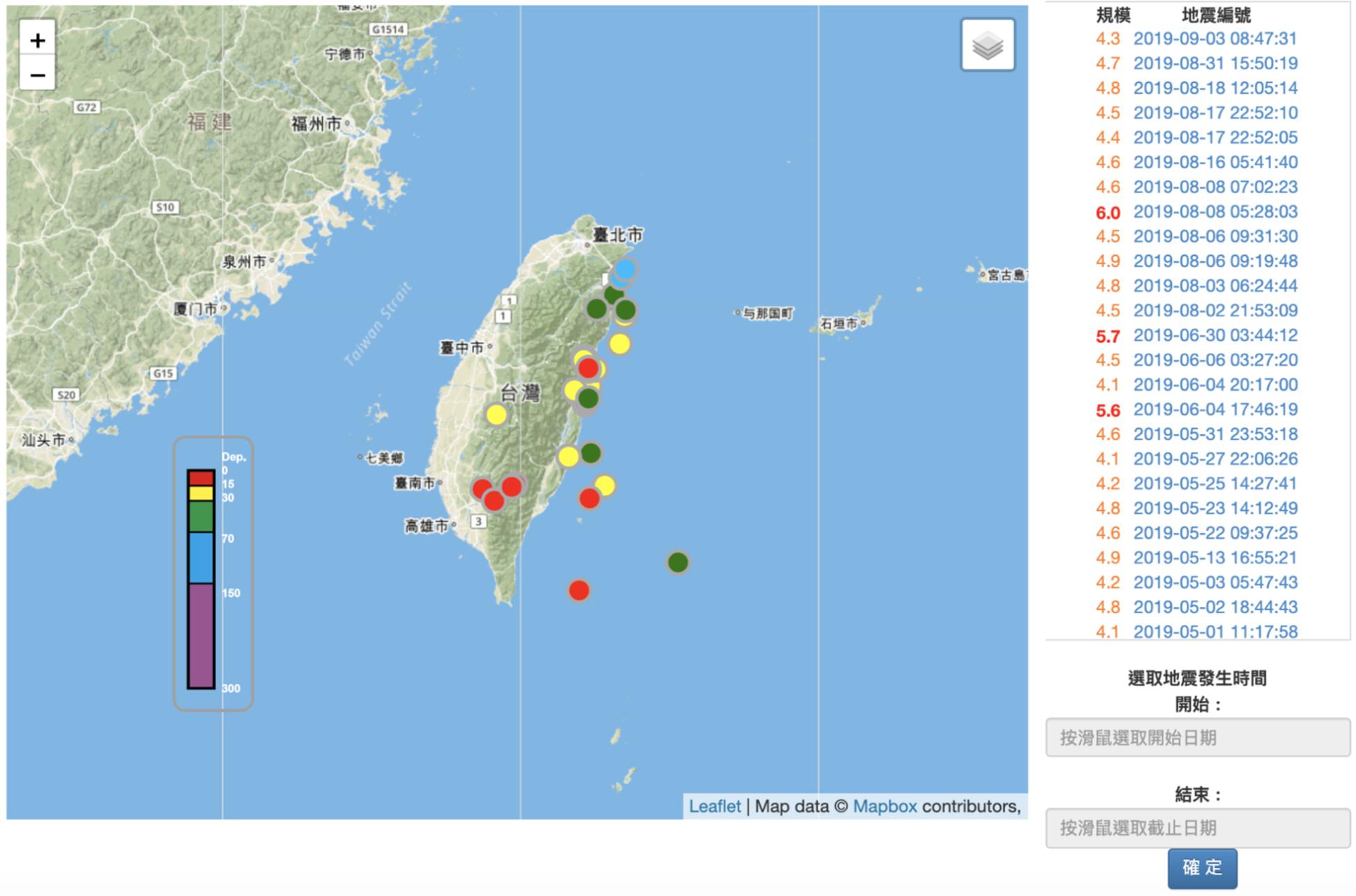
Figure 3. Website of School QCN network. Circles represent the recorded earthquakes colored coded by focal depth. The list of earthquakes on the right panel shows the date and magnitude for ML≥4 events, which allows the volunteers to access the waveforms in each QCN site.
Phase Ii: Near-Real Time Earthquake Game Competition and Teaching Resources
To make the recorded signals useful in classrooms and to allow the citizen to learn basic seismology, we developed an interactive tool called “the Near-Real Time Earthquake Game Competition (EGCnrt)1. This platform was announced along with online teaching resources in Nov. 2014. Within 10 min of a M≥4 earthquake event in Taiwan, the near-real time seismic data from the P-alert strong motion network (Wu et al., 2013, 2016) are released and the competition begins. The original purpose of the P-alert network is to provide on-site earthquake early warning and near real-time ground motion intensity measurements. Such island-wide earthquake early warning network could be beneficial for earthquake science learning (e.g., Kong et al., 2016), especially since the P-alert sensors are mostly installed in schools.
The EGCnrt competition platform allows citizen seismologists to report earthquake information by processing P- and S-wave arrivals (Finding Earthquakes game), peak ground motion (Measuring Earthquake Shaking and Sizing Up Earthquakes games), and first motion of P-waves (Measuring How a Fault Moves game). A series of simplified certificate games are designed in a training activity, to familiarize beginners with data processing steps. As shown in Figure 4A, four different processing skills are required in this training stage: (1) Finding the earthquake – by picking P- and S-wave arrivals at more than 3 stations, the epicenter of the earthquake can be found. (2) Measuring earthquake shaking – by picking the maximum amplitude in three components of seismograms, peak ground motion can be measured. (3) Sizing up earthquake – by picking the maximum amplitude in the horizontal component with the previously defined epicenter, earthquake magnitude can be measured. (4) Measuring how a fault moves – by picking initial motion polarities of P wave first arrival (up or down), the fault type (normal, thrust, or strike slip) can be determined. The certificate games corresponding to the above four components require the processing of at least three, five, ten, and 20 stations, respectively (Figure 4A). Once the certificates are achieved, the citizen seismologists can challenge themselves by taking part in the near-real time competition. As shown in Figure 5, the three components waveforms in each station can be viewed and processed when the users click the red circles in Figure 4B. The processing flow is listed in the left side of Figure 4B as (1) picking P and S arrivals at more than 3 stations (2) measuring peak ground acceleration (PGA) and magnitude at more than 7 stations (3) determining fault plane solution at more than 10 stations (4) creating the shake map at more than 15 stations. One can click the “locating earthquake” function to determine the hypocenter once three stations are processed. The competition steps are in a different order than the training steps. Here the processing of at least seven and ten stations are needed, to determine earthquake magnitude and fault plane solution, respectively. The score of the near-real time game is determined based on the precision of the location of the epicenter, magnitude, and fault plane solution. The algorithms are detailed in Liang et al. (2016) and also briefly summarized below.
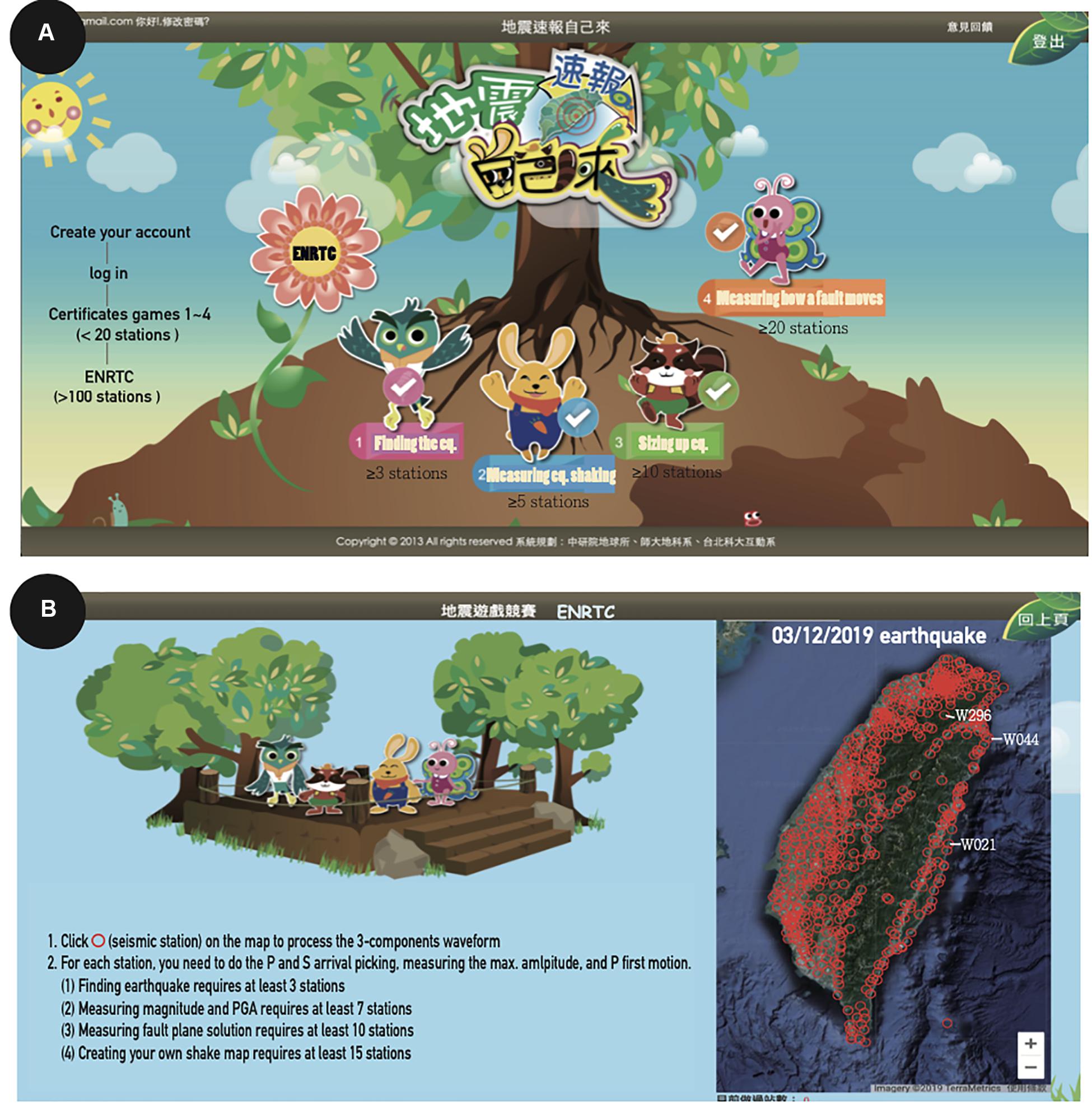
Figure 4. (A) The front page of the near-real-time earthquake games competition website. Each animal represents a different game. Once the certificate is achieved, the user can enter the competition platform (EGCnrt) to contribute to real earthquake information. (B) The EGCnrt page for a selected date of March 12, 2019. Station distribution for this event is shown by the red circles. The waveforms examples indicated by text are shown in Figure 5.
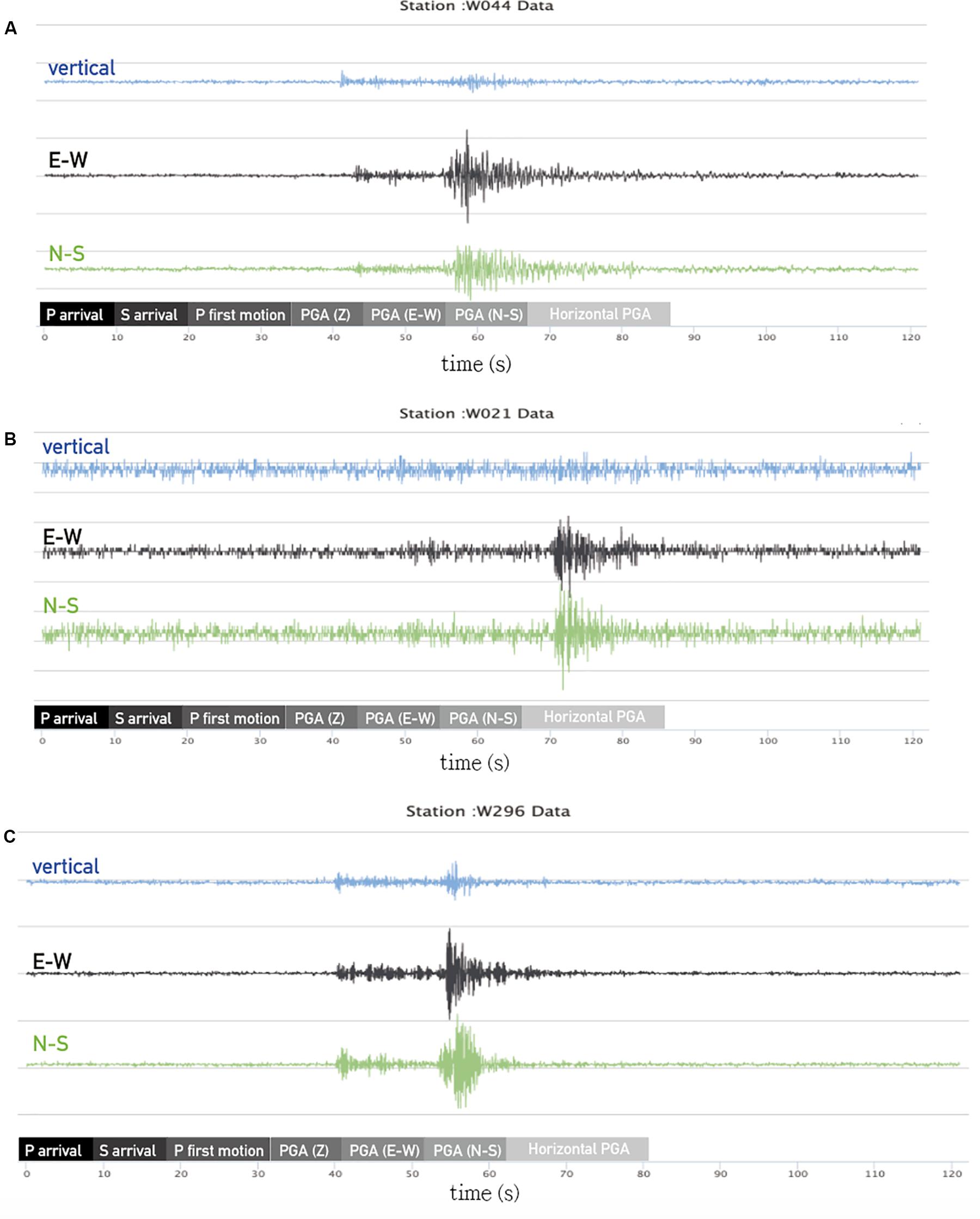
Figure 5. Waveform examples for three selected stations in Figure 4B. For each station the process flow is shown in the bottom.
1. Score for “Finding Earthquake”: (5 < Score_1 < 20)
X = Epicenter difference = (location determined by the user) – (location announced by Central Weather Bureau)
Score_1 = −0.6X + 20 if X < 25 km
Score_1 = 5, if X ≥ 25 km
2. Score for “Measuring earthquake shaking” Game: (5 < Score_2 < 20)
PGA difference Xi = (PGA determined by the user) – (PGA measured by the densest P-alert seismic network)
Score_2 = −0.2(ΣXi/n) + 20 if (ΣXi/n) < 75 cm/s2
Score_2 = 5 if (ΣXi/n) ≥ 75 cm/s2,
where n is the number of stations processed.
3. Score for “Sizing up earthquake” Game: (5 < Score_3 < 20)
Magnitude difference X = (Magnitude determined by the user) – (magnitude announced by Central Weather Bureau)
Score_3 = −10X + 20 if X < 1.5
Score_3 = 5 if X ≥ 1.5
4. Score for “Measuring how a fault moves” Game: (5 < Score_4 < 20)
Score_4 = 20 if the fault type is the same with the one announced by Real-Time Moment Tensor Monitoring System in Taiwan by Lee et al. (2013)2.
5. Additional score for number of stations processed: (5 < Score_5 < 20)
X = Number of stations processed
Score_5 = 0.2X + 10 if X < 50
Score_5 = 20 if X ≥ 50
Note that the final score is computed and accumulated when the above (1)–(4) are completed.
Six teachable units were also prepared, to closely link with different functions in EGCnrt. The summary of teachable units is listed below. (1) Orphan Tsunami: A story of mysterious tsunami in 1700 that stroke Japan along the coastline over a distance 1000 km without an apparent cause. The parent earthquake was found to locate in the western United States and Canada, in a region that was not known to have experienced an earthquake greater than Mw 7.5 in recorded times. Through geological evidence, the students will learn how the magnitude 8 (or higher) earthquake had written its own history. (2) Finding Earthquakes: An introduction to the skills needed for locating earthquakes – picking P- and S-wave arrivals at more than three stations. (3) The 2004 Sumatra Earthquake and Tsunami: A story of how the serious fatalities could happen during a disastrous tsunami, following the 2004 Mw 9.3 earthquake that occurred in northern Sumatra. The students will learn what are the precursory signals before a massive tsunami, how the amplitude of the seismic signal correlates with earthquake magnitude, and what information is required for effective tsunami warning. (4) Sizing Up Earthquakes: An introduction to the skills needed for determining earthquake magnitude and intensity – picking the maximum amplitude on three- component seismograms. (5) Forensic Seismology: A story of how the seismic signals help us to discriminate explosion/mine collapse from tectonic earthquake. (6) Making Fault Motions: An introduction to the skill needed for focal mechanism determination – picking the initial motion polarities of the first arrival of the P waves. These teaching materials are put into a several-mins-long youtube video, to prepare the citizen seismologist for EGCnrt. The original ppt and video clips are available on the website of https://katepili2003.wixsite.com/future-eq-school (in Chinese). Note that since students’ level may vary greatly from third to twelfth grade, the educational materials were designed differently to fit the student’s background knowledge. This was done during eight seed-teacher workshops where we worked with teachers from elementary, junior, and senior high schools in October 2013 to July 2014.
Phase Iii: Teacher Workshops and Development of Citizen Seismology Literacy (CSL)
Shortly after Phase II, we introduced the teaching materials in six teacher professional development workshops. With 15–80 participants in each workshop, we reached 121 first- to twelfth-grade teachers across the nation in 2014 and 2015. To collect feedback of the participants for future directions of this project, we developed 49 items to evaluate participants’ pre-knowledge, attitude, and skills in earthquake sciences, called Citizen Seismological Literacy (CSL). The CSL is compared with participants’ personality categorized into three sub-factors: Conscientiousness (a desire of personality to complete a task well), Intellect/Imagination (the ability of personality to understand or depict abstract ideas) and Grit (perseverance and passion for long-term goals). As detailed in Liang et al. (2017), we found that all three sub-factors in Personality are positively related to CSL dimensions of Attitude and Skills.
This indicates that that Conscientiousness, Intellect/imagination, and Grit may have an effect when developing one’s seismology literacy and that the CSL can act as a powerful approach to citizens’ learning paths for promoting citizen seismology in the future. Using Exploratory Factor Analysis (EFA), we also found some items in CSL questions are redundant. By removing the items with low factor loadings from the original 49 survey items, 15 items were extracted and re-categorized into new dimensions of Willingness, Awareness, and Technology fluency (Table 1), referring to the willingness to be educated and trained as contributors, the awareness of earthquakes and disaster prevention, and the self-efficacy on technical proficiency. The reliability can be indicated by Cronbach’s alpha that is commonly used to evaluate the internal consistency of the measurements within the same dimension. The value of higher than 0.7 and lower than 0.5 represent acceptable and unacceptable reliability, respectively. Here the alpha is calculated to be 0.756, 0.778, and 0.858 for Willingness, Awareness, and Technology fluency, respectively. The modified CSL model is now embedded in the EGCnrt game, which allows us to collect and analyze the players’ demographical information, pre-knowledge of seismology, and personality self-portraits.
In addition, we found that the most efficient learning happens when the participants are engaged in a competitive environment without a priori introduction of seismological background, indicating that the EGCnrt itself can be treated as self-learning tool. Despite the excellent learning progress through the EGCnrt, the degree of willingness of the participants to use EGCnrt in their own classrooms is found to be low. The feedback gathered in the individual interviews reveals the lack of confidence in teaching the earthquake science behind the game. The barriers for the teachers to make EGCnrt (with six teachable units) useful in the classroom mainly come from the limited teaching hours in Earth Sciences. In the exam-oriented education system of Taiwan, all the teaching hours are divided into five topics including astronomy, oceanography, atmospheric sciences, geology, and geophysics. The teachers often find it impossible to arrange additional hours to run all the teaching units associated with EGCnrt. We therefore changed our target audiences from teachers to students.
Phase Iv: Egcnrt Program in Schools and the Impact Assessment of Earthquake Game Competition
The outreach activities called EGCnrt program were initiated by an online registration system that allows the host teachers to sign in and arrange the time for the EGCnrt program in their classrooms. In summer 2016, we visited 12 schools selected by geographical diversity to run the pilot teaching. We reached 406 citizen seismologists (hereafter referred as players) who successfully participated in the game. Based on the experience in the previous workshops with teachers, the outreach activities were designed to facilitate the learning and thinking. As illustrated by the flowchart in Figure 6, the students are assigned to fill out the CSL survey before entering EGCnrt platform. The 1.5 h long EGCnrt program is composed of half an hour training activity and 1 h EGCnrt activity, as detailed in sections Training Activity (Certificate Game) and EGCnrt Activity.
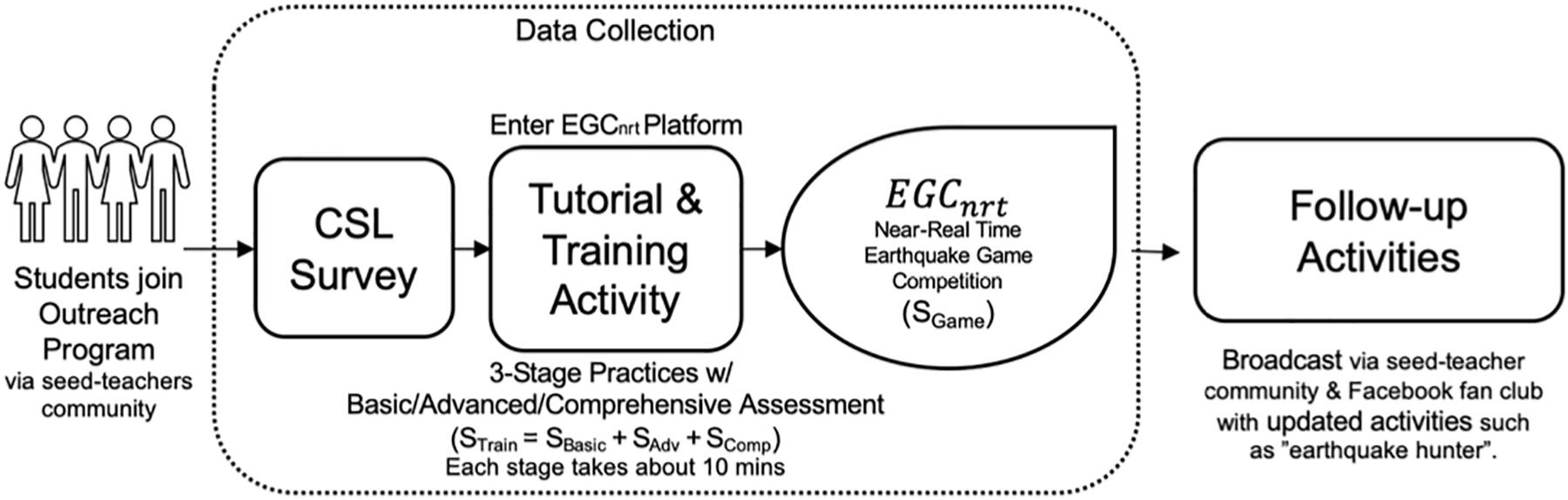
Figure 6. Flowchart of a 1.5 h long EGCnrt program at schools. This program is composed of CSL survey, training activity (four certificate games), and near-real time earthquake game competition. The tutorial in training activity only takes 5 min for introducing the structure and function of the EGCnrt platform.
Training Activity (Certificate Game)
The training activity requires the students to complete the four levels of certificates without lecture. A 5 min tutorial allows the students to (1) login to the system, (2) briefly understand the structure of this platform, and (3) learn how to view and process the seismic signals. Once they successfully got the certificates, nine questions will appear on the screen for bridging the game to some basic earthquake knowledge. Different from CSL, these questions are strongly tied with the knowledge behind the game. They can be classified into three sections: basic, advanced, and comprehensive knowledge. The three questions in each section are listed in Table 2. When the three questions are completed after each section, the correct answers will be displayed on the screen.
The section of basic knowledge (SBasic) refers to the recognition of the P and S first arrivals and their association with earthquake location, which is listed in high school curriculum. The section of advanced knowledge (SAdv) refers to an understanding of the relationship between maximum amplitude of seismogram and seismic intensity/earthquake magnitude. Although not listed in the high school curriculum, this concept introduced in this section is straightforward. The section of comprehensive knowledge (SComp) refers to the determination of fault plane solution using first motion of seismic waves, which requires an integration of seismic source and slip on the fault. It is a section bringing a new concept associating P wave radiation pattern and stereographic projection. The total score in this training activity (Strain) is 9, as STrain = SBasic + SAdv + SCom. Our experience shows that during the activity, getting the certificates requires only learning a specific skill or set of steps rather than requiring a deeper understanding. The knowledge behind each skill is only introduced in the pop-out questions that requires critical thinking. From our observation, in each classroom there exists only a handful of students who asked questions associated with logical connection between the skill and knowledge. These active learners can be identified during the activity.
EGCnrt Activity
The following 1 h EGCnrt activity requires the skills learned in the training stage to compete with each other using real data. The total score of up to 100 (SGame) is determined by the precision of the location of the epicenter, magnitude, and fault-plane solution. By examining the statistical significance of their correlation, we used Pearson Correlation to measure the linear association of two variables and k-mean clustering analysis to compare the difference between grouped variables.
Table 3 show the result of Pearson Correlation. We found that SGame is better correlated with SAdv and SComp than with SBasic, as revealed by r = 0.098, p = 0.049 for SAdv, r = 0.11, p = 0.027 for SComp, and p > 0.05 for SBasic. Here r and p represent Pearson correlation and statistical significance (p-value, > 0.05 means no significant correlation). This suggests that the knowledge gained during the training activity reflect their performance in EGCnrt activity. The performance in EGCnrt activity is also categorized into three groups as low (SGame = 0), medium (0 < SGame < 42), and high scores (SGame > 62) using k-means method, to compare with the scores in training activity. The k-means clustering method is commonly used due to its simplicity (MacQueen, 1967), which minimizes the distance between the cluster center and each point in the data set. As illustrated in Figure 7, it appears that the high score group in EGCnrt shows highest score in the training activity, which is consistent with the observation in Table 3. The increasing rate tends to be highest in advanced knowledge (Figure 7), which could be due to the fact that the skill learned for SAdv is heavily used in the EGCnrt activity. Note that the SGame is computed and accumulated once enough of stations are processed to obtain the basic earthquake information of location, magnitude, and fault plane solution (minimum requirement is 15 stations). From our observation, the large number of SGame = 0 happened when the students failed to stay focused on the EGCnrt activity. The coefficient of variation (cov) is a measurement of precision, smaller cov leads to greater precision. The cov of greater than 1 is considered to be high variance. In Figure 7, the largest cov (up to 0.82) appears in low game performance group, suggesting a less precise measure comparing with high and medium SGame group.
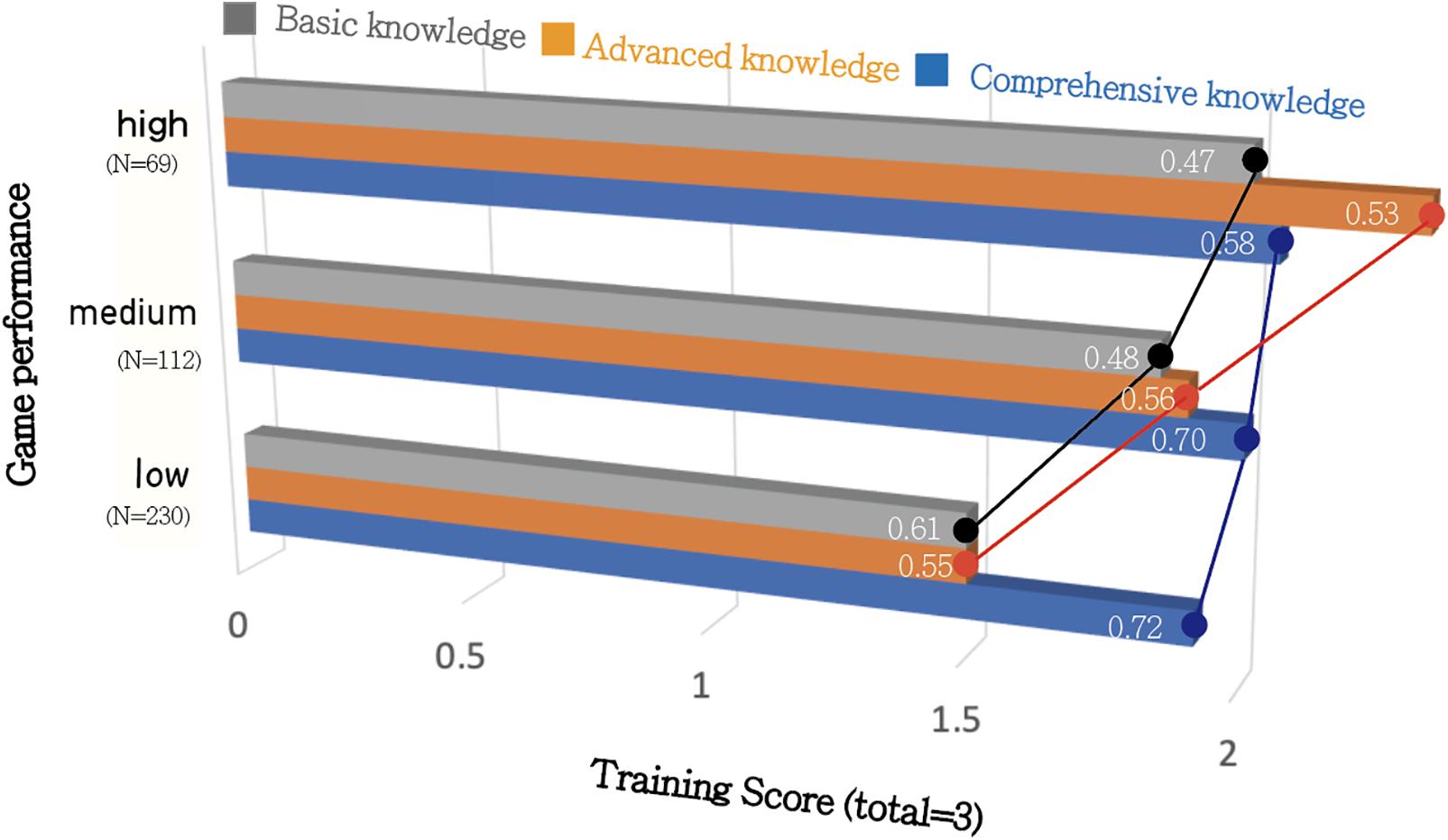
Figure 7. The relationship between training score and EGCnrt game performance. The number of students belonging to groups of low, medium, and high performance in EGCnrt is 230, 112, 69, respectively. In the total number of 425 samples, 14 went missing due to incorrect coordination. The number within each horizontal bar represent the coefficient of variation (the ratio of standard deviation to the mean).
Table 3 also shows the impact of EGCnrt on change in knowledge, attitude, and skill in earthquake sciences for students, as represented by CSL. The large p-value (0.167–0.827) indicates that the CSL does not reveal significant correlation with SGame and Strain. When the CSL is categorized into three levels from low, medium, and high for computing the corresponding SGame in Table 4, we found that the low CSL group tends to have lower SGame comparing with high and medium CSL groups. If comparing with the training score using the same CSL groups, the medium and high CSL also tend to show higher training scores, while the difference between medium and high CSL appears to be very small (Figure 8). This indicates that the correlation between scores in the EGCnrt activity (SGame and Strain) and CSL exists but is unlikely to follow a linear relation. Among three training sections, SComp and SBasic reveal strong dependency with CSL level, as the training score denoted by blue and gray symbols significantly increases from low CSL to medium/high CSL. SAdv, however, does not show such a tendency, implying that the core knowledge in SAdv may not be discriminable between different level of CSL groups. The effect of different CSL dimensions on the performance in training activity can be also seen in Figure 8. We found that when learners’ technology fluency and awareness increases, their performance and engagement in basic and comprehensive knowledge increases. The player’s willingness may not play an important role in the performance in the training activity, as the training score stands high even for low-willingness. In summary, we found that CSL could be regarded as an important indicator that reflects the students’ learning efficiency in seismology. Compared to willingness, technology fluency and awareness appear to play a more important role in the performance of training activity. The advanced knowledge gained during the training activity significantly correlates with the performance in EGCnrt activity, but no correlation with CSL scale is evident. This may be due to the fact that the advanced knowledge is relatively easy, so that even the low CSL group has fairly high training score. The coefficient of variation is found to be large (up to 0.97) in Figure 8, as a result of large cov in Scomp (gray triangles in low CSL group). Comparing with the dispersion in Sgame vs. Strain (Figure 7), the dispersion in CSL vs. Sgame (Table 4) and CSL vs. Strain (Figure 8) tends to be relatively large (up to > 0.90). This indicates that the association between CSL and training score/game performance is not as strong as the association between training score and game performance.
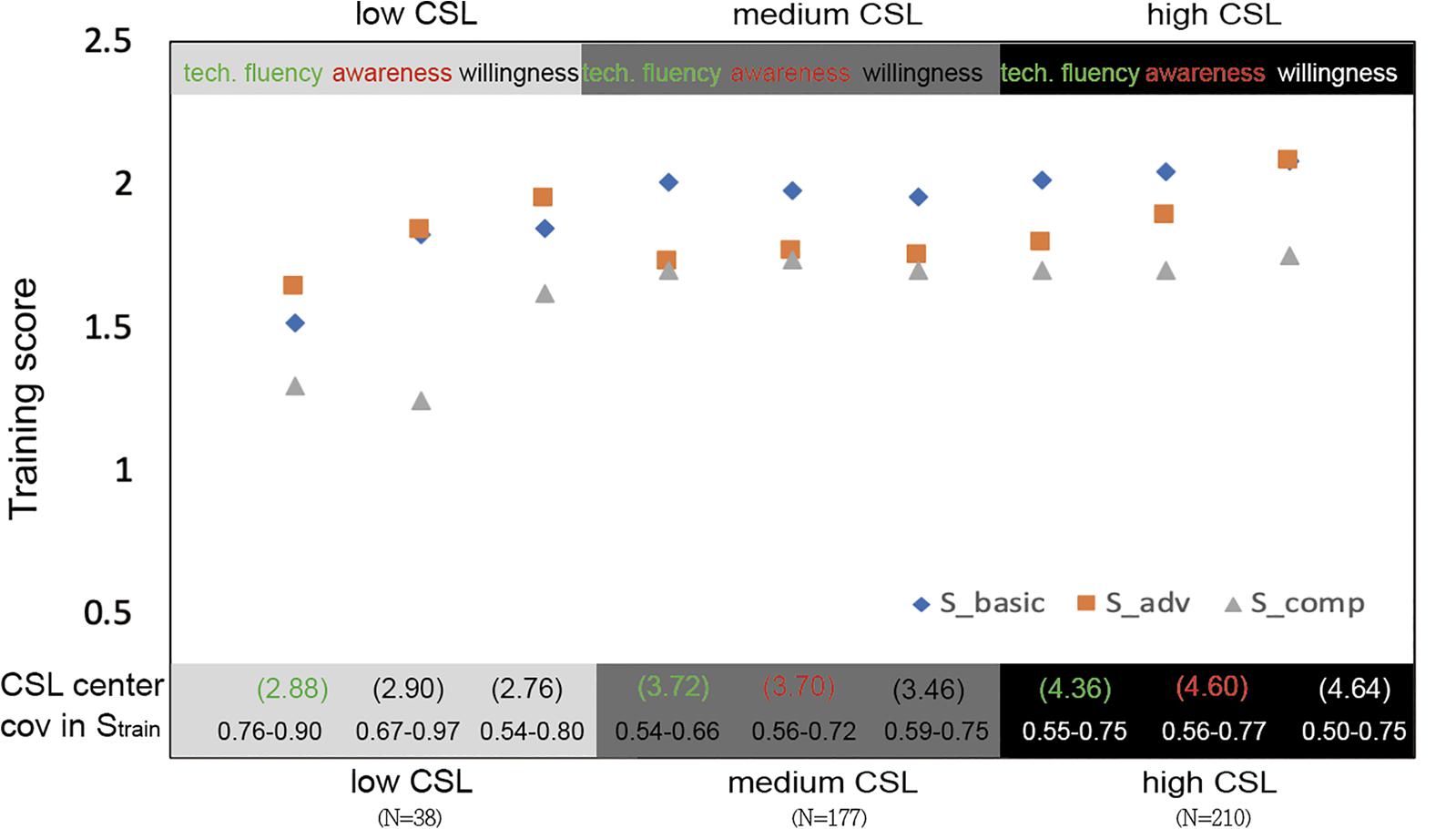
Figure 8. The relationship between the training scores and CSL. Low, medium, and high CSL is now divided into three dimensions (Technology fluency, awareness, and willingness), to correlate with training score. The number of samples that belong to groups of low, medium, and high CSL is 38, 177, and 210, respectively. In the bottom, the numbers in the first row (within the brackets) denote the clusters center for individual CSL group using k-means. The numbers in the second row denote the range of coefficient of variation in Sbasic, Sadv, and Scomp.
Discussion and Conclusion
By attempting to distribute the QCN in schools, the project of “Citizen Seismology in Taiwan” started with developing a near-real time earthquake game competition, followed by preparing the corresponding teachable units, and operating a series of outreach activities with formative assessment. The purposes are to encourage volunteers to interact with the real earthquake data and improve learning outcomes in earthquake science. Through professional development workshops with teachers, we found that teachers tend to lack confidence to run EGCnrt in their own classrooms. The barriers are mainly from the limited teaching hours in Earth Sciences (required course). In the exam-oriented education system in Taiwan, the teaching hours in high school are split into the five core knowledge units of astronomy, oceanography, atmospheric sciences, geology, and geophysics. The teachers often find it impossible to arrange additional hours to run all the teaching units associated with EGCnrt. Therefore, other than collaborating with primary and secondary educators (Liang et al., 2017), we also conducted pilot teaching in high school classrooms. Up to 2019, the total number of players reached 1319 since the EGCnrt was first online in late 2014. The time evolution of the number of players for all ML≥4 earthquakes on inland regions of Taiwan is shown in Figure 9. The corresponding location of earthquakes is shown in Figure 10. The largest number of players, 565, is reached for the 2016 ML6.4 Meinong earthquake, which is reinforced by a series of pilot courses intensely conducted during the summer of 2016 (as detailed in section 5), several months after the Meinong mainshock. Although the top-three players are announced real time on the EGCnrt platform and rewarded, only 23 out of 425 players who joined the 2016 summer outreach activities came back to the EGCnrt, to challenge with more earthquake data. These 23 players have an average score of 2, 1.96, and 1.96 for SBasic, SAdv and SComp, respectively, which are classified into the “high” performance in training activity illustrated by Figure 7. This again confirms the strong connection between the performance in training and EGCnrt activities. However, so far the number of players who returned to EGCnrt (outside the classroom) is too small to show a statistically meaningful trend in their CSL scale. In the future, more outreach activities are needed to investigate the personal characteristics for this group of volunteers. It is worthwhile to further study whether the long-term engagement is associated to the attributes proposed for CSL (e.g., awareness technology fluency, and willingness). To increase the return-rate and facilitate the long-term engagement, follow-up activity is necessary (Figure 6). We strongly encourage the host teachers/schools to run a “earthquake hunter” competition. For example, hunt for a major earthquake archived in the EGCnrt platform to report how much we know about this event. The nationwide competition with a strategic reward system can be expected as well to raise the public awareness to the EGCnrt platform.
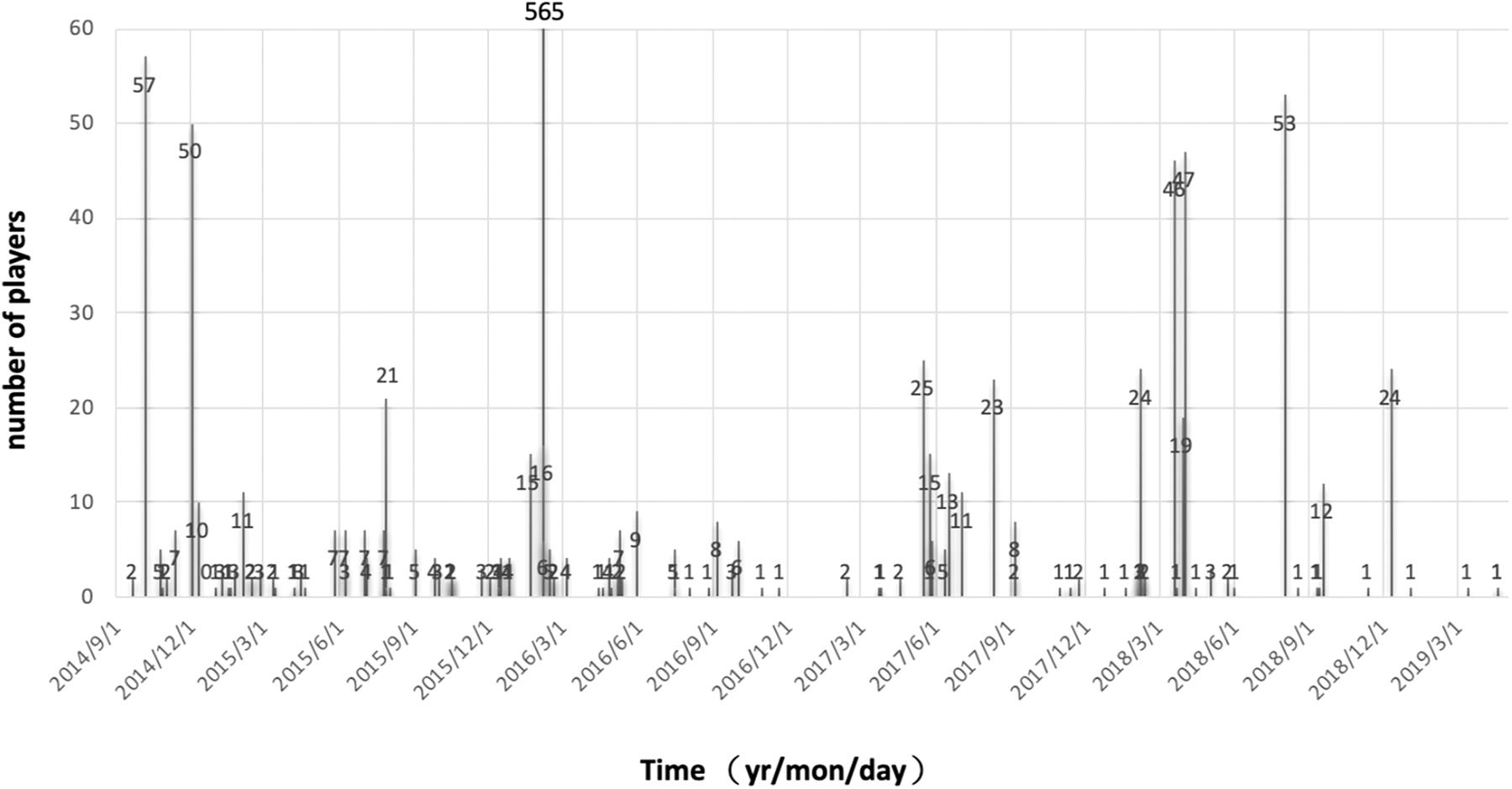
Figure 9. Number of players for the ML≥4 inland earthquakes since September 2014. For the 2016/2/6 Meinong earthquake, the number of volunteers reaches 565.
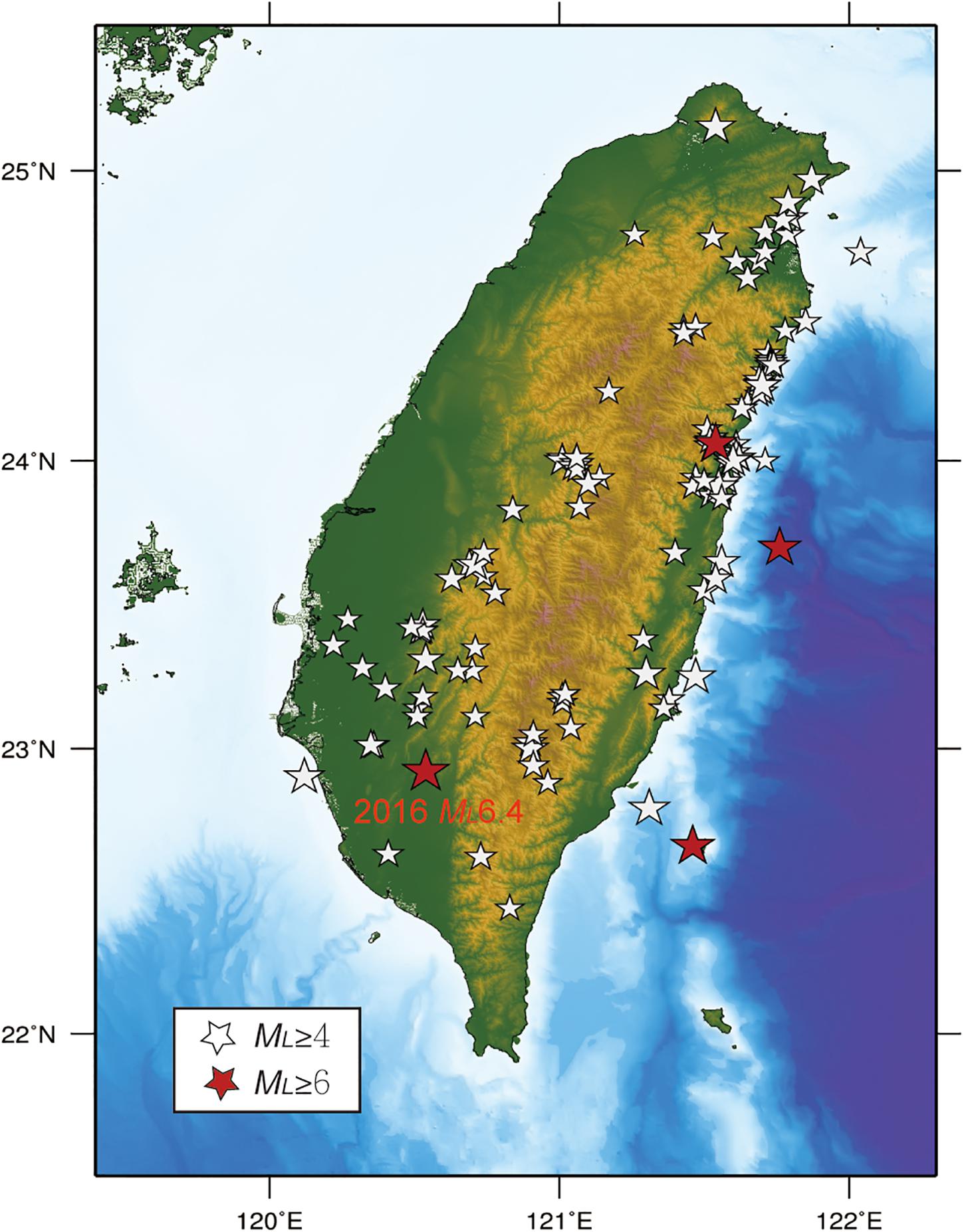
Figure 10. Location of the ML > 4 inland earthquakes in the earthquake game competition (the same events listed in Figure 9). White stars indicate events with magnitude greater than 4 but smaller than 6. Red stars indicate events with magnitude greater than 6.
Different from the traditional classroom, the EGCnrt program allows that students interact with each other across the room by discussing the skills needed in the activities and helping each other to get the certificates in training stage. From our observation, the most active discussion occurred when the questions appear on the screen after completing the certificate game(s) in each section. The students often asked around for the meaning of the questions and their connection with the certificate game(s). While the EGCnrt scores and ranking are shown online, a fun and competitive atmosphere can be naturally formed where the players attempt to outdo others and put each other into good humor. With such competition and lighter moments in class, the concentration in EGCnrt usually lasted longer than 30 mins. The host teachers were encouraged to participate in the activity as the observer. The interviews with the host teachers were conducted during and after the activity to learn (1) how do they teach earthquake science in the classroom, (2) what is the general behavior of students toward learning, (3) what is the problem encountered in teaching earthquake science, (4) how do the students behave differently when the competition platform is introduced in the classroom. Based on their observations, those players who showed excellent performance in EGCnrt (i.e., frequently helped others and attempted to understand the meaning of each game) are found to generally achieve low academic grades. Such an observation implies that the EGCnrt program has potential to foster learning and behavioral changes for the students who are less motivated in a traditional instruction classroom. In fact, mounting evidence has supported that the game-based elements in learning activities improve the learners’ motivation and engagement in educational environment (Dichev and Dicheva, 2017; Chen et al., 2019; Herrera et al., 2019). In this study, we present a game-based approach that uses real seismic data on an online digital platform, to extract information from students and assess the learning outcomes. This approach is composed of (1) pre-class Citizen Seismology Literacy (CSL) survey (2) half hour training activity with formative assessment (3) 1 h near-real time earthquake game competition (EGCnrt). Through statistical analysis, the above three elements are found to correlate with each other. We found that students with high CSL tend to have higher scores in game performance and training activity (especially for basic and comprehensive knowledge), however, such correlation does not follow a linear pattern. The score in the training activity is found to be more strongly correlated with performance in the EGCnrt, whereby the most significant factor is advanced knowledge. Such a result suggests that the CSL survey has potential to act as an indicator for the learning outcomes in seismology, while training program may efficiently improve the students’ performance in EGCnrt activity. In the near future, it is worthwhile to develop more dedicated CSL dimensions that might better depict the long-term engagement in citizen seismology throughout the programs and activities we offer.
Data Availability Statement
The datasets generated for this study are available on request to the corresponding author.
Ethics Statement
Ethical review and approval was not required for the study on human participants in accordance with the local legislation and institutional requirements (Center for Research Ethics and Research Ethics Committee in National Taiwan Normal University). The written consent was not required to participate in this study in accordance with the national legislation and the institutional requirement.
Author Contributions
W-TL developed the platform of near-real time earthquake game competition (EGCnrt) and maintained the QCN in Taiwan, while LW developed the Citizen Seismology Literacy. C-HL carried out the formative assessment. KC conducted the EGCnrt outreach activities in high schools and wrote the manuscript.
Funding
Support for this work was provided by MOST grant 108-2116-M-003-006-MY3 and 108-2511-H-033-003-MY2.
Conflict of Interest
The authors declare that the research was conducted in the absence of any commercial or financial relationships that could be construed as a potential conflict of interest.
Footnotes
References
Allen, R. M., Kong, W., and Martin-Short, R. (2019). The Myshake platform: a global vision for earthquake early warning. Pure Appl. Geophys. 177, 1699–1712.
Bossu, R., Laurin, M., Maze-Roux, G., Roussel, F., and Steed, R. (2015). The importance of smartphones as public earthquake-information tools and tools for the rapid engagement with eyewitnesses: a case study of the 2015 Nepal earthquake sequence. Seismol. Res. Lett. 86, 1587–1592.
Bossu, R., Mazet-Roux, V., Douet, S., Rives, S., Marin, S., and Aupetit, M. (2008). Internet users as seismic sensors for improved earthquake response. EOS Trans. 89, 225–226.
Bossu, R., Roussel, F., and Fallou, L. (2018). LastQuake: from rapid information to global seismic risk reduction. IInt. J. Dis. Risk Reduct. 28, 32–42. doi: 10.1016/J.IJDRR.2018.02.024
Chen, C. H., Law, V., and Huang, K. (2019). The roles of engagement and competition on learner’s performance and motivation in game-based science learning. Educ. Technol. Res. Dev. 67, 1003–1024.
Cochran, E. S., Lawrence, J. F., Christensen, C., and Jakka, R. (2009). The quake-catcher network: citizen science expanding seismic horizons. Seismol. Res. Lett. 80, 26–30.
Dichev, C., and Dicheva, D. (2017). Gamifying education: what is known, what is believed and what remains uncertain: a critical review. Int. J. Educ. Technol. High Educ. 14:9. doi: 10.1186/s41239-017-0042-5
Earle, P., Guy, M., Buckmaster, R., Ostrum, C., Horvath, S., and Vaughan, A. (2010). OMG earthquake! Can Twitter improve earthquake response? Seismol. Res. Lett. 81, 246–251.
Goldstein, P., Dodge, D., Firpo, M., and Minner, L. (2003). “SAC2000: Signal processing and analysis tools for seismologists and engineers, Invited contribution,” in The IASPEI International Handbook of Earthquake and Engineering Seismology, eds H. WHK Lee, P. C. Kanamori, and J. C. Kisslinger (London: Academic Press).
Guy, M., Earle, P., Ostrum, C., Gruchalla, K., and Horvath, S. (2010). Integration and dissemination of citizen reported and seismically derived earthquake information via social network technologies. Lect. Notes Comput. Sci. 6065, 42–53.
Herrera, R. F., Sanz, M. A., Montalban-Domingo, L., Garcia-Segura, T., and Pellicer, E. (2019). Impact of game-based learning on understanding lean construction principles. Sustainability 19:5294.
Huang, K. C., Kao, H., and Wu, Y. M. (2000). “The determination of ML – MW in Taiwan,” in Proceedings of the 8th Annual Meeting of Geophysical Society of China, Oxford 193–201.
Kong, Q., Allen, R. M., Schreier, L., and Kwon, Y. W. (2016). MyShake: a smartphone seismic network for earthquake early warning and beyond. Sci. Adv. 2:e1501055. doi: 10.1126/sciadv.1501055
Lee, S. J., Liang, W. T., Cheng, H. W., Tu, F. S., Ma, K. F., Tsuruoka, H., et al. (2013). Toward realtime regional earthquake simulation I: Real-time moment tensor monitoring (RMT) for regional events in Taiwan. Geophys. J. Int. 196, 432–446. doi: 10.1093/gji/ggt371
Liang, W. T., Chen, K. H., Wu, Y. F., Yen, E., and Chang, C. Y. (2016). Earthquake school in the cloud: citizen seismologists in Taiwan. Seismol. Res. Lett. 87:1. doi: 10.1785/0220150061
Liang, W. T., Lee, J. C., Chen, K. H., and Hsiao, N. C. (2017). Citizen earthquake science in Taiwan: from science to hazard mitigation. J. Disaster Res. 12, 1174–1181. doi: 10.20965/jdr.2017.p1174
MacQueen, J. B. (1967). “Some Methods for classification and Analysis of Multivariate Observations,” in Proceedings of 5-th Berkeley Symposium on Mathematical Statistics and Probability, (Berkeley: University of California Press), 281–297. doi: 10.1504/ijdmb.2015.067955
Ministry of Education (2014). Curriculum Guidelines of 12-Year Basic Education. Available online at: https://cirn.moe.edu.tw/Upload/file/32077/83646.pdf (accessed December 1, 2019).
Seno, T. (1977). The instantaneous rotation vector of the Philippine Sea Plate relative to the Eurasian plate. Tectonophysics 42, 209–226.
Seno, T., Stein, S., and Gripp, A. E. (1993). A model for the motion of the Phillippine Sea plate consistent with NUVEL-1 and geologic data. J. Geophys. Res. 98, 17941–17948.
Wald, D. J., Quitoriano, V., Worden, B., Hopper, M., and Dewey, J. W. (2011). USGS “Did you feel it?” internet-based macroseismic intensity maps. Ann. Geophys. 54, 688–707.
Wang, Y. J., Chan, C. H., Lee, Y. T., Ma, K. F., Shyu, J. B., Rau, R. J., et al. (2016). Probabilistic seismic hazard assessment for Taiwan. Terr. Atmos. Ocean. Sci. 27, 325–340. doi: 10.3319/TAO.2016.05.03.01(TEM)
Wu, Y. M., Chen, D. Y., Lin, T. L., Hsieh, C. Y., Chin, T. L., Chang, W. Y., et al. (2013). A high-density seismic network for earthquake early warning in Taiwan based on low cost sensors. Seismol. Res. Lett. 84, 1048–1054. doi: 10.1785/0220130085
Wu, Y. M., Liang, W. T., Mittal, H., Chao, W. A., Lin, C. H., Huang, B. S., et al. (2016). Performance of a low-cost earthquake warning system (P-alert) during the the 2016 ML6.4 Meinong (Taiwan) earthquake. Seismol. Res. Lett. 87, 1050–1059. doi: 10.1785/0220160058
Keywords: citizen seismology, QCN, earthquake game, citizen seismology literacy, formative assessment
Citation: Chen KH, Liang W-T, Lin C-H and Wu LY (2020) Citizen Seismology in Taiwan: Development, Outreach, and Formative Assessment of Near-Real Time Earthquake Game Competition Activities. Front. Earth Sci. 8:154. doi: 10.3389/feart.2020.00154
Received: 02 February 2020; Accepted: 27 April 2020;
Published: 29 May 2020.
Edited by:
Pier Paolo Bruno, University of Naples Federico II, ItalyReviewed by:
Elizabeth Cochran, United States Geological Survey (USGS), United StatesBernhard Maximilian Steinberger, Helmholtz Centre Potsdam, Germany
Copyright © 2020 Chen, Liang, Lin and Wu. This is an open-access article distributed under the terms of the Creative Commons Attribution License (CC BY). The use, distribution or reproduction in other forums is permitted, provided the original author(s) and the copyright owner(s) are credited and that the original publication in this journal is cited, in accordance with accepted academic practice. No use, distribution or reproduction is permitted which does not comply with these terms.
*Correspondence: Leon Yufeng Wu, leonwu@cycu.edu.tw