- 1Institute of Physics, Academia Sinica, Taipei, Taiwan
- 2Graduate Institute of Hydrological and Oceanic Sciences, National Central University, Taoyuan City, Taiwan
Even though the impact from large tsunamis are limited to coastal areas, these events are still devastating. Knowledge is crucial in minimizing the losses from natural disasters, as it can aid in creating better and more proactive preparation. Focusing on natural hazards’ mitigation in Asia, a collaboration between 10 Asian and two European countries, based on a deeper understanding approach, has been conducted since 2015. Deeper understanding aims to discover the physical mechanisms and drivers behind a hazard event. Innovative models and simulation facilities are developed correspondingly to achieve more accurate numerical simulations of the whole lifespan of the target event. An application framework composed from the knowledge, data, simulation facility, software tools, and case studies is designed to provide an advanced estimation of hazard risk and would be evolved progressively with more case studies and observation data. For tsunamis, based on the COMCOT (COrnell Multi-grid Coupled Tsunami Model), the simulation portal (iCOMCOT) implementing parallelized tsunami wave propagation calculation over distributed clouds had been established. The iCOMCOT system finished the simulation of the whole lifecycle of the 2011 Great East Japan Earthquake Tsunami in 1 min. In this regional collaboration, case studies on historical events and tsunami impact analysis were conducted. The goal is to capture the physical characteristics of the tsunami as much as possible, such as tsunami wave propagation, tsunami refraction, and tsunami run-up on land, as well as their drivers and root causes. The whole processes of the tsunami, from its initiation to its impacts in selected locations, then could be simulated accurately by iCOMCOT based on the scientific explorations and the quantitatively revised models. The Sulawesi Tsunami (2018) case is presented to demonstrate the processes of the deeper understanding approach and how to achieve the capacity building. At the same time, ways to take advantage of citizen science are also explored. The citizen science model is valuable in supporting data collection, such as data of run-up height, inundation range, flow depth, disruption information, impact area, from publication, news reports, and interviews from local people. According to experiences on case studies, suggestions to simplify and optimize the integration of the citizen science model with the deeper understanding approach to result in a lower operation cost are provided.
Introduction
Knowledge of the ever-changing earth is the foundation of effective hazard preparedness and mitigation. For example, significant progress in the fast determination of fault parameters and earthquake early warning signs based on discoveries of the physics and complexity of earthquakes in the past few decades have contributed to hazard mitigation (Kanamori, 2008). Tsunamis caused more casualties than other type of disaster in the past 30 years. The Indian Ocean tsunami in 2004, which is the deadliest tsunami in history, took the lives of over 230,000 people (Lay et al., 2005; Meltzner et al., 2006). The 2011 Tohoku tsunami, induced by the magnitude 9.0 undersea megathrust earthquake in Tohoku Japan, caused around 20,000 people to be killed (Fujiwara et al., 2011; Goto et al., 2011). Although tsunamis cannot be prevented, the impact of a tsunami can be alleviated through enhancement of knowledge, early warning, education, and adaptation.
After the largest and most damaging tsunami disasters, such as the 1960 Chile tsunami (Cisternas et al., 2005) and 1964 Alaska tsunami (Brocher et al., 2014), the international tsunami hazard community focused on hazard assessment and early warning systems based on numerical models in the 1970s (Vastano and Reid, 1967; Hwang and Divoky, 1970; Hwang et al., 1972). Stimulated by the 2004 Indian Ocean tsunami, tsunami hazard mapping and comprehensive risk understanding were improved significantly with the help of better numerical models, computational resources, and simulations (Spahn et al., 2010; Strunz et al., 2011). The extension of quantitative understanding of the vulnerability of various types of facilities on land due to tsunamis were intensified after the 2011 Japan tsunami (Suppasri et al., 2013; Muhari et al., 2015).
Discoveries of the physical mechanisms behind each tsunami event led directly to improved hazard preparedness and mitigation strategies after each disastrous tsunami (Løvholt et al., 2019). Unfortunately, tsunamis are quite rare. Only 11.32 tsunami events happen worldwide annually on average.1 In addition to their rarity, there are a few more obstacles to advancing the knowledge of hazards from detailed case studies. These obstacles include: (1) a limited period of consistent observations for large natural variability; (2) limited knowledge on the drivers and root causes of a disaster event; (3) the difficulty of performing experiments to understand the processes of a hazard on a similar scale; (4) the difficulty in transforming knowledge into simulation models and sharing this data.
The Disaster Mitigation Competence Centre (DMCC), supported by EGI-Engage2 (2015–2017) and EOSC-Hub3 (2018–2020), aligned with the UND project (deeper Understanding of Natural Disaster)4 (2018–2020), led by Academia Sinica and National Central University in Taiwan, adopt deeper understanding approaches to support regional disaster mitigation, including tsunamis, in collaboration with partners in nine Asian countries. The aim of DMCC is to achieve humanity’s sustainable development in Asia by reducing the impact of natural disasters. Centered on the primary barrier of disaster mitigation, DMCC aims to build up the capacity of disaster risk analysis through deeper understanding approaches. Deeper quantitative understanding and reproductions of the whole lifecycle of target hazards contribute directly to more accurate hazard risks analysis and could enhance prevention and mitigation strategies. DMCC has conducted case studies on tsunamis, storm surges, floods, forest fire monitoring, and dust transportation in partner countries and demonstrated the advantages of deeper understanding approaches using advanced numerical simulations. Additionally, local scientists and user communities were engaged in each case study collaboratively. A regional collaboration framework based on well-proved distributed computing infrastructure and technologies is enhanced in response to the requirements from case studies.
Based on the strategy to estimate potential risks quantitatively using what and how historical events happened, the collaboration platform supporting case study, numerical simulation, and information and knowledge sharing had been established. With the goal to push forward the knowledge and risk analysis capability of natural hazards, DMCC focuses on extending collaborations with more case studies, more partners, more types of hazards, and more observation data, as well as more analysis tools and methods based on local requirements. The open collaboration platform also targets the openness of the whole research lifecycle, such as open data, open access, open tools, and open standards, etc. The open science (Couch et al., 2019) principles and approaches will be adopted gradually. In order to foster the engagement of various local communities and to encourage good practice through collaborations with diverse parties, the citizen science (Blake et al., 2018) model has been incorporated to assist case studies, simulations, and training.
This paper is organized as follows. The deeper understanding approach and Sulawesi Tsunami case study are described in the next section. The citizen science model experiments from the collaboration platform for tsunami hazard risk analysis is explained in section “Application and Integration of Citizen Science Model.” Summary and future perspectives are wrapped up in the last section.
Deeper Understanding Approaches for Improving Hazard Risk Analysis
The goal of this study is to gather more data on disaster mitigation through the deeper understanding approach in the Asian region. Tsunamis are a target hazard since they are one of the primary common concerns in this region. Based on the deeper understanding approach, capacity development is implemented by case study, knowledge (root cause) discovery, and accurate simulation of the target hazard. Through the research on each case study, the hazard dynamics and science behind the event are explored. According to the discoveries, a more accurate numerical simulation is developed and the whole lifecycle of this event can be reproduced.
Tsunami Hazard Risk Analysis Through the Deeper Understanding Approach
The deeper understanding approach focuses on discovering the root causes and physical processes of target events. Simulation portals for tsunamis and meteorology-oriented processes are available for case studies in this collaboration. Case studies also allow researchers to learn from a specific historical event. Through deeper understanding activities, key factors to the simulation accuracy are investigated according to the lessons learned from each case study. As a direct outcome, processes of the whole life cycle of the target hazard could be reproduced more accurately. Both the enhanced simulation facility and the knowledge gained from case studies are shared through the application framework. The learning process and knowledge repository are key components of knowledge transformation and reuse. The application framework including those data, knowledge, workflow, simulation, and analysis facility, together with the configurations of each case study, becomes the foundation of an open collaboration platform for hazard risk analysis.
The typical case study workflow based on the deeper understanding approach starts from investigations of the root causes by the scientist group when the target case is confirmed. In order to reproduce the whole lifecycle of a target event accurately, the numerical model and parameterization will be fine-tuned according to the identified physical mechanisms. The expected outcome is not just more numerically accurate simulations but also optimized simulation procedures as well as observation data and initial and boundary conditions. Good quality observation data is crucial to the simulation process design and its results. New simulation portals will be developed accordingly, or the simulation facility would be revised following the requirements from case studies. The whole process will be carried out efficiently using scalable high-throughput computing schemes over the regional distributed cloud infrastructure. The workflow of tsunami event case studies based on the deeper understanding approach is depicted in Figure 1.
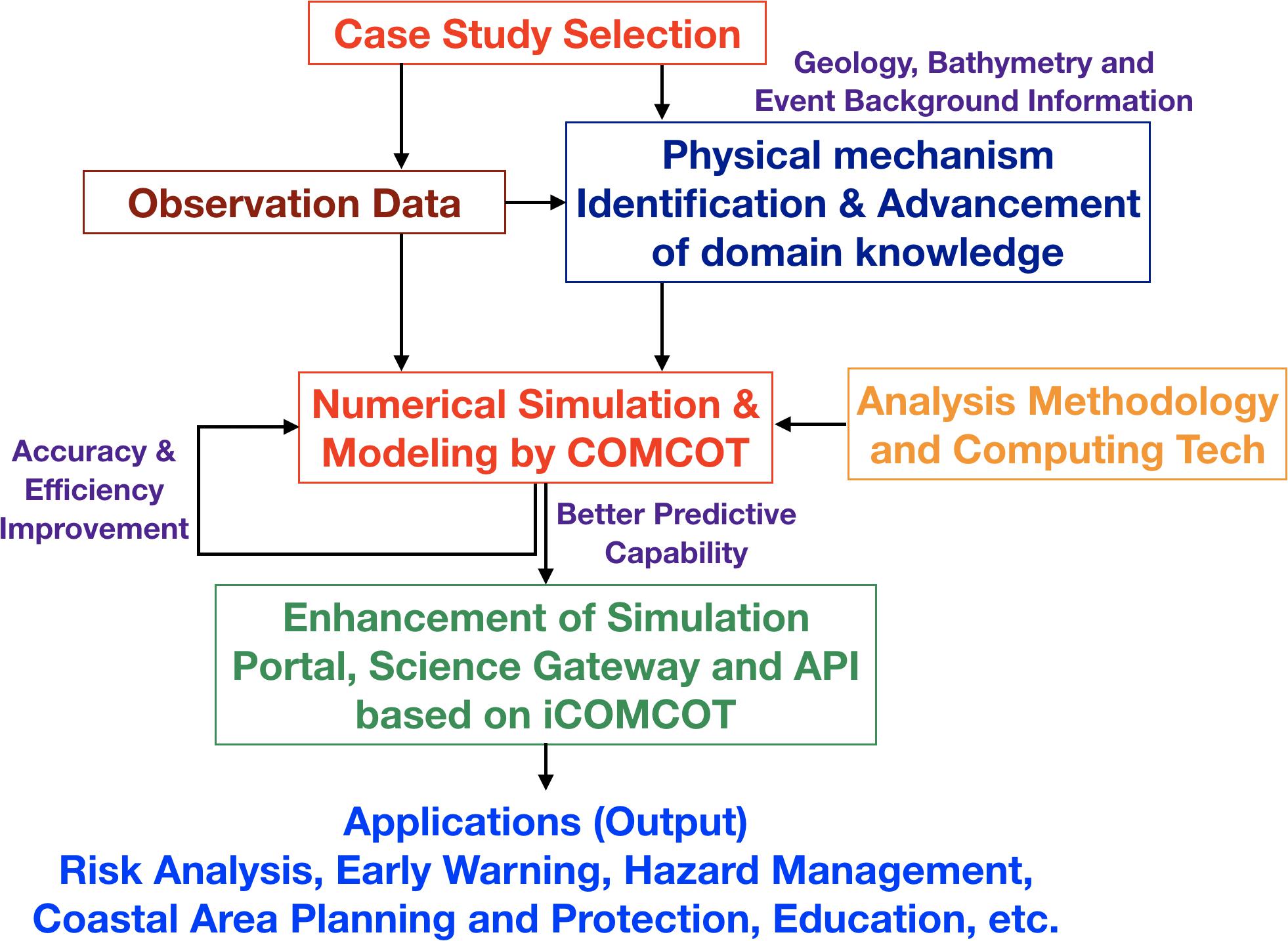
Figure 1. Typical workflow of a tsunami event case study based on the deeper understanding approach. The deeper understanding approach aims to advance the knowledge of the target hazard and develop an accurate numerical simulation model accordingly. The whole lifecycle of the event could be reproduced more accurately than before. All the data, numerical models, simulation portals, and the event simulation are the open accessible outcome of each case study. The numerical simulation model and results could be updated whenever there is new knowledge to the event, new observation data, and new applicable computing technologies.
iCOMCOT5 (Lin et al., 2015), which is based on the well-known COMCOT tsunami model (COrnell Multi-grid Coupled Tsunami Model) (Liu et al., 1998), is a tsunami simulation portal to support scalable and high-performance tsunami risk analysis over the cloud. Typically, when the earthquake source parameters (nine parameters: strike, dip, rake, focal depth, etc.) are confirmed and bathymetry data is in place, the initial free surface elevation could be evaluated by iCOMCOT in a few seconds. Afterwards, the whole processes of tsunami wave propagation are able to be efficiently simulated and the maximum tsunami wave height, tsunami arrival time, inundation, and the predicted time-history of the tsunami in certain locations will be generated in a few minutes. For example, in the case of the 2011 Japan earthquake, the iCOMCOT system with the spatial domain coverage of almost the whole Pacific Ocean can finish the simulation within 2 min. The impact analysis could be updated quickly whenever there is any change to the seismic source parameters, observation data, or bathymetry. The iCOMCOT simulation workflow and user interfaces, exemplified by an experimental case of the 2004 Indian Ocean earthquake and tsunami, is shown in Figure 2. The simulation results of iCOMCOT is also demonstrated in Figure 3.
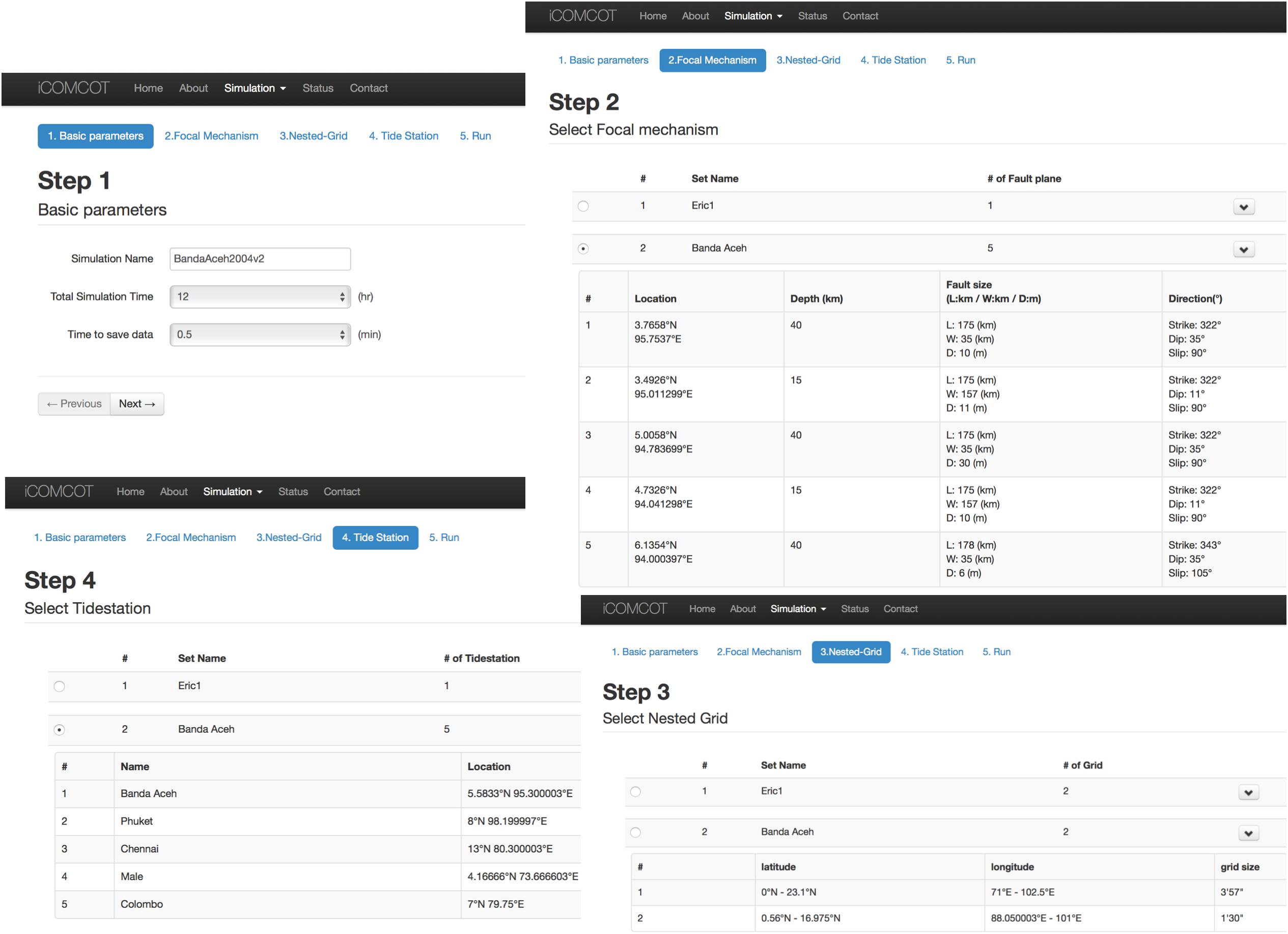
Figure 2. iCOMCOT simulation workflow exemplified by an experiment case of the 2004 Indian Ocean earthquake and tsunami. Before running the simulation, four configurations have to be defined in advance. Step 1 is to initialize the task by naming and defining the duration of the simulation time (in hours) and time interval (elapsed time between two saved simulation data). Step 2 is to set up focal mechanism parameters of the earthquake, including the epicenter location (latitude and longitude), depth, fault Size (length, width and depth in kilometer), and direction (strike, dip and rake in angles). Step 3 is to determine the nested grid domains of the simulation. Step 4 is to designate the observation sites (tidal stations).
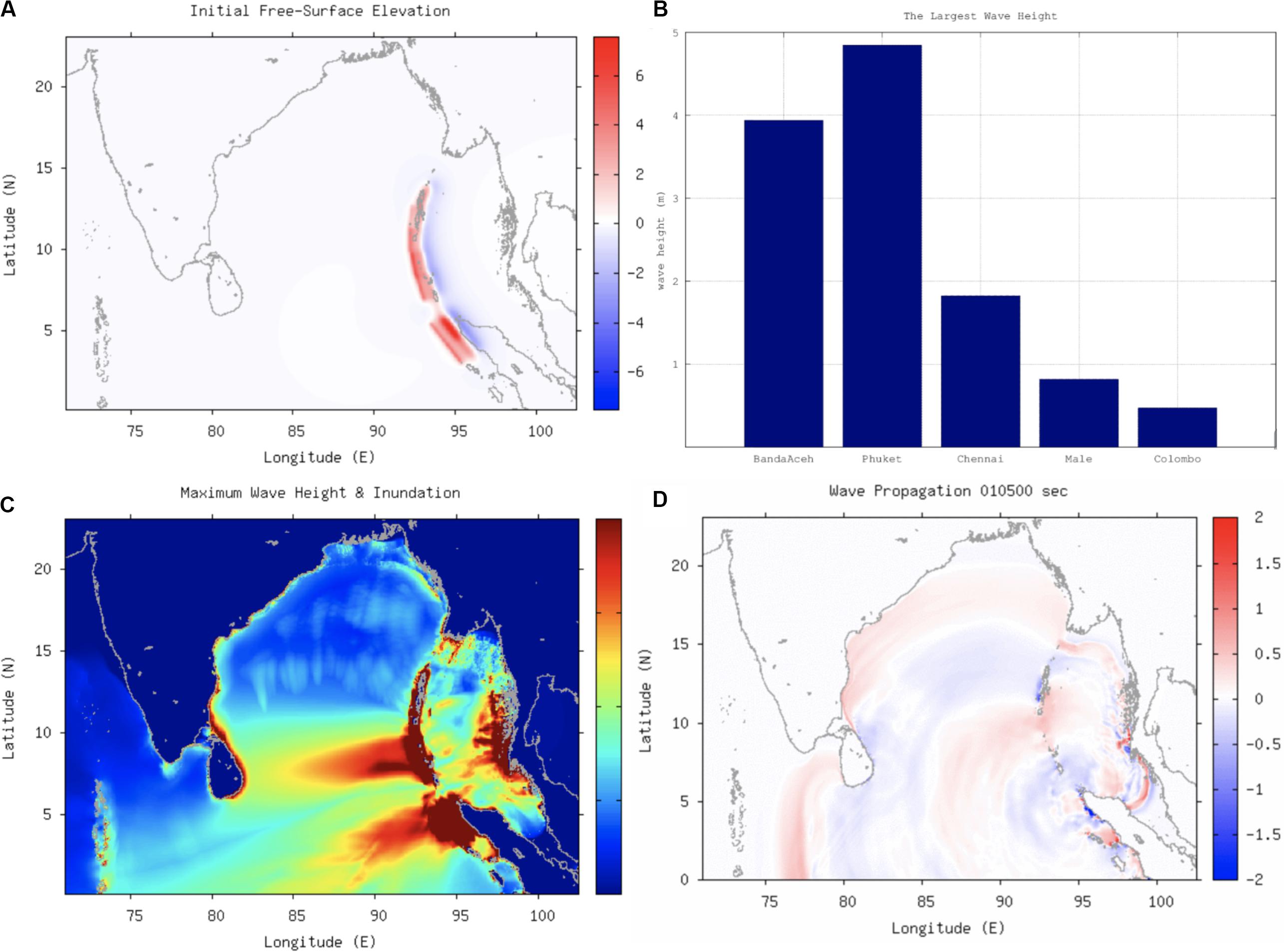
Figure 3. iCOMCOT simulation results of the experiment on the 2004 Indian Ocean earthquake and tsunami simulation experiment: (A) initial free surface elevation; (B) largest wave heights; (C) maximum wave height and inundation; (D) wave propagation animation.
iCOMCOT provides quantitative understanding of the vulnerability of tsunamis. The whole risk analysis workflow, platform (including the numerical simulation model and facility), and collaborations are improved progressively with more case studies, knowledge, and observation data. For each case study, facts (such as academic and government reports, news clips, videos, images, etc.) and observation data are collected. An accurate simulation facility is provided based on the deeper understanding approaches. The event itself is able to be reproduced and reinvestigated through the simulation portal. The numerical modeling and analysis methodology tool could be revised according to new findings and requirements. The ecosystem to investigate the tsunami event is available for applications of various aspects of disaster mitigation and preparedness, including education, awareness building, and communication, as well as coastal area planning and protection.
Sulawesi Tsunami (2018) Case Study
The 2018 Sulawesi Tsunami case study was conducted using DMCC collaborations because of the complexity in source identification and characterization. A very fast tsunami after a Mw 7.5 earthquake6 killed 4,340 people in northern Sulawesi. It is unusual that such a deadly tsunami was generated following a Mw 7.5 strike-slip earthquake rather than resulting from a big earthquake that happened in a long and straight trench. After confirming the capability of iCOMCOT from those disastrous tsunamis, it is a good opportunity for this collaboration to extend the deeper understanding approach to a different type of tsunami event.
Based on water-level records at the gauge station of Pantoloan, the tsunami propagated to the port of Pantoloan in just a few minutes after the mainshock and caused signals with higher frequency than the tides. The location of Pantoloan can be seen in Figure 4A. The Butterworth high-pass filter with the cutoff frequency of 6 h is adopted on the observed water-level data at Pantoloan to remove the tide effect. As can be seen from the de-tidal signal in Figure 4B, the tsunami amplitude reached 1.6 m at the port of Pantaloan. Based on video clips from the Internet,7 the tsunami waves arrived after about 3 min of the earthquake. For a seismogenic catastrophic tsunami, the wave is not possible to propagate to the city of Palu Bay within 3 min after the earthquake. More video analysis for the 2018 Sulawesi tsunami event can be found in Sunny et al. (2019) and Takagi et al. (2019).
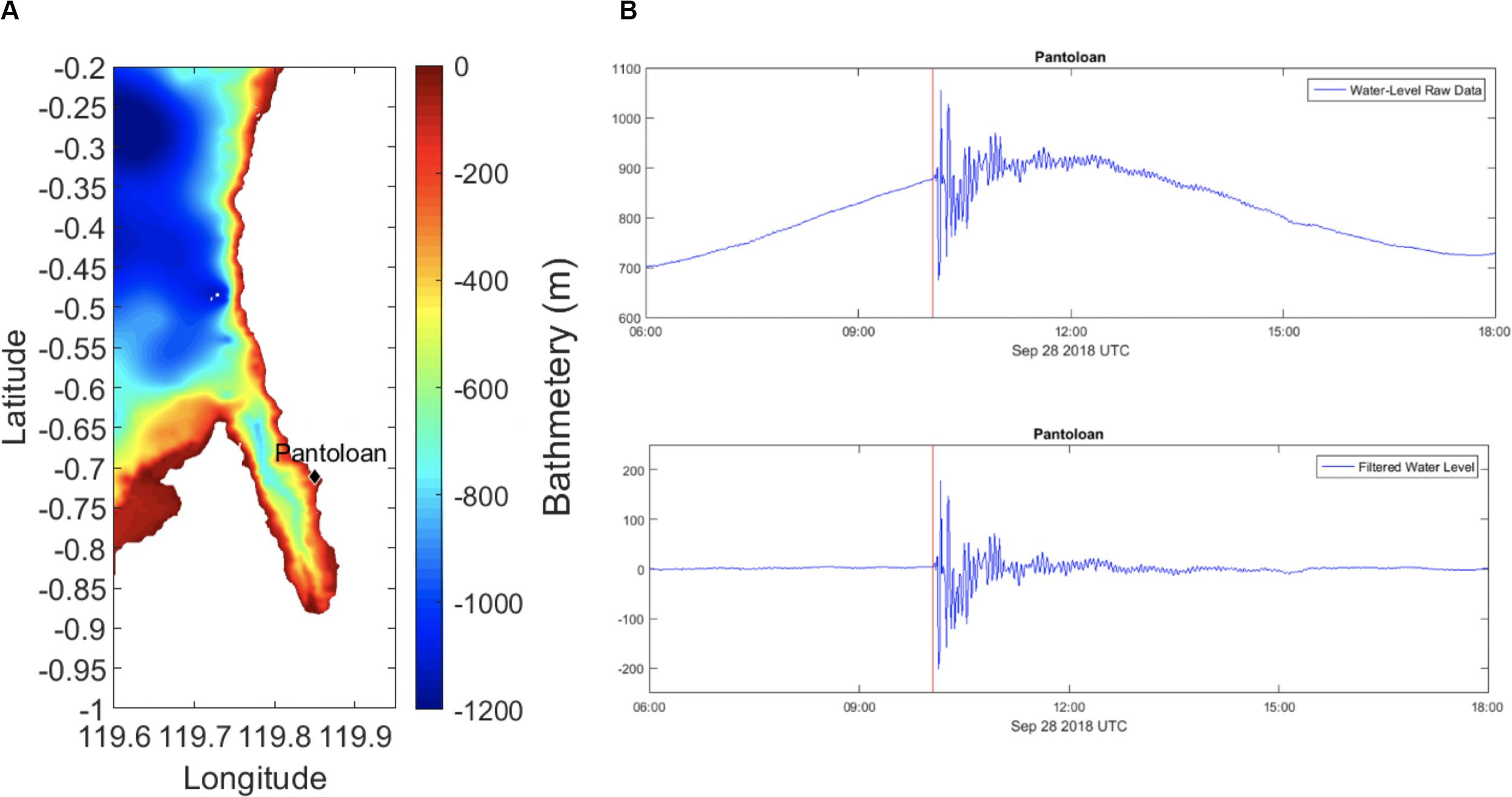
Figure 4. (A) Location of Pantoloan and the computational domain of COMCOT. The color shading depicts the water depth in meters. The station of Pantoloan located at (−0.7120 °E, 119.8554 °N) is labeled by the solid black diamond. The bathymetry data is from the BATNAS data bank of Badan Informasi Geospasial (BIG), Indonesia. (B) Upper panel: the observed wave level at Pantoloan in cm; lower panel: the time series of tsunami signal at Pantoloan by Butterworth high-pass filter with the cutoff frequency of 6 h in cm. The red line indicates the time of the mainshock of the earthquake (10:02 UTC, September 28, 2018).
According to the COMCOT simulation, the earthquake itself could only create a small tsunami with about 0.7 m wave height at Pantoloan and 0.65 m at Palu. Heidarzadeh et al. (2019) used a tsunami ray-tracing method to find out the locations of potential landslides, according to the discrepancy between observation data and simulations. Omira et al. (2019) conducted field surveys along the Palu Bay in the aftermath of the tsunami and identified several locations of the coastal landslides inside the bay. According to the scenario studies, we concluded tentatively that there are two tsunami sources in this event. A strike-slip earthquake tsunami dominates the impact outside the Palu Bay. Inside the Palu Bay, a landslide is one possible source. However, the locations of landslides are still under debate.
In this case study, the computational domain of COMCOT is (119.6–119.95 E) and (−1.0 to −0.2 N), as shown in Figure 4A. The nonlinear shallow water equations with the bottom friction are solved on the spherical coordinate. The Manning formula is used to model the bottom friction, and the Manning’s coefficient is 0.013, according to the analysis of Garzon and Ferreira (2016). In order to maintain numerical stability, the Courant–Friedrichs–Lewy (CFL) condition needs to be fulfilled. In COMCOT, the grid size is 0.05 arc-minutes (about 100 m) and the time step is set as 0.05 s. The bathymetry data used in the simulation is from the BATNAS data bank of Badan Informasi Geospasial (BIG), Indonesia. The resolution of BATNAS is 6 arc-seconds (0.1 arc-minutes).
The initial free surface elevation for the proposed scenario of a landslide tsunami at the mouth of Palu Bay is depicted in Figure 5. The landslide is assumed to move offshore because the crest of the tsunami waves arrived first, and were followed by the trough according to the observed tsunami signals at Pantoloan. The length and width of the uplift of the water surface disturbances are 4 and 8 km, respectively. The depression of the water surface has the same dimension as the uplift – 3 m uplift of water surface disturbances and – 4 m depression.
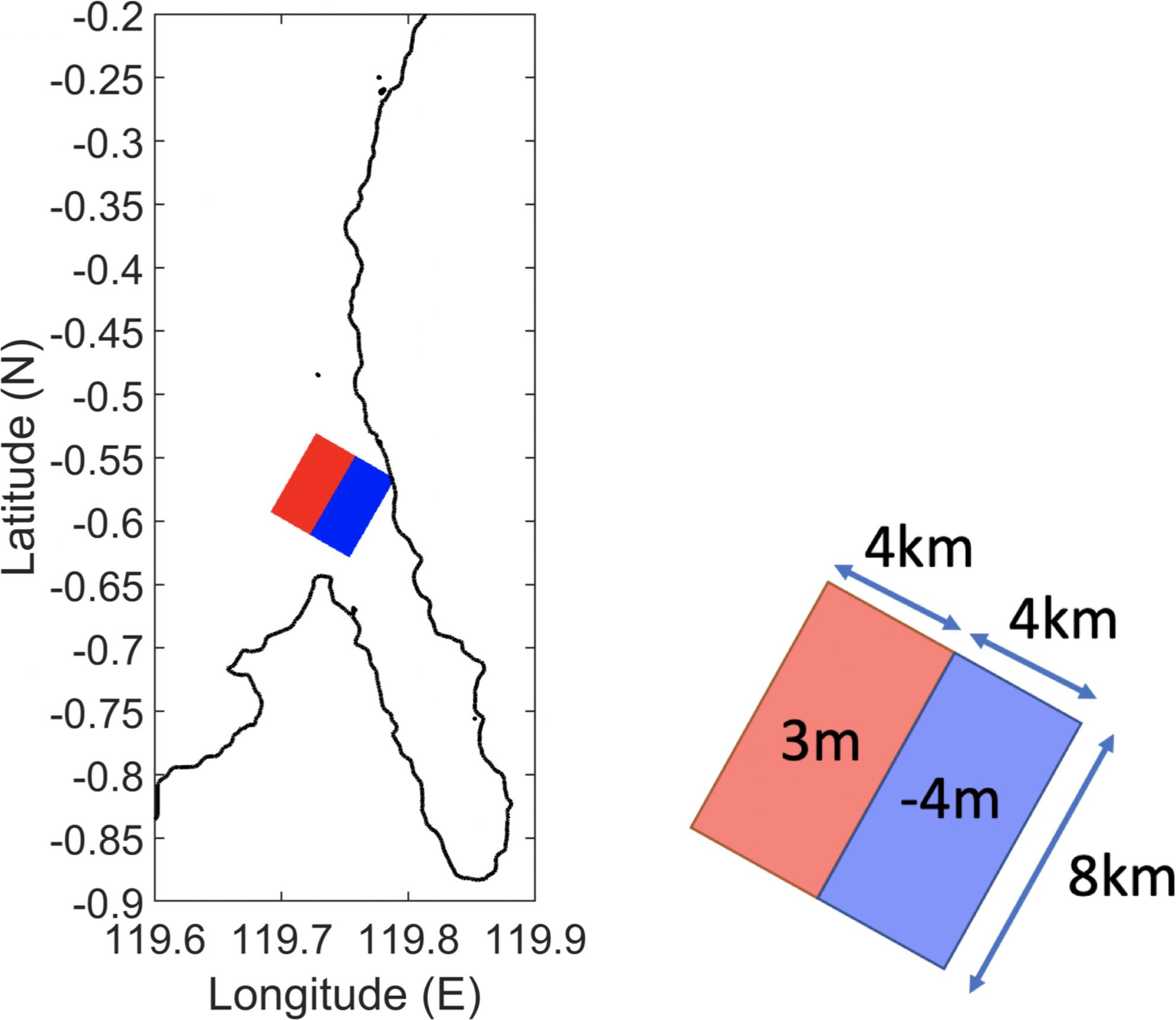
Figure 5. The initial free surface elevation for the proposed potential submarine landslide tsunami off the mouth of Palu Bay. The color shading indicates the water surface disturbances by the landslide: red – the uplift of the water surface disturbance; blue – the depression of the water level disturbance. The dimension of the initial free surface elevation is shown in the right panel.
The snapshots of computed tsunami propagation are shown in Figure 6. In the first 1–3 min after the earthquake, the leading negative tsunami waves propagate into Palu Bay. After 4 min of the mainshock, the tsunami wave front arrives in Pantoloan. Later, after 6 min of the mainshock, the positive tsunami waves arrive at Pantoloan.
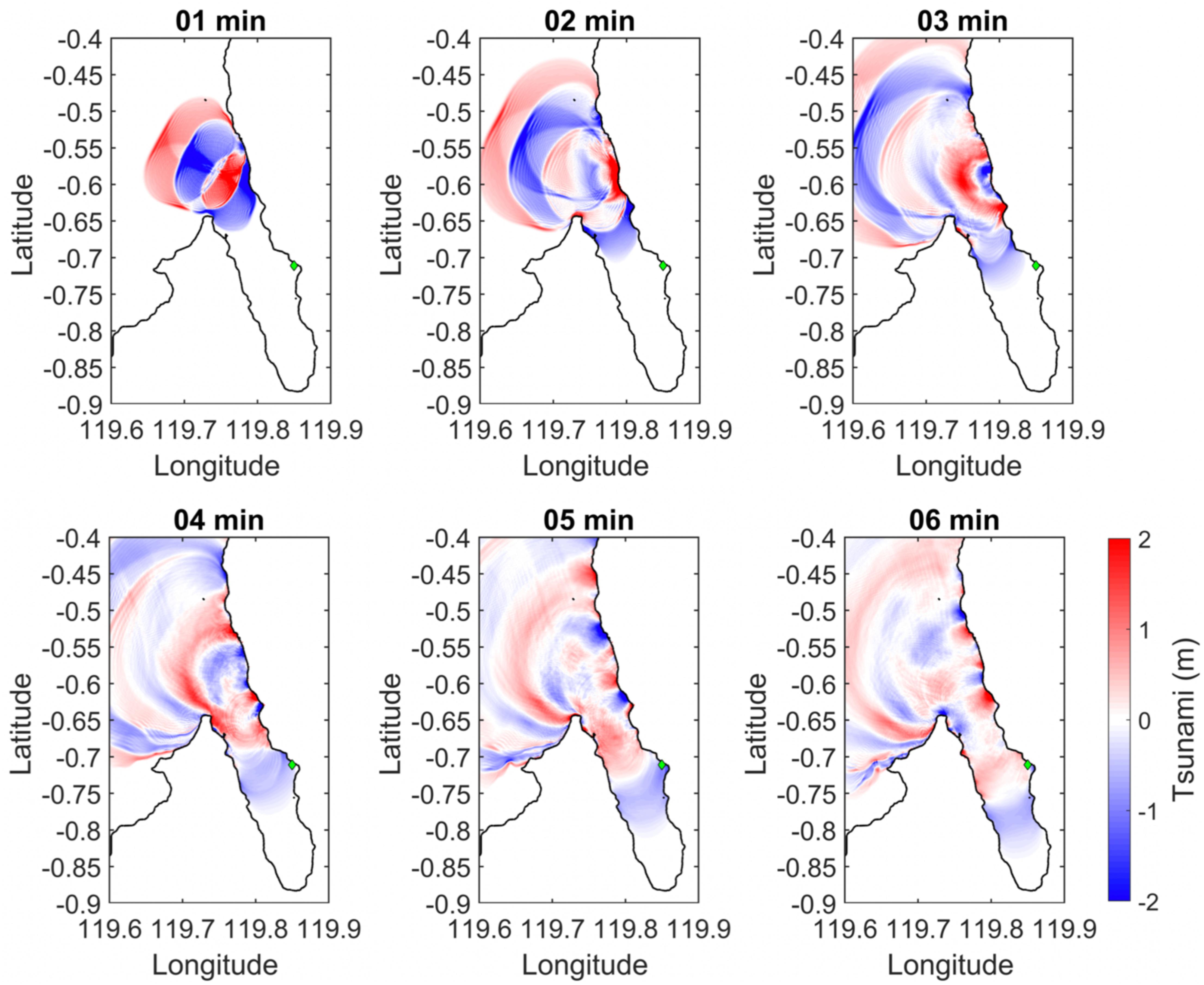
Figure 6. The snapshots of the tsunami propagation. The color shading indicates the free surface elevation in meters. The green diamond indicates the location of Pantoloan. The time depicts the instants after the mainshock of the earthquake (10:02 UTC, September 28, 2018).
Results of the simulated maximum free surface elevation is shown in Figure 7a. Near Pantoloan, the maximum free surface elevation caused by the proposed landslide tsunami is 3.0 m. Inside Palu Bay, the largest maximum free surface elevation is 3.8 m which occurs at the end of Palu Bay. In comparison to the observation data in Pantoloan, as shown in Figure 7b, the numerical results capture very well the first trough of the tsunami waves arriving at Pantoloan after 5 min of the mainshock. After 7 min of the earthquake, the simulation gives a good prediction on the first crest of the tsunami waves. At about 10 min, the simulated results give a faster prediction on the second trough, around 2 min faster than observed tsunami. Similar patterns can be found after 10 min.
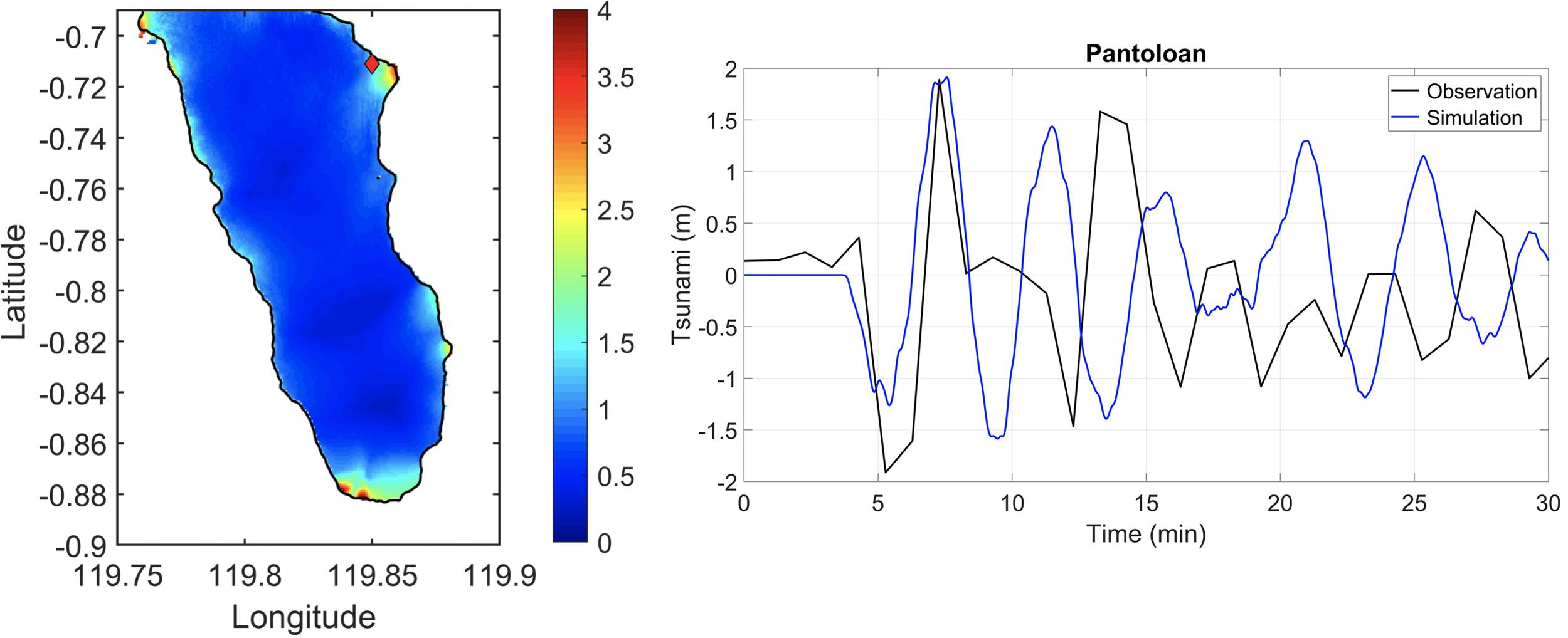
Figure 7. (Left panel) The computed maximum free surface elevation. The color shading indicates the maximum free surface elevation in meter. This plot has been zoomed in from the original computational domain to the regions of Palu Bay. The solid red diamond indicates the location of Pantoloan. (Right panel) The comparison between the simulated tsunami and the observed tsunami at Pantoloan. The blue line indicates the numerical results of COMCOT, and the black line indicates the observed tsunami. Note that the observed tsunami has been filtered by the high-pass filter with the 6-h cutoff frequency. The y-axis indicates the time after the mainshock of the earthquake (10:02 UTC, September 28, 2018).
An accurate and timely forecast of a hazard is the most practical solution to minimize potential losses. Development of the early warning system for the Indian Ocean tsunami had also kicked off in our collaborations with partner countries around the region. Based on the experiences of tsunami risk assessment (Wu and Huang, 2009; Wu, 2013) and early warning system development for the South China Sea (Lin et al., 2015), the large-scale trench-typed tsunami threats sources in the Indian Ocean will be explored carefully. Historical tsunami events in the Indian Ocean will be analyzed quantitatively. Potential sources, especially in the Java Trench, will be identified and their rupture length and width, the scale of seismic moment, the slip, and as the dip angle, etc., will be elaborated as well. iCOMCOT will be applied to simulate tsunami propagation, run-up, and inundation with multi-nested grids for a complete simulation of the process of a tsunami from the beginning to the inundation.
The deeper understanding approach has been advancing the knowledge of natural hazards and supporting more accurate risk analysis on selected case studies using simulation portals. Moreover, all the analysis is reproducible with shared data, knowledge, event background information, and analysis tools. All these cases could be further reinvestigated with innovative numerical models and updated data whenever they are available. Both the awareness of hazard risk and the capacity for disaster mitigation could be upgraded from all these outcomes through new case studies, education, communication, and better planning and adaption.
Application and Integration of Citizen Science Model
The practical framework for hazard risk evaluation based on the deeper understanding approach has been built and verified by several case studies of various types of hazards in different countries. The framework keeps on evolving progressively with more cases, including tsunami hazards, using iCOMCOT in this regional collaboration (Yen et al., 2018). New observation data and knowledge of the tsunami impacts and tsunami source characteristics will lead to the innovation of numerical models, risk estimation workflow, and analysis facility. Efficiency and functionality will also be advanced by user experiences and by adopting new information and communication technologies.
The collaboration takes advantage of the collective intelligence paradigm, such as the citizen science model, to extensively collect data of diversified data types and locations for tsunami case studies. In order to reduce the losses from future tsunamis, impact analysis and protection of local focus need to be enhanced from the lessons of historical events. Additionally, validation of impact analysis, education, early warning development, test of the workflow and functionality, and locally focused case studies all could benefit from the citizen science model. The framework integrating the deeper understanding approach and citizen science model is illustrated in Figure 8.
In accordance with the deeper understanding approach and the example of the Sulawesi Tsunami, basic steps of a case study include: (1) data collection, (2) source characterization, (3) simulation and verification, (4) impact analysis, (5) reproducibility test, and (6) training. The citizen science model is usually deployed to fill resource gaps or to extend participation and awareness. Observation data of gauges and local monitoring systems, bathymetry data, official reports and announcements, news, videos clips, information from social media, and field survey data from many countries are collected by partners in different countries since the impact region of a big tsunami, including both the near-field and far-field area, are very large. Based on the lessons learned from case studies, every partner could reproduce the tsunami hazard using iCOMCOT by focusing on different local impacts and local environments repeatedly. For instance, it could encourage intensified protection of important facilities, such as nuclear power plants, near the coastline and coastal region community planning. Influences of strong currents and floating debris from the possible wave height and inundation from a tsunami also have to be inspected seriously. Another example is the tsunami-induced coastal changes as proposed by the Indonesian partners. Volunteers around the world are able to learn the details of tsunami events or to validate the simulation results using iCOMCOT with the local data. The scientist group will review the report and initiate a new investigation task force if there are any significant new findings. Numerical simulations and case studies are very practical for the education of tsunami hazard mitigation in understanding the possible impacts and the processes. Partners also delivered knowledge of tsunamis through customized experiment designs using the numerical simulations. Training on using iCOMCOT to find out the possibilities of tsunami hazards according to the local environment and concerns are also a common use case in education by partners. Straight-forwardly, workflow and functions of the numerical simulation and risk analysis process could be improved from those various applications. Partners are encouraged to develop new analysis tools or applications and integrate these into the framework according to their local requirements.
Furthermore, all these outcomes, including education materials, new case studies, local simulations and experiments, new observation data, test results, new tools, and feed-back from local people, will be compiled into the collaboration platform by design. All materials in the platform will be shared based on the FAIR principle: findable, accessible, interpretable, and reusable.
The citizen science model is helpful in extending capacity, gaining popularity, and strengthening the practices of hazard mitigation from the experiences of the regional collaborators led by DMCC. Many hazard mitigation tasks could benefit from the citizen science model, but different strategies and implementation approaches are necessary. The citizen science model could be implemented by limited partners or volunteers instead of the general public only according to the goals and stages. For the deeper understanding approach in our collaborations, many tasks could be reinforced by the citizen science model in numerous forms, including data collection, impact analysis validation, education, early warning development support, testing, and local focused analysis. To develop the capacity, the collaboration initiated several representative or disastrous case studies of each selected hazard type using the simulation portals and collaboration platform. Subsequently, the collaboration is able to support more partners to carry out their own case studies using the platform. The subject matter is to engage local communities to initiate more case studies focusing on the local environment. With the strong motivation and obligations in our collaborations, the partners could achieve high quality in each task and take the roles as local coordinators of local groups and collaboration networks as well as dissemination and training events. On the other hand, the citizen science model was extended to trained volunteers on those exemplar case studies to further enrichment of the case studies. Trained volunteers are not just able to support new case studies but could also extend the capacity and strengthen the practices.
In terms of indicators for citizen science model implementation on tsunami hazard mitigation, such as the best achievable quality, it is suggested to extend the model to the general public step by step, from collaboration partners to trained volunteers first. Moreover, collaboration frameworks, simulation tools, well-defined workflow and guidelines, and clear goals for each task have to be in place before widely calling for volunteers. In the public volunteer level of citizen science model, the first issue is the quality of work is irregular even when all the previous mentioned prerequisites are available. Revalidation of contributions from public volunteers are required, although they are helpful in supporting data collection, local surveys, testing, and compilation of education materials. In coping with these, tools such as auto-checker, smart filter, error reduction assistance according to common faults, validation schemes, and intelligent tests are valuable to enhance the quality of citizen science model applications. Standard operation procedures, atomic task items, and intelligent error detection and error correction tools based on experiences are all necessary supporting tools. For the case study designed through our collaborations, the partner-only model is a special case of citizen science instances. Trained partners motivated by common goals could achieve expected throughput under constraints of cost and time. Applications could define indicators of the citizen science model according to their goals and limitations. Experiences and suggestions of implementing the citizen science model on tsunami hazard mitigation through our collaborations is summarized in Table 1.
Except for the purposes of science, hazard mitigation, including tsunamis, should attract many contributions from volunteers because of its significance for sustainability and public welfare. In our collaborations, all partners are trained to conduct more case studies according to their local needs using the collaboration platform. By learning from case studies, partners could develop their own customized collaboration model implementing the deeper understanding approach over the platform. The collaboration platform is a distributed computing infrastructure and will be advanced based on the requirements of applications and experiences of case studies. The citizen science model is applicable because people are eager to contribute to the safety and sustainability of their own community. In general, with a clear defined workflow, supporting tools, and training, the citizen science model could aggregate a lot of helpful input, test results, and even innovations to the local needs from knowledge advancement and risk assessments to the adaptation.
In general, the citizen science model is useful for enriching science with participants’ know-how and allowing for new points of view in addition to sharing time-consuming and labor-intensive tasks. It also makes science more open and encourages trust in and use of knowledge-based decision making. The deeper understanding approach and the collaboration platform are prepared to integrate contributions from broader types of participants in the production of tsunami disaster mitigation knowledge using the citizen science model. To the better advantage of the citizen science model, our strategy is to extend communication and complement this with extensive communities through education, discussion, and case studies over the distributed cloud platform with the iCOMCOT simulation portal.
Summary and Future Perspectives
In this study, the integration of the citizen science model to the deeper understanding approach using the 2018 Sulawesi Tsunami case study is demonstrated. Based on the goal of capacity building, the ecosystem (domain experts, supporting infrastructure, application providers, and collaboration platform) and good projects should be established first. Utilizing the citizen science model for disaster mitigation allows for the enhancement of communication and for support to be gained in the production of scientific knowledge with wider communities. Educating participants on a broader understanding of the scientific process and the nature of science through case studies could lead to a better quality and production of scientific workflow. Mutual learning between scientists and the public is essential in establishing trust with the scientific community. Effectiveness of disaster mitigation could also be improved from making people excited about science through citizen science projects. Opening the data, methodologies, outcomes, tools, projects, and labs, etc. are valuable ways to enable the transformation of science and research and facilitate innovations.
Based on the experiences gained from the collaboration, it has become apparent that a deeper understanding of the tsunami source characteristics, the whole process during its lifecycle, and its impacts especially in light of local environments, is essential to tsunami hazard mitigation. Extensive risk analysis and reinvestigation according to the new knowledge of the tsunami source and new observation data are necessary to update the risk assessment and preparedness in time. The citizen science model is one of the most feasible ways to motivate and aggregate intelligence from volunteers collectively.
To ensure the citizen science model could be implemented effectively, those necessary and supporting components have to be available first. In this regional collaboration, the citizen science model is implemented from partners only in the beginning, because the case study workflow and data policy have to be confirmed and the collaboration platform has to be in place at first. Using the citizen science model for data collection (including observation data, geological data, multimedia data from the mass media and social media, etc.) is very helpful. However, data quality requirements have to be clarified in the beginning. For example, data quality and evaluation methodology (including data inconsistency resolution), credibility, legality (licensing, ownership, privacy, liability and copyright), sustainability of the system or project must be established before the project begins. Citizen science could also be deployed for the validation of impact analysis, education, early warning development, testing of the workflow and functionality, and locally focused case studies according to the pilot experiments of our collaborations together with our Asian partners.
The deeper understanding approach has been proven to be able to advance the knowledge of hazards and to build up a better capacity of natural hazard risk analysis from case studies. In this regional collaboration, the goal of capacity building based on the deeper understanding approach is then implemented mainly on the basis of the following pillars: (1) improvement of numerical simulations through the integration of root cause discovery, (2) improvement of risk analysis by accurate numerical simulation, (3) capability to conduct a new case study from each partner, (4) case study reproduction using the simulation portals and collaboration platform, and (5) sharing of knowledge from case studies. Collective intelligence mechanisms could be integrated in the workflow of each perspective. The collaboration platform will grow with more case studies and more contributions from partners and volunteers from data, tools, analysis methodology, and knowledge.
Data Availability Statement
The datasets generated for this study are available on request to the corresponding author. The bathymetry data of BATNAS and the tide-gauge data at Pantoloan belong to Badan Informasi Geospasial (BIG), Indonesia. BATNAS data is publicly available on http://tides.big.go.id/DEMNAS/ and the information of Pantoloan tidal gauge can be referred to http://tides.big.go.id:8888/dash/.
Author Contributions
EY, SL, and T-RW designed the research and coordinated the collaborations. T-RW, Y-LT, and M-JC conducted the tsunami case studies and result analysis. EY and SL coordinated the development of iCOMCOT and data analysis. EY and Y-LT wrote the manuscript. All authors contributed to the article and approved the submitted version.
Funding
The Disaster Mitigation Competence Centre (DMCC) is partially supported by the EGI-Engage Project (co-funded by the European Union Horizon 2020 Programme under grant number 654142 during 2015 and 2017) with partners in the Philippines, Malaysia, Indonesia, and coordinated by the Academia Sinica Grid Computing Centre in Taiwan. DMCC+ is partially supported by the EOSC-Hub project (funded by the European Union’s Horizon 2020 Research and Innovation Programme under grant agreement No. 777536). The Deeper Understanding of Natural Disaster – Instrumental to Disaster Mitigation (UND) project is funded by Asi@Connect under grant agreement No. Asi@Connect-18-066. All these projects are supported by the Academia Sinica and National Central University in Taiwan.
Conflict of Interest
The authors declare that the research was conducted in the absence of any commercial or financial relationships that could be construed as a potential conflict of interest.
Acknowledgments
We thank all the partners who provided event data and work together on the case studies and collaboration platform. We also gratefully acknowledge Dr. Veerachai Tanpipat of Hydro-Informatics Institute (HII), Ministry of Higher Education, Science, Research, and Innovation (MHESI) in Thailand for his coordination of observation data collection on the 2018 Sulawesi Tsunami event; Dr. Philip L.-F. Liu and Dr. Pablo Higuera for advices on bathymetry data and the environment of the Palu Bay; and Dr. Xiaming Wang and Dr. Tung-Cheng Ho for discussions of data post-processing on the observation data of Pantaloon.
Footnotes
- ^ Global Historical Tsunami Database: https://www.ngdc.noaa.gov/hazard/tsu_db.shtml.
- ^ EGI-Engage: https://wiki.egi.eu/wiki/EGI-Engage:Main_Page.
- ^ EOSC-Hub: https://www.eosc-hub.eu.
- ^ UND Project: http://und.twgrid.org.
- ^ iCOMCOT Simulation Portal: http://icomcot.twgrid.org.
- ^ 2018 Sulawesi Earthquake information from USGS: https://earthquake.usgs.gov/earthquakes/eventpage/us1000h3p4/dyfi/intensity.
- ^ For example, a CCTV record at Palu Bay from YouTube, https://www.youtube.com/watch?v=8qaP7BCN87M.
References
Blake, D., Johnston, D., Leonard, G., McLaren, L., and Becker, J. (2018). A citizen science initiative to understand community response to the Kaikoura Earthquake and tsunami warning in Petone and Eastbourne, Wellington, Aotearoa/New Zealand. Bull. Seismol. Soc. Am. 108, 1807–1817. doi: 10.1785/0120170292
Brocher, T. M., Filson, J. R., Fuis, G. S., Haeussler, P. J., Holzer, T. L., Plafker, G., et al. (2014). The 1964 Great Alaska Earthquake and tsunamis-A modern perspective and enduring legacies: U.S. Geological. Surv. Fact Sheet 6, 2014–3018. doi: 10.3133/fs20143018
Cisternas, M., Atwater, B. F., Torrejón, F., Sawai, Y., Machuca, G., Lagos, M., et al. (2005). Predecessors of the giant 1960 Chile earthquake. Nature 437, 404–407. doi: 10.1038/nature03943
Couch, J., Theisz, K., and Gillanders, E. (2019). “Engaging the Public: Citizen Science,” in In Strategies for Team Science Success. eds K. Hall, A. Vogel, and R. Croyle (Berlin: Springer).
Fujiwara, T., Kodaira, S., No, T., Kaiho, Y., Takahashi, N., Kaneda, Y., et al. (2011). The 2011 Tohoku-oki earthquake: displacement reaching the trench axis. Science 334:1240. doi: 10.1126/science.1211554
Garzon, J. L., and Ferreira, C. M. (2016). Storm surge modeling in large estuaries: sensitivity analyses to parameters and physical processes in the Chesapeake Bay. J. Mar. Sci. Engin. 4:45. doi: 10.3390/jmse4030045
Goto, K., Chagué-Goff, C., Fujino, S., Goff, J., Jaffe, B., Nishimura, Y., et al. (2011). New insights of tsunami hazard from the 2011 Tohoku-oki event. Mar. Geol. 290, 46–50. doi: 10.1016/j.margeo.2011.10.004
Heidarzadeh, M., Muhari, A., and Wijanarto, A. B. (2019). Insights on the source of the 28 September 2018 Sulawesi tsunami, Indonesia based on spectral analyses and numerical simulations. Pure Appl. Geophys. 176, 25–43. doi: 10.1007/s00024-018-2065-9
Hwang, L.-S., Butler, H. L., and Divoky, D. J. (1972). Tsunami model: Generation and open-sea characteristics. Bull. Seismol. Soc. Am. 62, 1579–1596.
Kanamori, H. (2008). Earthquake physics, and real-time seismology. Nature 451, 271–273. doi: 10.1038/nature06585
Lay, T., Kanamori, H., Ammon, C., Nettles, M., Ward, S., Aster, R., et al. (2005). The Great Sumatra-Andaman Earthquake of 26 December 2004. Science 308, 1127–1133. doi: 10.1126/science.1112250
Lin, S. C., Wu, T. R., Yen, E., Chen, H. Y., Hsu, J., Tsai, Y. L., et al. (2015). Development of a tsunami early warning system for the South China Sea. Ocean Eng. 100, 1–18. doi: 10.1016/j.oceaneng.2015.02.003
Liu, P. L.-F., Woo, S. B., and Cho, Y. S. (1998). Computer programs for tsunami propagation and inundation. New York, NY: Cornell University.
Løvholt, F., Fraser, S., and Salgado-Gálvez, M. (2019). Global trends in advancing tsunami science for improved hazard and risk understanding. Contributing Paper to GAR, University of Hamburg: Germany doi: 10.1007/978-3-642-27737-5_642-1
Meltzner, A. J., Sieh, K., Abrams, M., Agnew, D. C., Hudnut, K. W., Avouac, J.-P., et al. (2006). Uplift and subsidence associated with the great Aceh-Andaman earthquake of 2004. J. Geophys. Res. 111:B02407. doi: 10.1193/1.2201969
Muhari, A., Charvet, I., Futami, T., Suppasri, A., and Imamura, F. (2015). Assessment of tsunami hazard in port and its impact on marine vessels from tsunami model and observed damage data. Natural Hazards 78, 1309–1328. doi: 10.1007/s11069-015-1772-0
Omira, R., Dogan, G. G., Hidayat, R., Husrin, S., Prasetya, G., Annunziato, A., et al. (2019). The September 28th, 2018, Tsunami In Palu-Sulawesi, Indonesia: A Post-Event Field Survey. Pure Appl. Geophys. 176, 1379–1395. doi: 10.1007/s00024-019-02145-z
Spahn, H., Hoppe, M., Vidiarina, H. D., and Usdianto, B. (2010). Experience from three years of local capacity development for tsunami early warning in Indonesia: challenges, lessons and the way ahead. Natural Hazards Earth Sys. Sci. 10, 1411–1429. doi: 10.5194/nhess-10-1411-2010
Strunz, G., Post, J., Zosseder, K., Wegscheider, S., Mück, M., Riedlinger, T., et al. (2011). Tsunami risk assessment in Indonesia. Nat. Hazards Earth Syst. Sci. 11, 67–82. doi: 10.5194/nhess-11-672011
Sunny, R. C., Cheng, W., and Horrillo, J. (2019). Video Content Analysis of the 2018 Sulawesi Tsunami, Indonesia: Impact at Palu Bay. Pure Appl. Geophys. 176, 4127–4138. doi: 10.1007/s00024-019-02325-x
Suppasri, A., Mas, E., Charvet, I., Gunasekera, R., Imai, K., Fukutani, Y., et al. (2013). Building damage characteristics based on surveyed data and fragility curves of the 2011 Great East Japan tsunami. Natural Hazards 66, 319–341. doi: 10.1007/s11069-012-0487-8
Takagi, H., Pratama, M. B., Kurobe, S., Esteban, M., Aránguiz, R., and Ke, B. (2019). Analysis of generation and arrival time of landslide tsunami to Palu City due to the 2018 Sulawesi earthquake. Landslides 16, 983–991. doi: 10.1007/s10346-019-01166-y
Vastano, A. C., and Reid, R. O. (1967). Tsunami response for islands: verification of a numerical procedure. J. Mar. Res. 25, 129–139.
Wu, T. R. (2013). “Tsunami Risk Assessment in Taiwan after the Event of 2011 Tohoku Tsunami,” in The Twenty-third International Offshore and Polar Engineering Conference (California: ISOPE).
Wu, T. R., and Huang, H. C. (2009). Modeling tsunami hazards from Manila trench to Taiwan. J. Asian Earth Sci. 36, 21–28. doi: 10.1016/j.jseaes.2008.12.006
Keywords: tsunami, disaster mitigation, citizen science, numerical simulation, distributed cloud, open platform
Citation: Yen E, Lin SC, Wu T-R, Tsai Y-L and Chung M-J (2020) Knowledge-Building Approach for Tsunami Impact Analysis Aided by Citizen Science. Front. Earth Sci. 8:315. doi: 10.3389/feart.2020.00315
Received: 30 January 2020; Accepted: 03 July 2020;
Published: 15 October 2020.
Edited by:
Kate Huihsuan Chen, National Taiwan Normal University, TaiwanReviewed by:
Yong Wei, University of Washington, United StatesRobert Lovas, Computer and Automation Research Institute (MTA), Hungary
Copyright © 2020 Yen, Lin, Wu, Tsai and Chung. This is an open-access article distributed under the terms of the Creative Commons Attribution License (CC BY). The use, distribution or reproduction in other forums is permitted, provided the original author(s) and the copyright owner(s) are credited and that the original publication in this journal is cited, in accordance with accepted academic practice. No use, distribution or reproduction is permitted which does not comply with these terms.
*Correspondence: Eric Yen, RXJpYy5ZZW5AdHdncmlkLm9yZw==