- 1Department of Earth and Environmental Science, University of Rochester, Rochester, NY, United States
- 2Department of Atmospheric and Oceanic Sciences, University of California, Los Angeles, Los Angeles, CA, United States
The remineralization depth of sinking organic particles controls the efficiency of the biological carbon pump by setting the sequestration timescale of remineralized carbon in the ocean interior. Oxygen minimum zones (OMZs) have been identified as regions of elevated particle transfer and efficient carbon sequestration at depth, but direct measurements remain sparse in these regions and only provide snapshots of the particle flux. Here, we use remineralization tracers to reconstruct time-mean particle flux profiles in the OMZs of the Eastern Tropical Pacific and the Arabian Sea. Compared to the surrounding tropical waters, both OMZs exhibit slow flux attenuation between 100 and 1000 m where suboxic waters reside, and sequester carbon beneath 1000 m more than twice as efficiently. Using a mechanistic model of particle sinking, remineralization, and disaggregation, we show that three different mechanisms might explain the shape of the OMZ flux profiles: (i) a significant slow-down of remineralization when carbon oxidation transitions from aerobic to anaerobic respiration (e.g., denitrification); (ii) the exclusion of zooplankton that mediate disaggregation of large particles from suboxic waters, and (iii) the limitation of remineralization by the diffusive supply of oxidants (oxygen and nitrate) into large particles. We show that each mechanism leaves a unique signature in the size distribution of particles, suggesting that observations with optical instruments such as Underwater Vision Profilers hold great promise for understanding the drivers of efficient carbon transfer though suboxic water columns. In turn, this will allow more accurate prediction of future changes in carbon sequestration as the ocean loses oxygen in a warming climate.
Introduction
The biological pump sequesters carbon out of contact with the atmosphere in deep ocean waters, owing to the formation of organic particles in the surface ocean followed by their sinking and remineralization at depth (Passow and Carlson, 2012). The depth of particle remineralization determines the “efficiency” of the biological pump, quantified as the amount of time remineralized carbon dioxide (CO2) remains sequestered in the ocean interior before returning to the surface (DeVries et al., 2012; Boyd et al., 2019). Recently, systematic large-scale patterns in particle remineralization depth have been identified from sediment trap data (Buesseler et al., 2007; Marsay et al., 2014) and confirmed by geochemical flux reconstructions, which provide a more spatially and temporally integrated view than the snapshots provided by sediment traps (Weber et al., 2016). Particles remineralize shallowest in the subtropics and deepest in high latitudes, which can be explained by a combination of temperature-sensitive remineralization rates and the effects of particle size and ballasting on particle sinking speed (Weber et al., 2016; DeVries and Weber, 2017; Laufkötter et al., 2017; Cram et al., 2018).
In addition to this large-scale pattern, sediment trap observations indicate that tropical oxygen minimum zones (OMZs) are regions of particularly efficient particle transfer to depth (Devol and Hartnett, 2001; Van Mooy et al., 2002; Keil et al., 2016), although this has not yet been confirmed by geochemical reconstructions based on dissolved tracer distributions. The mechanisms explaining efficient particle transfer through OMZs are not well understood, but multiple hypotheses have been advanced which fall into three broad categories, referred to here as Hypotheses 1–3.
Hypothesis 1 states that particle attenuation slows because the microbial community transitions from aerobic to anaerobic respiration – mostly denitrifying metabolisms – in oxygen deficient water (Devol, 1978), resulting in significantly slower organic carbon oxidation (Devol and Hartnett, 2001; Van Mooy et al., 2002). Hypothesis 2 states that the exclusion of zooplankton from oxygen deficient waters (Wishner et al., 2013; Wishner et al., 2018), or the suppression of zooplankton activity at low oxygen (O2) concentrations (Seibel, 2011; Kiko et al., 2016), prevents the disaggregation of large aggregates during zooplankton feeding, allowing more efficient transfer through the mesopelagic (Cavan et al., 2017). Hypothesis 3 revises Hypothesis 1 to state that organic carbon oxidation is governed by the physico-chemical “microenvironment” within sinking particles (Wright et al., 2012), rather than the ambient seawater chemistry. In oxygen deficient water columns, aerobic respiration and denitrification can exhaust O2 and nitrate (NO3) inside large particles faster than it is supplied diffusively (Ploug, 2001), preventing further carbon oxidation or slowing it significantly as the microbial community transitions to inefficient metabolisms such as sulfate reduction (Bianchi et al., 2018). Unlike Hypothesis 1, Hypothesis 3 does not invoke a substantial slowdown of carbon oxidation during denitrification relative to aerobic respiration, consistent with the similar free energy yield of the two pathways (Froelich et al., 1979).
Accurately quantifying particle fluxes through OMZs, and identifying their driving mechanisms, is critical for robust prediction of ocean carbon in a warming and deoxygenating ocean (Bopp et al., 2013; Laufkötter et al., 2017; Schmidtko et al., 2017), and for understanding the interaction of ocean oxygen content and carbon storage during past climate transitions (Lu et al., 2016). This paper serves two primary purposes. First, it presents new geochemical reconstructions that confirm and more firmly quantify efficient particle transfer through oxygen minimum zones (Section “Reconstruction of OMZ Particle Fluxes”). Second, it employs a mechanistic particle flux model (Section “Mechanistic Particle Size Spectrum Model”) to test which of the three hypotheses outlined above can explain the reconstructed flux profiles (Section “Simulated Particle Fluxes in the OMZs”), while demonstrating that each mechanism makes different predictions about particle size spectra within the OMZs (Section “Particle Size Spectra Distinguish Between Attenuation Mechanisms”). It is our hope that this will allow each hypothesis to be further scrutinized using burgeoning particle spectra datasets from Underwater Vision Profilers (UVPs) (Stemmann et al., 2012) in future work.
Reconstruction of OMZ Particle Fluxes
Previous work that reconstructed organic particle fluxes using dissolved geochemical tracers found that flux profiles averaged across tropical ocean regions exhibit intermediate attenuation over depth, sitting halfway between the fast-attenuating subtropics and slow-attenuating high latitudes (Weber et al., 2016). For this study, we repeated the geochemical reconstruction approach, but divided the Tropical Pacific and Indian oceans into suboxic OMZs (defined as [O2] < 5 μM at 400 m) and surrounding oxygenated waters (Figure 1A).
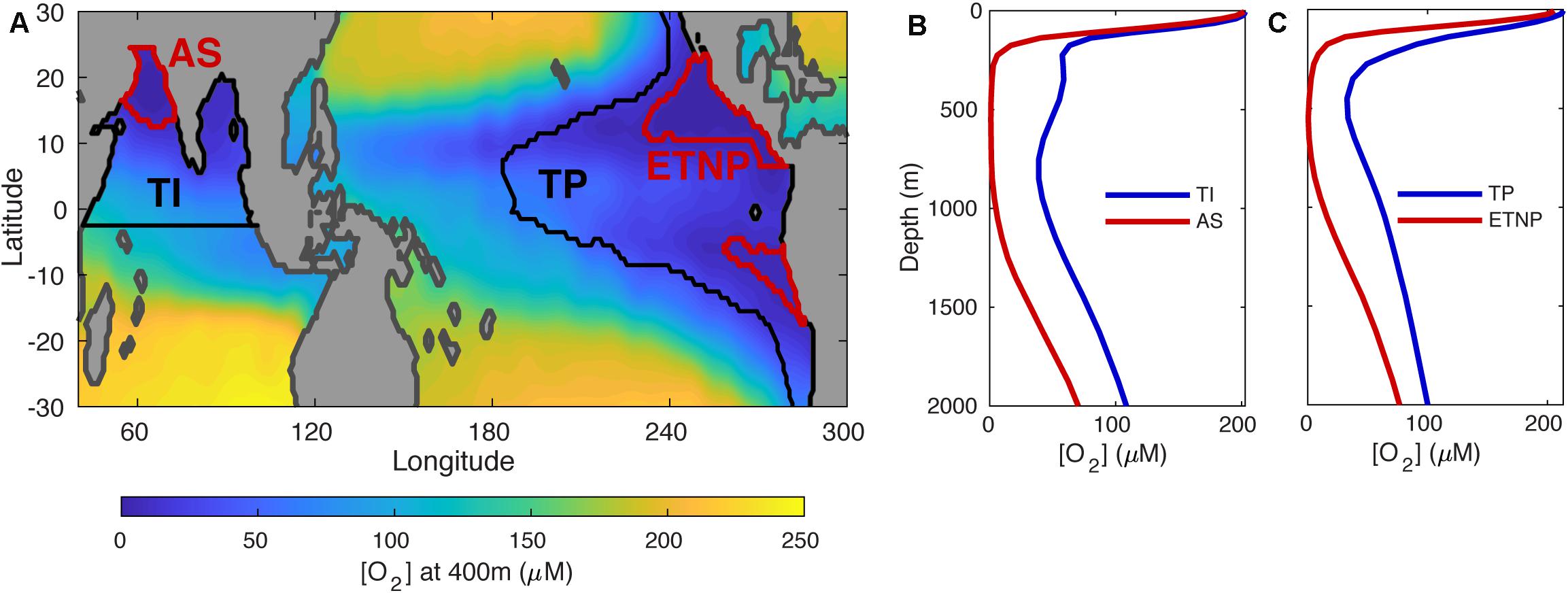
Figure 1. Regions used in our flux reconstruction analysis. (A) Contour map of annual-mean oxygen concentration at 400 m depth in the low latitude ocean, from the World Ocean Atlas 2013 (Garcia et al., 2013). Black and red contours delineate the regions over which reconstructed particle flux profiles are averaged. The areas inside of the black contours in the Indian and Pacific Oceans are defined as the oxygenated Tropical Indian (TI) and Tropical Pacific (TP) averaging regions, respectively, with the exception of the areas inside of the red contours, which enclose suboxic waters with [O2] < 5 μM at 400 m. These are defined as the OMZ averaging regions – Arabian Sea (AS) and Eastern Tropical North Pacific (ETNP). In the South Pacific, the area inside of the red contour is not incorporated into the TP region, but is not defined as independent averaging region because it spans too few gridcells on our model grid. (B) Depth profiles of annual-mean [O2] averaged across the TI and AS regions. (C), same as (B) but for the TP and ETNP regions.
The method for flux reconstruction is fully described in Weber et al. (2016). Briefly, an observationally constrained ocean circulation model is used to diagnose the accumulation rate of PO4 in the ocean interior (>100 m) due to particle remineralization, and the particulate organic phosphorus (POP) flux through a given depth horizon is estimated by integrating the remineralization that occurs beneath that horizon. Finally, fluxes are averaged into profiles across large ocean regions to minimize gridpoint-scale noise and identify large scale patterns in the particle flux. We note that in our interpretations, we implicitly assume that the shape of POP flux profiles is representative of all organic particle constituents, including particulate organic carbon (POC). This is a necessary limitation of our approach, given that POC flux profiles cannot be reconstructed more directly (Weber et al., 2016).
Four averaging regions are used here (Figure 1A). In the Indian Ocean, waters north of 2.5°S are integrated into the Tropical Indian (TI) region, with the exception of the Arabian Sea (AS) OMZ (Figure 1A), which houses a thick layer of suboxic water between ∼250 and 900 m (Figure 1B). In the Pacific, waters between 30°S and 30°N with a shallow nutricline [see (Weber et al., 2016)] are integrated into the Tropical Pacific (TP) region, with the exception of two OMZ regions at the eastern boundary (Figure 1A). To the north, the Eastern Tropical North Pacific (ETNP) is the world’s most expansive OMZ, where suboxic waters spread westward between 300 and 800 m (Figure 1C). To the south, the Eastern Tropical South Pacific (ETSP) is more coastally confined, and covers too few gridcells on our coarse model grid to allow for reliable flux reconstruction. This OMZ is not incorporated into the broader TP region, but is also not considered as a separate region in our analysis. The only further modification made here to the method of Weber et al. (2016) is that we use an updated ocean circulation model (DeVries and Holzer, 2019), and twelve model configurations with slightly different diffusive parameterizations are used to propagate circulation uncertainty into the flux reconstructions.
In the oxygenated TP and TI regions, reconstructed POP fluxes (Figures 2A,D) are very similar to those presented in Weber et al. (2016) for tropical regions. When normalized to the flux at ∼100 m (actually 114 m on our model grid), these flux profiles exhibit a similar shape to the canonical “Martin curve” (Martin et al., 1987), which attenuates over depth following a power-law relationship. At a depth of 1000 m, 15 ± 3% of the 100 m flux remains in the TP, and 18 ± 2% remains in the TI (Figures 2C,F), both similar to the 14% predicted by the Martin curve with canonical attenuation exponent of 0.858 (Martin et al., 1987).
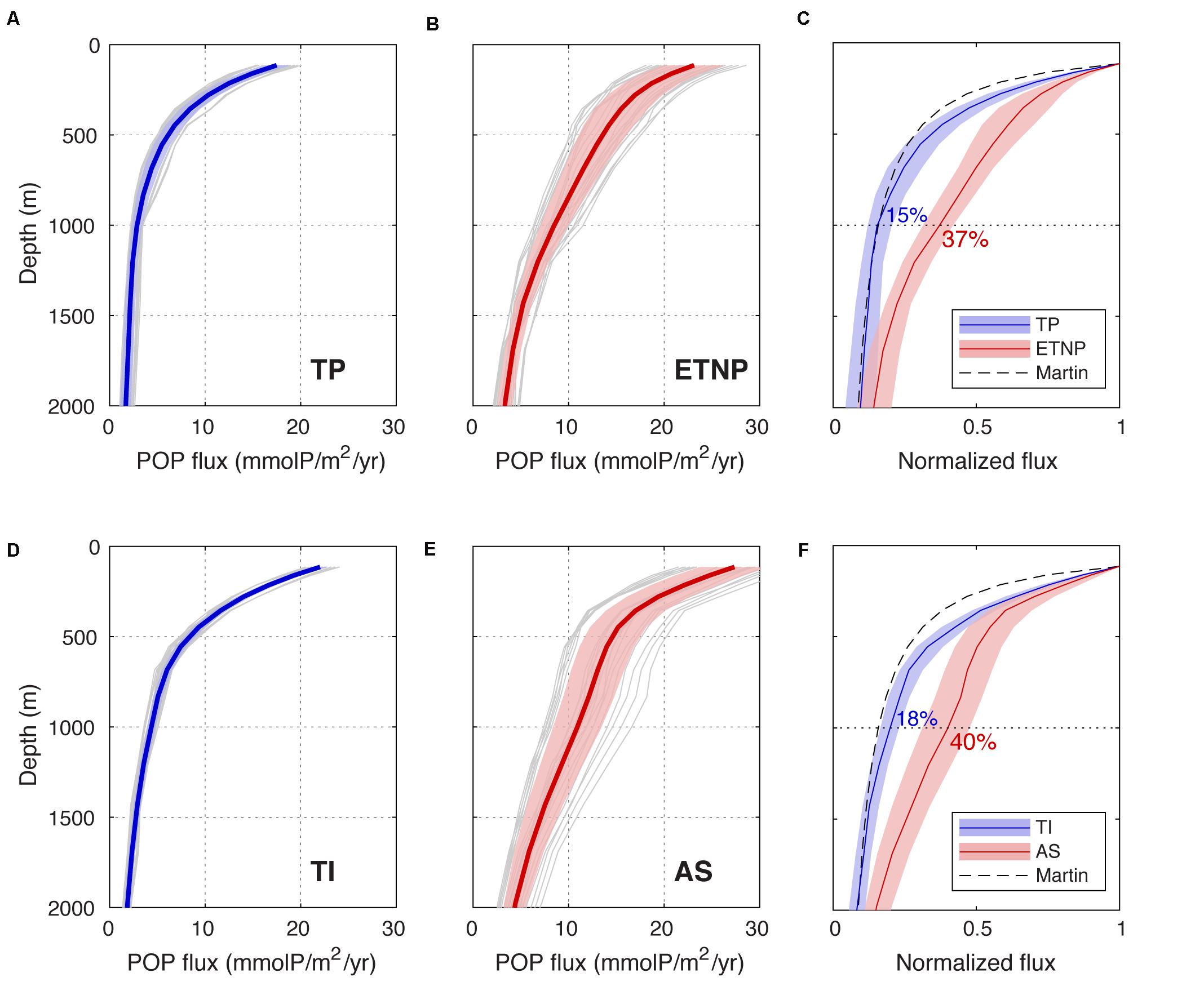
Figure 2. Reconstructed particle flux profiles. (A,B,D,E), Flux profiles of particulate organic phosphorus (POP) averaged across the TP, ETNP, TI and AS regions, respectively, reconstructed by diagnosing PO4 accumulation in an ocean circulation model. Gray lines show the individual profiles derived from an ensemble of different ocean circulation models. Central red and blue lines show the ensemble-means, and shaded envelopes delineate ± 1σ from the mean. (C), Ensemble-mean flux profiles and uncertainty envelopes from the TP and ETNP regions are normalized to the flux at the base of the euphotic zone, facilitating comparison between profile shapes in the oxygenated tropics and the OMZ. The fraction of the flux remaining at 1000 m is labeled for each region, and the canonical Martin curve (dashed black line) is shown for reference. (F) Same as (C) but for TI and AS regions.
Flux profiles in the ETNP and AS OMZ regions differ from the surrounding tropical waters in two ways. First, the export flux at the base of the euphotic zone is ∼30% higher in the OMZs than the oxygenated tropics in both ocean basins (Figures 2B,E), which can be attributed to the fact that OMZs are located in productive coastal upwelling regions. Second, and more importantly, the normalized flux profiles do not follow the same simple power-law shape over depth (Figures 2C,F). In the shallow subsurface (100–200 m), OMZ particle fluxes attenuate almost as quickly in the OMZs as in the surrounding tropics, but the attenuation slows significantly beneath 200 m, and the ETNP and AS profiles begin to diverge from the TP and TI profiles, respectively (Figures 2C,F). This divergence reaches its maximum at 1000 m, where 37 ± 4% of the 100 m flux remains in the ETNP, and 40 ± 8% remains in the AS. In both Pacific and Indian Oceans, particle transfer through the mesopelagic zone (100–1000 m) is therefore more than twice as efficient in OMZs than in oxygenated tropical waters. In the lower oxyclines beneath 1000 m, the ETNP and AS particle fluxes attenuate more rapidly again, re-converging with the TP and TI profiles, respectively. This provides strong evidence that the slow particle attenuation is specifically linked to water column suboxia, and not caused by unrelated differences such as larger particles or lower temperatures in the OMZs.
The results presented here are consistent with sediment trap observations, which also find particle transfer efficiency of ∼40% to 1000 m in OMZs (Devol and Hartnett, 2001; Van Mooy et al., 2002), and confirm that slow flux particle attenuation is a systematic and time-mean feature of OMZs. Our finding that slow attenuation is specifically associated with the suboxic layer is consistent with a recent study using thorium isotopes to reconstruct particle fluxes in the ETSP (Pavia et al., 2019), but our results do not support their finding that fluxes attenuate faster in the upper oxycline of the OMZ than in oxygenated water columns.
Mechanistic Particle Size Spectrum Model
To investigate the mechanisms driving differences in flux attenuation between OMZs and oxygenated tropical waters, we employed the size-resolved particle spectrum model developed by DeVries et al. (2014) and updated by Bianchi et al. (2018). In this model, particles are produced in the surface euphotic zone (<100 m) following a power-law size spectrum, in which log10 (particle number density) declines linearly with log10 (diameter), and the relative abundance of large and small particles is controlled by the “slope” of the spectrum. The spectrum is discretized into ∼200 size classes between limits of 50 μm and 5 mm, and the organic carbon mass of each particle class is related to its diameter (d, in mm) by POC = cmdα, where cm (μgC) is the reference carbon mass of a 1 mm particle and α (unitless) is an exponent that accounts for the fractal nature of marine organic particles (Alldredge, 1998).
The simulated particle size spectrum then evolves through the water column due to remineralization, disaggregation, and size-dependent sinking, which are each parameterized based on empirically derived relationships and observed particle properties (Bianchi et al., 2018). Remineralization is represented by first-order mass loss from each particle at carbon-specific rate cr (day–1), which means that each particle shrinks and its sinking speed slows over depth, resulting in attenuation of the particle flux. When particles shrink beneath the lower size limit of 50 μm they are assumed to stop sinking and are transferred to a suspended particle class that remineralize in situ. Disaggregation is represented as first order mass loss from each size class at rate kd (day–1), which can be thought of as the probability of a given particle fragmenting in a unit time. Particles are assumed to fragment into a spectrum of smaller sized particles, which means their mass is re-apportioned among all smaller size classes (Bianchi et al., 2018). Because disaggregation transfers mass from large, fast-sinking size classes to small, slow-sinking classes, it also results in significant attenuation of the particle flux over depth, consistent with observations (Briggs et al., 2020).
The default model configuration used here (referred to as Model 0, see Table 1) is the same as that described in Bianchi et al. (2018), with two modifications. First, the model of Bianchi et al. (2018) simulates internal particle chemistry, allowing remineralization to be partitioned between different redox pathways. In Model 0, this behavior is “deactivated”, and particles are assumed to remineralize homogeneously due to aerobic respiration. Second, we reformulate the remineralization rate constant cr as function of temperature, to be consistent with laboratory cultures (Iversen and Ploug, 2013) and other recent modeling efforts (DeVries and Weber, 2017; Laufkötter et al., 2017; Cram et al., 2018):
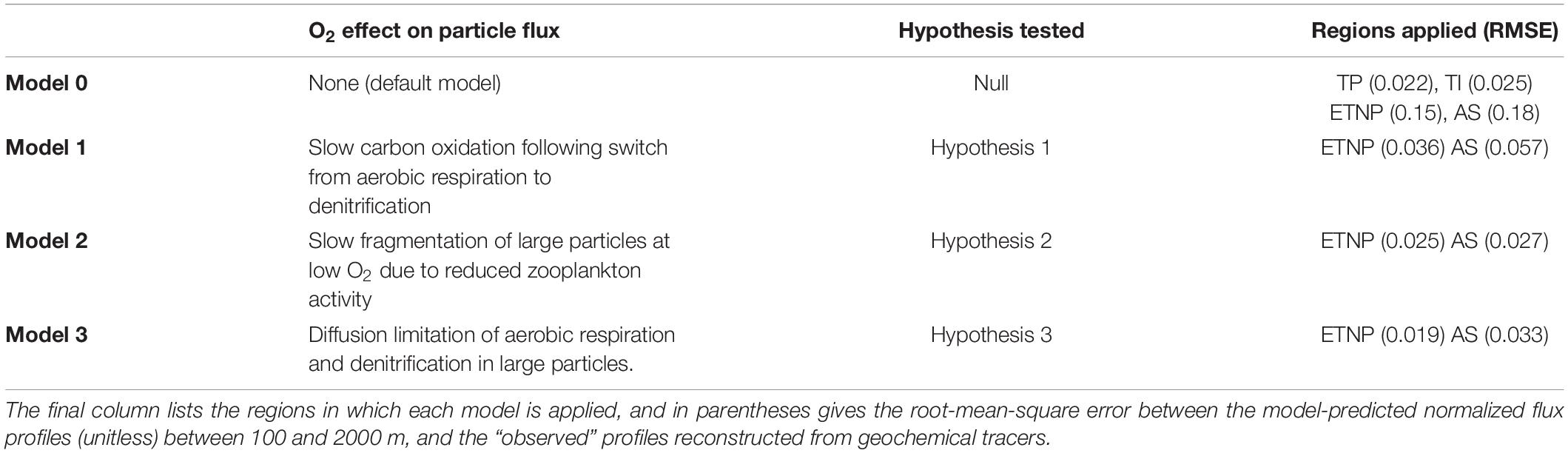
Table 1. Summary of O2 effects on the particle flux in each model configuration, and the hypothesis that is tested by the model.
In Eq.1, cref is the carbon-specific oxidation rate at the reference temperature Tref (here 20°C), and Q10 is the factor by which this rate increases/decreases for each 10°C of warming/cooling.
We developed three further configurations of the model (Models 1–3, Table 1), designed to formalize the three different effects of O2 on particle fluxes proposed in Hypotheses 1–3 (see Section “Introduction”). Each of these configurations makes a single modification to Model 0, independently of one another. While a combination of the O2 effects may operate in real OMZs, our “end-member” simulations serve to explore the flux profiles and particle spectra that would be produced when each mechanism operates in isolation.
Model 1 imposes a simple O2 threshold effect on the cr, representing slower carbon oxidation following a transition from aerobic respiration to denitrification as the sole remineralization pathway in suboxic waters (Hypothesis 1):
In Eq 2, εd is the ratio of the carbon oxidation rate achieved during denitrification to the rate achieved during aerobic respiration, and the transition between metabolisms occurs sharply when O2 drops below O2,crit (Devol, 1978).
Model 2 represents the slowing of particle disaggregation in OMZs due to declining zooplankton activity at low [O2] (Cavan et al., 2017) (Hypothesis 2). In Bianchi et al. (2018), the disaggregation rate constant kd was chosen to ensure that large particle classes lose mass due to remineralization and disaggregation at relatively even rates, consistent with constraints from thorium isotopes (Lam and Marchal, 2015), and new evidence suggesting that fragmentation is responsible for up to 50% of the particle flux attenuation (Briggs et al., 2020). In Model 2, we redefine:
In Eq. 3, kd∞ is the fragmentation rate constant when O2 is abundant, εf is the fraction of this rate that is maintained under anoxic conditions (which can be thought of as the fraction of disaggregation attributed to processes other zooplankton feeding) and KO2 is the half-saturation O2 concentration for zooplankton activity.
Finally, Model 3 “reactivates” the full simulation of internal particle chemistry described in Bianchi et al. (2018), to represent the diffusion-limitation of carbon oxidation in suboxic water columns (Hypothesis 3). Briefly, this model represents the diffusive supply of oxidants into each particle, and their stepwise consumption in the particle interior by carbon oxidation. O2 is consumed first, and if the demand exceeds the O2 supply a denitrifying microenvironment is formed where NO3 is reduced and carbon oxidation proceeds at ∼99% of the aerobic rate, reflecting the relative free energy yields of the pathways (Froelich et al., 1979). If NO3 also becomes exhausted, a final internal microenvironment is formed in which Bianchi et al. (2018) assumed that carbon is oxidized extremely slowly by sulfate reduction. For simplicity, we instead assume that organic carbon oxidation cannot proceed at all in this NO3-free zone.
Our goal is to determine which of the three mechanisms formalized in Models 1–3 can adequately explain the differences in reconstructed particle flux profiles between OMZs and the surrounding tropical waters (Figures 2C,F). To achieve this, we first fit Model 0 to the reconstructed flux profiles from the TP and TI regions, by optimizing cref and Q10 to minimize the root-mean-square error (RMSE) between the model prediction and the mean normalized flux profile from our ensemble of 12 different reconstructions (central blue line in Figures 2C,F). All other model parameters are left unchanged from Bianchi et al. (2018). The values of cref and Q10 determined for the TP region were propagated into all simulations of the ETNP, and the values determined for TI were propagated into all simulations of the AS. In the ETNP and AS regions, Model 0 was first applied to test the null hypothesis that OMZ flux profiles can simply be explained by differences in water column temperature relative to the surrounding tropics, with no O2 effect. Then, Models 1–3 were applied and optimized to assess Hypotheses 1–3.
In each optimization, a small number of parameters that control the strength of the O2 effect on particle flux attenuation were adjusted to minimize the RMSE between the model and the reconstructed flux profiles (central red line in Figures 2C,F): In Model 1, εd and O2,crit were adjusted; in Model 2, εf and KO2 were adjusted; in Model 3, a single free parameter (cm) was adjusted, which controls the carbon content of particles and therefore determines how quickly diffusion-limitation of carbon oxidation is reached (see Bianchi et al., 2018). Following model optimization, Hypotheses 1–3 can be assessed based on how well Models 1–3 reproduce the reconstructed OMZ flux profiles, and whether this can be achieved without violating prior constraints on the parameter values (see Section “Simulated Particle Fluxes in the OMZs”).
All water column data required for these simulations (temperature for remineralization, O2 and NO3 for boundary conditions in Model 3) are taken from the World Ocean Atlas 2013 climatology (Garcia et al., 2013) and averaged across the regions shown in Figure 1. The particle spectrum slope at the base of the euphotic zone of all regions is set to −3.4, which was identified as characteristic value for low latitude systems in Bianchi et al. (2018).
Simulated Particle Fluxes in the OMZs
Following parameter optimization, Model 0 accurately reproduced the time-mean particle flux profiles that we reconstructed for the oxygenated TP and TI regions (Figures 3A,C, Table 1). To achieve this, the model required carbon oxidation rate constants at 20°C of ∼0.6 day–1 and ∼0.5 day–1 in the TP and TI regions, respectively, which fall within the range of rates measured by particle-interceptors in warm low latitude regions (McDonnell et al., 2015). It also required Q10 of ∼2.4 and ∼2.1 in TP and TI, respectively, similar to the values selected by other data-constrained models (DeVries and Weber, 2017; Cram et al., 2018).
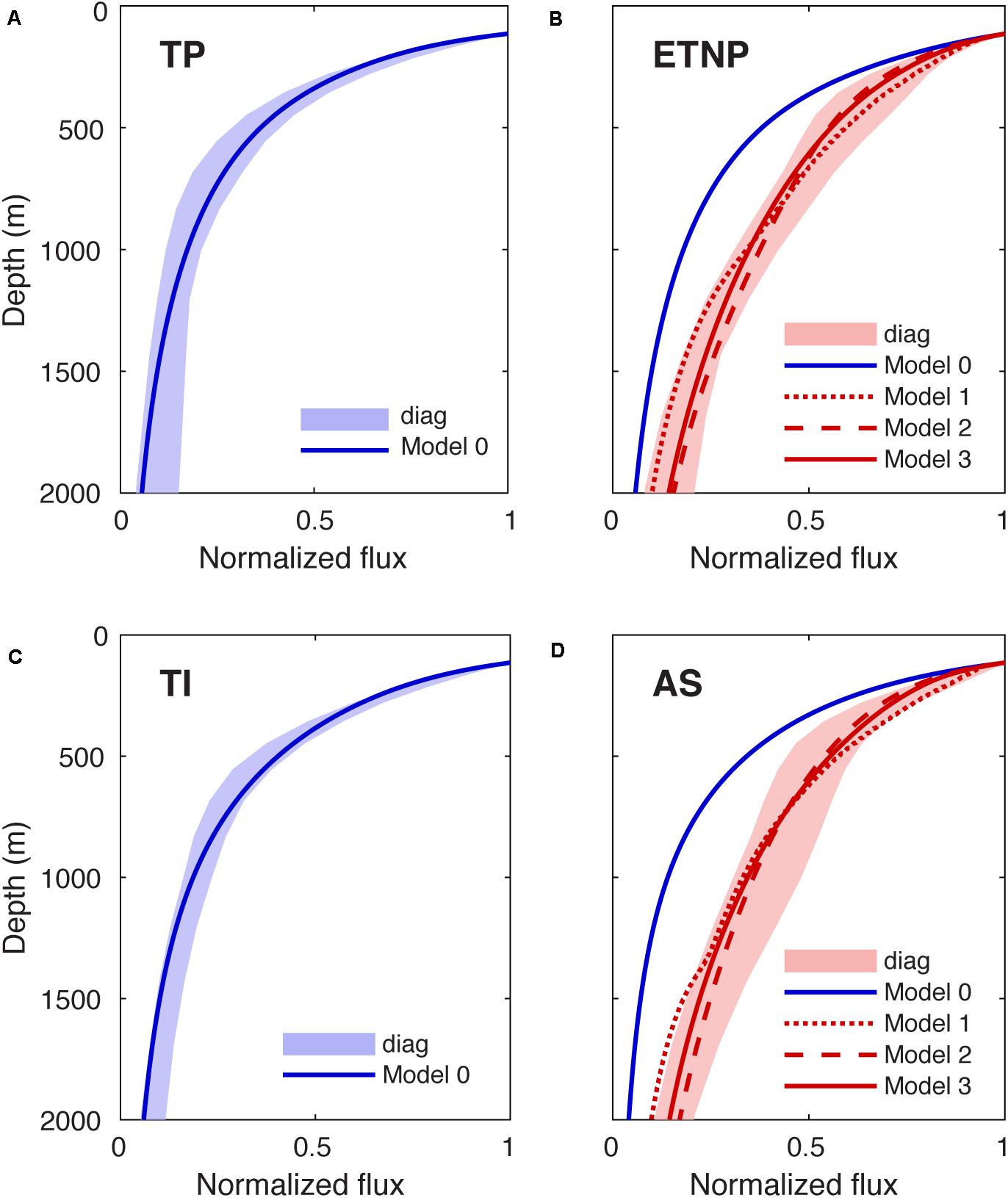
Figure 3. Particle flux profiles predicted by a mechanistic size-resolved model. (A) Normalized particle flux predicted by the default Model 0 configuration (blue line) in the TP region, compared to the uncertainty envelope of the reconstructed flux (blue shading, repeated from Figure 2C). (B) Predicted particle fluxes in the ETNP region from Model 0 (blue line) and Models 1–3 (red lines), which simulate different O2 effects on particle flux attenuation, compared to the reconstructed profile (red shading). (C,D) Same as (A,B) but for the TI and AS regions, respectively.
Reconstructed flux profiles in the ETNP and AS could not be reproduced by Model 0, which predicts flux profiles that attenuate far too rapidly over depth, demonstrating that temperature is not an important factor driving efficient particle transfer through OMZs (Figures 3B,D, Table 1). However, Models 1–3 could all reproduce the reconstructed OMZ flux profiles reasonably well following the parameter optimizations outlined in Section “Mechanistic Particle Size Spectrum Model” (Figures 3B,D, Table 1). Of the three configurations, Model 2 fit the reconstructed flux profile best in the AS region (RMSE≈0.03, unitless) and Model 3 fit best in the ETNP region (RMSE≈0.02), whereas Model 1 was least skillful in both regions (∼0.06 in AS; ∼0.04 in ETNP). This is because Model 1 predicts that the particle flux attenuates slightly faster than observed in the lower oxycline beneath the suboxic layer (Figures 3B,D). However, all three models can largely fit the reconstructed fluxes within their uncertainty envelopes, suggesting the shape of the particle flux profiles does not place a strong constraint on the mechanism driving slow attenuation in the OMZs.
In Model 1, our optimization process selects εd of ∼0.19 and ∼0.17 in ETNP and AS, suggesting that carbon oxidation must slow down more than 80% following the transition from aerobic respiration to denitrification, in order to best explain the reconstructed fluxes. This is not consistent with the difference in free energy yield between the two process, which is only ∼1% (Froelich et al., 1979), but might be explained by lag time associated with particle colonization by the denitrifying microbial community, which remains poorly quantified to date (Bristow, 2018), or other processes that limit the efficiency of anaerobic metabolisms. The optimization also selects O2,crit of 15 μM and 20 μM in ETNP and AS, respectively, which is much higher than the range of 2–5 μM observed to select for denitrifying metabolisms in cultures (Devol, 1978). Taken together, these parameter selections cast doubt on the idea that slow carbon oxidation during denitrification is the primary driver of slow flux attenuation in OMZs (Hypothesis 1).
In Model 2, our optimization selects εf of ∼0.02 in both ETNP and AS regions, which means that disaggregation must be almost entirely curtailed in suboxic water in order to explain the reconstructed flux profiles, requiring that very little disaggregation occurs due to processes other than zooplankton feeding. It also selects KO2 of ∼22 μM in ETNP and ∼40 μM in AS, consistent with observations that zooplankton generally adjust their migration depth to avoid hypoxic ([O2] < 60 μM) regions of the water column, not just suboxic waters (Bianchi et al., 2013).
Finally, in Model 3 our optimization process selects cm (carbon content of a 1 mm particle) of 6.8 μgC in ETNP and 7.2 μgC in AS, which both fall within the observationally constrained range of 5.5–8 μgC defined in Bianchi et al. (2018). This model predicts that large NO3-depleted microenvironments develop in the interior of particles >0.5 mm in the suboxic layers of the ETNP and AS, and particles >1 mm also harbor these microenvironments in the upper and lower oxyclines, dramatically curtailing remineralization of large particles through the water column.
Particle Size Spectra Distinguish Between Attenuation Mechanisms
Even though Models 1–3 achieve relatively similar flux profiles (Figures 3B,D), they make very different predictions about how the particle size spectrum evolves through the OMZ, and how it diverges from the spectra generated in oxygenated water columns (Figure 4). Here, we analyze particle spectra only from simulations of the TP and ETNP regions for brevity, although similar conclusions can be drawn from the TI and AS regions.
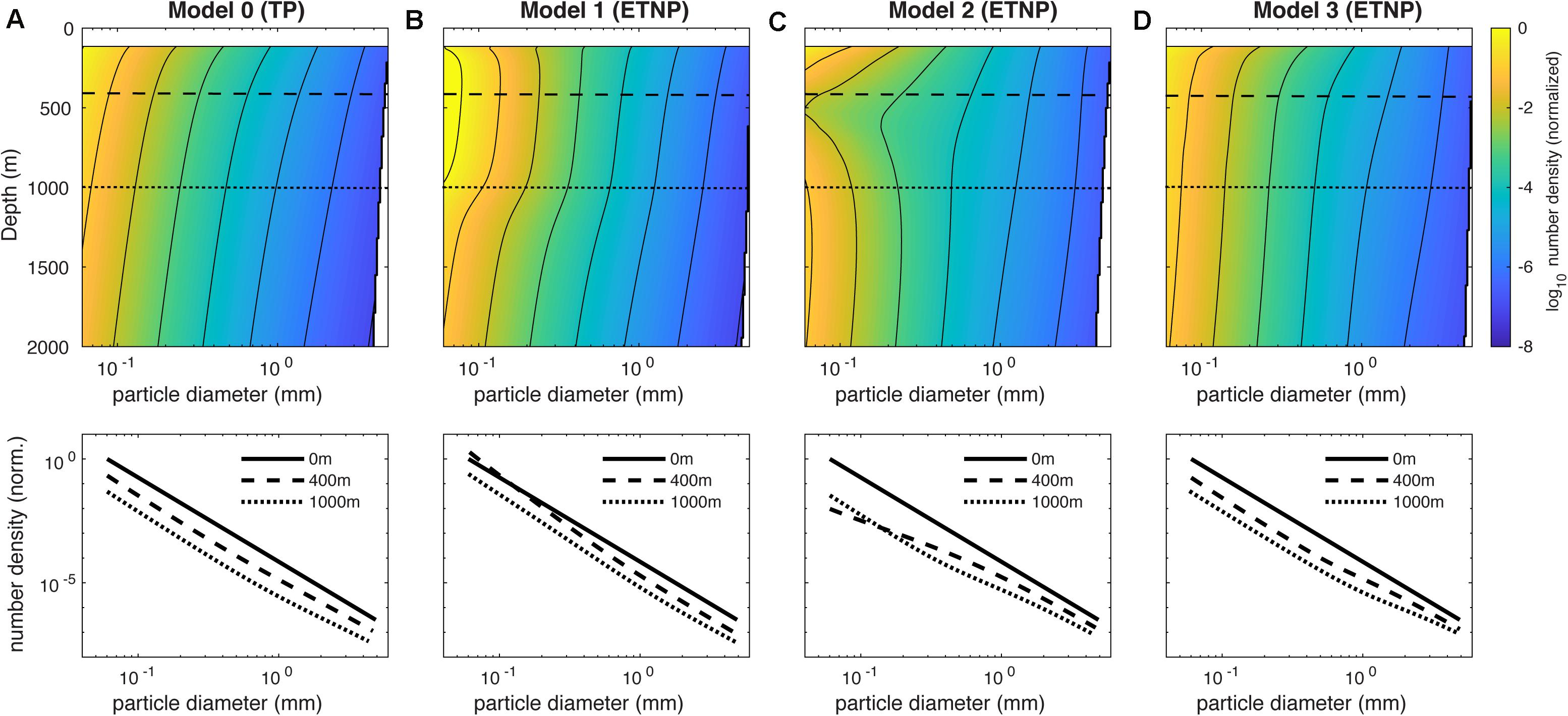
Figure 4. Predicted particle size spectra in our mechanistic model. (A), Upper panel shows the particle number density predicted in the TP region by Model 0, as a function of depth and particle diameter. Number density in #mm–1 is normalized to the abundance of the smallest size class in the surface ocean, yielding a dimensionless size spectrum. Lower panel shows the surface size spectrum (solid line, slope = –3.4) and “slices” through the spectrum at depths of 400 m (oxygen minimum) and 1000 m (lower oxycline), which are indicated on the upper panel as dashed and dotted lines, respectively. (B–D), Same as (A), but showing the size spectra predicted by Models 1–3 in the ETNP region. Each of these models predicts unique differences in the OMZ size spectra relative to the oxic water column.
In our model, changes in the size spectrum over depth are largely driven by the balance of remineralization, disaggregation, and sinking. While all particles remineralize at the same first-order rate, smaller particles spend a longer time within each depth interval due to their slower sinking speed, and so remineralize shallower in the water column. The effect of remineralization is therefore a “flattening” of the particle spectrum over depth, due to the preferential loss of particles from the small end of the spectrum. Disaggregation has the opposite effect: by redistributing mass from large to small particle size classes, it tends to “steepen” the particle size spectrum. In the default configuration of our model (Model 0), the relatively even rates of disaggregation and remineralization means their opposing effects on the particle size spectrum largely balance one another, and the particles are lost evenly from all size classes over depth (Figure 4A-upper) in the TP region, causing little variation in the size spectrum slope (Figures 4A-lower, 5A). This is consistent with a compilation of UVP particle observations from the oxic Tropical Atlantic Ocean, which revealed no systematic variations over depth (Bianchi et al., 2018).
Models 1–3 each predict particle spectra in the OMZ that diverge from this simple behavior in unique ways. In Model 1, particles smaller than ∼100 μm actually increase in abundance moving from the surface into the suboxic layer, while large particle abundance declines (Figure 4B-upper). This is because when remineralization is suppressed at O2 < O2,crit (Eq. 2), the transfer of mass from large to small size classes by disaggregation becomes the primary mechanism shaping the particle size spectrum. The spectrum slope therefore steepens moving into the core of suboxic layer (Figures 4B-lower, 5A), before flattening again toward the original value of −3.4 in the lower oxycline (Figure 5A).
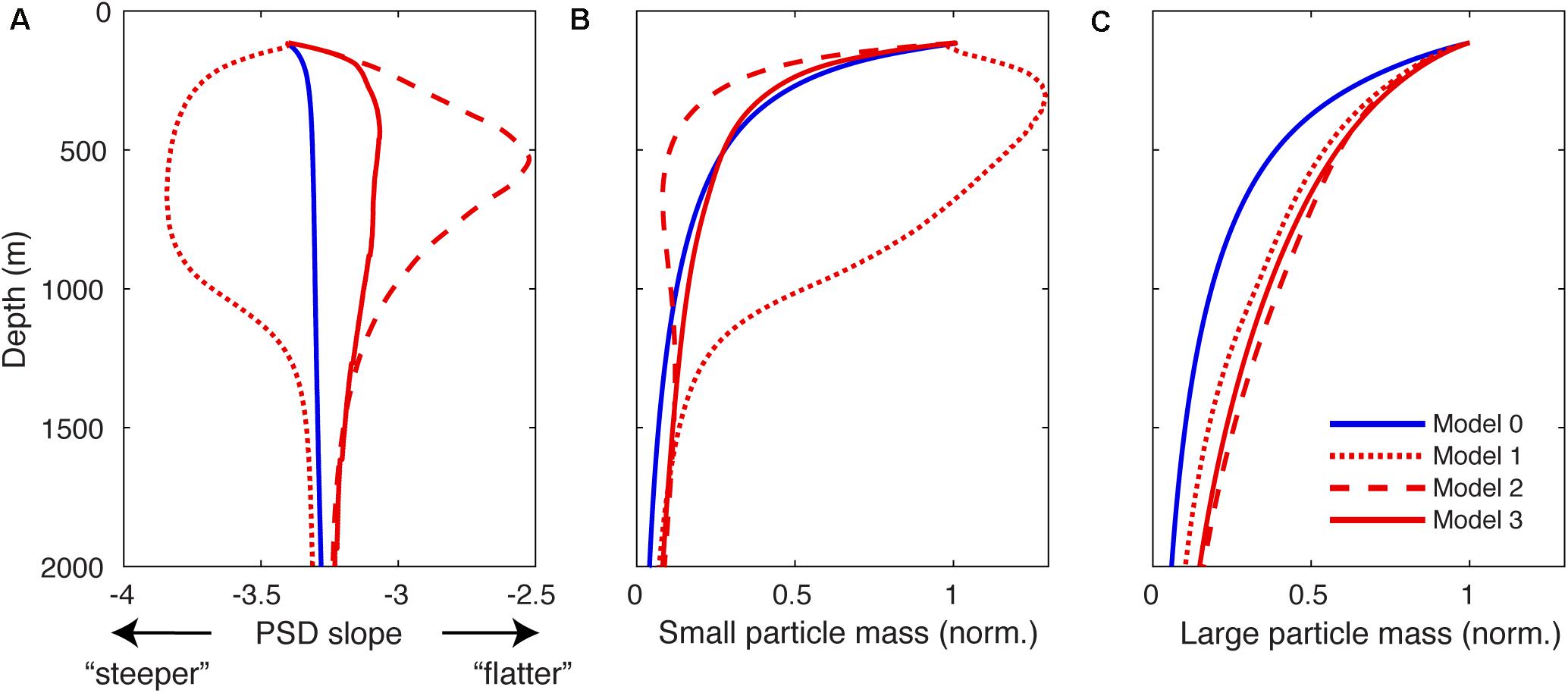
Figure 5. Summary statistics of simulated particle spectra. (A), Slope of the particle spectrum as a function of depth predicted by Model 0 in the TP region and by Models 1–3 in the ETNP region, derived by fitting a straight line through the simulated spectrum at each model depth horizon. More negative numbers indicate a “steeper” spectrum that is more dominated by small particles, and less negative numbers indicate a “flatter” spectrum that is more dominated by large particles. (B) Mass of particulate organic matter (POM) integrated across small particle size classes, defined here as < 0.5 mm, and normalized to the mass at 100 m. (C) Same as (B) but for large aggregate particles >0.5 mm.
In Model 2, small particles are lost very rapidly moving into the OMZ (Figure 4C-upper), compared to their loss rate over the same depth interval in the oxygenated water column (Figure 4A-upper). With disaggregation suppressed at low O2 (Eq. 3), the preferential loss of small particles due to remineralization becomes the dominant process shaping the particle spectrum, and the spectral slope sharply flattens moving into the suboxic layer (Figure 4C-lower), reaching a value of −2.5 at ∼500 m (Figure 5A). The spectrum then steepens again in the lower oxycline (Figures 4C-lower, 5A) where disaggregation resumes in waters that become habitable for zooplankton once more (Wishner et al., 1998).
In Model 3, particles larger than 0.5 mm are preferentially preserved through the suboxic layer due to the diffusion-limitation of carbon oxidation (Figure 4D-upper), resulting in flattening of the particle flux, followed by a gradual steepening through the lower oxycline (Figures 4D-upper, 5A). Although Models 2 and 3 both predict flattening size spectra over depth in the OMZ, indicating that large particles become more abundant relative to small particles, the two mechanisms can be distinguished based on a closer analysis of the spectra. Model 3 predicts a much subtler flattening of the spectrum than Model 2, reaching a minimum slope of −3.1 compared to −2.5 in Model 2 (Figure 5A). In Model 3, even though small particles are preferentially lost due to remineralization in the OMZ, they are rapidly replenished through disaggregation. Therefore, in comparison to an oxygenated water column, Hypothesis 3 predicts a relatively similar concentration profile of small particles (<0.5 mm) in the OMZ, whereas Hypothesis 2 predicts a much more rapid decline in small particle concentration in the suboxic layer (Figure 5B). By contrast, Hypothesis 1 uniquely predicts that the concentration of small particles actually increases over the same depth interval (Figure 5B). This analysis suggests that the smaller end of the particle spectrum contains the most important clues for distinguishing between different mechanisms of slow flux attenuation through OMZs, whereas all mechanisms predict higher concentrations of large particles relative to the oxic water column (Figure 5C).
Identifying the unique particle size spectrum signatures of O2 effects on remineralization and disaggregation opens a promising new pathway for understanding the mechanisms driving the efficient biological pump of OMZ regions, through analysis of UVP size spectrum observations. These instruments allow accurate enumeration of particles between the size limits of 100 μm−2.6 mm (Picheral et al., 2010), spanning most of the size range simulated in our model, and therefore provide ideal datasets for evaluating our model formalizations of Hypotheses 1–3.
A compilation 6 UVP size spectrum profiles from the ETSP (Bianchi et al., 2018), found that the spectral slope increased slightly between 100 and 1000 m, largely driven by increased abundance of large particles relative to an oxic water column, rather than decreased abundance of small particles. This is not consistent with Hypothesis 1 (slow remineralization during denitrification) and is most consistent with the predictions of Hypothesis 3 (diffusion limitation of carbon oxidation in large particles). However, this analysis was far too limited to draw firm conclusions, and further analysis of UVP observations from all three of the ocean’s major OMZs is required in order to assess systematic patterns in their particle size spectra.
Because each UVP image serves only as a snapshot of the particle flux, and can be strongly influenced by pulses of organic matter, compilation of numerous UVP profiles from a single location or region will be critical to identify coherent features of the particle spectrum. The new Ecotaxa repository for UVP images (Picheral et al., 2017) may now contain sufficient data to construct meaningful composites for comparing OMZs to oxic tropical regions, but this analysis is beyond the scope of the current study. By showing that different flux attenuation mechanisms leave an imprint in particle size spectra, we hope to motivate further work scrutinizing the existing UVP archives, and new field campaigns that collect targeted UVP data across oxygen gradients in the ocean.
Given that a combination of oxygen effects likely operates in the OMZs (with different mechanisms potentially dominating in the upper and low oxyclines and the OMZ core) size spectra from these regions may exhibit a complex blend of the end-member patterns shown in Figure 4. In this case, UVP data alone may be insufficient to adequately determine the relative importance of each mechanism, and future work will greatly benefit from collecting additional particulate data alongside UVP profiles. Because disaggregation can produce particles smaller than the lower UVP detection limit of ∼100 μm (Lam and Marchal, 2015), extending the spectral range using alternative particle-counting instruments (Stemmann and Boss, 2012) or microscopy (Durkin et al., 2015) would help quantify the difference in disaggregation rates between OMZs and oxygenated waters. Additionally, measuring trace-metal (Janssen et al., 2014) and genomic (Ganesh et al., 2015) indicators of particle interior redox conditions would further elucidate the degree to which diffusion-limited microenvironments impede carbon oxidation in OMZs. When supplied with this broad range of observational constraints, our mechanistic particle model will be better equipped to assess the balance of processes that control particle fluxes through OMZs.
It is also worth noting that while our model includes the main processes that contribute to particle flux attenuation with depth, it does not consider other processes that may contribute to weaker flux attenuation in OMZ. These include particle formation at depth (e.g., by autotrophy or zooplankton vertical migration), zooplankton detritivory, and various effects of ballasting material, among others (Keil et al., 2016). For completeness, these processes should be considered in future modeling of OMZ particle fluxes.
Conclusion
The key contribution of this study is the new constraints we have placed on the efficiency of the biological pump in OMZ regions, by reconstructing time-mean particle flux profiles for the Eastern Tropical North Pacific and the Arabian Sea from geochemical tracer data. These reconstructions revealed slow particle flux attenuation over depth in the suboxic water column, consistent with previous evidence from sediment trap “snapshots” (Devol and Hartnett, 2001; Keil et al., 2016) and confirming that this is a systematic feature of OMZ particle fluxes. Compared to surrounding tropical waters, the fraction of organic matter sinking from the euphotic zone that penetrates the mesopelagic zone to 1000 m is more than twice as large in OMZs. The convergence of OMZ flux profiles toward those from oxic water columns revealed in our reconstructions beneath 1000 m providers compelling new evidence that suboxia itself is the factor driving slow flux attenuation (Figures 2C,F).
Using a mechanistic particle flux model we showed that the shape of OMZ flux profiles can be explained by any of three oxygen effects: (i) very slow carbon oxidation during denitrification (Devol and Hartnett, 2001; Van Mooy et al., 2002); (ii) dramatically reduced fragmentation in suboxic waters due to exclusion of zooplankton (Cavan et al., 2017); (iii) diffusion limitation of carbon oxidation in large particles (Bianchi et al., 2018). However, each of these mechanisms makes unique predictions about changes in particle size spectra through the water column, opening the possibility to extract mechanistic information from UVP observations. The idealized end-member cases presented here serve as a starting point for interpreting patterns in UVP spectral data from OMZ regions.
We suggest that combining existing UVP archives, new targeted observations across oxygen gradients, and mechanistic models like the one used here is a promising approach for unraveling the processes driving efficient biological pump of OMZs. In turn, this will allow for improved prediction of carbon sequestration in the future ocean, where continued warming and deoxygenation are likely to reshape the efficiency of the biological pump (Laufkötter et al., 2017; Cram et al., 2018).
Data Availability Statement
The datasets presented in this study can be found in online repositories. The names of the repository/repositories and accession number(s) can be found below: 10.6084/m9.figshare. 12401000.
Author Contributions
TW and DB designed the study and analyzed the results. TW conducted the flux reconstruction analysis, implemented the mechanistic particle flux model, and wrote the manuscript, with input from DB. Both authors contributed to the article and approved the submitted version.
Funding
This work was supported by NSF grants OCE-1635414 awarded to TW and OCE-1635632 awarded to DB.
Conflict of Interest
The authors declare that the research was conducted in the absence of any commercial or financial relationships that could be construed as a potential conflict of interest.
Acknowledgments
We thank Tim DeVries for providing access to the updated version of the Ocean Circulation Inverse Model (OCIM) used in our flux reconstructions, and Jacob Cram for discussions that helped motivate this study.
References
Alldredge, A. (1998). The carbon, nitrogen and mass content of marine snow as a function of aggregate size. Deep Sea Res. Part I Oceanogr. Res. Papers 45, 529–541. doi: 10.1016/s0967-0637(97)00048-4
Bianchi, D., Galbraith, E. D., Carozza, D. A., Mislan, K. A. S., and Stock, C. A. (2013). Intensification of open-ocean oxygen depletion by vertically migrating animals. Nat. Geosci. 6, 545–548. doi: 10.1038/ngeo1837
Bianchi, D., Weber, T. S., Kiko, R., and Deutsch, C. (2018). Global niche of marine anaerobic metabolisms expanded by particle microenvironments. Nat. Geosci. 11, 263–268. doi: 10.1038/s41561-018-0081-0
Bopp, L., Resplandy, L., Orr, J. C., Doney, S. C., Dunne, J. P., Gehlen, M., et al. (2013). Multiple stressors of ocean ecosystems in the 21st century: projections with CMIP5 models. Biogeosciences 10, 3627–3676. doi: 10.5194/bgd-10-3627-2013
Boyd, P. W., Claustre, H., Levy, M., Siegel, D. A., and Weber, T. (2019). Multi-faceted particle pumps drive carbon sequestration in the ocean. Nature 568, 327–335. doi: 10.1038/s41586-019-1098-2
Briggs, N., Dall’olmo, G., and Claustre, H. (2020). Major role of particle fragmentation in regulating biological sequestration of CO⁢sub>2⁢/sub> by the oceans. Science 367:791. doi: 10.1126/science.aay1790
Buesseler, K. O., Lamborg, C. H., Boyd, P. W., Lam, P. J., Trull, T. W., Bidigare, R. R., et al. (2007). Revisiting Carbon Flux Through the Ocean’s Twilight Zone. Science 316, 567–570.
Cavan, E. L., Trimmer, M., Shelley, F., and Sanders, R. (2017). Remineralization of particulate organic carbon in an ocean oxygen minimum zone. Nat. Commun. 8:14847.
Cram, J. A., Weber, T., Leung, S. W., McDonnell, A. M. P., Liang, J.-H., and Deutsch, C. (2018). The Role of Particle Size, Ballast, Temperature, and Oxygen in the Sinking Flux to the Deep Sea. Glob. Biogeochem. Cycles 32, 858–876. doi: 10.1029/2017gb005710
Devol, A. H. (1978). Bacterial oxygen uptake kinetics as related to biological processes in oxygen deficient zones of the oceans. Deep Sea Res. 25, 137–146. doi: 10.1016/0146-6291(78)90001-2
Devol, A. H., and Hartnett, H. E. (2001). Role of the oxygen-deficient zone in transfer of organic carbon to the deep ocean. Limnol. Oceanogr. 46, 1684–1690. doi: 10.4319/lo.2001.46.7.1684
DeVries, T., and Holzer, M. (2019). Radiocarbon and Helium Isotope Constraints on Deep Ocean Ventilation and Mantle-3He Sources. J. Geophys. Res. 124, 3036–3057. doi: 10.1029/2018jc014716
DeVries, T., Liang, J.-H., and Deutsch, C. (2014). A mechanistic particle flux model applied to the oceanic phosphorus cycle. Biogeosciences 11, 5381–5398. doi: 10.5194/bg-11-5381-2014
DeVries, T., Primeau, F., and Deutsch, C. (2012). The sequestration efficiency of the biological pump. Geophys. Res. Lett. 39, 1–5.
DeVries, T., and Weber, T. (2017). The export and fate of organic matter in the ocean: new constraints from combining satellite and oceanographic tracer observations. Glob. Biogeochem. Cycles 31, 535–555. doi: 10.1002/2016gb005551
Durkin, C. A., Estapa, M. L., and Buesseler, K. O. (2015). Observations of carbon export by small sinking particles in the upper mesopelagic. Mar. Chem. 175, 72–81. doi: 10.1016/j.marchem.2015.02.011
Froelich, P. N., Klinkhammer, G., Bender, M. A. A., Luedtke, N., Heath, G. R., Cullen, D., et al. (1979). Early oxidation of organic matter in pelagic sediments of the eastern equatorial Atlantic: suboxic diagenesis. Geochim. Cosmochim. Acta 43, 1075–1090. doi: 10.1016/0016-7037(79)90095-4
Ganesh, S., Bristow, L. A., Larsen, M., Sarode, N., Thamdrup, B., and Stewart, F. J. (2015). Size-fraction partitioning of community gene transcription and nitrogen metabolism in a marine oxygen minimum zone. ISME J. 9, 2682–2696. doi: 10.1038/ismej.2015.44
Garcia, H. E., Locarnini, R. A., Boyer, T. P., Antonov, J. I., Baranova, O. K., Zweng, M. M., et al. (2013). “World Ocean Atlas 2013, Volume 4: dissolved Inorganic Nutrients (phosphate, nitrate, silicate), in NOAA Atlas NESDIS 68, Ed. S. Levitus (Washington, DC: U.S. Government Printing Office).
Iversen, M., and Ploug, H. (2013). Temperature effects on carbon-specific respiration rate and sinking velocity of diatom aggregates–potential implications for deep ocean export processes. Biogeosciences 10, 4073–4085. doi: 10.5194/bg-10-4073-2013
Janssen, D. J., Conway, T. M., John, S. G., Christian, J. R., Kramer, D. I., Pedersen, T. F., et al. (2014). Undocumented water column sink for cadmium in open ocean oxygen-deficient zones. Proc. Natl. Acad. Sci. U.S.A. 111, 6888–6893. doi: 10.1073/pnas.1402388111
Keil, R. G., Neibauer, J. A., Biladeau, C., Elst, K. V. D., and Devol, A. H. (2016). A multiproxy approach to understanding the “enhanced” flux of organic matter through the oxygen-deficient waters of the Arabian Sea. Biogeosciences 13, 2077–2092. doi: 10.5194/bg-13-2077-2016
Kiko, R., Hauss, H., Buchholz, F., and Melzner, F. (2016). Ammonium excretion and oxygen respiration of tropical copepods and euphausiids exposed to oxygen minimum zone conditions. Biogeosciences 13, 2241–2255. doi: 10.5194/bg-13-2241-2016
Lam, P. J., and Marchal, O. (2015). Insights into particle cycling from thorium and particle data. Ann. Rev. Mar. Sci 7, 159–184. doi: 10.1146/annurev-marine-010814-015623
Laufkötter, C., John, J. G., Stock, C. A., and Dunne, J. P. (2017). Temperature and oxygen dependence of the remineralization of organic matter. Glob. Biogeochem. Cycles 31, 1038–1050. doi: 10.1002/2017gb005643
Lu, Z., Hoogakker, B. A. A., Hillenbrand, C.-D., Zhou, X., Thomas, E., Gutchess, K. M., et al. (2016). Oxygen depletion recorded in upper waters of the glacial Southern Ocean. Nat. Commun. 7:11146.
Marsay, C. M., Sanders, R. J., Henson, S. A., Pabortsava, K., and Achterberg, E. P. (2014). Attenuation of sinking particulate organic carbon flux through the mesopelagic ocean. PNAS 112, 1089–1094. doi: 10.1073/pnas.1415311112
Martin, J. H., Knauer, G. A., Karl, D. M., and Broenkow, W. W. (1987). VERTEX: carbon cycling in the northeast Pacific. Deep Sea Res. Part A Oceanogr. Res. Papers 34, 267–285. doi: 10.1016/0198-0149(87)90086-0
McDonnell, A. M. P., Boyd, P. W., and Buesseler, K. O. (2015). Effects of sinking velocities and microbial respiration rates on the attenuation of particulate carbon fluxes through the mesopelagic zone. Glob. Biogeochem. Cycles 29, 175–193. doi: 10.1002/2014gb004935
Passow, U., and Carlson, C. (2012). The biological pump in a high CO2 world. Mar. Ecol. Progr. Ser. 470, 249–271.
Pavia, F. J., Anderson, R. F., Lam, P. J., Cael, B. B., Vivancos, S. M., Fleisher, M. Q., et al. (2019). Shallow particulate organic carbon regeneration in the South Pacific Ocean. Proc. Natl. Acad. Sci. U.S.A. 116:9753. doi: 10.1073/pnas.1901863116
Picheral, M., Colin, S., and Irisson, J.-O. (2017). EcoTaxa, a tool for the taxonomic classification of images. Available online at: http://ecotaxa.obs-vlfr.fr
Picheral, M., Guidi, L., Stemmann, L., Karl, D. M., Iddaoud, G., and Gorsky, G. (2010). The Underwater Vision Profiler 5: an advanced instrument for high spatial resolution studies of particle size spectra and zooplankton. Limnol. Oceanogr. 8, 462–473. doi: 10.4319/lom.2010.8.462
Ploug, H. (2001). Small-scale oxygen fluxes and remineralization in sinking aggregates. Limnol. Oceanogr. 46, 1624–1631. doi: 10.4319/lo.2001.46.7.1624
Schmidtko, S., Stramma, L., and Visbeck, M. (2017). Decline in global oceanic oxygen content during the past five decades. Nature 542, 335–339. doi: 10.1038/nature21399
Seibel, B. A. (2011). Critical oxygen levels and metabolic suppression in oceanic oxygen minimum zones. J. Exp. Biol. 214:326. doi: 10.1242/jeb.049171
Stemmann, L., and Boss, E. (2012). Plankton and particle size and packaging: from determining optical properties to driving the biological pump. Ann. Rev. Mar. Sci. 4, 263–290. doi: 10.1146/annurev-marine-120710-100853
Stemmann, L., Picheral, M., Guidi, L., Lombard, F., Prejger, F., Claustre, H., et al. (2012). “Assessing the spatial and temporal distributions of zooplankton and marine particles using the Underwater Vision Profiler,” in Sensors for Ecology: Towards Integrated Knowledge of Ecosystems, J.F. Le Galliard, J.F. Guarini, and F. Gaill (France: Institut Ecologie et Environnement), 119.
Van Mooy, B. A. S., Keil, R. G., and Devol, A. H. (2002). Impact of suboxia on sinking particulate organic carbon: enhanced carbon flux and preferential degradation of amino acids via denitrification. Geochim. Cosmochim. Acta 66, 457–465. doi: 10.1016/s0016-7037(01)00787-6
Weber, T., Cram, J. A., Leung, S. W., Devries, T., and Deutsch, C. (2016). Deep ocean nutrients imply large latitudinal variation in particle transfer efficiency. Proc. Natl. Acad. Sci. U.S.A. 113, 8606–8611. doi: 10.1073/pnas.1604414113
Wishner, K. F., Gowing, M. M., and Gelfman, C. (1998). Mesozooplankton biomass in the upper 1000m in the Arabian Sea: overall seasonal and geographic patterns, and relationship to oxygen gradients. Deep Sea Res. Part II Top. Stud. Oceanogr. 45, 2405–2432. doi: 10.1016/s0967-0645(98)00078-2
Wishner, K. F., Outram, D. M., Seibel, B. A., Daly, K. L., and Williams, R. L. (2013). Zooplankton in the eastern tropical north Pacific: boundary effects of oxygen minimum zone expansion. Deep Sea Res. Part I Oceanogr. Res. Papers 79, 122–140. doi: 10.1016/j.dsr.2013.05.012
Wishner, K. F., Seibel, B. A., Roman, C., Deutsch, C., Outram, D., Shaw, C. T., et al. (2018). Ocean deoxygenation and zooplankton: very small oxygen differences matter. Sci. Adv. 4:eaau5180. doi: 10.1126/sciadv.aau5180
Keywords: biological pump, ocean carbon sequestration, particle flux, oxygen minimum zones, remineralization, disaggregation, particle size spectra
Citation: Weber T and Bianchi D (2020) Efficient Particle Transfer to Depth in Oxygen Minimum Zones of the Pacific and Indian Oceans. Front. Earth Sci. 8:376. doi: 10.3389/feart.2020.00376
Received: 30 May 2020; Accepted: 11 August 2020;
Published: 04 September 2020.
Edited by:
Makio Honda, Japan Agency for Marine-Earth Science and Technology (JAMSTEC), JapanReviewed by:
Morgan Reed Raven, University of California, Santa Barbara, United StatesFrank Pavia, California Institute of Technology, United States
Copyright © 2020 Weber and Bianchi. This is an open-access article distributed under the terms of the Creative Commons Attribution License (CC BY). The use, distribution or reproduction in other forums is permitted, provided the original author(s) and the copyright owner(s) are credited and that the original publication in this journal is cited, in accordance with accepted academic practice. No use, distribution or reproduction is permitted which does not comply with these terms.
*Correspondence: Thomas Weber, t.weber@rochester.edu