- 1Guangdong Open Laboratory of Geospatial Information Technology and Application, Guangzhou Institute of Geography, Guangdong Academy of Sciences, Guangzhou, China
- 2Guangdong Geological Disaster Emergency Technology Research Center, Guangzhou, China
Because of the physical character of soil from granite weathering and the typhoon rains in South China, the model for early warnings of existing shallow landslides cannot be well applied in that area. This study developed a new shallow landslide model based on the effect of soil macropores for determining the rainfall threshold to post an early warning of the possibility of a shallow landslide in South China. We studied the hydrological effects of macropore flow and proposed a mechanistic model of the formation of shallow landslides by introducing the macropore coefficient of granite residual soil. The rainfall threshold of each slope unit was calculated by combining the hydrological model with the proposed shallow landslide model. Lastly, we tested the calculated result in the Maguihe watershed, Guangdong province, South China, which experienced a group of massive shallow landslides on 21 September 2010. The study results showed that the macropores of vegetation roots had a significant effect by increasing the permeability of granite residual soil. Coniferous forest land has the highest initial infiltration rate and stable infiltration rate, followed by shrub forest land, and then bare land. Statistical verification showed that the accurate prediction rate of the proposed model was 80.65%, which is adequate for early warning of shallow landslides in South China. We discuss the application conditions and parameter calibrations of the proposed model, and offer recommendations for future research.
Introduction
Shallow landslides induced by heavy rainfall are common phenomena around the world and are frequently accompanied by serious loss of life and property, particularly in mountainous and hilly areas (Asch et al., 2007; Beville et al., 2010; Chien et al., 2015; Palladino et al., 2018; Liu X. et al., 2022; Liu Z. J. et al., 2022). South China is located in a strong monsoon interaction zone. Under the influence of global climate change and intensifying human activities, landslide disasters have been increasing in frequency and people’s lives and property in hilly and mountainous areas are facing huge threats (Dai et al., 1999; Gong, 2014; Gong et al., 2021; Shou and Chen, 2021). For example, on 21 September 2010, typhoon Fanapi caused a heavy rainstorm with a single-day rainfall of 814 mm. These conditions resulted in more than 2,000 shallow landslides in Gaozhou City, Guangdong Province, causing 73 deaths and direct economic losses of about 2.2 billion yuan (Wang and Xia, 2012). On 10 June 2019, thousands of shallow landslides occurred in Longchuan County, Heyuan City, Guangdong Province, causing the evacuation of 19,769 people and a direct economic loss exceeding 200 million yuan (Sohu news, 2019). History reminds us that engineering measures cannot always protect us against the forces of nature. Thus, an effective warning system based on well-calibrated rainfall readings is extremely important to mitigate the risks associated with shallow landslides caused by rainstorm in South China.
Currently, the commonly used approaches for analyzing shallow landslides are statistical or physical methods (Adams and Sidle, 1987; Kim et al., 2016; Palladino et al., 2018; Ran et al., 2018; Vandromme et al., 2020; Loche et al., 2022). The statistical method cannot address the physical mechanism or dynamic behavior of landslides and factors such as soil cohesion and friction angle have little correlation with landslide occurrence. The premise of this method for early warning of shallow landslides is to have long-term, relatively complete rainfall data (Florence et al., 2018; Bhardwaj et al., 2019; Gao et al., 2019; Hawas et al., 2019; Juliev et al., 2019; Mekonnen et al., 2022). However, in the mountainous and hilly areas of South China, landslide observation sites are scarce, the research foundation is weak, and relatively few historical records are available. Thus, the essential data such as the time of landslide occurrence and the corresponding accumulated rainfall are often lacking. In this case, the margin of error when using statistical methods to generate early warnings is relatively large. At present, most existing landslide warning systems in South China are based on this method, wherein a warning is issued when an observed value reaches a statistical threshold.
Physical and mechanical methods are based on rainfall infiltration, hydrological effects, and soil instability as a function of the slope to determine the rainfall threshold, or an analysis of the critical hydrological threshold of landslides by studying the movement mechanism and runoff process (Romano et al., 1998; Nguyen et al., 2014; Jiang et al., 2017; Liu et al., 2017; Wu et al., 2020; Qiu et al., 2022; Wang et al., 2022; Zhu et al., 2022). This is presently the most effective method for determining the risk of shallow landslides. With this approach, we can clearly describe the migration of precipitation on the surface and its infiltration into the ground, as well as observe and analyze changes in pore water pressure of soils that occur because of precipitation. In addition, specific changes in slope and the corresponding safety factor value can be calculated using the slope stability model (Wang et al., 2014; Fattah et al., 2017; Liang, 2022). Currently, the main physical models used to evaluate shallow landslides associated with rainfall are the Shallow Slope Stability model (SHALSTAB) (Pradhan and Kim, 2016; Kim, et al., 2019; Shou and Chen, 2021), the Stability Index MAPping model (SINMAP) (Michel et al., 2014; Edirisooriya et al., 2018), and the Transient Rainfall Infiltration and Grid-based Regional Slope Stability model (TRIGRS) (Alvioli and Baum, 2016; Marin et al., 2020; He et al., 2021). These physical models are mainly founded on the infinite slope method based on Mohr-Coulomb failure criteria to reveal landslide potential. The plane sliding model is representative of those methods in which it is assumed that slope instability can be extended indefinitely. Safety factors and the possible location of failure at the surface can be obtained using the limit equilibrium method. Therefore, this paper used the physical method to calculate the threshold for warning against shallow landslides induced by heavy rainfall in South China.
Because of the particular type of weathered granite soil in South China, the formation mechanism is quite different from that of the loess landslides in the northwest and the gravel landslides in the southwest. The surface layers of shallow landslides in South China consist mostly of strongly weathered granite and residual slope accumulation layers, with well-developed surface joints and fissures. Heavy rain can scour the pores, gradually enlarging them to form channels for surface infiltration, turning the precipitation into a preferential flow through large pores. A temporary groundwater level is formed in the loose layer with a larger hydraulic conductivity that causes floating drag and scouring of the rock and soil mass, and eventually leads to the large-scale occurrence of shallow landslides (Dai et al., 1999; Letto and Cella, 2016; Gong et al., 2017; Chen et al., 2022; Liao et al., 2022; Silva et al., 2022). At present, most relevant physical models assume that under conditions of no surface runoff, the mechanism of how macropore flow influences shallow landslides is still unclear. Most research has focused on conducting laboratory tests on soil macropore flow (Ilek et al., 2019; Muddle and Briggs, 2019; Kotlar et al., 2020; Tao et al., 2020; Jarvis and Larsbo, 2022); related studies evaluating the effect of macropore flow on shallow landslide stability are relatively rare, and no relevant physical model incorporating the soil macropore coefficient has been established so far.
Therefore, the overall objective of this study was to develop an innovative model of the mechanism of shallow landslides that takes into account the effect of soil macropore flow. We also propose a method for determining the rainfall threshold for generating early warnings of shallow landslides in South China by combining the proposed physical model and the hydrological model. The specific objectives were 1) to analyze the effect of root macropores on soil permeability by field experiments using double-ring infiltration meters, 2) to develop a mechanistic model of shallow landslides including the effect of macropores, 3) to propose a method for determining the rainfall threshold for early warnings of shallow landslides based on the proposed model, and 4) to test the performance of the proposed method based on historical disaster data and from actual field data. To achieve these objectives, the Shenshui watershed, located in western Guangdong Province, South China, was selected as the study area. Because of the complex interaction of natural and artificial factors, this area has suffered repeatedly from shallow landslides from 2010 to 2021, causing serious loss of life and property. The study results provide a scientifically reliable theoretical basis for the mitigation of shallow landslides in South China.
Materials and methods
Study area
The Shenshui watershed, located in Magui town, Maoming City, Guangong Province, South China, was selected as the study area (Figure 1). The watershed area is approximately 9.52 km2 with a maximum elevation of approximately 836 m, and the length of the main channel is nearly 4.71 km. The river bed gradient is relatively large, ranging from 0° to 58.34°. The range of a typical channel slope, accounting for 39.51% of the study area, is between 30° and 40°. The characteristic parameters of the debris flow gully watershed are listed in Table 1.
The study area is a subtropical monsoon region, with a climate strongly influenced by the South China Sea. The average annual temperature ranges from 21.3°C to 23.2°C (Wang and Xia, 2012). The average annual rainfall is 2160 mm, the maximum average annual rainfall is 3150 mm, and the maximum annual rainfall is 3175 mm as recorded by the Magui hydrological observation station (Liu 2011). Lithologically, the main exposed strata are mainly Late Proterozoic gneissic fine-grained and medium-grained monzonitic granite, Sinian feldspar quartz sandstone mixed with silty slate and phyllite, Jurassic metamorphic rock formations, and Quaternary clay silt and sandy gravel sandwiched in clay siltstone. The parent material of the natural soil is mainly granite, with gneiss and its weathered elements. The study area is subject to heavy rains from typhoons and other severe convective weather. Because of its peculiar natural and geographical conditions, the heavy rains frequently lead to collapses, shallow landslides, debris flows and other disasters. For example, on 21 September 2010, Magui town suffered from many collapses, landslides, and debris flows because of typhoon Fanapi (Figure 2), resulting in the deaths of at least 73 people and damage to 8300 houses costing 2.2 billion yuan in economic losses (Wang and Xia, 2012), and having a serious impact on the environment and the lives of local residents. The shallow landslides occurred mostly at the top of the hill and halfway up the mountain, where loose solid materials were abundant. We determined the occurrence of shallow landslides induced by this event from the remote-sensing image data of the study area (Figure 2). It can be seen that the area of shallow landslides was nearly 0.60 km2; therefore, the early warning of the risk of shallow landslides in this area was extremely important.
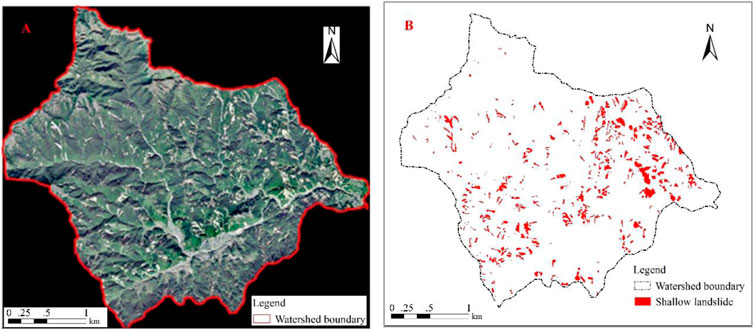
FIGURE 2. Distribution of shallow landslides in the Shenshui watershed induced by typhoon Fanapi. (A) Remote-sensing images after the event and (B) distribution of shallow landslides.
Field testing of effects of macropore flow on permeability enhancement
Numerous mass disaster events in South China, such as the Magui disaster on 21 September 2010 and the Longchuan disaster on 10 June 2019, show that mass shallow landslides are mainly concentrated on the mountains with well-developed vegetation. However, shallow landslides rarely occur in mountains with poor vegetation development. This is because the macropores of vegetation roots have a significant effect on increasing the permeability of granite residual soil. To discuss the effect of root macropores to the permeability of granite residual soil in South China, we measured the infiltration properties of granite residual soils under representative vegetation in South China using the double-loop method. Three cover types are used: coniferous forest, shrub forest and bare land. Every cover type conducted three groups of field tests. The field test of double-loop method was carried out according to the relevant specifications, mainly measuring the initial infiltration rate and stable infiltration rate of granite residual soils.
Mechanistic model of shallow landslides and the effect of macropores
According to the rainstorm infiltration mechanism of landslide formation, the surface runoff is taken as the node with two conditions being considered. With little surface runoff of rain, the groundwater infiltration and slope stability coefficient are controlled by the amount of rainfall. In the second case, with surface runoff, the volume of groundwater infiltration depends on the presence of large soil pores, which causes the soil to become saturated; thus, rainfall infiltration is mainly affected by the hydraulic conductivity of the saturated soil. It is assumed that the landslide body is relatively impermeable, a temporarily confined aquifer is located below the slip surface, and groundwater flows and circulates in deep layers along the confined aquifer. In South China, the shallow landslides are mainly induced under the second case. Thus, this study focused on landslide stability under the condition of surface runoff during a heavy rainstorm.
Under the action of rain, the flotation force
where
Innovatively considering the influence of macropore flow on landslide formation, the macropore coefficient (
where
When the seismic force is ignored and surface runoff occurs, the slope stability coefficient
where
Divide both the numerator and denominator of the above equation by
Let
Divide both the numerator and denominator of the above equation by
where
where
Rainfall threshold of shallow landslides
When
The main reason for shallow landslides during heavy rainfall in South China is the rise in groundwater level, soil saturation and the reduction in effective shear strength caused by continuous heavy rainfall. The limit equilibrium theory can well express the coupling relationship between hydrology and geology. Therefore, according to the runoff characteristics and mechanical limit equilibrium of slopes in small watersheds, an early warning threshold model of shallow landslides occurring in groups can be established based on the hydrological-mechanical mechanism. The hydrological model of this study used the improved SCS model to simulate the relationship between rainfall and infiltration, and the established slope limit equilibrium formula to analyze the stability of the slope under heavy rainfall. The calculation unit used in this study is the slope unit. The small watershed digital elevation model (DEM) is used to realize the automatic division of the slope unit, and each unit is coded and given element attribute information. On this basis, GIS spatial analysis is used to calculate the warning threshold of each division unit.
Results and discussion
Field test of the double-loop method
The test result of the initial infiltration rate and stable infiltration rate of granite residual soils in the three cover types are shown in Table 2. The processes of soil infiltration on different vegetation plots are shown in Figure 3.
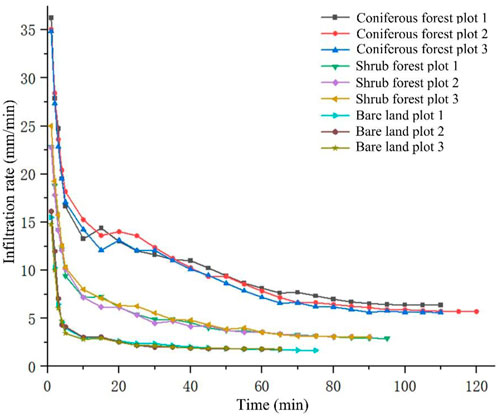
FIGURE 3. Infiltration process of different vegetation coverage in granite residual soil in South China.
It can be seen that although there are differences in the specific infiltration process on the different vegetation plots, the overall trend is the same. The soil infiltration rate was high in the initial stage, and decreased rapidly over a short period of time; then, the rate of decrease of infiltration slowed down, and the final infiltration rate approached a stable value.
In this paper, two infiltration indicators, initial infiltration rate and stable infiltration rate, were selected to analyze and evaluate the granite residual soil permeability in each plot (Table 2). It can be seen that the initial infiltration rate and the stable infiltration rate of coniferous forest land were the highest, followed by shrub forest land, and bare land. This is because the decomposing leaves form humus, and soil under the action of humus forms aggregates with a large pore structure. In addition, the coniferous forest soil is more transparent with many developed roots, root-soil gaps, rotted root channels and other large pores. The macropores of vegetation roots have a significant effect on increasing the permeability of granite residual soil. Therefore, a determination of the stability of a slope covered by vegetation must take into account the effect of macropore flow, which strongly influences slope stability; otherwise, the prediction of shallow landslides will be very different from the actual situation.
Calculated result of rainfall threshold of shallow landslides
Division of early warning unit
On the ArcGIS 10.5 platform, according to the digital elevation model (DEM) of the study area, the source-cutting method was adopted, and the ridge line and the valley line were used as the boundaries. The slope unit in the study area was divided into 624 slope subunits (Figure 4).
Results of model parameter calculations
In our proposed physical model of shallow landslides, the main parameters were terrain gradient (
1) Terrain gradient of the slope unit (
Slope is the most influential element in the determination of the risk factor for shallow landslides. Using GIS software, we first calculated the slope of each grid, and then used the spatial statistics function to count the average slope of each slope unit. The result is shown in Figure 5A.
2) The ratio of the area of rain collection (
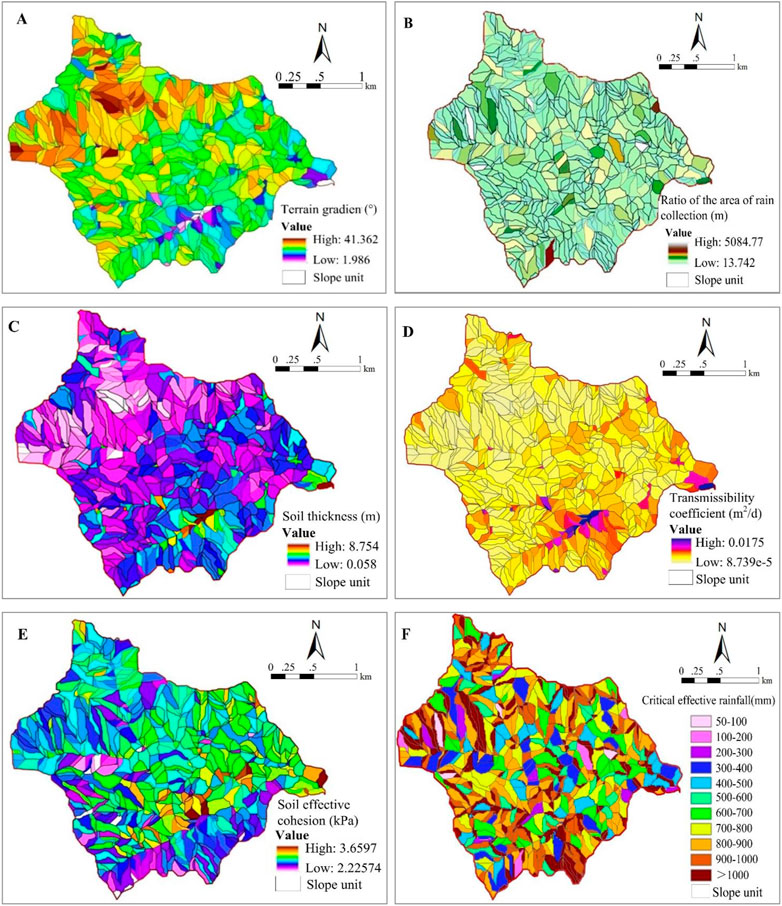
FIGURE 5. Calculated results of proposed model parameters and the critical effective rainfall of each slope unit in the study area. (A) Terrain gradient; (B) ratio of the area of rain collection; (C) soil thickness; (D) soil transmissibility coefficient; (E) soil effective cohesion; and (F) critical effect rainfall.
The ratio of the area of rain collection was calculated as:
in which
3) The soil thickness (
Soil thickness is one of the important factors affecting shallow landslides. In this study, in order to obtain the distribution of soil thickness in study area, field measurements of soil depth were made. The main measurements were soil thickness and surface slope. The measurement position of soil layer thickness was selected in the exposed section area, which was performed manually using an auger and tape measure. Based on the results of field measurements, a regression analysis was carried out on the relationship between slope and soil layer thickness, as:
where
4) The soil transmissibility coefficient (
The soil transmissibility coefficient was calculated by the following equation:
where
5) The soil effective cohesion (
A slope is a unified system composed of its upper soil layer and covered vegetation, and the effective cohesion of the soil is the combination of soil cohesion and plant root cohesion. In this study, the effective cohesion of the soil as related to vegetation coverage was calculated by the following formula:
where
6) The density ratio of water to soil (
The soil macropore coefficient (
Lastly, according to Eq. 9 and the algebraic calculation of each factor in ArcGIS software, the critical effective rainfall amount triggering shallow landslides in each slope unit can be obtained (Figure 5F).
It can be seen that the terrain slope of the slope unit in the study area varied from 1.986° to 41.362°. The upstream slope of the small watershed was larger, and the slope of the valley and downstream areas was relatively gentle (Figure 5A). The ratio of the area of rain collection in the study area ranged from 13.742 to 5084.77 (Figure 5B). The thickness of granite residual soil in the Shenshui watershed was relative high, with a maximum value of 8.754 m (Figure 5C). The transmissibility coefficient was between 0.00008739 m2/d and 0.0175 m2/d (Figure 5D). The soil effective cohesion was relatively small, with a maximum value of 3.6597 kPa (Figure 5E). It can be seen that when the effective rainfall was greater than 50 mm, some slope units slided (Figure 5F). The greater the effective rainfall, the greater the number of unstable slope units and the larger the group of landslides occurring. We compared the actual measured rainfall with the critical rainfall, and obtained the unstable state of the slope unit under the actual rainfall, so as to carry out early warning and forecasting for each slope.
Verification of proposed model
On 21 September 2010, the residents of Magui town experienced many shallow landslides due to the strong rains associated with typhoon Fanapi. The total rainfall reached 829.7 mm (from 19 September, at 20:00 h, to 23 September, at 20:00 h). The time of heavy precipitation in Magui Town on 21 September occurred from 00:00 to 12:00, with two peaks of very heavy precipitation. One peak occurred at about 02:00 h and the precipitation was 74 mm/h. The other peak was at 11:00 h, and the peak precipitation reached 105.5 mm/h. The rainfall process of this event is shown in Figure 6.
By inputting the above precipitation process data into the improved hydrological SCS model, we were able to obtain the actual effective rainfall threshold triggering shallow landslides on each slope unit. Then, by comparison with the critical effective rainfall calculated by the physical model proposed in this study, the spatial distribution of unstable slope units calculated by the proposed theoretical model can be illustrated (Figure 7).
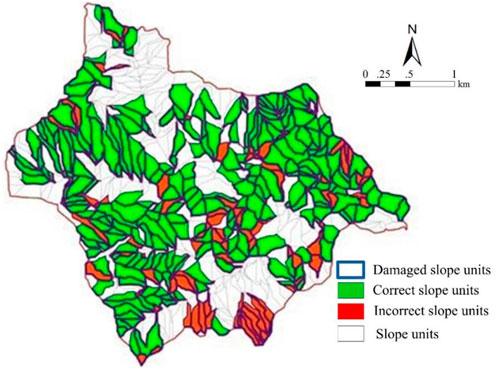
FIGURE 7. Comparison between the calculated results from the landslide early warning model and the actual occurrence.
Figure 7 shows that within the scope of the disaster, there were 336 slope units on which shallow landslides occurred. According to the results of the proposed model calculations, landslides happened on 271 slope units, and the accuracy of the prediction rate was 80.65%. Thus, the model proposed in this study was successfully used for early warning of shallow landslides in the Magui River Basin.
Discussion
The key parameters of the proposed physical model for early warning of shallow landslides can be roughly divided into five categories, namely topographic parameters, geological parameters, hydrological parameters, vegetation cover parameters, and meteorological parameters. The key parameters and the methods for obtaining them are shown in Table 3.
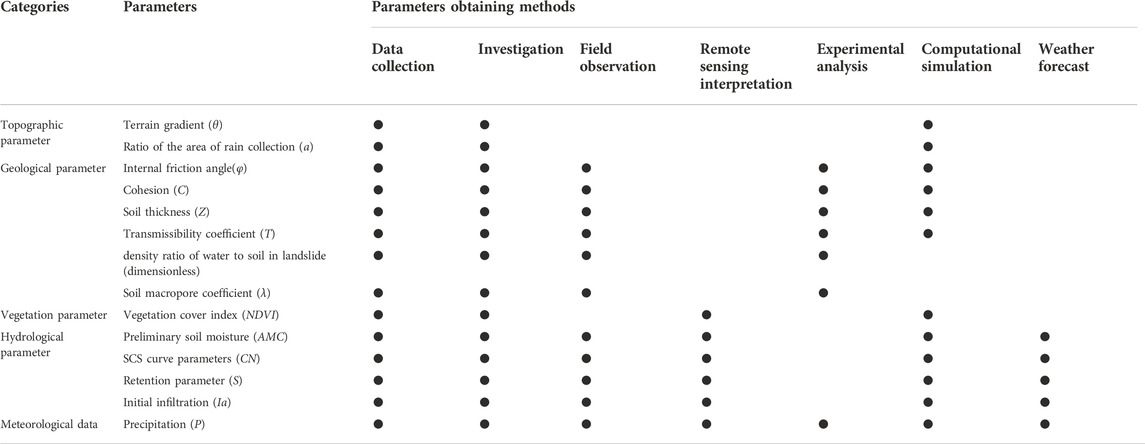
TABLE 3. Method of obtaining the key parameters of the proposed physical model of shallow landslides.
The above model parameters are very important for the calculation of shallow landslide warning thresholds. Therefore, in specific applications, it is necessary to test and calibrate these parameters, to ensure that they are in agreement with the actual results, and only in that way can the predicted warning threshold be accurate. The proposed model can be used in those areas with soil composed of weathered granites and subject to typhoon rainstorms, such as South China. In the future, we will continue to test this model on other watersheds where shallow landslides have occurred, so as to increase its accuracy for use in other regions.
Conclusion
In this study, we developed a physical model of shallow landslides, influenced by the effect of soil macropore flow, and proposed a method for determining the rainfall threshold for early warnings of shallow landslides based on the hydrological model and the proposed physical model in South China. The conclusions are:
1) We measured the infiltration properties of granite residual soils under three representative vegetation covers in South China using the double-loop method. The initial infiltration rate and the stable infiltration rate of coniferous forest land were highest, followed by shrub forest land, and bare land. The macropores of vegetation roots had a significant effect on increasing the permeability of granite residual soil; therefore, the type of vegetation coverage and the infiltration effect of macropores must be considered in determining the stability of a developed slope, otherwise the prediction of shallow landslides will be very different from the actual situation.
2) Considering the effect of macropore flow on shallow landslides, we introduced the macropore coefficient of soil into the shallow landslide mechanism model, and established a revised shallow landslide physical model of granite residual soil in South China.
3) We applied the proposed physical model to calculate the rainfall threshold for early warning of shallow landslides. The massive number of shallow landslides induced by the heavy rainfall on 21 September 2010 was used to verify the calculated results, and revealed a prediction accuracy of 80.65%. Thus, the model proposed in this study provided useful early warnings of the shallow landslides in the Magui River Basin.
Data availability statement
The raw data supporting the conclusion of this article will be made available by the authors, without undue reservation.
Author contributions
JW carried out the field investigation, data analysis, and manuscript writing. QG involved in manuscript supervision. SY undertook the field survey, participated in the discussion and decision-making process. JC was involved in writing reviewing, manuscript editing, and supervision. All the authors participated and contributed to the final manuscript.
Funding
This research work was supported by the National Natural Science Foundation of China (42101084; 41977413), Natural Science Foundation of Guangdong Province (2022A1515011898), Young S&T Talent Training Program of Guangdong Provincial Association for S&T (GDSTA), China (SKXRC202201), and 2022 National Park Construction Project, China (2021GJGY029).
Conflict of interest
The authors declare that the research was conducted in the absence of any commercial or financial relationships that could be construed as a potential conflict of interest.
Publisher’s note
All claims expressed in this article are solely those of the authors and do not necessarily represent those of their affiliated organizations, or those of the publisher, the editors and the reviewers. Any product that may be evaluated in this article, or claim that may be made by its manufacturer, is not guaranteed or endorsed by the publisher.
References
Adams, P. W., and Sidle, R. C. (1987). Soil conditions in three recent landslides in Southeast Alaska. For. Ecol. Manage. 18 (2), 93–102. doi:10.1016/0378-1127(87)90136-8
Alvioli, M., and Baum, R. L. (2016). Parallelization of the TRIGRS model for rainfall-induced landslides using the message passing interface. Environ. Model. Softw. 81, 122–135. doi:10.1016/j.envsoft.2016.04.002
Asch, T., Beek, l., and Bogaard, T. A. (2007). Problems in predicting the mobility of slow moving landslides. Eng. Geol. 91 (1), 46–55. doi:10.1016/j.enggeo.2006.12.012
Beville, S. H., Mirus, B. B., Ebel, B. A., Mader, G. G., and Loague, K. (2010). Using simulated hydrologic response to revisit the 1973 Lerida Court landslide. Environ. Earth Sci. 61 (6), 1249–1257. doi:10.1007/s12665-010-0448-z
Bhardwaj, A., Wasson, R. J., Ziegler, A. D., Chow, W. T. L., and Sundriyal, Y. P. (2019). Characteristics of rain-induced landslides in the Indian himalaya: A case study of the mandakini catchment during the 2013 flood. Geomorphology 330, 100–115. doi:10.1016/j.geomorph.2019.01.010
Chen, P. J., Liu, X., Yu, S., Xu, J. H., Hong, B., Ma, J., et al. (2022). Stability assessment of the restored Benggang units in a weathered granite crust region of South China. Ecol. Eng. 182, 106709. doi:10.1016/j.ecoleng.2022.106709
Chien, L. K., Hsu, C. F., and Yin, L. C. (2015). Warning model for shallow landslides induced by extreme rainfall. Water 7, 4362–4384. doi:10.3390/w7084362
Dai, F. C., Lee, C. F., and Wang, S. J. (1999). Analysis of rainstorm-induced slide-debris flows on natural terrain of Lantau Island, Hong Kong. Eng. Geol. 51 (4), 279–290. doi:10.1016/S0013-7952(98)00047-7
Edirisooriya, K. V. D., Vitanage, N. S., Uluwaduge, P., and Senevirathna, E. M. T. K. (2018). Understanding disaster risk and mitigation special reference to Ratnapura District. Procedia Eng. 212, 1034–1039. doi:10.1016/j.proeng.2018.01.133
Fattah, M. Y., Al-Mosawi, M. J., and Al-Americ, A. F. I. (2017). Stresses and pore water pressure induced by machine foundation on saturated sand. Ocean. Eng. 16, 268–281. doi:10.1016/j.oceaneng.2017.09.055
Florence, W. Y., Frankie, K., and Lo, L. C. (2018). From landslide susceptibility to landslide frequency: A territory-wide study in Hong Kong. Eng. Geol. 242, 12–22. doi:10.1016/j.enggeo.2018.05.001
Gao, J., Zahng, Z., Wang, C. Z., Liu, J., and Zhang, L. (2019). Susceptibility assessment of landslides triggered by earthquakes in the Western Sichuan Plateau. Catena 175, 63–76. doi:10.1016/j.catena.2018.12.013
Gong, Q. H. (2014). DEM and GIS based analysis of topographic and geomorphologic factors of shallow landslide in red soil hilly region of South China. Int. J. Earth Sci. Eng. 7 (2), 393–399.
Gong, Q. H., Huang, G. Q., Zhang, D. L., and Xiong, H. X. (2017). On the application of the slope element unit method to the shallow landslide risk-prevention mapping with a case study sample of Songgang watershed. J. Saf. Environ. 17 (2), 615–620.
Gong, Q. H., Wang, J., Zhou, P., and Guo, M. (2021). A regional landslide stability analysis method under the combined impact of rainfall and vegetation roots in South China. Adv. Civ. Eng. 2021, 1–12. doi:10.1155/2021/5512281
Hawas, K., Muhammad, S., Muhammad, A., Bacha, M. A., Shah, S. U., and Calligaris, C. (2019). Landslide susceptibility assessment using Frequency Ratio, a case study of northern Pakistan. Egypt. J. Remote Sens. Space Sci. 22 (1), 11–24. doi:10.1016/j.ejrs.2018.03.004
He, J. Y., Qiu, H. J., Qu, F. H., Hu, S., Yang, D. D., Zhang, Y., et al. (2021). Prediction of spatiotemporal stability and rainfall threshold of shallow landslides using the TRIGRS and Scoops3D models. CATENA 197, 104999. doi:10.1016/j.catena.2020.104999
Ilek, A., Kucza, J., and Witek, W. (2019). Using undisturbed soil samples to study how rock fragments and soil macropores affect the hydraulic conductivity of forest stony soils: Some methodological aspects. J. Hydrology 570, 132–140. doi:10.1016/j.jhydrol.2018.12.067
Jarvis, N., and Larsbo, M. (2022). Macropores and macropore flow. Reference Module Earth Syst. Environ. Sci 2022, 1–8. doi:10.1016/B978-0-12-822974-3.00098-7
Jiang, S. H., Huang, J. S., Yao, C., and Yang, J. H. (2017). Quantitative risk assessment of slope failure in 2-D spatially variable soils by limit equilibrium method. Appl. Math. Model. 47, 710–725. doi:10.1016/j.apm.2017.03.048
Juliev, M., Mergili, M., Mondal, I., Nurtaev, B., Pulatov, A., and Hubl, J. (2019). Comparative analysis of statistical methods for landslide susceptibility mapping in the Bostanlik District, Uzbekistan. Sci. Total Environ. 653, 801–814. doi:10.1016/j.scitotenv.2018.10.431
Kim, M., An, H., Kim, J., Kim, S., Oh, H. J., and Song, Y. S. (2019). Assessment of sudden sediment source areas incurred by extreme rainfall in a mountainous environment: Approach using a subsurface hydrologic concept. Quat. Int. 519, 232–244. doi:10.1016/j.quaint.2018.10.031
Kim, M. S., Onda, Y., Uchida, T., and Kim, J. K. (2016). Effects of soil depth and subsurface flow along the subsurface topography on shallow landslide predictions at the site of a small granitic hillslope. Geomorphology 271, 40–54. doi:10.1016/j.geomorph.2016.07.031
Kotlar, A. M., Lier, Q. J., Andersen, H. E., Norgaard, T., and Iversen, B. V. (2020). Quantification of macropore flow in Danish soils using near-saturated hydraulic properties. Geoderma 375, 114479. doi:10.1016/j.geoderma.2020.114479
Letto, F., and Cella, P. F. (2016). Geotechnical and landslide aspects in weathered granitoid rock masses (Serre Massif, southern Calabria, Italy). CATENA 145, 301–315. doi:10.1016/j.catena.2016.06.027
Liang, W. L. (2022). Dynamics of pore water pressure at the soil–bedrock interface recorded during a rainfall-induced shallow landslide in a steep natural forested headwater catchment, Taiwan. J. Hydrology 587, 125003. doi:10.1016/j.jhydrol.2020.125003
Liao, D. L., Deng, Y. S., Duan, X. Q., Cai, C. Q., and Ding, S. W. (2022). Variations in weathering characteristics of soil profiles and response of the Atterberg limits in the granite hilly area of South China. CATENA 215, 106325. doi:10.1016/j.catena.2022.106325
Liu, C. Y., Ku, C. Y., Xiao, J. E., Huang, C. C., and Hsu, S. M. (2017). Numerical modeling of unsaturated layered soil for rainfall-induced shallow landslides. J. Environ. Eng. Landsc. Manag. 25 (4), 329–341. doi:10.3846/16486897.2017.1326925
Liu, J. H. (2011). Analysis of heavy mountain flood on 21th, september, 2010 in Gaozhou caojiang river. Guangdong Water Resources and Hydropower, 33–37.
Liu, X., Lan, H. X., Li, L. P., and Cui, P. (2022). An ecological indicator system for shallow landslide analysis. CATENA 214, 106211. doi:10.1016/j.catena.2022.106211
Liu, Z. J., Zhu, Y. R., Liu, Y., Yang, D. D., Ma, S. Y., Zhang, J. J., et al. (2022). Efficient identification and monitoring of landslides by time-series InSAR combining single- and multi-look phases. Remote Sens. 14, 1026. doi:10.3390/rs14041026
Loche, M., Alvioli, M., Marchesini, I., Bakka, H., and Lombardo, L. (2022). Landslide susceptibility maps of Italy: Lesson learnt from dealing with multiple landslide types and the uneven spatial distribution of the national inventory. Earth-Science Rev. 232, 104125. doi:10.1016/j.earscirev.2022.104125
Marin, R. J., García, E. F., and Aristizábal, E. (2020). Effect of basin morphometric parameters on physically-based rainfall thresholds for shallow landslides. Eng. Geol. 278, 105855. doi:10.1016/j.enggeo.2020.105855
Mekonnen, A. A., Raghuvanshi, T. K., Suryabhagavan, K. V., and Kassawmar, T. (2022). GIS-based landslide susceptibility zonation and risk assessment in complex landscape: A case of beshilo watershed, northern Ethiopia. Environ. Challenges 8, 100586. doi:10.1016/j.envc.2022.100586
Michel, G. P., Kobiyama, M., and Goerl, R. F. (2014). Comparative analysis of SHALSTAB and SINMAP for landslide susceptibility mapping in the Cunha River basin, southern Brazil. J. Soils Sediments 14 (7), 1266–1277. doi:10.1007/s11368-014-0886-4
Muddle, D. M., and Briggs, K. M. (2019). Macropore structure and permeability of clay fill samples from a historic clay fill earthwork. Transp. Geotech. 19, 96–109. doi:10.1016/j.trgeo.2019.02.003
Nguyen, M. N., Bui, T. Q., Yu, T., and Hirose, S. (2014). Isogeometric analysis for unsaturated flow problems. Comput. Geotech. 62, 257–267. doi:10.1016/j.compgeo.2014.08.003
Palladino, M. R., Viero, A., Turconi, L., Brunetti, M. T., Peruccacci, S., Melillo, M., et al. (2018). Rainfall thresholds for the activation of shallow landslides in the Italian alps: The role of environmental conditioning factors. Geomorphology 303, 53–67. doi:10.1016/j.geomorph.2017.11.009
Pradhan, A. M. S., and Kim, Y. T. (2016). Evaluation of a combined spatial multi-criteria evaluation model and deterministic model for landslide susceptibility mapping. CATENA 140, 125–139. doi:10.1016/j.catena.2016.01.022
Qiu, H., Zhu, Y., Zhou, W., Sun, H., He, J., and Liu, Z. (2022). Influence of DEM resolution on landslide simulation performance based on the Scoops3D model. Geomat. Nat. Hazards Risk 13 (1), 1663–1681. doi:10.1080/19475705.2022.2097451
Ran, Q. H., Hong, Y. Y., Li, W., and Gao, J. H. (2018). A modelling study of rainfall-induced shallow landslide mechanisms under different rainfall characteristics. J. Hydrology 563, 790–801. doi:10.1016/j.jhydrol.2018.06.040
Romano, N., Brunone, B., and Santini, A. (1998). Numerical analysis of one-dimensional unsaturated flow in layered soilsflow in layered soils. Adv. Water Resour. 21 (4), 315–324. doi:10.1016/S0309-1708(96)00059-0
Shou, K. J., and Chen, J. R. (2021). On the rainfall induced deep-seated and shallow landslide hazard in Taiwan. Eng. Geol. 288, 106156. doi:10.1016/j.enggeo.2021.106156
Silva, A. C. D., Resende, I., Costa, R. C. D., Uagoda, R. E. S., and Avelar, A. D. S. (2022). Geophysical for granitic joint patern and subsurface hydrology related to slope instability. J. Appl. Geophys. 199, 104607. doi:10.1016/j.jappgeo.2022.104607
Sohu New (2019). The 6.10 flood in Longchuan caused economic losses of about 80 million yuan and affected 28,000 people. AvaliableAt: https://www.sohu.com/a/319885598_669237.
Tao, Y., Zou, Z. Q., Guo, L., He, Y. B., Lin, L. R., Lin, H., et al. (2020). Linking soil macropores, subsurface flow and its hydrodynamic characteristics to the development of Benggang erosion. J. Hydrology 586, 124829. doi:10.1016/j.jhydrol.2020.124829
Vandromme, R., Thiery, Y., Sedan, S., and Sedan, O. (2020). ALICE (assessment of landslides induced by climatic events): A single tool to integrate shallow and deep landslides for susceptibility and hazard assessment. Geomorphology 367, 107307. doi:10.1016/j.geomorph.2020.107307
Wang, G. H., Zhang, D. X., Furuya, G., and Yang, J. (2014). Pore-pressure generation and fluidization in a loess landslide triggered by the 1920 haiyuan earthquake, China: A case study. Eng. Geol. 174, 36–45. doi:10.1016/j.enggeo.2014.03.006
Wang, L. Y., Qiu, H. J., Zhou, W. Q., Zhu, Y. R., Liu, Z. J., Ma, S. Y., et al. (2022). The post-failure spatiotemporal deformation of certain translational landslides may follow the pre-failure pattern. Remote Sens. 14, 2333. doi:10.3390/rs14102333
Wang, Y., and Xia, B. (2012). Eco-restoration strategies and measures for the soil and water conservation of typhoon-hit areas in Western Guangdong province: A case study of Magui town, Gaozhou city. Sci. Soil Water Conservation 10 (1), 88–93.
Wu, L. Z., Zhu, S. R., and Peng, J. B. (2020). Application of the Chebyshev spectral method to the simulation of groundwater flow and rainfall-induced landslides. Appl. Math. Model. 80, 408–425. doi:10.1016/j.apm.2019.11.043
Keywords: shallow landslide, formation mechanism, soil macropore flow, rainfall threshold, granites weathering crust
Citation: Wang J, Gong Q, Yuan S and Chen J (2023) Combining soil macropore flow with formation mechanism to the development of shallow landslide warning threshold in South China. Front. Earth Sci. 10:1048427. doi: 10.3389/feart.2022.1048427
Received: 19 September 2022; Accepted: 20 October 2022;
Published: 11 January 2023.
Edited by:
Haijun Qiu, Northwest University, ChinaCopyright © 2023 Wang, Gong, Yuan and Chen. This is an open-access article distributed under the terms of the Creative Commons Attribution License (CC BY). The use, distribution or reproduction in other forums is permitted, provided the original author(s) and the copyright owner(s) are credited and that the original publication in this journal is cited, in accordance with accepted academic practice. No use, distribution or reproduction is permitted which does not comply with these terms.
*Correspondence: Jun Wang, d2FuZ2p1bkBnZGFzLmFjLmNu; Qinghua Gong, Z29uZ3FoQGdkYXMuYWMuY24=