- 1Department of Earth and Environmental Sciences, Indian Institute of Science Education and Research Bhopal, Bhopal, India
- 2Helmholtz-Zentrum Hereon Institute, Geesthacht, Germany
This study aims to provide a comprehensive and balanced assessment of recent scientific studies on the evolution, temporal variability and prediction of tropical cyclones (TCs), focusing on the North Indian Ocean (NIO). The involvement of earth’s components in TC genesis and intensification has been elaborated in a confined way. The advancement of multidisciplinary approaches for comprehending the TCs is highlighted after a brief description of the involvement of oceanic, atmospheric, and land surface processes. Only a few studies illustrate how land surface plays a role in TC intensification; however, the role of latent heat flow, moisture, and convection in cyclogenesis is well documented. Despite two to 3 decades of advancement and significant development in forecasting techniques and satellite products, the prediction of TC’s intensity, dissipation, track, and landfall remains a challenge. The most noticeable improvements in NIO TC’s prediction have been achieved in the last couple of decades when concord techniques are utilized, especially the data assimilation methods and dynamical coupled atmosphere-ocean regional models. Through diverse methodologies, algorithms, parameterization, in-situ observational data, data mining, boundary layer, and surface fluxes, significant research has been done to increase the skills of standalone atmospheric models and air-sea coupled models. However, some crucial issues still exist, and it is suggested that they should be addressed in future studies.
1 Introduction
Tropical cyclone (TC) is a broad term for a non-frontal synoptic scale low-pressure system that develops over the warm tropical oceans with organized convective processes (Wang and Wu, 2004). Upper ocean heat content (UOHC) and sea surface temperature (SST) are primary oceanic components that make favourable conditions for convection, which results in the development of a low-pressure area (Tiwari et al., 2021). TCs have a horizontal scale of hundreds to thousand kilometres extending throughout the troposphere’s height (Wang et al., 2012). TCs are demarcated by different names in various oceanic basins such as “typhoon” (Western Pacific), “hurricane” (Atlantic and Eastern Pacific), and “tropical cyclone” (north Indian Ocean). The World Meteorological Organization and India Meteorological Department (IMD) have classified the TCs over the north Indian Ocean (NIO) with 3-min maximum sustained wind (MSW) speed (Table 1); however, the criterion for MSW differs for different regions. A low-pressure area with MSW 31–50 km/h is characterized as depression followed by deep depression (51–62 kmph), cyclonic storm (CS, 63–88 km/h), severe cyclonic storm (SCS, 89–117 km/h), etc. (Table 1).
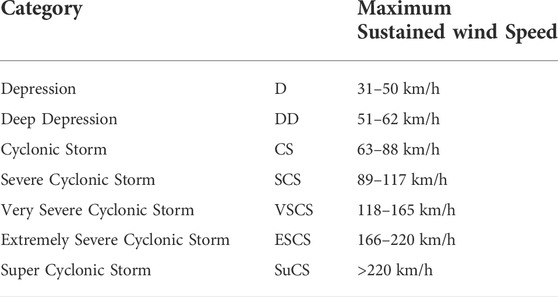
TABLE 1. North Indian Ocean tropical cyclone intensity scale by IMD based on 3-min average maximum sustained wind speed.
The NIO, with a coastline of ∼7,516 km, is accountable for 6–8% of TCs that develop over the globe dominated by the Bay of Bengal (BoB) (Das et al., 2014; Espejo et al., 2016; Mohapatra, 2016; Gupta et al., 2019; Mohanty et al., 2019). The NIO shows a bimodal distribution of TCs activities over the BoB and Arabian Sea (ARB) basins. The primary peak lies in the post-monsoon season (October-December) and another in the pre-monsoon season, i.e., April-June. The trend of the propagation of NIO TCs is northwestwards. Therefore, most of the BoB TCs cause devastation on the eastern coast of India (Gupta et al., 2019; Nadimpalli et al., 2021). Since the last decade, an increase in the frequency of pre-monsoon intense ARB TCs have been observed, and they mostly showed north-eastward propagation (Sriver, 2011; Wang et al., 2012). Thus, it is crucial to understand the synoptic and mesoscale circulations that help in the TC genesis and evolution.
Usually, the TC activities in the BoB are approximately four times higher than in the ARB (Deshpande et al., 2021). However, recent studies have reported an increasing trend of very intense ARB TCs in the changing climate scenario due to the rise in the ARB SST (Sriver, 2011; Wang et al., 2012; Gupta et al., 2019). Deshpande et al. (2021) observed a significant change in the frequency, duration, and intensity of the ARB and BoB CS and VSCS during 1982–2019. The frequency of ARB CS has increased by 52% in the recent epoch (2001–2019), whereas the frequency of BoB CS showed a decrease in the same period. The year 2019 was the most active period for NIO, with 5 TCs in the ARB and three in the BoB (Deshpande et al., 2021). Figure 1 demonstrates the monthly frequency of NIO TCs taken from the IMD data for a period of 130 years (1891–2020). 49.8% of the NIO TCs were formed in the post-monsoon season, while 28.9% were in the pre-monsoon season. The overall contribution of these 6 months was approximately 80%.
Figure 2 depicts the decadal frequency and trend of NIO TCs for three seasons: March-May (MAM), June-September (JJAS), and October-December (OND), as well as the annual period from 1891 to 2020. The MAM TCs (Figure 2A) has declined slightly, with a trend value of −0.004 and an average of 10 TCs each decade. Above a 90% confidence level, the Mann-Kendal test confirms that the trend is not significant. With a mean of 14.5 TCs per decade, JJAS (Figure 2B) has shown a significant decreasing trend (above 90% confidence level). However, with an average of 25.2 TCs each decade, the OND season has shown an increasing trend (not significant at 90% confidence level) (Figure 2C). With a trend value of −0.159 and 50.5 TCs per decade, yearly NIO TCs have demonstrated a decreasing trend (not significant above 90% confidence level) (Figure 2D).
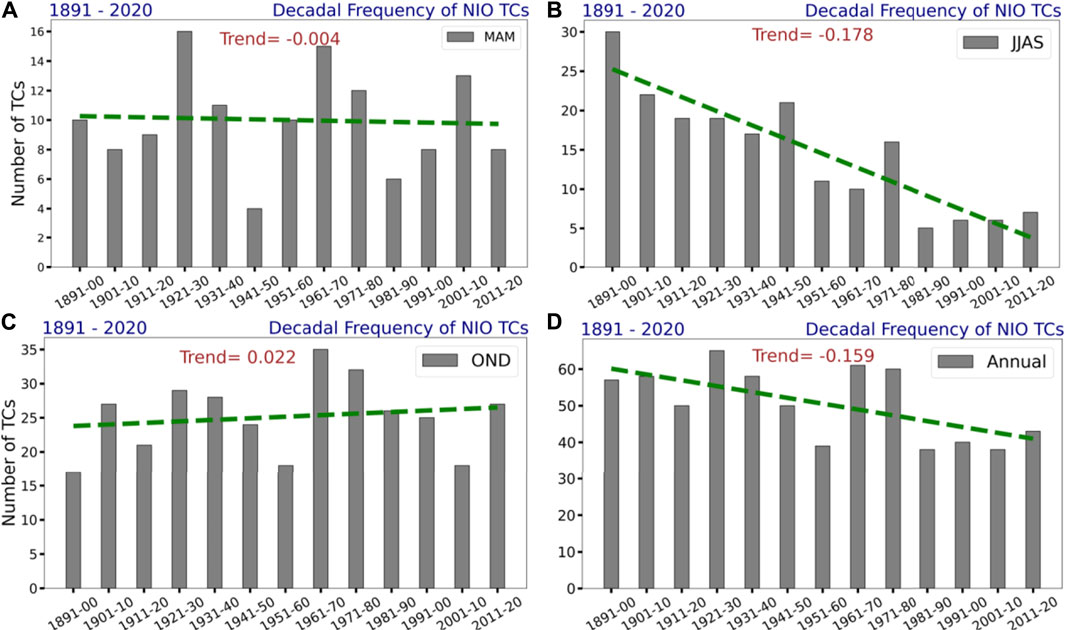
FIGURE 2. Decadal frequency of NIO TCs and their trends during 1891–2020 for the seasons (A) MAM, (B) JJAS, (C) OND, and (D) Annual.
Furthermore, Figure 3 shows the decadal frequency of ARB TCs for the same seasons and duration as Figure 2. 34 TCs were identified for the MAM season, with a slightly declining trend from 1891 to 2020. In the JJAS and OND seasons, 39 and 59 TCs were formed with an increasing trend, showing a ratio of 1:1.74 for the ARB and OND seasons. In the recent 2 decades (2001–10 and 2011–20), the number of ARB TCs for OND has more than doubled. Over the last 4 decades, the frequency of yearly TCs has monotonically increased, with an overall increasing trend.
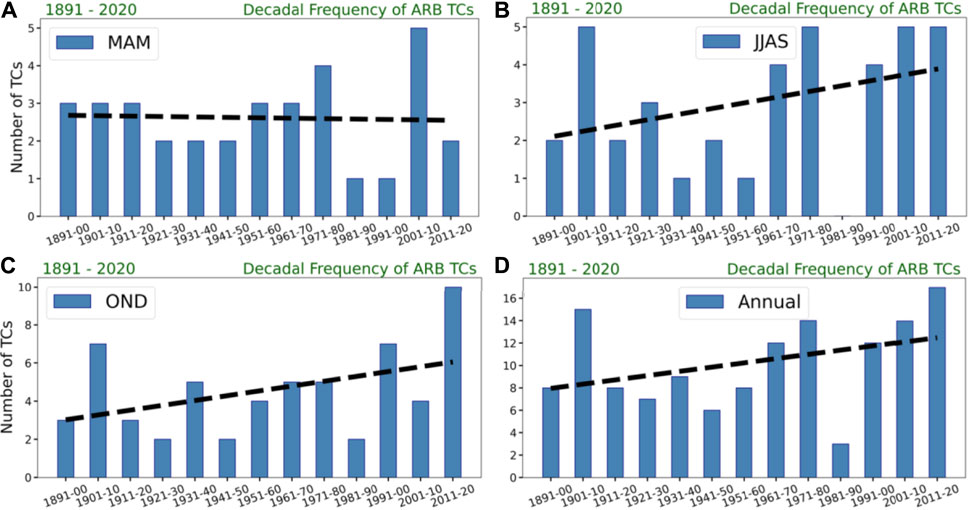
FIGURE 3. Decadal frequency of ARB TCs during 1891–2020 for the seasons (A) MAM, (B) JJAS, (C) OND, and (D) Annual.
Mondal et al. (2021) examined the characteristics of BoB TCs from 1982 to 2020 in the context of the El Nino Southern Oscillation (ENSO). They reported that TC activities have increased during ENSO years, with some shifts in their genesis locations. The majority of the TCs were developed between 5o and 15oN. During La Nina (El Nino) conditions, almost two-thirds of TCs were headed eastward (westward). The TCs of La Nina years were found to have a longer lifespan than those of El Nino. La Nina years had an average of 8.92 annual TC days, which was twice as many as El Nino years (4.51 days).
In recent years, studies have been carried out to understand the dynamics and thermodynamics of TCs’, physics of the numerical weather prediction (NWP) models including boundary layer parameterizations, and vortex initialization to improve the TC forecast eventually to prevent devastations and huge losses. The intense TCs cause unfair losses of lives, infrastructure, and agriculture, especially near the coastal region (Figure 4). Therefore, it cannot be ignored that with the advancement in the TC forecast, there will be a significant reduction in the loss of lives. Few recent perfidious TCs like Amphan (2020), Fani (2019), Hudhud (2014), and Phailin (2013), due to very to extremely severe intensity, resulting in a loss of $6-7 billion. It extended the space of forecast improvement to the numerical models and forecasting community (Gupta et al., 2019).
In this review article, following with the Introduction, the authors provide an overview of the role of earth’s components associated with TC genesis and intensification in section 2. Section 3 focuses on multidisciplinary approaches to study the TC. Revolution in NIO TCs prediction in the satellite era is discussed in section 4. Concluding remarks are given in the last section.
2 Earth’s components and cyclogenesis
A TC is a very complex phenomenon resulting from the steady-state changes in the composite environment. A low-pressure area develops over the ocean and concentrates into depression and deep depression with favourable conditions intensifying into a TC expanding in the troposphere. Usually, after the landfall, it again concentrates into a depression (Chauhan et al., 2021). Therefore, analysis of all the environmental components contributing to TC activities would be helpful to develop a better understanding of the TC formation and precisely forecast its characteristics such as intensity, eye formation, track, landfall, heavy rainfall, storm surge, etc.
2.1 Role of ocean
A warm ocean with a low-pressure system is the niche for the formation of TCs. However, the thermal gradient of the NIO consisting of BoB and ARB gives rise to many furious TCs. Several studies have been done to understand the dynamics and thermodynamics of the ocean involved in the TCs’ formation (Webster et al., 2005). In addition, there are studies done in the past to understand the impact of TC on the BoB region. The findings from the studies confirm the rise in the NIO SST (Tiwari et al., 2021). Mishra et al. (2020) reported a strong role of ARB warming on increasing weather extremes. In addition to this, a study by Mohanty et al. (2012) confirms the role of SST in increasing the number of SCS in the ARB. Also, there are recent studies done so far to understand the ocean’s role in TC formation and its activities. For example, Prakash and Pant (2017) studied the dominant role of mixed layer heat budget in TC genesis. The study’s findings confirmed the reduction in the magnitude and diurnal periodicity of the net surface heat fluxes interconnected with the cloud cover during the cyclone. On the other hand, Patwardhan and Bhalme (2001) and Jadhav and Munot (2009) identified a significant decrease in the trend of TC frequency seasonally despite increasing SST in recent decades. Therefore, to simulate the accurate TC obtaining a realistic SST is a big challenge to researchers. However, there are studies conducted to do sensitivity experiments of models to get realistic SST. SST obtained through satellite corresponds to TC (Mandal and Mohanty, 2010; Mohanty et al., 2019). While few contrasting studies (Demaria and Kalpana, 1994) reveal that SST has a relatively less impact on the intensification and propagation of TC after the formation. Bhatla et al. (2020) mentioned that global warming had increased the upper troposphere temperature and also the SST. This rise in SST could be a reason for intense TCs (Chauhan et al., 2021). Another study by Frank and Young (2007) also confirmed the role of SST in the formation and increased intensity of TC.
A 28-years (1990–2017) mean SST in the pre-monsoon (AMJ) and post-monsoon (OND) seasons over the NIO has been shown in Figures 5A,B using the Hadley Centre Global Sea Ice and Sea Surface Temperature (HadISST) data. The pre-monsoon season is associated with boreal summer, and thus the SST in this season was relatively high (Figure 5A). Southeast ARB was warmer than other regions, and most of the intense ARB TCs formed over this region. Due to the Indian Summer Monsoon Rainfall season from June to September, the SST during the post-monsoon becomes low but sufficient to conceive TCs (Figure 5B). Central to south-east BoB was comparatively warm, and most of the intense BoB TCs developed over this region.
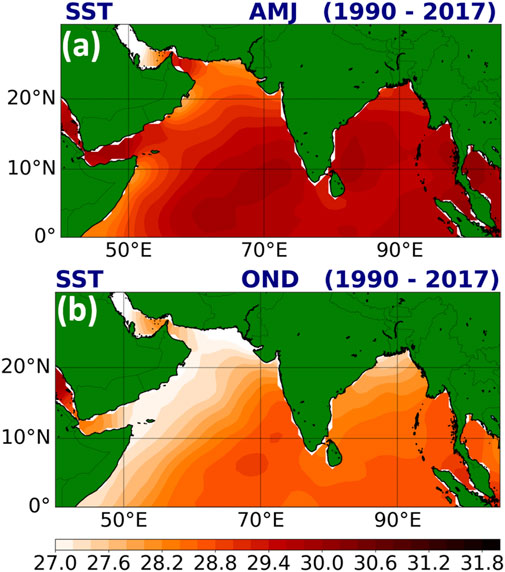
FIGURE 5. The mean SST (oC) over the NIO during 1990–2017 for (A) April-June (AMJ) and (B) October-December (OND). The SST data was obtained from Hadley Centre Global Sea Ice and Sea Surface Temperature dataset.
Other oceanic subsurface parameters play a vital role in modifying the TCs genesis and other characteristics. Tropical Cyclone Heat Potential (TCHP), currents, and eddies over the warm oceans help in the TC’s rapid intensification (RI). Moreover, a trace of eddies formation near TC tracks could assess the RI phase (Jangir et al., 2020). Lin et al. (2013) also mentioned the role of TCHP and eddy currents in the TC’s RI. A spatial demonstration of TCHP over the BoB during the life of VSCS Titli is shown in Figure 6. A cyclonic system like VSCS Titli that encounter the TCHP and eddy currents are more disastrous during the landfall and further inland progression. Mawren and Reason (2017) and Patnaik et al. (2014) also mentioned the contribution of eddies in the TC intensification over the NIO. In addition, there are sparse studies carried out using the oceanic numerical models to understand and forecast the TC. For example, Das et al. (2014) used Princeton Ocean Model (POM) to study the thermodynamics of oceans over BoB.
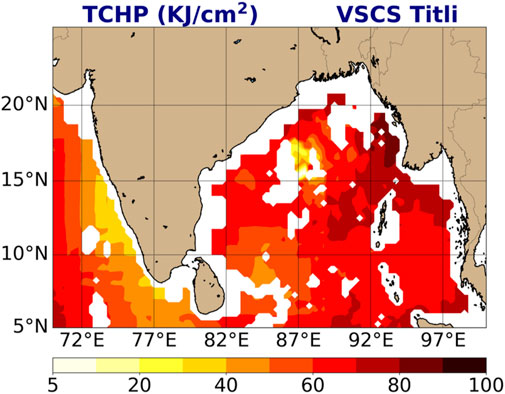
FIGURE 6. Spatial distribution of tropical cyclone heat potential (KJ/cm2) over the BoB valid for 10 October 2018 during the life of a very severe cyclonic storm Titli. The data was obtained from the National Remote Sensing Centre- Indian Space Research Organisation, India.
2.2 Role of atmosphere
TC is always a concern for meteorologists because it results from synoptic and mesoscale interactions accompanied by other natural phenomena like strong winds, heavy rainfall, and storm surge. Likewise, ocean components other essential factors of the weather system that contribute to the formation of TCs are Coriolis force, vorticity, low vertical wind shear, moisture and moist convection. Moreover, in tropical regions, moist convection dominates the process of transporting mass, energy, and momentum through the atmosphere (Kumar et al., 2017). Albert et al. (2022) reported a 0.58 correlation between power dissipation index (PDI) and SCS category TCs from 1979 to 2019. They also found that relative humidity at 600 hPa, positive relative vorticity at 850 hPa, and reduced outgoing longwave radiation at 500 hPa have significantly contributed to the NIO’s increased TC frequency. Balaji et al. (2018) used the accumulated cyclone energy (ACE) metric to analyze TC activities over the NIO from 1981 to 2014 and observed an increasing trend in recent years. SST, UOHC, atmospheric water vapour, and the genesis potential index (GPI) were all strongly associated with the growth and variability of ACE. According to Duan et al. (2021), the lower and middle troposphere’s relative humidity and vertical wind shear are the two key contributors to TC genesis. The combination of these two variables drives the bimodal seasonal cycle of TCs’ in both the ARB and the BoB. Tiwari et al. (2021) investigated the characteristics of post-monsoon season TCs’ over the BoB from 1979 to 2018 using various metrices such as ACE and PDI. They also used correlation and principal component analysis on the VSCS, SCS, and CS category TCs, taking into account SST, vertical wind shear, MSW, minimum sea level pressure (MSLP), relative vorticity, and specific rainwater content, among other parameters and revealed that relative vorticity is the most influential among all the controlling elements that impact the final intensity of the BoB TCs’. A study by Chan (2005) explained the importance of the baroclinic process in the TC. However, the energy and heat transfer through convection modifies the temperature gradient and vertical wind shear (Osuri et al., 2010). Again, the representation of dynamical changes in the NWP model is a big challenge. However, this has been further improved with satellite products that help to develop the better initial vortex position and structure of TC in the NWP models.
Further to confirm the data dependencies for position and structure of TC, Courtney et al. (2019) used different best-track datasets from IMD and Joint Typhoon Warning Center (JTWC) to analyze the intensity of VSCS Hudhud. They found different results with both the dataset as underestimation in one and overestimation in other due to different criteria of sources for MSW. Mohanty et al. (2020) portray the impact of dry air intrusion on the ESCS Fani caused by asymmetric wind. This dry air significantly impacts the vortex initialization that affects the structure, landfall, and TC intensity. However, few studies focused on improving the vortex initialization by using better satellite and reanalysis data (Nadimpalli, 2020a; Nadimpalli, 2020b). In addition to SST, atmospheric high temperature and diabatic heating also plays a dominant role in the evolution of the intensity and determining the track of TC (Singh and Bhaskaran, 2020). Henceforth, many studies confirmed the direct correlation between seasonal changes and TC formation. Therefore, the NIO favours the formation of more intense BoB TCs in the post-monsoon season and less frequent but intense ARB TCs in the pre-monsoon season (Nadimpalli et al., 2021).
2.3 Role of land surface
The land is a sink for TCs, and they get energetic when crossing the lands (Pattanayak and Mohanty, 2010). Advancements in data assimilation techniques have improved the forecast skills of the NWP models in wide ranges during pre-and post-monsoon seasons. Also, land surface features have helped to enhance the mesoscale features such as drying, precipitation, and deep convection. It further helps in better representation of Land Surface Models (LSM), Land use and Land Cover (LULC), soil moisture and other parameters (Osuri et al., 2017). Raju et al. (2011) progressively showed the importance of land data assimilation in improving the TC’s intensity prediction through better representation of boundary layer flux exchange. Rajesh et al. (2017) confirmed the same conclusion of the role of the land surface in mesoscale moist convection. Scholarly articles indicate the inland role in a TC, whereas the “Brown Ocean” concept was also introduced, indicating the role of wetland in mimicking the ocean to fuel moisture to the TC intensification over the many regions across the world (Andersen and Shepherd, 2017). Nair et al. (2019) reported the role of LULC change, soil moisture, heat flux in the intensification of TC over land. Mohanty et al. (2001) mentioned in the Indian Ocean Experiment (INDOEX) that deep offshore plume-like structure resulting from diurnal variability and topography heterogeneity plays a vital role in modulating the local and large-scale circulation patterns. Chang et al. (2009) have signified the role of soil condition before the storm in predicting the landfalling storm. Kishtawal et al. (2012) analyzed the role of change in soil bulk density and change in land features over the decay of the TCs and post-landfall intensity changes. However, there are very few studies to evaluate the role of land in TC’s intensification and weakening. Also, the forecast of accurate landfall and post-landfall intensification is a question of debate. Henceforth, many studies suggested the use of improved land force parameters for better prediction of TCs (Mohanty et al., 2001; Corsaro and Toumi, 2017; Rajesh et al., 2017).
3 Multidisciplinary approaches addressing the cyclones
3.1 Numerical modelling (standalone models)
In numerical models of the earth’s climate system and mesoscale weather events, the atmosphere, ocean, land, and cryosphere, among other elements, are mathematically represented. One of the best examples of such models is NWP models, which have been the mainstay of operational weather prediction for the last 2 decades or so. As new modelling algorithms, parameterization schemes, and faster computing resources become available, NWP is a complex and specialized field that is constantly evolving. The NWP models cover both hydrostatic and non-hydrostatic assumptions. The conservation of momentum, conservation of mass, conservation of energy, the first law of thermodynamics, equation of state, and the relationship among pressure, temperature, and density are all addressed in the fundamental governing equations of NWP models, and some of them are shown below:
Eq. 1, 2, and 3 are the horizontal momentum equation, continuity equation, and hydrostatic equation, respectively. Here, V is the horizontal wind velocity, Ps is the surface pressure, T is the temperature, and
Thus, a set of partial differential equations that explain the dynamic and thermodynamic processes in the earth’s atmosphere are used to generate the prediction or forecast. The equation set and model performance are highly influenced by the horizontal and vertical grid structure, initial and boundary conditions, and model domain.
There are various numerical models with different resolutions for predicting and projecting TCs worldwide, including NWP models, regional climate models (RCMs), and global climate models (GCMs) (Table 2). However, due to inaccurate vortex initialization of TCs, incomplete representation of complex physical processes, error in parameterization, and coarse resolution of the models, there are biases in the predictions of TC intensity, genesis and landfall that are still a challenge for forecasters (Chen et al., 2020). Osuri et al. (2012) performed numerical experiments to improve the TC intensity prediction by assimilating satellite-derived wind data. Further research improves uncertainty in the NWP system in predicting heavy rainfall associated with TC through Doppler Weather Radar (DWR) data assimilation (Mohanty et al., 2014). An example of TC simulation using the WRF model is provided in Figure 7. Simulated MSLP (Figure 7A) and surface wind at 10-m height (Figure 7B) during a VSCS Titli (2018) over the BoB was captured well by the model that shows the model’s potential to predict the TC’s over the region.
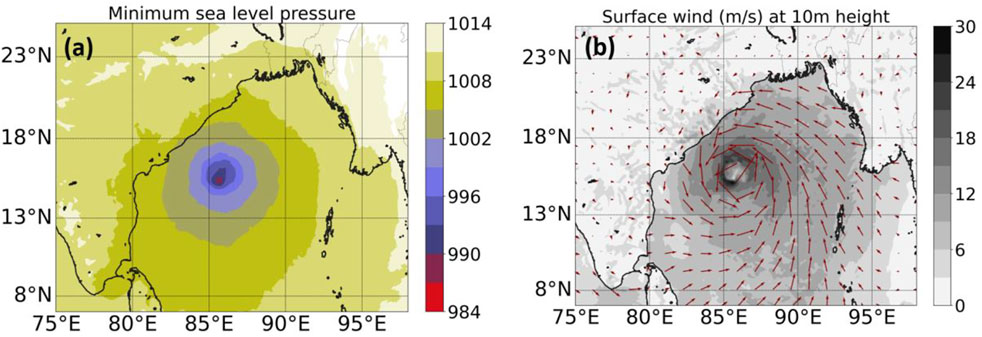
FIGURE 7. Spatial distribution of (A) MSLP and (B) 10-m surface wind during the life of cyclone Titli (2018) over the ARB using the high-resolution WRF model.
The prediction skill of the NWP model is highly reliable on the exactness of the initial state of the atmosphere. The conventional measurements like Sonde, Pilot, Profiler, Airep, Buoy, Ship, etc., have benefited the weather predictions, including the tropical belt but to a lesser extent (Guerbette et al., 2016). However, space-borne sensors provide continuous data at a high spatiotemporal resolution over sparse areas, mainly oceans. TCs are one of the deadliest natural hazards over the tropical regions to predict in terms of location and intensity to mitigate the devastation (Kumar and Shukla, 2019). The forecasting of severe weather events highly depends on moisture distribution and pre-convection environment transportation (Sieglaff et al., 2009). Though various conventional and satellite observations are routinely assimilated in the NWP model to produce a precise estimate of the initial model state (Montmerle et al., 2007), the use of satellite radiances perform an important role in the present operational data assimilation system (Kelly and Thépaut, 2007; Zupanski, 2013).
Due to a notable diversity in the observations, the Earth system modelling and its evaluation using satellite data have become challenging. Previous studies suggested that assimilation of clear-sky or cloud-cleared radiance assimilation from Microwave (MW) and Infrared (IR) sensors have improved the temperature and moisture analysis (Madhulatha et al., 2018). Zou et al. (2013) assimilated the Advanced Technology Microwave Sounder (ATMS) radiances in the HWRF model to analyze its influence on track and intensity forecasts. They suggested a consistently positive impact on model predictions. The accuracy in predicting severe weather events by numerical models can be further improved by incorporating precipitation and cloud affected radiances from MW sensors (Madhulatha et al., 2018). In practice, it is difficult to separate the effect of cloud and precipitation in the temperature and moisture observations, therefore the assimilation of such data in the NWP models is still a significant challenge (Kumar and Shukla, 2019).
In the assimilation of clear-sky radiances, a large amount of information is discarded due to the non-linearity of the system, spatial and temporal discontinuity of the clouds and precipitation, and limitations in the model dynamics. Also, most of the data assimilation techniques forcefully transform the nonlinear processes into the linearized form (Ohring and Bauer, 2011; Kumar and Shukla, 2019). To avoid these difficulties to some extent, many agencies use the clear-sky IR/MW radiance in operational forecasts (Madhulatha et al., 2018). As a result, the clear sky radiance from different MW sensors has shown a positive impact on the NWP model forecasts.
Ice and precipitation-affected radiances have a massive potential to improve accuracy (Ohring and Bauer, 2011; Zupanski, 2013; Kumar and Shukla, 2019). Modification in observation thinning and quality control allowed Zhu et al. (2016) to assimilate all-sky satellite radiances in the Grid-point Statistical Interpolation (GSI) analysis system and evaluate the outputs over the clear-sky approach. The all-sky assimilation system provided more realistic brightness temperature (TB) results and cloud water analysis increments. Yang et al. (2016) analyzed the impact of clear-sky and all-sky AMSR2 radiances in predicting Hurricane Sandy. All-sky AMSR2 assimilation experiment showed the improved forecast of MSLP, cloud distribution, and warm-core structure compared to clear-sky radiance because more precipitation/cloud-affected data was assimilated through all-sky radiance around hurricane core areas. Assimilation of MW radiometers measured radiances in cloudy and rainy areas has shown recent progress in the humidity analysis (Yang et al., 2016; Zhu et al., 2016). Previously, Guerbette et al. (2016) showed the sensitivity of the Sondeur Atmosphérique du Profil d’Humidité Intertropicale par Radiométrie (SAPHIR) sounder within cloud systems to solid precipitating hydrometeors. Singh et al. (2013) compared the NWP model simulated radiances from SAPHIR using input profiles retrieved from Atmospheric Infra-Red Sounder (AIRS) data and radiosonde profiles. Singh et al. (2013) found good agreement between SAPHIR and Microwave Humidity Sounder (MHS) TB. A representation of SAPHIR TB during the cyclone Ockhi (2017) over the NIO is also demonstrated in Figure 8.
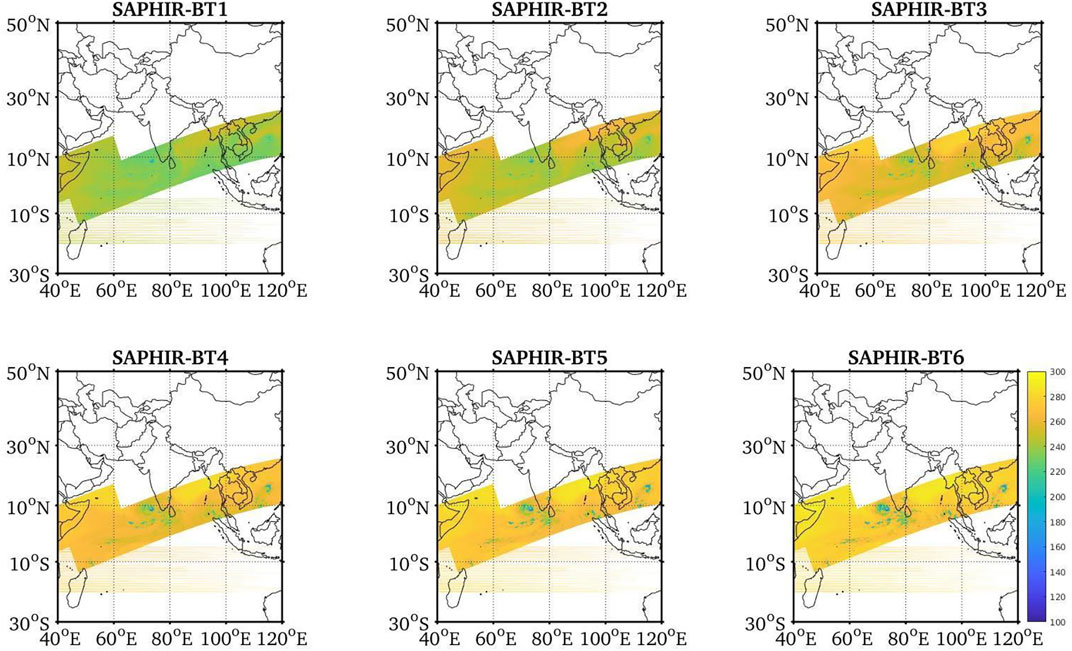
FIGURE 8. An overview of the brightness temperature dataset obtained from the 6-channels of SAPHIR, Megha-Tropiques satellite during the life of a very severe cyclonic storm Ockhi (29 November 2017–6 December 2017) over the NIO.
3.1.1 A NIO TC simulation instance with standalone WRF model
We have performed the simulation of TC Titli (2018) over the BoB basin of NIO using the WRF model. The initial and boundary conditions were taken from the “final analysis” product of the National Centers for Environmental Prediction (NCEP). The model simulation was started at 00 UTC on 9 October 2018, for a period of 120 h, and the results were compared to the “best-track” data from the IMD. The model simulation of cyclone Titli’s genesis point was southwest of the observed location. The simulated storm crossed the observed track three to four times within the first 24 h (Figure 9). Then it proceeded continually on the left side of the observed track and made landfall on India’s east coast about 6 h later, to the southwest of the actual landfall region. Further, we evaluated the mean absolute error in simulated TC’s track (Figure 10A) and intensity errors (in terms of MSW; Figure 10B) to the IMD best-track data (Figure 10). The model performed excellently for the first 24 h, with a track error of less than 50 km (Figure 10A). However, the model began to exhibit substantial errors (more than 100 km) after 30 h of lead time and continued to do so until the simulation was completed. Similarly, the model produced a reasonably good intensity simulation with a 1-day lead time (Figure 10B). The model showed a relatively large error (underprediction) at 30 and 36 h and an overestimation in the TC’s intensity simulation from 42 h onwards. Except for a few steps, the intensity error was less than or close to five hPa. In general, the model has demonstrated the ability to address the characteristics of NIO TC, while improved techniques such as 4DVar data assimilation can produce more accurate results.
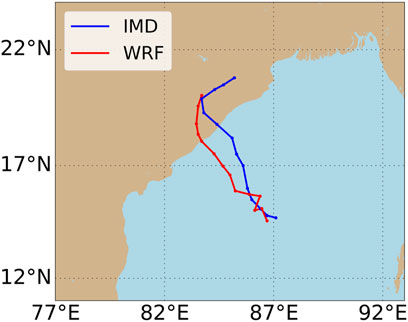
FIGURE 9. Observed (IMD best-track) and simulated (WRF) tracks of TC Titli (2018) over the NIO initialized at 00 UTC 2018–10-09 using the input forcings from the NCEP 1o × 1o final analysis product.
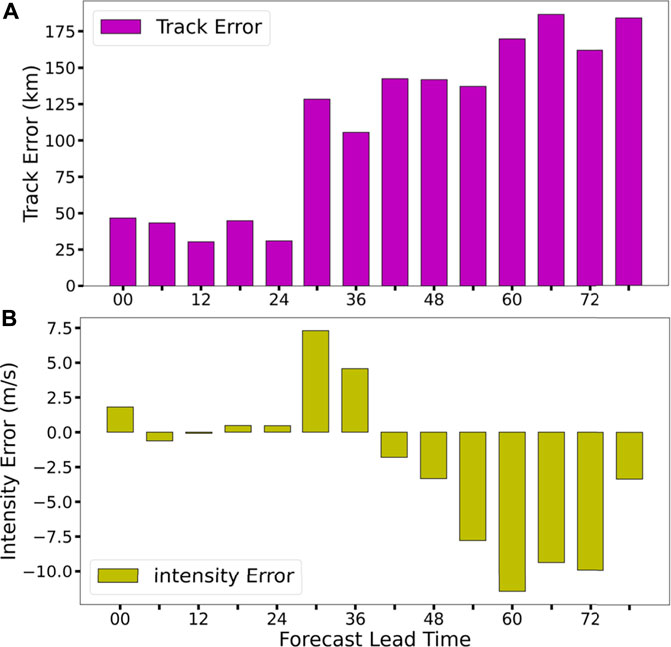
FIGURE 10. 6-hourly (A) track error (km) and (B) intensity error (m/s) in terms of MSW for the simulation of TC Titli initialized at 00 UTC 2018–10-09 using WRF model. The outputs are validated against the IMD best-track data.
The performance of the WRF model over the NIO is analogous to those of other basins. For example, Islam et al. (2015) reproduced Typhoon Haiyan’s track movement and strength over the West Pacific Ocean. The model performed well in the track simulation, but it significantly underestimated the intensity simulation. Tiwari et al. (2019) used the WRF model to simulate super typhoon Maysak over the Northwest Pacific Ocean, and the model provided reasonable results compared to observations.
3.2 Coupled models (two-way or three-way)
It is noticed that atmosphere, ocean, and land all together have a role in the TC genesis through the exchange of fluxes that ultimately affect TC intensification and track changes. Moreover, it is confirmed by Mohanty et al. (2001) that land-air-sea interaction has a significant impact on the regional circulation pattern. There is a developed strong feedback teleconnection, interaction and feedback between the regional and global scale features. Recent research mentioned the importance of the air-sea interface for intensity prediction. Following the same, there are many studies over the influence of air-sea interaction on TC physical activity and its simulation. It is well established that air-sea moisture difference and surface entropy flux increase the intensity and hence destructiveness. Single standalone models used for the TC forecasts are more biased and inaccurate due to the unrealistic feedback in the model. Such static SST is provided in the NWP models, with a high probability of biases in the results (Bender and Ginis, 2000; Bender et al., 2007). Henceforth, coupled models emerged as a helping tool to the modelling community in improving the predictability skills of the model. Many studies have been done so far to test the predicting skills of coupled ocean-atmosphere models over NIO and other oceans. For example, the sensitivity experiment of the HWRF-POM/HYCOM coupled ocean-atmosphere model was conducted to demonstrate the predicting skills of these widely used regional models (Mohanty et al., 2019). Another study using WRF-ROMS demonstrated the benefits of a coupled atmosphere-ocean model for obtaining realistic simulations of atmospheric and oceanic parameters under the extreme weather conditions associated with a very intense cyclone such as TC Phailin (Prakash and Pant, 2017). There are more studies observed using coupling between ocean-atmosphere but three ways coupled models are significantly less explored. However, few studies demonstrated the usefulness of the inclusion of realistic land surface features has the potential to improve the predicting skills of models.
3.3 Statistical approach
Studies show that using a statistical approach is a challenge. The statistical models and methods have limitations in TC prediction beyond 24 h over a heterogeneous environment like NIO (Mohanty and Gupta, 1997; Gupta, 2006). However, these methods are not far behind in contributing to the improvement in TC forecast. Statistical approaches are used to correct the data, fill the gaps for data, bias correction, downscaling, and many more ways to explore the correlation between environmental drivers and TC activity. A study was conducted by Lee et al. (2020) using the statistical downscaling approach to investigate the TC’s frequency, and the result indicates that future TCs will be more devastating (more intense). Data used in NWP models is always a hindrance in the TC forecast. Statistical approaches help improve the quality of input data for initial and boundary conditions and post-processing.
3.4 Machine learning methods
Orthodox statistical models often fail to address the nonlinear and complex relationship between TC predictors (Demaria et al., 2005; Lee et al., 2015; Wang et al., 2015). To solve such challenges, the concept of machine learning is being used to explore the observational datasets to improve the TCs forecast skills (Chen et al., 2020). It also helps to improve the uncertainties in the NWP models by enhancing the pre-processing, i.e., refining the initial condition of a model through data assimilation techniques. Artificial intelligence, a part of machine learning, is a new development to the current century. According to their applications, we can divide the machine learning algorithms into three categories: feature selection, clustering, and regression (Chen et al., 2020). Richman et al. (2017) applied the support vector regression (SVR) method to an initial predictor pool to reduce the TC seasonal prediction errors. Alemany et al. (2019) proposed a fully connected recurrent neural network to predict the TCs trajectory. Their method successfully predicted the hurricane’s track up to 120 h with reduced error.
3.5 Observational datasets
Once the TC approaches the land, the automatic weather station (AWS) and conventional observations such as sonde, pilot, profiler, airep, buoy, ship, and doppler weather radar data are relevant for TC prediction. Because conventional observations over the ocean are unavailable, satellite data are being used and have shown to be significant for studying and understanding TC characteristics (Jaiswal et al., 2017). Microwave scatterometers have been effective in studying cyclogenesis in its early phases (Sharp et al., 2002). The use of a sea-winds scatterometer has been valuable in understanding tropical disturbances (Sharp et al., 2002; Li et al., 2003). Jaiswal and Kishtawal (2011) and Jaiswal et al. (2013) applied the scatterometer derived surface wind. They used a wind pattern matching based technique that relied on the availability of a cyclonic disturbance during the satellite overpass.
Apart from conventional observations, the reanalysis products from the European Centre for Medium-Range Weather Forecasts (ERA-I, ERA-40, and ERA-5, and others), National Centers for Environmental Prediction—National Center for Atmospheric Research, Modern-Era Retrospective Analysis for Research and Applications, etc., are widely used in many kinds of literature to study the TCs, such as Evan et al. (2006); Balaji et al. (2018); Duan et al. (2021); Tiwari et al. (2021); Ranji et al. (2022); etc.
3.6 Intercomparison of strength and weakness of various approaches
The numerical modelling approach is demarcated as best suited for operational forecasting; however, the coupled models are best to perform the cyclone related research (Table 3).
3.7 Performance comparison of various approaches
The simulation of cyclone Phailin induced storm surge and inundation was carried out by means of ADvanced CIRCulation (ADCIRC) model by Kumar et al. (2015). Phailin made landfall on 12 October 2013 at Odisha (east coast of India). Within a few days of landfall, the model-simulated inundation extent corresponded closely with field surveys at Ganjam, Odisha. Furthermore, the model nicely simulated the temporal evolution of the surge residual based on measurements from a tide gauge in Paradip. However, the model slightly underestimated the magnitude compared to observations, which might be attributed to the model’s paucity of wave setup and uncertainty in wind and pressure parameters. More or less a similar kind of study was performed by Murty et al. (2014) by implementing a coupled wave + surge hydrodynamic modeling system to simulate storm surge caused by cyclone Phailin. The coupled model setup provides a realistic representation of the dynamic interaction of currents, waves, tides, and wind, which is important for operational forecasts.
Saxby et al. (2021) compared the performance of a regional convection-permitting atmosphere-ocean coupled model, i.e., the Met Office Unified Model atmosphere-only configuration and three-dimensional dynamical ocean model for the simulations of BoB TCs. Results showed that the atmosphere-only configuration produced stronger TCs than the coupled configuration; however, both model configurations reasonably represented the TC dynamics. Sahoo et al. (2019) evaluated the impact of cyclone Phailin’s strong winds and heavy rainfall on the power distribution network. The data from the tailored WRF model was found to be highly effective in managing power distribution and transmission networks in electric power grids. A thorough inspection of the results reveals that the atmospheric model fared well to capture power line tripping time. This study’s overall knowledge offers a greater scope for developing a framework for efficient power network planning operations, resource allocation, and disaster preparedness. Kumar et al. (2017) evaluated the WRF model with nested domains (horizontal resolutions of 27 and 9 km for outer and inner domains, respectively) to simulate cyclone Phailin. Compared to the domain-1 simulation, the cyclone’s intensity was well simulated in domain-2. In the domain-2 simulation, the average root mean square error and standard deviations of surface wind and MSLP were significantly lower. The track of cyclone Phailin was also well demonstrated by domain-2 simulation with respect to IMD best-track observation.
4 Current status of the NIO cyclones prediction
Many studies in recent years have shown remarkable advances in NIO cyclones’ track prediction; nonetheless, intensity prediction and rapid intensity prediction remain a crucial challenge for researchers and the scientific community. This section discusses the literature on the current status of NIO TCs, covering characteristics such as genesis, track, intensification, landfall, rainfall, and so on.
4.1 Status of cyclones genesis, track, intensification, and RI
For NIO cyclones real-time prediction, the IMD in New Delhi introduced some in-house global and limited area NWP models (Mohapatra et al., 2014, Mohapatra et al., 2013; Mohanty et al., 2015; Nadimpalli et al., 2020a). IMD employed the Quasi-Lagrangian model as a TC operational model with a coarser resolution and only 16 vertical levels in the twenty-first century’s first decade. Under the auspices of the ‘Forecast Demonstration Project of Landfalling TCs,’ some prestigious research centres and academic institutes in India also provide real-time TC forecasts to IMD for official usage (Mohapatra et al., 2011; Nadimpalli et al., 2020a). Since 2007, some operational centres have adopted the WRF model for real-time TC prediction. Adapting such mesoscale models like WRF and HWRF for the NIO region has been a significant development in recent years. Some works of literature such as Kanase and Salvekar (2015); Mahala et al. (2021); Osuri et al. (2012); Pattanayak et al. (2012a), and Raju et al. (2011) conducted dedicated simulations to determine the optimal parameterization scheme set up within the WRF model for the NIO region. The WRF model was used by Osuri et al. (2013) to simulate 17 NIO TCs. The TCs movement had an eastward and sluggish bias. Track errors ranged from 113 to 375 km with a model resolution of 27 km. Pattanayak et al. (2012b) and Mohanty et al. (2013) found better results with the HWRF model for TCs’ track prediction and improved intensity prediction over the BoB. Nadimpalli et al. (2020a) evaluated the forecasts from WRF and HWRF models in a quasi-operational setup for 10 BoB TCs from 2013 to 2017. Both models performed well for forecasts up to 30 h; however, HWRF generated more accurate results for longer forecasts.
Vinodhkumar et al. (2022) studied the climatology of NIO cyclone RI behaviour from 1990 to 2019. If the MSW speed of a cyclone increases by 15.4 m/s or greater in 24 h, it is classified as RI. During the time period given above, 46 NIO cyclones had the RI feature, with a considerable increase beginning in the year 2000. The post-monsoon season proved more favourable for RI TCs. While around 70% of RI TCs migrated north-westward or westward, eastern states of India (Tamil Nadu, and Andhra Pradesh) are more vulnerable to RI TCs. A possible cause behind the increase in the RI TCs over the NIO could be the higher moisture and SST over the cyclogenesis locations than the non-RI TCs (Nadimpalli et al., 2021). Munsi et al. (2021) used the WRF model to perform three-dimensional variational-Ensemble Kalman Filter data assimilation for three cyclones over the NIO: Fani, Ockhi, and Luban. To investigate the RI of these TCs, upper-air observations, radiometer wind, and radiance data were assimilated. In all cases, the model framework correctly simulated the RI.
4.2 Status of cyclones landfall and rainfall
Osuri et al. (2013) reported the WRF model to be more efficient in predicting the landfall location than the other attributes. Govindankutty et al. (2010) showed a positive impact of assimilating the conventional and Doppler weather radar radial wind assimilation on the intensity and rainfall distribution of NIO TCs. Sandeep et al. (2017) assimilated the Doppler weather radar wind profiles in the 3DVar and Hybrid 3DVar mode and found a minimal improvement in the TC’s zonal and meridional winds simulation. Gopalakrishnan and Chandrasekar (2018) performed the assimilation of satellite-derived winds, satellite radiance, and conventional observations with 3DVar and four-dimensional variational (4DVar) mode for the first time over the NIO for 4 TCs. The 4DVar experiments revealed better results than 3DVar in terms of track and intensity and rainfall prediction.
5 Conclusion
This study has provided the literature review on the climatology and recent progress in the tropical cyclone (TC) prediction over the North Indian Ocean (NIO) along with various methods to study them. Although the authors have tried to include as many aspects as possible, some studies could have been missed because of the rapid advancement of this research area. Several important facets of TC have been discussed, including the earth’s components and multidisciplinary approaches for TC prediction. In recent years, a rise in the NIO sea surface temperature, especially in the Arabian Sea, has been a primary factor for the genesis of highly intense TCs. Along with SST, vertical profiles of tropical cyclone heat potential in the ocean also affect the TC formation and intensification. A direct correlation of TC frequency with seasonal changes successfully claims the genesis of intense Arabian Sea (Bay of Bengal) TCs in the pre-monsoon (post-monsoon) season. At India Meteorological Department in New Delhi, operational forecasting of the NIO TCs is mainly being done by numerical modelling systems, including Weather Research and Forecasting and Hurricane Weather Research Forecasting models. Recent studies have shown significant improvements in TCs prediction with the assimilations of remote sensing observations. However, the improvement in the intensity and rapid intensity prediction of NIO cyclones is still of major concern. There is sufficient literature on three-dimensional variational (3DVar) data assimilations; very few studies on four-dimensional variational (4DVar) data assimilations over the NIO region are available. Being a more accurate technique, further scientific development with the 4DVar data assimilation method for NIO TCs prediction is on high priority in the present scenario.
Author contributions
All authors listed have made a substantial, direct, and intellectual contribution to the work and approved it for publication.
Funding
The first author is thankful to the Department of Science and Technology, Government of India, for giving the DST-INSPIRE research fellowship, registration number IF160165. Indian Institute of Science Education and Research (IISER) Bhopal has provided the research facilities and lab environment. PK acknowledges funding from the Science and Engineering Research Board (SERB), Department of Science and Technology (DST), Government of India, Grant Number CRG/2021/001227.
Conflict of interest
The authors declare that the research was conducted in the absence of any commercial or financial relationships that could be construed as a potential conflict of interest.
Publisher’s note
All claims expressed in this article are solely those of the authors and do not necessarily represent those of their affiliated organizations, or those of the publisher, the editors and the reviewers. Any product that may be evaluated in this article, or claim that may be made by its manufacturer, is not guaranteed or endorsed by the publisher.
References
Albert, J., Krishnan, A., Bhaskaran, P. K., and Singh, K. S. (2022). Role and influence of key atmospheric parameters in large-scale environmental flow associated with tropical cyclogenesis and ENSO in the North Indian Ocean basin. Clim. Dyn. 58, 17–34. doi:10.1007/s00382-021-05885-8
Alemany, S., Beltran, J., Perez, A., and Ganzfried, S. (2019). “Predicting hurricane trajectories using a recurrent neural network,” in The Thirty-Third AAAI Conference on Artificial Intelligence, Hilton Hawaiian Village, Honolulu, Hawaii, USA, Jan 27–Feb 1, 2019.
Andersen, T., and Shepherd, M. (2017). “Inland tropical cyclones and the “Brown ocean” concept,” in Hurricanes and climate change. Editors J. Collins, and K. Walsh (Cham: Springer). doi:10.1007/978-3-319-47594-3_5
Balaji, M., Chakraborty, A., and Mandal, M. (2018). Changes in tropical cyclone activity in north Indian Ocean during satellite era (1981–2014). Int. J. Climatol. 38, 2819–2837. doi:10.1002/joc.5463
Bender, M. A., and Ginis, I. (2000). Real-case simulations of hurricane–ocean interaction using A high-resolution coupled model: Effects on hurricane intensity. Mon. Weather Rev. 128, 917–946. doi:10.1175/1520-0493(2000)128<0917:rcsoho>2.0.co;2
Bender, M. A., Tuleya, R., Thomas, B., and Marchok, T. (2007). The operational GFDL coupled hurricane–ocean prediction system and a summary of its performance. Mon. Weather Rev. 135, 3965–3989. doi:10.1175/2007mwr2032.1
Bhatla, R., Raj, R., Mall, R. K., and Shivani, (2020). “Tropical cyclones over the north Indian ocean in changing climate,” in Techniques for disaster risk management and mitigation. Editors P. K. Srivastava, S. K. Singh, U. C. Mohanty, and T. Murty. 1st ed. (Hoboken, New Jersey, United States: Wiley), 63–76. doi:10.1002/9781119359203.ch5
Chan, J. C. L. (2005). The physics of tropical cyclone motion. Annu. Rev. Fluid Mech. 37, 99–128. doi:10.1146/annurev.fluid.37.061903.175702
Chang, H.-I., Niyogi, D., Kumar, A., Kishtawal, C. M., Dudhia, J., Chen, F., et al. (2009). Possible relation between land surface feedback and the post-landfall structure of monsoon depressions: Land surface and monsoon depressions. Geophys. Res. Lett. 36, 1–6. doi:10.1029/2009GL037781
Chauhan, A., Singh, R. P., Dash, P., and Kumar, R. (2021). Impact of tropical cyclone “Fani” on land, ocean, atmospheric and meteorological parameters. Mar. Pollut. Bull. 162, 111844. doi:10.1016/j.marpolbul.2020.111844
Chen, R., Zhang, W., and Wang, X. (2020). Machine learning in tropical cyclone forecast modeling: A review. Atmosphere 11, 676. doi:10.3390/atmos11070676
Corsaro, C. M., and Toumi, R. (2017). A self‐weakening mechanism for tropical cyclones. Q. J. R. Meteorol. Soc. 143, 2585–2599. doi:10.1002/qj.3109
Courtney, J. B., Langlade, S., Sampson, C. R., Knaff, J. A., Birchard, T., Barlow, S., et al. (2019). Operational perspectives on tropical cyclone intensity change Part 1: Recent advances in intensity guidance. Trop. Cyclone Res. Rev. 8, 123–133. doi:10.1016/j.tcrr.2019.10.002
Das, Y., Mohanty, U. C., Jain, I., Subba Rao, M., and Murty, A. S. N. (2014). Modeling on the aspects of thermal response of Bay of bengal to tropical cyclone TC05B 1999 using Princeton Ocean model (POM): Preliminary results. Am. J. Model. Optim. 2, 47–59. doi:10.12691/ajmo-2-2-2
Demaria, M., and Kaplan, J. (1994). A statistical hurricane intensity prediction scheme (SHIPS) for the atlantic basin. Wea. Forecast. 9, 209–220. doi:10.1175/1520-0434(1994)009<0209:aships>2.0.co;2
Demaria, M., Mainelli, M., Shay, L. K., Knaff, J. A., and Kaplan, J. (2005). Further improvements to the statistical hurricane intensity prediction scheme (SHIPS). Weather Forecast. 20, 531–543. doi:10.1175/waf862.1
Deshpande, M., Singh, V. K., Ganadhi, M. K., Roxy, M. K., Emmanuel, R., and Kumar, U. (2021). Changing status of tropical cyclones over the north Indian Ocean. Clim. Dyn. 57, 3545–3567. doi:10.1007/s00382-021-05880-z
Duan, W., Yuan, J., Duan, X., and Feng, D. (2021). Seasonal variation of tropical cyclone genesis and the related large-scale environments: Comparison between the Bay of bengal and Arabian Sea sub-basins. Atmosphere 12, 1593. doi:10.3390/atmos12121593
Espejo, A., Mendez, F. J., Diez, J., Medina, R., and Al-Yahyai, S. (2016). Seasonal probabilistic forecasting of tropical cyclone activity in the North Indian Ocean: Forecasting tropical cyclone activity. J. Flood Risk Manag. 9, 379–389. doi:10.1111/jfr3.12197
Frank, W. M., and Young, G. S. (2007). The interannual variability of tropical cyclones. Mon. Weather Rev. 135, 3587–3598. doi:10.1175/mwr3435.1
Gopalakrishnan, D., and Chandrasekar, A. (2018). On the improved predictive skill of WRF model with regional 4DVar initialization: A study with north Indian Ocean tropical cyclones. IEEE Trans. Geosci. Remote Sens. 56, 3350–3357. doi:10.1109/tgrs.2018.2798623
Govindankutty, M., Chandrasekar, A., and Pradan, D. (2010). Impact of 3DVAR assimilation of Doppler Weather Radar wind data and IMD observation for the prediction of a tropical cyclone. Int. J. Remote Sens. 31, 6327–6345. doi:10.1080/01431160903413689
Guerbette, J., Mahfouf, J. F., and Plu, M. (2016). Towards the assimilation of all-sky microwave radiances from the SAPHIR humidity sounder in a limited area NWP model over tropical regions. Tellus A Dyn. Meteorology Oceanogr. 68, 28620. doi:10.3402/tellusa.v68.28620
Gupta, A. (2006). Current status of Tropical Cyclone track prediction techniques and forecast errors. Mausam 57, 151–158. doi:10.54302/mausam.v57i1.464
Gupta, S., Jain, I., Johari, P., and Lal, M. (2019). “Impact of climate change on tropical cyclones frequency and intensity on Indian coasts,” in Proceedings of International Conference on Remote Sensing for Disaster Management, Visakhapatnam, India, October 11–13, 2017. Editors P. J. Rao, K. N. Rao, and S. Kubo (Springer International Publishing), 359–365. doi:10.1007/978-3-319-77276-9_32
Islam, T., Srivastava, P. K., Rico-Ramirez, M. A., Dai, Q., Gupta, M., and Singh, S. K. (2015). Tracking a tropical cyclone through WRF–ARW simulation and sensitivity of model physics. Nat. Hazards (Dordr). 76, 1473–1495. doi:10.1007/s11069-014-1494-8
Jadhav, S. K., and Munot, A. A. (2009). Warming SST of Bay of Bengal and decrease in formation of cyclonic disturbances over the Indian region during southwest monsoon season. Theor. Appl. Climatol. 96, 327–336. doi:10.1007/s00704-008-0043-3
Jaiswal, N., Kishtawal, C. M., and Pal, P. K. (2013). Prediction of tropical cyclogenesis in North Indian Ocean using Oceansat-2 scatterometer (OSCAT) winds. Meteorol. Atmos. Phys. 119, 137–149. doi:10.1007/s00703-012-0230-8
Jaiswal, N., Kishtawal, C. M., and Pal, P. K. (2017). “Tropical cyclogenesis prediction in the north Indian ocean during 2013 using OSCAT derived surface wind observations,” in Tropical cyclone activity over the north Indian ocean. Editors M. Mohapatra, B. Bandyopadhyay, and L. Rathore (Cham: Springer). doi:10.1007/978-3-319-40576-6_14
Jaiswal, N., and Kishtawal, C. M. (2011). Prediction of tropical cyclogenesis using scatterometer data. IEEE Trans. Geosci. Remote Sens. 49, 4904–4909. doi:10.1109/tgrs.2011.2153862
Jangir, B., Swain, D., and Ghose, S. K. (2020). Influence of eddies and tropical cyclone heat potential on intensity changes of tropical cyclones in the North Indian Ocean. Adv. Space Res. 68, 773–786. S0273117720300296. doi:10.1016/j.asr.2020.01.011
Kanase, R. D., and Salvekar, P. S. (2015). Effect of physical parameterization schemes on track and intensity of cyclone LAILA using WRF model. Asia. Pac. J. Atmos. Sci. 51, 205–227. doi:10.1007/s13143-015-0071-8
Kelly, G., and Thépaut, J. N. (2007). Evaluation of the impact of the space component of the global observing system through observing system experiments. ECMWF Newsl. 113, 16–28.
Kishtawal, C. M., Niyogi, D., Kumar, A., Bozeman, M. L., and Kellner, O. (2012). Sensitivity of inland decay of North Atlantic tropical cyclones to soil parameters. Nat. Hazards (Dordr). 63, 1527–1542. doi:10.1007/s11069-011-0015-2
Kumar, P., and Shukla, M. V. (2019). Assimilating INSAT‐3D thermal infrared window imager observation with the particle filter: A case study for vardah cyclone. J. Geophys. Res. Atmos. 124, 1897–1911. doi:10.1029/2018jd028827
Kumar, S., Routray, A., Tiwari, G., Chauhan, R., and Jain, I. (2017). “Simulation of tropical cyclone ‘Phailin’ using WRF modeling system,” in Tropical cyclone activity over the north Indian ocean. Editors M. Mohapatra, B. K. Bandyopadhyay, and L. S. Rathore (Midtown Manhattan, New York: Springer International Publishing), 307–316. doi:10.1007/978-3-319-40576-6_21
Kumar, T. S., Murty, P. L. N., Kumar, M. P., Kumar, M. K., Padmanabham, J., Kumar, N. K., et al. (2015). Modeling storm surge and its associated inland inundation extent due to very severe cyclonic storm Phailin. Mar. Geod. 38 (4), 345–360. doi:10.1080/01490419.2015.1053640
Lee, C.-Y., Camargo, S. J., Sobel, A. H., and Tippett, M. K. (2020). Statistical–dynamical downscaling projections of tropical cyclone activity in a warming climate: Two diverging genesis scenarios. J. Clim. 33, 4815–4834. doi:10.1175/JCLI-D-19-0452.1
Lee, C. Y., Tippett, M. K., Camargo, S. J., and Sobel, A. H. (2015). Probabilistic multiple linear regression modeling for tropical cyclone intensity. Mon. Weather Rev. 143, 933–954. doi:10.1175/mwr-d-14-00171.1
Li, T., Fu, B., Ge, X., Wang, B., and Peng, M. (2003). Satellite data analysis and numerical simulation of tropical cyclone formation. Geophys. Res. Lett. 30, 2122. doi:10.1029/2003gl018556
Lin, I.-I., Goni, G. J., Knaff, J. A., Forbes, C., and Ali, M. M. (2013). Ocean heat content for tropical cyclone intensity forecasting and its impact on storm surge. Nat. Hazards (Dordr). 66, 1481–1500. doi:10.1007/s11069-012-0214-5
Madhulatha, A., Rajeevan, M., Bhowmik, S. R., and Das, A. K. (2018). Impact of assimilation of conventional and satellite radiance GTS observations on simulation of mesoscale convective system over Southeast India using WRF-3DVar. Pure Appl. Geophys. 175, 479–500. doi:10.1007/s00024-017-1689-5
Mahala, B. K., Mohanty, P. K., Xalxo, K. L., Routray, A., and Misra, S. K. (2021). Impact of WRF parameterization schemes on track and intensity of extremely severe cyclonic storm ‘‘Fani. Pure Appl. Geophys. 178, 245–268. doi:10.1007/s00024-020-02629-3
Mandal, M., and Mohanty, U. C. (2010). Simulation of severe land-falling Bay of bengal cyclones during 1995–1999 using mesoscale model MM5. Mar. Geod. 33, 315–337. doi:10.1080/01490419.2010.518070
Mawren, D., and Reason, C. J. C. (2017). Variability of upper-ocean characteristics and tropical cyclones in the South West Indian Ocean. J. Geophys. Res. Oceans 122, 2012–2028. doi:10.1002/2016JC012028
Mishra, A. K., Dwivedi, S., Di Sante, F., and Coppola, E. (2020). Thermodynamical properties associated with the Indian summer monsoon rainfall using a regional climate model. Theor. Appl. Climatol. 141, 587–599. doi:10.1007/s00704-020-03237-w
Mohanty, S., Nadimpalli, R., Mohanty, U. C., Mohapatra, M., Sharma, A., Das, A. K., et al. (2020). Quasi-operational forecast guidance of extremely severe cyclonic storm Fani over the Bay of Bengal using high-resolution mesoscale models. Meteorol. Atmos. Phys. 133, 331–348. doi:10.1007/s00703-020-00751-4
Mohanty, U. C., and Gupta, A. (1997). Deterministic methods for prediction of Tropical Cyclone tracks. Mausam 48, 257–272. doi:10.54302/mausam.v48i2.4008
Mohanty, U. C., Nadimpalli, R., Mohanty, S., and Osuri, K. K. (2019). Recent advancements in prediction of tropical cyclone track over north Indian Ocean basin. Mausam 70, 57–70. doi:10.54302/mausam.v70i1.167
Mohanty, U. C., Niyogi, D., Raman, S., and Sarkar, A. (2001). Numerical study of the role of land-air-sea interactions for the northeasterly monsoon circulations over Indian Ocean during INDOEX. Curr. Sci. 80, 60–68.
Mohanty, U. C., Osuri, K. K., and Pattanayak, S. (2013). A study on high resolution mesoscale modeling systems for simulation of tropical cyclones over the Bay of Bengal. Mausam 64, 117–134. doi:10.54302/mausam.v64i1.661
Mohanty, U. C., Osuri, K. K., and Pattanayak, S. (2014). “Mesoscale modelling for tropical cyclone forecasting over the north Indian ocean,” in Monitoring and prediction of tropical cyclones in the Indian ocean and climate change. Editors U. C. Mohanty, M. Mohapatra, O. P. Singh, B. K. Bandyopadhyay, and L. S. Rathore (Dordrecht, Netherlands: Springer Netherlands), 274–286. doi:10.1007/978-94-007-7720-0_24
Mohanty, U. C., Osuri, K. K., Pattanayak, S., and Sinha, P. (2012). An observational perspective on tropical cyclone activity over Indian seas in a warming environment. Nat. Hazards (Dordr). 63, 1319–1335. doi:10.1007/s11069-011-9810-z
Mohanty, U. C., Osuri, K. K., Tallapragada, V., Marks, F. D., Pattanayak, S., Mohapatra, M., et al. (2015). A great escape from the Bay of bengal “super sapphire–phailin” tropical cyclone: A case of improved weather forecast and societal response for disaster mitigation. Earth Interact. 19, 1–11. doi:10.1175/EI-D-14-0032.1
Mohapatra, M., Bandyopadhyay, B. K., and Tyagi, A. (2014). “Status and plans for operational tropical cyclone forecasting and warning systems in the north Indian ocean region,” in Monitoring and prediction of tropical cyclones in the Indian ocean and climate change. Editors U. C. Mohanty, M. Mohapatra, O. P. Singh, B. K. Bandyopadhyay, and L. S. Rathore (Dordrecht: Springer).
Mohapatra, M. (2011). Forecast demonstration project (FDP) for improving track, intensity and landfall of Bay of Bengal tropical cyclones implementation of pilot phase, 2010- a report. New Delhi: IMD.
Mohapatra, M. (2016). “Monitoring and forecasting of tropical cyclones over north Indian ocean,” in Advanced numerical modeling and data assimilation techniques for tropical cyclone prediction. Editors U. C. Mohanty, and S. G. Gopalakrishnan (Dordrecht, Netherlands: Springer Netherlands), 409–447. doi:10.5822/978-94-024-0896-6_16
Mohapatra, M., Sikka, D. R., Bandyopadhyay, B. K., and Tyagi, A. (2013). Outcomes and challenges of forecast demonstration project (FDP) on landfalling cyclones over the Bay of Bengal. Mausam 64, 1–12. doi:10.54302/mausam.v64i1.650
Mondal, M., Biswas, A., Haldar, S., Mandal, S., Bhattacharya, S., and Paul, S. (2021). Spatio-temporal behaviours of tropical cyclones over the Bay of bengal basin in last five decades, Trop. Cyclone Res. Rev., 11, 1–15. doi.org/doi:10.1016/j.tcrr.2021.11.004
Montmerle, T., Rabier, F., and Fischer, C. (2007). Relative impact of polar-orbiting and geostationary satellite radiances in the Aladin/France numerical weather prediction system. Q. J. R. Meteorol. Soc. 133, 655–671. doi:10.1002/qj.34
Munsi, M., Kesarkar, A., Bhate, J., Panchal, A., Singh, K., Kutty, G., et al. (2021). Rapidly intensified, long duration North Indian Ocean tropical cyclones: Mesoscale downscaling and validation. Atmos. Res. 259, 105678. doi:10.1016/j.atmosres.2021.105678
Murty, P. L. N., Sandhya, K. G., Bhaskaran, P. K., Jose, F., Gayathri, R., Nair, T. M. B., et al. (2014). A coupled hydrodynamic modeling system for PHAILIN cyclone in the Bay of Bengal. Coast. Eng. 93, 71–81. doi:10.1016/j.coastaleng.2014.08.006
Nadimpalli, R., Mohanty, S., Pathak, N., Osuri, K. K., Mohanty, U. C., and Chatterjee, S. (2021). Understanding the characteristics of rapid intensity changes of tropical cyclones over north Indian ocean. SN Appl. Sci. 3, 68. doi:10.1007/s42452-020-03995-2
Nadimpalli, R., Osuri, K. K., Mohanty, U. C., Das, A. K., Kumar, A., Sil, S., et al. (2020a). Forecasting tropical cyclones in the Bay of Bengal using quasi-operational WRF and HWRF modeling systems: An assessment study. Meteorol. Atmos. Phys. 132, 1–17. doi:10.1007/s00703-019-00669-6
Nadimpalli, R., Srivastava, A., Prasad, V. S., Osuri, K. K., Das, A. K., Mohanty, U. C., et al. (2020b). Impact of INSAT-3D/3DR radiance data assimilation in predicting tropical cyclone Titli over the Bay of bengal. IEEE Trans. Geosci. Remote Sens. 58, 6945–6957. doi:10.1109/TGRS.2020.2978211
Nair, U. S., Rappin, E., Foshee, E., Smith, W., Pielke, R. A., Mahmood, R., et al. (2019). Influence of land cover and soil moisture based Brown ocean effect on an extreme rainfall event from a Louisiana gulf coast tropical system. Sci. Rep. 9, 17136. doi:10.1038/s41598-019-53031-6
Ohring, G., and Bauer, P. (2011). The use of cloud and precipitation observations in data assimilation (CPDA). Q. J. R. Meteorol. Soc. 137, 1933. doi:10.1002/qj.946
Osuri, K. K., Mohanty, U. C., Routray, A., Kulkarni, M. A., and Mohapatra, M. (2012). Customization of WRF-ARW model with physical parameterization schemes for the simulation of tropical cyclones over North Indian Ocean. Nat. Hazards (Dordr). 63, 1337–1359. doi:10.1007/s11069-011-9862-0
Osuri, K. K., Mohanty, U. C., Routray, A., Mohapatra, M., and Niyogi, D. (2013). Real-time track prediction of tropical cyclones over the north Indian ocean using the ARW model. J. Appl. Meteorology Climatol. 52, 2476–2492. doi:10.1175/jamc-d-12-0313.1
Osuri, K. K., Nadimpalli, R., Mohanty, U. C., Chen, F., Rajeevan, M., and Niyogi, D. (2017). Improved prediction of severe thunderstorms over the Indian Monsoon region using high-resolution soil moisture and temperature initialization. Sci. Rep. 7, 41377. doi:10.1038/srep41377
Osuri, K. K., Routray, A., Mohanty, U. C., and Kulkarni, M. A. (2010). “Simulation of tropical cyclones over Indian seas: Data impact study using WRF-var assimilation system,” in Indian Ocean tropical cyclones and climate change. Editor Y. Charabi (Dordrecht, Netherlands: Springer Netherlands), 115–124. doi:10.1007/978-90-481-3109-9_15
Patnaik, K. V. K. R. K., Maneesha, K., Sadhuram, Y., Prasad, K. V. S. R., Ramana Murty, T. V., and Brahmananda Rao, V. (2014). East India Coastal Current induced eddies and their interaction with tropical storms over Bay of Bengal. J. Operational Oceanogr. 7, 58–68. doi:10.1080/1755876X.2014.11020153
Pattanayak, S., Mohanty, U. C., and Gopalakrishnan, S. G. (2012b). Simulation of very severe cyclone mala over Bay of bengal with HWRF modeling system. Nat. Hazards 63, 1413–1437. doi:10.1007/s11069-011-9863-z
Pattanayak, S., Mohanty, U. C., and Osuri, K. K. (2012a). Impact of parameterization of physical processes on simulation of track and intensity of tropical cyclone nargis (2008) with WRF-NMM model. Sci. World J., 2012, 1–18. doi:10.1100/2012/671437
Pattanayak, S., and Mohanty, U. C. (2010). “Simulation of track and intensity of gonu and sidr with WRF-NMM modeling system,” in Indian Ocean tropical cyclones and climate change. Editor Y. Charabi (Dordrecht, Netherlands: Springer Netherlands), 83–89. doi:10.1007/978-90-481-3109-9_12
Patwardhan, S. K., and Bhalme, H. N. (2001). A study of cyclonic disturbances over India and the adjacent ocean. Int. J. Climatol. 2, 527–534. doi:10.1002/joc.615
Prakash, K. R., and Pant, V. (2017). Upper oceanic response to tropical cyclone Phailin in the Bay of Bengal using a coupled atmosphere-ocean model. Ocean. Dyn. 67, 51–64. doi:10.1007/s10236-016-1020-5
Rajesh, P. V., Pattnaik, S., Mohanty, U. C., Rai, D., Baisya, H., and Pandey, P. C. (2017). Land surface sensitivity of monsoon depressions formed over Bay of Bengal using improved high-resolution land state. Dyn. Atmos. Oceans 80, 155–172. doi:10.1016/j.dynatmoce.2017.10.007
Raju, P. V. S., Potty, J., and Mohanty, U. C. (2011). Sensitivity of physical parameterizations on prediction of tropical cyclone Nargis over the Bay of Bengal using WRF model. Meteorol. Atmos. Phys. 113, 125–137. doi:10.1007/s00703-011-0151-y
Ranji, Z., Zarifsanayei, A. R., Cartwright, N., and Soltanpour, M. (2022). Climate change impacts on tropical cyclones of the Arabian Sea: Projections and uncertainty investigations. Int. J. Climatol. 1, 21. doi:10.1002/joc.7523
Richman, M. B., Leslie, L. M., Ramsay, H. A., and Klotzbach, P. J. (2017). Reducing tropical cyclone prediction errors using machine learning approaches. Procedia Comput. Sci. 114, 314–323. doi:10.1016/j.procs.2017.09.048
Sahoo, B., Bhaskaran, P. K., and Pradhan, A. K. (2019). Application of weather forecasting model WRF for operational electric power network management-a case study for Phailin cyclone. Theor. Appl. Climatol. 137, 871–891. doi:10.1007/s00704-018-2639-6
Sandeep, A., Prasad, V. S., and Johny, C. J. (2017). Quality and impact of Indian Doppler weather radar wind profiles: A diagnostic study. Pure Appl. Geophys. 174, 2847–2862. doi:10.1007/s00024-017-1544-8
Saxby, J., Crook, J., Peatman, S., Birch, C., Schwendike, J., Valdivieso da Costa, M., et al. (2021). Simulations of Bay of Bengal tropical cyclones in a regional convection-permitting atmosphere–ocean coupled model. Weather Clim. Dynam. Discuss. [preprint], in review, doi:10.5194/wcd-2021-46
Sharp, R. J., Bourassa, M. A., and Brien, J. J. O. (2002). Early detection of tropical cyclones using seawinds-derived vorticity. Bull. Am. Meteorol. Soc. 83, 879–889. doi:10.1175/1520-0477(2002)083<0879:edotcu>2.3.co;2
Sieglaff, J. M., Schmit, T. J., Menzel, W. P., and Ackerman, S. A. (2009). Inferring convective weather characteristics with geostationary high spectral resolution IR window measurements: A look into the future. J. Atmos. Ocean. Technol. 26, 1527–1541. doi:10.1175/2009jtecha1210.1
Singh, K. S., and Bhaskaran, P. K. (2020). Prediction of landfalling Bay of bengal cyclones during 2013 using the high-resolution weather research and forecasting model. Meteorol. Appl. 27. doi:10.1002/met.1850
Singh, R., Ojha, S. P., Kishtawal, C. M., and Pal, P. K. (2013). Quality assessment and assimilation of Megha-Tropiques SAPHIR radiances into WRF assimilation system. J. Geophys. Res. Atmos. 118, 6957–6969. doi:10.1002/jgrd.50502
Tiwari, G., Kumar, S., Routray, A., Panda, J., and Jain, I. (2019). A high-resolution mesoscale model approach to reproduce super typhoon Maysak (2015) over northwestern Pacific ocean. Earth Syst. Environ. 3, 101–112. doi:10.1007/s41748-019-00086-0
Tiwari, G., Rameshan, A., Kumar, P., Javed, A., and Mishra, A. K. (2021). Understanding the post-monsoon tropical cyclone variability and trend over the Bay of Bengal during the satellite era. Q. J. R. Meteorol. Soc. 148, 1–14. doi:10.1002/qj.4189
Vinodhkumar, B., Busireddy, N. K. R., Ankur, K., Nadimpalli, R., and Osuri, K. K. (2022). On occurrence of rapid intensification and rainfall changes in tropical cyclones over the North Indian Ocean. Int. J. Climatol. 42, 714–726. doi:10.1002/joc.7268
Wang, B., Xu, S., and Wu, L. (2012). Intensified Arabian Sea tropical storms. Nature 489, E1–E2. doi:10.1038/nature11470
Wang, Y., Rao, Y., Tan, Z. M., and Schönemann, D. (2015). A statistical analysis of the effects of vertical wind shear on tropical cyclone intensity change over the western north pacific. Mon. Weather Rev. 143, 3434–3453. doi:10.1175/mwr-d-15-0049.1
Wang, Y., and Wu, C.-C. (2004). Current understanding of tropical cyclone structure and intensity changes? A review. Meteorol. Atmos. Phys. 87, 257–278. doi:10.1007/s00703-003-0055-6
Webster, P. J., Holland, G. J., Curry, J. A., and Chang, H.-R. (2005). Changes in tropical cyclone number, duration, and intensity in a warming environment. Science 309, 1844–1846. doi:10.1126/science.1116448
Yang, C., Liu, Z., Bresch, J., Rizvi, S. R., Huang, X. Y., and Min, J. (2016). AMSR2 all-sky radiance assimilation and its impact on the analysis and forecast of Hurricane Sandy with a limited-area data assimilation system. Tellus A Dyn. Meteorology Oceanogr. 68, 30917. doi:10.3402/tellusa.v68.30917
Zhu, Y., Liu, E., Mahajan, R., Thomas, C., Groff, D., Van Delst, P., et al. (2016). All-sky microwave radiance assimilation in NCEP’s GSI analysis system. Mon. Weather Rev. 144, 4709–4735. doi:10.1175/mwr-d-15-0445.1
Zou, X., Weng, F., Zhang, B., Lin, L., Qin, Z., and Tallapragada, V. (2013). Impacts of assimilation of ATMS data in HWRF on track and intensity forecasts of 2012 four landfall hurricanes. J. Geophys. Res. Atmos. 118, 11–558. doi:10.1002/2013jd020405
Keywords: tropical cyclone, north indian ocean, track, intensity, numerical weather prediction
Citation: Tiwari G, Kumar P and Tiwari P (2022) The appraisal of tropical cyclones in the North Indian Ocean: An overview of different approaches and the involvement of Earth’s components. Front. Earth Sci. 10:823090. doi: 10.3389/feart.2022.823090
Received: 29 November 2021; Accepted: 21 July 2022;
Published: 25 August 2022.
Edited by:
Liguang Wu, Fudan University, ChinaReviewed by:
Suman Paul, Sidho Kanho Birsha University, IndiaEric Hendricks, National Center for Atmospheric Research (UCAR), United States
Raghavendra Ashrit, National Centre for Medium Range Weather Forecasting, India
Copyright © 2022 Tiwari, Kumar and Tiwari. This is an open-access article distributed under the terms of the Creative Commons Attribution License (CC BY). The use, distribution or reproduction in other forums is permitted, provided the original author(s) and the copyright owner(s) are credited and that the original publication in this journal is cited, in accordance with accepted academic practice. No use, distribution or reproduction is permitted which does not comply with these terms.
*Correspondence: Pankaj Kumar, a3VtYXJwQGlpc2VyYi5hYy5pbg==