- 1Beijing Institute of Technology Chongqing Innovation Center, Chongqing, China
- 2Radar Research Lab, School of Information and Electronics, Beijing Institute of Technology, Beijing, China
- 3Faculty of Engineering, China University of Geosciences, Wuhan, China
- 4School of Resources and Geosciences, China University of Mining and Technology, Xuzhou, China
- 5School of Civil Engineering, Chongqing University, Chongqing, China
A landslide susceptibility map (LSM) is the basis of hazard and risk assessment, guiding land planning and utilization, early warning of disaster, etc. Researchers are often overly keen on hybridizing state-of-the-art models or exploring new mathematical susceptibility models to improve the accuracy of the susceptibility map in terms of a receiver operator characteristic curve. Correlation analysis of the causal factors is a necessary routine process before susceptibility modeling to ensure that the overall correlation among all factors is low. However, this overall correlation analysis is insufficient to detect a high local correlation among the causal factor classes. The objective of this study is to answer three questions: 1) Is there a high correlation between causal factors in some parts locally? 2) Does it affect the accuracy of landslide susceptibility assessment? and 3) How can this influence be eliminated? To this aim, Wanzhou County was taken as the test site, where landslide susceptibility assessment based on 12 causal factors has been previously performed using the frequency ratio (FR) model and random forest (RF) model. In this work, we conducted a local spatial correlation analysis of the “altitude” and “rivers” factors and found a sizeable spatial overlap between altitude-class-1 and rivers-class-1. The “altitude” and “rivers” factors were reclassified, and then the FR model and RF model were used to reevaluate the susceptibility and analyze the accuracy loss caused by the local spatial correlation of the two factors. The results demonstrated that the accuracy of LSMs was markedly enhanced after reclassification of “altitude” and “rivers,” especially for the RF model–based LSM. This research shed new light on the local correlation of causal factors arising from a particular geomorphology and their impact on susceptibility.
Introduction
The landslide susceptibility map represents the spatial probability of landslide occurrence, is the basis for landslide hazard and risk assessment (Fell et al., 2008; Pellicani et al., 2017), and is used in practice for land planning (Cascini 2008; Chen et al., 2019), quantitative risk analysis (Chen et al., 2016; Yan et al., 2020), early warning systems (Segoni et al., 2018; Rosi et al., 2021), etc. In the past several decades, hazard susceptibility assessment has always been a hot spot for research on all kinds of regional scales, including local-scale (Yang J. et al., 2019), basin-scale (Bueechi et al., 2019; Huang et al., 2021a), and national-scale (Bălteanu et al., 2020). The relationship between existing landslides and their causal factors is modeled to obtain the landslide probability for the whole study area, which is the basic framework of landslide susceptibility. The internal geological and external environmental factors are the main incentives of landslides, characterized by altitude, slope, aspect, lithology, curvature, human engineering activities, rivers, traffic, etc. (Xiao et al., 2019). In recent years, to improve the accuracy of susceptibility evaluation, lots of new statistical (Segoni et al., 2016; Reichenbach et al., 2018) and machine learning methods (Catani et al., 2013; Lagomarsino et al., 2017; Huang et al., 2020), or multiple mixed-matching models (Rossi et al., 2010; Shirzadi et al., 2017; Huang et al., 2021b), have been introduced in susceptibility mapping.
After the susceptibility calculation, a receiver operator characteristic (ROC) curve is always required for accurate analysis (Xiao et al., 2020). The model with the highest AUC is considered the best model suitable for this test site (Canavesi et al., 2020; Sun et al., 2020) and, at the same time, provides a reference for other research areas. Researchers are overly keen on hybridizing state-of-the-art models (Schicker and Moon, 2012; Kornejady et al., 2018; Luo and Liu, 2018) or exploring new mathematical susceptibility models (Chen et al., 2017; Yang Y. et al., 2019; Paryani et al., 2020; Wu et al., 2020), often ignoring the interrelationships between causal factors. It is a well-known fact that each study area has its specific geomorphological features. By analyzing the correlation of the causal factors, factors with high overall correlation were excluded (Liu et al., 2019; Mind'je et al., 2020; Zhao and Chen, 2020). However, the remaining causal factors may be highly correlated in some micro-topography parts, which cannot be detected by the overall correlation analysis and have not been mentioned in the literature. Given this, several issues need to be discussed: Is there a high correlation between causal factors in some parts locally? Does it affect the accuracy of landslide susceptibility assessment? How can this influence be eliminated?
In Wanzhou County, Chongqing, China, the Yangtze River flows through the entire area from southwest to northeast, causing many landslides along both sides of the Yangtze River (Yang et al., 2017; Wang et al., 2019; Huang et al., 2021; Wang et al., 2021). Both sides of the Yangtze River are highly susceptible to landslides, and the region is characterized by low elevation and proximity to rivers (Yang et al., 2018; Deng et al., 2021; Hu et al., 2021; Wang et al., 2021). Therefore, it is necessary to explore whether “altitude” and “rivers” factors are highly correlated in the region and their influence on susceptibility mapping.
This study aims to show that local spatial correlation on causal factors could exist and reduce the accuracy of susceptibility mapping. We conducted a local spatial correlation analysis on the “altitude” and “rivers” in the study area to discuss their valid contribution to susceptibility, taking Wanzhou County as an example. The “altitude” and “rivers” were reclassified; then, the frequency ratio (FR) model and random forest (RF) model were used to reevaluate the susceptibility and analyze the accuracy loss caused by the local spatial correlation of these factors. The results shed new light on local correlations of factors arising from a particular geomorphology and their impact on susceptibility.
Test Site Description
Wanzhou County is located in the Three Gorges Area of the Yangtze River basin (Chongqing Municipality, southwestern China) between 107° 55′ 22″–108° 53′ 25″ E and 30° 24′ 25″–31° 14′ 58″ N, covering an area of approximately 3,457 km2 (Figure 1).
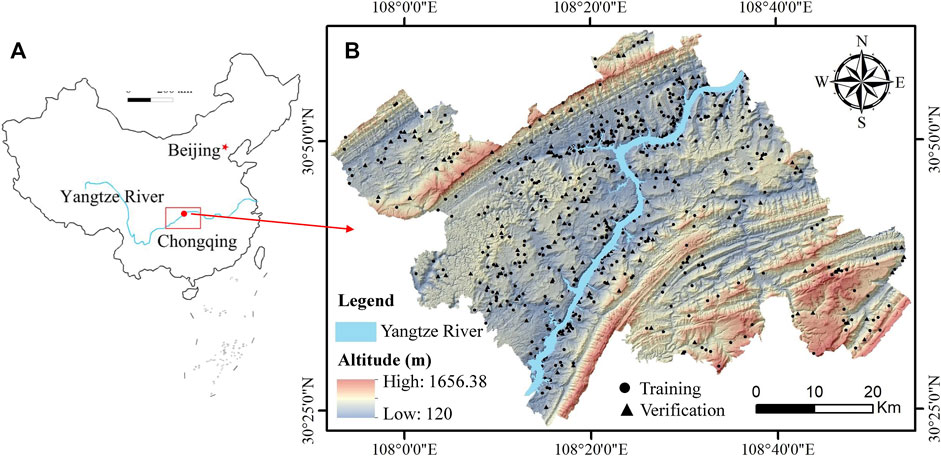
FIGURE 1. Location of study area (the coordinate system used is Xi'an 80). (A) Location of Wanzhou County, Chongqing, in China; (B) the topography map and landslide distribution in Wanzhou County.
The study area extends into the subtropical humid monsoon zone and features a mild climate with abundant sunshine and mean annual precipitation of 1,191.3 mm, mainly concentrated from May to September (about 90% of the yearly rainfall). During summer, the rain is characterized by short and intense rainstorms (up to 100 mm/day). The Yangtze River runs throughout the study area from southwest to northeast, and 93 large and small streams form a complex surface runoff network. The elevation gradually decreases from east to west, forming a hilly landscape, with an overall step-like morphology formed by multilevel fluvial terraces, which resulted from the combination of repeated tectonic uplift stages and the Yangtze River erosion. According to the information provided by Chongqing Natural Resources Bureau, more than 600 landslides were identified in the study area. Since the impoundment of the Three Gorges Reservoir in 2003, many dormant landslides have been reactivated, mainly triggered by water level fluctuation and rainfall. The well-known Anlesi Landslide, Caojiezi Landslide, and Taibaiyan Landslide are all ancient landslides with a volume of more than 10 million cubic meters, and they all developed in subhorizontally dipping sandstone and mudstone interbedded strata.
The bedrock lithology encompasses sandstones, mudstones, shales, and limestones (Table 1), with nearly horizontal stratifications. Extending from both sides of the Yangtze River, the outcropping bedrock mainly increases in age from Triassic to Jurassic (2.3–137 Ma), with sporadic Permian (299–252 Ma) and Quaternary bedrock (from 2.5 Ma). The middle Jurassic Shaximiao Group, consisting of alternating layers of sandstone and mudstone, is the most widely distributed geological unit.
Input Data and Methodology
Modeling Algorithms
1) Frequency ratio (FR) model.
The frequency ratio model is a relatively simple statistical model (Kumar and Anbalagan, 2015). Each factor is classified according to a specific method, and the contribution degree of each factor category is calculated based on statistical analysis. The contribution degree set of all factors is the Landslide Susceptibility Index (LSI), and the formula is
where S1 is the landslide area within the classification, S is the area within the classification, A1 is the total landslide area of the study area, and A is the total area of the study area.
2) Random forest (RF) model.
The random forest model is a nonparametric multivariate technology based on ensemble learning algorithm. This technology was proposed by Breiman and was widely used in various research fields because of its excellent performance, including landslide disaster susceptibility evaluation (Breiman, 1996a, 1996b; Breiman, 2001). Random forest model is considered to be a relatively effective method in classification, regression, and unsupervised learning. It contains some classification numbers for prediction, and this classification tree is randomly generated by using “bagging” to generate multiple independent training sets. The main advantages of this model are as follows: It is suitable for analyzing nonlinear variables without considering multicollinearity and has strong robustness to outliers; it can deal with high-dimensional data, take into account discrete data and continuous data, and has no fixed standardization requirements for the input data set; the data processing speed is fast and can obtain the variable importance sorting; and compared with other models, it has a strong anti-noise ability.
Input Data and Methodology
Twelve landslide susceptibility causal factors of Wanzhou County and two models, namely, frequency ratio (FR) and random forest (RF), are used in this research. The selected 12 causal factors are altitude, slope, aspect, plan curvature, profile curvature, Stream Power Index (SPI), bedding structure, lithology, land use, geological structure, rivers, and roads. In the past, we have done many studies on susceptibility assessment, including the susceptibility mapping of Wanzhou County based on these two models and 12 factors (for more details, see the article by Xiao et al., 2019). The classification and frequency ratio contribution of the factors are shown in Table 2. The receiver operator characteristic (ROC) curves were used to test the accuracy of the susceptibility results, with 72.8 and 79.9% accuracy under the FR model and RF model, respectively.
In the study area, massive landslides were induced by the Yangtze River, heavily skewing the landslide distribution toward lower altitudes. The altitude range of the study area is 120–1,656 m, divided into six classes: 120–350, 350–500, 500–700, 700–900, 900–1,100, and 1,100–1,656 m (Table 3). According to their scale, the water systems were divided into three types: I) the main stem of the Yangtze River, II) secondary tributaries of the Yangtze River, and III) seasonal streams. The influence of the river on landslide development is related to the type of river and the distance from the slope to the river. The rivers factor was divided into five classes by distance to each water system shown in Table 4.
In the previous susceptibility evaluation, the Spearman correlation coefficient between altitude and rivers was only −0.14 (Table 5), indicating that overall the correlation between these two factors was low. The altitude-class-1 zone (less than 350 m) has the highest frequency ratio contribution (Table 2), attributed to the rivers’ effect in the initial analysis. The water level of the Yangtze River reservoir fluctuates between 145 and 175 m, affecting slopes mostly below 350 m, thus exhibiting a tendency for landslides to be distributed at different altitudes. After in-depth consideration of the causal factors in the study area, it was found that river development is highly related to topographic elevation, so there may be a considerable spatial overlap between the altitude-class-1 zone and rivers-class-1 zone.
Therefore, there are three possible issues: Is there a high correlation between altitude-class-1 and rivers-class-1 zones; Does it affect the accuracy of landslide susceptibility assessment; and How can this influence be eliminated? Exploring and answering the three issues are the main research objectives of this study. The research idea includes the following steps:
- First, altitude-class-1 and rivers-class-1 were divided into three zones: a, b, and c. As shown in Figure 2, “a” is the common area for altitude-class-1 and rivers-class-1, and “b” and “c” are separate areas for altitude-class-1 and rivers-class-1, respectively. The frequency ratios of landslides in zones a, b, and c were counted and compared with altitude-class-1 and rivers-class-1 to reflect the actual contribution of the two factors. This step can answer the question of whether there is a high correlation between altitude-class-1 and rivers-class-1 regions.
- The altitude and rivers factors were reclassified, and then the susceptibility of Wanzhou County was re-evaluated. The altitude was divided into seven classes, where classes-2 to 6 remained the same, and class-1 was split into class-1a and class-1b. The rivers factor was divided into six classes, where classes-2 to 5 were left as they were, and class-1 was split into class-1a and class-1c. Altitude-class-1a and rivers-class-1a are, spatially, the exact same area. Susceptibility was reassessed using FR and RF models based on reclassified altitude and rivers and the original ten other causal factors. This step can be considered a preliminary stage to directly illustrate the impact on the accuracy of the susceptibility evaluation while providing quantitative data for analysis in a further step.
- Quantitative and pixel-by-pixel analysis of susceptibility maps: The receiver operator characteristic (ROC) curve was used to verify the accuracy of the susceptibility results, and pixel-by-pixel for going through where the susceptibility map changed after factor reclassification.
Results
Figure 3 presents a visual inspection that clearly exemplifies the distribution of landslides in altitude-class-1 and rivers-class-1 areas. The dark gray “a” zone represents the common area for altitude-class-1 and rivers-class-1, while the blue “c” and orange “b” are the separate areas for altitude-class-1 and rivers-class-1, respectively. All landslides in the study area are superimposed on the map in black rasters, showing the differential distribution of landslides in areas a, b, and c. We can see at a glance that the landslides in the gray area are less than those in the dark gray and the blue areas. As a quantitative comparison, landslide frequency ratio statistics were performed for each a, b, or c area (Table 6). The data show that the frequency of landslide distribution in areas a, b, and c varies greatly. The landslide frequency ratio in the common area a is 2.72, the landslide frequency ratio in altitude-class-1 rises from 2.98 to 3.49 after removing area a, and the landslide frequency ratio in rivers-class-1 plummets from 1.41 to 0.46 after removing area a. It can be tentatively inferred that the common area of altitude-class-1 and rivers-class-1 to some extent influences the judgment of the actual contribution of altitude and rivers factors to landslide development. That is, the initially calculated landslide frequency ratios of altitude and rivers are not entirely reliable.
“Altitude-class-1” was reclassified into “altitude-class-1a” and “altitude-class-1b,” while “rivers-class-1” was divided into “rivers-class-1a” and “rivers-class-1c.” Table 7 shows the original classes and new classes, concluding the percentage of domain in the total domain and frequency ratio contribution of each class. At the same time, a Coxcomb chart (Figure 4) clearly expressed all the information in Table 7. The arc of the sector represents the PDTD of each class, and its radius stands for the FR value. The red stripes represent the original class-1, and the reclassified areas 1a and 1b (1c) are indicated in blue and green, respectively, to reflect the contribution of each area to landslide development by the length of the sector radius.
It is evident from Figure 4 that the landslide frequency distribution in class-1 is not uniform, especially for the “rivers-class-1” area: “Rivers-class-1a” far exceeds the average contribution of “rivers-class-1.” In contrast, the true gift of “rivers-class-1c” is minimal. It follows that a reclassification of the area was absolutely necessary to better reflect the contribution of causal factors to landslides. To verify the effects of reclassifying “altitude-class-1” and “rivers-class-1,” the 12 causal factor system of the previous susceptibility assessment in Table 2 was used in the landslide susceptibility assessment in this test. Except for altitude and rivers, the remaining ten causal factors continued the previous classification.
The LSM of Wanzhou County was recalculated using the FR model and RF model based on improved factors; then, the area under the receiver operating characteristic (ROC) curve (AUC) was applied to evaluate the accuracy of each result. The ROC curve mainly reflects the change of the number of landslides in each susceptibility interval from high to low. As shown in Figure 5, after reclassification of altitude-class-1 and rivers-class-1, the accuracy of LSM based on the FR model was improved by 0.5% (72.8–73.3%), and the accuracy of LSM based on the RF model was significantly improved by 5.1% (79.9–85.0%).
The LSM was divided into 10 zones with 10% spacing according to the susceptibility value (i.e., the landslide probability of occurrence), and pixel-by-pixel counted the number of landslide pixels and all pixels in each region, respectively. It is evident that the number of landslide points is directly proportional to the susceptibility value (Figure 6A). For the two models, the percentages of landslides in the range of the top 20% interval of the occurrence probability were improved 8.1% (FR model, 18.10–26.2%) and 24.87% (RF model, 24.2–48.98%), respectively. In contrast, pixels were primarily located in zones with susceptibility value below 40% (Figure 6B).
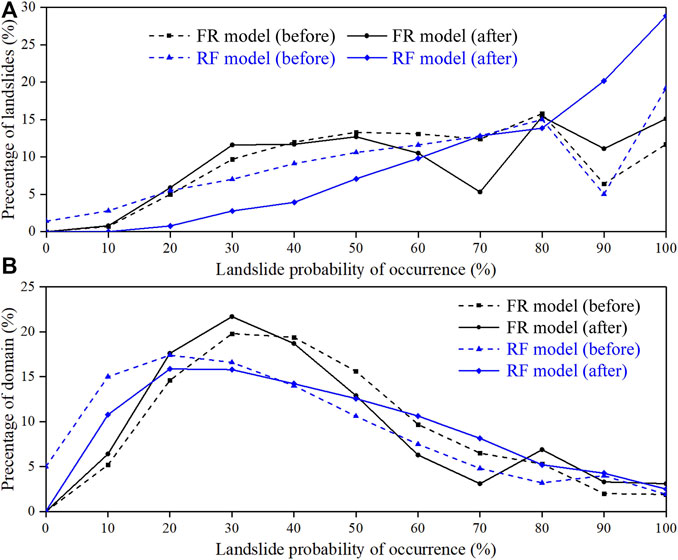
FIGURE 6. Distribution of points versus the landslide probability of occurrence. (A) Landslide points; (B) all pixels in the domain.
The susceptibility value was divided into five zones by equal interval: very low (0–20%), low (20–40%), moderate (40–60%), high (60–80%), and very high (80–100%). The landslide statistics of different susceptibility levels are shown in Table 8 and Figure 7. The frequency ratio value for the very high susceptibility areas varied considerably. The frequency ratio value based on the FR model increased from 4.09 to 4.64, and the value based on the RF model increased from 4.10 to 7.23.
The above results demonstrated that the accuracy of the very high susceptibility zone was markedly enhanced after reclassification of “altitude-class-1” and “rivers-class-1,” especially for the RF model–based LSM.
Discussion
The two LSMs based on the RF model are shown in Figure 8. Although the improved LSM has a 5.9% higher AUC, it is not easy to see the difference when comparing these two graphs with the naked eye. A visual comparison of the two maps was made, and their values were subtracted to define their differences (Figure 9). Since the raster value of each susceptibility map is between 0 and 1, the value of the comparison map could potentially range from − 1 to 1. A simple visual inspection of Figure 9 reveals that there are apparent differences between the two susceptibility maps. The value range of Figure 9 is −0.9731–0.9482, with pure blue representing −1, pure red representing 1, and a gradual blue–yellow–red transition between −1 and 1. Most importantly, the differences between the two LSMs are not evenly distributed, and some spatial patterns of rivers can be recognized in the comparison map.
Concerning the method proposed by Xiao et al. (2020) for understanding and interpreting the different results of LSM, the values of the comparison map were interrupted at ±0.5 and divided into three classes, namely, “underestimation” (UN), “approximation” (APR), and “overestimation” (OV). Table 9 shows the range of values and percentages for each classification. 97.13% of the comparison map pixels are located in the APR region, and only scattering pixels are UN or OV.
To explore the critical class of the rivers factor that led to differences between susceptibility maps, a simple count of the UN and OV points for each class of rivers was performed (Table 10). In the statistics of Table 10, rivers-class-1a only accounts for 9.68% of the total area, but it contains 26.53% of UN pixels. Meanwhile, rivers-class-1c accounts for only 13.27% of the total area, but it has 38.16% OV pixels.
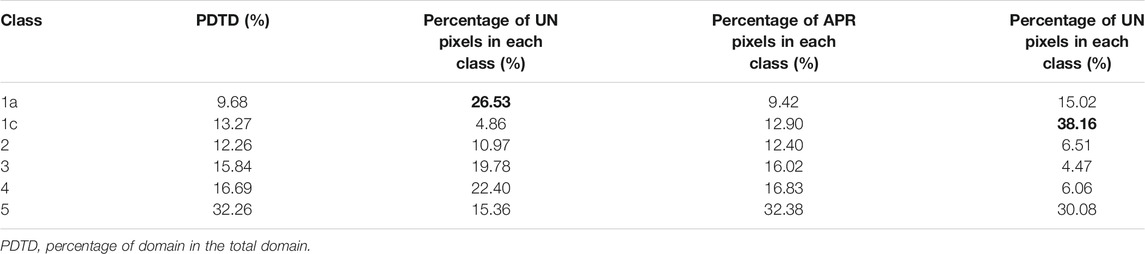
TABLE 10. Simple statistical properties of UN/APR/OV pixel distribution across each class of the rivers factor.
In the original RF model–based susceptibility assessment, rivers-class-1 was not differentiated into area 1a and area 1c. This statistical result indicates that the susceptibility value in rivers-class-1a is underestimated, and rivers-class-1c is overestimated in the original LSM. The deviation of the susceptibility results is exactly the same as that in the factor contribution analysis (Table 7; Figure 4B). The landslide contribution in the rivers-class-1a area was underestimated, where the calculated susceptibility values were underestimated. For rivers-class-1c, both landslide contribution and susceptibility value were overestimated. After reclassifying the rivers factor, the RF model improved the LSM accuracy in the rivers-class-1 area, thus improving the accuracy in the high susceptibility area and the whole area.
Rivers-classes-1a and 1c are visually inspected and explicitly represented in Figure 10 concerning the UN or OV pixels. In Figure 10A, the rivers-class-1a area is marked in yellow, the rivers-class-1c area is indicated in blue, and the other classes are uniformly noted in light gray. UN and OV pixels are displayed in black and red, respectively, scattered sporadically throughout the study area. Zooming in on the two regions of Figures 10B,C, one can clearly see that the red OV pixels tend to be distributed on class-1c, again in agreement with the statistical properties of Table 10.
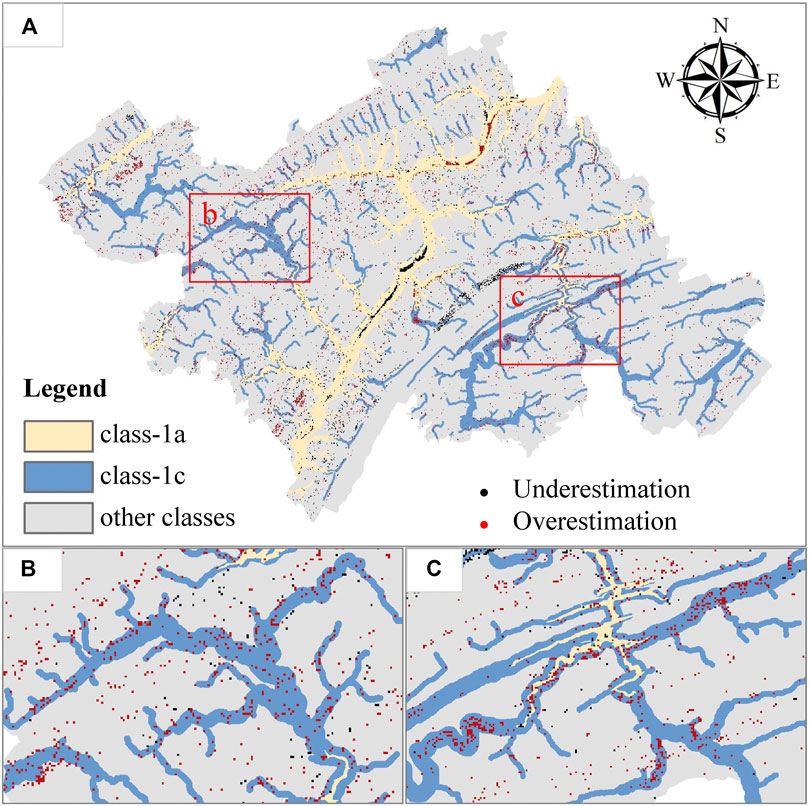
FIGURE 10. Spatial location of underestimations and overestimations in relation to rivers-class-1. (A) Whole study area; (B) typical region; (C) typical region.
Previous studies of landslide susceptibility have included correlation analysis of the causal factors, but only for each causal factor as a whole. The study in this work demonstrated the existence of a high local correlation between classifications of altitude and rivers. In other words, the high local correlation of factor classifications cannot be detected by the overall correlation analysis. In this study, the conjecture about altitude and rivers comes entirely from the in-depth knowledge of the topography and river system in the study area. On the basis of this conjecture, a local correlation analysis and a quantitative study of its effect on the accuracy of LSM were performed. The results show that the high local correlation of altitude and rivers factors does exist and truly affects the accuracy of LSM. Meanwhile, a simple reclassification of factors can eliminate this effect and improve the accuracy of LSM.
Conclusion
This study shows that the local correlation of causal factors could exist and reduce the accuracy of susceptibility assessment. A simple method of factor reclassification was proposed to improve the accuracy of LSM effectively. Taking Wanzhou County as the test site, where landslide susceptibility assessment was based on 12 causal factors, the FR model and RF model were previously completed. In this work, we conducted a local spatial correlation analysis of the “altitude” and “rivers” factors and found a large spatial overlap between altitude-class-1 and rivers-class-1. “Altitude-class-1” was reclassified into “altitude-class-1a” and “altitude-class-1b,” while “rivers-class-1” was divided into “rivers-class-1a” and “rivers-class-1c,” where “altitude-class-1a” was spatially identical to the “rivers-class-1a” area. The FR model and RF model were used to reevaluate the susceptibility. The area under the receiver operating characteristic curve (AUC) was applied to evaluate the accuracy of each LSM. The results demonstrated that the accuracy of LSMs was markedly enhanced after reclassification of “altitude-class-1” and “rivers-class-1,” especially for the RF model–based LSM. A pixel-by-pixel comparison of the two LSMs based on the RF model was performed and visually inspected with rivers-class-1. In previous susceptibility mapping, the calculated susceptibility value in the rivers-class-1a area tends to be underestimated, and the opposite is seen for the rivers-class-1c area. This research shed new light on the local correlation of causal factors arising from a particular geomorphology and their impact on susceptibility.
Finally, the following points can be summarized for the cases in this study.
- The overall correlation between the altitude and rivers factor is low, but there is a considerable spatial overlap between altitude-class-1 and rivers-class-1. The presence of this common overlap area has led to the underestimation and overestimation of the contribution of altitude-class-1 and rivers-class-1 to landslides, respectively, in previous susceptibility assessments.
- The accuracy of the LSMs was improved by 0.5% (FR model) and 5.1% (RF model) after reclassification of “altitude-class-1” and “rivers-class-1,” respectively, especially for the accuracy of the very high susceptibility zone of the RF model–based LSM.
- Since the FR model does not consider the weight coefficients of the causal factors, the FR model–based LSM is not sensitive enough to the reclassification of the altitude and rivers factors. The RF model performs better not only in modeling the relationship between causal factors and landslides but also in distinguishing the differences of each factor class.
Data Availability Statement
The raw data supporting the conclusion of this article will be made available by the authors, without undue reservation.
Author Contributions
TX organized and analyzed the data and wrote the manuscript, LW provided and analyzed the data, LY analyzed the data and wrote the manuscript, WT and TX were responsible for the project, and CZ analyzed the data. All authors have read and agreed to the published version of the article.
Funding
This research was supported in part by the Natural Science Foundation of Chongqing, China, under Grant cstc2020jcyj-jqX0008; in part by the National Natural Science Foundation of China under Grants 61960206009, 61971037, and 31727901; and in part by Chongqing Key Laboratory of Geological Environment Monitoring and Disaster Early-Warning in Three Gorges Reservoir Area under Grant MP2020B0301.
Conflict of Interest
The authors declare that the research was conducted in the absence of any commercial or financial relationships that could be construed as a potential conflict of interest.
Publisher’s Note
All claims expressed in this article are solely those of the authors and do not necessarily represent those of their affiliated organizations, or those of the publisher, the editors, and the reviewers. Any product that may be evaluated in this article, or claim that may be made by its manufacturer, is not guaranteed or endorsed by the publisher.
Abbreviations
AUC, area under the receiver operating characteristic curve; APR, approximation; FR model, frequency ratio model; LSM, landslide susceptibility map; OV, overestimation; PDTD, percentage of domain in total domain; PLTL, percentage of landslide in total landslide; RF model, random forest model; ROC, receiver operator characteristic; SPI, Stream Power Index; UN, underestimation.
References
Bălteanu, D., Micu, M., Jurchescu, M., Malet, J.-P., Sima, M., Kucsicsa, G., et al. (2020). National-scale Landslide Susceptibility Map of Romania in a European Methodological Framework. Geomorphology 371, 107432. doi:10.1016/j.geomorph.2020.107432
Breiman, L. (2001). Statistical Modeling: The Two Cultures (With Comments and a Rejoinder by the Author). Statist. Sci. 16 (3), 199–231. doi:10.1214/ss/1009213726
Bueechi, E., Klimeš, J., Frey, H., Huggel, C., Strozzi, T., and Cochachin, A. (2019). Regional-scale Landslide Susceptibility Modelling in the Cordillera Blanca, Peru-a Comparison of Different Approaches. Landslides 16 (2), 395–407. doi:10.1007/s10346-018-1090-1
Canavesi, V., Segoni, S., Rosi, A., Ting, X., Nery, T., Catani, F., et al. (2020). Different Approaches to Use Morphometric Attributes in Landslide Susceptibility Mapping Based on Meso-Scale Spatial Units: A Case Study in Rio de Janeiro (Brazil). Remote Sensing 12 (11), 1826. doi:10.3390/rs12111826
Cascini, L. (2008). Applicability of Landslide Susceptibility and hazard Zoning at Different Scales. Eng. Geology. 102 (3-4), 164–177. doi:10.1016/j.enggeo.2008.03.016
Catani, F., Lagomarsino, D., Segoni, S., and Tofani, V. (2013). Landslide Susceptibility Estimation by Random Forests Technique: Sensitivity and Scaling Issues. Nat. Hazards Earth Syst. Sci. 13 (11), 2815–2831. doi:10.5194/nhess-13-2815-2013
Chen, L., Guo, Z., Yin, K., Shrestha, D. P., and Jin, S. (2019). The influence of land use and land cover change on landslide susceptibility: a case study in Zhushan Town, Xuan'en County (Hubei, China). Nat. Hazards Earth Syst. Sci. 19 (10), 2207–2228. doi:10.5194/nhess-19-2207-2019
Chen, L., van Westen, C. J., Hussin, H., Ciurean, R. L., Turkington, T., Chavarro-Rincon, D., et al. (2016). Integrating Expert Opinion with Modelling for Quantitative Multi-hazard Risk Assessment in the Eastern Italian Alps. Geomorphology 273, 150–167. doi:10.1016/j.geomorph.2016.07.041
Chen, W., Shirzadi, A., Shahabi, H., Ahmad, B. B., Zhang, S., Hong, H., et al. (2017). A Novel Hybrid Artificial Intelligence Approach Based on the Rotation forest Ensemble and Naïve Bayes Tree Classifiers for a Landslide Susceptibility Assessment in Langao County, China. Geomatics, Nat. Hazards Risk 8 (2), 1955–1977. doi:10.1080/19475705.2017.1401560
Deng, Y., Hu, C., Tian, W., and Zhao, Z. (2021). A Grid Partition Method for Atmospheric Phase Compensation in GB-SAR. IEEE Trans. Geosci. Remote Sens., 1–13. doi:10.1109/TGRS.2021.3074161
Fell, R., Corominas, J., Bonnard, C., Cascini, L., Leroi, E., and Savage, W. Z. (2008). Guidelines for Landslide Susceptibility, hazard and Risk Zoning for Land-Use Planning. Eng. Geology. 102 (3-4), 99–111. doi:10.1016/j.enggeo.2008.03.014
Hu, C., Deng, Y., and Tian, W. (2021). Multistatic Ground-Based Differential Interferometric MIMO Radar for 3D Deformation Measurement. Sci. China Inf. Sci. 64 (12), 227301. doi:10.1007/s11432-021-3352-y
Huang, C., Zhou, Q., Zhou, L., and Cao, Y. (2021). Ancient Landslide in Wanzhou District Analysis from 2015 to 2018 Based on ALOS-2 Data by QPS-InSAR. Nat. Hazards, 1–24. doi:10.1007/s11069-021-04898-0
Huang, F., Cao, Z., Jiang, S.-H., Zhou, C., Huang, J., and Guo, Z. (2020). Landslide Susceptibility Prediction Based on a Semi-supervised Multiple-Layer Perceptron Model. Landslides 17 (12), 2919–2930. doi:10.1007/s10346-020-01473-9
Huang, F., Tao, S., Chang, Z., Huang, J., Fan, X., Jiang, S.-H., et al. (2021a). Efficient and Automatic Extraction of Slope Units Based on Multi-Scale Segmentation Method for Landslide Assessments. Landslides, 1–17. doi:10.1007/s10346-021-01756-9
Huang, F., Ye, Z., Jiang, S.-H., Huang, J., Chang, Z., and Chen, J. (2021b). Uncertainty Study of Landslide Susceptibility Prediction Considering the Different Attribute Interval Numbers of Environmental Factors and Different Data-Based Models. Catena 202, 105250. doi:10.1016/j.catena.2021.105250
Kornejady, A., Ownegh, M., Rahmati, O., and Bahremand, A. (2018). Landslide Susceptibility Assessment Using Three Bivariate Models Considering the New Topo-Hydrological Factor: HAND. Geocarto Int. 33 (11), 1155–1185. doi:10.1080/10106049.2017.1334832
Kumar, R., and Anbalagan, R. (2015). Landslide Susceptibility Zonation in Part of Tehri Reservoir Region Using Frequency Ratio, Fuzzy Logic and Gis. J. Earth Syst. Sci. 124 (2), 431–448. doi:10.1007/s12040-015-0536-2
Lagomarsino, D., Tofani, V., Segoni, S., Catani, F., and Casagli, N. (2017). A Tool for Classification and Regression Using Random forest Methodology: Applications to Landslide Susceptibility Mapping and Soil Thickness Modeling. Environ. Model. Assess. 22 (3), 201–214. doi:10.1007/s10666-016-9538-y
Liu, L., Li, S., Li, X., Jiang, Y., Wei, W., Wang, Z., et al. (2019). An Integrated Approach for Landslide Susceptibility Mapping by Considering Spatial Correlation and Fractal Distribution of Clustered Landslide Data. Landslides 16 (4), 715–728. doi:10.1007/s10346-018-01122-2
Luo, W., and Liu, C.-C. (2018). Innovative Landslide Susceptibility Mapping Supported by Geomorphon and Geographical Detector Methods. Landslides 15 (3), 465–474. doi:10.1007/s10346-017-0893-9
Mind’je, R., Li, L., Nsengiyumva, J. B., Mupenzi, C., Nyesheja, E. M., Kayumba, P. M., et al. (2020). Landslide Susceptibility and Influencing Factors Analysis in Rwanda. Environ. Dev. Sustain. 22 (8), 7985–8012. doi:10.1007/s10668-019-00557-4
Paryani, S., Neshat, A., Javadi, S., and Pradhan, B. (2020). Comparative Performance of New Hybrid ANFIS Models in Landslide Susceptibility Mapping. Nat. Hazards 103, 1961–1988. doi:10.1007/s11069-020-04067-9
Pellicani, R., Argentiero, I., and Spilotro, G. (2017). GIS-based Predictive Models for Regional-Scale Landslide Susceptibility Assessment and Risk Mapping along Road Corridors. Geomatics, Nat. Hazards Risk 8 (2), 1012–1033. doi:10.1080/19475705.2017.1292411
Reichenbach, P., Rossi, M., Malamud, B. D., Mihir, M., and Guzzetti, F. (2018). A Review of Statistically-Based Landslide Susceptibility Models. Earth-Science Rev. 180, 60–91. doi:10.1016/j.earscirev.2018.03.001
Rosi, A., Segoni, S., Canavesi, V., Monni, A., Gallucci, A., and Casagli, N. (2021). Definition of 3D Rainfall Thresholds to Increase Operative Landslide Early Warning System Performances. Landslides 18 (3), 1045–1057. doi:10.1007/s10346-020-01523-2
Rossi, M., Guzzetti, F., Reichenbach, P., Mondini, A. C., and Peruccacci, S. (2010). Optimal Landslide Susceptibility Zonation Based on Multiple Forecasts. Geomorphology 114 (3), 129–142. doi:10.1016/j.geomorph.2009.06.020
Schicker, R., and Moon, V. (2012). Comparison of Bivariate and Multivariate Statistical Approaches in Landslide Susceptibility Mapping at a Regional Scale. Geomorphology 161-162, 40–57. doi:10.1016/j.geomorph.2012.03.036
Segoni, S., Tofani, V., Lagomarsino, D., and Moretti, S. (2016). Landslide Susceptibility of the Prato-Pistoia-Lucca Provinces, Tuscany, Italy. J. Maps 12 (Suppl. 1), 401–406. doi:10.1080/17445647.2016.1233463
Segoni, S., Tofani, V., Rosi, A., Catani, F., and Casagli, N. (2018). Combination of Rainfall Thresholds and Susceptibility Maps for Dynamic Landslide hazard Assessment at Regional Scale. Front. Earth Sci. 6, 85. doi:10.3389/feart.2018.00085
Shirzadi, A., Bui, D. T., Pham, B. T., Solaimani, K., Chapi, K., Kavian, A., et al. (2017). Shallow Landslide Susceptibility Assessment Using a Novel Hybrid Intelligence Approach. Environ. Earth Sci. 76 (2), 60. doi:10.1007/s12665-016-6374-y
Sun, D., Xu, J., Wen, H., and Wang, Y. (2020). An Optimized Random Forest Model and its Generalization Ability in Landslide Susceptibility Mapping: Application in Two Areas of Three Gorges Reservoir, China. J. Earth Sci. 31 (6), 1068–1086. doi:10.1007/s12583-020-1072-9
Wang, J., Schweizer, D., Liu, Q., Su, A., Hu, X., and Blum, P. (2021). Three-dimensional Landslide Evolution Model at the Yangtze River. Eng. Geology. 292, 106275. doi:10.1016/j.enggeo.2021.106275
Wang, L., Yin, Y., Huang, B., and Dai, Z. (2020). Damage Evolution and Stability Analysis of the Jianchuandong Dangerous Rock Mass in the Three Gorges Reservoir Area. Eng. Geology. 265, 105439. doi:10.1016/j.enggeo.2019.105439
Wang, L., Zhang, Z., Huang, B., Ming, H., and Chen, Z. (2021). Triggering mechanism and possible evolution process of the ancient Qingshi landslide in the Three Gorges Reservoir. Geomatics Nat. Hazards Risk 12 (1), 3160–3174. doi:10.1080/19475705.2021.1998230
Wu, Y., Ke, Y., Chen, Z., Liang, S., Zhao, H., and Hong, H. (2020). Application of Alternating Decision Tree with AdaBoost and Bagging Ensembles for Landslide Susceptibility Mapping. Catena 187, 104396. doi:10.1016/j.catena.2019.104396
Xiao, T., Segoni, S., Chen, L., Yin, K., and Casagli, N. (2020). A Step beyond Landslide Susceptibility Maps: a Simple Method to Investigate and Explain the Different Outcomes Obtained by Different Approaches. Landslides 17 (3), 627–640. doi:10.1007/s10346-019-01299-0
Xiao, T., Yin, K., Yao, T., and Liu, S. (2019). Spatial Prediction of Landslide Susceptibility Using GIS-Based Statistical and Machine Learning Models in Wanzhou County, Three Gorges Reservoir, China. Acta Geochim 38 (5), 654–669. doi:10.1007/s11631-019-00341-1
Yan, S., He, S., Deng, Y., Liu, W., Wang, D., and Shen, F. (2020). A Reliability-Based Approach for the Impact Vulnerability Assessment of Bridge Piers Subjected to Debris Flows. Eng. Geology. 269, 105567. doi:10.1016/j.enggeo.2020.105567
Yang, B., Yin, K., Xiao, T., Chen, L., and Du, J. (2017). Annual Variation of Landslide Stability under the Effect of Water Level Fluctuation and Rainfall in the Three Gorges Reservoir, China. Environ. Earth Sci. 76 (16), 1–17. doi:10.1007/s12665-017-6898-9
Yang, H. F., Yang, S. L., Xu, K. H., Milliman, J. D., Wang, H., Yang, Z., et al. (2018). Human Impacts on Sediment in the Yangtze River: A Review and New Perspectives. Glob. Planet. Change 162, 8–17. doi:10.1016/j.gloplacha.2018.01.001
Yang, J., Song, C., Yang, Y., Xu, C., Guo, F., and Xie, L. (2019). New Method for Landslide Susceptibility Mapping Supported by Spatial Logistic Regression and GeoDetector: A Case Study of Duwen Highway Basin, Sichuan Province, China. Geomorphology 324, 62–71. doi:10.1016/j.geomorph.2018.09.019
Yang, Y., Yang, J., Xu, C., Xu, C., and Song, C. (2019). Local-scale Landslide Susceptibility Mapping Using the B-GeoSVC Model. Landslides 16 (7), 1301–1312. doi:10.1007/s10346-019-01174-y
Keywords: landslide susceptibility, altitude and rivers, local correlation, reclassification of causal factors, accuracy of landslide susceptibility map
Citation: Xiao T, Yu L, Tian W, Zhou C and Wang L (2021) Reducing Local Correlations Among Causal Factor Classifications as a Strategy to Improve Landslide Susceptibility Mapping. Front. Earth Sci. 9:781674. doi: 10.3389/feart.2021.781674
Received: 23 September 2021; Accepted: 11 October 2021;
Published: 22 November 2021.
Edited by:
Faming Huang, Nanchang University, ChinaCopyright © 2021 Xiao, Yu, Tian, Zhou and Wang. This is an open-access article distributed under the terms of the Creative Commons Attribution License (CC BY). The use, distribution or reproduction in other forums is permitted, provided the original author(s) and the copyright owner(s) are credited and that the original publication in this journal is cited, in accordance with accepted academic practice. No use, distribution or reproduction is permitted which does not comply with these terms.
*Correspondence: Luqi Wang, d2xxOTNAY3F1LmVkdS5jbg==