- 1Geoscience Australia, Canberra, ACT, Australia
- 2Istituto Nazionale di Geofisica e Vulcanologia, Roma, Italy
At far-field coasts the largest tsunami waves may occur many hours post-arrival, and hazardous waves may persist for more than 1 day. Such tsunamis are often simulated by nesting high-resolution nonlinear shallow water models (covering sites of interest) within low-resolution reduced-physics global-scale models (to efficiently simulate propagation). These global models often ignore friction and are mathematically energy conservative, so in theory the modeled tsunami will persist indefinitely. In contrast, real tsunamis exhibit slow dissipation at the global-scale with an energy e-folding time of approximately 1 day. How strongly do these global-scale approximations affect nearshore tsunamis simulated at far-field coasts? To investigate this we compare modeled and observed tsunamis at sixteen nearshore tide-gauges in Australia, generated by the following earthquakes:
Introduction
Large earthquake-tsunamis can be hazardous even at far-field coasts. For example the 1946 Aleutian earthquake-tsunami caused 159 deaths in Hawaii; the 1960 Chile earthquake-tsunami caused 142 deaths in Japan; the 2004 Sumatra earthquake-tsunami led to 300 deaths in Somalia (Fritz and Borrero, 2006; Okal, 2011). Smaller yet more-common tsunamis are also of interest for risk management because they can generate hazardous currents and minor inundation, with potential to harm people, damage assets, and disrupt economic activity at ports (e.g., Beccari, 2009; Borrero et al., 2015b). To mitigate the risk, early warning systems and hazard assessments are employed to guide emergency management and planning (Kânoğlu et al., 2015; Grezio et al., 2017; Davies and Griffin, 2018). They are underpinned by numerical models of tsunami propagation and inundation which exploit various approximations for computational tractability (e.g., Gica et al., 2008; Greenslade et al., 2011; Lorito et al., 2015; Setiyono et al., 2017; Volpe et al., 2019; Davies and Griffin, 2020). It is important to understand how well such models simulate key quantities of interest for applications (e.g., maximum wave heights and current speeds, their timing, and the duration of dangerous waves). Herein we focus on the tsunami wave size at far-field coasts in the period from 0-to-60 h post-earthquake. This is motivated by the fact that hazardous waves can persist for several days (Tang et al., 2012). Some historical tsunami observations in Australia and New Zealand exhibited wave maxima arriving 1-to-2 days post earthquake, long after the initial tsunami arrival (Pattiaratchi and Wijeratne, 2009; Borrero et al., 2015a).
For tsunami modeling applications at far-field sites, it is often advantageous to simulate global-scale propagation with linear models, noting nonlinearity should be small because ocean depths are generally much larger than tsunami amplitudes (Shuto, 1991). Linear models are typically faster to solve numerically (e.g., Liu et al., 2008; Baba et al., 2014), and if many scenarios are required then even greater speedups follow by constructing solutions
Here
Tsunami propagation models at ocean-basin scales also often neglect friction, which has little effect on deep ocean tsunami simulations for a few hours post-arrival (Tang et al., 2012; Fujii and Satake, 2013; Allgeyer and Cummins, 2014; Baba et al., 2017; Heidarzadeh et al., 2018; Davies, 2019). However the frictionless shallow water equations are energy conservative, assuming smooth solutions, which mathematically implies the tsunami persists forever (Arakawa and Hsu, 1990; Fjordholm et al., 2011; Tang et al., 2012). In contrast real global-scale tsunamis eventually dissipate. Observations at coastal and deep-ocean gauges in the Pacific and Indian Oceans suggest an exponential time-decay of available potential energy, with an e-folding timescale about
Quadratic bottom friction (e.g., Manning or Chezy) is most common in tsunami models, but the solutions cannot be represented with unit-sources (Eq. 1) over timescales for which the nonlinear dissipation is important. To represent global-scale tsunami dissipation within a linear hydrodynamic model, Fine et al. (2013) and Kulikov et al. (2014) combined the linear shallow water equations (LSWE) with a constant linear-friction term. Although not justified from hydrodynamic theory, this model implies an exponential time-decay of the tsunami’s energy which is consistent with observational studies. The linear-friction coefficient may thus be estimated using observed tsunami decay timescales (Fine et al., 2013; Kulikov et al., 2014). The model was implemented using numerical methods that have good energy conservation to prevent numerical dissipation from dominating the results at late times, which was reportedly easier to achieve by modifying a linear model (Fine et al., 2013; Kulikov et al., 2014). Numerical dissipation is often significant for models solving the nonlinear shallow water equations (NSWE) and can even exceed the physical dissipation of global scale tsunamis at computationally practical resolutions (Popinet, 2011; Tang et al., 2012; Tolkova, 2014). This has been used to explain persistent under-estimation of late time tsunami wave heights in some nested nearshore models, even without any friction in the global propagation model (Tang et al., 2012; Tolkova, 2014). However, in the absence of significant numerical dissipation, friction is necessary to represent the late-time energy decay observed in global-scale tsunamis.
Modeled coastal tsunami wave heights are likely to be qualitatively affected at sufficiently late times by the treatment of global-scale dissipation. This has the potential to affect tsunami hazard assessments (e.g., runup maxima) and warnings (e.g., warning cancellation). Our study seeks to better understand the practical significance of this issue, focusing on the empirical performance of alternative models rather than the physics of tsunami dissipation. To this end we test several global-to-local scale nested-grid tsunami models by comparison with tsunami observations at tide-gauges in Australia, for the period 0–60 h post-earthquake. The alternative models differ only in their treatment of friction on the global-scale grid (where propagation is simulated with some variant of the LSWE); in all cases the tsunami at nearshore sites of interest is simulated on nested grids which solve the NSWE with Manning-friction. The tsunami initial conditions are derived from published earthquake-source inversions. We focus on relatively simple tsunami models which are computationally cheap and very practical in applications, while acknowledging that tsunami propagation may be simulated more accurately using computationally intensive approaches that include higher order physics such as dispersion, loading, seawater density stratification, and self-gravitation (Allgeyer and Cummins, 2014; Baba et al., 2017). Model improvements may also be sought through use of higher quality elevation data outside the primary area of interest, combined with higher model resolution, to better represent remote reflections (Kowalik et al., 2008; Geist, 2009). The practical benefit of very high-accuracy propagation modeling may be limited for hazard assessments, because plausible source variations can have an even greater effect on the simulated tsunami (Davies, 2019), although further study of this issue is warranted.
The paper is structured as follows. Section 2.1 presents the earthquake-tsunami sources (initial water-surface perturbations) used to model each historic event. Section 2.2 presents the tide-gauge data used to test each model. Section 2.3 reviews the tsunami model setup. Section 2.4 details the alternative reduced-physics hydrodynamic models that are tested. Section 3.1 illustrates each model’s performance using examples from three historic tsunamis. Sections 3.2 and 3.3 compare the modeled and observed tsunami maxima for all sites and events. Section 3.4 considers how the model errors are affected by the tsunami source.
Materials and Methods
Earthquake Source Models for Historical Tsunamis
Five historic tsunamigenic earthquake events were analyzed in this study:
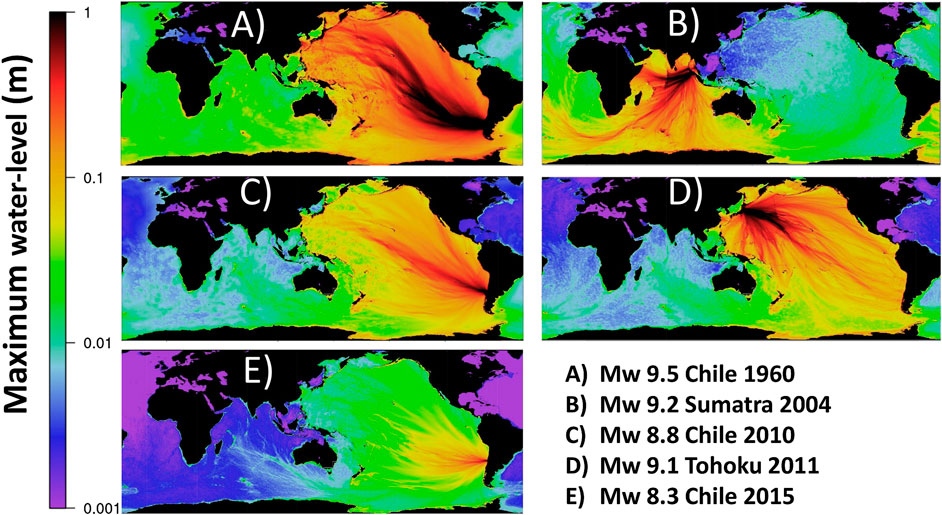
FIGURE 1. Tsunami events considered in this study. The images are model outputs derived using the solver from Section 2.4.3 on the global grid and a subset of the source models in Figure 2 [(A): H19, (B): F07, (C): L11, (D): Y18, (H): W17].
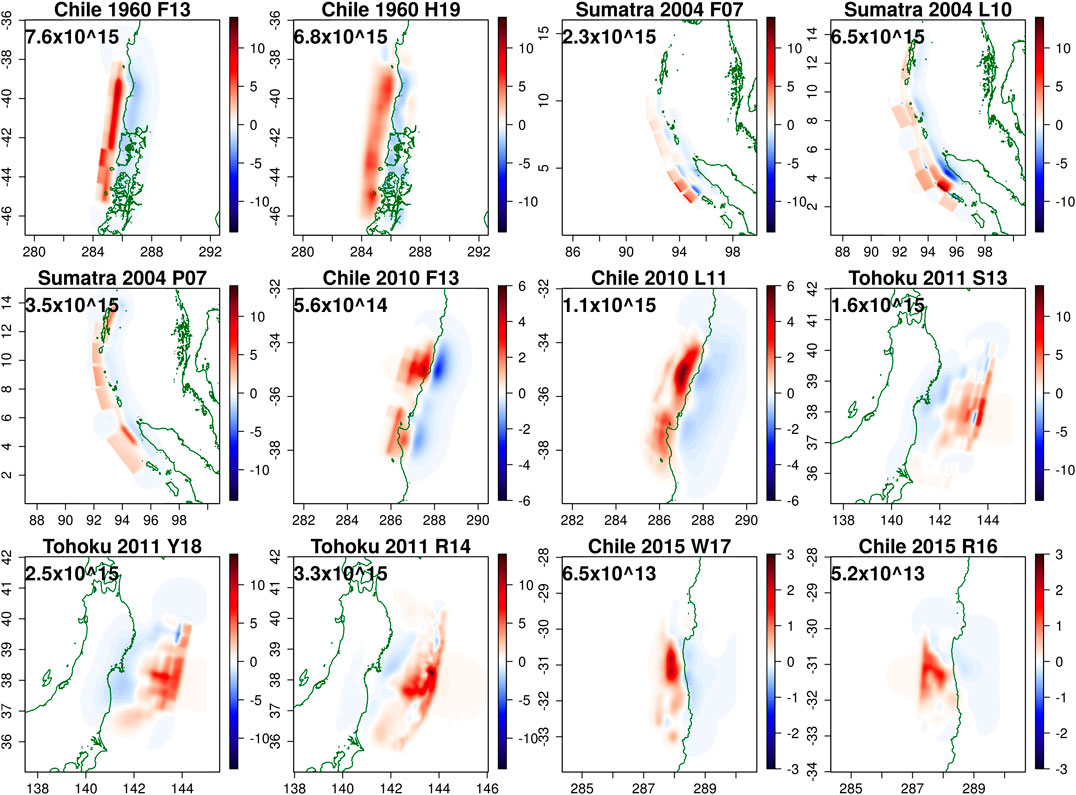
FIGURE 2. The vertical co-seismic displacement for all source models. Text beneath the title gives the initial available potential energy of the ocean surface displacement (kg m2/s2, derived by integrating Eq. 5 in space; there is no contribution from deformation on land). The sources are based on finite-fault inversions in the following publications: F13 (Fujii and Satake, 2013); H19 (Ho et al., 2019); F07 (Fujii and Satake, 2007); L10 (Lorito et al., 2010); P07 (Piatanesi and Lorito, 2007); L11 (Lorito et al., 2011); S13 (Satake et al., 2013); Y18 (Yamazaki et al., 2018); R14 (Romano et al., 2014); W17 (Williamson et al., 2017); R16 (Romano et al., 2016).
Most tsunami initial conditions in Figure 2 were derived by computing the vertical co-seismic deformation from the published fault-geometries and slip vectors, assuming instantaneous rupture in a homogeneous elastic half-space (Okada, 1985; Meade, 2007). The effect of horizontal deformation over a sloping seabed (Tanioka and Satake, 1996) was not included as this tends to add shorter waves to the source which may not be well simulated with our long-wave hydrodynamic model (Saito et al., 2014). However source R14 was taken directly from the finite-element model of Romano et al. (2014); this includes horizontal components so is rougher than our other sources (Figure 2). Source H19 was derived from water-surface unit-sources following Ho et al. (2019). A Kajiura filter (Kajiura, 1963; Glimsdal et al., 2013) was applied to all models except the water-surface inversion H19.
The studies on which our source models are based generally inverted the source using deep sea and/or coastal tsunami data, sometimes combined with geodetic data (GPS, InSAR, leveling) and occasionally teleseismic data. None made use of the Australian tide-gauge data studied herein. All inverted the source with linear combinations of earthquake and/or tsunami Green’s functions, in some cases including regularization constraints (L11, Y14, R16, W17, Y18). Most simulated the co-seismic displacement assuming a homogeneous elastic half-space, although R14 used a 3D finite-element model to account for material heterogeneity. Tsunami Green’s functions were mostly modeled with shallow water schemes, although R14, R16 and Y18 employed a non-hydrostatic model. Some papers reported both a best-fitting source model and an average source model; in such cases we always used the former. Full details are available in the repository (see Data Availability Statement).
Test Sites and Data
The model is compared with data at 16 sites in Australia that have good bathymetry and relatively good quality tsunami observations at tide-gauges (Figure 3). Most gauges only record one of the modeled tsunami events, although Fort Denison in Sydney Harbor records all five (Figure 3). By using multiple sites with good quality data for each historic tsunami, we reduce the risk that site-specific factors limiting the model performance are mistakenly attributed to source/propagation model biases (e.g., undiagnosed errors in the bathymetry or tide-gauge records). No gauge observations were rejected on the basis of disagreement with our tsunami model, to avoid biasing the results.
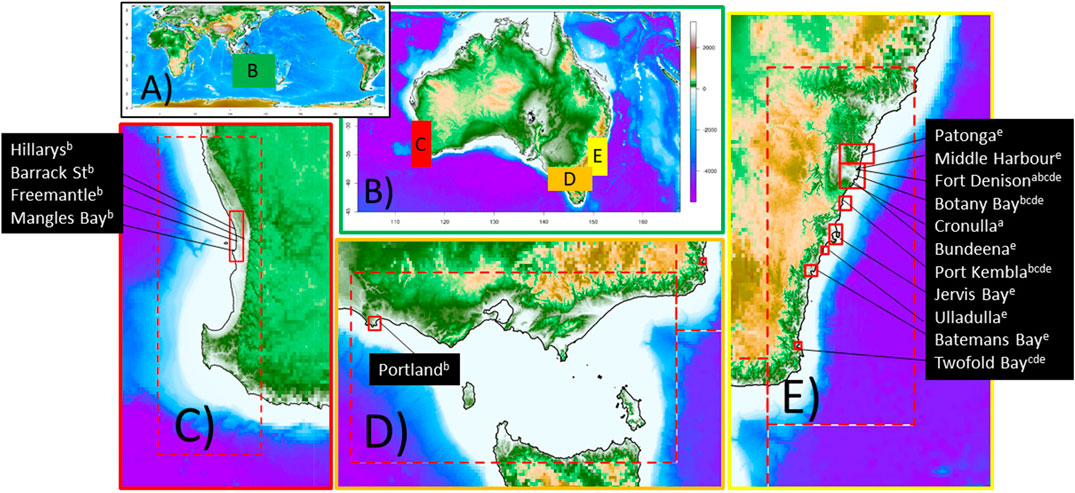
FIGURE 3. The model extent and tide-gauge locations. Superscripts on tide-gauges (a,b,c,d,e) indicate the tsunamis for which we have data, corresponding to the 1960, 2004, 2010, 2011, 2015 events, respectively. (A) The global grid has a spatial resolution of 1-arc-minute and is modeled with reduced-physics approaches; (B) zoom near Australia which shows the three regions where refined grids are used and the NSWE are solved; (C–E) the refined grids around western and south-eastern Australia. Dashed boxes denote regions with 1/7-arc-minute spatial resolution (
Tide-gauge data was obtained from a range of sources (see Acknowledgments). To detide the observations we first subtracted tidal predictions, which were either provided with the tide-gauge data, or obtained from TPXO7.2 (Egbert and Erofeeva, 2002). Next a high pass filter was used to remove the residual long-period sea-level variations by applying a discrete Fourier transform and zeroing the amplitude of waves with period exceeding a threshold. At most sites the tides are semi-diurnal and the high pass filter threshold was 3 h. At our Western Australia sites the tides are diurnal and a longer threshold was used (6 h); the 3 h threshold led to non-stationary oscillations in the non-tsunami sea-level component at the time of the Sumatra 2004 tsunami, suggesting a longer threshold is appropriate. Irrespective this has little impact on the tsunami signal. In all cases the detided tsunami record might still be affected by other physical processes (e.g., seiching due to transient atmospheric forcings) or measurement errors (e.g., excess mechanical smoothing in the gauge, Satake et al., 1988); however it represents our best estimate of the tsunami signal.
Most gauges used herein have a sampling frequency of 1-min. For the Sumatra 2004 event, three of the four gauges used in Western Australia have 5-min sampling frequency (excepting Hillarys which has a 1-min frequency). For the 1960 Chile event only two gauges are available (Fort Denison and Cronulla), both of which were digitized at approximately 2-min sampling frequency from scans of the analogue tide-gauge records (the former by Wilson et al., 2018). Although in recent decades additional 15-min tide-gauge data is available at several sites, this was not used because it under-samples the tsunami making interpretation more difficult (Rabinovich et al., 2011). At Port Kembla the outer-harbour gauge is used; the inner-harbour gauge was not used based on advice from the data custodians (NSW Port Authority, personal communication 2020) and the issues noted in Allen and Greenslade (2016).
Tsunami Model
The tsunami is simulated from source to the tide-gauges using the open-source hydrodynamic model SWALS, which solves several variants of the shallow water equations in spherical or Cartesian coordinates and was used for the 2018 Australian Probabilistic Tsunami Hazard Assessment (Davies and Griffin, 2018). The source code includes a validation test-suite of more than 20 analytical, laboratory and field problems, including well-known tests such as those in NTHMP (2012) (excluding landslides) and two recent field-scale NTHMP problems (Macías et al., 2020). It uses structured grids with two-way nesting, and flux-correction to enforce conservation at nested grid boundaries. Different grids can employ different solvers simultaneously; this is used in the current study to test a range of reduced-physics solvers on the global grid (only) while solving the NSWE on refined grids.
The global scale tsunami propagation is simulated on a 1 arc-minute grid (Figure 3A) using bathymetry derived from GEBCO 2014 (Weatherall et al., 2015) and GA250 (Whiteway, 2009) (details in Davies and Griffin, 2018). East-West periodic boundary conditions are used. The southern boundary (79° S) is covered by land. At the northern boundary (68° N) a reflective wall is imposed; while artificial this closes the model and facilitates energy conservation calculations (as SWALS does not track energy fluxes through boundaries). Physically this is reasonable given little tsunami energy will radiate through either Bering strait (which is small) or north Atlantic (which is very far from our tsunami sources). The reduced-physics solvers used on this grid are described in Sections 2.4.2–2.4.4.
Nearshore regions containing good-quality tide-gauge observations are simulated on refined grids (1/7 and 1/49 arc-minutes, (Figures 3C–E), which are linked with the global grid and each other via two-way nesting. Good quality nearshore elevation data is used on the highest-resolution grids, mostly derived from LIDAR and gap-filled using available single-beam bathymetric surveys and gridded elevation from prior studies (see Acknowledgments; Allen and Greenslade, 2016; Wilson and Power, 2018). Breakwalls close to our tide-gauges were burned into the model’s elevation to ensure they are represented irrespective of the grid resolution. On these nearshore grids the tsunami is simulated using the NSWE with Manning-friction (Section 2.4.1).
To check the numerical convergence of the model at the default resolution (1, 1/7 and 1/49 arc-minute nested grids), higher resolution simulations (1/2, 1/14, 1/98 arc-minute) were run for two of our sources (F07, Y18), using the reduced physics solver of Section 2.4.3 on the coarsest grid. Results were compared with the default model at twelve sites with corresponding good-quality observations, as this is where the model results will be used. Waveforms were similar although not completely convergent, with tsunami maxima showing changes ranging from −12% to +21% with a median of 2% (typical examples in Figure 4). In general greater differences are anticipated between the models and data, so the default resolution was considered adequate for the current study.
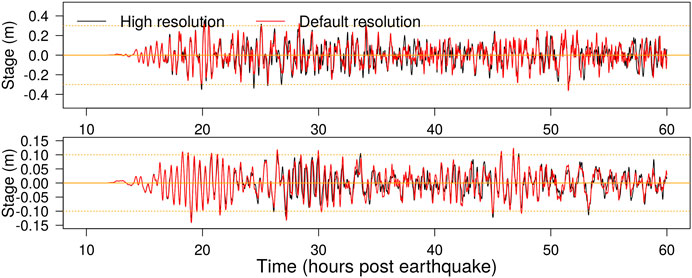
FIGURE 4. Comparison of nearshore gauge results in two models; one with default resolution (1, 1/7, 1/49 arc-minute nested grids) and one with higher-resolution (1/2, 1/14, 1/98 arc-minute nested grids). Locations correspond to tide-gauges at Port Kembla (top) and Botany Bay (bottom); results elsewhere were qualitatively similar. The simulation corresponds to the Tohoku 2011 Y18 source, using Manning-friction in the global model.
Hydrodynamic Simplifications for Global Scale Tsunami Propagation
Several reduced-physics solvers are tested on the global grid, all based on the linear shallow water equations (LSWE) with alternative dissipation models:
(1) Frictionless (Section 2.4.2)
(2) Nonlinear Manning-friction (Section 2.4.3)
(3) Constant linear-friction (Section 2.4.4)
These represent computationally efficient alternatives to solving the full NSWE for global tsunami propagation.
The frictionless LSWE were tested because they are often used to simulate large-scale tsunami propagation (e.g., Choi et al., 2003; Burbidge et al., 2008; Thio, 2015; Li et al., 2016; Davies and Griffin, 2020) and arguably represent the simplest model of this kind. The Manning-friction approach was tested because it is closer to the full NSWE; however over timescales where dissipation is important, the nonlinearity will prevent a unit-source implementation. The constant linear-friction approach (Fine et al., 2013; Kulikov et al., 2014) was tested because it enables dissipation to be included in a linear framework, with all the associated efficiency benefits. Furthermore, although unit-sources are not employed in this study, below it is shown that solutions of the constant linear-friction model can be well approximated with a simple transformation of solutions of the frictionless LSWE, which is convenient because the latter are available in several existing tsunami propagation databases (e.g., Thio, 2015; Li et al., 2016; Davies and Griffin, 2018).
Nonlinear Shallow Water Equations
The 2D NSWE with friction are (e.g., Baba et al., 2015; Behrens and Dias, 2015):
Here
If friction is neglected (
Here the depth-integrated energy density
with water density ρ (kg/m3), and
By definition the integral of
On refined grids the model herein solves Eq. 2 in flux-conservative form with a constant Manning-friction
Linear Shallow Water Equations Without Friction
In the deep ocean even large earthquake tsunamis are of small amplitude relative to ocean depths and velocities are slight compared to the gravity wave speed. Under these conditions Eq. 2 is well approximated with the frictionless LSWE:
Here
but is included in Eq. 6 to facilitate extensions below. These equations are also energy conservative.
Herein the LSWE are solved numerically with the classical leap-frog scheme (Goto and Ogawa, 1997). Importantly this scheme has good numerical energy conservation; in our twelve simulations that used the frictionless LSWE on the global grid the numerically integrated energy (Eq. 3) varied within [−2.7%, 0.25%] over 60 h. Minor dissipation is expected in the full model because refined grids solve the NSWE with friction and employ a more dissipative finite-volume numerical scheme; minor energy increase can occur because the leap-frog scheme is not perfectly energy conservative. In comparison the literature suggests global tsunami propagation simulations often have much greater numerical dissipation; Tang et al. (2012) and Tolkova (2014) used several variants of the MOST and CLIFFS solvers to simulate the Tohoku tsunami globally without friction and found
Linear Shallow Water Equations With Manning-Friction
To add dissipation to the global model it is natural to consider a Manning-friction closure in Eq. 6. For computational efficiency our approach exploits the approximation
where n is the Manning-friction coefficient, which was set to 0.035 on the global grid (slightly larger than our nearshore Manning coefficient of 0.03). Real tsunamis likely dissipate due to a mixture of nonlinear bottom friction in shallow regions, and other deep-ocean dissipation mechanisms which are important for simulating tides (Egbert and Ray, 2000; Buijsman et al., 2015; Kleermaeker et al., 2019). Our global model poorly resolves most shallow nearshore areas, neglects nonlinear tidal interactions, and ignores other dissipation mechanisms, so the Manning model is likely a strong simplification of reality. In preliminary simulations we also tried a smaller global Manning coefficient (
Equation 8 is nonlinear so solutions cannot be constructed from unit-sources (at least not over timescales long enough for nonlinear dissipation to be important). However the term in large parenthesis is time-invariant which reduces the computational expense of our implementation. A semi-implicit discretization is used to include Eq. 8 in the leap-frog scheme, with
For global tsunami propagation this model is relatively close to the NSWE with Manning-friction (Eq. 2). Figure 5 compares solutions at some nearshore tide-gauges when using the above reduced-physics model on the global grid, vs. the full NSWE (the latter were solved with a leap-frog numerical scheme combined with an upwind treatment of nonlinear advection, for similarity with our reduced-physics solver, Goto and Ogawa, 1997; Liu et al., 1995). At our nearshore gauges of interest, which are inside the refined grids and thus simulated with the NSWE finite-volume scheme (Section 2.4.1), the difference between the two models is smaller than differences caused by grid refinement (compare Figures 4 and 5). However the full simulation ran three times faster when using the simpler model on the global grid (about 2 vs. 6 h for the 60 h simulation with all nested grids, using 8 CPU nodes of the Gadi supercomputer NCI, 2020), due purely to a factor-of-6.6 speedup in the global grid calculation.
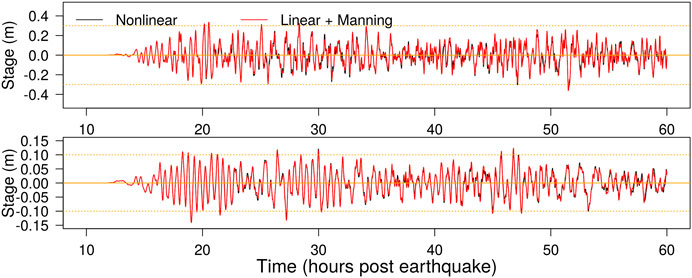
FIGURE 5. Comparison of nearshore gauge results in two models which use different solvers on the global grid; one solves the full NSWE, the other solves the LSWE with a nonlinear Manning-friction term. In both cases the NSWE are solved on refined grids. The site locations and scenario details are the same as in Figure 4.
Linear Shallow Water Equations With Constant Linear-Friction, and Approximation via Frictionless Solutions
To represent global-scale tsunami dissipation with a linear model, Fine et al. (2013) and Kulikov et al. (2014) appended constant linear-friction to the LSWE (Eq. 6) by replacing
where f is a constant linear drag coefficient. Fine et al. (2013) and Kulikov et al. (2014) show Eqs 6 and 9 cause the globally integrated energy to decay exponentially in time with e-folding timescale of
Linear-friction is attractive, if it works well enough in practice, because the model retains all the practical benefits of linearity for simulating global propagation (e.g., unit-source solutions are exact). The use of a constant f is not essential to preserve linearity; often linear friction is implemented by linearizing a quadratic drag model about a reference depth and velocity (e.g., Tolkova, 2015). However the use of a constant f has a practical advantage, above those noted in Fine et al. (2013) and Kulikov et al. (2014): it is possible to approximate solutions of the model with negligible error by using transformed solutions of the frictionless LSWE (Eqs 6 and 7). To illustrate this point, Figure 6 compares a numerically derived solution to the constant linear-friction model Eqs 6 and 9 with an “approximate linear-friction” solution defined as:
Here the superscript a denotes the approximate linear-friction solution, and the superscript 0 denotes the frictionless LSWE solution which is prescribed the same initial conditions as the solution of interest.
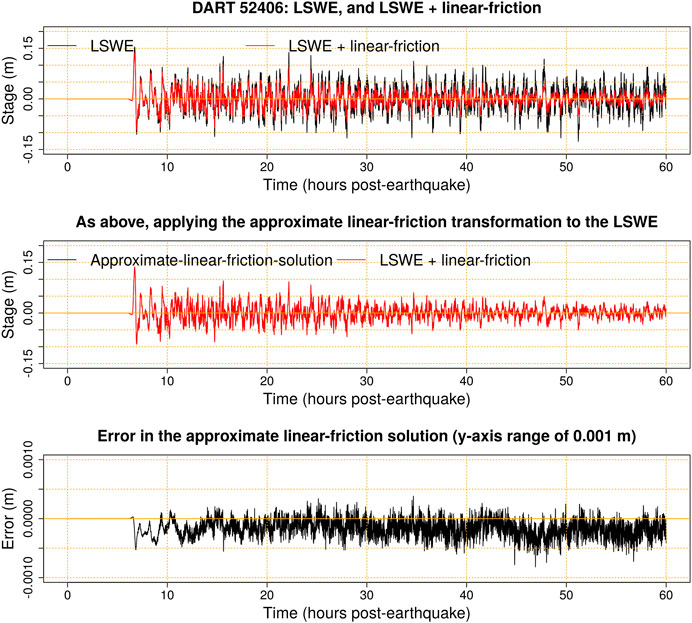
FIGURE 6. Illustration of the approximate linear-friction solution at a deep ocean site (DART 52406), using the Y18 source. Top: Comparison of numerical solutions using the frictionless LSWE (Section 2.4.2) and the LSWE with constant linear-friction (Section 2.4.4). Middle panel: Comparison of the approximate linear-friction solution (derived with the LSWE solution via Eq. 10) and the LSWE with constant linear-friction. They are visually indistinguishable. Bottom: Difference between the two solutions in the middle panel, using a vertical scale of
Although Eq. 10 does not produce an exact solution of the constant linear-friction model, the error is negligible in practice (Figure 6) for global-scale tsunamis and other waves having a period much smaller than the decay timescale
Justification of the Approximate Linear-Friction Solution (Eq. 10)
It is standard to convert the frictionless LSWE to a single wave equation for the free surface by applying
where
For the approximate linear-friction solution (Eq. 10), the analogous equation follows by substituting
Equation 13 is the same as Eq. 12 except for the second RHS source term, which has magnitude
Results
Section 3.1 provides examples to illustrate how the global dissipation models affect nearshore tsunami simulations in comparison with data. This gives context for a subsequent statistical analysis but necessarily represents a small sample of the results; figures comparing models and data at all sites are available via the repository (see Data Availability Statement). Section 3.2 uses statistical techniques to evaluate each model by comparing all simulations and observations simultaneously, focusing on biases in the modeled tsunami maxima and their relation to the simulation duration. These results motivate tests of several modified linear-friction models on the global grid (Section 3.3). Section 3.4 considers how the model performance varies among the source-inversions.
Effect of Global Dissipation at Nearshore Gauges: Examples
Fort Denison, Chile 1960, H19 Source Model
The 1960 Chile tsunami was observed widely on Australia’s east-coast where it induced widespread marine hazards and minor inundation (Beccari, 2009). Based on our simulations, the tsunami reached the eastern Australian mainland
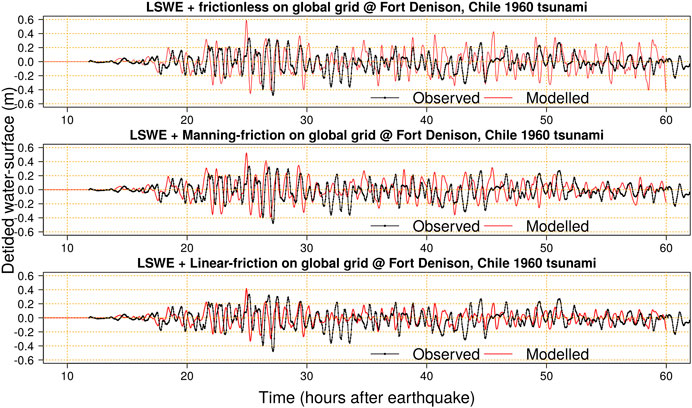
FIGURE 7. Simulations and observations of the Chile 1960 tsunami at Fort Denison near Sydney. All simulations use the Chile 1960 H19 source model. Top: Using the frictionless LSWE on the global grid. Middle: Using LSWE with Manning-friction on the global grid. Bottom: Using LSWE with linear-friction on the global grid.
At Fort Denison the models with frictionless and Manning-friction global grid solvers give similar results prior to the observed maxima, with predicted waves slightly larger than observed (Figure 7). Linear-friction on the global grid produces slightly smaller initial waves. Constant linear-friction induces greater dissipation in the deep-ocean compared to Manning-friction which dissipates most energy in nearshore areas; at later times the cumulative effect of nearshore propagation leads to significant global energy dissipation with Manning-friction, but this has little influence on the early arrivals. All models well approximate the timing of the maximum wave but differ in its size (Figure 7). In this case linear-friction best represents the observed tsunami maxima, but at later times consistently under-predicts the tsunami size. Manning-friction better simulates the size of late arriving waves, while the frictionless global model leads to nearshore waves that are too large at late times.
The data has a phase-lag relative to all models (Figure 7). This is expected given our use of the LSWE on the global grid. The phase-lag should be better simulated by including additional terms in the global model related to loading, seawater density stratification, and self-gravitation (Watada et al., 2014; An and Liu, 2016), although this would be computationally expensive (Allgeyer and Cummins, 2014; Baba et al., 2017).
Hillarys, Sumatra 2004, F07 Source Model
The Sumatra 2004 tsunami induced significant marine hazards in Western Australia, including 35 ocean rescues, damages to boats and marinas, and minor inundation at a number of coastal towns (Anderson, 2015). It took about 6 h to reach Hillarys in Western Australia (Figure 8), which is the shortest travel time among the tsunamis considered herein. The fourth wave was the largest at Hillarys and occurred a few hours after the tsunami arrival, although waves around 21 h post-earthquake were only slightly smaller (Figure 8). Pattiaratchi and Wijeratne (2009) noted these later waves were the largest observed at several other sites in Western Australian, and attributed them to tsunami energy reaching Australia via reflections off distant Indian Ocean topography.
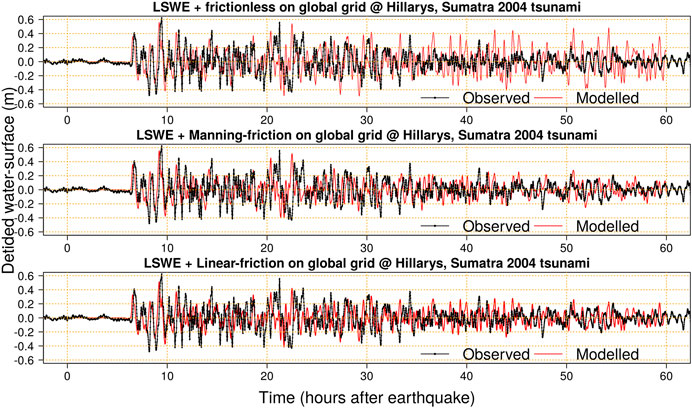
FIGURE 8. Simulations and observations of the Sumatra 2004 tsunami at Hillarys in Western Australia. All simulations use the Sumatra 2004 F07 source model. Top: Using the frictionless LSWE on the global grid. Middle: Using LSWE with Manning-friction on the global grid. Bottom: Using LSWE with linear-friction on the global grid.
At Hillarys the simulated leading waves are not strongly affected by the choice of global dissipation model (Figure 8). About 30 h post-earthquake the frictionless global model results in larger nearshore waves than simulations with friction, although initially it is not obvious that any model better agrees with data. However from about 36 h post-earthquake the frictionless global model consistently predicts larger nearshore waves than were observed, as seen in the previous Chile 1960 example, indicating a lack of global dissipation. At late times the models with global friction predict smaller waves and agree much better with data in the nearshore (Figure 8).
The data evidences some phase-lag relative to the model, as noted in the previous Chile 1960 example. For instance the modeled long-period wave around 20–23 h arrives slightly earlier than the observed wave (Figure 8), and a similar result is obtained with the P07 and L10 source models (see online repository). These phase-lags may be better modeled by accounting for loading, seawater density stratification, and self-gravitation (Baba et al., 2017). The neglect of wave dispersion is also likely to be significant for the Sumatra 2004 example, which shows much more short-period wave energy than the Chile 1960 example (e.g., compare Figures 7 and 8). Non-dispersive shallow-water wave theory will over-estimate the celerity of shorter period waves, as previously noted in the context of the 2004 Sumatra tsunami (Kulikov, 2006).
Twofold Bay, Tohoku 2011, S13 Source Model
The 2011 Tohoku tsunami was widely observed in eastern Australia (Hinwood and Mclean, 2013). The initial waves reached Australia’s east-coast via straits around eastern Papua New Guinea, the Solomon Islands and Vanuatu, while at later times much of the wave energy arrived via the ocean north of New Zealand (Hinwood and Mclean, 2013). Our models suggest the leading wave reached South America around 20–22 h post-earthquake where it was prominently reflected into the Pacific Ocean, adding significantly to the late-time wave energy in the southwest Pacific Ocean. In eastern Australia the tide-gauges at Fort Denison and Twofold Bay exhibited late-time maxima (46–50 h post-earthquake, Figure 9) while gauges at Botany Bay and Port Kembla exhibited earlier maxima (18–20 h post-earthquake) but nonetheless showed relatively large late-time waves that we attribute to reflections from the eastern Pacific. This is similar to reports from New Zealand where Borrero et al. (2015a) found some sites (but not all) experienced late-time tsunami maxima up to 2 days post-earthquake.
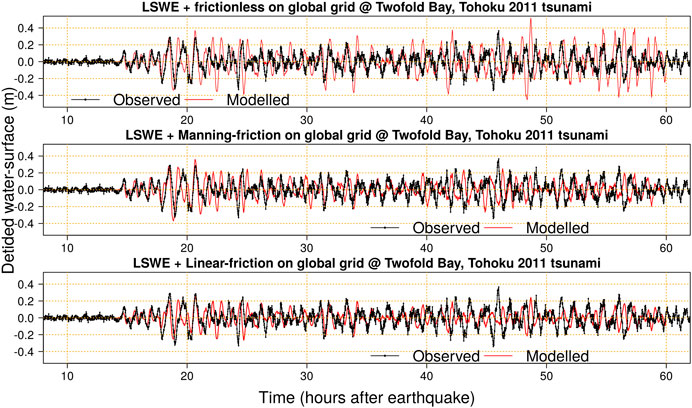
FIGURE 9. Simulations and observations of the Tohoku 2011 tsunami at Twofold Bay. Note the observed late-time maxima 46 h post-earthquake. All simulations use the Tohoku 2011 S13 source model. Top: Using the frictionless LSWE on the global grid. Middle: Using LSWE with Manning-friction on the global grid. Bottom: Using LSWE with linear-friction on the global grid.
At Twofold Bay the first few waves (up to 20 h post-earthquake) are similar in the models with frictionless and Manning-friction global grid solvers, and agree quite well with data (Figure 9). Linear-friction produces smaller initial waves than the Manning model, as noted for above for the Chile 1960 tsunami, which is attributed to its greater dissipation in the deep ocean. At late times the frictionless global model produces overly large waves in the nearshore compared to data (as in the previous examples). In this case the Manning and linear-friction models predict late-time waves smaller than were observed. These results again highlight the sensitivity of later waves to the global dissipation model.
Effect of Global Dissipation at Nearshore Gauges: Tsunami Maxima Statistics
To compare the models and observations at all gauges simultaneously the tsunami maxima (i.e. detided water-level maxima) is used following Allen and Greenslade (2016) and Adams and LeVeque (2017). The results above suggest that model biases may be related to the simulation duration, and to assess this both simulations and data are truncated to various time-intervals (Figure 10):
(1) 0–8 h after the tsunami arrival. The arrival time is defined, for each model and gauge separately, as the time that the modeled stage absolute value exceeds
(2) 0–36 h post-earthquake.
(3) 0–60 h post-earthquake.
(4) The last day of the 60 h simulation (i.e., 36–60 h post-earthquake).
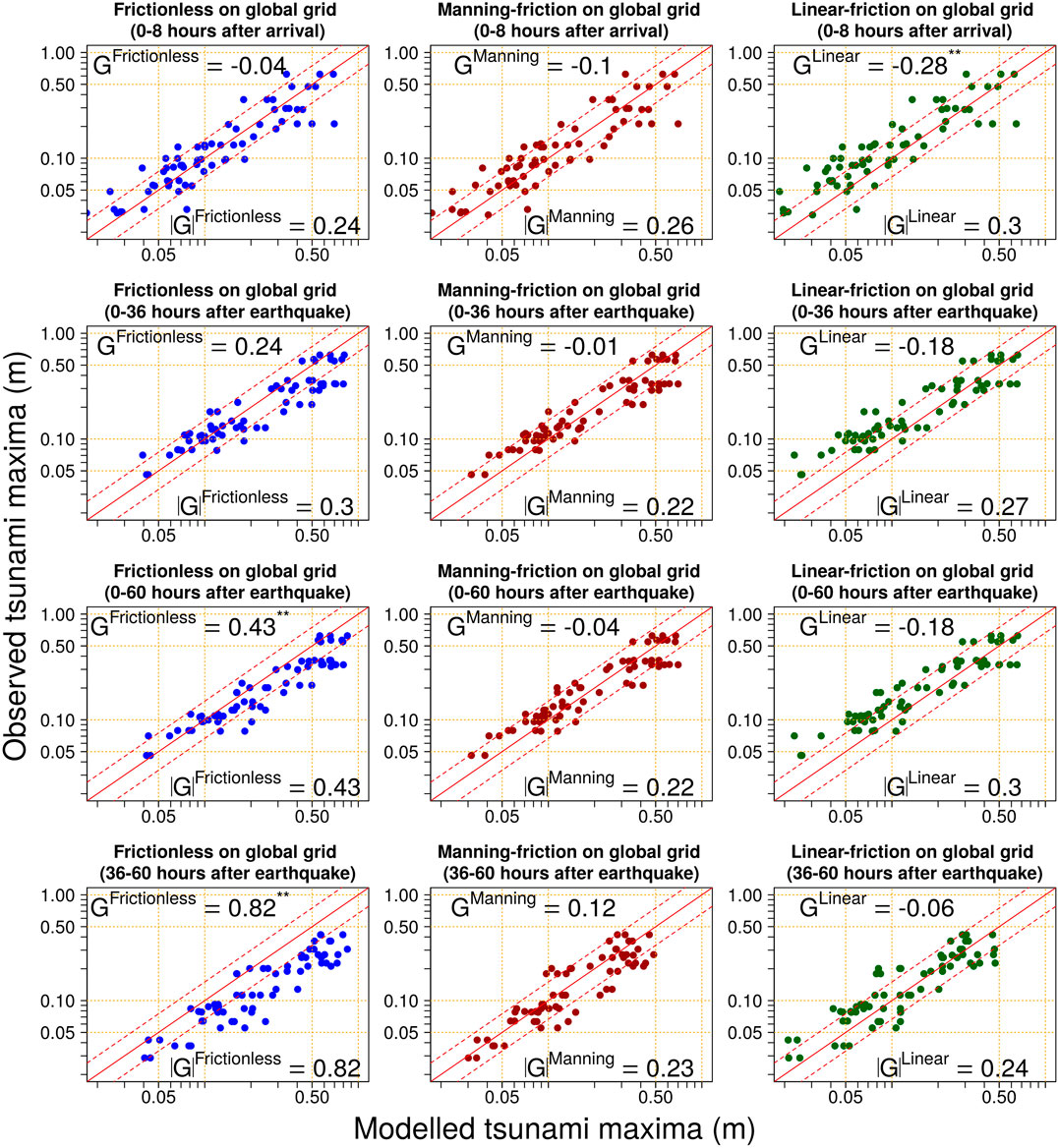
FIGURE 10. Modelled-vs-observed tsunami maxima for different global model types (frictionless, Manning-friction, linear-friction), and different temporal subsets of the simulation (0–8 h after tsunami arrival; 0–36 h after earthquake; 0–60 h after earthquake, 36–60 h after earthquake). Diagonal solid line is
All but one observed time-series extends for the full 60 h simulation; the 1960 Cronulla tide-gauge record is truncated at 29 h post-earthquake and dropped from comparison with longer simulations.
Statistics describing the model bias (
The bias statistic
Here
The superscript“**” is appended to
The accuracy statistic
Thus
Figure 10 highlights the interaction of the simulation duration and the model bias, most prominently for the frictionless global model. If simulations are restricted to 0–8 h following tsunami arrival, this model exhibits small bias and a typical accuracy around 24% (top-left panel of Figure 10). For a 36 h simulation there is increasing positive bias (
Manning-friction in the global model leads to much better agreement with observed tsunami-maxima for long simulations (Figure 10). For short simulations (0–8 h post-arrival) it performs similarly to the frictionless model, suggesting friction has limited influence on early arriving waves. But over long simulations the cumulative effect of nearshore dissipation at the global scale reduces the nearshore wave heights, in a manner broadly consistent with observations (Figure 10).
Linear-friction in the global model induces a moderate negative bias in the early (0–8 h post-arrival) nearshore tsunami maxima (
To gauge the model accuracy it is useful to cross-reference the
Alternative Linear-Friction Models With Reduced Bias for Earlier Waves
Because linear models have significant practical benefits (e.g., unit sources), herein two variations of the linear-friction model are tested. Both aim to reduce downward bias in nearshore waves 0–8 h after arrival, as compared with the constant linear-friction model, while retaining the benefits of friction for longer simulations. In addition, both models retain the convenient property that their solutions can be approximated from solutions of the frictionless model:
(1) Reduced-linear-friction. This model uses
(2) Delayed-linear-friction. This model is frictionless for the first 12 h, and subsequently uses
where
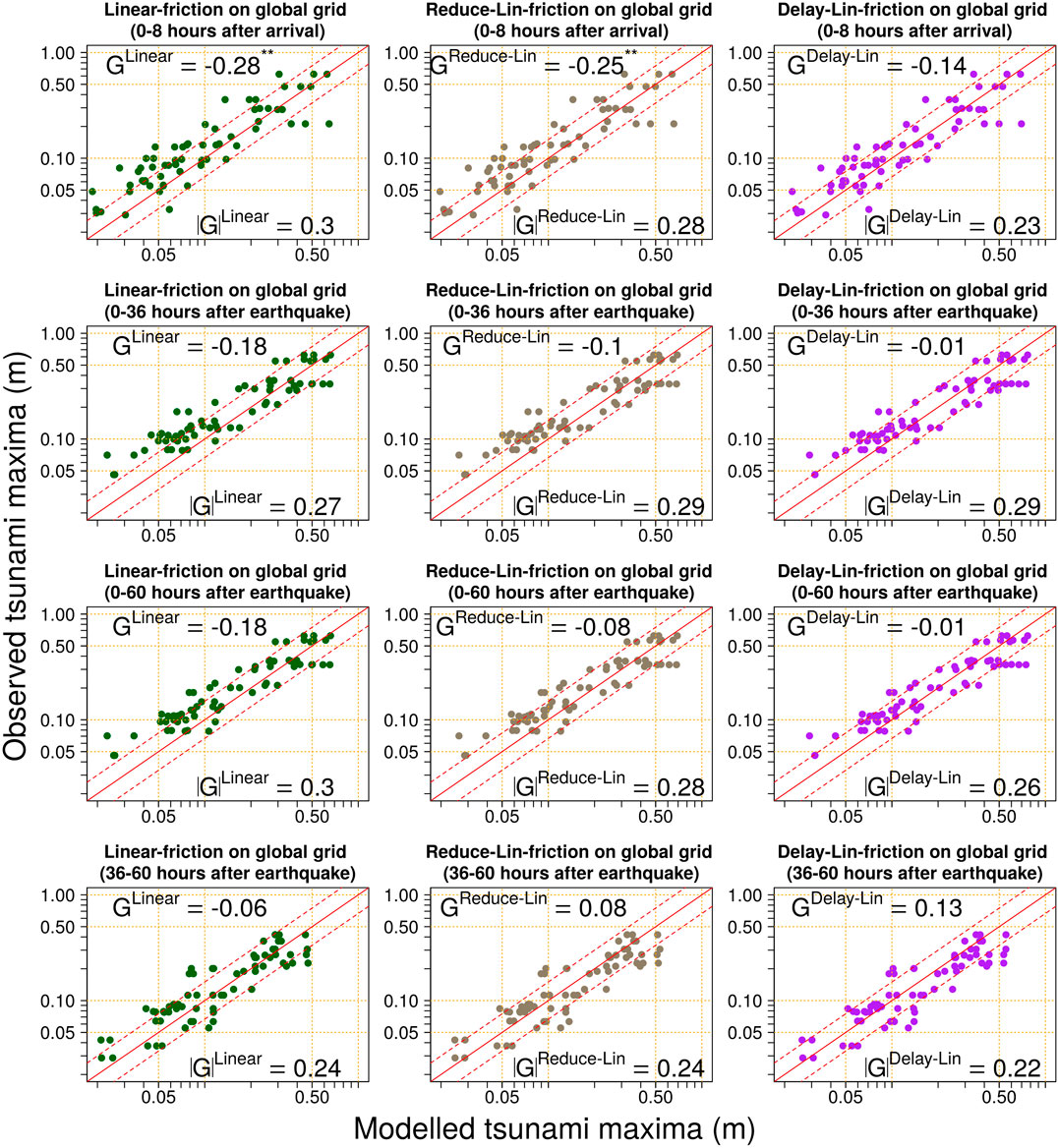
FIGURE 11. Modelled-vs-observed tsunami maxima for different global model types (linear-friction, linear-friction with a lower friction factor, linear-friction with delayed onset of 12 h), and different temporal subsets of the simulation (0–8 h after tsunami arrival; 0–36 h after earthquake; 0–60 h after earthquake, 36–60 h after earthquake). Diagonal solid line is
The reduced-linear-friction model continues to show downward bias for waves 0–8 h after arrival, even though its friction coefficient is a-priori too small (Figure 11). If constant linear-friction were a good approximation of dissipation for early-arriving waves, then the a-priori small friction coefficient should lead to overestimation of tsunami maxima, but this does not occur. That indicates weaknesses in the constant linear-friction parameterization of tsunami dissipation; friction is still too large in the deep ocean. However the model performs reasonably well for longer simulations (Figure 11).
In contrast, the delayed-linear-friction model performs better in the 0–8 h post-arrival period than any other linear model with friction (Figure 11). Furthermore, over longer simulations it continues to exhibit relatively low-bias in the tsunami maxima, with results comparable to the Manning-friction model (Figure 10). Among the linear models tested herein, this appears the most promising overall for simulating global-scale tsunami propagation.
Source-Inversion Effects on Modeled Tsunami Maxima
The previous section highlights that error in the modeled tsunami maxima varies from gauge-to-gauge and also depends on the simulation duration. However there is also a significant component related to the source-inversion itself (Figure 12). For simplicity Figure 12 only depicts results using the Manning-friction and delayed-linear-friction global models, which exhibited the least overall bias above, although comparable variations between source-inversions exist for the other models.
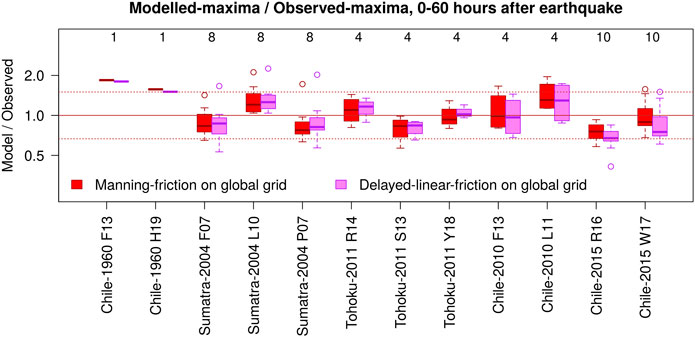
FIGURE 12. Per-inversions boxplots of ratio between modeled and observed tsunami maxima at all gauges, for simulations of 60 h, and two model types. The integers above the boxes count the tide-gauge observations. Dotted horizontal lines are
For a given historical tsunami, inversions with a larger model/observed ratio in Figure 12 tend to have greater available potential energy in their ocean-displacement (reported in Figure 2). For example consider the Chile 2010 tsunami; the L11 inversion produces higher model/obs ratios than the F13 inversion, while the former also exhibits greater available potential energy (Figures 2, 12). For the Chile 2015 tsunami the W17 inversion exhibits greater model/obs than the R16 inversion, and has greater initial available potential energy (Figures 2, 12). Other cases behave similarly with one exception; for Sumatra 2004 the P07 inversion has slightly smaller model/observed than the F07 inversion (Figure 12), despite having a slightly larger available potential energy (Figure 2). This may reflect that compared to F07, the P07 inversion has greater co-seismic displacement toward the north of the rupture area (Figure 2), which may have less effect on Australia than deformation in the southeast due to tsunami dynamics in the Bay of Bengal. In any case this result is consistent with the suggestion of Titov et al. (2016) that far-field tsunamis are sensitive to the location and energy of the initial ocean-surface displacement, with other source details having a secondary role.
Discussion
Linear models provide a very convenient basis for global-scale tsunami scenario databases, and these are often implemented without friction (Burbidge et al., 2008; Li et al., 2016; Davies and Griffin, 2020). Our results suggest this approach is adequate for simulating tsunami maxima up to 8 h following tsunami arrival, when combined with nearshore models based on the NSWE with Manning-friction. Herein 8 h post-arrival corresponds to a median of 20 h post-earthquake; for hazard applications this duration would typically include the most significant waves for the most hazardous scenarios, where sites of interest are well exposed to the tsunami’s leading wave. However, tsunamis may remain dangerous for a considerably longer duration. Over longer simulations the lack of global model dissipation leads to slow divergence of the frictionless model wave heights, as compared to both data and models with friction. It is difficult to estimate a “threshold” duration after which the frictionless model biases become important. In comparison to observed tsunami maxima, our results for 36 h simulations suggest bias
The late-time model biases are largely removed using nonlinear Manning-friction in the global propagation model with
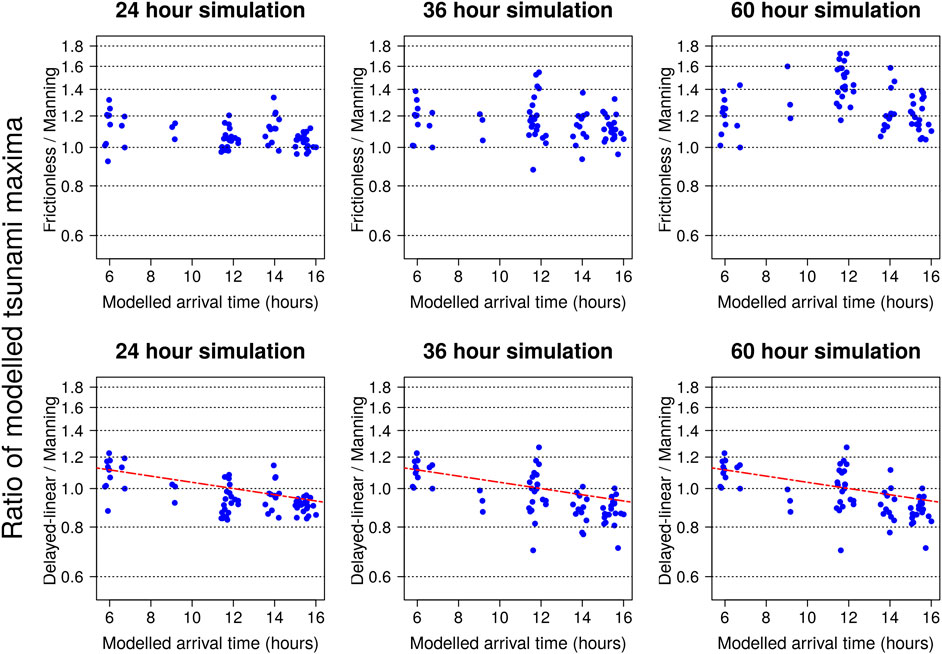
FIGURE 13. Ratios of modeled tsunami maxima, at nearshore sites with tide-gauge data, using different offshore friction treatments: Top row frictionless/Manning; Bottom row Delayed-linear-friction/Manning-friction. In the bottom row the dashed line is
In some instances unit-source based treatments of global tsunami propagation are essential; for example if many tsunami scenarios must be simulated for a small nearshore area, global propagation modeling may be computationally prohibitive. In such circumstances the delayed-linear friction model is worth considering, particularly for long simulation durations where biases in the frictionless model are anticipated. While not motivated from hydrodynamic theory, this model addresses the early-time bias of constant linear-friction, which was found to underestimate observed tsunami maxima 0–8 h post-arrival. A key result of this study is that the model can be implemented, to high-accuracy, via a trivial transformation of frictionless solutions (Eq. 16). Thus the model is very easy to implement with existing unit-source databases based on the frictionless LSWE (e.g., Thio, 2015; Davies and Griffin, 2020).
Herein delayed-linear-friction was applied with a 12 h frictionless period
One may estimate the relative change r in the tsunami maxima that would result from this approach, vs. the use of
where
Finally we emphasize limitations of this study and directions for further work. All results are based on global-scale propagation models with little numerical dissipation. Clearly these techniques should not be applied to models where numerical dissipation is already comparable to or greater than the required physical dissipation, as this could exaggerate any under-estimation of late arriving waves. Our models neglect various physical processes which can affect tsunami propagation (dispersion, loading, self-gravitation, density stratification), and we make no attempt to resolve coastal inundation outside our areas of interest in Australia although this may affect remote wave reflections; future work should consider the influence of these approximations on modeled waves at the coast. Following previous work (Allen and Greenslade, 2016; Adams and LeVeque, 2017) we used the tsunami-maxima statistic to quantify model biases, and while this is useful, there is much more information available in the full time-series that may be extracted using other statistics and provide new insights into the model performance. The tests herein consider only five historic tsunamis using sources derived from 12 finite-fault inversions, with a total of 28 tide-gauge records. The testing should be extended to consider more historic events and sites, and other types of data (e.g., inundation footprints and depths; tsunami currents). Of particular interest is the global-record of historical tsunami energy-decay timescales (e.g., van Dorn, 1987; Rabinovich et al., 2011, 2013); further insights into dissipation models would likely be obtained by simulating these observations with alternative models. The tests should also be extended to consider tsunami scenarios derived for hazard assessment (Davies, 2019), including characterization of the nearshore performance of random hazard scenarios.
Conclusion
When modeling far-field tsunamis in the nearshore it is often convenient to nest high-resolution nonlinear shallow water models (which cover coastal regions of interest) within reduced-physics global-scale models (which efficiently simulate tsunami propagation). In this context we evaluated the performance of several reduced-physics global propagation models which combine the LSWE with alternative treatments of friction. Nearshore tsunamis were simulated with a nested nonlinear model and compared with coastal tide-gauge observations in Australia. Tsunami initial conditions were derived from published source-models. Our results suggest the commonly used frictionless global-scale model is adequate for simulating far-field coastal tsunamis for 0–8 h following arrival. However for sufficiently long simulations the frictionless model overestimates coastal wave heights because it is mathematically energy conservative (which is well approximated with our numerical methods) and thus does not represent global-scale tsunami dissipation. In our simulations this bias was clear from comparison with data 36–60 h post-earthquake; it is difficult to define a precise time at which the bias becomes significant. Better estimates of late-time wave heights can be obtained by appending Manning-friction to the global model, although this renders the model nonlinear. For unit-source based implementations it is preferable work with linear models, and so variants of linear-friction were tested; these are not derived from hydrodynamic theory yet can mimic observed tsunami energy decay rates. Among these, delayed-linear-friction most accurately simulated tsunami maxima at nearshore gauges. Solutions of this model can be conveniently derived by transforming frictionless LSWE solutions with Eq. 16, making it easy to implement via existing unit-source databases that are derived with the frictionless LSWE (e.g., Davies and Griffin, 2020). These results may facilitate improved simulation of late-time tsunami wave heights for hazard and early warning applications in the far-field.
Data Availability Statement
The datasets presented in this study can be found in online repositories. The names of the repository/repositories and accession number(s) can be found below: https://github.com/GeoscienceAustralia/ptha/tree/master/misc/nearshore_testing_2020.
Author Contributions
GD led the study design and implementation and wrote the first draft manuscript, with guidance from FR and SL. FR provided code and data to construct the sources R14 and R16. All authors contributed to the final manuscript.
Acknowledgments
This paper was published with the permission of the CEO, Geoscience Australia, and was undertaken with the assistance of resources and services from the National Computational Infrastructure (NCI), which is supported by the Australian Government. Discussions with Jon Hinwood and reviews by Kaya Wilson, Phil Cummins, Alexander Rabinovich and Efim Pelinovsky improved the work. Tide-gauge data was provided by the NSW Department of Planning, Industry and Environment, Manly Hydraulics Laboratory, the NSW Port Authority, the WA Department of Transport, and the Australian Bureau of Meteorology. The elevation data was based on a range of elevation products available from ELVIS http://elevation.fsdf.org.au/, The Australian Oceans Data Network https://portal.aodn.org.au/, Ausseabed http://www.ausseabed.gov.au/data, and the WA Department of Transport https://catalogue.data.wa.gov.au/dataset/composite-surfaces-multibeam-lidar-laser.
Conflict of Interest
The authors declare that the research was conducted in the absence of any commercial or financial relationships that could be construed as a potential conflict of interest.
References
Adams, L. M., and LeVeque, R. J. (2017). Technical Report. GeoClaw model tsunamis Compared to tide gauge results, final report. University of Washington (Accessed November 3, 2017).
Allen, S. C. R., and Greenslade, D. J. M. (2016). A pilot tsunami inundation forecast system for Australia. Pure Appl. Geophys. 173, 3955–3971. doi:10.1007/s00024-016-1392-y
Allgeyer, S., and Cummins, P. (2014). Numerical tsunami simulation including elastic loading and seawater density stratification. Geophys. Res. Lett. 41, 2368–2375. doi:10.1002/2014GL059348
An, C., and Liu, P. L.-F. (2016). Analytical solutions for estimating tsunami propagation speeds. Coastal Engg. 117, 44–56. doi:10.1016/j.coastaleng.2016.07.006
Arakawa, A., and Hsu, Y. G. (1990). Energy conserving and potential-enstrophy dissipating schemes for the shallow water equations. Mon. Weather Rev. 118, 1960–1969. doi:10.1175/1520-0493(1990)118<1960:ECAPED>2.0.CO;2
Audusse, E., Bouchut, F., Bristeau, M.-O., Klein, R., and Perthame, B. (2004). A fast and stable well-balanced scheme with hydrostatic reconstruction for shallow water flows. SIAM J. Sci. Comput. 25, 2050–2065. doi:10.1137/s1064827503431090
Baba, T., Allgeyer, S., Hossen, J., Cummins, P. R., Tsushima, H., Imai, K., et al. (2017). Accurate numerical simulation of the far-field tsunami caused by the 2011 Tohoku earthquake, including the effects of Boussinesq dispersion, seawater density stratification, elastic loading, and gravitational potential change. Ocean Model. 111, 46–54. doi:10.1016/j.ocemod.2017.01.002
Baba, T., Takahashi, N., Kaneda, Y., Ando, K., Matsuoka, D., and Kato, T. (2015). Parallel implementation of dispersive tsunami wave modeling with a nesting algorithm for the 2011 tohoku tsunami. Pure Appl. Geophys. 172, 3455–3472. doi:10.1007/s00024-015-1049-2
Baba, T., Takahashi, N., Kaneda, Y., Inazawa, Y., and Kikkojin, M. (2014). “Tsunami inundation modeling of the 2011 tohoku earthquake using three-dimensional building data for Sendai, Miyagi prefecture, Japan,” in Tsunami events and lessons learned. Editors Kontar, Y. A., Takahashi, T., and Santiago-Fandino, V. (Amsterdam: Springer).
Beccari, B. (2009). Technical Report. Measurements and impacts of the Chilean tsunami of may 1960 in new south wales, Australia. NSW State Emergency Service.
Behrens, J., and Dias, F. (2015). New computational methods in tsunami science. Philos. Trans. R. Soc. London A 373, 20140382. doi:10.1098/rsta.2014.0382
Borrero, J. C., Goring, D. G., Greer, S. D., and Power, W. L. (2015a). Far-field tsunami hazard in New Zealand ports. Pure Appl. Geophys. 172, 731–756. doi:10.1007/s00024-014-0987-4
Borrero, J. C., Lynett, P. J., and Kalligeris, N. (2015b). Tsunami currents in ports. Phil. Trans. Math. Phys. Eng. Sci. 373, 20140372. doi:10.1098/rsta.2014.0372
Buijsman, M., Arbic, B., Green, J., Helber, R., Richman, J., Shriver, J., et al. (2015). Optimizing internal wave drag in a forward barotropic model with semidiurnal tides. Ocean Model. 85, 42–55. doi:10.1016/j.ocemod.2014.11.003
Burbidge, D., Cummins, P., Mleczko, R., and Thio, H. (2008). A probabilistic tsunami hazard assessment for western Australia. Pure Appl. Geophys. 165, 2059–2088. doi:10.1007/s00024-008-0421-x
Choi, B. H., Pelinovsky, E., Kim, K. O., and Lee, J. S. (2003). Simulation of the trans-oceanic tsunami propagation due to the 1883 krakatau volcanic eruption. Nat. Hazards Earth Syst. Sci. 3, 321–332. doi:10.5194/nhess-3-321-2003
Davies, G., Griffin, J., Løvholt, F., Glimsdal, S., Harbitz, C., Thio, H. K., et al. (2017). A global probabilistic tsunami hazard assessment from earthquake sources. Geol. Soc. London Spec. Publ. 456, 219–244. doi:10.1144/sp456.5
Davies, G., and Griffin, J. (2018). Technical Report, Geoscience Australia Record 2018/41. The 2018 Australian probabilistic tsunami hazard assessment: hazards from earthquake generated tsunamis. doi:10.11636/Record.2018.041
Davies, G., and Griffin, J. (2020). Sensitivity of probabilistic tsunami hazard assessment to far-field earthquake slip complexity and rigidity depth-dependence: case study of Australia. Pure Appl. Geophys. 177, 1521–1548. doi:10.1007/s00024-019-02299-w
Davies, G., and Roberts, S. (2015). Open source flood simulation with a 2D discontinuous-elevation hydrodynamic model. Proc. of MODSIM 2015, GoldCoast. doi:10.36334/modsim.2015.l5.davies.
Davies, G. (2019). Tsunami variability from uncalibrated stochastic earthquake models: tests against deep ocean observations 2006-2016. Geophys. J. Int. 218, 1939–1960. doi:10.1093/gji/ggz260
Egbert, G. D., and Erofeeva, S. Y. (2002). Efficient inverse modeling of barotropic ocean tides. J. Atmos. Ocean. Technol. 19, 183–204. doi:10.1175/1520-0426(2002)019〈0183:EIMOBO〉2.0.CO;2
Egbert, G. D., and Ray, R. D. (2000). Significant dissipation of tidal energy in the deep ocean inferred from satellite altimeter data. Nature 405, 775–778. doi:10.1038/35015531
Fine, I. V., Kulikov, E. A., and Cherniawsky, J. Y. (2013). Japans 2011 tsunami: characteristics of wave propagation from observations and numerical modelling. Pure Appl. Geophys. 170, 1295–1307. doi:10.1007/s00024-012-0555-8
Fjordholm, U. S., Mishra, S., and Tadmor, E. (2011). Well-balanced and energy stable schemes for the shallow water equations with discontinuous topography. J. Comput. Phys. 230, 5587–5609. doi:10.1016/j.jcp.2011.03.042
Fritz, H. M., and Borrero, J. C. (2006). Somalia field survey after the december 2004 Indian ocean tsunami. Earthq. Spectra. 22, 219–233. doi:10.1193/1.2201972
Fujii, Y., and Satake, K. (2007). Tsunami source of the 2004 sumatra-andaman earthquake inferred from tide gauge and satellite data. Bull. Seismol. Soc. Am. 97, S192. doi:10.1785/0120050613
Fujii, Y., and Satake, K. (2013). Slip distribution and seismic moment of the 2010 and 1960 Chilean earthquakes inferred from tsunami waveforms and coastal geodetic data. Pure Appl. Geophys. 170, 1493–1509. doi:10.1007/s00024-012-0524-2
Geist, E. (2009). Phenomenology of tsunamis: statistical properties from generation to runup. Adv. Geophys. 51, 107–169. doi:10.1016/S0065-2687(09)05108-5
Gica, E., Spillane, M. C., Titov, V. V., Chamberlin, C. D., and Newman, J. C. (2008). Technical Report No. 139. Development of the forecast propagation database for NOAA’s short-term inundation forecast for tsunamis (SIFT). NOAA Technical Memorandum OAR PMEL, Seattle, WA.
Glimsdal, S., Pedersen, G., Harbitz, C., and Løvholt, F. (2013). Dispersion of tsunamis: does it really matter? Nat. Hazards Earth Syst. Sci. 13, 1507–1526. doi:10.5194/nhess-13-1507-2013
Goto, C., and Ogawa, Y. (1997). Technical Report No. 35. Numerical method of tsunami simulation with the leap-frog scheme. IUGG/IOC Time Project.
Greenslade, D. J. M., Allen, S. C. R., and Simanjuntak, M. A. (2011). An evaluation of tsunami forecasts from the T2 scenario database. Pure Appl. Geophys. 168, 1137–1151. doi:10.1007/s00024-010-0229-3
Grezio, A., Babeyko, A., Baptista, M. A., Behrens, J., Costa, A., Davies, G., et al. (2017). Probabilistic tsunami hazard analysis: multiple sources and global applications. Rev. Geophys. 55, 1158–1198. doi:10.1002/2017RG000579. 2017RG000579
Heidarzadeh, M., Satake, K., Takagawa, T., Rabinovich, A., and Kusumoto, S. (2018). A comparative study of far-field tsunami amplitudes and ocean-wide propagation properties: insight from major trans-Pacific tsunamis of 2010-2015. Geophys. J. Int. 215, 22–36. doi:10.1093/gji/ggy265
Hinwood, J. B., and Mclean, E. J. (2013). Effects of the march 2011 Japanese tsunami in bays and estuaries of SE Australia. Pure Appl. Geophys. 170, 1207–1227. doi:10.1007/s00024-012-0561-x
Ho, T.-C., Satake, K., Watada, S., and Fujii, Y. (2019). Source estimate for the 1960 Chile earthquake from joint inversion of geodetic and transoceanic tsunami data. J. Geophys. Res. Solid Earth. 124, 2812–2828. doi:10.1029/2018JB016996
Kânoğlu, U., Titov, V., Bernard, E., and Synolakis, C. (2015). Tsunamis: bridging science, engineering and society. Philos. Trans. R. Soc. London. 373, 20140369. doi:10.1098/rsta.2014.0369
Kleermaeker, S. D., Apecechea, M. I., Verlaan, M., Mortlock, T., and Rego, J. L. (2019). “Global-to-local scale storm surge modelling: operational forecasting and model sensitivities,” in Australasian coasts & ports 2019 conference, Hobart, September 10–13, 2019.
Kowalik, Z., Horrillo, J., Knight, W., and Logan, T. (2008). Kuril Islands tsunami of november 2006: 1. Impact at crescent city by distant scattering. J. Geophys. Res. 113, C01020. doi:10.1029/2007JC004402
Kulikov, E. A., Fine, I. V., and Yakovenko, O. I. (2014). Numerical modeling of the long surface waves scattering for the 2011 Japan tsunami: case study. Izvestiya Atmos. Ocean. Phys. 50, 498–507. doi:10.1134/S0001433814050053
Kulikov, E. (2006). Dispersion of the Sumatra Tsunami waves in the Indian Ocean detected by satellite altimetry. Russ. J. Earth Sci. 8, 1–5. doi:10.2205/2006es000214
Li, L., Switzer, A. D., Chan, C.-H., Wang, Y., Weiss, R., and Qiu, Q. (2016). How heterogeneous coseismic slip affects regional probabilistic tsunami hazard assessment: a case study in the South China Sea. J. Geophys. Res. Solid Earth. 121, 6250–6272. doi:10.1002/2016JB013111. 2016JB013111
Liu, P. L. F., Cho, Y.-S., Briggs, M. J., Kanoglu, U., and Synolakis, C. E. (1995). Runup of solitary waves on a circular island. J. Fluid Mech. 302, 259–285. doi:10.1017/S0022112095004095
Liu, Y., Shi, Y., Yuen, D. A., Sevre, E. O. D., Yuan, X., and Xing, H. L. (2008). Comparison of linear and nonlinear shallow wave water equations applied to tsunami waves over the China sea. Acta Geotechnica. 4, 129–137. doi:10.1007/s11440-008-0073-0
Llewellyn Smith, S. G., and Young, W. R. (2002). Conversion of the barotropic tide. J. Phys. Oceanogr. 32, 1554–1566. doi:10.1175/1520-0485(2002)032〈1554:COTBT〉2.0.CO;2
Lorenz, E. N. (1955). Available potential energy and the maintenance of the general circulation. Tellus 7, 157–167. doi:10.1111/j.2153-3490.1955.tb01148.x
Lorito, S., Piatanesi, A., Cannelli, V., Romano, F., and Melini, D. (2010). Kinematics and source zone properties of the 2004 Sumatra-Andaman earthquake and tsunami: nonlinear joint inversion of tide gauge, satellite altimetry, and GPS data. J. Geophys. Res. 115, B02304. doi:10.1029/2008jb005974
Lorito, S., Romano, F., Atzori, S., Tong, X., Avallone, A., McCloskey, J., et al. (2011). Limited overlap between the seismic gap and coseismic slip of the great 2010 Chile earthquake. Nat. Geosci. 4, 173–177. doi:10.1038/ngeo1073
Lorito, S., Selva, J., Basili, R., Romano, F., Tiberti, M., and Piatanesi, A. (2015). Probabilistic hazard for seismically induced tsunamis: accuracy and feasibility of inundation maps. Geophys. J. Int. 200, 574–588. doi:10.1093/gji/ggu408
Macías, J., Castro, M. J., Ortega, S., and González-Vida, J. M. (2020). Performance assessment of Tsunami-HySEA model for NTHMP tsunami currents benchmarking. Field cases. Ocean Model. 152, 101645. doi:10.1016/j.ocemod.2020.101645
Meade, B. J. (2007). Algorithms for the calculation of exact displacements, strains, and stresses for triangular dislocation elements in a uniform elastic half space. Comput. Geosci. 33, 1064–1075. doi:10.1016/j.cageo.2006.12.003
Miller, G. R., Munk, W. H., and Snodgrass, F. E. (1962). Long-period waves over California’s continental borderland part ii. tsunamis. J. Mar. Res. 20, 31–41.
Miranda, J. M., Baptista, M. A., and Omira, R. (2014). On the use of Green’s summation for tsunami waveform estimation: a case study. Geophys. J. Int. 199, 459–464. doi:10.1093/gji/ggu266
Mofjeld, H. O., González, F. I., Bernard, E. N., and Newman, J. C. (2000). Forecasting the heights of later waves in pacific-wide tsunamis. Nat. Hazards. 22, 71–89. doi:10.1023/A:1008198901542
Molinari, I., Tonini, R., Lorito, S., Piatanesi, A., Romano, F., Melini, D., et al. (2016). Fast evaluation of tsunami scenarios: uncertainty assessment for a Mediterranean Sea database. Nat. Hazards Earth Syst. Sci. 16, 2593–2602. doi:10.5194/nhess-16-2593-2016
Munk, W. (1963). “Some comments regarding diffusion and absorption of tsunamis,” in Proceedings Tsunami Meetings, Tenth Pacific Science Congress, Honolulu (IUGG Monograph), Vol. 24, 53–72.
Munk, W. (1997). Once again: once again―tidal friction. Prog. Oceanogr. 40, 7–35. doi:10.1016/S0079-6611(97)00021-9
NCI (2020). [Dataset] National computational infrastructure hpc systems. Available at:https://nci.org.au/our-systems/hpc-systems.
NTHMP(2012). Technical Report. Proceedings and results of the 2011 NTHMP model benchmarking workshop. National Tsunami Hazard Mitigation Program, NOAA Special Report.
Nyland, D., and Huang, P. (2013). Forecasting wave amplitudes after the arrival of a tsunami. Pure Appl. Geophys. 171, 3501–3513. doi:10.1007/s00024-013-0703-9
Oh, I., and Rabinovich, A. (1994). Manifestation of hokkaido southwest (okushiri) tsunami 12 July, 1993, at the coast of korea. Sci. Tsunami Hazards. 12, 93–116.
Okada, Y. (1985). Surface deformation due to shear and tensile faults in a half-space. Bull. Seismol. Soc. Am. 75, 1135–1154.
Okal, E. A. (2011). Tsunamigenic earthquakes: past and present milestones. Pure Appl. Geophys. 168, 969–995. doi:10.1007/s00024-010-0215-9
Pattiaratchi, C., and Wijeratne, E. M. S. (2009). Tide gauge observations of 2004-2007 Indian Ocean tsunamis from Sri Lanka and Western Australia. Pure Appl. Geophys. 166, 233–258. doi:10.1007/s00024-008-0434-5
Percival, D. M., Percival, D. B., Denbo, D. W., Gica, E., Huang, P. Y., Mofjeld, H. O., et al. (2014). Automated tsunami source modeling using the sweeping window positive elastic net. J. Am. Stat. Assoc. 109, 491–499. doi:10.1080/01621459.2013.879062
Piatanesi, A., and Lorito, S. (2007). Rupture process of the 2004 sumatra–andaman earthquake from tsunami waveform inversion. Bull. Seismol. Soc. Am. 97, S223–S231. doi:10.1785/0120050627
Popinet, S. (2011). Quadtree-adaptive tsunami modelling. Ocean Dynam. 61, 1261–1285. doi:10.1007/s10236-011-0438-z
Rabinovich, A. B., Candella, R. N., and Thomson, R. E. (2011). Energy decay of the 2004 Sumatra tsunami in the world ocean. Pure Appl. Geophys. 168, 1919–1950. doi:10.1007/s00024-011-0279-1
Rabinovich, A. B., Candella, R. N., and Thomson, R. E. (2013). The open ocean energy decay of three recent trans-pacific tsunamis. Geophys. Res. Lett. 40, 3157–3162. doi:10.1002/grl.50625
Romano, F., Piatanesi, A., Lorito, S., Tolomei, C., Atzori, S., and Murphy, S. (2016). Optimal time alignment of tide-gauge tsunami waveforms in nonlinear inversions: application to the 2015 Illapel (Chile) earthquake. Geophys. Res. Lett. 43, 11226–11235. doi:10.1002/2016GL071310. 2016GL071310
Romano, F., Trasatti, E., Lorito, S., Piromallo, C., Piatanesi, A., Ito, Y., et al. (2014). Structural control on the Tohoku earthquake rupture process investigated by 3D FEM, tsunami and geodetic data. Sci. Rep. 4, 5631. doi:10.1038/srep05631
Saito, T., Inazu, D., Miyoshi, T., and Hino, R. (2014). Dispersion and nonlinear effects in the 2011 Tohoku-oki earthquake tsunami. J. Geophys. Res. Oceans. 119, 5160–5180. doi:10.1002/2014jc009971
Satake, K., Fujii, Y., Harada, T., and Namegaya, Y. (2013). Time and space distribution of coseismic slip of the 2011 tohoku earthquake as inferred from tsunami waveform data. Bull. Seismol. Soc. Am. 103, 1473–1492. doi:10.1785/0120120122
Satake, K., Okada, M., and Abe, K. (1988). Tide gauge response to tsunamis: measurements at 40 tide gauge stations in Japan. J. Mar. Res. 46, 557–571. doi:10.1357/002224088785113504
Setiyono, U., Gusman, A. R., Satake, K., and Fujii, Y. (2017). Pre-computed tsunami inundation database and forecast simulation in Pelabuhan Ratu, Indonesia. Pure Appl. Geophys. 174, 3219–3235. doi:10.1007/s00024-017-1633-8
Shuto, N. (1991). Numerical simulation of tsunamis—its present and near future. Nat. Hazards. 4, 171–191. doi:10.1007/BF00162786
Tang, L., Titov, V. V., Bernard, E. N., Wei, Y., Chamberlin, C. D., Newman, J. C., et al. (2012). Direct energy estimation of the 2011 Japan tsunami using deep-ocean pressure measurements. J. Geophys. Res.: Oceans. 117, C08008. doi:10.1029/2011JC007635
Tang, L., Titov, V. V., and Chamberlin, C. D. (2009). Development, testing, and applications of site-specific tsunami inundation models for real-time forecasting. J. Geophys. Res. 114, C12025. doi:10.1029/2009JC005476
Tanioka, Y., and Satake, K. (1996). Tsunami generation by horizontal displacement of ocean bottom. Geophys. Res. Lett. 23, 861–864. doi:10.1029/96GL00736
Thio, H. (2015). “Tsunami hazards of the pacific rim,” in Proceedings of the 10th pacific conference on earthquake engineering, Sydney, November 6–8, 2015
Titov, V., Song, Y. T., Tang, L., Bernard, E. N., Bar-Sever, Y., and Wei, Y. (2016). Consistent estimates of tsunami energy show promise for improved early warning. Pure Appl. Geophys. 173, 3863–3880. doi:10.1007/s00024-016-1312-1
Tolkova, E. (2014). Land-water boundary treatment for a tsunami model with dimensional splitting. Pure Appl. Geophys. 171, 2289–2314. doi:10.1007/s00024-014-0825-8
Tolkova, E. (2015). Tsunami penetration in tidal rivers, with observations of the Chile 2015 tsunami in rivers in Japan. Pure Appl. Geophys. 173, 389–409. doi:10.1007/s00024-015-1229-0
van Dorn, W. G. (1984). Some tsunami characteristics deducible from tide records. J. Phys. Oceanogr. 14, 353–363. doi:10.1175/1520-0485(1984)014〈0353:STCDFT〉2.0.CO;2
van Dorn, W. G. (1987). Tide gauge response to tsunamis. part 2: other oceans and smaller seas. J. Phys. Oceanogr. 17, 1507–1516. doi:10.1175/1520-0485(1987)017<1507:TGRTTP>2.0.CO;2
Volpe, M., Lorito, S., Selva, J., Tonini, R., Romano, F., and Brizuela, B. (2019). From regional to local SPTHA: efficient computation of probabilistic tsunami inundation maps addressing near-field sources. Nat. Hazards Earth Syst. Sci. 19, 455–469. doi:10.5194/nhess-19-455-2019
Watada, S., Kusumoto, S., and Satake, K. (2014). Traveltime delay and initial phase reversal of distant tsunamis coupled with the self-gravitating elastic earth. J. Geophys. Res. Solid Earth. 119, 4287–4310. doi:10.1002/2013jb010841
Weatherall, P., Marks, K. M., Jakobsson, M., Schmitt, T., Tani, S., Arndt, J. E., et al. (2015). A new digital bathymetric model of the world’s oceans. Earth Space Sci. 2, 331–345. doi:10.1002/2015EA000107
Whiteway, T. (2009). Technical Report, Geoscience Australia Record 2009/21. Australian bathymetry and topography grid, June 2009.
Williamson, A., Newman, A., and Cummins, P. (2017). Reconstruction of coseismic slip from the 2015 Illapel earthquake using combined geodetic and tsunami waveform data. J. Geophys. Res. 122, 2119–2130. doi:10.1002/2016JB013883
Wilson, K. M., Allen, S. C. R., and Power, H. E. (2018). The tsunami threat to Sydney Harbour, Australia: modelling potential and historic events. Sci. Rep. 8, 15045. doi:10.1038/s41598-018-33156-w
Wilson, K. M., and Power, H. E. (2018). Seamless bathymetry and topography datasets for new south wales, Australia. Scientific Data. 5, 180115. doi:10.1038/sdata.2018.115
Yamazaki, Y., Cheung, K. F., and Lay, T. (2018). A self-consistent fault slip model for the 2011 tohoku earthquake and tsunami. J. Geophys. Res. Solid Earth. 123, 1435–1458. doi:10.1002/2017JB014749
Yue, H., Lay, T., Li, L., Yamazaki, Y., Cheung, K. F., Rivera, L., et al. (2015). Validation of linearity assumptions for using tsunami waveforms in joint inversion of kinematic rupture models: application to the 2010 MentawaiMw7.8 tsunami earthquake. J. Geophys. Res. Solid Earth. 120, 1728–1747. doi:10.1002/2014jb011721
Keywords: tsunamis, shallow water equations, friction, tsunami observations, numerical methods
Citation: Davies G, Romano F and Lorito S (2020) Global Dissipation Models for Simulating Tsunamis at Far-Field Coasts up to 60 hours Post-Earthquake: Multi-Site Tests in Australia. Front. Earth Sci. 8:598235. doi:10.3389/feart.2020.598235
Received: 24 August 2020; Accepted: 02 October 2020;
Published: 30 October 2020.
Edited by:
Chong Xu, National Institute of Natural Hazards, ChinaReviewed by:
Alexander B. Rabinovich, P.P. Shirshov Institute of Oceanology (RAS), RussiaEfim Pelinovsky, Institute of Applied Physics (RAS), Russia
Copyright © 2020 Davies, Romano and Lorito. This is an open-access article distributed under the terms of the Creative Commons Attribution License (CC BY). The use, distribution or reproduction in other forums is permitted, provided the original author(s) and the copyright owner(s) are credited and that the original publication in this journal is cited, in accordance with accepted academic practice. No use, distribution or reproduction is permitted which does not comply with these terms.
*Correspondence: Gareth Davies, Z2FyZXRoLmRhdmllc0BnYS5nb3YuYXU=