- 1Key Laboratory of Mesoscale Severe Weather/Ministry of Education, Nanjing University, Nanjing, China
- 2School of Atmospheric Sciences, Nanjing University, Nanjing, China
- 3School of Environment, Nanjing Normal University, Nanjing, China
Two high-resolution Chinese regional reanalysis (CNRR) datasets at a resolution of 18 km during the period of 1998–2009 are generated by Gridpoint Statistical Interpolation (GSI) data assimilation system and spectral nudging (SN) method. The precipitation from CNRR is comprehensively evaluated against the observational datasets and global reanalysis ERA5 over East-Asia. The climatology mean, seasonal variability, extreme events, and summer diurnal cycle of precipitation are analyzed. Results show that CNRR reasonably reproduces the observed characteristics of rainfall, although some biases exist. The spatial distribution of climatology mean precipitation is well simulated by CNRR, while overestimation exists especially on the west side of Tibetan-Plateau (TP). CNRR reproduces the unimodal feature of the annual cycle with overestimations of summer precipitation, and well produces the probability of light and moderate rainfall but tend to overestimate heavy and extreme precipitation over most regions in China. The overall spatial distribution of extreme precipitation indices can be captured by CNRR. The diurnal cycle of summer precipitation, as well as the amplitude of diurnal cycle, are better reproduced by CNRR-GSI, capturing eastward propagation of diurnal phase from TP along the Yangtze River. CNRR-GSI generally outperforms CNRR-SN over most regions of China except in reproducing heavy and extreme rainfall in the Yangtze River Basin (YRB) and South China (SC) regions. CNRR-GSI shows comparable results with the latest ERA5 and outperforms it in simulating the diurnal cycle of precipitation. This dataset can be considered as a reliable source for precipitation related applications.
Introduction
Precipitation is a vital component of the hydrological cycle and the predominant and direct sources of water for the land surface water budget (Trenberth, 1999; Maurer et al., 2001; Risi et al., 2010; Zhou et al., 2011; Schneider et al., 2017). By affecting drinking water, lake and river, precipitation casts a remarkable influence on human life. Meanwhile, large economic losses and serious damage to both urban and rural areas can be caused by floods coming with extreme precipitation (Kunkel, 2003; Wang and Zhou, 2005; Li et al., 2011; Powell and Reinhard, 2016). Long-term precipitation data is the fundamental of climate research. Thus, accurate and reliable precipitation data at high spatiotemporal resolution is highly desirable for a wide range of applications from global freshwater budget (Veldkamp et al., 2016; Keune and Miralles, 2019; Llovel et al., 2019) to local hydrology (Orth et al., 2016; Lei et al., 2017).
In recent decades, atmospheric reanalysis datasets have been widely used in climate researches. Reanalysis datasets are derived from numerical weather prediction (NWP) systems anchored on long-term, observed climate data which are reanalyzed with data-assimilation techniques. Several global reanalysis datasets have been developed, such as the National Centers for Environmental Prediction/National Center for Atmospheric Research (NCEP/NCAR) global reanalysis (Kalnay et al., 1996), the European Center for Medium-Range Weather Forecast (ECMWF) reanalysis ERA-Interim (Dee et al., 2011) and ERA5 (Hersbach et al., 2020), the Modern-Era Retrospective Analysis for Research and Application (MERRA) reanalysis (Rienecker et al., 2011) and the Japan Meteorological Agency JRA-55 (Kobayashi et al., 2015), and they have been applied in numerous studies, including the detection of climate variability, the investigation of climate change and the evaluation of climate models (Sylla et al., 2010; Rudeva and Gulev, 2011; Olauson and Bergkvist, 2015; Hawcroft et al., 2017; Chen et al., 2019). Global reanalysis with high horizontal resolution is a milestone for climate researches. For example, the ERA5 global reanalysis with a 0.25-degree horizontal grid spacing released since 2018 introduced significant improvements in representing the atmosphere with much higher vertical and temporal resolutions compared to prior reanalyses (Zhang et al., 2019; Delhasse et al., 2020; Dullaart et al., 2020; Nogueira, 2020; Tarek et al., 2020; Taszarek et al., 2020). However, ERA5 performed unsuitable for trend analysis (Gleixner et al., 2020; Nogueira, 2020), and shows positive bias of temperature over complex topography, such as the Rocky Mountain area, possibly due to some processes not being accounted for in ERA5 (Tarek et al., 2020). Over TP, WRF-driven regional reanalysis shows advantage in simulating precipitation compared to ERA5 (He et al., 2019). The ability of such global reanalysis datasets in depicting regional-scale, complex terrain climate and diurnal-scale variability requires further evaluation and improvement (von Storch, 1999; Sotillo et al., 2005; Bromwich et al., 2016; Zhang et al., 2017). Thus, long-term reanalysis datasets with higher spatial and temporal resolutions are still in need for regional climate researches and investigating phenomena such as extreme rainfall events and mesoscale weather systems.
Regional reanalysis datasets have been developed to meet the demand for local applications with finer spatial resolutions (at about 10–30 km) that could provide more detailed meteorological information. To avoid the assumption of a perfect model and to reduce the errors at a regional scale, observations are also assimilated by a data assimilation framework in regional reanalysis systems. The North American Regional Reanalysis (NARR) was the first successful regional reanalysis utilizing NCEP Eta Model and its Data Assimilation System for the North American domain (Mesinger et al., 2006) and proved to be useful with detailed precipitation information. NARR has been widely used to study the precipitation characteristics in North America, such as understanding the characteristics of daily precipitation (Becker et al., 2009), investigating the precipitation recycling variability (Dominguez et al., 2008), and studying the suitability for hydrologic modeling and analysis in complex terrain (Trubilowicz et al., 2016). After NARR, there are many regional reanalysis datasets generated by regional models and data assimilation techniques over Europe (Bollmeyer et al., 2015; Dahlgren et al., 2016), Arctic (Bromwich et al., 2016), Australia (Su et al., 2019) and Indian monsoon region (Mahmood et al., 2018). Previous studies showed that regional reanalysis datasets with higher resolution are able to well reproduce local extreme events and reflect the spatial and temporal variability of precipitation. Comparison between two European regional reanalysis developed in the European Reanalysis and Observations For Monitoring (EURO4M) project and the global reanalysis revealed that regional reanalysis could improve the representation of precipitation, especially in representing high−threshold events and extreme events (Jermey and Renshaw, 2016). Besides the traditional data assimilation method, the spectral nudging (SN) technique (von Storch et al., 2000) is also used to produce a regional reanalysis dataset (Kanamaru and Kanamitsu, 2007). When applying the SN technique, the regional atmosphere model simulated variables are nudged to the large-scale global reanalysis forcing at the inner model domain. Using the dynamical downscaling method with SN technique, von Storch et al. (2017) developed a different procedure to produce the regional reanalysis without local data, which permits to generate additional regional details in observation-sparse regions.
Although several regional reanalysis datasets have been constructed and used in climate researches around the world, very limited reanalysis dataset is available over China. Zhang et al. (2017) constructed a high-resolution (18 km) regional reanalysis in China and its adjacent area via data assimilation and spectral nudging (SN), which was the preliminary and the first experimental attempt to construct a high-resolution reanalysis for China mainland. To extend the CNRR data to decadal length, two 12-year long CNRR datasets (1998–2009) have been generated by using the data assimilation method based on GSI and SN technique. This study aims to (1) comprehensively evaluate the capability of CNRR in reproducing the mean climate, seasonal cycle, extreme rainfall, daily and diurnal variability of precipitation over China, (2) compare the effects of GSI data assimilation technique and SN method in generating precipitation in CNRR. The paper is organized as follows. Section “Data and Methods” offers the details of CNRR datasets, the reference data, and validation methods. In Section “Results,” the evaluation of CNRRs in reproducing the precipitation over China and its adjacent areas is presented. A summary and discussion are given in Section “Conclusion and Discussion.”
Data and Methods
Chinese Regional Reanalysis Datasets
The Weather Research and Forecasting (WRF, version 3.8.1, Skamarock et al., 2008) model is used to generate the CNRR dataset. The CNRR domain covers mainland China and most parts of East Asia, with the domain center at 30°N, 115°E, and 481 × 361 grid points in the east-west and south-north directions at 18 km horizontal resolution (Figure 1). The model physical parameterizations configuration is same as that used in Zhang et al. (2017). Two WRF experiments are conducted to produce the CNRR datasets, one is using the GSI data assimilation technique (Shao et al., 2016) (CNRR-GSI), and the other one is using the SN method (CNRR-SN). The WRF simulations are initialized at 0000 UTC on 01 July 1997 and integrated to 0000 UTC 01 January 2010, with the first half-year as spin-up time. The 12-year period (1998–2009) is used for the evaluation of precipitation. The CNRR precipitation is at an hourly temporal scale with 18 km horizontal resolution.
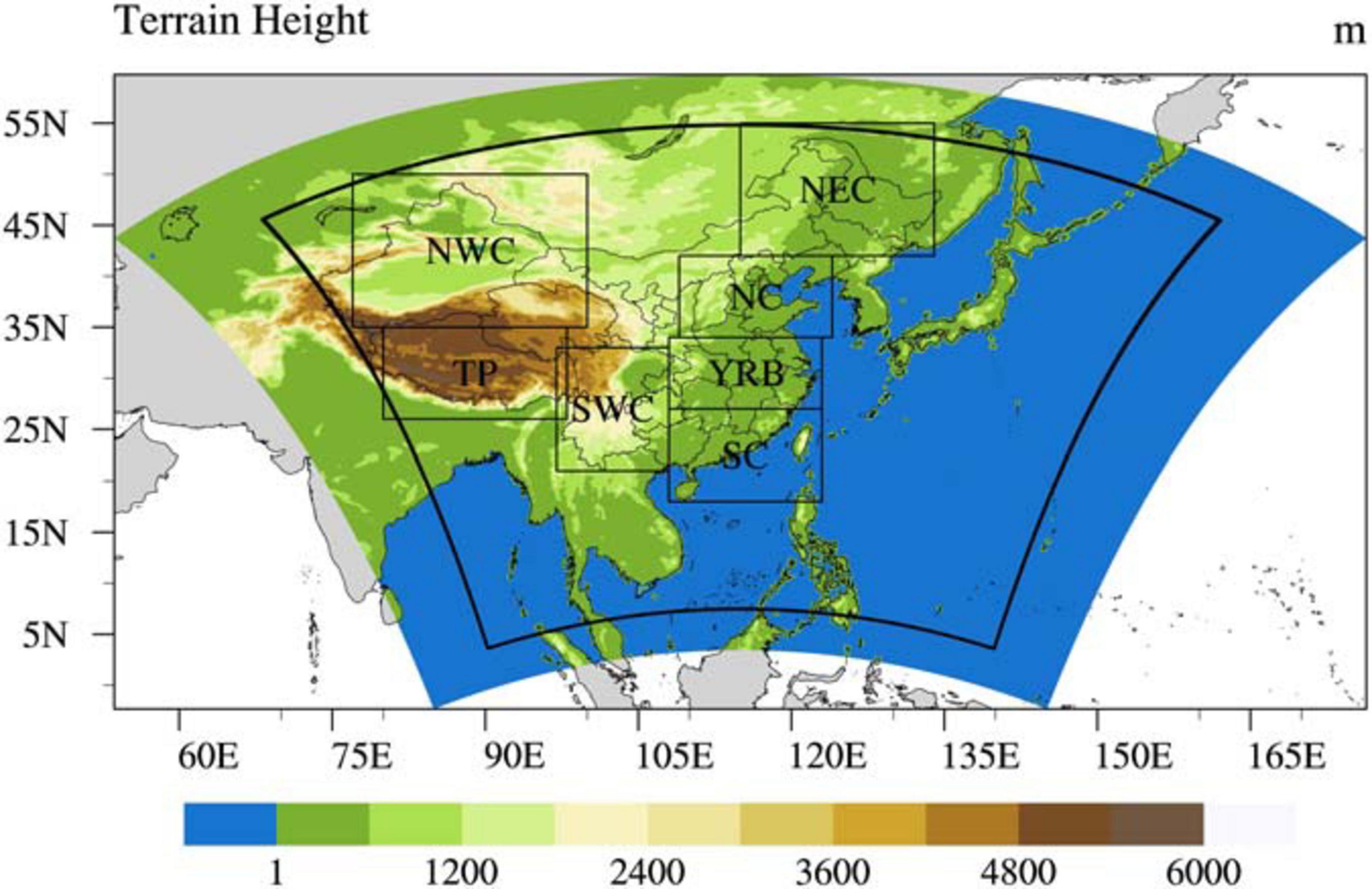
Figure 1. Simulation domain, evaluation domain and the topography of CNRR (unit: m). Sub-domains (NWC, NEC, NC, TP, YRB, SWC and SC) are marked.
Data Used for Evaluation
To assess the general capability of the CNRR in reproducing precipitation, observations including satellite-based datasets and gridded gauge-based datasets, and global reanalysis dataset are collected as references.
The satellite derived precipitation dataset used in this study is the Climate Prediction Center morphing technique (CMORPH v1.0) (Joyce et al., 2004), which is a high-resolution precipitation dataset provided by the Climate Prediction Center (CPC), National Oceanic and Atmospheric Administration (NOAA). It has been widely used to study precipitation characteristics from seasonal mean to diurnal cycle in China. The 3-hourly CMORPH with corrected bias (CMORPH-CRT) precipitation product, which has a spatial resolution of 0.25 latitude by 0.25 longitude, is used for evaluation of both spatial-temporal features, daily and diurnal variations of CNRRs.
The gridded gauge-based land precipitation over the simulation domain (named as OBS-Land) is constructed by combining the CN05.1 dataset (Wu and Gao, 2013) in China, and the daily precipitation produced by the project Asian Precipitation-Highly Resolved Observational Data Integration Towards the Evaluation of Water Resources (APHRODITE) (Yatagai et al., 2012) over the land area outside China. The CN05.1 contains gridded daily precipitation data with a resolution of 0.25 latitude by 0.25 longitude based on the interpolation of over 2400 observing stations in China. Due to the lack of in-situ observations over western China such as TP, gridded precipitation data over such regions may have potential uncertainties. The APHRODITE aims to develop state-of-the-art daily precipitation datasets on high-resolution grids covering the whole of Asia, including monsoon Asia, Russia, and the Middle East. The daily precipitation is generated from the Global Telecommunication Systems (GTS) reports, precompiled data and individual data collected by the APHRODITE project and rain gauge data provided by the National Hydrological and Meteorological Services (NHMs). The combined gridded daily precipitation product over land area (OBS-Land) has a spatial resolution of 0.25°×0.25°, and covers the period from 1998 to 2009.
Global reanalysis dataset ERA5 is released by ECMWF (Hersbach et al., 2020) since 2018, with a spatial resolution of 0.25 degree (∼31 km), covering a period of 1950-present. The reanalysis using a significantly more advanced 4D-Var assimilation technique, providing several improvements compared to its previous generation ERA-Interim.
Evaluation Methods
Since the characteristic of precipitation varies in space and time over China and its adjacent areas, 7 sub-regions are further selected for detailed evaluation (Figure 1): Northeast China (NEC), North China (NC), Northwest China (NWC), Yangtze River Basin (YRB), South China (SC), Southwest China (SWC) and Tibetan Plateau (TP). Comparison is conducted on climatological mean, seasonal cycle, daily and extreme precipitation, and diurnal variations. A threshold of 0.1 mm day–1 is chosen to separate dry and wet days. For the assessment of CNRR’s capability of simulating diurnal variation of precipitation, we calculate and standardize the annual, summer (June, July and August, JJA) and winter (December, January and February, DJF) mean of the diurnal cycle of precipitation amount during 1998–2009. The harmonic analysis is also applied on the precipitation diurnal variation, and the first harmonic is chosen to represent the diurnal cycle of precipitation (Dai and Trenberth, 2004; Koo and Hong, 2010; Mascaro, 2017). The first harmonic fits the following model:
where R(tk) is the accumulated-rainfall amount from time tk–1 to tk. For CNRR-GSI and CNRR-SN which are hourly data, tk = 0,1,2,…,23, for CMORPH whose temporal resolution is 3 hours, tk = 0,3,6,9,…,21; R(tk) is the value of rainfall amount at time tk; stands for daily mean precipitation, equals to zero here because of the standardization, Ai and φi are the amplitude and the phase of the given ith harmonic.
To quantify the performance of CNRR precipitation datasets, the root mean square error (RMSE) and the correlation coefficient between CNRR and observations are calculated in the study. The CNRR datasets are firstly interpolated to the grids of CMORPH, and OBS_Land, respectively, when calculating correlation and RMSE between them.
The equations of RMSE and correlation are:
where Mi is the value from CNRR at grid i, Oi is the value from observations at grid i, is the spatially averaged value from CNRR, is the value from observations, and n is the total number of grid points.
Considering the climatology of China, 4 extreme precipitation indices, namely consecutive dry days (CDD), consecutive wet days (CWD), very wet day precipitation (R95p), maximum 5-day precipitation amount (Rx5) established by the widely used Expert Team on Climate Change Detection and Indices (ETCCDI), are selected in this study to analyze extreme rainfall. The former two indices deal with rainfall frequency and the latter two indices deal with rainfall intensity. The definitions of the indices are given in Table 1.
Results
To detailed evaluate the precipitation characteristics in CNRR products, the mean climatology, seasonal cycle of precipitation, daily precipitation and diurnal variations of precipitation are assessed against the CMORPH, OBS_Land, and, ERA5.
Mean Climatology
Figure 2 depicts the spatial distribution of 12-year annual, summer, and winter mean precipitation of observations (CMORPH and OBS_Land), CNRRs and, ERA5 over East Asia. According to the reference data, the 12-year annual mean precipitation is distributed as decreasing from south near the equator of 10 mm/day to north around 1 mm/day, with more rainfall over the ocean than the land. Over the China mainland, precipitation decreases from the southeast to the northwest. The reanalysis datasets can well reproduce annual mean precipitation compared to the observations with spatial correlations coefficients (SCCs) all above 0.79 and RMSEs below 2.13 mm/day over the simulation domain. CNRR-GSI tends to overestimate precipitation over southern China, while CNRR-SN tends to overestimate precipitation over southwestern China. Over ocean, both CNRR-GSI and CNRR-SN well produce the main rainfall belts over Northwest Pacific compared to CMORPH, although CNRR-GSI overestimates precipitation over tropical regions. Seasonal mean precipitation has similar spatial distribution as the annual mean, and most of the precipitation occurred in summer. In summer, CNRR-GSI can simulate the spatial patterns of precipitation over most regions in East Asia, except for the significant overestimation over southern China. CNRR-SN produces wet bias over SWC and NEC. It is worth noting that both CNRR-GSI and CNRR-SN have large wet biases over TP. The summer precipitation over ocean is evidently overestimated by CNRR-SN over Bay of Bengal. For winter precipitation, CNRR-GSI and CNRR-SN produce wet bias over regions south of YRB in China.
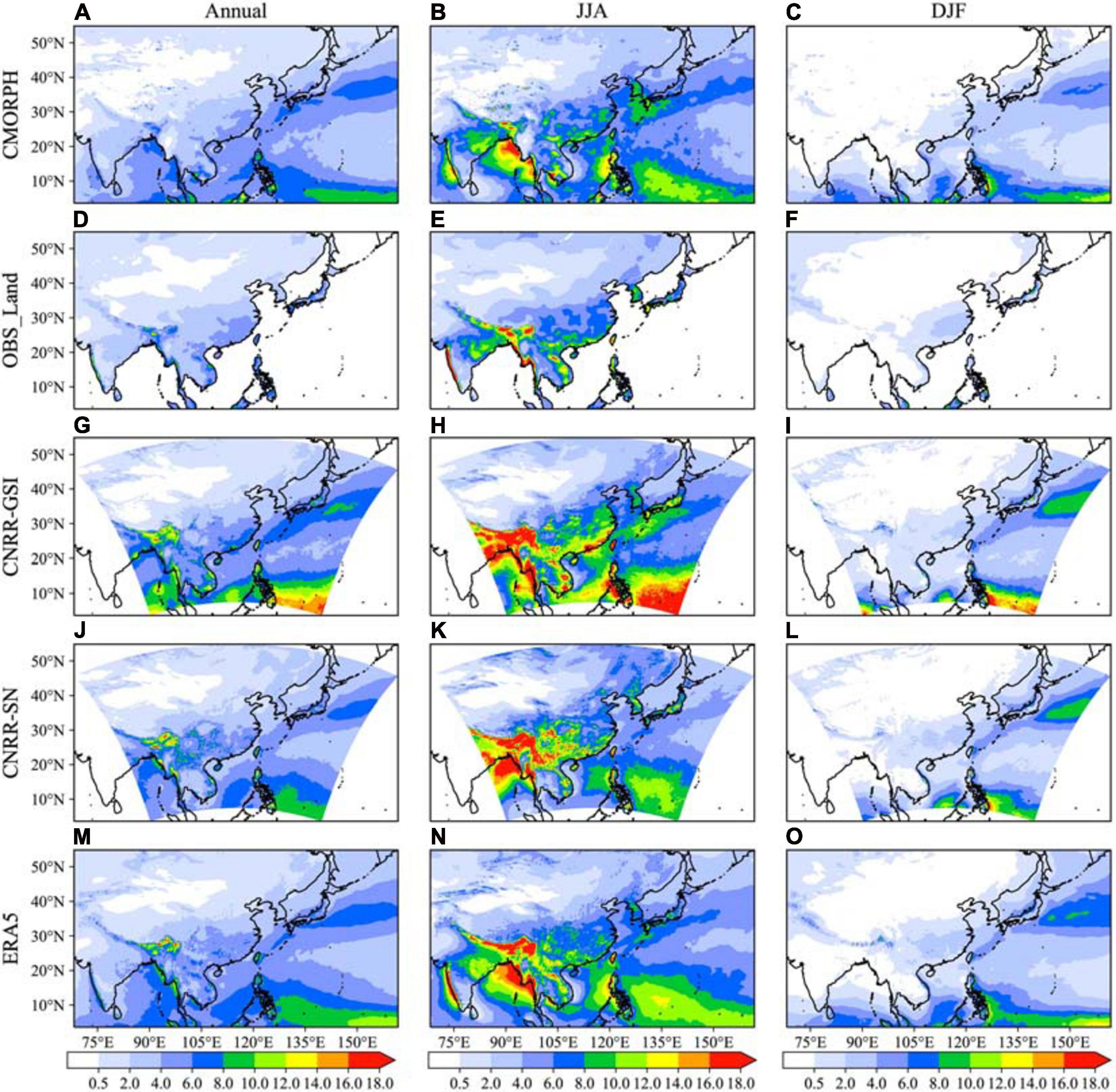
Figure 2. Distribution of the annual, summer (JJA) and winter (DJF) precipitation from observations (A–F), CNRRs (G–L) and ERA5 (M–O) (1998–2009), unit: mm/day.
To quantitively assess the CNRR precipitation products, the SCCs and RMSEs of annual, summer, and winter mean precipitation over the whole domain, ocean, and land areas between the CNRRs and observations are computed and listed in Table 2. CNRRs perform well with most of the SCCs above 0.8. Generally, CNRR-GSI results are relatively close to ERA5, and outperforms CNRR-SN in simulating the spatial distribution of climatology precipitation with higher SCCs, while it generates relative larger RMSEs over ocean. Over land area, CNRR-GSI generates better spatial distribution with higher SCCs and lower RMSEs of annual precipitation compared to the others.
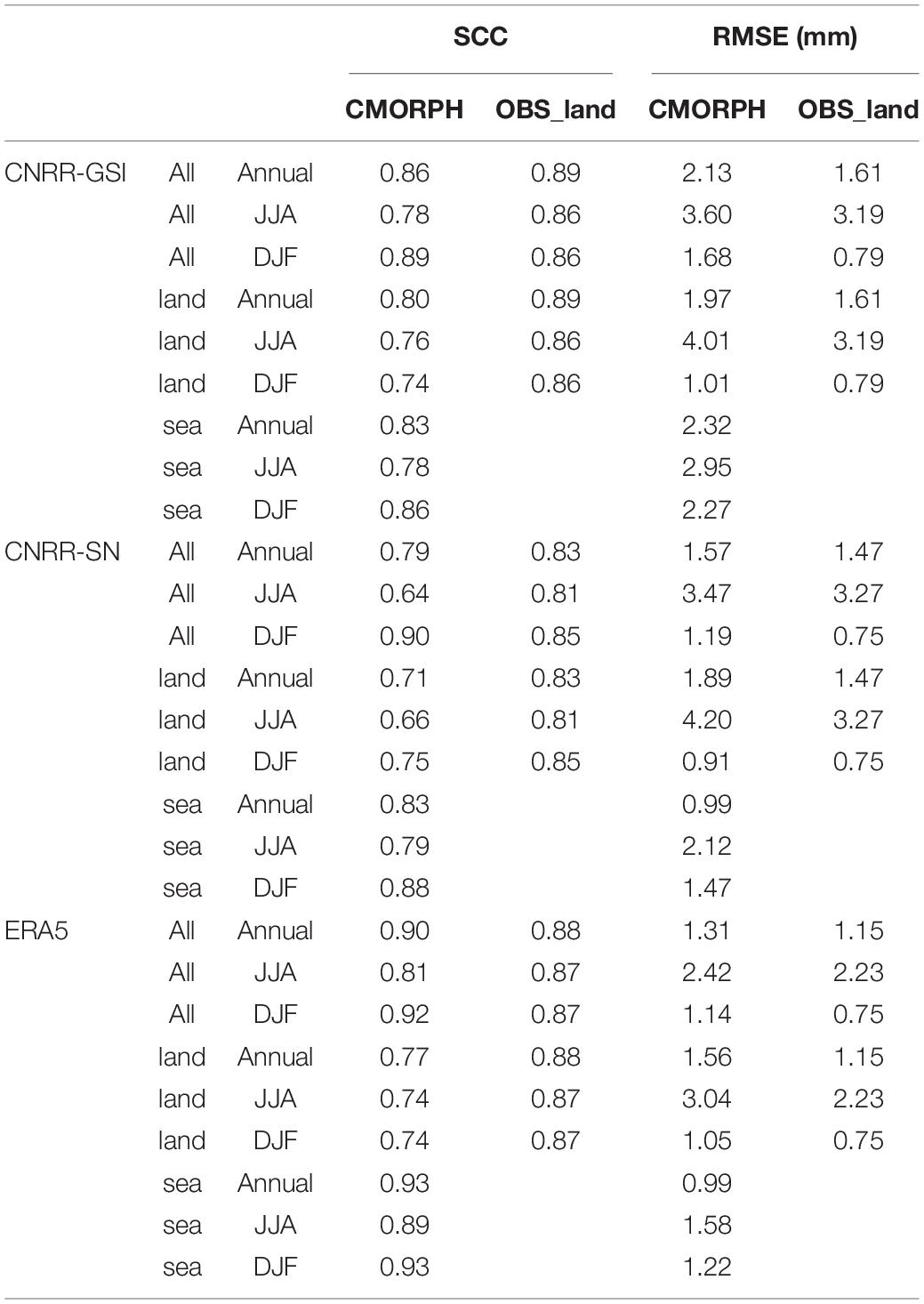
Table 2. The SCCs and RMSEs of annual, summer (JJA) and winter (DJF) precipitation between reanalyses and observations over land and ocean.
Seasonal Cycle
The seasonal cycles of regional averaged precipitation over the 7 sub-regions are displayed in Figure 3. Overall, the reanalysis datasets can generally reproduce the seasonal variations of precipitation over all sub-regions with the temporal correlation coefficients (TCCs) above 0.93, but obvious differences exist over different regions. Over the northern regions of China (NC, NEC, and NWC), CNRRs can portray the annual cycles of precipitation well with TCCs above 0.97. CNRR-SN significantly overestimates the precipitation amounts over these regions during warm season. CNRR-GSI shows better results in NEC and NWC than CNRR-SN and ERA5. ERA5 simulates better over NEC and NC than the CNRRs. For YRB region, ERA5 and CNRR-SN performs better on simulating the seasonal cycle with a TCC above 0.98, while CNRR-GSI simulates the peak of the monthly precipitation in July which is one month later than that in the observations. Over SC region, the observed precipitation seasonal cycle has two peaks in June and August, respectively. CNRRs and ERA5 can simulate the seasonal cycle with the two peaks, although wetter biases exist in CNRR-GSI in warm season. In SWC and TP regions, the peaks of the seasonal cycle are reasonably captured by the CNRRs. ERA5 and CNRR-SN yields significant larger overestimations over the two regions, reaching up to twice of the observations.
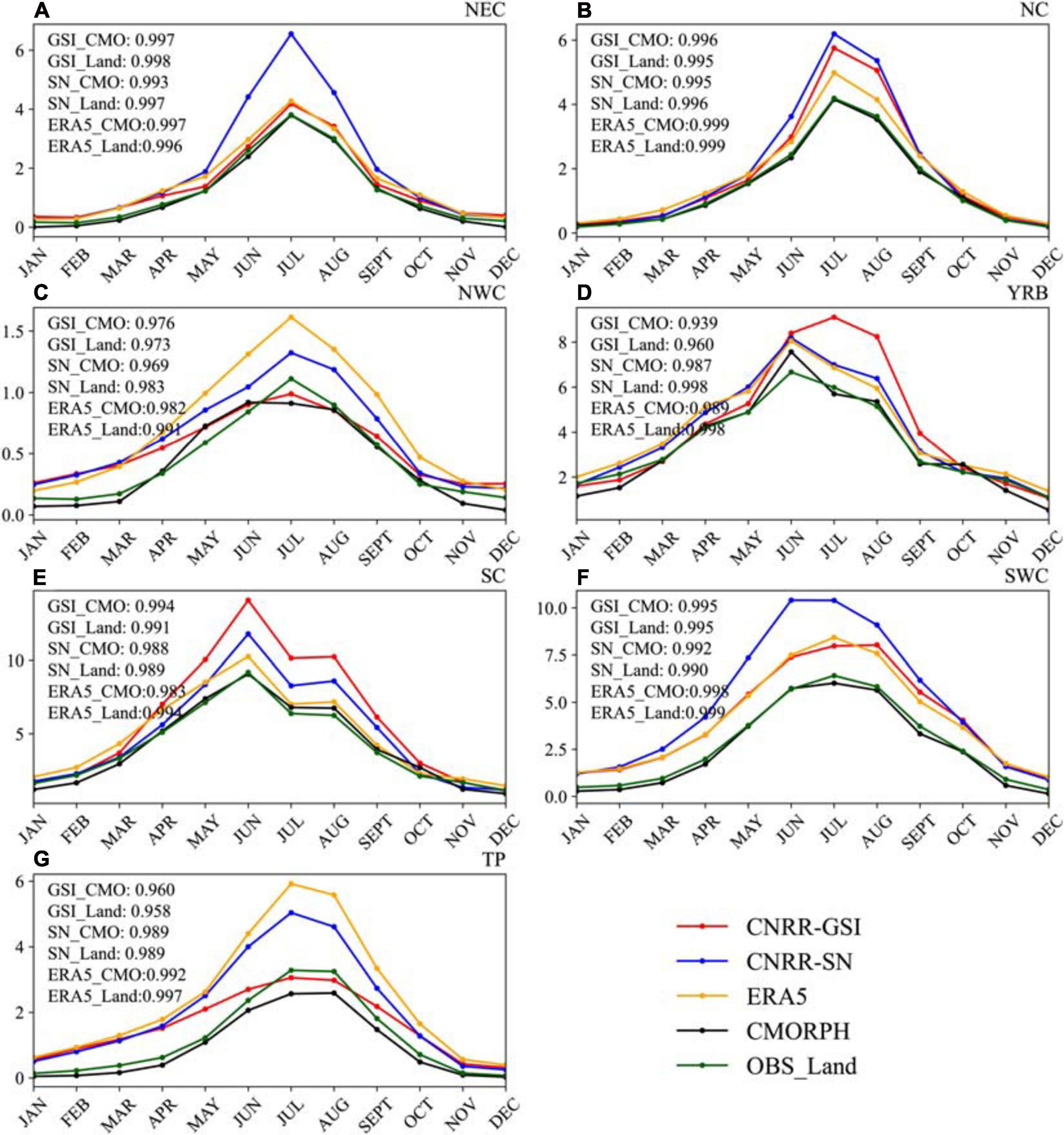
Figure 3. Monthly precipitation produced by the CNRRs, observations and ERA5 over 7 sub-domains over China. The correlation coefficients are calculated for the different sub-domains. GSI_CMO, SN_CMO and ERA5_CMO stand for the correlation between CNRRs and CMORPH. GSI_Land, SN_Land and ERA5_Land stand for the correlation between CNRRs and OBS_Land.
Figure 4 demonstrates the seasonal variations of CNRRs, EAR5 and observed meridional mean (105°E∼120°E) precipitation, which represents the evolution of monsoon precipitation. As can be seen from the references, the time of peak precipitation varies with latitude under the influence of the propagation of monsoon precipitation. The reanalyses can reasonably reproduce the propagation, but clear biases exist in CNRRs. Over regions south of 20N, both CNRR-GSI and CNRR-SN capture the peak precipitation in August and September. Over regions between 20N and 30N, the observed rainfall peaks in June, and the propagation is well captured by CNRRs, while larger wet biases can be found during warm season in CNRR-GSI compared to CNRR-SN. Over northern regions above 30N, CNRRs can portray the precipitation pattern well.
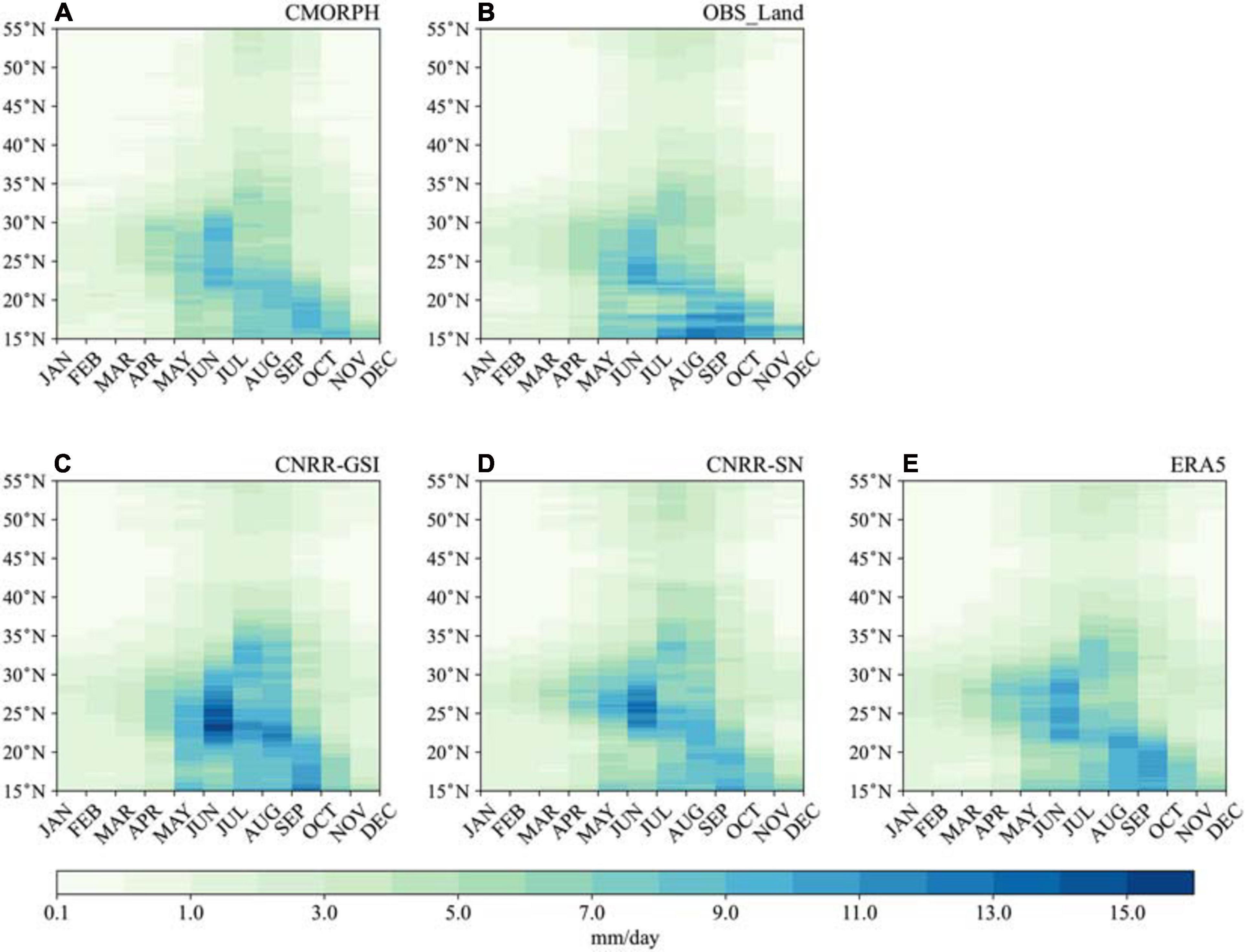
Figure 4. Hovemueller diagrams of the observations (A–B), CNRRs (C–D) and ERA5 (E) precipitation seasonal variations over Eastern China (105−−120), units: mm/day.
Daily Precipitation
An examination of the daily precipitation of CNRRs and ERA5 is conducted by comparing the wet day (defined as daily precipitation >0.1 mm/day) probability between the reanalysis and observations using several different thresholds, namely 0.1 mm∼10 mm, 10 mm∼25 mm, and 25 mm∼50 mm, representing light rainfall, moderate rainfall, and heavy rainfall, respectively. Figure 5 shows the spatial distributions of wet day probability at different precipitation intensities and the SCCs and RMSEs between CNRR, ERA5 and references are listed in Table 3. For light rainfall, ERA5 and CNRRs reproduced the patterns well over the land compared to the OBS_Land observation with the SCCs above 0.72 (Table 3). CNRRs tend to underestimate the light rainfall frequency over TP and Sichuan Province, while ERA5 reduces such bias. The reanalyses tend to generate more light rainfall events over ocean compared to CMORPH, and ERA5 produces larger biases than CNRRs. For moderate rainfall events, CNRR-GSI can well simulate the spatial pattern of precipitation probability with the SCC larger than 0.8. However, CNRR-SN clearly overestimates the probability, especially over southwestern China. Both CNRR-GSI and CNRR-SN can simulate the distribution of moderate rainfall probability over ocean, with positive biases exist compared to CMORPH, while ERA5 simulates more moderate rainfall events than CNRRs. For heavy rainfall events, CNRR-GSI reproduces the distribution of precipitation probability, and the SCCs between CNRR-GSI and observations are all above 0.75. CNRR-SN again simulates larger biases over southwestern China than CNRR-GSI. ERA5 shows similar performance to CNRR-GSI, and has better results over the land with higher SCC at 0.82. Over the ocean, the reanalyses tend to underestimate the heavy rainfall frequency, and ERA5 produces larger bias. Based on Table 3, it can be found that CNRR-GSI outperforms CNRR-SN in simulating the distribution of precipitation probability for all three precipitation intensity events, with higher SCCs and lower RMSEs. ERA5 provides better light and heavy rainfall over land than CNRR-GSI, while CNRR-GSI shows better results over the entire domain. The probability density function (PDF) of precipitation offers further details into the error structure of the CNRR precipitation datasets. The PDFs of daily precipitation for the seven sub-regions in CNRR, ERA5 and the OBS_Land are depicted in Figure 6. Only daily precipitation above 0.1 mm/day is used to generate PDFs. Precipitation probability generally decreases as the precipitation intensity increases. Differences in the PDFs exist between the sub-regions owing to the spatial variation in precipitation intensity. For instance, the southern region of China (SEC and SWC) exhibits larger probabilities of daily precipitation above 25 mm/day. In general, CNRRs and ERA5 well reproduced the observed PDFs, although overestimated probability at heavy precipitation intensities exists in CNRRs. CNRR-GSI generally captures light rainfall probability more accurately over all 7 sub-regions, while ERA5 shows obvious overestimation. Moderate rainfall events are generally overestimated by CNRR-SN and ERA5, especially over NEC, NC, and SWC. Obviously, both CNRR-GSI and CNRR-SN generate too many heavy rainfall events in NEC, NC, NWC, SWC, and TP, but comparable results for heavy rainfall events in YRB and SC. CNRR-GSI generates even more extreme rainfall events (daily precipitation >50.0 mm/day) in NC, YRB, and SC than CNRR-SN. ERA5 simulates the probability of heavy rainfall events well except over NWC. Over TP region, the light and moderate rainfall frequency is well simulated by CNRRs compared to observations, while significant overestimation exists for the frequency of rainfall daily precipitation intensity above 15 mm/day especially in CNRR-SN, and ERA5 shows similar results as CNRR-GSI.
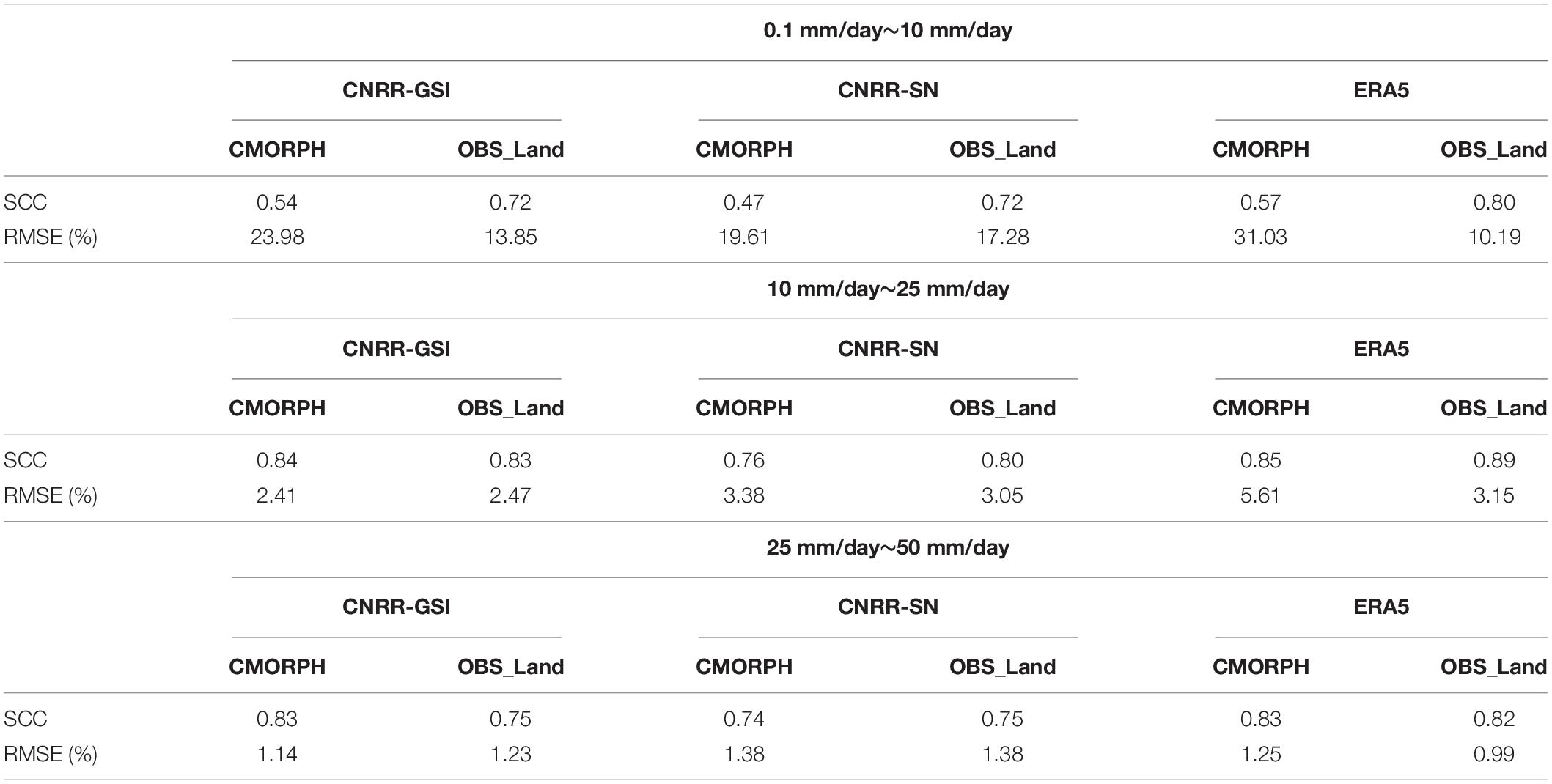
Table 3. The SCCs and RMSEs of wet day probability for the three rainfall bands between CNRRs, ERA5 and observations.
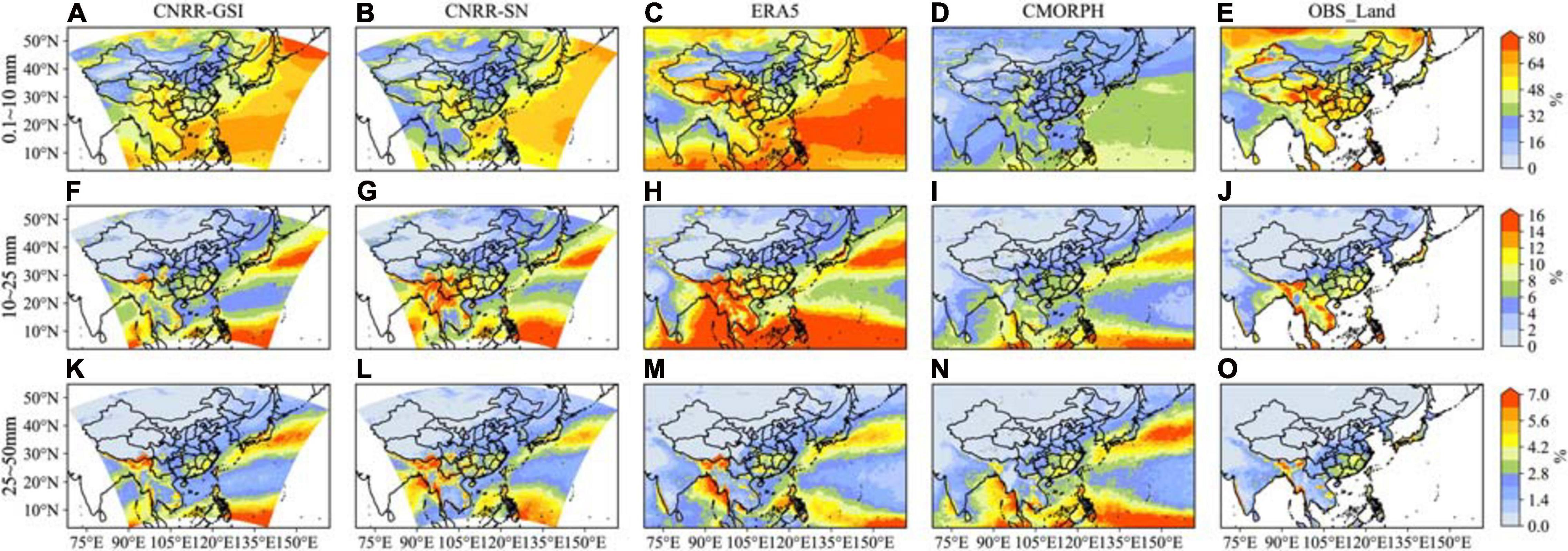
Figure 5. Distribution of the wet day probability from CNRRs, observations and ERA5 (1998–2009) of rainfall between 0.1–10 mm/day (A–E), 10–25 mm/day (F–J) and 25–50 mm/day (K–O), unit: %.
To further assess the CNRRs’ capability of producing the extreme precipitation events, the spatial distributions of 4 extreme precipitation indices (CDD, CWD, Rx5, R95p) from ETCCDI over land area are calculated and shown in Figure 7. According to OBS_Land, large CDDs above 100 days are mainly located at northwestern China. Although ERA5 and CNRRs can well simulate the distribution of CDD, they tend to overestimate CDD over Xinjiang province especially in CNRR-SN experiment. Both ERA5 and CNRRs reproduce the large CWD over southwestern China, and CNRR-GSI can also simulate the spatial pattern of CWD over the Indochina Peninsula where underestimation (overestimation) clearly exits in CNRR-SN (ERA5). For the extreme precipitation intensity indices (Rx5 and R95p), the spatial distributions can be well simulated with the SCCs above 0.93 in CNRR experiments, while overestimation clearly exists in southern China especially in CNRR-GSI experiment. Generally, CNRRs and ERA5 reasonably capture the observed distributions of extreme precipitation indices. CNRR-GSI better simulates the patterns of CDD and CWD with higher SCCs and lower RMSEs, while it has larger RMSEs for Rx5 and R95p (as shown in Table 4).
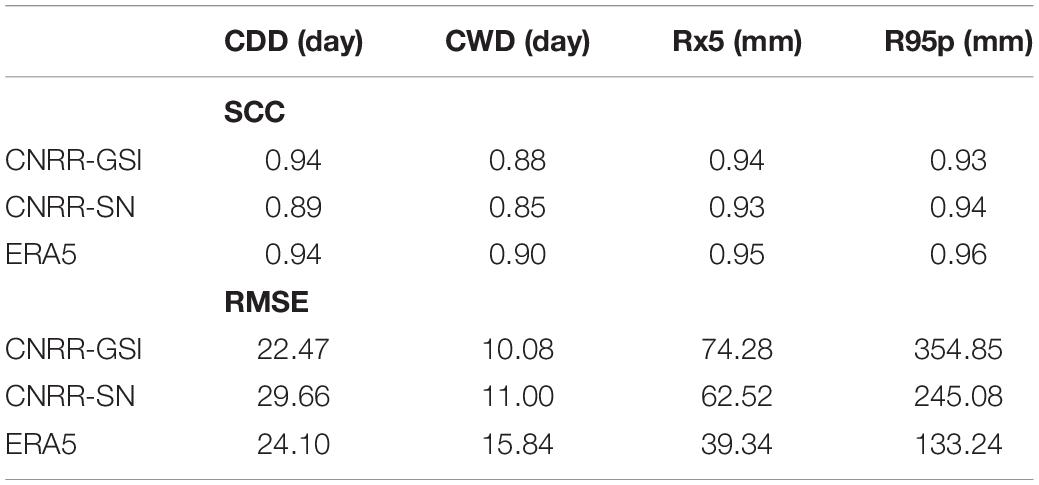
Table 4. The SCCs and RMSEs of the extreme precipitation indices (CDD, CWD, Rx5 and R95p) between CNRRs, ERA5 and OBS_Land.
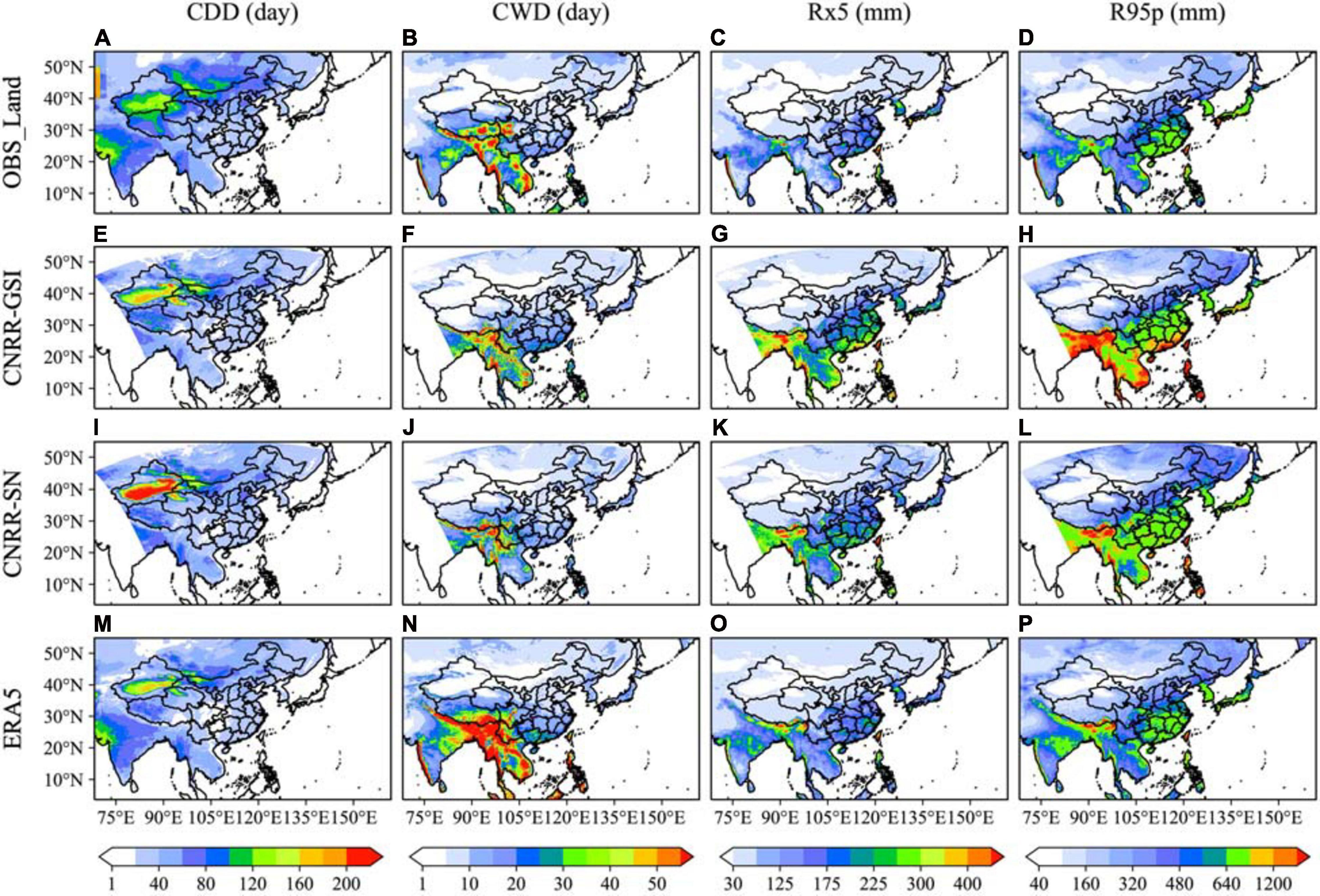
Figure 7. Spatial distribution of the extreme rainfall indices (CDD, CWD, Rx5 and R95p) produced by OBS-Land (A–D), CNRR-GSI (E–H), CNRR-SN (I–L) and ERA5 (M–P) over land.
Diurnal Variation
The diurnal cycle of precipitation is an important aspect of regional climate. Evaluation of the diurnal variation of precipitation is helpful to further assess the ability of CNRR in producing the spatiotemporal characteristics of precipitation. In this study, only the diurnal variation of summer precipitation is discussed. Local Standard Time (LST) is used to represent the diurnal cycle.
The CMORPH satellite derived precipitation which represents 3 hourly rain rate on the 0.25°× 0.25° latitude−longitude grids is used as observation. The harmonic analysis is applied to characterize the diurnal cycle (24-h period) of precipitation, the first harmonic component is used to calculate the amplitudes and phases of diurnal variations. Figure 8 shows the distribution of the phase of the diurnal cycle of precipitation amount (PA) and frequency (PF), reflecting the occurring time of daily maximum PA and PF, respectively. From the observation, precipitation mainly occurs in the afternoon (from 1500 to 1800 LST) over most land areas except for southwestern China where rainfall usually occurs at night to early morning. An eastward propagation of peak time of PA can be observed starting from TP to YRB (marked in Figure 8A). The spatial pattern of the peak time of PA over land area produced by CNRR-GSI is consistent with that of CMORPH, reflecting the spatial evolution of the diurnal phase of rainfall, although a bias of 1∼2 h exists in certain areas. The eastward propagation of peak time of PA is best reproduced in CNRR-GSI with a 2-h delay over TP. CNRR-SN can simulate the spatial distribution of the peak time of PA with the occurrence time about 2 h earlier over most regions in China and about 4 h earlier over Indochina Peninsula compared to observation. Also, it fails to simulate the eastward propagation of the diurnal phase. ERA5 captures the spatial distribution, and well reproduced the phase over TP and south China. Both CNRR and ERA5 can reproduce the peak time of diurnal variation of PA over ocean with the maximum precipitation occurs in daytime, and CNRR-GSI better simulates the pattern of peak time over Bay of Bengal. The distribution of the phase of the diurnal cycle of PF is quite similar to that of PA. CNRR-GSI can capture the characteristic of maximum precipitation frequency over most regions over land with the occurrence time about 2 h earlier than observation over NEC, Inner Mongolia, and TP. CNRR-SN shows similar pattern to ERA5, and CNRR-SN and ERA5 both beat CNRR-GSI in reproducing the phase of the diurnal cycle of PF over NE and Inner Mongolia, but they simulate earlier peak time of PF over most land areas especially over SC and Indochina Peninsula, and do not reproduce the peak time of frequency at night over southwestern China. Both CNRRs and ERA5 can simulate the maximum precipitation frequency in morning over most offshore areas (East China Sea, Yellow Sea, etc.), and CNRR-GSI performs slightly better results than CNRR-SN over South China Sea and Bay of Bengal.
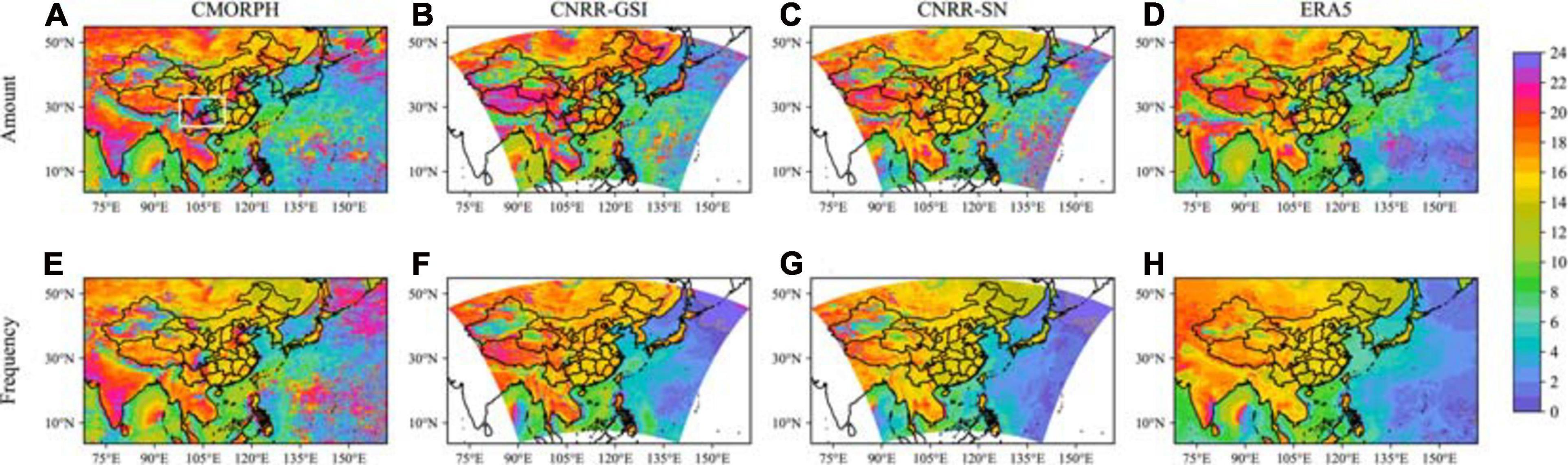
Figure 8. Distribution of the diurnal harmonic phase of precipitation amount (PA) (A–D) and precipitation frequency (PF) (E–H) from CNRRs, ERA5 and CMORPH. Local Standard Time (LST) is used to represent the diurnal cycle. The white-line square in panel (A) marks the location of eastward propagation of PA phase from TP.
Figure 9 depicts the distribution of the normalized amplitudes of diurnal harmonic of PA from CMORPH, CNRRs and ERA5. The amplitude is normalized by dividing them by the daily mean precipitation. From the CMORPH (Figure 9A), the maximum normalized amplitude of PA mainly locates over the TP and along the coastal regions in southern China and Indochina Peninsula. CNRR-GSI can reproduce the distribution of the diurnal harmonic of precipitation amplitude, with the strongest diurnal rainfall amplitudes over TP and along the coastal regions in SC and Indochina Peninsula. And the SCC between CNRR-GSI and CMORPH is the highest, reaching 0.89. ERA5 captures the spatial pattern of PA amplitude well, while it underestimates the amplitude over TP and south east of China. Although CNRR-SN can simulate the spatial pattern of precipitation amplitude with the SCC at 0.88, it clearly overestimates the normalized amplitude over southern China, Indochina Peninsula, northern and northwestern China.
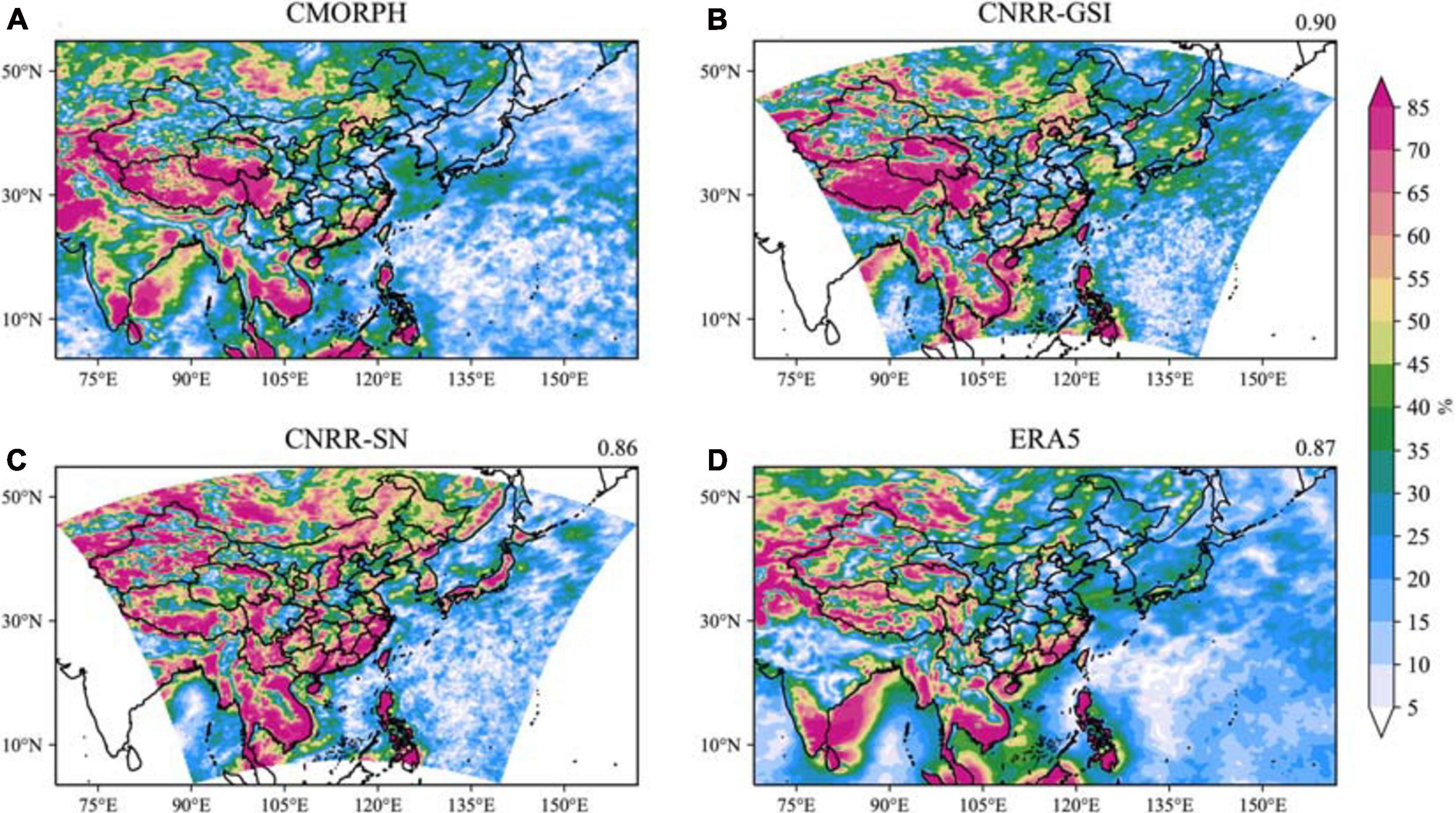
Figure 9. Distribution of the normalized diurnal harmonic amplitude of precipitation amount (PA) from CMORPH (A), CNRR-GSI (B), CNRR-SN (C) and ERA5 (D). The amplitude is normalized by dividing daily average precipitation. Unit: %.
To further reveal the regional features of diurnal cycles of PA and PF in China, Figure 10 compares the normalized diurnal harmonic cycle of summer PA and PF from the CNRRs, ERA5 and CMORPH over the 7 sub-regions. Obvious diurnal variations in precipitation can be observed in all 7 sub-regions in China. Most regions (NEC, NWC, YRB, SC) show that both PA and PF peak in the afternoon between 1500 LST and 1700 LST, consistent with previous studies (Yu et al., 2007; Zhou et al., 2008; Chen et al., 2018). PA in TP and SWC peaks during midnight. NEC, NWC, SC, SWC, and TP regions exhibited larger diurnal variations of PA and PF. CNRR-GSI simulates the diurnal cycle of PA much closer to the observation especially in NC and SWC with correlation coefficients exceed 0.89, although a 2 h bias exists in regions such as NEC and NWC. ERA5 simulates the diurnal cycle of PA quite close to CMORPH with the TCC up to near 1.0 over NEC and SC, but its performance over different regions is unstable, with 2∼9 h bias existing and TCC below 0.5 over SWC and TP, and the TCC over NC reaches −0.28. CNRR-SN produces identical diurnal variations of PA among all 7 sub-regions with significant overestimation of afternoon PA and underestimates the early morning PA in SWC and TP regions. In terms of PF, the peak frequency occurs during the afternoon (14:00 to 17:00 LST) in all 7 sub-regions. Both CNRRs and ERA5 well simulate the diurnal variations of PF in which CNRR-SN significantly overestimates the magnitude of diurnal variation of PF. CNRR-GSI outperforms CNRR-SN and ERA5 with higher TCCs, although a 1–2 h delay exists over most regions of China.
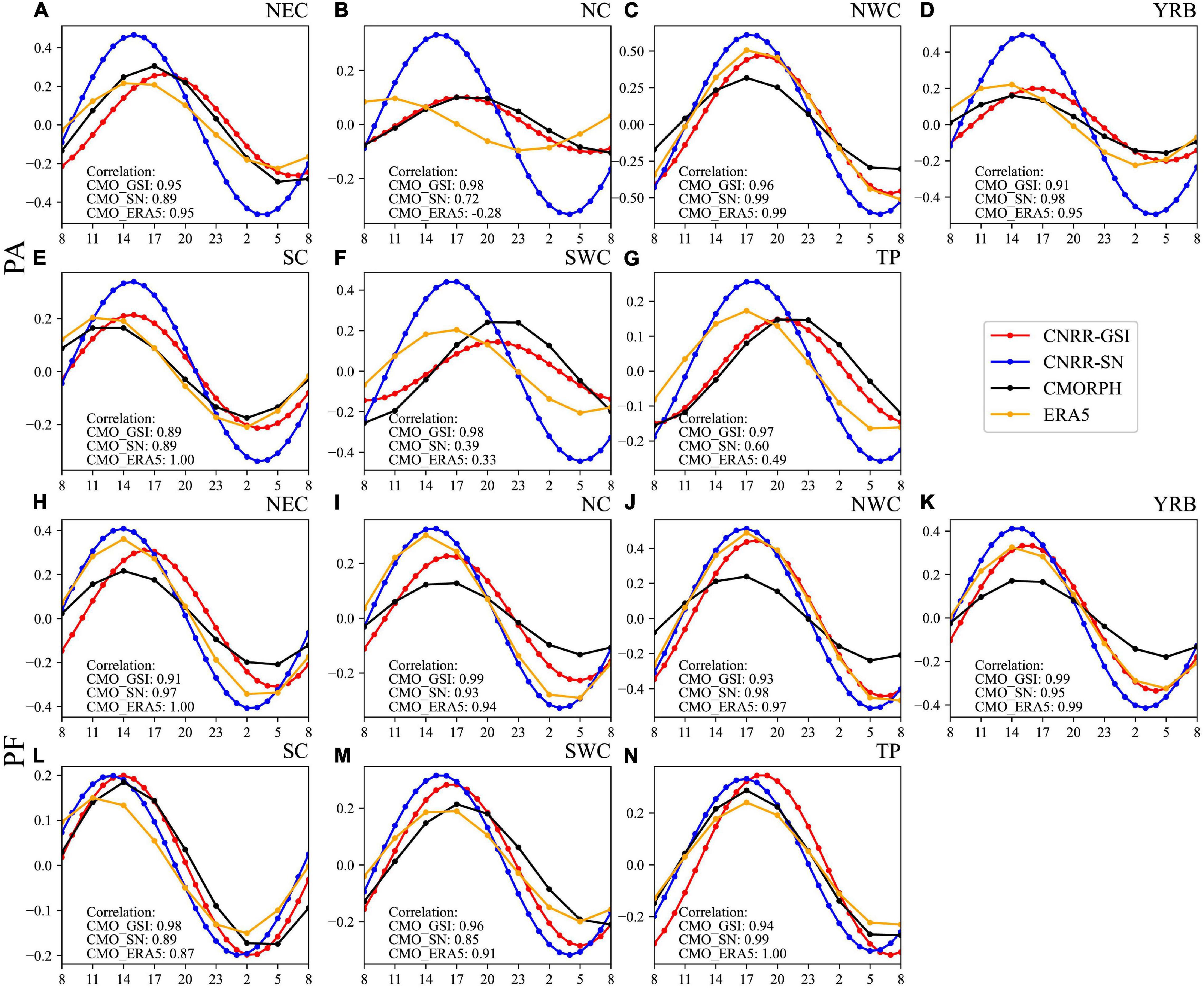
Figure 10. The normalized diurnal harmonic cycle of PA (A–G) and PF (H–N) from CNRRs, ERA5, and CMORPH.
Conclusion and Discussion
Two Chinese regional reanalysis datasets, namely the CNRR-GSI and CNRR-SN, are generated at a horizontal resolution of 18 km from 1998 to 2012 utilizing data assimilation technique and SN method, respectively. The satellite data based global precipitation data CMORPH and the gauge-based gridded land precipitation over the simulation domain (OBS_Land) constructed using CN05.1 and APHRODITE, and the global reanalysis dataset ERA5 are used to comprehensively evaluate the skills of CNRRs in reproducing the key features of the climatology, seasonal, daily, and diurnal precipitation and extreme rainfall over East Asia.
Both CNRR-GSI and CNRR-SN are reliable in depicting the spatial distribution of precipitation in a climatological view, but overestimation of warm season rainfall especially over the south of TP exists. In general, CNRR-GSI generates better spatial distribution with higher SCCs but more rainfall with higher RMSEs than CNRR-SN and ERA5 over land. In terms of the seasonal cycle, CNRRs can reproduce the seasonal variations of precipitation over China as well as the evolution of monsoon precipitation, and generates the results close to ERA5. CNRR-GSI outperforms CNRR-SN in reproducing summer rainfall in most regions of China except YRB and SC.
Analysis of the daily precipitation reveals that CNRRs are capable of reproducing the distribution of precipitation occurrence at different precipitation intensities. CNRRs slightly underestimate the light rainfall probability and overestimate heavy rainfall probability over the simulation domain. For different regions in China, CNRRs overestimate heavy rainfall probability, especially over TP and NWC. CNRR-GSI outperforms CNRR-SN in reproducing precipitation probability at different intensities over most regions except that CNRR-GSI produces more heavy and extreme rainfall over YRB and SC. The probability of moderate and heavy rainfall can be well captured by ERA5, while CNRRs simulate the light rainfall occurrence and underestimate the extreme rainfall frequency better than ERA5. CNRRs also reasonably capture the observed distributions of extreme indices, and CNRR-GSI best simulates the patterns of CDD and CWD with higher SCCs and lower RMSEs compared to CNRR-SN and ERA5, while it has larger RMSEs for Rx5 and R95p.
Comparison of peak time of summer PA and PF in China between CMORPH, CNRRs and ERA5 reveals that CNRR-GSI outperforms CNRR-SN and ERA5 in capturing the regional characteristic of the diurnal cycle of precipitation, especially over eastern China. The eastward propagation of peak time PA starting from the TP and along the Yangtze River is well reproduced in CNRR-GSI but failed in CNRR-SN, regardless of the slight shifts of diurnal phases around 1–2 hours. CNRR-GSI and ERA5 also reasonably captures the amplitude of the diurnal cycle while significant overestimations exist in CNRR-SN over most regions in the simulation domain. Diurnal variations of PA and PF in different regions in China are better revealed by CNRR-GSI with higher SCCs and lower RMSEs.
With the refinement of the dynamical and physical processes in regional numerical weather models and the application of 3D-Var data assimilation and SN techniques, the CNRR datasets can provide reliable precipitation products. CNRR-GSI generally outperforms the CNRR-SN in simulating the characteristics of precipitation over China, shows comparable results with the latest ERA5 and outperforms it in simulating the diurnal cycle of precipitation. Overestimations of heavy and extreme rainfall are observed over YRB, SC, and south of TP in CNRR-GSI. Special care should be taken when using CNRR datasets to study the regional climate over TP as well as in the case of extreme rainfall. With the increasing computing resources, optimized assimilation techniques (e.g., 4D-Var, ensemble Kalman filter, ensemble transform Kalman filter, etc.), and the selection of observations, CNRR could be further improved to provide more reliable precipitation products. Thus, there is a great potential for the application of the CNRR products in precipitation related researches and applications in East Asia.
Authors Biographies
YL is a second-year postgraduate student from School of Atmospheric Sciences at Nanjing University, Nanjing, China. Her major field of study is regional climate change and simulation.
She studied and earned her BA degree from School of Atmospheric Sciences at Nanjing University. By far, she has had interest in the data assimilation methods and evaluation of regional reanalysis data.
Miss Lu is a member of Key Laboratory of Mesoscale Severe Weather/Ministry of Education, Nanjing University. Her e-mail address is mg1928018@smail.nju.edu.cn
MS earned his Ph.D. degree from George Mason University from College of Science, Geography and Geoinformation Science Department, United States in 2017, mainly focusing on data assimilation and remote sensing.
He works as Associate Professor in School of Environment, Nanjing Normal University. From 2017.6 to 2018.4 he worked as Research Associate in School of the Environment, Nanjing University and as Postdoc at Department of Atmospheric and Planetary Science, Hampton University/NASA LaRC. He is now working on Atmospheric and air quality modeling.
Dr. Shao can be contacted by e-mail mshao@masonlive.gmu.edu
JF earned her Ph.D., Meteorology from School of Atmospheric Sciences, Nanjing University, Nanjing China in 2000 by studying geostrophic adjustment frontogenesis.
She works as Professor at School of Atmospheric Sciences in Nanjing University, China. She has devoted to studying tropical cyclone dynamics.
Prof Fang is awarded Excellent Scientific and Technological Fellow in Meteorology by Chinese Meteorological Society, the First Prize of the Advancement of Science and Technology by Ministry of Education of the People’s Republic of China, the Prize of the excellent PH. D. Thesis by Jiangsu Education Commission and “Tu Changwang” Young Meteorological Investigator Award by Chinese Meteorological Society. Prof Fang’s e-mail address is fangjuan@nju.edu.cn
YP earned his Ph.D., Meteorology from School of Atmospheric Sciences, Nanjing University, Nanjing, China in 2003.
He works as Associate Professor in School of Atmospheric Sciences, Nanjing University, Nanjing, China currently, teaching Principle of Synoptic Meteorology. His research interest focuses on mesoscale synoptic analysis and numerical simulation.
Prof Pan is awarded Outstanding Individual of Employment Guidance by Nanjing University in 2004, the Second Prize of the Advancement of Science and Technology, Province Jiangsu in 1999 and is nominated the First Prize of National Award for Natural Sciences. Prof Pan’s e-mail address is pyn@nju.edu.cn
JT earned his Ph.D. in Meteorology from School of Atmospheric Sciences, Nanjing University, Nanjing, China in 2004. His major field is regional climate modeling.
He works as Professor in School of Atmospheric Sciences, Nanjing University, Nanjing, China from 2016 currently. He is devoted to studying regional climate modeling, extremes, land-atmosphere interaction and diurnal cycle of precipitation and convective systems. His teaching interest focuses on Statistical Weather Forecasting and Statistical methods in meteorological big data analysis.
Prof Tang is a member of Key Laboratory of Mesoscale Severe Weather/Ministry of Education, Nanjing University. His e-mail address is jptang@nju.edu.cn
Data Availability Statement
The raw data supporting the conclusions of this article will be made available by the authors, without undue reservation.
Author Contributions
YL conceived the work, analyzed the results, and wrote the manuscript. MS and JT made contribution to the conception and design the study, reviewed and edited the manuscript. JF and YP read and approved the manuscript. All authors contributed to the article and approved the submitted version.
Funding
This work is jointly supported by the National Key Research and Development Program of China (2018YFA0606003) and the National Natural Science Foundation of China (41875124).
Conflict of Interest
The authors declare that the research was conducted in the absence of any commercial or financial relationships that could be construed as a potential conflict of interest.
Acknowledgments
The authors would like to express their gratitude to the following data centers: the National Climatic Data Center (NCDC) of National Oceanic and Atmospheric Administration (NOAA) for providing satellite observation CMORPH (https://www.ncdc.noaa.gov/cdr/atmospheric/precipitation-cmorph) and the NCAR/UCAR Climate Data Guide for providing APHRODITE (https://climatedataguide.ucar.edu/climate-data/aphrodite-asian-precipitation-highly-resolved-observational-data-integration-towards). The authors would also thank Dr. Jia Wu from the National Climate Center (NCC) of China Meteorological Administration (CMA) for providing the CN05.1 gridded dataset. All data and assimilation outputs used in this study can be accessed by contacting JT (anB0YW5nQG5qdS5lZHUuY24=).
References
Becker, E. J., Berbery, E. H., and Higgins, R. W. (2009). Understanding the characteristics of daily precipitation over the United States using the North American Regional Reanalysis. J. Clim. 22, 6268–6286. doi: 10.1175/2009JCLI2838.1
Bromwich, D. H., Wilson, A. B., Bai, L. S., Moore, G. W., and Bauer, P. (2016). A comparison of the regional Arctic System Reanalysis and the global ERA-Interim Reanalysis for the Arctic. Q. J. R. Meteorol. Soc. 142, 644–658. doi: 10.1002/qj.2527
Bollmeyer, C., Keller, J. D., Ohlwein, C., Wahl, S., Crewell, S., Friederichs, P., et al. (2015). Towards a high-resolution regional reanalysis for the European CORDEX domain. Q. J. R. Meteorol. Soc. 141, 1–15. doi: 10.1002/qj.2486
Chen, G., Lan, R., Zeng, W., Pan, H., and Li, W. (2018). Diurnal variations of rainfall in surface and satellite observations at the monsoon coast (South China). J. Clim. 31, 1703–1724. doi: 10.1175/JCLI-D-17-0373.1
Chen, S., Gan, T. Y., Tan, X., Shao, D., and Zhu, J. (2019). Assessment of CFSR, ERA-Interim, JRA-55, MERRA-2, NCEP-2 reanalysis data for drought analysis over China. Clim. Dyn. 53, 737–757. doi: 10.1007/s00382-018-04611-1
Dahlgren, P., Landelius, T., Kållberg, P., and Gollvik, S. (2016). A high-resolution regional reanalysis for Europe. Part 1: three-dimensional reanalysis with the regional HIgh-Resolution Limited-Area Model (HIRLAM). Q. J. R. Meteorol. Soc. 142, 2119–2131. doi: 10.1002/qj.2807
Dai, A., and Trenberth, K. E. (2004). The diurnal cycle and its depiction in the Community Climate System Model. J. Clim. 17, 930–951.
Dee, D. P., Uppala, S. M., Simmons, A., Berrisford, P., Poli, P., Kobayashi, S., et al. (2011). The ERA-Interim reanalysis: configuration and performance of the data assimilation system. Q. J. R. Meteorol. Soc. 137, 553–597. doi: 10.1002/qj.828
Delhasse, A., Kittel, C., Amory, C., Hofer, S., van As, D., Fausto, R. S., et al. (2020). Brief communication: evaluation of the near-surface climate in ERA5 over the Greenland Ice Sheet. Cryosphere 14, 957–965.
Dominguez, F., Kumar, P., and Vivoni, E. R. (2008). Precipitation recycling variability and ecoclimatological stability–A study using NARR data. Part II: North American monsoon region. J. Clim. 21, 5187–5203. doi: 10.1175/2008JCLI1760.1
Dullaart, J. C., Muis, S., Bloemendaal, N., and Aerts, J. C. (2020). Advancing global storm surge modelling using the new ERA5 climate reanalysis. Clim. Dyn. 54, 1007–1021.
Gleixner, S., Demissie, T., and Diro, G. T. (2020). Did ERA5 improve temperature and precipitation reanalysis over East Africa? Atmosphere 11:996.
Hawcroft, M., Dacre, H., Forbes, R., Hodges, K., Shaffrey, L., and Stein, T. (2017). Using satellite and reanalysis data to evaluate the representation of latent heating in extratropical cyclones in a climate model. Clim. Dyn. 48, 2255–2278. doi: 10.1007/s00382-016-3204-6
He, J., Zhang, F., Chen, X., Bao, X., Chen, D., Kim, H. M., et al. (2019). Development and evaluation of an ensemble-based data assimilation system for regional reanalysis over the Tibetan Plateau and surrounding regions. J. Adv. Model. Earth Syst. 11, 2503–2522.
Hersbach, H., Bell, B., Berrisford, P., Hirahara, S., Horányi, A., Muñoz-Sabater, J., et al. (2020). The ERA5 global reanalysis. Q. J. R. Meteorol. Soc. 146, 1999–2049.
Jermey, P., and Renshaw, R. (2016). Precipitation representation over a two-year period in regional reanalysis. Q.J. R. Meteorol. Soc. 142, 1300–1310. doi: 10.1002/qj.2733
Joyce, R. J., Janowiak, J. E., Arkin, P. A., and Xie, P. (2004). CMORPH: a method that produces global precipitation estimates from passive microwave and infrared data at high spatial and temporal resolution. J. Hydrometeorol. 5, 487–503.
Kalnay, E., Kanamitsu, M., Kistler, R., Collins, W., Deaven, D., Gandin, L., et al. (1996). The NCEP/NCAR 40-year reanalysis project. Bull. Am. Meteorol. Soc. 77, 437–472.
Kanamaru, H., and Kanamitsu, M. (2007). Fifty-seven-year California reanalysis downscaling at 10 km (CaRD10). Part II: Comparison with North American regional reanalysis. J. Clim. 20, 5572–5592. doi: 10.1175/2007jcli1522.1
Keune, J., and Miralles, D. G. (2019). A precipitation recycling network to assess freshwater vulnerability: challenging the watershed convention. Water Resour. Res. 55, 9947–9961. doi: 10.1029/2019WR025310
Kobayashi, S., Ota, Y., Harada, Y., Ebita, A., Moriya, M., Onoda, H., et al. (2015). The JRA-55 reanalysis: General specifications and basic characteristics. J. Meteorol. Soc. Jpn. Ser. II 93, 5–48. doi: 10.2151/jmsj.2015-001
Koo, M. S., and Hong, S. Y. (2010). Diurnal variations of simulated precipitation over East Asia in two regional climate models. J. Geophys. Res. Atmos. 115:D05105. doi: 10.1029/2009JD012574
Kunkel, K. E. (2003). North American trends in extreme precipitation. Nat. Hazards 29, 291–305. doi: 10.1023/A:1023694115864
Lei, Y., Yao, T., Yang, K., Sheng, Y., Kleinherenbrink, M., Yi, S., et al. (2017). Lake seasonality across the Tibetan Plateau and their varying relationship with regional mass changes and local hydrology. Geophys. Res. Lett. 44, 892–900. doi: 10.1002/2016GL072062
Li, Z., Liu, W.-Z., Zhang, X.-C., and Zheng, F.-L. (2011). Assessing the site-specific impacts of climate change on hydrology, soil erosion and crop yields in the Loess Plateau of China. Clim. Change 105, 223–242. doi: 10.1007/s10584-010-9875-9
Llovel, W., Purkey, S., Meyssignac, B., Blazquez, A., Kolodziejczyk, N., and Bamber, J. (2019). Global ocean freshening, ocean mass increase and global mean sea level rise over 2005–2015. Sci. Rep. 9, 1–10. doi: 10.1038/s41598-019-54239-2
Mahmood, S., Davie, J., Jermey, P., Renshaw, R., George, J. P., Rajagopal, E., et al. (2018). Indian monsoon data assimilation and analysis regional reanalysis: configuration and performance. Atmos. Sci. Lett. 19:e808. doi: 10.1002/asl.808
Mascaro, G. (2017). Multiscale spatial and temporal statistical properties of rainfall in central Arizona. J. Hydrometeorol. 18, 227–245. doi: 10.1175/JHM-D-16-0167.1
Maurer, E. P., O’Donnell, G. M., Lettenmaier, D. P., and Roads, J. O. (2001). Evaluation of the land surface water budget in NCEP/NCAR and NCEP/DOE reanalyses using an off-line hydrologic model. J. Geophys. Res. Atmos. 106, 17841–17862. doi: 10.1029/2000JD900828
Mesinger, F., DiMego, G., Kalnay, E., Mitchell, K., Shafran, P. C., Ebisuzaki, W., et al. (2006). North American regional reanalysis. Bull. Am. Meteorol. Soc. 87, 343–360. doi: 10.1175/bams-87-3-343
Nogueira, M. (2020). Inter-comparison of ERA-5, ERA-interim and GPCP rainfall over the last 40 years: process-based analysis of systematic and random differences. J. Hydrol. 583:124632.
Olauson, J., and Bergkvist, M. (2015). Modelling the Swedish wind power production using MERRA reanalysis data. Renew. Energy 76, 717–725. doi: 10.1016/j.renene.2014.11.085
Orth, R., Zscheischler, J., and Seneviratne, S. (2016). Record dry summer in 2015 challenges precipitation projections in Central Europe. Sci. Rep. 6:28334. doi: 10.1038/srep28334
Powell, J., and Reinhard, S. (2016). Measuring the effects of extreme weather events on yields. Weather Clim. Extrem. 12, 69–79. doi: 10.1016/j.wace.2016.02.003
Rienecker, M. M., Suarez, M. J., Gelaro, R., Todling, R., Bacmeister, J., Liu, E., et al. (2011). MERRA: NASA’s modern-era retrospective analysis for research and applications. J. Clim. 24, 3624–3648. doi: 10.1175/JCLI-D-11-00015.1
Risi, C., Bony, S., Vimeux, F., Frankenberg, C., Noone, D., and Worden, J. (2010). Understanding the Sahelian water budget through the isotopic composition of water vapor and precipitation. J. Geophys. Res. Atmos. 115:D24110. doi: 10.1029/2010JD014690
Rudeva, I., and Gulev, S. K. (2011). Composite analysis of North Atlantic extratropical cyclones in NCEP–NCAR reanalysis data. Monthly Weather Rev. 139, 1419–1446. doi: 10.1175/2010MWR3294.1
Schneider, U., Finger, P., Meyer-Christoffer, A., Rustemeier, E., Ziese, M., and Becker, A. (2017). Evaluating the hydrological cycle over land using the newly-corrected precipitation climatology from the Global Precipitation Climatology Centre (GPCC). Atmosphere 8:52. doi: 10.3390/atmos8030052
Shao, H., Derber, J., Huang, X. Y., Hu, M., Newman, K., Stark, D., et al. (2016). Bridging research to operations transitions: status and plans of community GSI. Bull. Am/. Meteorol. Soc. 97, 1427–1440. doi: 10.1175/bams-d-13-00245.1
Skamarock, W. C., Klemp, J. B., Dudhia, J., Gill, D. O., Barker, D. M., Duda, M. G., et al. (2008). A Description of the Advanced Research WRF Version 3Rep. Boulder, CO: National Center For Atmospheric Research Boulder Co Mesoscale and Microscale.
Sotillo, M., Ratsimandresy, A., Carretero, J., Bentamy, A., Valero, F., and González-Rouco, F. (2005). A high-resolution 44-year atmospheric hindcast for the Mediterranean Basin: contribution to the regional improvement of global reanalysis. Clim. Dyn. 25, 219–236. doi: 10.1007/s00382-005-0030-7
Su, C.-H., Eizenberg, N., Steinle, P., Jakob, D., Fox-Hughes, P., White, C. J., et al. (2019). BARRA v1. 0: the Bureau of Meteorology atmospheric high-resolution regional reanalysis for Australia. Geosci. Model Dev. 12, 2049–2068. doi: 10.5194/gmd-12-2049-2019
Sylla, M. B., Coppola, E., Mariotti, L., Giorgi, F., Ruti, P., Dell’Aquila, A., et al. (2010). Multiyear simulation of the African climate using a regional climate model (RegCM3) with the high resolution ERA-interim reanalysis. Clim. Dyn. 35, 231–247. doi: 10.1007/s00382-009-0613-9
Tarek, M., Brissette, F. P., and Arsenault, R. (2020). Evaluation of the ERA5 reanalysis as a potential reference dataset for hydrological modelling over North America. Hydrol. Earth Syst. Sci. 24, 2527–2544.
Taszarek, M., Allen, J. T., Púčik, T., Hoogewind, K. A., and Brooks, H. E. (2020). Severe convective storms across Europe and the United States. Part II: ERA5 environments associated with lightning, large hail, severe wind, and tornadoes. Journal of Climate 33, 10263–10286.
Trenberth, K. E. (1999). “Conceptual framework for changes of extremes of the hydrological cycle with climate change,” in Weather and Climate Extremes, eds T. R. Karl, N. Nicholls, and A. Ghazi (Dordrecht: Springer), 327–339. doi: 10.1007/978-94-015-9265-9_18
Trubilowicz, J. W., Shea, J., Jost, G., and Moore, R. (2016). Suitability of North American Regional Reanalysis (NARR) output for hydrologic modelling and analysis in mountainous terrain. Hydrol. Process. 30, 2332–2347. doi: 10.1002/hyp.10795
Veldkamp, T., Wada, Y., Aerts, J., and Ward, P. (2016). Towards a global water scarcity risk assessment framework: incorporation of probability distributions and hydro-climatic variability. Environ. Res. Lett. 11:024006. doi: 10.1088/1748-9326/11/2/024006
von Storch, H. (1999). “The global and regional climate system,” in Anthropogenic Climate Change, eds H. von Storch and G. Flöser (Berlin: Springer), 3–36. doi: 10.1007/978-3-642-59992-7_1
von Storch, H., Langenberg, H., and Feser, F. (2000). A spectral nudging technique for dynamical downscaling purposes. Mon. Weather Rev. 128, 3664–3673. doi: 10.1175/1520-0493(2000)128<3664:Asntfd>2.0.Co;2
von Storch, H., Feser, F., Geyer, B., Klehmet, K., Li, D., Rockel, B., et al. (2017). Regional reanalysis without local data: exploiting the downscaling paradigm. J. Geophys. Res. Atmos. 122, 8631–8649. doi: 10.1002/2016JD026332
Wang, Y., and Zhou, L. (2005). Correction to “Observed trends in extreme precipitation events in China during 1961–2001 and the associated changes in large-scale circulation. Geophys. Res. Lett. 32:L09707. doi: 10.1029/2005GL023769
Wu, J., and Gao, X.-J. (2013). A gridded daily observation dataset over China region and comparison with the other datasets. Diqiu Wuli Xuebao 56, 1102–1111.
Yatagai, A., Kamiguchi, K., Arakawa, O., Hamada, A., Yasutomi, N., and Kitoh, A. (2012). APHRODITE: Constructing a long-term daily gridded precipitation dataset for Asia based on a dense network of rain gauges. Bull. Am. Meteorol. Soc. 93, 1401–1415. doi: 10.1175/BAMS-D-11-00122.1
Yu, R., Zhou, T., Xiong, A., Zhu, Y., and Li, J. (2007). Diurnal variations of summer precipitation over contiguous China. Geophys. Res. Lett. 34:L01704. doi: 10.1029/2006GL028129
Zhang, Q., Pan, Y., Wang, S., Xu, J., and Tang, J. (2017). High-resolution regional reanalysis in China: Evaluation of 1 year period experiments. J. Geophys. Res. Atmos. 122, 10801–10819. doi: 10.1002/2017JD027476
Zhang, Y., Cai, C., Chen, B., and Dai, W. (2019). Consistency evaluation of precipitable water vapor derived from ERA5, ERA-Interim, GNSS, and radiosondes over China. Radio Sci. 54, 561–571.
Zhou, T., Yu, R., Chen, H., Dai, A., and Pan, Y. (2008). Summer precipitation frequency, intensity, and diurnal cycle over China: a comparison of satellite data with rain gauge observations. J. Clim. 21, 3997–4010. doi: 10.1175/2008JCLI2028.1
Keywords: evaluation, precipitation, regional reanalysis, spectral nudging, data assimilation
Citation: Lu Y, Shao M, Fang J, Pan Y and Tang J (2021) Evaluation of Precipitation in the Chinese Regional Reanalysis Using Satellite Estimates, Gauge-Based Observations and Global Reanalysis. Front. Earth Sci. 9:647580. doi: 10.3389/feart.2021.647580
Received: 30 December 2020; Accepted: 29 March 2021;
Published: 16 April 2021.
Edited by:
Xing Yuan, Nanjing University of Information Science and Technology, ChinaReviewed by:
Shanhu Jiang, Hohai University, ChinaLiwei Zou, Institute of Atmospheric Physics (CAS), China
Copyright © 2021 Lu, Shao, Fang, Pan and Tang. This is an open-access article distributed under the terms of the Creative Commons Attribution License (CC BY). The use, distribution or reproduction in other forums is permitted, provided the original author(s) and the copyright owner(s) are credited and that the original publication in this journal is cited, in accordance with accepted academic practice. No use, distribution or reproduction is permitted which does not comply with these terms.
*Correspondence: Min Shao, bXNoYW9AbWFzb25saXZlLmdtdS5lZHU=; Jiangping Tang, anB0YW5nQG5qdS5lZHUuY24=