- 1Institute of Arctic Climate and Environment Research, JAMSTEC, Yokosuka, Japan
- 2Institute for Space—Earth Environmental Research, Nagoya University, Nagoya, Japan
- 3Institute of Arctic Climate and Environment Research, JAMSTEC, Yokohama, Japan
Water age is a useful metric to evaluate the influence of anthropogenic and natural forcings on the terrestrial water cycle. Current climate warming is enhancing the warming of permafrost soil in the Arctic. Although permafrost is a crucial component of the Arctic terrestrial water cycle, its influence on processes regulating the fluxes and ages of Arctic terrestrial water, particularly soil storage and evapotranspiration, is not well understood. In this study, a water age calculation scheme was implemented into the coupled hydrological and biogeochemical model (CHANGE) to assess the mechanisms through which climate warming affects the soil water storage–evapotranspiration–water age feedback cycle in a boreal forest. Continuous air temperature increase from 1980 to 2016 caused earlier snowmelt and soil thawing, inducing decreasing age trends in snow- and rain-sourced water. The younger water contributed to higher spring evapotranspiration. In summer, the higher evapotranspiration dried the surface soil layer. In turn, the drier surface layer increased the loss of fresh rainwater. Autumn precipitation, preserved in the frozen winter soil until the following spring, became an additional source of water and enhanced plant transpiration in the following summer. This increase accounted for 4.2% of the annual total transpiration. These results suggest that permafrost warming, characterized by earlier soil thawing and later freezing, induced higher evapotranspiration, thereby shortening the residence time of precipitation-sourced water in the active layer and further rejuvenating water in soil layers and in evapotranspiration. Under future climate warming conditions, this effect is expected to intensify and the water cycle will accelerate.
1 Introduction
Water age is defined as the time interval between the moment a water molecule enters the hydrological system and the moment it flows out (Sprenger et al., 2019). It is a useful metric to assess changes in water storages–fluxes interactions within the hydrological system (Pfister et al., 2017; Sprenger et al., 2018). In the Arctic terrestrial system, the hydrological processes regulating water storages and fluxes are completely different in winter, when soil freezes, and in summer, when soil thaws. Thus, the hydrological system, dormant during winter, experiences considerable phase changes in warmer seasons. These seasonal changes are driven primarily by air temperature. Meteorological observation data have revealed an increasing temperature trend in the Arctic over the past several decades, with particularly marked warming during spring (Bekryaev et al., 2010). Warmer air temperatures affect snowmelt (Kim et al., 2015; Zhang and Ma, 2018), soil thawing (Park et al., 2016a), and vegetation phenological and physiological activity (Ohta et al., 2014). In turn, such changes enhance water flux variations, increasing water loss via evapotranspiration and runoff (Holmes et al., 2015; Wang et al., 2021; Zhang et al., 2021). Water cycle alterations are reflected in water age variations (Hrachowitz et al., 2016).
Previous studies have estimated the non-stationary age of stream water by directly measuring inputs and outputs of isotopic tracers at catchments (Tetzlaff et al., 2014; Birkel and Soulsby, 2015; Rinaldo et al., 2015). Several process-based numerical models have also been developed, in which the incorporation of isotopic tracers effectively allowed scientists to estimate water ages in the absence of direct in situ measurements, and to better understand the physical processes for runoff generation in global catchments under different climates (Maneta and Silverman, 2013; Stadnyk et al., 2013; van Huijgevoort et al., 2016; Ala-aho et al., 2017, 2018; Piovano et al., 2019). Water loss by evapotranspiration to the atmosphere is a crucial process affecting terrestrial water storages and fluxes and, ultimately, water ages. Few water age studies have focused on evapotranspiration rather than on stream water. However, evapotranspiration measurements have shown an isotopic connection to groundwater sources (Good et al., 2015), while water ages have been estimated from evapotranspiration with process-based, coupled ecohydrological models incorporating isotopic tracers to calculate the energy balance in the atmosphere–vegetation–soil system (Sprenger et al., 2015; Kuppel et al., 2018). Other modeling studies have evaluated the influence of cold-region processes on evapotranspiration and source water ages (Smith et al., 2019). Unfortunately, quantitative information on the influence of permafrost on water flux ages in northern cold regions, using observations or tracer-based models, remains limited.
Permafrost is an important component of the hydrological system in the terrestrial Arctic. It has been increasingly affected by climate-change-induced warming and thawing (Biskaborn et al., 2019). Permafrost warming during summer caused an increase in the active layer thickness (ALT), defined as the maximum soil thawing depth (Li et al., 2022), thereby increasing water storage capacity in the active layer and, possibly, enhancing evapotranspiration (Suzuki et al., 2021). Observations also showed the sensitivity of evapotranspiration to higher soil moisture in the active layer of a Siberian boreal forest (Ohta et al., 2014; Kotani et al., 2019). Active-layer soil moisture depends primarily on inputs from snow meltwater in spring and precipitation in summer (Ma and Zhang, 2022), and on outputs from evapotranspiration. In addition, the water content of the active layer before soil thaws in spring is representative of moisture conditions from the preceding autumn (Park et al., 2021). Similarly, active-layer soil moisture conditions reflect climatic and ecohydrological events. This suggests that calculating the water age of evapotranspiration generated by this moisture might provide valuable information on the influence of permafrost warming on the soil freezing/thawing process, thus on evapotranspiration and soil moisture variations.
We have developed a water age calculation module, which uses five water isotopic tracers as modeling targets, and coupled it to our process-based land surface model (Park et al., 2021). The main objective of this study was to quantify, using the modified land surface model, the influence of climate-driven permafrost variations on model-simulated water storages, fluxes, and ages, particularly evapotranspiration water ages, of a boreal forest in 1980–2016. Using the model-simulated water ages, this study specifically investigated an important property of permafrost: the delay it induced on the response of soil water from precipitation to hydrometeorological forcings.
2 Materials and methods
2.1 General model description
This study used the process-based, coupled hydrological and biogeochemical land surface model (CHANGE: Park et al., 2011). CHANGE incorporates principles of hydrology, biology, ecology, geochemistry, and physiology to calculate momentum, heat, water and carbon quantities and partitioning, and tracer fluxes in the atmosphere–vegetation–snow–soil system, including all interactions between components and processes within the system (Park et al., 2011, 2018). The energy budget is calculated for the canopy, snow, and soil surface separately. The resulting available energy is used to derive evapotranspiration, snowmelt, and ground heat flux, as well as canopy, snow, and soil temperature. The mass conservation principle is also applied to solve the water budget for the surface layer. During snowmelt or precipitation events, the water input in the surface soil layer is divided into infiltration and surface runoff. The infiltrated water becomes a source of soil moisture and plant transpiration and generates a subsurface flow evacuated either in the permafrost table or at the bottom boundary layer. CHANGE explicitly represents water dynamics and heat fluxes in a soil column with a depth of 70 m and accounts for the freezing/thawing phase changes and the effects of organic carbon on the soil hydrothermal properties. The simulated ice content in frozen soil layers increases their impedance to water flows. The layer impedance is parameterized as the soil moisture stress conditions associated with drying or freezing. Then, it is coupled to stomatal conductance and to the maximum carboxylation rate, which controls plant productivity and phenology. CHANGE also includes a coupled dynamic vegetation module that mechanistically simulates biogeochemical processes, including the carbon and nitrogen cycles in vegetation, litter, and soil, across multiple biomes.
Moreover, CHANGE includes a water isotope tracer module designed to characterize the spatiotemporal variability of isotopes and water sources, originating from precipitation and ground ice, in the hydrological system (Park et al., 2021). With this module, it is possible to quantify the contribution of ground ice meltwater induced by permafrost thawing to soil water storages and to the subsequent subsurface flow and evapotranspiration, especially the water footprint of permafrost degradation caused by climate warming.
2.2 Water age module
In a hydrological system, water input/output variations directly affect the water fluxes, thereby influencing the water age. In the new CHANGE module, water ages in storages (i.e., canopy, snowpack, and soil) are estimated along the one-dimensional water flow direction, with a scheme (Figure 1) similar to that used for tracer flux calculation (Park et al., 2021). The volumetric water age (Ai) variation in the lower storage (i), following an input of water (Wi) from the upper storage (i−1) at time step (t), is expressed as:
where ai−1 is the water age in the upper storage, Fw,i is the water flux from the upper storage, △t is the time step, and t−1 represents the previous time step. Conversely, the water age variation in the upper storage, induced by an upward water flux from the lower storage, is calculated with Eq. 4 and 5 of Park et al. (2021).
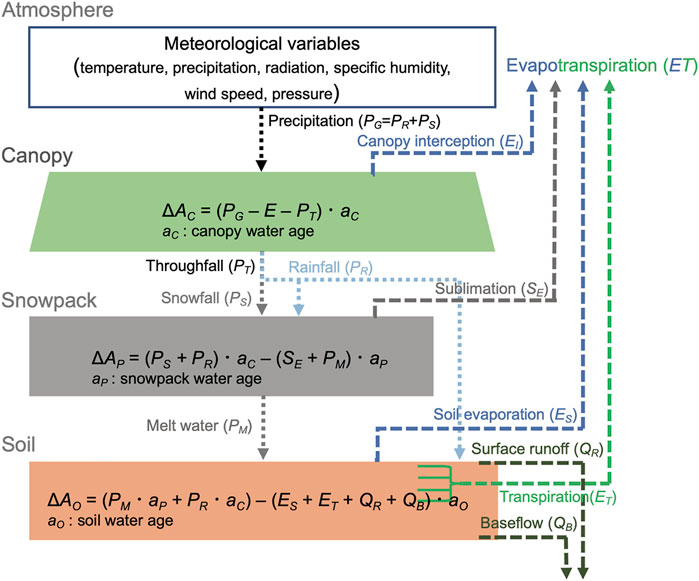
FIGURE 1. Schematic diagram of the water age calculation scheme for a one-dimensional water flow. Downward arrows represent water fluxes from upper to lower water storage pools, initiated in the atmosphere; upward arrows show water losses by evapotranspiration in individual storage pools.
The simplifying assumption is that the water flux between storages is fully mixed, because the model essentially considers bulk tracer content values in fully mixed storages. The water mixing assumption is also applied to water age. In the water age calculation, the precipitation input age is set to zero. For example, the age of water intercepted by a dry canopy is zero, and thus the drip and evaporation rates are also zero. However, the age of water remaining on the canopy increases after each time step, so that new precipitation results in a younger canopy water age. Soil evaporation and plant transpiration remove water from a storage. The volumetric water age for evaporation (AEi) from a storage (i) to the atmosphere at time step (t) is expressed as:
where Ew,i represents the evaporative flux from the lower storage. Eq. 4 is also used to calculate water age variations from plant transpiration, derived using a partitioning function, and runoff. The age of water extracted by plant roots in each soil layer depends on the model-simulated plant transpiration and on soil water age. Therefore, the water age for plant transpiration is obtained by integrating over the individual layers. Additionally, dew formation is treated as an additional water input, resulting in younger water ages in the storages. For the snowpack, however, sublimation from the snow surface limits the production of snow meltwater, thus the snow age decreases. The snowpack age is also influenced by snowmelt and snowfall events.
The water flow between components of the hydrological system (i.e., canopy, snow, and soil) in the land surface scheme occurs sequentially downward, from the canopy to the snow then to the soil (Figure 1). Water ages for individual components are updated at each time step depending on the water fluxes. In permafrost, there is no water flux, thus the water age continuously increases. The water age scheme also assumes that the seasonal freezing/thawing phase change in the active layer does not affect soil water age. However, when the active layer thickens, the water age of the new active layer is expectedly younger, because of mixing with water from the upper layers or soil water loss by subsurface outflow. In our simulations, the water age of each soil layer was initialized to zero, because age observation data for frozen water or permafrost ice were not available. Therefore, simulated water ages for the permafrost layers below the active layer were nearly identical.
2.3 Study site and observations
The study site is located in eastern Siberia (62.2°N, 128.5°E), approximately 20 km north of Yakutsk. Climatological records at the study site from 1998 to 2010 indicate an annual mean air temperature (Ta) of −10.4°C and an annual mean precipitation (PG) of 260 mm. Annual mean snowfall during winter (October–March) represents 40% of the annual mean precipitation, yielding a maximum snow depth of approximately 60 cm. Observations of the hydrometeorological variables at the study site were described by Kotani et al. (2019) and Hiyama et al. (2021). The study site is representative of a typical boreal forest landscape. A species of larch (Larix cajanderi) dominates the overstory, with a mean tree height of 18 m. The forest floor is covered by dense cowberry (Vaccinium vitis-idaea) and underlain by continuous, ice-rich permafrost. The study site has coarse humidified permafrost soils on heterogeneous sandy–loamy carbonate ground, textured by sand (74%), clay (15%), and loam (11%) with soil organic carbon content of 20 kg m−3.
Meteorological records at the study site cover a limited time period and include dates without observations. These issues limit the model applicability for long-term simulations. To complement the observation records, the meteorological data were combined with ERA-Interim reanalysis data from the European Centre for Medium-Range Weather Forecasts (Dee et al., 2011). Continuous daily data from ERA-Interim were selected to reflect the local climatic conditions and to construct the primary forcing data for CHANGE by assimilating the observations acquired at the study site. Generation of the daily forcing data was described by Miyazaki et al. (2015).
2.4 Model simulations
A static land cover type, the boreal deciduous needleleaf forest, was set up for the CHANGE simulations. Vegetation phenology and physiology were prognostically estimated from the model output for carbon and nitrogen contents. Vertical profiles of thermal and hydraulic soil parameters were explicitly estimated on the basis of the measured soil texture fractions, which were then updated at each time step to account for the simulated soil organic carbon variations. The spin-up CHANGE simulation was conducted over 1,200 years with the detrended forcing data of the first 20 years and a CO2 atmospheric concentration of 350 ppm. Dynamic equilibrium of the carbon and nitrogen contents for the total ecosystem was reached during the spin-up period. In this study, the hydrogen and oxygen isotopic ratios (δ2H and δ18O) were excluded from the modeling variables, because dedicated CHANGE simulation results for δ2H and δ18O have previously been analyzed and validated (Park et al., 2021). Water ages were initialized to zero in all soil layers then updated by water exchanges caused by precipitation and evapotranspiration events during the spin-up period, to minimize the influence of initialization values on the simulation results, at least in the active layer.
The warming of the Arctic climate, particularly significant in the cold seasons (Bekryaev et al., 2010), is a major forcing factor affecting terrestrial hydrological processes. The Arctic terrestrial air temperature has increased by approximately 1.5–2.0°C during the past 4 decades (Bekryaev et al., 2010; Park et al., 2017). The study site has also recorded extreme increases in summer precipitation of 30–70 mm during 2004–2008 relative to the average of 150 mm during 1979–2013 (Iijima et al., 2016). These rates of change were adopted as thresholds for investigating the model sensitivity to extreme climatic events as well as plant responses to such changes. To investigate the sensitivity of water age to climate warming, four simulations were conducted for the period 1980–2016, with the settings of the original simulation but with perturbed values of the air temperature and precipitation: a constant increase of the daily mean Ta by 2°C and 4°C (simulations EXT2 and EXT4, respectively), a combined increase of Ta by 2°C and PG by 30% (simulation EXT2C_P30), and a simulation based on EXT2 but with a root depth increased to 1.06 m from the original depth of 0.58 m (EXT2_RT). We also conducted an additional simulation to investigate the influence of autumn precipitation on evapotranspiration in the following year, also based on EXT2 but with PG values reduced by 30% in August–September. The simulation results were then compared with the reference simulation.
3 Results
3.1 Soil moisture and water age
Climatological daily averages (averages for the same day over the whole study period) were calculated from the simulation results from 1980 to 2016, for the ALT and for vertical profiles of the soil moisture and of the water age. Their seasonal variations are presented in Figure 2. The ALT results showed typical seasonality, with soil thawing starting in mid-April and reaching its maximum in early October (Figure 2A). In April, snow began to melt at the study site. Snow meltwater infiltrated the soil and saturated the surface layer down to a depth of approximately 0.1 m (Figure 2B). Surface layer water was displaced downward by incoming precipitation in the growing season, when the active layer develops. The maximum daily mean ALT remained within 1.4–1.9 m from 1980 to 2016. In summer, the soil depth of approximately 0.6 m was comparatively drier than the deeper layers, because of soil water loss caused by higher plant transpiration and soil evaporation (Park et al., 2021). In autumn, when evapotranspiration was quite low, the surface soil layer of 0.1 m depth was wetted again. Water remained in the surface layer as frozen water until the following spring (Figure 2B).
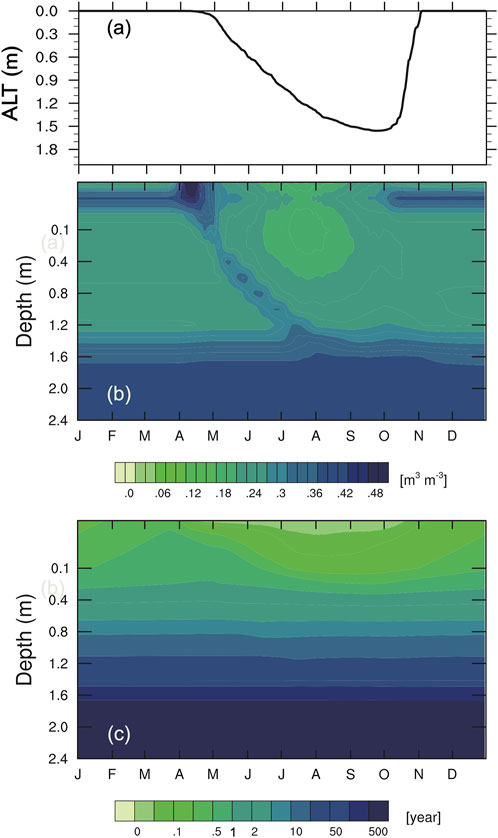
FIGURE 2. Seasonal variability, for the reference simulation, of climatological (1980–2016) daily averages for (A) the active layer thickness, and for the vertical profiles of (B) soil moisture and (C) water age. The vertical scale for the 0–0.4 m soil layer in Panels (B)–(C) is logarithmic.
The vertical profile of the simulated soil water age exhibited a clear monotonous distribution in all seasons, with younger ages in the surface layer and increasingly older ages with increasing depth (Figure 2C). At the study site, plant roots were primarily distributed within the 0–0.6 m surface soil column (Park et al., 2021). At these depths, water uptake by plant roots (Figure 2B) enhanced mixing of the existing water with fresh rainwater in summer, yielding younger water ages with a clear seasonal variability. Evaporation from the soil surface also increased water mixing and rejuvenation. The estimated water age in the surface soil column indicated full replacement of surface soil water by precipitation water within 1 year. Higher evapotranspiration also reduced infiltration of precipitation water into deeper soil layers. Thus, infiltration was effectively limited to depths of 0.8 m or less in summer (Figure 2B). The resulting limited mixing in deeper soil layers prevented rejuvenation and the stored water aged continuously. For example, the soil water age at a depth of 1.0 m was older than 10 years (Figure 2C). In permafrost, at depths of 1.9 m and below, water flow rarely occurred. The simulated water ages increased constantly, up to the length of the spin-up period (1,200 years).
3.2 Evapotranspiration and water age
Seasonal variations of the simulated climatological daily mean snow water equivalents (SWE), evapotranspiration subcomponents (plant transpiration, canopy interception and soil evaporation) separated according to the water sources (snow and rain), and the corresponding simulated water ages are displayed in Figure 3. The SWE showed typical seasonality, with accumulation starting in early autumn and reaching its maximum in March, concurrently with the maximum snow water age (80 days, Figure 3A). Snow began to melt at the end of March (Figure 3A), thus initiating soil evaporation (ES, Figure 3B) when the ES water was oldest (160 days), which reflected the water age of the surface soil layer. In April, the water age for soil evaporation sharply decreased to less than 20 days despite few rainfall events (Figure 3A), but a notable amount of water evaporated from the soil surface, explaining a surface soil layer age younger than in March (Figure 2C). From late April, the fraction of rain-sourced water evaporating from the surface markedly increased, while water ages decreased to less than 10 days in summer, indicating frequent precipitation events. Lower evapotranspiration and intermittent soil-freezing in October resulted in slightly older winter water ages for soil evaporation (Figure 3B). Annual mean water ages for interception by the vegetation canopy were considerably younger than those calculated for soil evaporation (Table 1).
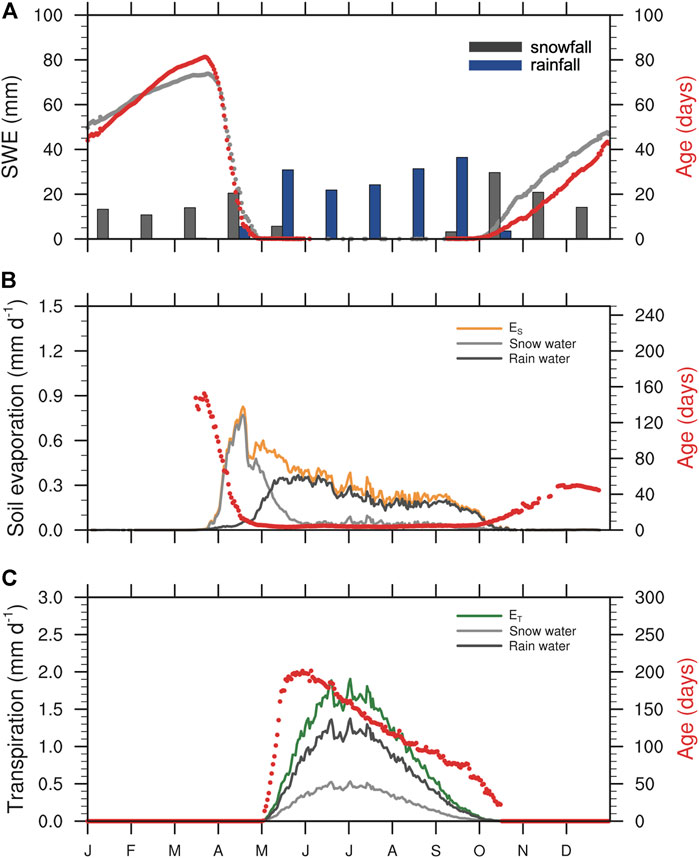
FIGURE 3. Seasonal variability, for the reference simulation, of climatological (1980–2016) daily averages for (A) the snow water equivalents (gray curve), and for the contributions of snowfall and rainfall to (B) soil evaporation and (C) plant transpiration. Daily mean water ages are indicated by red dots. Panel (A) also shows the climatological monthly average snowfall and rainfall amounts.
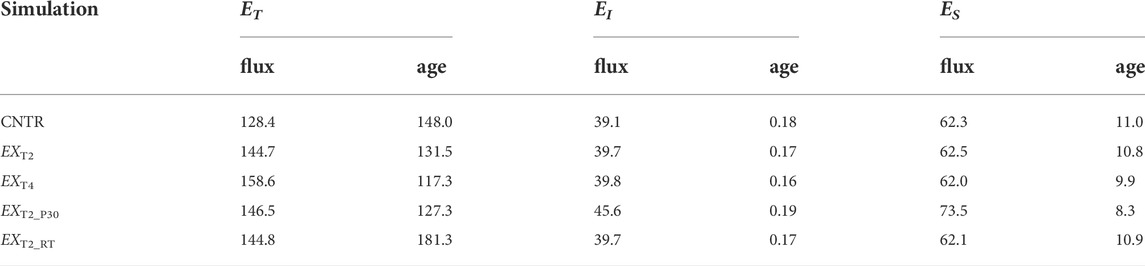
TABLE 1. Climatological (1980–2016) annual average water flux (mm yr−1) and age (days) for transpiration (ET), interception (EI), and soil evaporation (ES) in the reference and sensitivity simulations. Here, the averaged ages represent the daily flux-weighted annual median water ages for each evapotranspiration subcomponent.
Plnt roots extracted a large fraction of rain-sourced water from soil layers, accounting for 72% of the annual plant transpiration value (ET). The ET variations showed typical seasonality, peaking in June–July then decreasing, consistently with both the snow-sourced and rain-sourced transpiration values. In May, when the transpiration-induced water flux started, the corresponding water age increased rapidly. The deepening of the active layer in May allowed plant roots to reach deeper layers and extract older soil water. Subsequently, transpiration-induced water ages decreased continuously during the growing season. In turn, summer precipitation wetted the soil previously dried by higher plant transpiration. The alternating drying and wetting phases rejuvenated soil water in summer, explaining the relatively younger transpiration water age. Soil freezing in the cold season caused the younger soil water to age, capturing it until the following spring (Figure 2C). Daily flux-weighted annual median water ages, for transpiration and soil evaporation in the reference simulation, were 148 days and 11 days, respectively (Table 1). For comparison, previous model simulations yielded transpiration water ages of 200 ± 10 days and 150–360 days in northern Sweden (Smith et al., 2019) and in the Scottish Highlands (Kuppel et al., 2020), respectively.
3.3 Trends
In this study, trends were calculated with the Mann–Kendall test for the water age module variables shown in Figure 4 (blue symbols represent statistically significant values, p < 0.1). Air temperature is an important driver of Arctic hydrological processes. Statistically significant warming trends were identified in the climatological daily mean Ta values in spring, autumn, and winter (Figure 4A). The warmer Ta caused stronger sublimation and earlier snowmelt in spring, explaining the decreasing trend in the snow water age (Figure 4C). Snow meltwater saturated the surface soil layer (Figure 2B). Snow-sourced water contributed to soil evaporation in spring (Figure 4E) and to the decreasing trend of the corresponding water age in April (Figure 4G). There was no perceptible transpiration water age trend (values within ±0.1 day yr−1) in summer (Figure 4G). In October, when ES was lowest (Figure 3B), the soil evaporation water age showed a persistent, statistically significant negative trend (Figure 4G), indicating that water age was directly influenced by the increasing rainfall (Figure 4B).
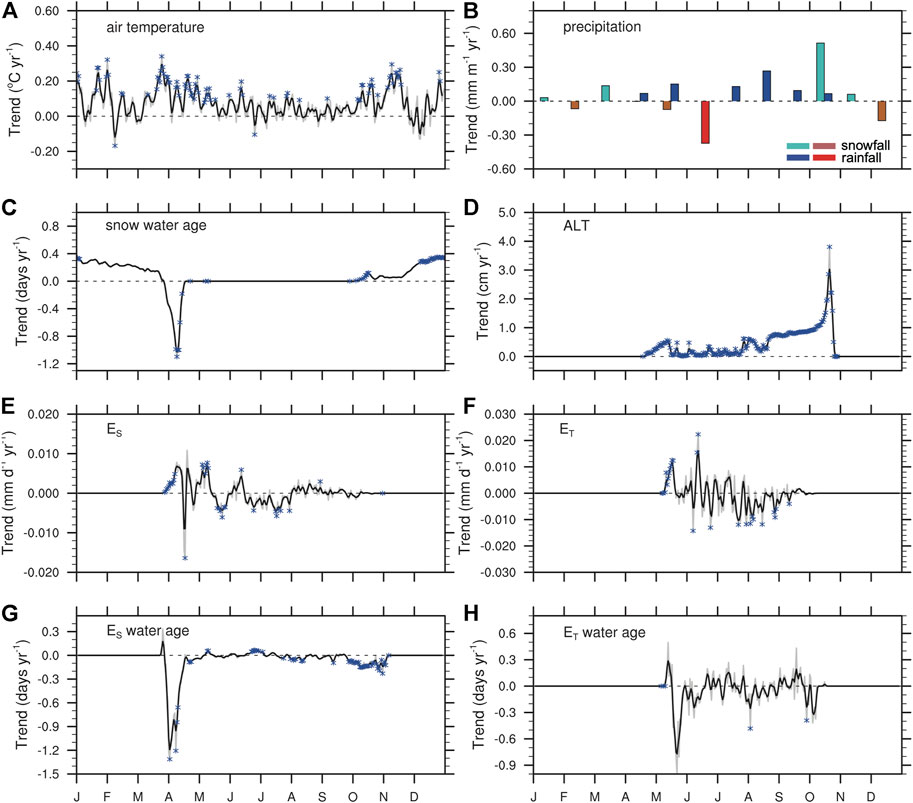
FIGURE 4. Seasonal variability, for the reference simulation, of climatological (1980–2016) daily average trends in (A) air temperature, (C) snow-sourced water age, (D) active layer thickness, (E) soil evaporation, (F) plant transpiration, (G) water age for soil evaporation, and (H) water age for plant transpiration. Panel (B) shows the climatological monthly average trends in snowfall and rainfall. The thin gray and thick black curves represent the original data and the 3-day average, respectively. Blue asterisks indicate statistically significant data (p < 0.1).
Once the snow cover had completely melted, the frozen soil began to thaw. The warmer Ta hastened soil thawing and induced a significant thickening trend in the active layer in summer, with a maximum ALT increase of 4 cm yr−1 in mid-October when the active layer extended to its maximum depth (Figure 4D). In spring, the active layer also thickened under the influence of the warming climate, which enhanced the ice–liquid water phase change. Under climate warming conditions, the phenological and physiological vegetation activity also increased, with plant roots extracting markedly more moisture from the soil (Parazoo et al., 2018). Plant transpiration exhibited a significant increasing trend in early May (Figure 4F), explained by the earlier start of plant transpiration and a deficit of snow meltwater (Figure 4B) under warmer Ta conditions, resulting in older ET water ages. In late May, the ET water age became rapidly younger (Figure 4H), because the increasing rainfall (Figure 4B) enhanced the mixing of young rain water with older soil moisture. Summer climatological trends of daily mean ET and of ET water age (Figures 4F,H, respectively) were alternately positive and negative, reflecting the influence of precipitation events.
Warming air temperatures also modified the soil freezing and thawing dates at the study site. In this study, freezing and thawing dates in the 0–5 cm topmost soil column were determined with the method of Park et al. (2016b). The simulated spring thawing date showed an overall negative trend of −0.21 days yr−1 (p < 0.04) over the 1980–2016 study period (Figure 5), indicating earlier soil thawing in response to increasing Ta (Figure 4A). The Ta warming trend also delayed soil freezing significantly (0.35 days yr−1, p < 0.01). As a consequence of earlier soil thawing and later soil freezing, the growing season length (defined as the number of non-freezing days in 1 year) showed a statistically significant (p < 0.01) increasing trend over the study period (Figure 5), which resultantly contributed to the annual evapotranspiration (sum of ES and ET) increasing trends (r = 0.19, p < 0.28; Ma et al., 2022).
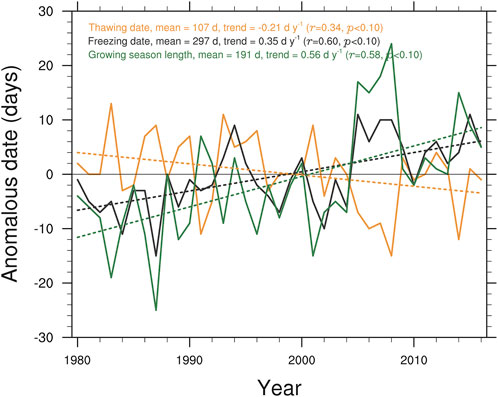
FIGURE 5. Interannual variability (1980–2016) of the simulated freezing and thawing anomalous dates and of the growing season length (yearly number of non-freezing days), and corresponding temporal trends (dashed lines) in the reference simulation.
3.4 Sensitivity simulations
Four sensitivity simulations, characterized by specific perturbations of Ta and PG and described in Section 2.4, were conducted to investigate the influence of climate warming on evapotranspiration and water age within the hydrological system. Figure 6 shows the seasonal variability of the anomalies, defined as the differences between the sensitivity simulation results and the reference simulation. Warmer air temperatures (+2°C and +4°C for Ta in simulations EXT2 and EXT4, respectively) enhanced sublimation from the snowpack while reducing the contribution of snowfall to PG, explaining lower SWE during the cold season (October–March) and earlier spring snowmelt than in the reference simulation (Figure 6A). As expected, a precipitation increase (+30% for PG, simulation EXT2_P30) compensated the influence of warmer Ta on the snowpack, resulting in positive SWE anomalies (Figure 6A). Modifications of the snow-related processes relatively to the reference simulation yielded consistently larger ES values and earlier start dates in spring (Figure 6C), and younger ES water ages (Figure 6E). Warmer Ta also induced an ET increase caused by earlier vegetation activity (Figure 6D). Conversely, this enhanced activity dried the surface soil layer (Figure 6B), explaining the negative ES anomalies in summer (Figure 6C). Soil dryness (magnitude of the negative anomaly) was directly related to the Ta increase, as illustrated by the larger negative anomalies for simulation EXT4 (Figure 6B), combined with higher losses by canopy interception (EI in Table 1). Similarly to its influence on the snowpack, a PG increase (EXT2_P30) could compensate the summer soil drying induced by higher Ta values (Figure 6B). Furthermore, under climate warming conditions, plants largely used rain-sourced soil water for transpiration, yielding larger ET anomalies for larger temperature increases, as shown in Figure 3C.
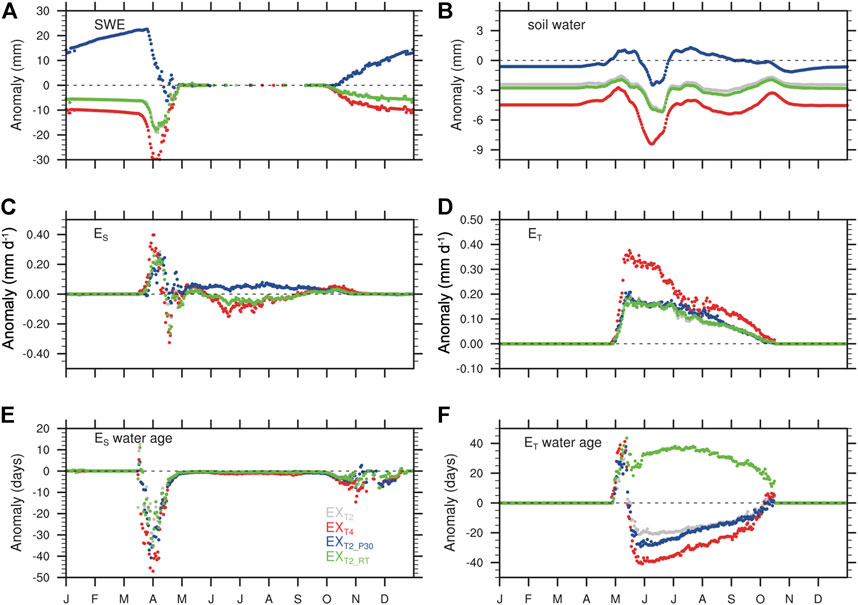
FIGURE 6. Seasonal variability (1980–2016) of daily average anomalies (differences between the sensitivity simulation results and the reference simulation) for (A) the snow water equivalents, (B) the 0–1 m soil column water content, (C) soil evaporation, (D) plant transpiration, (E) water age for soil evaporation, and (F) water age for plant transpiration.
Earlier vegetation phenological activity caused by warmer Ta was also closely implicated to earlier and deeper soil thawing in spring. The resulting increase in plant water extraction from the active layer explained the anomalously older ET water age in early spring (Figure 6F). This higher ET quickly dried the soil (Figure 6B), which was then wetted again by increased precipitation. Therefore, under warming conditions, ET was associated with anomalously younger water ages (Figure 6F). Climate warming possibly causes the root biomass and the rooting depth to increase. To investigate the influence of root depth on water ages, a fourth simulation was conducted on the basis of EXT2, but with a root depth extended to 1.06 m (EXT2_RT). This resulted in anomalously older ET water ages (Figure 6F), because a downward water flux generally requires longer transit time to reach deeper soil layers.
4 Discussion
In this study, the CHANGE model was coupled with a water tracer module to assess the influence of climate warming on evapotranspiration regimes and the subsequent soil water budget variations in a boreal forest. The tracer module, originally designed to isolate the contributions of each water source to hydrological processes, was modified to incorporate water age dynamics. Dedicated model simulations were conducted to quantify the influence of climate warming on water fluxes–storages–ages interactions in the evapotranspiration process.
4.1 Influence of climate warming on evapotranspiration and water age
At the study site, the climate-induced evapotranspiration increase was larger in spring than in autumn, despite consistent Ta increases in both seasons (Figure 4A), possibly because solar radiation levels are higher in spring than in autumn (nearly reaching the yearly maximum) and because vegetation transitions from winter dormancy to the onset of the active growing season. The earlier start of the growing season, induced by warmer air temperatures, relaxes the low-temperature constraints on photosynthesis and on gas and water exchanges on the canopy, and enhances plant growth (Parazoo et al., 2018). Earlier vegetation activity and soil thawing allow plant roots to access soil water earlier in spring, yielding larger ET values (Figure 6D). These findings are generally consistent with a previous study that demonstrated high correlation between the spring thawing date and the evapotranspiration intensity, using freezing/thawing records derived from satellite-based microwave remote sensing observations in the Northern Hemisphere high latitudes (Zhang et al., 2011). The positive influence of the earlier growing season onset on evapotranspiration was reinforced by the increase in snow meltwater availability, which limited plant moisture stress. The inflow of snow-sourced water into the surface soil layer showed a rejuvenating trend, explained by a shorter snowpack period and increased sublimation. The shorter freezing period (Figure 5) and the larger spring rainfall (Figure 4B) both contributed to surface soil water rejuvenation. Consequently, water ages for the spring evapotranspiration showed a decreasing trend over the study period (Figures 4G,H).
Conversely, this earlier and larger evapotranspiration in spring enhanced soil water loss, thus soil drying, in early summer (Figure 6B). The warming-driven leaf growth enhancement resulted in a larger quantity of canopy leaves that increased water losses by interception, thereby decreasing precipitation throughfall and causing the soil to dry. The low-soil-moisture constraint on evapotranspiration generally induced daily negative trends in summer (Figures 4E,F). However, rainwater from precipitation events, after reaching the dried soil, mixed with the older soil water. In turn, the mixed water was used for evapotranspiration (Tetzlaff et al., 2021), which explains the younger water ages calculated for soil evaporation and plant transpiration. This feedback cycle was enhanced under warming conditions, as confirmed by the sensitivity simulations in which warmer Ta induced considerable ES and ET increases (Figures 6C,D). Soil dryness is projected to increase further under future climate warming conditions (Andresen et al., 2020). Thus, more precipitation water will be consumed by plant transpiration, resulting in younger ET water ages (Figure 6F). In autumn, plants enter the dormancy or senescence stage. In this case, the influence of warmer air temperatures or larger precipitation on photosynthesis and plant transpiration is mitigated by the seasonally lower solar radiation and shorter photoperiod. Warmer autumn air temperature delayed snow accumulation and soil freezing (Figure 5). This delay also contributed to the decreasing age trends calculated for soil water and plant transpiration in the following spring. Our results suggest that subarctic climate warming has accelerated the terrestrial water cycle by increasing same-season use of precipitation-sourced young water for evapotranspiration.
4.2 Influence of permafrost warming on evapotranspiration and water age
Soil freezing in winter increased soil water ages (Figure 2B). This was reflected in the early spring ES variations when mixing of the frozen, older soil water with younger snow meltwater and rain water induced a rapid decrease of the water ages for the surface soil layer (Figure 2B) and the ES (Figure 3B). Conversely, seasonal variability of the ET water ages was completely different, particularly at the onset of plant transpiration in May, with ET values reaching their maximum within 1 month (Figure 3C). In CHANGE, the soil moisture stress associated with drying or freezing is represented by a normalized parameter (values within 0–1) and coupled to the plant photosynthetic and physiological processes. The ET water age variations in the early growing season reflected the reduction of soil freezing stress after the active layer developed (Figure 3C). This was inconsistent with the ET water age variation pattern identified in a non-permafrost Scottish Highlands site, characterized by winter soil frost and snow cover (Smith et al., 2019). On the contrary, the pattern identified by Smith et al. (2019) showed similar seasonality with our ES water age estimates. The maximum ET water age (approximately 200 days, Figure 3C), reached at the start of the growing season at the study site, clearly showed a connection between plant roots and the water accumulated during the preceding autumn. The ET water ages then continuously decreased over the growing season, indicating the ecohydrological transition to a rainfall-driven phase during which the surface soil layer water storage was recharged by summer rainfall. Rain-sourced water accounted for a large fraction of the ET water (Figure 3C). Analyzing the seasonal variability of ET water ages has provided valuable insights into water dynamics associated with the active layer freezing/thawing process. It has also contributed to uncertainty reduction and better characterization of the relative contributions of model-estimated water sources to the ET water. These contributions had not been validated with observational data prior to this study.
Winter freezing preserves the soil water conditions, established in the preceding autumn, until the following spring. This preserved water status represents the initial conditions for the growing season and strongly influences the related ecohydrological processes. An additional simulation was conducted to investigate the influence of autumn precipitation on evapotranspiration in the following year, with PG values reduced by 30% in August–September based on EXT2. Differences with the reference simulation were consistently negative during the growing season (Figure 7A). The lower PG values reduced the ET by 4.2% relatively to the reference values, demonstrating the influence of autumn precipitation on the ET water ages. Monthly mean ET ages differences (Figure 7B) between the reference (solid curves) and the additional simulation (dashed curves) were mostly found in soil layers below the surface but not deeper than 0.8 m (Figure 7B). In summer, ET and ES often caused the surface soil layer to dry. The remaining surface soil moisture was nearly fully displaced by the frequent precipitation inflow. This consistently occurs in the reference and additional simulations, regardless of the soil moisture level in early spring, consequently low ET water age differences. In the additional simulation, the drier soil probably enhanced mixing of soil water with younger precipitation water infiltrated from the upper layers (Park et al., 2021). Water mixing and mobilization produced younger soil water in soil layers below the surface layer (Figure 7B). The presence of younger ET water under a dried soil layer was broadly verified by the temperature-only sensitivity simulations EXT2 and EXT4 (Figure 6F). These results suggest that autumn precipitation water trapped in the soil by winter freezing causes deviations of the evapotranspiration and water ages from the hydrometeorological forcings in the following growing season. Sugimoto et al. (2003) established, in a larch forest of eastern Siberia, that the autumn soil water stored in the 0–1.2 m active layer column contributed to ET in the following summer. A 1-year delayed correlation between soil water and evapotranspiration was also identified at the Kolyma watershed in eastern Siberia, underlain by continuous permafrost (Zhang et al., 2019; Suzuki et al., 2021). However, the warmer temperature increases permafrost thawing, leading to wetted surface layers and thus greater evapotranspiration. The increased evapotranspiration ultimately causes a drier surface, which is in turn fed back as a limitation on evapotranspiration. This feedback might become strengthened under the influence of future climate change. Climate models have projected long-term drying of the surface soil in permafrost regions (Andresen et al., 2020).
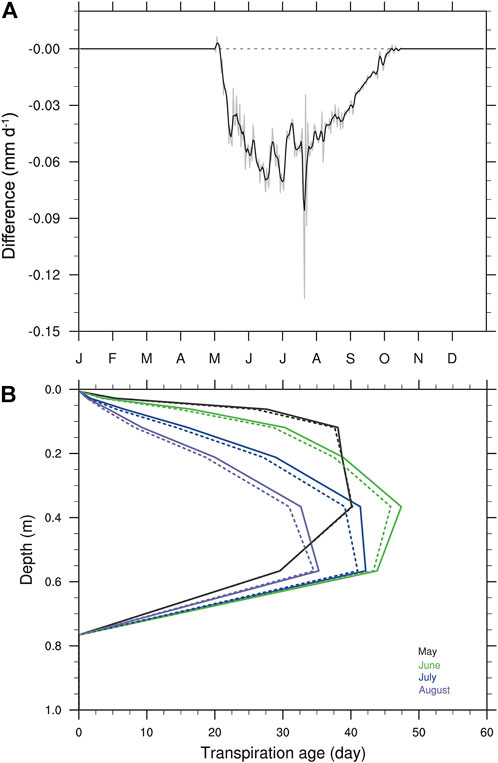
FIGURE 7. Comparison between the reference simulation and a simulation with a 30% reduction in August–September precipitation for (A) the seasonal variability (1980–2016) of daily average plant transpiration anomalies and (B) monthly average vertical profiles of plant transpiration water ages.
4.3 Model limitations and scope of the study
Previous observational studies investigated the climate-induced winter snow increase at the same study site and the influence of the resulting soil wetness on vegetation activity and on evapotranspiration (Ohta et al., 2014; Kotani et al., 2019). In CHANGE, ecohydrological interactions are reproduced by a numerical model that includes a water tracer module to analyze the dynamics of precipitation-sourced water storages in the surface–subsurface system. The model calculated high contributions of summer rainwater to the increased evapotranspiration at the study site and characterized the evaporation-induced water isotopic enrichment, validated by observational data (Park et al., 2021). However, in this study, although a lack of observational data for water flux age calculations prevented us from validating the simulated evapotranspiration water ages, the coupling of the new water age calculation module with CHANGE produced encouraging results. The seasonal variability of evapotranspiration water ages was clearly associated with the active layer freezing/thawing process.
Simulated soil water ages were older in the deeper layers, because of the low water exchange at these depths (Figure 2). There was no indication of water movement by capillary rise from the deeper soil layers toward the upper layers under current climate conditions, because moisture conditions within the upper layers remained beyond the wilting point in summer (Figure 2B). This result was also verified for future climate warming scenarios. In the EXT4 simulation, for example, anomalous ET water ages were younger than in the reference simulation (Figure 6F), indicating a larger dependence of ET on summer precipitation than on water upwelling from deep soil layers. Moreover, when considering a plant root depth larger by 50 cm than that of the reference simulation (simulation EXT2_RT), anomalous ET water ages remained approximately 20 days older than the reference throughout the growing season (Figure 6F) as a result of increased extraction by the plant roots of older water from deeper soil layers. Evidence of the root depth influence was also identified by comparing the EXT2 and EXT2_RT simulations. The comparison showed ET water age differences of approximately 40 days despite an identical Ta increase of 2°C in both simulations (Figure 6F). Under future climate warming scenarios, root depth extension, larger spatial heterogeneities, and ALT increase are nearly certain. In such conditions, increased connection between plant transpiration and permafrost-sourced water is expected. Therefore, the root profile, as currently configured, is a likely source of uncertainties in simulations of ecohydrological processes associated with the model-simulated water sources and ages. Furthermore, the Ta and PG perturbation defined in our simulations are not sufficient to characterize future climate warming conditions, which also depend on complex variations of meteorological variables such as humidity, surface solar radiation, or wind speed (Carvalho et al., 2022). In that sense, the sensitivity simulations presented in this study are not sufficiently realistic for accurate projections of evapotranspiration and water ages under future climate warming scenarios. Nevertheless, the main objective of this study was not the evaluation of future projections for evapotranspiration and water ages, but the analysis of evapotranspiration and water age responses to current climate warming conditions. Therefore, uncertainties on the forcing data should not preclude meaningful analyses with the newly implemented water age calculation scheme.
5 Conclusion
We incorporated a new water age calculation scheme into the tracer module of the process-based land surface model CHANGE and examined the influence of hydrological process modifications induced by permafrost warming on evapotranspiration and water ages. Climate warming caused earlier snowmelt and soil thawing, thereby enhancing soil evaporation and plant transpiration that, in turn, consumed increasingly younger water in spring. The progressively younger water ages for evapotranspiration in summer and autumn illustrated the dependence of the evapotranspiration on precipitation, because of the drying soil. These findings were consistent with our sensitivity model simulations. We also established that winter soil freezing contributed to summer plant transpiration by storing older soil water from the preceding autumn. However, climate warming shortened the freezing period. Thus, the early spring flux water ages became gradually younger. Our results demonstrated that climate warming strengthened the connection between evapotranspiration and precipitation-sourced freshwater in the soil system. Future climate-induced temperature increases will enhance permafrost warming and increase the contribution of summer rainfall to precipitation, also resulting in larger evapotranspiration. Therefore, we expect that future evapotranspiration increases will further accelerate the water cycle in boreal forests, with increasingly larger loss of younger precipitation water, a phenomenon that we identified under current climate conditions.
Data availability statement
The raw data supporting the conclusion of this article will be made available by the authors, without undue reservation.
Author contributions
HP developed the ideas, wrote most of this paper and drew most of the figures. All authors participated in data processing and preliminary analysis; HP coordinated the model experiments and analyzed the simulation results, KS and TH contributed the result analysis and manuscript writing and discussion.
Funding
This study was partly supported by the Japan Society for the Promotion of Science Grants-in-Aid for Scientific Research (KAKENHI) funding program, Grant Numbers 19H05668, 21H04934, and 22H03758. It was also supported by the Belmont Forum of the Japan Science and Technology Agency, Grant Number JPMJBF2003, and the Arctic Challenge for Sustainability II project funded by the Ministry of Education, Culture, Sports, Science and Technology, Japan, Grant Number JPMXD1420318865.
Acknowledgments
We thank Eric Dupuy, from Edanz (https://jp.edanz.com/ac) for editing a draft of this manuscript.
Conflict of interest
The authors declare that the research was conducted in the absence of any commercial or financial relationships that could be construed as a potential conflict of interest.
The reviewer NM declared a past co-authorship with the author HP to the handling editor.
Publisher’s note
All claims expressed in this article are solely those of the authors and do not necessarily represent those of their affiliated organizations, or those of the publisher, the editors and the reviewers. Any product that may be evaluated in this article, or claim that may be made by its manufacturer, is not guaranteed or endorsed by the publisher.
References
Ala-aho, P., Tetzlaff, D., McNamara, J. P., Laudon, H., Kormos, P., and Soulsby, C. (2018). Modeling the isotopic evolution of snowpack and snowmelt: Testing a spatially distributed parsimonious approach. Water Resour. Res. 53, 5813–5830. doi:10.1002/2017WR020650
Ala-aho, P., Tetzlaff, D., McNamara, J. P., Laudon, H., and Soulsby, C. (2017). Using isotopes to constrain water flux and age estimates in snow-influenced catchments using the STARR (Spatially distributed Tracer-Aided Rainfall–Runoff) model. Hydrol. Earth Syst. Sci.BSyst. Sci. 21, 5089–5110. doi:10.5194/hess-21-5089-2017
Andresen, C. G., Lawrence, D. M., Wilson, C. J., McGuire, A. D., Koven, C., Schaefer, K., et al. (2020). Soil moisture and hydrology projections of the permafrost region – A model intercomparison. Cryosphere 14, 445–459. doi:10.5194/tc-14-445-2020
Bekryaev, R. V., Polyakov, I. V., and Alexeev, V. A. (2010). Role of polar amplification in long-term surface air temperature variations and modern Arctic warming. J. Clim. 23, 3888–3906. doi:10.1175/2010jcli3297.1
Birkel, C., and Soulsby, C. (2015). Advancing tracer-aided rainfall-runoff modelling: A review of progress, problems and unrealised potential. Hydrol. Process. 29, 5227–5240. doi:10.1002/hyp.10594
Biskaborn, B. K., Smith, S. L., Noetzli, J., Matthes, H., Vieira, G., Streletskiy, D. A., et al. (2019). Permafrost is warming at a global scale. Nat. Commun. 10, 264. doi:10.1038/s41467-018-08240-4
Carvalho, D., Rafael, S., Monteiro, A., Rodrigues, V., Lopes, M., and Rocha, A. (2022). How well have CMIP3, CMIP5 and CMIP6 future climate projections portrayed the recently observed warming. Sci. Rep. 12, 11983. doi:10.1038/s41598-022-16264-6
Dee, D. P., Uppala, S. M., Simmons, A. J., Berrisford, P., Poli, P., Kobayashi, S., et al. (2011). The ERA-Interim reanalysis; configuration and performance of the data assimilation system. Q. J. R. Meteorol. Soc. 137, 553–597. doi:10.1002/qj.828
Good, S. P., Noone, D., and Bowen, G. J. (2015). Hydrologic connectivity constrains partitioning of global terrestrial water fluxes. Science 349, 175–177. doi:10.1126/science.aaa5931
Hiyama, T., Ueyama, M., Kotani, A., Iwata, H., Nakai, T., Okamura, M., et al. (2021). Lessons learned from more than a decade of greenhouse gas flux measurements at boreal forests in eastern Siberia and interior Alaska. Polar Sci. 27, 100607. doi:10.1016/j.polar.2020.100607
Holmes, R. M., Shiklomanov, A. I., Tank, S. E., McClelland, J. W., and Tretiakov, M. (2015). River discharge. Arct. Rep. Card. 2015, 60–65.
Hrachowitz, M., Benettin, P., van Breukelen, B. M., Fovet, O., Howden, N. J. K., Ruiz, L., et al. (2016). Transit times – The link between hydrology and water quality at the catchment scale. WIREs. Water 3, 629–657. doi:10.1002/wat2.1155
Iijima, Y., Nakamura, T., Park, H., Tachibana, Y., and Fedorov, A. (2016). Enhancement of Arctic storm activity in relation to permafrost degradation in eastern Siberia. Int. J. Climatol. 36, 4265–4275. doi:10.1002/joc.4629
Kim, Y., Kimball, J. S., Robinson, D. A., and Derksen, C. (2015). New satellite climate data records indicate strong coupling between recent frozen season changes and snow cover over high northern latitudes. Environ. Res. Lett. 10, 084004. doi:10.1088/1748-9326/10/8/084004
Kotani, A., Saito, A., Kononov, A. V., Petrov, R. E., Maximov, T. C., Iijima, Y., et al. (2019). Impact of unusually wet permafrost soil on understory vegetation and CO2 exchange in a larch forest in eastern Siberia. Agric. For. Meteorol. 265, 295–309. doi:10.1016/j.agrformet.2018.11.025
Kuppel, S., Tetzlaff, D., Maneta, M. P., and Soulsby, C. (2020). Critical zone storage controls on the water ages of ecohydrological outputs. Geophys. Res. Lett. 47, e2020GL088897. doi:10.1029/2020GL088897
Kuppel, S., Tetzlaff, D., Maneta, M. P., and Soulsby, C. (2018). EcH&lt;sub&gt;2&lt;/sub&gt;O-iso 1.0: Water isotopes and age tracking in a process-based, distributed ecohydrological model. Geosci. Model Dev. 11, 3045–3069. doi:10.5194/gmd-11-3045-2018
Li, C., Wei, Y., Liu, Y., Li, L., Peng, L., Chen, J., et al. (2022). Active layer thickness in the northern Hemisphere: Changes from 2000 to 2018 and future simulations. J. Geophys. Res.-Atmosphere 127, e2022JD036785. doi:10.1029/2022JD036785
Ma, N., Szilagyi, J., and Zhang, Y. (2022). Calibration-free complementary relationship estimates terrestrial evapotranspiration globally. Water Resour. Res. 57, e2021WR029691. doi:10.1029/2021WR029691
Ma, N., and Zhang, Y. (2022). Increasing Tibetan Plateau terrestrial evapotranspiration primarily driven by precipitation. Agric. For. Meteorol. 317, 108887. doi:10.1016/j.agrformet.2022.108887
Maneta, M. P., and Silverman, N. L. (2013). A spatially distributed model to simulate water, energy, and vegetation dynamics using information from regional climate models. Earth Interact. 17, 1–44. doi:10.1175/2012ei000472.1
Miyazaki, S., Saito, K., Mori, J., Yamazaki, T., Ise, T., Arakida, H., et al. (2015). The GRENE-TEA model intercomparison project (GTMIP): Overview and experiment protocol for stage 1. Geosci. Model Dev. 8, 2841–2856. doi:10.5194/gmd-8-2841-2015
Ohta, T., Kotani, A., Iijima, Y., Maximov, T. C., Ito, S., Hanamura, M., et al. (2014). Effects of waterlogging on water and carbon dioxide fluxes and environmental variables in a Siberian larch forest, 1998–2011. Agric. For. Meteorol. 188, 64–75. doi:10.1016/j.agrformet.2013.12.012
Parazoo, N. C., Arneth, A., Pugh, T. A. M., Smith, B., Steiner, N., Luus, K., et al. (2018). Spring photosynthetic onset and net CO2 uptake in Alaska triggered by landscape thawing. Glob. Chang. Biol. 24, 3416–3435. doi:10.1111/gcb.14283
Park, H., Iijima, Y., Yabuki, H., Ohta, T., Walsh, J., Kodama, Y., et al. (2011). The application of a coupled hydrological and biogeochemical model (CHANGE) for modeling of energy, water, and CO2 exchanges over a larch forest in eastern Siberia. J. Geophys. Res. 116, D15102. doi:10.1029/2010JD015386
Park, H., Kim, Y., and Kimball, J. S. (2016a). Widespread permafrost vulnerability and soil active layer increases over the high northern latitudes inferred from satellite remote sensing and process model assessments. Remote Sens. Environ. 175, 349–358. doi:10.1016/j.rse.2015.12.046
Park, H., Launiainen, S., Konstantinov, P. Y., Iijima, Y., and Fedorov, A. N. (2018). Modeling the effect of moss cover on soil temperature and carbon fluxes at a tundra site in Northeastern Siberia. J. Geophys. Res.-Biogeosciences 123, 3028–3044. doi:10.1029/2018JG004491
Park, H., Tanoue, M., Sugimoto, A., Ichiyanagi, K., Iwahana, G., and Hiyama, T. (2021). Quantitative separation of precipitation and permafrost waters used for evapotranspiration in a boreal forest: A numerical study using tracer model. JGR. Biogeosciences 126, e2021JG006645. doi:10.1029/2021JG006645
Park, H., Yoshikawa, Y., Oshima, K., Kim, Y., Ngo-Duc, T., Kimball, J. S., et al. (2016b). Quantification of warming climate-induced changes in terrestrial Arctic river ice thickness and phenology. J. Clim. 29, 1733–1754. doi:10.1175/JCLI-D-15-0569.1
Park, H., Yoshikawa, Y., Yang, D., and Oshima, K. (2017). Warming water in Arctic terrestrial rivers under climate change. J. Hydrometeorol. 18, 1983–1995. doi:10.1175/JHM-D-16-0260.1
Pfister, L., Martínez-Carreras, N., Hissler, C., Klaus, J., Carrer, G. E., Stewart, M. K., et al. (2017). Bedrock geology controls on catchment storage, mixing and release: A comparative analysis of 16 nested catchments. Hydrol. Process. 31, 1828–1845. doi:10.1002/hyp.11134
Piovano, T. I., Tetzlaff, D., Carey, S. K., Shatilla, N. J., Smith, A., and Soulsby, C. (2019). Spatially distributed tracer-aided runoff modelling and dynamics of storage and water ages in a permafrost-influenced catchment. Hydrol. Earth Syst. Sci. 23, 2507–2523. doi:10.5194/hess-23-2507-2019
Rinaldo, A., Benettin, P., Harman, C. J., Hrachowitz, M., McGuire, K. J., van der Velde, Y., et al. (2015). Storage selection functions: A coherent framework for quantifying how catchments store and release water and solutes. Water Resour. Res. 51, 4840–4847. doi:10.1002/2015WR017273
Smith, A., Tetzlaff, D., Laudon, H., Maneta, M., and Soulsby, C. (2019). Assessing the influence of soil freeze–thaw cycles on catchment water storage–flux–age interactions using a tracer-aided ecohydrological model. Hydrol. Earth Syst. Sci. 23, 3319–3334. doi:10.5194/hess-23-3319-2019
Sprenger, M., Herbstritt, B., and Weiler, M. (2015). Established methods and new opportunities for pore water stable isotope analysis. Hydrol. Process. 29, 5174–5192. doi:10.1002/hyp.10643
Sprenger, M., Stumpp, C., Weiler, M., Aeschbach, W., Allen, S. T., Benettin, P., et al. (2019). The demographics of water: A review of water ages in the critical zone. Rev. Geophys. 57, 800–834. doi:10.1029/2018RG000633
Sprenger, M., Tetzlaff, D., Buttle, Z., Laudon, H., and Soulsby, C. (2018). Water ages in the critical zone of long-term experimental sites in northern latitudes. Hydrol. Earth Syst. Sci. 22, 3965–3981. doi:10.5194/hess-22-3965-2018
Stadnyk, T., Delavau, C., Kouwen, N., and Edwards, T. W. D. (2013). Towards hydrological model calibration and validation: Simulation of stable water isotopes using the isoWATFLOOD model. Hydrol. Process. 27, 3791–3810. doi:10.1002/hyp.9695
Sugimoto, A., Naito, D., Yanagisawa, N., Ichiyanagi, K., Kurita, N., Kubota, J., et al. (2003). Characteristics of soil moisture in permafrost observed in East Siberian taiga with stable isotopes of water. Hydrol. Process. 17, 1073–1092. doi:10.1002/hyp.1180
Suzuki, K., Park, H., Makarieva, O., Kanamori, H., Hori, M., Matsuo, K., et al. (2021). Effect of permafrost thawing on discharge of the Kolyma river, northeastern Siberia. Remote Sens. (Basel). 13, 4389. doi:10.3390/rs13214389
Tetzlaff, D., Birkel, C., Dick, J., Geris, J., and Soulsby, C. (2014). Storage dynamics in hydropedological units control hillslope connectivity, runoff generation and the evolution of catchment transit time distributions. Water Resour. Res. 50, 969–985. doi:10.1002/2013WR014147
Tetzlaff, D., Buttle, J., Carey, S. K., Kohn, M. J., Laudon, H., McNamara, J. P., et al. (2021). A preliminary assessment of water partitioning and ecohydrological coupling in northern headwaters using stable isotopes and conceptual runoff models. Hydrol. Process. 35, 5153–5173. doi:10.1002/hyp.10515
van Huijgevoort, M. H. J., Tetzlaff, D., Sutanudjaja, E. H., and Soulsby, C. (2016). Using high resolution tracer data to constrain water storage, flux and age estimates in a spatially distributed rainfall-runoff model. Hydrol. Process. 30, 4761–4778. doi:10.1002/hyp.10902
Wang, P., Huang, Q., Pozdniakov, S. P., Liu, S., Ma, N., Wang, T., et al. (2021). Potential role of permafrost thaw on increasing Siberian river discharge. Environ. Res. Lett. 16, 034046. doi:10.1088/1748-9326/abe326
Zhang, K., Kimball, J. S., Kim, Y., and McDonald, K. C. (2011). Changing freeze-thaw seasons in northern high latitudes and associated influences on evapotranspiration. Hydrol. Process. 30, 4142–4151. doi:10.1002/hyp.8350
Zhang, Y., He, B. I. N., Guo, L., and Liu, D. (2019). Differences in response of terrestrial water storage components to precipitation over 168 global river basins. J. Hydrometeorol. 20, 1981–1999. doi:10.1175/jhm-d-18-0253.1
Zhang, Y., Ma, N., Park, H., Walsh, J. E., and Zhang, K. (2021). “Evaporation processes and changes over the northern regions,” in Arctic hydrology, permafrost and ecosystems. Editors D. Yang, and D. L. Kane (Springer Nature Switzerland), 101–131. doi:10.1007/978-3-030-50930-9_4
Keywords: evapotranspiration, permafrost, water age, tracer module, climate warming
Citation: Park H, Hiyama T and Suzuki K (2022) Contribution of water rejuvenation induced by climate warming to evapotranspiration in a Siberian boreal forest. Front. Earth Sci. 10:1037668. doi: 10.3389/feart.2022.1037668
Received: 06 September 2022; Accepted: 13 October 2022;
Published: 31 October 2022.
Edited by:
Chunqiao Song, Nanjing Institute of Geography and Limnology (CAS), ChinaReviewed by:
Haohao Wu, Nanjing Institute of Geography and Limnology (CAS), ChinaNing Ma, Institute of Geographic Sciences and Natural Resources Research(CAS), China
Copyright © 2022 Park, Hiyama and Suzuki. This is an open-access article distributed under the terms of the Creative Commons Attribution License (CC BY). The use, distribution or reproduction in other forums is permitted, provided the original author(s) and the copyright owner(s) are credited and that the original publication in this journal is cited, in accordance with accepted academic practice. No use, distribution or reproduction is permitted which does not comply with these terms.
*Correspondence: Hotaek Park, park@jamstec.go.jp