Landslide Susceptibility Modeling Using a Hybrid Bivariate Statistical and Expert Consultation Approach in Canada Hill, Sarawak, Malaysia
- 1Department of Geology, Faculty of Science, University of Malaya, Kuala Lumpur, Malaysia
- 2Technical Services Division, Department of Mineral and Geoscience Malaysia, Ipoh, Malaysia
- 3Southeast Asia Disaster Prevention Research Initiative (SEADPRI-UKM), Universiti Kebangsaan Malaysia, Bandar Baru Bangi, Malaysia
Landslide susceptibility assessment was conducted in Canada Hill, Sarawak, Malaysia through a combined bivariate statistics and expert consultation approach using geographical information system, which captures landslide-conditioning parameters specific to the study area; to ensure its usefulness in practice. Over the past four decades, many landslide parameters and increasingly sophisticated statistical methods have been used in landslide research. However, the findings have had very limited use in practice as the actual ground conditions are not well represented. The weakness is due to poor quality of data in landslide inventories and inadequate understanding of landslide-conditioning parameters. In this study, bivariate statistical method was used in conjunction with an iterative process of expert consultation. Thirteen original landslide-conditioning parameters were narrowed down to six, with the addition of a unique parameter, planar failure potential, which was selected based on expert input. The parameter captures planar failure landslides, which has the highest impact in the study area, causing loss of lives and property destruction. The inaugural landslide susceptibility map for the study area has five classes; very low, low, moderate, high and very high susceptibility. All major planar failures and most smaller circular failures fall within the very high susceptibility class, with a success rate of 75.8%. The approach used in this study has improved the quality of the landslide inventory and delineated key conditioning parameters. The resultant map captures local conditions, which is useful for landslide management.
1 Introduction
Landslides are common in areas with rugged topography and while their occurrence cannot be prevented they can be mitigated to lessen the impact on society (Crozier, 1986). Aspects that influence landslide occurrence include geological features such as rock-type, structure, soil type and weathering depth as well as other factors such as slope gradient, ground saturation and land cover, among others. Landslide susceptibility essentially refers to the potential for failures to occur in a given area based on surficial and subsurface conditions and processes. Landslide susceptibility modeling is based on the premise that past events leave recognizable morphological features that can be identified and mapped through field observation or remote sensing (Rib and Liang, 1978; Varnes, 1978; Hansen, 1984; Hutchinson, 1988; Dikau et al., 1996). Such incidents are controlled by physical principles that can be quantitatively analyzed. Both past and present landslides provide valuable information on conditions of failure to anticipate future areas of occurrences. Landslide susceptibility modeling results in maps that can be used for land use planning and reducing the risk of infrastructure and communities to landslides.
Landslide susceptibility models delineate areas where landslides are likely to occur based on local geological, geomorphological and other physical conditions. Landslide susceptibility models can be developed using qualitative or quantitative techniques. Quantitative susceptibility modeling is conducted using deterministic (analytically driven), heuristic (knowledge-driven) or data-driven statistical bivariate, multivariate and machine learning approaches (collectively referred to as statistical approaches in this paper). The deterministic approach is best suited for terrain with similar landslide types, geological setting and geomorphological conditions; thus have limited use for susceptibility modeling over larger areas (Soeters and van Westen, 1996). The heuristic approach is semi-quantitative, relying on ranking and weighting of known instability factors based on their importance in causing landslides, using expert knowledge. This approach is subject to misconception, landform misinterpretation and uncertainty for those unfamiliar with the area under investigation (Reichenbach et al., 2018). Bivariate or multivariate approaches involve linear correlation analysis between landslide events and conditioning factors. Machine learning approaches address non-linear correlation analysis between the events and factors. There has been much advancement in remote sensing technology, Geographical Information System (GIS) and computing capability since the 1990s. It has made landslide susceptibility modeling using statistical approaches more efficient and inexpensive. High-resolution maps covering large tracts of areas that were previously inaccessible can now be easily produced.
A landmark review of landslide susceptibility modeling based on statistical approaches revealed that the parameters used over the past four decades have remained similar but the significance of its selection with respect to ground conditions and understanding of landslide processes is poorly justified (Reichenbach et al., 2018). In some cases, landslide susceptibility modeling using bivariate statistical methods have yielded better results compared to multivariate approaches. Bivariate statistical approaches are also very reliable when combined with expert inputs (van Westen et al., 2003). Machine learning techniques have proliferated recently but its use in landslide susceptibility modeling is limited (Merghadi et al., 2020). It is due to shortcomings associated with algorithm selection, poor quality of data in landslide inventories and inadequate understanding of landslide-conditioning parameters. Poor data quality and inadequate understanding are issues in all statistical methods. While statistical approaches have become increasingly sophisticated in the research domain, its use in actual practice has also been questioned, particularly with respect to its precision in reflecting the actual factors that cause a slope to fail (Hearn and Hart, 2019). The importance of understanding the terrain and landslide condition parameters to develop a reliable inventory based on primary and secondary data from field investigations was emphasized. This is supported by recent findings which indicate that landslide inventories based on a combination of high-resolution images and field information provide better estimates of areas that are prone to shallow landslides (Bordoni et al., 2020; Merghadi et al., 2020). A comparative study revealed that machine learning models are more accurate than general statistical approaches limited by its linear analysis, and heuristic methods using subjective weights (Huang et al., 2020). The single study uses the same landslide inventory in developing the susceptibility models. While the study is very sophisticated in its statistical approach, there was no mention of the quality of the landslide inventory. The relationship between contributing factors and landslide incidents was also not elaborated. The landslide inventory for statistical approaches can comprise about 50 to over 600 landslide events (Pradhan, 2011; Abedini et al., 2019; Bordoni et al., 2020; Huang et al., 2020; Wu et al., 2020). However, the number of events recorded does not reflect the quality of the landslide inventory. The quality of the landslide inventory is reflected by records from field observations on failure characteristics and landslide-conditioning parameters.
A limited number of landslide susceptibility studies have been conducted in Malaysia over the past decade, primarily using statistical approaches (Pradhan, 2011; Sharir et al., 2017; Jeong et al., 2018; Sameen et al., 2020). Areas covered include Cameron Highlands, Penang Hill, Putrajaya and parts of Kuala Lumpur in Peninsular Malaysia, as well as Kundasang, located in the state of Sabah in East Malaysia (Pradhan, 2011; Sharir et al., 2017; Jeong et al., 2018; Sameen et al., 2020). In Peninsular Malaysia, the rock types are mainly granites and metamorphic rocks, and the soil profile is thick. The dominant mode of failure is rotational slide, while other modes include soil flow and shallow translational slide (Pradhan, 2011; Sameen et al., 2020), with deep seated landslides reported in the Kuala Lumpur area (Jeong et al., 2018). The number of landslide-conditioning parameters vary; up to a maximum of 12 factors have been used covering geological, geomorphological and other aspects. In Sabah, landslide density maps were developed to delineate susceptibility zones using four conditioning parameters in the area: slope angle, slope aspect, lithology and soil type. (Sharir et al., 2017). The landslide density is high in natural slopes steeper than 35° and cut slopes of 25°–35° as well as soils derived from metasedimentary rocks. Many of the studies in the country have reported model performance in terms of fit and prediction capability. The studies also noted that the quality of modeling is dependent on the property and completeness of the inventory, particularly with respect to the observed landslide-conditioning parameters as well as their linkages (Pradhan, 2011; Sharir et al., 2017).
This paper focuses on landslide susceptibility modeling of Canada Hill in Miri, Sarawak, which is part of East Malaysia in north west Borneo Island. It is the first attempt to develop a landslide susceptibility model for the area. The study combines the statistical bivariate method with expert consultation to develop a landslide susceptibility model for the area (van Westen et al., 2003). The bivariate method is simple and reliable for incorporating landslide-conditioning parameters, while expert consultation provides insights on the significance of the parameters. This hybrid approach was taken to overcome the limitation of statistical methods in reflecting the actual factors that cause a slope to fail and ensure its usefulness in actual practice (Reichenbach et al., 2018; Hearn and Hart, 2019). It also facilitates the establishment of an inventory that could be further developed to test more sophisticated statistical analysis to cover larger tracts of area. The next section comprises a brief overview of the study area with respect to the geology and landslide characteristics. Subsequently, a brief account is provided on data acquisition and the methods deployed. The results and discussion are centered on the landslide-conditioning parameters, susceptibility maps, model performance, and recommendations for future work, followed by the final concluding section.
2 Overview of the Study Area
Canada Hill is a narrow northeast-southwest trending ridge located in Miri, Sarawak (Figure 1). The hill, which extends up to 8 km is underlain primarily by the middle Miocene-Pliocene (∼10 Ma) Miri Formation, consisting of interbedded deltaic sandstone and shale (Liechti et al., 1960). The late Miocene Seria Formation is in conformity with the Miri Formation and is almost similar in lithology, comprising laminated thin sandstone, sandy shale and shale with some lignite (Liechti et al., 1960). The Pleistocene Terrace deposits comprise well sorted loose quarzitic sands deposited in the near-shore environment, which were uplifted to the present position by block faulting (Kessler and Jong, 2014) (Figure 1). The flat plain adjacent to the hill consists of Holocene marine deposits and peat. Canada Hill is part of the faulted Miri Anticlinal structure, an open fold which extends to the sea in a south-westward direction and plunges toward the northeast (Wannier et al., 2011). The strata of the north-western limb of the anticline mainly dip north-westerly between 20° and 30°, while those on the south-eastern limb dips south-easterly between 30° and 60°. The strata are cut by numerous faults and joints.
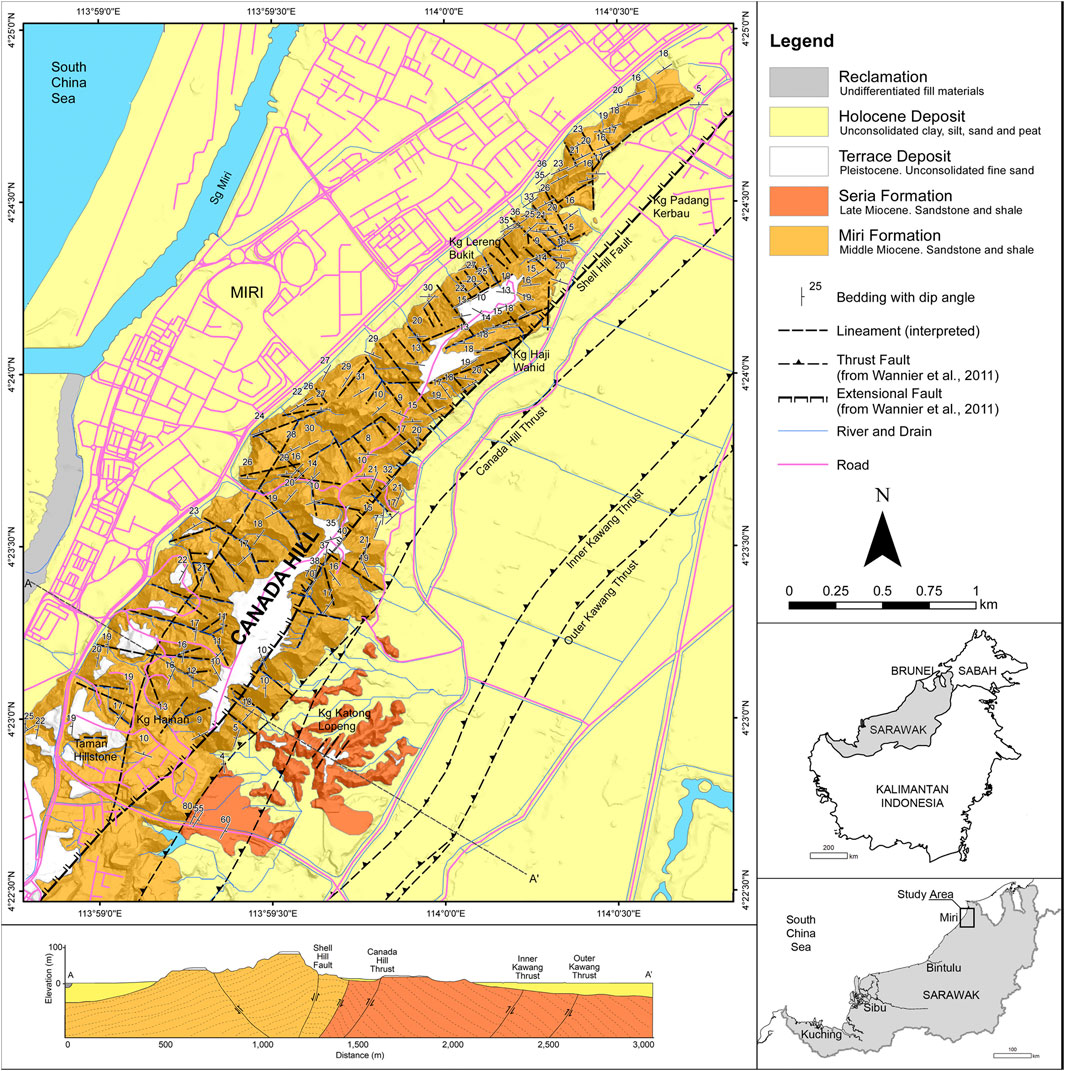
FIGURE 1. Geological map and cross section of Canada Hill in Miri, Sarawak. Extension and thrust faults, and naming of Miocene geological units after Wannier et al. (2011).
A total of 62 landslides were recorded in Canada Hill comprise dominantly shallow rotational failures followed by planar failures and toppling failures. Two major landslides (LS1 and LS2) that occurred in 2009 have caused significant damage to properties and two fatalities. The slip surfaces of these two landslides are along the sandstone-shale beds with an average slope gradient of about 25°. Landslide occurrences in Canada Hill are common during prolonged periods of heavy rainfall during the north-east monsoon, due to water seepage and surface water runoff (Banda et al., 2009; Mohd, 2014).
3 Materials and Methods
Data Acquisition
The study commenced with data acquisition from a variety of sources (Figure 2). A base map of Canada Hill was prepared using topographic maps, Shuttle Radar Topography Mission (SRTM) data, satellite imageries and geological maps by Wannier et al. (2011). The 1 arc-second SRTM was obtained from a public domain run by USGS (https://earthexplorer.usgs.gov/) while the topographic map of 1 m elevation intervals was obtained from the Department of Mineral and Geoscience Malaysia. The topographic map was derived from erial LiDAR data acquired by the Malaysian Public Works Department for the landslide investigation of Canada Hill by the Department of Mineral and Geoscience Malaysia (Mohd, 2014). A digital elevation model (DEM) with a 1 m cell size was generated. The DEM was overlain with satellite imageries from Google Earth to aid in the base map digitization, identification of spatial locations of past landslides and field investigation. The resultant maps were converted into geotiff files and uploaded into “Avenza Maps”, a mobile application utilized for verification and updating geological and landslide information in the field.
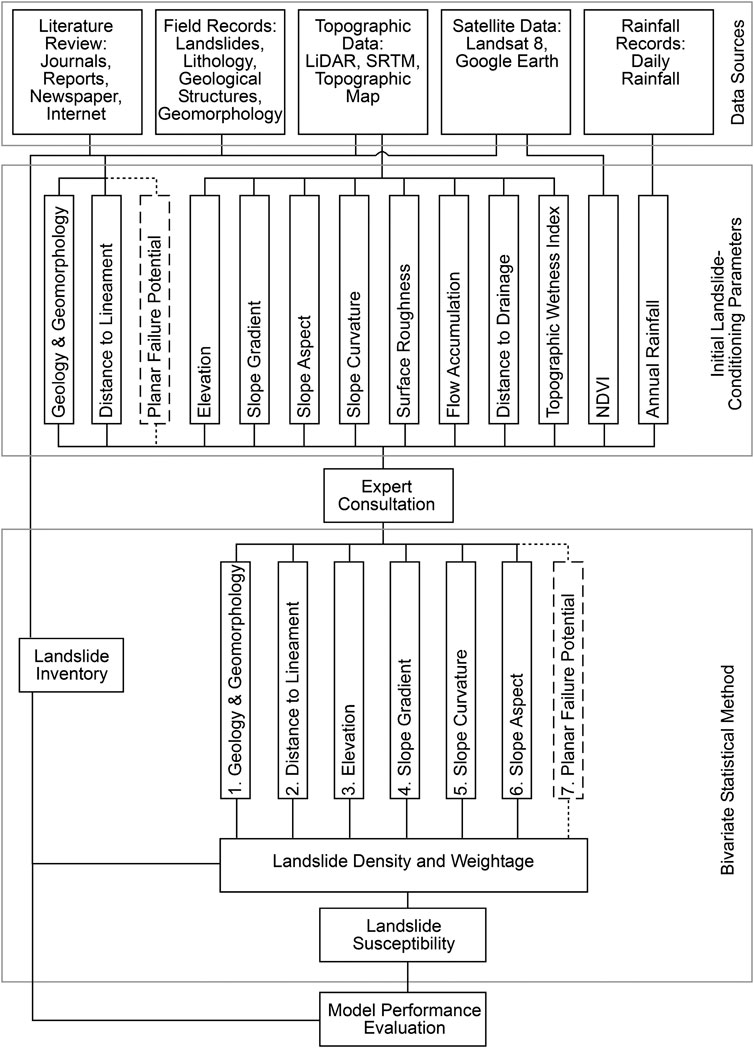
FIGURE 2. Flow chart of the landslide susceptibility modeling using a hybrid approach, where expert opinion was used for the selection of landslide-conditioning parameters for bivariate statistical analysis.
The landslide inventory was developed from field mapping and observations, interpretation of satellite imageries and DEM, and historical records from the Minerals and Geoscience Department of Malaysia and the newspapers. The tropical climate in Malaysia promotes rapid erosion and overgrowth of vegetation that easily wipes out evidence of slope movements within a few years. Thus, sequential Google Earth images ranging from 5 to 7 years over 14 years were interpreted and compared to obtain the best sense of slope movement, changes of land use and other human activities (van Westen et al., 1997). Field verification was conducted in cases where past minor shallow failures were difficult to demarcate accurately on the map.
Landslide-Conditioning Parameters
Thirteen landslide-conditioning parameter maps were initially created for the susceptibility analysis. They are: geology and geomorphology; distance to lineament; planar failure potential; distance to drainage; normalized differentiated vegetation index (NDVI); annual rainfall; slope gradient; elevation; slope aspect; slope curvature; topographic wetness index (TWI); surface roughness; and flow accumulation. The last seven are primary and secondary geomorphometric parameters derived from the DEM.
The geological map was produced primarily from field investigations and information from Wannier et al. (2011). Geomorphology was obtained from field observations and interpretation of shaded relief maps derived from the DEM. The geomorphology of the study area is closely related to the geology and it can be divided into five classes: Miri Formation; Seria Formation; Terrace Deposits, Holocene Deposits and Fill Material. The Miri Formation mainly occupies the severely eroded and deeply incised slopes of Canada Hill. The terrain is consisting of narrow V-shaped valleys and narrow ridges. The relief is between 10 and 50 m. The Seria Formation forms low elongated hills with relief of between 5 and 15 m. Soils from Miri and Seria formations comprise of highly erodible sand and clay with low plasticity (Muol, 2009). The Terrace deposits are 1–3 m thick and occupy the flat top and the gentle flanks of the plateau-like Canada Hill. The Holocene deposits are comprising of marine clay and organic soils of the coastal plain, peat occupying coastal swamps and beach sand along the coast. The estuary of the Miri River has been reclaimed using undifferentiated fill materials.
Major joints and faults present around the hill form prominent negative lineaments observed in the shaded relief maps and satellite imageries. In addition, several major thrust and normal faults have been interpreted from subsurface exploration data (Wannier et al., 2011). The faults can introduce tectonic stress and cause shearing of the bedrock. The joints and faults are pathway for the ingress of water and caused increased weathering intensity. The distance to lineament classes are more than 250 m; 150 m–250 m; 75 m–150 m; 25 m–75 m; and less than 25 m.
Slope gradient is considered a very important parameter in landslide susceptibility assessment (Reichenbach et al., 2018) and was used in this study to understand the morphological character, rate of soil erosion and mass wasting. The slope gradient parameter was derived from DEM and divided into six classes: 0°–5°; 5°–15°; 15°–25°; 25°–35°; 35°–60° and 60°–90°.
The effects of elevation on the landslide occurrences depend on the lithological character of the units. Higher elevations may promote the development of first order streams, resulting in slope steepening (Mandal and Mandal, 2017). The elevation of the study area was derived from the DEM and divided into five classes; 0 m–5 m; 5 m–25 m; 25 m–50 m; 50 m–75 m; and more than 75 m.
Convex and concave slopes have different responses to stability based on their moisture retention capabilities. The surface of concave slopes can retain moisture for a long time and may become a catchment for sediments to accumulate from overland flow. Meanwhile, on convex slopes, moistures are drained out immediately and transporting along sediments. This makes convex slopes more prone to landslide occurrences. The slope curvature parameter was derived from the DEM and divided into three classes: flat; convex; and concave.
The slope aspect may be used to identify slope segments that are most susceptible to landslides due to exposure to sunlight, drying winds, rainfall and discontinuities (Yalcin et al., 2011). The visual representation of slope aspect in a map also enables the representation of the direction of flow based on the orientation of ridges, spurs and valleys which in turn, can delineate places of potential water surplus region (Mandal and Mandal, 2017). Canada Hill exhibits a relatively simple anticline structure, as shown by the orientation of bedding which mainly strikes parallel to the trend of the hill. This can be used to indicate the influence of geological structure to landslide occurrences. The slope aspect was derived from the DEM and divided into nine classes; flat; North; Northeast; East; Southeast; South; Southwest; West; and Northwest.
The surface roughness was obtained from the standard deviation of elevation calculated using focal statistics over a 15 cell by 15 cell moving window (LaHusen et al., 2016). It is assumed that areas affected by landslides have a different roughness signature. The TWI is used to indicate ground moisture and areas accumulating water flow. It was calculated from the equation: ln (A/tanβ), where A is the upslope contributing area and tanβ is the local slope (Moore et al., 1991; Sørensen et al., 2006). The flow accumulation calculates the cumulative number of upslope cells that drain into it. It is often used to identify areas of concentrated flow, such as drainage channels.
Drainage was obtained from the topographic map. Buffer distances of 0–25 m, 25–50 m, 50–100 m, 100–250 m and more than 250 m were used. The NDVI was calculated from the red and near infrared bands of Landsat-8 imagery. The NDVI is divided into water, barren, sparsely vegetated, moderately vegetated and densely vegetated areas using break values selected by comparing the land cover and vegetation density of the classified area in the true color imagery.
Planar failure potential is a parameter that is used to assess the kinematic possibility of sliding. The parameter can be determined for the study area because the geological structure is simple, where dip and dip directions can be interpolated from discrete bedding points using circular statistics. The method from de Kemp (1998) was used to extract the direction cosines of the bedding data. The dip direction and dip angle maps were created separately. The first step involves calculating the angle between the slope aspect and dip direction of the bedding. The second step is to determine the angle between the slope gradient, dip angle of the bedding and friction angle. Based on observations of past planar failures, a friction angle of 25° is assumed and the angles between 15° and 25° are considered marginal. The bedding plane that strikes within 0°–30° to the slope face is considered the most vulnerable. This parameter is divided into five classes of in relation to potential instability caused by bedding controlled planar failure; very high, high, moderate, low and very low.
Average annual rainfall was calculated from daily rainfall data from five rainfall stations near the study area for the period between 2007 and 2016. The average annual rainfall of the five stations was used to interpolate the rainfall for the entire study area using the kriging method.
Bivariate Statistical Method
The bivariate statistical method is based on the analysis of the functional statistical relationship between landslide-conditioning parameters and the known distribution of landslides (Carrara, 1983; Naranjo et al., 1994; Guzzetti et al., 1999; van Westen et al., 2003; Reichenbach et al., 2018). The tendency of an area to experience landslide is determined from the functional relationship, where weights are calculated for each parameter from the landslide density.
The importance of each landslide-conditioning parameter is analyzed individually by comparing the map of the parameter with the landslide distribution map. Landslide densities were calculated using the equation of van Westen (1993) for each parameter map to obtain the weight (Eq. 1). The densities are equivalent to the number of landslides over the area occupied by the parameter.
where.
Wi = weight of a certain parameter class (e.g., a rock type, or a slope class)
Densclass = landslide density within the parameter class
Densmap = landslide density within the entire map
Npix (Si) = number of pixels, which contain landslides, in certain parameter classes
Npix (Ni) = total number of pixels in a certain parameter class.
The analysis utilizes the pixel cells in a map and therefore maps that were initially in vector format were converted into rasters. The raster maps were then analyzed to obtain the number of pixels and landslide density for each parameter class. Weight is calculated from the landslide density within a class with the landslide density of the whole area.
Negative weights would mean that landslide density is lower than normal, positive weights if the landslide density is higher than normal and zero weight if there are no landslide occurrences in a certain parameter class or the class may or may not contribute to landslide occurrences (van Westen, 1997). However, certain subclasses that do not contain any landslide occurrences would return a “no pixel data” or 0 Npix (Si) value for certain subclasses due to the absence of landslide occurrences. This problem leads to errors in calculation for the weights as the incorporation of 0 Npix (Si) value in the equation would approach infinitesimal. Therefore, an initiative to quantitatively assign a relatively lower value than the weight was done although this would not exactly show the information value of the area (Oliveira et al., 2015). The weights were then used to reclassify the chosen landslide-controlling parameters to be used in the overlay analysis for the generation of landslide susceptibility map.
The final landslide susceptibility map is a summation of the reclassified parameter maps. The resultant map yielded a continuous value and was normalized for subsequent reclassification into five classes to denote areas of very low, low, moderate, high and very high susceptibility to landslide occurrences. The susceptibility classes were obtained through manual classification adapted from Australian Geomechanics Society (AGS), 2007 and Fell et al. (2008). In this classification, the very high susceptibility class contains more than 50% of the landslide pixels, high susceptibility 25%–50%, moderate susceptibility 12.5%–25%, low susceptibility 6.25%–12.5% and the very low susceptibility 0%–6.25% of the landslide pixels.
Expert Consultation
An iterative expert consultation process was instituted to enable peer review and provision of inputs to the study. The process is also a means of controlling the quality of data in the landslide inventory. Inputs were obtained in the development of the landslide inventory, identification and screening of landslide-conditioning processes and review of the bivariate statistical results (Figure 2). The international panel of experts comprised practitioners from the private sector and government as well as researchers, with at least two years of experience working on landslides in tropical terrain. Several had intimate knowledge of the geology, geomorphology and slope failures in the study area. Initially, a menu of landslide-conditioning parameters was presented to seek opinions in terms of their suitability for landslide susceptibility modeling (Daniel and Ng, 2018). The menu was then modified where parameters were selected, dropped or added based on the experiential and local knowledge of the expert panel, as well as results from the bivariate statistical analysis. Statistical analysis generally does not utilize expert inputs and rely on objective correlation of landslide occurrence with conditioning parameters. The use of expert judgment is usually employed in the heuristic analysis. The combination of the bivariate statistics method and expert consultation in this study is referred to as a hybrid approach.
Evaluation of Model Performance
The success rate of the landslide susceptibility maps produced from the different approaches were calculated and compared by means of a validation curve, also known as receiver operating characteristic (ROC). The ROC-curve was plotted using the obtained cumulative percentage of landslide occurrences (y-axis) and the percentage of landslide susceptibility classes arranged from the highest to the lowest values (x-axis). A totally random prediction would yield a hypothetical validation “curve” coinciding with a diagonal line from 0 to 1 and the position of the validation curve resulting from the landslide data of a study area relative to the hypothetical “curve” would determine the model’s predictive value and capability (Remondo et al., 2003). The landslide susceptibility classes were re-categorized into 10 classes from 0–100 with the interval of 10 prior to calculating the area under the ROC curve (Sameen et al., 2020).
4 Results
Landslide Distribution
The landslide inventory of Canada Hill currently consists of 62 landslides. These include 52 rotational, five translational, three planar and two toppling failures. Thirteen initial landslide-conditioning parameters were screened by experts for landslide susceptibility modeling (Figure 2).
In the first iteration with the experts, seven parameters were considered unsuitable for the study area and omitted from the assessment. These are surface roughness, flow accumulation, distance to drainage, TWI, NDVI, annual rainfall and planar failure potential (Table 1). Of the unsuitable parameters, surface roughness, distance from drainage and TWI show poor correlation with landslides. The NDVI is not reliable when calculated from an imagery because it does not reflect the vegetation density during the actual landslide event. The date of most landslide occurrences in not known and Canada Hill experiences fluctuations in vegetation density due to forest fires and modification of land use, making NDVI an unsuitable parameter. Rainfall that is more of a triggering factor than a predisposing factor and flow accumulation, which is more suitable for debris flow are both omitted. Planar failure potential was also not considered in the first iteration because it is a subjective parameter derived from the combination of slope aspect, slope gradient and bedding orientation.
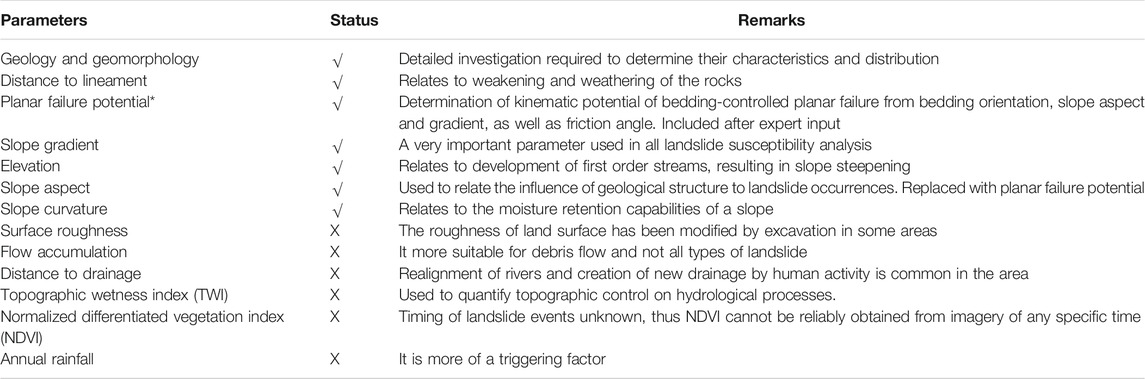
TABLE 1. An overview of landslide-conditioning parameters used in the study. The expert consultation process resulted in 6 parameters being omitted and replacement of slope aspect with planar failure potential.
The first iteration with experts led to the selection of six parameters for the modeling (Figure 3). These are geology and geomorphology, distance to lineament, slope gradient, elevation, slope curvature and slope aspect (Table 2). The majority of the landslides occur in the Miri Formation. The remaining occur in the terrace deposit. Generally, there is an increase in landslide occurrences with decreasing distance to lineament. Slopes with an angle of 15°–25° recorded the highest number of landslides (35.5%), followed by 25°–35° slopes (24.2%) and 35°–60° slopes (22.6%). No landslide was recorded in the steepest slopes (60–90°) probably because these slopes occupy only a very small areal extent of 0.03%. There is a general increase of landslides with increasing elevation. The highest occurrence of landslides (56.4%) falls in the elevation class of 50 m–75 m. Only 12.9% of landslides fall in areas above 75 m, probably due to its smaller areal extent and the presence of relatively flat plateau at the top of the Canada Hill. Approximately 64.5% of the landslides in the study area occur in convex slopes while 35.5% are in the concave slopes. With respect to slope aspect, landslide occurrences are common in the slopes facing E, SW, W, and NW, with the highest number of occurrences recorded in NW slopes (19.4%). As the landslide distribution did not record major planar failures that caused loss of lives in either the high or very high classes of susceptibility, the parameters selected were reappraised.
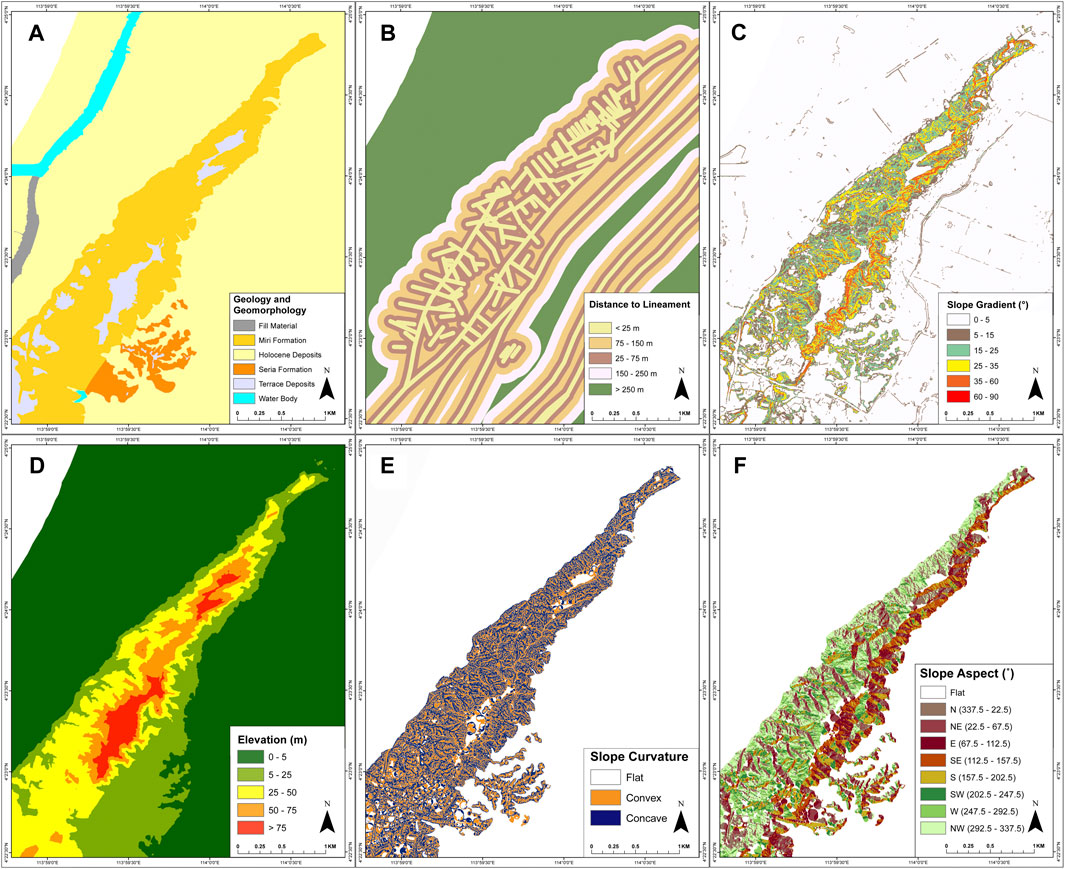
FIGURE 3. Landslide-conditioning parameter maps. (A) Geology and geomorphology (B) distance to lineament (C) slope gradient (D) elevation (E) slope curvature, and (F) slope aspect.
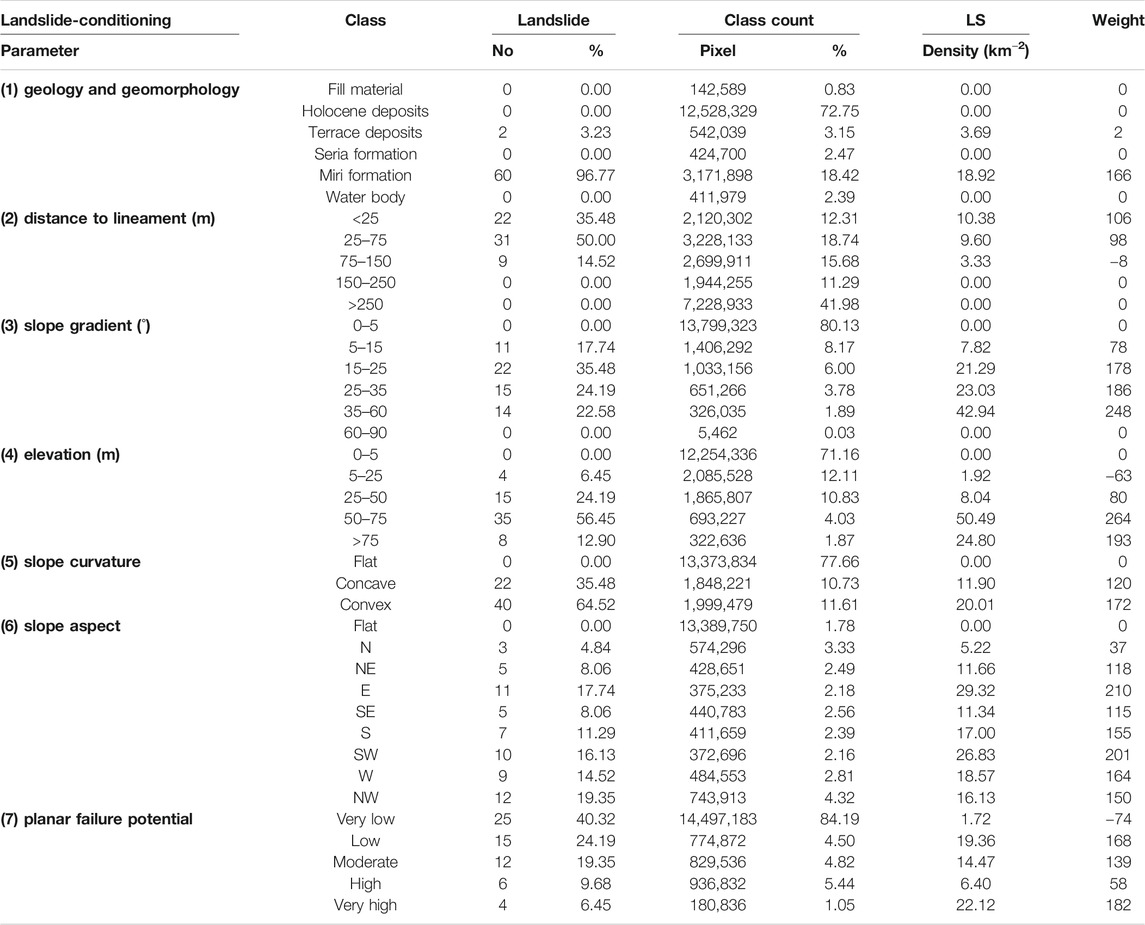
TABLE 2. The weights and the relationship between landslide-controlling parameters and landslide distribution using the bivariate statistical approach. Only the first six parameters were used in the first analysis. In the second analysis slope aspect was replaced with planar failure potential.
The second iteration with experts led to the substitution of slope aspect with planar failure potential in order to improve the susceptibility map for planar failures. Most of the landslides at Canada Hill occurred in the area of very low in terms of planar failure potential, which accounts for 40.3% of the landslide occurrence while the high and very high potential area accounts for 16.1% (Table 2). A probable explanation for this number is that most of the landslides recorded in the inventory are shallow minor landslides that failed rotationally as opposed to having a planar failure mechanism. Major landslides LS1 and LS2 fell in the very high potential area, indicating that this parameter can enhance the landslide susceptibility assessment by capturing the infrequent but extensive planar failures occurring in the study area.
Landslide Susceptibility
The landslide susceptibility map is shown in Figure 4. Areas with very high and high susceptibility are mainly located on the upper slopes of Canada Hill, immediately below the flat plateau. The high susceptibility areas also occur below the ridges at the mid-slopes. These areas underlain by Miri Formation are deeply incised by erosion. The moderately susceptible areas mainly occur in the lower slopes. Areas with low susceptibility include the foot slopes of Canada Hill and low hills underlain by Seria Formation, while the very low susceptibility areas are the coastal plain covered by Holocene deposits, the flat to gently sloping areas underlain by the Terrace deposits and Seria Formation.
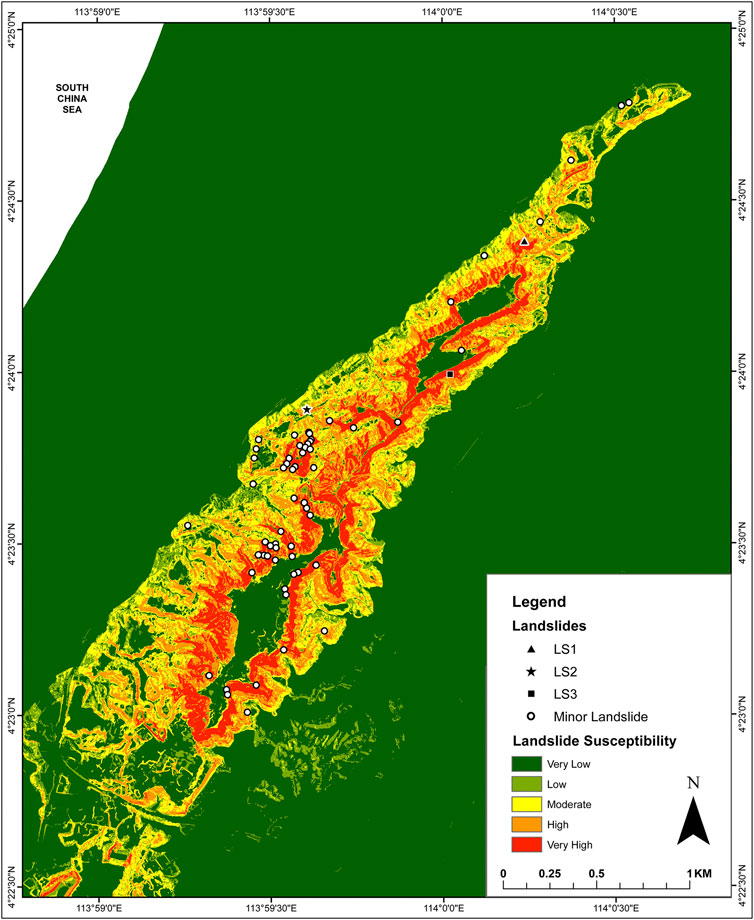
FIGURE 4. Landslide susceptibility map of Canada Hill produced from six parameters; geology and geomorphology, distance to lineament, slope gradient, elevation, slope curvature, and slope aspect.
The landslide susceptibility assessment using the bivariate statistical approach utilized the weights in Table 2. The first iteration of the bivariate analysis resulted in a landslide susceptibility map (Figure 4) that shows very satisfactory agreement to landslide occurrences. The success rate is 80.8% (Figure 5). However, the map failed to place a major planar landslide (LS2) in the high or very high susceptibility zone. The major landslide LSI falls within the high susceptibility zone. The scarcity of data in the inventory, especially on planar failures impaired the integration of the planar failures in the susceptibility map. The second bivariate statistical analysis was carried out to refine the process by replacing the slope aspect with planar failure potential parameter (Figure 6) in the assessment based on expert consultation. The landslide susceptibility map was produced using the same weights as shown in Table 2. The landslide susceptibility map produced in the second iteration (Figure 7) captured both major planar failure occurrences in the very high susceptibility zone. The success rate of the revised map is within the very satisfactory range although there is an overall reduction to 75.8% (Figure 5). The inclusion of the planar failure potential parameter originated from the expert consultation. The introduction of this element in a structured way to the bivariate statistical model, appears to have strengthened this approach by incorporating vital local knowledge.
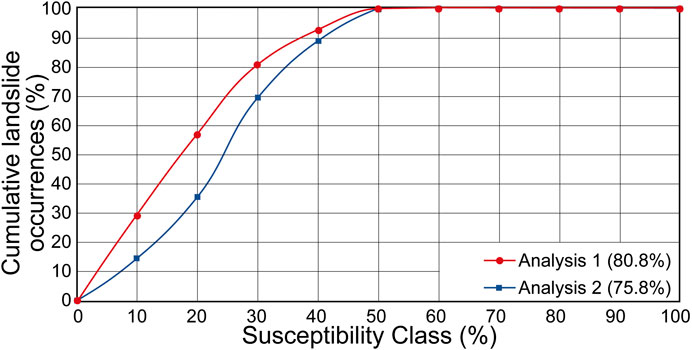
FIGURE 5. Plot of cumulative percentage of landslide occurrences vs. percentage of landslide susceptibility classes. The success rate is calculated as the area under the curve. Analysis 1 was calculated from the six initial parameters (geology and geomorphology, distance to lineament, slope gradient, elevation, slope curvature, and slope aspect) and in analysis 2, slope aspect was replaced with planar failure potential.
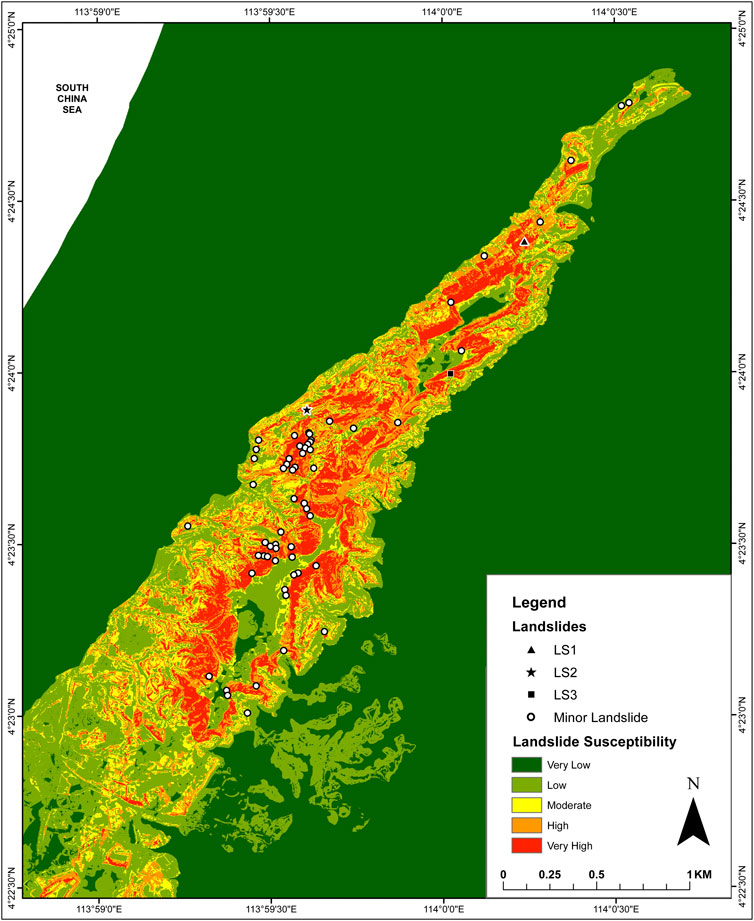
FIGURE 7. Revised landslide susceptibility map of Canada Hill where the parameter slope aspect was replaced with planar failure potential.
5 Discussion
The reliability and completeness of a landslide susceptibility map depends on the quality of the input parameters used in the landslide susceptibility assessment, the degree of experience of the person and the complexity of the study area geologically (Magliulo et al., 2009). The initial inventory of landslides for Canada Hill was developed from DEM, satellite images, previous literature and recent field investigation. Landslide inventories are of better quality based on a combination of high-resolution images and thorough field information (Bordoni et al., 2020; Merghadi et al., 2020). During the expert consultation, the quality of the landslide inventory was found to be deficient. This was primarily due to the difficulties in identifying relict shallow landslides and areas that were difficult to access during the fieldwork as well as insufficient high-quality erial photos from previous decades. It was highlighted that fast growing thick vegetation in tropical terrain rapidly mask failures in this region. This requires data to be continuously collected immediately after an event. The availability of unpublished field records provided by experts with extensive experience in the study area provided additional information to improve the quality of the landslide inventory.
The selection of landslide-conditioning parameters with respect to the geology, geomorphology and ground conditions have to be carefully understood and justified (Reichenbach et al., 2018). The understanding of the process that induce landslide occurrences gives an idea of landscape evolution, with regards of whether it being site-specific or homogenous across different areas. The understanding of the parameters was enhanced in the hybrid approach. The expert consultation enabled the delineation of bedding controlled planar failure, which occurs in the area. This mode of failure is generally confined to this particular geological setting, and is not commonly mentioned in the literature. The analysis of planar failure is normally conducted in detailed scale at specific sites. The expert consultation contributed to the extrapolation of the bedding orientation from specific location points to the entire study area. This enabled the planar failure potential to be assessed as a landslide-conditioning parameter that is unique to the area in the bivariate statistical method.
The iterative process of expert consultation has resulted in a susceptibility map that captured major planar failures in the very high susceptibility class. The initial susceptibility map that was generated using the bivariate statistical method without the input of this local information did not record the major planar failures in the very high susceptibility class. This confirms previous findings that bivariate statistical methods are very reliable when combined with expert inputs (van Westen et al., 2003). It may be argued that planar failure potential is a subjective landslide-conditioning parameter that cannot be replicated because it originated from expert input. However, the process of combining expert consultation when deploying the bivariate statistical method can be easily replicated. The process of instituting expert consultation to provide local knowledge of an area will be the same, though the nature of the final parameters used will differ based on local geological and geomorphological conditions. If the method is replicated in another area, the set of parameters used to create the susceptibility map may not be the same as those used in the present study. Nevertheless, the process of consultation enables better selection of landslide-conditioning parameters that represent the real field situation. The resultant landslide susceptibility map would then be relevant for actual practice.
A landslide inventory for statistical approaches may comprise nearly 600 events (Pradhan, 2011; Abedini et al., 2019; Bordoni et al., 2020; Huang et al., 2020; Wu et al., 2020). However, the quality of the data-set is more important than the number of events in the record. All studies should mention limitations of the landslide inventory and provide field information on actual contributing factors that cause a slope to fail. Furthermore, the applicability of the work in practice is also important. In using the hybrid approach, the expert consultation in this study comprised practitioners from the private sector and government as well as researchers. This provided additional experiential knowledge while at the same time securing the buy-in of practitioners on the use of the findings for landslide management. Thus, the composition of the expert panel is also important, to enhance the use of the findings beyond the study.
Many investigations have assessed model performance in terms of fit and prediction capability but very few have reported on uncertainties; these are primarily in multi-criteria statistical approaches (Reichenbach et al., 2018). It has been recommended model fit and prediction performances be complemented with uncertainty analysis to improve landslide susceptibility assessment. The bivariate method in this paper used receiver operating characteristic (ROC) to test the model performance, which is common in landslide susceptibility modeling. The aspect on uncertainty will be considered for future work in the study area.
6 Conclusion
Landslide susceptibility assessment was conducted in Canada Hill, Sarawak, Malaysia using a hybrid approach. The approach involved combining bivariate statistics with an iterative process of expert consultation. Thirteen landslide-conditioning parameters were narrowed down to six with an additional unique parameter selected based on expert input. These are geology and geomorphology, slope gradient, elevation, distance to lineament, slope curvature and slope aspect as well as planar failure potential. The use of bivariate statistics generated a susceptibility map with five classes; very low, low, moderate, high and very high. The initial map did not capture major planar failures in the very high susceptibility class. The success rate of the landslide susceptible model is 80.8%. The replacement of slope aspect with the unique planar failure potential based on expert consultation resulted in a map where all major planar failures and most smaller circular failures fall within the very high susceptibility class, with a success rate of 75.8%. Expert consultation has resulted in a map that captures local conditions, which is more useful for landslide management. The process of expert consultation also enabled quality control during landslide inventory development, enabling the capture of appropriate field observations on failure characteristics and landslide-conditioning parameters. The process of consultation has enabled the selection of landslide-conditioning parameters that is appropriate and representative of the field situation. The hybrid approach has potential for resulting in landslide susceptibility map that is relevant for practice.
Data Availability Statement
The raw data supporting the conclusions of this article will be made available by the authors, without undue reservation.
Author Contributions
JP mobilized resources for the study. NT and JP conceptualized and designed the study. MD and MA conducted the field investigation. MD analyzed the data. MD and NT wrote the manuscript. JP revised the manuscript. All authors read and approved the manuscript.
Funding
This research was supported by Newton-Ungku Omar Fund (Application ID: 59348-455144, UM project no. IF002–2017, UKM project no. XX-2017-002).
Conflict of Interest
The authors declare that the research was conducted in the absence of any commercial or financial relationships that could be construed as a potential conflict of interest.
Acknowledgments
The authors would like to thank the Editor for the opportunity to be part of this special issue. Thanks are also due to the Department of Mineral and Geoscience Malaysia for the digital data and field assistance, Malaysian Meteorological Department and Department of Irrigation and Drainage Malaysia for the rainfall data. The authors would also like to thank geologists and academicians from the British Geological Survey, SEADPRI, Geomapping Technology, Geoxpert and other organizations that have provided useful inputs for this study.
References
Abedini, M., Ghasemian, B., Shirzadi, A., Shahabi, H., Chapi, K., Pham, B. T., et al. (2019). A novel hybrid approach of bayesian logistic regression and its ensembles for landslide susceptibility assessment. Geocarto Int. 34, 1427–1457. doi:10.1080/10106049.2018.1499820
Australian Geomechanics Society (AGS) (2007). Guideline for landslide susceptibility, hazard and risk zoning for land use planning. Aust. Geomech. J. 42 (1), 13–36. doi:10.1016/j.enggeo.2008.03.022
Banda, R., Zakaria, M., and Kamaludin, H. (2009). Geological significance of landslide occurrences in Canada hill, Miri, Sarawak. Paper presented at the GEOSEA 2009: eleventh regional congress on geology, mineral and energy resources of Southeast asia. Hotel IstanaMalaysia: Kuala Lumpur.
Bordoni, M., Galanti, Y., Bartelletti, C., Persichillo, M. G., Barsanti, M., Giannecchini, R., et al. (2020). The influence of the inventory on the determination of the rainfall-induced shallow landslides susceptibility using generalized additive models. Catena 193, 104630. doi:10.1016/j.catena.2020.104630
Carrara, A. (1983). Multivariate models for landslide hazard evaluation. Math. Geol. 15 (3), 403–426. doi:10.1007/bf01031290
Daniel, M. T., and Ng, T. F. (2018). Assessment of landslide susceptibility in sedimentary rocks: a case study from Miri, Sarawak. Paper presented at the fifteenth regional congress on geology, Minerals and energy resources of Southeast asia. Hanoi, Vietnam: GEOSEA XV.
de Kemp, E. A. (1998). Three-dimensional projection of curvilinear geological features through direction cosine interpolation of structural field observations. Comput. Geosci. 24 (3), 269–284. doi:10.1016/S0098-3004(97)00066-6
Dikau, R., Brunsden, D., Schrott, L., and Ibsen, M. L. (1996). Landslide recognition. Identification, movements and causes. Chichester, England: Wiley.
Fell, R., Corominas, J., Bonnard, C., Cascini, L., Leroi, E., and Savage, W. Z. (2008). Guidelines for landslide susceptibility, hazard and risk zoning for land use planning. Eng. Geol. 102, 85–98. doi:10.1016/j.enggeo.2008.03.022
Guzzetti, F., Carrara, A., Cardinali, M., and Reichenbach, P. (1999). Landslide hazard evaluation: a review of current techniques and their application in a multi-scale study, Central Italy. Geomorphology 31, 181–216. doi:10.1016/s0169-555x(99)00078-1
Hansen, A. (1984). “Landslide hazard analysis” in Slope instability. Editors D. Brunsden, and D. B. Prior (New York: Wiley)), 523–602.
Hearn, G. J., and Hart, A. B. (2019). Landslide susceptibility mapping: a practitioner’s view. Bull. Eng. Geol. Environ. 78, 5811–5826. doi:10.1007/s10064-019-01506-1
Huang, F., Cao, Z., Guo, J., Jiang, S. H., Li, S., and Guo, Z. (2020). Comparisons of heuristic, general statistical and machine learning models for landslide susceptibility prediction and mapping. Catena 191, 104580. doi:10.1016/j.catena.2020.104580
Hutchinson, J. N. (1988). General report: morphological and geotechnical parameters of landslides in relation to geology and hydrology. Rotterdam, Balkema: Paper presented at the 5th International Symposium on Landslides.
Jeong, S., Kassim, A., Hong, M., and Saadatkhah, N. (2018). Susceptibility assessments of landslides in Hulu Kelang area using a geographic information system-based prediction model. Sustainability 10, 2941. doi:10.3390/su10082941
Kessler, F. L., and Jong, J. (2014). The origin of Canada Hill – a result of strike-slip deformation and hydraulically powered uplift at the Pleistocene/Holocene border?. Bull. Geol. Soc. Malays. 60, 35–44. doi:10.7186/bgsm60201404
LaHusen, S. R., Duvall, A. R., Booth, A. M., and Montgomery, D. R. (2016). Surface roughness dating of long-runout landslides near Oso, Washington (USA), reveals persistent postglacial hillslope instability. Geology 44 (2), 111–114. doi:10.1130/G37267.1
Liechti, P., Roe, F. W., and Haile, N. S. (1960). The geology of Sarawak, Brunei and the western part of north Borneo. Sarawak: Geological Survey Department British Territories in Borneo: Kuching.
Magliulo, P., Lisio, A. D., and Russo, F. (2009). Comparison of GIS-based methodologies for the landslide susceptibility assessment. Geoinformatica 13, 253–265. doi:10.1007/s10707-008-0063-2
Mandal, B., and Mandal, S. (2017). Landslide susceptibility mapping using modified information value model in the Lish river basin of Darjiling Himalaya. Spat. Inf. Res. 25, 205–218. doi:10.1007/s41324-017-0096-4
Merghadi, A., Yunus, A. P., Dou, J., Whiteley, J., ThaiPham, B., Bui, D. T., et al. (2020). Machine learning methods for landslide susceptibility studies: a comparative overview of algorithm performance. Earth Sci. Rev. 207, 103225. doi:10.1016/j.earscirev.2020.103225
Mohd, F. A. K. (2014). Siasatan tanah runtuh di Kampong padang kerbau, Miri, bahagian Miri, Sarawak. (JMG.SWK(GBN)1/2014). Kuching: Department of Mineral and Geoscience Malaysia.
Moore, I. D., Grayson, R. B., and Ladson, A. R. (1991). Digital terrain modelling: a review of hydrological, geomorphological, and biological applications. Hydrol. Proc. 5, 3–30. doi:10.1002/hyp.3360050103
Muol, E. (2009). Laporan tanah runtuh di Kg. Lereng bukit, bukit kanada, Miri, Sarawak. (JMG.SWK(GBN) 1/2009). Kuching, Sarawak: Department of Mineral and Geoscience Malaysia.
Naranjo, J. L., van Westen, C. J., and Soeters, R. (1994). Evaluating the use of training areas in bivariate statistical landslide hazard analysis– a case study in Colombia. ITC J. 1994-3, 292–300.
Oliveira, S. C., Zêzere, J. L., Catalão, J., and Nico, G. (2015). The contribution of PSInSAR interferometry to landslide hazard in weak rock-dominated areas. Landslides 12 (4), 703–719. doi:10.1007/s10346-014-0522-9
Pradhan, B. (2011). Use of GIS-based fuzzy logic relations and its cross application to produce landslide susceptibility maps in three test areas in Malaysia. Environ. Sci. 63, 329–349. doi:10.1007/s12665-010-0705-1
Reichenbach, P., Rossi, M., Malamud, B. D., Mihir, M., and Guzzetti, F. (2018). A review of statistically-based landslide susceptibility models. Earth Sci. Rev. 180, 60–91. doi:10.1016/j.earscirev.2018.03.001
Remondo, J., González, A., Terán, J. R. D. D., Cendrero, A., Fabbri, A., and Chung, C. J. F. (2003). Validation of landslide susceptibility maps; examples and applications from a case study in Northern Spain. Nat. Hazards 30, 437–449. doi:10.1023/B:NHAZ.0000007201.80743.fc
Rib, H. T., and Liang, T. (1978). “Recognition and identification” in Landslide analysis and control, Washington transportation research board special report 176. Editors R. L. Schuster, and R. J. Krizek (Washington: National Academy of Sciences), 34–80.
Sameen, M. I., Pradhan, B., Bui, D. T., and Alamri, A. M. (2020). Systematic sample subdividing strategy for training landslide susceptibility models. Catena 187, 104358. doi:10.1016/j.catena.2019.104358
Sharir, K., Roslee, R., Lee, K. E., and Simon, N. (2017). Landslide factors and susceptibility mapping on natural and artificial slopes in Kundasang, Sabah. Sains Malays 46 (9), 1531–1540. doi:10.17576/jsm-2017-4609-23
Soeters, R., and van Westen, C. J. (1996). “Slope instability recognition analysis and zonation” in Landslides: investigation and mitigation, transportation research board special report 247. Editors K. T. Turner, and R. L. Schuster (Washington: National Academy Press), 129–177.
Sørensen, R., Zinko, U., and Seibert, J. (2006). On the calculation of the topographic wetness index: evaluation of different methods based on field observations. Hydrol. Earth Syst. Sci. 10, 101–112. doi:10.5194/hess-10-101-2006
van Westen, C. J. (1993). Application of geographic information systems to landslide hazard zonation. [Enschede, The Netherlands]: International Institute for Geo-Information Science and Earth Observation. [doctoral dissertation].
van Westen, C. J., Rengers, N., and Soeters, R. (2003). Use of geomorphological information in indirect landslide susceptibility assessment. Nat. Hazards 30, 399–419.
van Westen, C. J., Rengers, N., Terlien, M. T. J., and Soeters, R. (1997). Prediction of the occurrence of slope instability phenomena through GIS-based hazard zonation. Geol. Rundschau 86, 404–414. doi:10.1007/s005310050149
van Westen, C. J. (1997). “Statistical landslide hazard analysis” in ILWIS application guide. Editors C. V. Westen, A. S. López, P. U. Cornejo and G. C. Ardanza (Enschede: ITC Publication), 73–84.
Varnes, D. J. (1978). “Slope movements, type and processes” in Landslide analysis and control. Transportation research board special report 176. Editors R. L. Schuster, and R. J. Krizek (Washington: National Academy of Sciences), 11–33.
Wannier, M., Lesslar, P., Lee, C., Raven, H., Sorkhabi, R., and Ibrahim, A. (2011). Geological excursions around Miri, Sarawak. Sarawak, Malaysia: MiriEcoMedia Software.
Wu, Y., Ke, Y., Chen, Z., Liang, S., Zhao, H., and Hong, H. (2020). Application of alternating decision tree with AdaBoost and bagging ensembles for landslide susceptibility mapping. Catena 187, 104396. doi:10.1016/j.catena.2019.104396
Yalcin, A., Reis, S., Aydinoglu, A. C., and Yomralioglu, T. (2011). A GIS-based comparative study of frequency ratio, analytical hierarchy process, bivariate statistics and logistics regression methods for landslide susceptibility mapping in Trabzon, NE Turkey. Catena 85, 274–287. doi:10.1016/j.catena.2011.01.014
Keywords: bivariate statistics, expert consultation, landslide-conditioning parameter, landslide susceptibility, geographic information system, Malaysia
Citation: Daniel MT, Ng TF, Abdul Kadir MF and Pereira JJ (2021) Landslide Susceptibility Modeling Using a Hybrid Bivariate Statistical and Expert Consultation Approach in Canada Hill, Sarawak, Malaysia. Front. Earth Sci. 9:616225. doi: 10.3389/feart.2021.616225
Received: 11 October 2020; Accepted: 26 January 2021;
Published: 11 March 2021.
Edited by:
Fang Chen, Institute of Remote Sensing and Digital Earth (CAS), ChinaReviewed by:
Jinhu Bian, Institute of Mountain Hazards and Environment (CAS), ChinaAmod Mani Dixit, National Society for Earthquake Technology Nepal (NSET), Nepal
Copyright © 2021 Daniel, Ng, Abdul Kadir and Pereira. This is an open-access article distributed under the terms of the Creative Commons Attribution License (CC BY). The use, distribution or reproduction in other forums is permitted, provided the original author(s) and the copyright owner(s) are credited and that the original publication in this journal is cited, in accordance with accepted academic practice. No use, distribution or reproduction is permitted which does not comply with these terms.
*Correspondence: Tham Fatt Ng, ntf@um.edu.my