- 1Department of Oceanography and Coastal Sciences, College of the Coast and Environment, Louisiana State University, Baton Rouge, LA, United States
- 2Coastal Studies Institute, Louisiana State University, Baton Rouge, LA, United States
- 3Bert S. Turner Department of Construction Management, Louisiana State University, Baton Rouge, LA, United States
Because of its ubiquitous nature and catastrophic impacts, flood information should be readily available and continually evaluated, to maximize utility for the public and professionals. Web-based tools can fill existing needs for actionable information to inform decisions regarding flood damage mitigation for new and existing structures. The goal of this research is to identify the current capabilities, gaps, and future demands of Web-based flood communication systems. To understand the current and potential niche of Web-based flood tools, a review of the literature concerning the effectiveness of mass media, grassroots-based “citizen science” efforts, and social media in communicating flood risk is conducted. Then, the strengths of 50 major, freely-available, Web-based flood information portals are reviewed. Results suggest that mass media often provide actionable information, especially for short-term benefit immediately before, during, and immediately after the flood for individuals and community leaders. Citizen science and grassroots efforts encourage planning strategies to prevent or mitigate flood. Social media is most beneficial in raising awareness of the flood hazard and communicating short- and long-term mitigation and adaptation strategies. However, while mass media, citizen science, and social media have revolutionized the way that people plan for, survive, and recover from floods, their utility is largely restricted to addressing short-term information needs at the meso-scale or broader and/or conveying information about singular events to scientists and/or other professional interests. Actionable information to inform long-term planning and mitigating flood, for both the public and community leaders, remains lacking. A particular need is for communication mechanisms that satisfy several criteria simultaneously: wide broadcast, appealing delivery method, and focus on enhancing decision-making for long-term needs rather than solely for short-term benefit. Particularly useful would be a new, webtool that provides sufficient functionality to enhance flood risk reduction decision making, considering both the costs and benefits of mitigation.
Introduction: Communication for Minimizing Flood Risk
Perennially the world’s most ubiquitous and expensive natural hazard, flooding increases in notoriety as populations and economic investment in coastal and other flood-prone areas continue to climb sharply (Dewan et al., 2006; Bushra et al., 2021). One way to enhance flood risk preparedness is through improved flood risk communication (Maidl and Buchecker 2015), especially that which provides actionable information such as previous, current, and future flood damage (Mostafiz et al., 2021a) and hazard risk, mitigation and adaptation approaches, and cost-benefit analysis. Actionable information enables informed decision making for disaster preparedness, mitigation, response, and recovery. Among the challenges in this regard are that judgments about whether a piece of information is actionable vary (Zade et al., 2018) and that “actionable” information must be filtered from “noise” (He et al., 2017), although automated categorization information based on actionability is possible to assist with these challenges (Zahera et al., 2021). Technological advances and enhanced grassroots efforts have traditionally improved communications regarding the flood risk, before, during, and after flood disasters. Such endeavors via mass media, citizen science, and social media are helpful but continue to fall short in some ways, as evidenced by the fact that losses in flood-prone areas continue to increase (Mostafiz et al., 2021b, 2021c).
The overarching goal of this research is to identify the publicly available, actionable information on existing flood risk communication tools that might help to fill the communication gap regarding the flood hazard. To accomplish this goal, we address three questions about communicating the flood risk: 1) What information exists about the success (or lack thereof) of various vehicles for communicating the flood hazard and their effects on flood hazard mitigation? 2) What actionable information is available online and required for citizens and community leaders? 3) What is the functionality for the intended users of online tools to provide actionable information to aid flood risk reduction decision making?
To support the goal, this research addresses the gap in summarizing and synthesizing the existing literature. We analyze strengths and weaknesses in each of the traditional areas of mass media, citizen science and other outreach, and social media, vis-à-vis flood risk communication. We then review online webtools that can address some of these existing gaps, with emphasis on analyzing the strengths and areas for improvement of existing Web-based portals, particularly as these relate to functionality for the intended user. Finally, we discuss the actionable information needed for laypeople and community officials to enhance long-term flood planning and mitigation strategies to build safer structures and reduce flood loss. This research provides a snapshot of the current state of flood risk communication, so that in future years when such websites are no longer available or are in greatly changed form, the scientific community will have a documentation of the state of the science and art in 2022.
Actionable Information
Actionable information as defined here refers to relevant data that span flood magnitude and occurrence, damage resulting from flood hazards, alternatives to minimize flood damage, and the associated cost of these alternatives. The time scale on which information can be “actionable” ranges, but a distinction can be considered here between short-term and long-term actionable information, with “short-term” referring to information that informs decisions in the immediate preparation and aftermath of the flood hazard, and “long-term” referring to that information that is useful for mitigating the impacts of the hazard, at the preparation or recovery stages. This distinction is important because short-term actionable information enhances the prospect of survival of the hazard, while long-term actionable information enhances resilience. In addition, both short- and long-term information can be characterized based on the primary intended audience of the message. Here we distinguish only between “individual-” and “community-” level audiences, with the former dominated by homeowners or prospective homeowners concerned about enhancing flood resilience for their investment and the latter consisting of elected officials, builders, and planners seeking to enhance the quality of life for their constituents. The present research directly considers the following as specific types of long-term actionable information, at both the individual- and community-level, against which the risk communication outlets are measured: hazard properties (i.e., flood frequency and magnitude), effects of hazards (i.e., flood damage and loss), mitigation options (e.g., flood barriers), structure resiliency (e.g., elevation, wet flood proofing), effectiveness of combinations of both hazard and building mitigation (e.g., sandbag plus elevated structure) customized for the individual’s particular situation (e.g., livable area, presence of basement, number of stories, length of time that the user plans to use the structure, whether structure is insured and if so, amount of coverage and deductible), community information (e.g., community rating system (CRS) score and steps needed and benefits of enhancing the community’s rating, existing community freeboard requirements), and economic parameters (e.g., interest and discount rates, mortgage period).
Mass Media Effectiveness
The wide audience of mass media allows it to provide critical information for short-term flood mitigation and response, particularly at the individual level, such as in broadcasting official forecasted flood warnings and in post-disaster relief distribution. Altinay et al. (2021) reminded that mass media can also assist in long-term flood communication, particularly by informing risk perception (e.g., Wahlberg and Sjoberg 2000; Fleming et al., 2006; You and Ju 2017; Martins et al., 2019; Heidari et al., 2021) and disaster preparedness (Tekeli-Yeşil et al., 2011). In general, consumers tend to feel that mass media provide more information about natural hazards that are experienced locally and frequently (Karanikola et al., 2015). The demand for mass media-generated public information, including a widening scope of roles, increases during and after disaster events (Miller and Goidel 2009).
Sources of information broadcasted via mass media vary widely in degree of expertise, influence, and perceived credibility, including topical experts, community leaders, public eyewitnesses, and anecdotal comments from broadcasters or citizens. The extent to which expertise/influence of the source (Takahashi and Tandoc 2016) and the admission of uncertainty in broadcasted statements (Gustafson and Rice 2019) affect public credibility has been examined. However, differences in perceived trust of such sources regarding flood risk perception and actionability remain uninvestigated.
The mass media has been criticized for its coverage of local flood events, particularly in the last 2 decades. Using the example of the North Sea Flood of 1953, Hall (2011) suggested that catastrophic events in the infancy of instantaneous mass media were initially likely to have been presented as opportunities for community resilience and solidarity, but later gave way to opportunities for assigning blame and accountability. Perez-Lugo (2001) concluded that the media fell short in communicating warning due to a 1998 flood in Puerto Rico, but that even proper warnings of impending disaster are likely to be misinterpreted as merely nuisance. Karanikola et al. (2015) cautioned that exaggerations of hazards by media sources in their own self-interests can be problematic. Bright and Bagley (2017) noticed that election cycles can impact the extent of coverage of media events. Valencio and Valencio (2018) somewhat apologetically highlighted the many dilemmas involved in mass media reporting of catastrophic events, with the need to balance different expectations, perspectives, and needs desired by mass media consumers, all amid various logistical and financial limitations, leading to possible reduction of trust in the mass media. Consumers have also perceived that mass media effectiveness is less than ideal (Ajaero et al., 2016).
On the whole, Vyncke et al. (2017) confirmed that mass media remain important, even in present times, particularly through elimination of misinformation and quelling of fears, perhaps as a counterbalance in an environment of escalating importance of social media. Information from mass media sources may contain actionable information, especially for short-term benefit immediately before, during, and in the direct aftermath of the disaster, and especially for laypeople. However, there remains a lack of actionable information for the long-term mitigation and decision-making process for individual homeowners and community officials (e.g., cost-benefit analysis of flood mitigation options). The utility and credibility of mass media-generated products could be improved by individual and local customization via Web-based data-sharing systems that would enhance awareness of long-term flood mitigation and adaptation options (e.g., as the wisdom of purchasing flood insurance, building outside of flood zone, and elevating new or existing homes).
Effectiveness of Citizen Science and Related Grassroots Efforts
While mass media information can be regarded as a “top-down” information flow, grassroots efforts are usually “bottom-up” flows. Grassroots efforts that include public information fairs, Earth Day events, peaceful rallies, educational programs, outreach programs, and other similar efforts are termed “citizen science.” Intended consumers can be both at the individual and community levels, for both short- and long-term benefit.
Citizen science has been particularly useful in data collection about past, present, and future floods, for minimizing flood risk. Regarding understanding of past floods, Usón et al. (2016) used participatory interviews to understand relationships among actors and agencies involved in the flood risk management in Santiago, Chile. Glas et al. (2020) also used citizen science-based survey completion about floods in the past, but with a focus on Haiti. Citizen science has proven particularly useful in “heat of the moment” flood danger, such as storm surge monitoring (Spicer et al., 2021), identifying flooded areas in real time (Sy et al., 2019; Yang et al., 2019), and recognizing spatial variability in urban flash floods (Smith and Rodriguez 2017). Efforts at understanding future risk have taken the form of clarification of flood scenarios (Dhiman et al., 2019), improving spatial coverage of pluvial flood events (Michelson and Chang 2019), community mapping (Petersson et al., 2020), and data collection in remote areas (Pandeya et al., 2020).
Other citizen-science-based research has the potential to crosscut efforts to understand past, present, and future flood hazard mitigation. For example, cultural and linguistically diverse community leaders were found to serve as important gatekeepers in communicating flood details (Shepherd and van Vuuren 2014). Hamilton et al. (2018); Keech et al. (2019) examined the role of public ad campaigns and informal communication, respectively, as a means of deterring the dangerous behavior of driving within floodwaters. Citizen engagement in implementing flood risk governance has proven to be successful and shows potential for future success in Europe (Wehn et al., 2015a; Wehn et al., 2015b; Mees et al., 2016; Mees et al., 2018) and elsewhere (Matczak and Hegger 2020), along with other “bottom-up” flood risk management initiatives (Paul et al., 2018; Seebauer et al., 2019).
The shortcomings of citizen science and related grassroots approaches have also been noted. A major disadvantage is data reliability (e.g., Lukyanenko et al., 2016), particularly in evaluating flood risk (Sy et al., 2019). Alves et al. (2021) found that consuming messages from such grassroots efforts was associated with a low coping capacity even among those who had experienced flood. However, other recent work has shown that such uncertainties can be ameliorated to provide valuable information about the flood risk (Tian et al., 2019). In summarizing the state of citizen science and related grassroots approaches regarding flood risk communication, Cheung and Feldman (2019) reaffirmed the importance while also calling for more collaboration between scientists and the lay public on applied problems using theoretical principles.
On the whole, citizen science appears to have an important and growing role in flood risk management. Its strengths are in enhancing public awareness of the necessity for long-term planning strategies to prevent or at least mitigate the flood hazard, thereby enhancing both individual- and community-level efforts. Toward this end, modern Internet capabilities allow for additional advances in citizen-science-based data collection for natural hazards (De Longueville et al., 2010), including flood risk assessment. Likewise, modern communication tools such as Web apps (Ferri et al., 2020) simultaneously across wide geographical areas (McCallum et al., 2016; Hicks et al., 2019) offers increased promise for citizen science as a means of mitigating the flood hazard. However, citizen science and grassroots efforts generally lack the provision of direct actionable information to individual homeowners or community officials at the micro-level, including cost-benefit analysis of mitigation options (e.g., elevated home, wet proofing, dry proofing, and sandbags).
Social Media Effectiveness
As social media share the advantage of mass media in reaching a wide audience quickly, it is not surprising that in recent years, flood information conveyed by social media has received increasing attention. The 2011 Bangkok flood may have been the first major flood event for which the role of social media was studied extensively, with the conclusion that flood losses were reduced by an average of 37 percent due to social media-acquired information (Allaire, 2016). Social media’s role in mitigating the flood hazard has been praised, especially for its timeliness (Kwon and Kang, 2016), which provides short-term benefits.
In recent years, Twitter® has become a particularly important social media platform for flood communication and for analysis of its effectiveness in mitigating disaster risks, including that due to flood. Niles et al. (2019) reported that use of Twitter varies by the various stages of disaster and by the type of disaster, with use in hurricane events peaking at the preparation stage and in flood and tornado events at the “during” or recovery stage. Wang et al. (2021) found that Twitter use has a positive correlation with hurricane resiliency, suggesting that it can be a tool to enhance resilience. Machine learning techniques have revealed the temporal evolution of the various “stages” of Tweet applications in the context of a single disaster (Arapostathis, 2021), with other research calling for further integration of machine-learning-derived information in this regard (Dwarakanath et al., 2021).
Social media-derived data can also be effective in managing long-term flood risk through enhancing scientific data availability. For example, social media are useful for identifying water height points (Li et al., 2018) and areas likely to be submerged (Smith et al., 2017), serving as a proxy for streamflow (Restrepo-Estrada et al., 2018), and monitoring flood waters (Sattaru et al., 2021), including mapping by use of images circulated on social media itself (Rajeshkannan and Kogilavani 2021). Bayesian statistical modeling has been conducted to consider data of various types (i.e., remotely sensed, high-resolution maps, and social media) in estimating flood inundation probability (Rosser et al., 2017).
Social media can minimize flood risk during the human component of the disaster in both short and long terms through optimization of the quality and quantity of communication (Lovari and Bowen, 2020). At the planning stage, information planning and training possibilities gathered via social media are most effective on enhancing flood preparedness, and flood response and recovery (Abimbola et al., 2020). During the flood, social media can disseminate news about sudden onset of the disaster (Vieweg et al., 2014) to provide more effective warnings. The most effective niche for social media may be at the response stage (Stephenson et al., 2018), though Cheng et al. (2019) found that disaster response has been a largely underutilized tool in China. Social media has been found to be useful for immediate-post-disaster damage assessment (Kryvasheyeu et al., 2016), relief and rescue attempts (Basu et al., 2021), and recruiting and managing volunteers and gathering equipment in the flood aftermath (Sharp and Carter, 2020). At even longer time scales, the recovery process can benefit from greater investment in and more efficient information utilization from social media (Yeo et al., 2020). Anderson (2020) noted that evidence of success during the recovery process can be derived from social media communications, in the form of a shift of pronouns from first person singular to first person plural (i.e., from “I, me, my” to “we, us, our”), as the former set of pronouns provides evidence of self-preservation (Pennebaker, 2013) while the latter signifies collectivity (Pavlidou, 2014).
Among all stages of these uses of social media associated with the flood hazard, the analysis of consumer preferences is an important consideration (Feldman et al., 2016). To that end, development of network structures of information dissemination among civilians has been characterized (Olanrewaju et al., 2016), including use of agent-based modeling to identify structures (Du et al., 2017). Perhaps chief among such structures are cultural groups that go beyond ethnicity to include faith-based groups, non-profits, and others, all of which may create both conduits and barriers for communication among victims (Yeo et al., 2018). Perhaps the next development will be to characterize spatio-temporal response stages based on a large collection of events.
In general, social media is most beneficial in raising awareness of the flood hazard and communicating short- and long-term mitigation and adaptation strategies. It is the easiest and quickest way for local authorities to assimilate actionable information to the masses before, during, and after the flood event. However, reliance on social media for mitigating flood impacts has also taken criticism in some of the same ways as for other natural disasters, particularly for providing slowing rates of information diffusion as the crisis continues (Yoo et al., 2016), for being a source of information overload during a stressful period of information processing (Imran et al., 2020), and for the lack of identifiability of direct actionable information (McCreadie et al., 2019), especially for long-term planning. One specific type of actionable information missing from social media sources is user-defined, point- or polygon-specific, flood mitigation cost-benefit analysis at the individual- and community-level.
Resources and Functionality of Webtools
Publicly-accessible webtools have become important for communicating and minimizing flood risk, especially as a means of addressing the shortcomings of the more traditional sources of flood information (i.e., mass media, citizen science, and social media). As hundreds of webtools provide information related to floods and search engines may point to some more readily than others, the inclusion criteria here emphasize the most popular webtools and those that provide the most valuable flood-related information, while excluding any that are non-English language. A review of the available resources reveals several categories based on the primary objective, with some overlapping functionality: Flood forecasting, flood zoning, flood insurance, historical flood analysis, flood monitoring, flood hazard risk and cost, water management, and general flood information. Although this research is not intended to include all of the existing webtools for flood risk communication, a review of the major attributes of 15 sampled online flood risk resources is shown in Table 1 by objective. The resources included in Table 1 and among the 50 sampled here were selected based on the authors’ pre-existing knowledge, along with the prominence of the tools as revealed in online searches by the authors.
Flood Forecasting
The Global Flood Awareness System (GloFAS; Hirpa et al., 2018; Chen et al., 2019; Baugh et al., 2020; Harrigan et al., 2020; Passerotti et al., 2020; Senent-Aparicio et al., 2021), produced by the Copernicus Emergency Management Service (CEMS), is an operational system for monitoring and forecasting floods globally. GloFAS consists of a hydrometeorological forecasting system that is connected to a monitoring system for providing forecasts out to the monthly and seasonal scales (Alfieri et al., 2013). The system provides a quick overview of current and forecasted hydrometeorological events, including floods. GloFAS output can be used for streamflow forecasting and flood early warning (Alfieri et al., 2013) at the macro-scale, but based on the information available, a user would be unlikely to be able to use the tool to mitigate long-term flood impacts at the micro-scale. Enhancing Flood Early Warning Services (EFEWS, 2022) increases flood forecast lead time with an operational 15-days flood forecast using GloFAS, thereby supporting flood resilience in the Hindu Kush Himalaya region. Likewise, the Flood Forecasting and Warning Centre (FFWC, 2022) provides flood forecast and flood warning information using GloFAS for Bangladesh.
The Global Flood Monitoring System (GFMS; Wu et al., 2018), developed at the University of Maryland, inputs real-time precipitation data from the Tropical Rainfall Measuring Mission (TRMM; Santos et al., 2019) Multi-satellite Precipitation Analysis (TMPA) and the Integrated Multi-SatellitE Retrievals for Global Precipitation Measurement (IMERG and GPM; Kidd and Huffman 2011; Tapiador et al., 2012; Guo et al., 2016; Mahmoud et al., 2018) to produce hydrological runoff and routing model output, at a gridded spatial scale of 0.125° from 50°N to 50°S. TRMM includes an array of international satellites that generate global observations of rain and snow by combining data from all passive-microwave instruments in the GPM suite (Huffman et al., 2015). Flood detection/intensity forecasts are based on 13 years of retrospective model runs with TMPA input, with flood thresholds derived for each grid point using surface water storage statistics (95th percentile plus parameters related to basin hydrologic characteristics). Streamflow, surface water storage, and inundation variables are also calculated at 1-km resolution (Wu et al., 2014). The latest real-time GFMS couples the satellite-derived precipitation totals, runoff magnitude estimation and routing, and flood identification. Wu et al. (2019) found that GFMS offers complementary information with the Soil Moisture Active Passive (SMAP) satellite data, available since 2015, to provide enhanced flood forecast information. GFMS data have been used for localized flood extent mapping, including in combination with U.S. Army Corps of Engineers (USACE) Hydrologic Engineering Center’s River Analysis System (HEC-RAS; Kumar et al., 2020). Kar et al. (2020) integrated output from GloFAS and GFMS to classify flood severity at the drainage basin scale. The flood forecasting, based on precipitation forecasts and streamflow modeling, assists in short-term disaster preparedness by facilitating flood monitoring, including floods that can result from other hazards, such as tropical cyclones, and cause still other hazards, such as landslides. However, long-term actionable information is missing at the micro-scale for use by laypersons.
The iowa Flood Information System (IFIS; Demir and Krajewski 2013; Demir et al., 2018) is a Google Maps®–based Web platform developed by the iowa Flood Center (IFC) and represents one of the most comprehensive state-level resources of its kind. IFIS integrates and displays real-time meteorological, hydrological, and soil moisture conditions and forecasts by stream and watershed. This comprehensive data-driven system offers a flood hazard calculator tool with user-defined annual probabilities, to calculate the likelihood of a point flooding. One advantage is that IFIS helps to dispel the common misunderstanding that the flood return period determines the number of floods that can occur in a given return period. A shortcoming of IFIS is that even though it serves over 1,000 iowa communities, it has limited utility at scales more localized than the community level.
National Oceanic and Atmospheric Administration (NOAA) has had a leading role in interactive hydrologic forecasting since the early 1990s (Adams, 2016). NOAA developed the Advanced Hydrologic Prediction Service (AHPS; McEnery et al., 2005; National Research Council, 2006), an accurate, information-rich, Web-based forecast product. AHPS is particularly useful for generating extended probabilistic stream forecasts using ensemble precipitation and temperature forcing (Mullusky et al., 2003; Schaake, 2003; Schaake et al., 2004) to inform risk-based decisions (Connelly et al., 1999). The most recent version of AHPS displays the magnitude and uncertainty of flood/drought events at time scales from hours to months, via user-friendly graphical products including hydrographs. Output includes flood forecast levels and time to crest, along with the likelihood of minor, moderate, or major flooding of a selected stream, the probability of stage exceeding a certain level, along with forecasted discharge during 90-day periods and an inundation map showing nearby infrastructure such as roads, railways, and landmarks relative to past floods. Gronewold et al. (2011) used AHPS to characterize seasonal and inter-annual Great Lakes water levels but cautioned that AHPS dampens the variability relative to observed values. NOAA also produces the Automated Flood Warning System (AFWS; Keeney et al., 2012), which uses gauge data from various sources including the U.S. National Weather Service (NWS), and other federal, state, and local agencies. AFWS data are incorporated not only into emergency planning, but also such routine and fundamental services as enhancing navigation and trade, evaluating water supply, treating wastewater, generating power, and enhancing structural integrity, often in situations for which no other data are available (NWS, 2022).
Other flood forecasting tools are specifically designed for use at the regional scale outside the U.S.A. and particularly across political boundaries. For example, NOAA’s (2022) Sea Level Rise Viewer shows the sea level rise and potential coastal flooding and inundation depth. Another useful webtool for flood forecasting is European Flood Awareness System (EFAS), provided by CEMS (2022). EFAS emphasizes the large watersheds that cross national boundaries in Europe. In general, planning and decision-making regarding transboundary flooding issues are facilitated by Global Environment Facility (2022), which sponsors an online tool for integrating present and future hydrometeorological scenarios including both flood and drought. The scenarios are useful even at the local (water utility) level. A notable example of a meso-(i.e., watershed-) level flood forecasting tool for the Global South is the Outil de Prédiction des Inondations dans la Delta Intérieur du Niger (OPIDIN. 2022), which forecasts flood inundation in the Inner Niger Delta.
These flood forecasting Web resources are designed for assessing flood risk and for promulgating real-time information regarding upcoming floods to professionals such as scientists, researchers, government officials, emergency managers, and media. While the webtools described above offer an important indirect service to the lay public via the mass media, they stop short of providing actionable information to enhance long-term individual or community resilience. For example, none of the tools described above make recommendations on the height to which particular buildings should be elevated.
Flood Zoning
Most U.S. counties provide online Flood Insurance Rate Maps (FIRMs) for the 100-years flood. For example, the Georgia Flood Map Program (2022) shows FIRMs for any property. A main advantage of such websites is a generally quick and easy determination of the flood zone, which fixes flood insurance rates and availability. A major disadvantage of such sites is that they generally lack interactivity and detailed information, especially on a near-real-time basis. Local or city-level products are also available for similar purposes to assist in flood zoning/insurance needs in the form of “Digital FIRMs” (DFIRMs). One example is that for the City of Galveston (2022), which helps homeowners and prospective homeowners to identify their flood zone.
Two Federal Emergency Management Agency (FEMA) products are also useful for flood zoning and other similar applications. The National Flood Hazard Layer (NFHL) viewer (FEMA, 2022a) depicts the current effective FIRM, flood zone, base flood elevation, levee location, and other information for the U.S., where maps have been modernized. NFHL compiles spatial data from the most recent FIRM database with Letters of Map Revision (LOMRs). The Flood Map Service Center (MSC; FEMA, 2022b) is the official public source for flood hazard information, including flood zones, produced in support of the National Flood Insurance Program (NFIP). MSC provides not only flood maps but also an array of other products useful for mitigating the flood hazard and communicating flood risk. The second product, FEMA (2022c) FloodMaps, provides new and preliminary FIRMs. The current flood map, FEMA standard flood hazard determination form, and FEMA’s letter of map amendment are all available.
Another tool that falls into the category of flood zoning is the Virginia Flood Risk Information System (VFRIS; Virginia Department of Conservation and Recreation, 2022). VFRIS incorporates data from the NFIP, DFIRMs, NFHL, and other sources. Flood zone delineations, base flood elevation, sea level rise inundation areas, dam break inundation zones, 100-years return period flood depth, and parcel and building footprints are all depicted graphically. A similar product is available for Louisiana, called Louisiana’s FloodMaps Portal (Skinner, 2022) that includes flood zone, ground elevation, effective FIRM, and historic FIRM data for that state at the individual building level.
The primary advantage of such flood zoning Web resources is that the flood zone information is typically shown at the micro-scale or even at the individual building level. Such flood zone information alerts users to the need for purchasing flood insurance and other regulatory requirements. However, in general, like the tools or websites described above, these tend to provide little actionable information (e.g., the flood zone category for each building) for individual homeowners or community for long-term, flood-resilient construction.
Flood Insurance
Several useful tools are available for consumers to evaluate the economics of flood insurance for their properties. For example, FEMA’s (2022d) “FloodSmart” provides details of flood insurance including its importance, types of flood insurance, coverage and premiums, insurance providers, and the process of purchasing insurance and filing claims. National Flood Services LLC’s (2022) “My Flood Quote” also provides information regarding flood insurance (i.e., importance, cost, and process of purchase), claims, and assistance in the different stages of flood disaster. In general, these and similar sites are directed toward a lay public audience, but they also provide useful information for local-level community planning. For example, individual buildings where flood insurance is mandatory in the U.S. due to location within the 100-years flood zone are often identified. A shortcoming of flood insurance sites is that they typically lack quantitative information availability, such as the short- and long-term insurance premium savings for constructing above the minimum required elevation (i.e., freeboard).
Historical Flood Analysis
Archived information for historical flood events is available through the U.S. Flood Inundation Map Repository (USFIMR) project (Johnson et al., 2019) housed at the University of Alabama. This product includes 10-30 m-resolution satellite-based imagery of past U.S. flood events (Cohen et al., 2018), with shapefiles available for download. Such information can be used as a basis of comparison for flood modeling and prediction. USFIMR does not attempt to integrate real-time meteorological or hydrological information. The extension of this project, known as the Global Flood Inundation Map Repository (GloFIMR), is also administered by the University of Alabama.
Similarly, U.S. Geological Survey (USGS) provides the WaterWatch (Beigi and Tsai, 2014; Oubeidillah et al., 2014) service, which includes a Web-based map, graphs, and tables describing historical as well as near-real-time streamflow conditions in the U.S. at over 3,000 long-term USGS stream gauges. WaterWatch can also bundle streamflow output by hydrologic region, for daily, weekly, bi-weekly, or monthly mean periods for more precise analysis of hydrometeorological conditions back to 1901 (Jian et al., 2008).
The United Nations Satellite Centre (UNOSAT) flood portal [United Nations Institute for Training and Research (UNITAR. 2022)] provides vectorized GIS files for satellite-derived historical flood data since 2007. Functionality includes the ability to import, incorporate, and analyze additional data to enhance decision-making for risk reduction.
All of the above historical flood webtools offer advantages for establishing precedents for flooding at the location of interest. Potential homeowners may use this information to build safe and stronger homes. However, the resources provide little other actionable information for the individual homeowner, such as the current and future probability of flooding, and damage and loss estimates from floods of various magnitudes.
Flood Monitoring
In addition to GloFAS and other tools described previously in the context of other objectives, Dartmouth Flood Observatory (DFO; Lin et al., 2017) provides not only historical, but also near-real-time, maps of flooding globally, based on satellite imagery and data such as total precipitable water (i.e., precipitation that would fall if the entire column of water vapor above it condensed and precipitated), observed rainfall, and river and reservoir levels (Kettner et al., 2021). Kundzewicz et al. (2013) used this product to develop a flood climatology for Europe, and Chen et al. (2020) did similar work for mainland Southeast Asia.
Another useful product is from NASA Goddard Space Flight Center’s (GSFC) Hydrology Laboratory, which operationalizes near-real-time global flood mapping using available satellite data resources. Currently, the system utilizes the twice-daily overpass of the Moderate-resolution Imaging Spectrometer (MODIS) instrument aboard the Terra and Aqua satellites. This system produces global daily surface and flood water maps at approximately 250-m resolution, in 10 × 10° tiles (Nigro et al., 2014). Brakenridge and Anderson (2006) described early uses of MODIS in flood monitoring. Regional flood potential has been derived using terrestrial modeled water storage from the Gravity Recovery and Climate Experiment (GRACE) along with precipitation observations from the Global Precipitation Climatology Project (GPCP; Reager and Famiglietti, 2009.
The Automated Disaster Analysis and Mapping system (ADAM; World Food Programme (WFP) 2022) provides current flood alerts, events, and monitoring information globally. ADAM also tracks longer-term flood events that could have catastrophic consequences for human health, food security, and/or transportation.
Many websites, such as that of the Australian Government Bureau of Meteorology (2022a), monitor floods at the national level. Likewise, the Government of Canada (2022) monitors the water level and discharge data at the Canadian province and territory level. Pakistan (Flood Forecasting Division, 2022), France (Vigicrues, 2022), and Germany (Bundesanstalt für Gewässerkunde, 2022) are other examples of nations with similar offerings. These flood monitoring websites and webtools provide actionable information for the individual and community officials to prepare for imminent disaster. However, they generally lack information for long-term planning to promote resilient site selection and construction.
Flood Hazard Risk and Cost
Samu and Kentel (2018) recently produced a comprehensive economic analysis of flood in Zimbabwe, but similar micro-scale analyses are difficult and lacking. In a useful overview of the advantages and limitations of global flood risk models (GFIRMs), Ward et al. (2015) cautioned that GFIRMs are often mismatched to their intended uses, especially at the local level. The Aqueduct Global Flood Analyzer (AGFA; Iceland, 2015; Sutanudjaja et al., 2017; Ward, 2017) represents a zoomable, meso-resolution offering for determining riverine or coastal flood risk and cost-benefit analysis for either an “optimistic” or “pessimistic” scenario, for 2030, 2050, or 2080, according to the user-defined projection model. The tool provides a customizable, expected, annual urban damage and potential avoided loss by flood protection mechanisms (e.g., levee, dam; Ward et al., 2015). Samu and Akıntuğ (2020) used AGFA successfully to assess economic risk of flood and drought in Zimbabwe. Nevertheless, as with many other systems described above, AGFA does not offer analysis at scales more localized than the watershed level.
USGS (2022) provides the Flood Inundation Mapper (FIM), which shows flood locations and potential loss estimates interactively, based on user-selected stream conditions. Historical flood information is also available. The “what if” flood scenarios available through FIM provide useful risk assessments and flood planning tools at the community level.
The main limitation of existing flood hazard risk and cost webtools or websites is that the analysis is typically at city-to-global level instead of at the micro–level, which limits the utility for decision making by individuals before constructing or purchasing a home. Flood Factor®, produced by First Street Foundation (2020a), addresses such shortcomings by offering a micro-scale (i.e., individual building-level) analytic approach to understanding the flood risk for homes and other structures. First Street Foundation (2020b) also produces a national-level flood risk assessment from these data, which is now being used in as a tool for other research, such as that by Armal et al. (2020), who used Flood Factor to quantify past and future economic impacts due to flood.
Water Management
One useful tool for water management is USACE’s Corps Water Management System (CWMS; Hu et al., 2006), which is an information system that integrates data management and short-term modeling tools. CWMS collects, validates, and stores information such as precipitation, river stage, and floodgate settings, on a real-time basis from platforms via Geostationary Operational Environmental Satellite, NWS Advanced Weather Interactive Processing System, and other sources. The values are then disseminated to users primarily through Web technology and are used to calibrate hydrologic and hydraulic models to represent current conditions. Stage and runoff forecasting is done using several engineering models. The control and visualization interface (CAVI) provides user functionality, such as in executing model runs, visualizing data, and selecting outputs (Charley and Luna, 2007). Agriculturalists can benefit greatly from such water management websites, not only because of information needed to inform planning regarding irrigation water availability, but also for harvest scheduling, reservoir water supply budgeting, and flooding following opening of dam reservoir gates. The constraint of such resources is that the utility is for a limited audience of agriculturalists. However, flood forecasting from water management websites may have untapped potential for assisting communities in flood preparation.
General Flood Information
Many websites provide general flood information for education and public awareness of flood impacts. For example, the Australian Government Bureau of Meteorology (2022b) includes flood warning services and text regarding flood preparation and understanding floods. Such information was useful to Australians before, during, and after Flood Victoria (Molino, 2009). In addition to its use for flood insurance described previously, FEMA’s (2022d) “FloodSmart” includes more general information regarding cost of flooding and flood maps. Ready (2022), an official website of the U.S. government, provides useful information for the preparation, action, and recovery stages of flood. Colorado Division of Homeland Security and Emergency Management Department of Safety (2022) includes basic flood information along with similar guidelines on what should be done before, during, and after a flood. Another example is City of New Orleans (2022), which offers information on flood insurance, flood risk reduction, and flood resilience for new construction. These and similar websites serve the public in an education/outreach capacity regarding the short-term actions that should be taken to reduce the flood impact, but they offer limited actionable information for long-term planning, such as location specific comparative cost-benefit analyses under different mitigation options.
Overall Assessment of Webtools
Comprehensive assessment of the relative usefulness of the webtools is difficult. While data regarding use statistics and the types of data that users felt were most and least helpful would provide valuable information for webtool comparison, such data would likely be biased toward the webtools that serve areas that have the highest population density and experienced the most flooding. Few would use even the most comprehensive webtool if no ominous flood event exists in the region that it covers, and many would use even the least comprehensive webtool if there is an ominous flood event in the region that it covers. Similarly, the variety of scales covered across the webtools is also likely to bias any assessment of usefulness based on “hit” statistics. Moreover, while assessing what the users gain from the webtools would be useful, such an analysis would be most appropriate for an interview or questionnaire format on the webtool itself, but such data are unavailable to the authors.
Nevertheless, it is clear that the existing tools provide some actionable information for enhancing resilience, but with much room for improvement (Table 2). A major shortcoming is that, with the notable exception of Flood Factor, they tend to be useful only at the meso-scale or broader, and most tend to be geared toward short-term flood danger mitigation. While progress made in webtool development in recent years to mitigate the flood hazard, especially with applications such as Flood Factor, represents impressive advancement of science communication, there remains an absence of tools that optimize freeboard benefit-to-cost ratio at the individual building scale, for both new and existing residence owners, renters, developers, engineers, architects, and planners, in a bottom-up approach. Such a flood risk communication webtool is urgent so that individuals and community leaders can make decisions based on quantitative, long-term actionable information. However, as Salvati et al. (2016) cautioned, such a development should not come at the expense of advances in other forms of flood communication designed for the layperson.
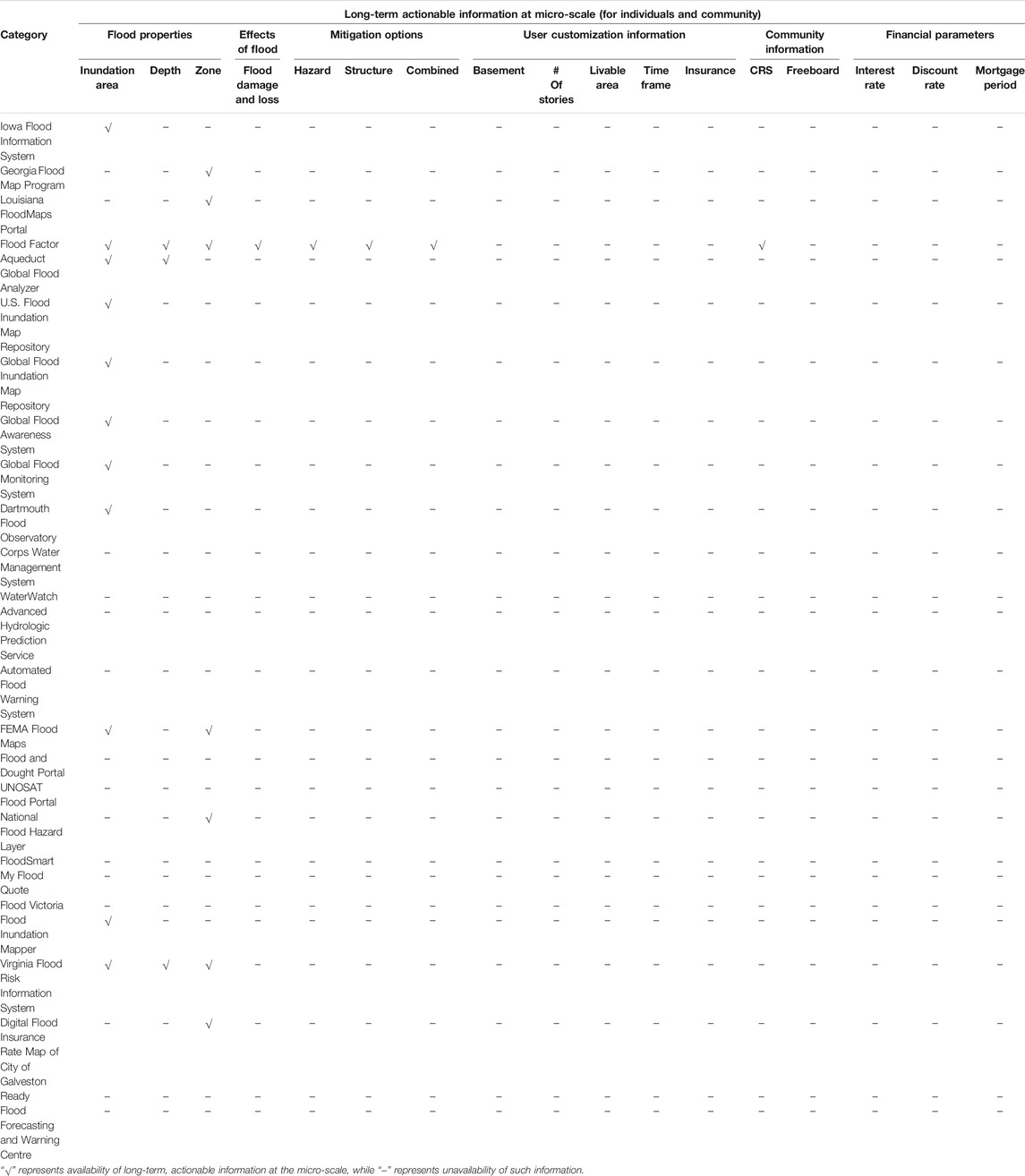
TABLE 2. Types of long-term actionable information at the micro-scale in selected existing web resources.
Recently, researchers at Louisiana State University and University of New Orleans began developing the Flood Safe Home (2022) webtool to assist individual homeowners in the decision-making process based on life-cycle cost-benefit analysis (Dong and Frangopol, 2017). Available information will include the optimal elevation above ground level for the residence, for deriving the maximum benefit from not only avoiding flood loss but also from savings on insurance premiums. Such tools seem to be the next step in the provision of actionable information regarding flood hazard mitigation.
Summary and Conclusion
Results of this analysis suggest that while mass media, citizen science, and social media have revolutionized the way that people plan for, survive, and recover from floods, their utility is largely restricted to addressing short-term needs for information and/or conveying information about singular events to scientists. More robust applications of these well-established forms of media might include broadcast of more specific, actionable information based on sound science from trusted voices to enhance long-term planning. However, availability of such actionable information to both community leaders and the lay public to satisfy needs for long-term planning for and mitigation of the flood hazard remains limited. A particular area of need is media that satisfy several criteria simultaneously: a delivery method that reaches the most people and that people find most appealing, while enhancing decision-making for long-term needs rather than for short-term gain. The most likely solution is a webtool that builds on Flood Factor by providing additional functionality to support improved flood risk reduction decision making, especially one that considers both the costs and benefits of mitigation. Such a tool would enhance resilience to the world’s most widespread and impactful hazard.
Author Contributions
RM developed the detailed methodology, collected and analyzed the data, and developed the initial text. RR helped in the literature review, and edited early and late drafts of the text. CF helped to organize the paper and tables, and revised the text. Y-CL provided oversight on analysis and edited the text.
Funding
This was funded by the Louisiana Sea Grant College Program (Omnibus cycle 2020–2022; Award Number: NA18OAR4170098; Project Number: R/CH-03), the Gulf Research Program of the National Academies of Sciences, Engineering, and Medicine under the Grant Agreement number: 200010880 “The New First Line of Defense: Building Community Resilience through Residential Risk Disclosure,” and the U.S. Department of Housing and Urban Development (HUD; 2019–2022; Award No. H21679CA, Subaward No. S01227-1). Any opinions, findings, conclusions, and recommendations expressed in this manuscript are those of the author and do not necessarily reflect the official policy or position of the funders. Publication of this article was subsidized by the Performance Contractors Professorship in the College of Engineering at LSU.
Conflict of Interest
The authors declare that the research was conducted in the absence of any commercial or financial relationships that could be construed as a potential conflict of interest.
Publisher’s Note
All claims expressed in this article are solely those of the authors and do not necessarily represent those of their affiliated organizations, or those of the publisher, the editors and the reviewers. Any product that may be evaluated in this article, orclaim that may be made by its manufacturer, is not guaranteed or endorsed by the publisher.
References
Abimbola, A. F., Bakar, H. S. A., Mat, M. Z., and Adebambo, O. H. (2020). Evaluating the Influence of Resident Agencies' Participation in Flood Management via Social Media, in Nigeria. Jssh 28 (4), 2765–2785. doi:10.47836/pjssh.28.4.15
Adams, T. E. (2016). “Flood Forecasting in the United States NOAA/National Weather Service,” in Flood Forecasting: A Global Perspective. Editors T. E. AdamsIII, and T. C. Pagano (Academic Press), 249–310. doi:10.1016/b978-0-12-801884-2.00010-4
Ajaero, I. D., Okoro, N. M., and Ajaero, C. K. (2016). Perception of and Attitude toward Mass media Reportage of the 2012 Flood in Rural Nigeria. SAGE Open 6 (3), 215824401666688. doi:10.1177/2158244016666887
Alfieri, L., Burek, P., Dutra, E., Krzeminski, B., Muraro, D., Thielen, J., et al. (2013). GloFAS - Global Ensemble Streamflow Forecasting and Flood Early Warning. Hydrol. Earth Syst. Sci. 17 (3), 1161–1175. doi:10.5194/hess-17-1161-2013
Allaire, M. C. (2016). Disaster Loss and Social media: Can Online Information Increase Flood Resilience? Water Resour. Res. 52 (9), 7408–7423. doi:10.1002/2016WR019243
Altinay, Z., Rittmeyer, E., Morris, L. L., and Reams, M. A. (2021). Public Risk Salience of Sea Level Rise in Louisiana, United States. J. Environ. Stud. Sci. 11, 523–536. in press. doi:10.1007/s13412-020-00642-5
Alves, P. B. R., Cordão, M. J. d. S., Djordjević, S., and Javadi, A. A. (2021). Place-based Citizen Science for Assessing Risk Perception and Coping Capacity of Households Affected by Multiple Hazards. Sustainability 13 (1), 302. Art. No. 302. doi:10.3390/su13010302
Anderson, A. A. (2020). Expressions of Resilience: Social Media Responses to a Flooding Event. Risk Anal. 41, 1600–1613. in press. doi:10.1111/risa.13639
Arapostathis, S. G. (2021). A Methodology for Automatic Acquisition of Flood‐event Management Information from Social Media: the Flood in Messinia, South Greece, 2016. Inf. Syst. Front. 23, 1127–1144. doi:10.1007/s10796-021-10105-z
Armal, S., Porter, J., Lingle, B., Chu, Z., Marston, M., and Wing, O. (2020). Assessing Property Level Economic Impacts of Climate in the US, New Insights and Evidence from a Comprehensive Flood Risk Assessment Tool. Climate 8 (10), 116. Art. No. 116. doi:10.3390/cli8100116
Australian Government Bureau of Meteorology (2022b). Australia Rainfall and River Conditions. Available at: http://www.bom.gov.au/australia/flood/?ref=ftr (Last accessed 1 25, 2022).
Australian Government Bureau of Meteorology (2022a). Flood Knowledge centre. Available at: http://www.bom.gov.au/australia/flood/knowledge-centre/ (Last accessed 1 25, 2022).
Basu, M., Jana, A., and Ghosh, S. (2021). “Utilizing Online Social media for Coordinating post-disaster Relief in Indian Cities,” in Advances in Urban Planning in Developing Nations. Editor A. Jana (London: Routledge India), 199–210. doi:10.4324/9781003091370-10
Baugh, C., de Rosnay, P., Lawrence, H., Jurlina, T., Drusch, M., Zsoter, E., et al. (2020). The Impact of SMOS Soil Moisture Data Assimilation within the Operational Global Flood Awareness System (GloFAS). Remote Sensing 12 (9), 1490. doi:10.3390/rs12091490
Beigi, E., and Tsai, F. T. C. (2014). GIS-based Water Budget Framework for High-Resolution Groundwater Recharge Estimation of Large-Scale Humid Regions. J. Hydrologic Eng. 19 (8), 05014004. doi:10.1061/(ASCE)HE.1943-5584.0000993
Brakenridge, R., and Anderson, E. (2006). “MODIS-based Flood Detection, Mapping, and Measurement: The Potential for Operational Hydrological Applications,” in Transboundary Floods: Reducing Risks through Flood Management. Nato Science Series: IV: Earth and Environmental Sciences. Editors J. Marsalek, G. Stancalie, and G. Balint (Dordrecht: Springer), 72. doi:10.1007/1-4020-4902-1_1
Bright, C. F., and Bagley, B. (2017). Elections, News Cycles, and Attention to Disasters. Dpm 26 (4), 471–478. doi:10.1108/DPM-02-2017-0018
Bundesanstalt für Gewässerkunde (2022). Current Water Levels of the Federal Waterways. Available atV https://www.bafg.de/DE/06_Info_Service/01_Wasserstaende/wasserstaende_node.html (Last accessed 1 25, 2022).
Bushra, N., Mostafiz, R. B., Rohli, R. V., Friedland, C. J., and Rahim, M. A. (2021). Technical and Social Approaches to Study Shoreline Change of Kuakata, Bangladesh. Front. Mar. Sci. 8, 730984. doi:10.3389/fmars.2021.730984
Charley, W. J., and Luna, M. (2007). Corps Water Management System (CWMS) Decision Support Modeling and Integration Use in the June 2007 Texas Floods. American Geophysical Union Fall Meeting Abstracts, H11C‒0681. Available at: https://ui.adsabs.harvard.edu/abs/2007AGUFM.H11C0681C/abstract (Last accessed 1 25, 2022).
Chen, A., Giese, M., and Chen, D. (2020). Flood Impact on Mainland Southeast Asia between 1985 and 2018-The Role of Tropical Cyclones. J. Flood Risk Manag., 13(2), Art. No. e12598. doi:10.1111/jfr3.12598
Chen, K., Guo, S., Wang, J., Qin, P., He, S., Sun, S., et al. (2019). Evaluation of GloFAS-Seasonal Forecasts for cascade Reservoir Impoundment Operation in the Upper Yangtze River. Water 11 (12), 2539. Art. No. 2539. doi:10.3390/w11122539
Cheng, X., Han, G., Zhao, Y., and Li, L. (2019). Evaluating Social media Response to Urban Flood Disaster: Case Study on an East Asian City (Wuhan, China). Sustainability 11 (19), 5330. Art. No. 5330. doi:10.3390/su11195330
Cheung, W., and Feldman, D. (20191961). Can Citizen Science Promote Flood Risk Communication? Water 11 (10), 1961. doi:10.3390/w11101961
City of Galveston (2022). Digital Flood Insurance Rate Map (Effective Date: 08/15/2019). Available at: https://galveston.maps.arcgis.com/apps/webappviewer/index.html?id=ef5190792f794b44a8bcb84b02ac4c7c (Last accessed 1 25, 2022).
City of New Orleans, (2022). NOLA Ready. Available at: https://ready.nola.gov/rain/ (Last accessed 1 25, 2022).
Cohen, S., Brakenridge, G. R., Kettner, A., Bates, B., Nelson, J., McDonald, R., et al. (2018). Estimating Floodwater Depths from Flood Inundation Maps and Topography. J. Am. Water Resour. Assoc. 54 (4), 847–858. doi:10.1111/1752-1688.12609
Colorado Division of Homeland Security (2022). Emergency Management Department of Safety. Available at: https://dhsem.colorado.gov/info-center/readycolorado/colorado-hazard-information/flood (Last accessed 1 25, 2022).
Connelly, B. A., Braatz, D. T., Halquist, J. B., DeWeese, M. M., Larson, L., and Ingram, J. J. (1999). Advanced Hydrologic Prediction System. J. Geophys. Res. 104 (D16), 19655–19660. doi:10.1029/1999JD900051
Copernicus Emergency Management Service (2022). Copernicus Emergency Management Service (CEMS). Available at: https://www.efas.eu/en/copernicus-emergency-management-service-cems (Last accessed 1 25, 2022).
De Longueville, B., Luraschi, G., Smits, P., Peedell, S., and Groeve, T. (2010). Citizens as Sensors for Natural Hazards: A VGI Integration Workflow. Geomatica 64 (1), 41–59. doi:10.5623/geomat-2010-0005
Demir, I., and Krajewski, W. F. (20132013). Towards an Integrated Flood Information System: Centralized Data Access, Analysis, and Visualization. Environ. Model. Softw. 50, 77–84. doi:10.1016/j.envsoft.2013.08.009
Demir, I., Yildirim, E., Sermet, Y., and Sit, M. A. (2018). FLOODSS: Iowa Flood Information System as a Generalized Flood Cyberinfrastructure. Int. J. River Basin Manag. 16 (3), 393–400. doi:10.1080/15715124.2017.1411927
Dewan, A. M., Kankam-Yeboah, K., and Nishigaki, M. (2006). Using Synthetic Aperture Radar (SAR) Data for Mapping River Water Flooding in an Urban Landscape: a Case Study of Greater Dhaka, Bangladesh. J. Jpn. Soc. Hydrol. Water Resour. 19 (1), 44–54. doi:10.3178/jjshwr.19.44
Dhiman, R., VishnuRadhan, R., Eldho, T. I., and Inamdar, A. (2019). Flood Risk and Adaptation in Indian Coastal Cities: Recent Scenarios. Appl. Water Sci. 9 (1), 1–16. doi:10.1007/s13201-018-0881-9
Dong, Y., and Frangopol, D. M. (20172017). Probabilistic Life-Cycle Cost-Benefit Analysis of Portfolios of Buildings under Flood hazard. Eng. Structures 142, 290–299. doi:10.1016/j.engstruct.2017.03.063
Du, E., Cai, X., Sun, Z., and Minsker, B. (2017). Exploring the Role of Social Media and Individual Behaviors in Flood Evacuation Processes: An Agent-Based Modeling Approach. Water Resour. Res. 53 (11), 9164–9180. doi:10.1002/2017WR021192
Dwarakanath, L., Kamsin, A., Rasheed, R. A., Anandhan, A., and Shuib, L. (2021). Automated Machine Learning Approaches for Emergency Response and Coordination via Social media in the Aftermath of a Disaster: A Review. IEEE Access 9, 68917–68931. doi:10.1109/ACCESS.2021.3074819
Enhancing Flood Early Warning Services (2022). Enhancing Flood Early Warning Services (EWS). Available at: https://www.servirglobal.net/ServiceCatalogue/details/5bc8824b51ebdcae79683356 (Last accessed 1 25, 2022).
Federal Emergency Management Agency (2022c). FEMA Flood Maps. Available at: https://www.newfloodmap.com/ (Last accessed 1 25, 2022).
Federal Emergency Management Agency (2022b). Flood Map Service Center. Available at: https://msc.fema.gov/portal/home (Last accessed 1 25, 2022).
Federal Emergency Management Agency (2022a). National Flood Hazard Layer (NFHL) Viewer. Available at: https://hazards-fema.maps.arcgis.com/apps/webappviewer/index.html?id=8b0adb51996444d4879338b5529aa9cd (Last accessed 1 25, 2022).
Federal Emergency Management Agency (2022d). National Flood Insurance Program. Available at: https://www.floodsmart.gov/ (Last accessed 1 25, 2022).
Feldman, D., Contreras, S., Karlin, B., Basolo, V., Matthew, R., Sanders, B., et al. (2016). Communicating Flood Risk: Looking Back and Forward at Traditional and Social media Outlets. Int. J. Disaster Risk Reduction 15, 43–51. doi:10.1016/j.ijdrr.2015.12.004
Ferri, M., Wehn, U., See, L., Monego, M., and Fritz, S. (2020). The Value of Citizen Science for Flood Risk Reduction: Cost-Benefit Analysis of a Citizen Observatory in the Brenta-Bacchiglione Catchment. Hydrol. Earth Syst. Sci. 24 (12), 5781–5798. doi:10.5194/hess-24-5781-2020
First Street Foundation (2020a). First Street Foundation Technical Documentation. Available at: https://assets.firststreet.org/uploads/2020/06/FSF_Flood_Model_Technical_Documentation.pdf (Last accessed 1 25, 2022).
First Street Foundation (2020b). The First National Flood Risk Assessment: Defining America’s Growing Risk. Available at: https://assets.firststreet.org/uploads/2020/06/first_street_foundation__first_national_flood_risk_assessment.pdf (Last accessed 1 25, 2022).
Fleming, K., Thorson, E., and Zhang, Y. (2006). Going beyond Exposure to Local News media: An Information-Processing Examination of Public Perceptions of Food Safety. J. Health Commun. 11 (8), 789–806. doi:10.1080/10810730600959705
Flood Forecasting and Warning Centre (2022). Flood Forecasting and Warning Centre (FFWC). Available at: http://www.ffwc.gov.bd/ (Last accessed 1 25, 2022).
Flood Forecasting Division (2022). Current & Daily Basis Min & Max Temps and Graphs. Available at: http://www.pmd.gov.pk/FFD/cp/floodpage.htm (Last accessed 1 25, 2022).
Flood Safe Home (2022). What Is the Optimal Freeboard? Available at: https://floodsafehome.lsu.edu/# (Last accessed 1 25, 2022).
Georgia Flood Map Program (2022). Georgia Flood Map Program. Available at: https://map.georgiadfirm.com/ (Last accessed 1 25, 2022).
Glas, H., De Maeyer, P., Merisier, S., and Deruyter, G. (2020). Development of a Low‐cost Methodology for Data Acquisition and Flood Risk Assessment in the Floodplain of the River Moustiques in Haiti. J. Flood Risk Manag. 13 (2), e12608. doi:10.1111/jfr3.12608
Global Environment Facility (2022). Flood and Drought portal. Available at: https://www.flooddroughtmonitor.com/home (Last accessed 1 25, 2022).
Government of Canada, (2022). Real-Time Hydrometric Data Map Search. Available at: https://wateroffice.ec.gc.ca/google_map/google_map_e.html?searchBy=p&province=AB&doSearch=Go (Last accessed 1 25, 222).
Gronewold, A. D., Clites, A. H., Hunter, T. S., and Stow, C. A. (2011). An Appraisal of the Great Lakes Advanced Hydrologic Prediction System. J. Great Lakes Res. 37 (3), 577–583. doi:10.1016/j.jglr.2011.06.010
Guo, H., Chen, S., Bao, A., Behrangi, A., Hong, Y., Ndayisaba, F., et al. (2016). Early Assessment of Integrated Multi-Satellite Retrievals for Global Precipitation Measurement over China. Atmos. Res. 176-177, 121–133. doi:10.1016/j.atmosres.2016.02.020
Gustafson, A., and Rice, R. E. (2019). The Effects of Uncertainty Frames in Three Science Communication Topics. Sci. Commun. 41 (6), 679–706. doi:10.1177/1075547019870811
Hall, A. (2011). The Rise of Blame and Recreancy in the United Kingdom: A Cultural, Political and Scientific Autopsy of the North Sea Flood of 1953. Environ. Hist. Camb 17 (3), 379–408. doi:10.3197/096734011X13077054787145
Hamilton, K., Peden, A. E., Keech, J. J., and Hagger, M. S. (20182018). Changing People's Attitudes and Beliefs toward Driving through Floodwaters: Evaluation of a Video Infographic. Transportation Res. F: Traffic Psychol. Behav. 53, 50–60. doi:10.1016/j.trf.2017.12.012
Harrigan, S., Zsoter, E., Alfieri, L., Prudhomme, C., Salamon, P., Wetterhall, F., et al. (2020). GloFAS-ERA5 Operational Global River Discharge Reanalysis 1979-present. Earth Syst. Sci. Data 12 (3), 2043–2060. doi:10.5194/essd-12-2043-2020
He, X., Lu, D., Margolin, D., Wang, M., Idrissi, S. E., and Lin, Y. R. (2017).The Signals and Noise: Actionable Information in Improvised Social media Channels during a Disaster. In Proceedings of the 2017 Association for Computing Machinery on Web Science Conference. Troy, NY, 33–42.
Heidari, M., Sayfouri, N., and Heidari, S. (2021). Lessons Learned from the Higher Risk Perception and the Efficient Flood Mitigation in Haji-Abad, Golestan Province, Iran, 2019. Disaster Med. Public Health Prep. 15 (3), 339–343. doi:10.1017/dmp.2020.14
Hicks, A., Barclay, J., Chilvers, J., Armijos, M. T., Oven, K., Simmons, P., et al. (2019). Global Mapping of Citizen Science Projects for Disaster Risk Reduction. Front. Earth Sci. 7, 226. doi:10.3389/feart.2019.00226
Hirpa, F. A., Salamon, P., Beck, H. E., Lorini, V., Alfieri, L., Zsoter, E., et al. (2018). Calibration of the Global Flood Awareness System (GloFAS) Using Daily Streamflow Data. J. Hydrol. 566, 595–606. doi:10.1016/j.jhydrol.2018.09.052
Hu, H. H., Kreymborg, L. R., Doeing, B. J., Baron, K. S., and Jutila, S. A. (2006). Gridded Snowmelt and Rainfall-Runoff CWMS Hydrologic Modeling of the Red River of the North Basin. J. Hydrol. Eng. 1111 (2), 912–100. doi:10.1061/(ASCE)1084-069910.1061/(asce)1084-0699(2006)11:2(91)
Huffman, G. J., Bolvin, D. T., and Nelkin, E. J. (2015). Day 1 IMERG Final Run Release Notes. Greenbelt, MD, USA: NASA/GSFC. Available at: https://scholar.google.com/scholar?cluster=9959834544933202456&hl=en&as_sdt=0,22# (Last accessed 1 25, 2022).
Iceland, C. (2015). “The Aqueduct Global Flood Analyzer,” in EGU General Assembly Conference Abstracts, 1815. Available at: https://ui.adsabs.harvard.edu/abs/2015EGUGA..17.1815I/abstract (Last accessed 1 25, 2022).
Imran, M., Ofli, F., Caragea, D., and Torralba, A. (2020). Using AI and Social media Multimodal Content for Disaster Response and Management: Opportunities, Challenges, and Future Directions. Inf. Process. Manag. 57 (5), 102261. Art. No. 102261. doi:10.1016/j.ipm.2020.102261
Jian, X., Wolock, D., and Lins, H. (2008). WaterWatch – Maps, Graphs, and Tables of Current, Recent, and Past Streamflow Conditions. Available at: http://pubs.usgs.gov/fs/2008/3031/index.html (Last accessed 1 25, 2022).
Johnson, J. M., Munasinghe, D., Eyelade, D., and Cohen, S. (2019). An Integrated Evaluation of the National Water Model (NWM)-Height above Nearest Drainage (HAND) Flood Mapping Methodology. Nat. Hazards Earth Syst. Sci. 19 (11), 2405–2420. doi:10.5194/nhess-19-2405-2019
Kar, B., Bausch, D., Wang, J., SharmaChen, P. Z., Chen, Z., Schumann, G., et al. (2020). Integrated Model of Models for Global Flood Alerting. WIT Trans. Built Environ. 194, 73–83. doi:10.2495/FRIAR200071
Karanikola, P., Panagopoulos, T., Tampakis, S., Karantoni, M. I., and Tsantopoulos, G. (2015). Facing and Managing Natural Disasters in the Sporades Islands, Greece. Nat. Hazards Earth Syst. Sci. 14 (2), 995–1005. doi:10.5194/nhess-14-995-2014
Keech, J. J., Smith, S. R., Peden, A. E., Hagger, M. S., and Hamilton, K. (2019). The Lived Experience of Rescuing People Who Have Driven into Floodwater: Understanding Challenges and Identifying Areas for Providing Support. Health Promot. J. Austral 30 (2), 252–257. doi:10.1002/hpja.181
Keeney, H. J., Buan, S., and Diamond, L. (2012). “Multi-hazard Early Warning System of the United States National Weather Service,” in Institutional Partnerships in Multi-Hazard Early Warning Systems. Editor M. Golnaraghi (Berlin, Heidelberg: Springer), 115115–157157. doi:10.1007/978-3-642-25373-7_6
Kettner, A. J., Brakenridge, G. R., Schumann, G. J.-P., and Shen, X. (2021). DFO-flood Observatory. Earth Observation Flood Appl. Prog. Perspect., 147–164. doi:10.1016/B978-0-12-819412-6.00007-9
Kidd, C., and Huffman, G. (2011). Global Precipitation Measurement. Met. Apps 18 (3), 334–353. doi:10.1002/met.284
Kryvasheyeu, Y., Chen, H., Obradovich, N., Moro, E., Van Hentenryck, P., Fowler, J., et al. (2016). Rapid Assessment of Disaster Damage Using Social media Activity. Sci. Adv., 2(3), Art. No. e1500779. doi:10.1126/sciadv.1500779
Kumar, N., Kumar, M., Sherring, A., Suryavanshi, S., Ahmad, A., and Lal, D. (2020). Applicability of HEC-RAS 2D and GFMS for Flood Extent Mapping: A Case Study of Sangam Area, Prayagraj, India. Model. Earth Syst. Environ. 6 (1), 397–405. doi:10.1007/s40808-019-00687-8
Kundzewicz, Z. W., Pińskwar, I., and Brakenridge, G. R. (2013). Large Floods in Europe, 1985-2009. Hydrological Sci. J. 58 (1), 1–7. doi:10.1080/02626667.2012.745082
Kwon, H. Y., and Kang, Y. O. (2016). Risk Analysis and Visualization for Detecting Signs of Flood Disaster in Twitter. Spat. Inf. Res. 24 (2), 127–139. doi:10.1007/s41324-016-0014-1
Li, Z., Wang, C., Emrich, C. T., and Guo, D. (2018). A Novel Approach to Leveraging Social media for Rapid Flood Mapping: a Case Study of the 2015 South Carolina Floods. Cartography Geogr. Inf. Sci. 45 (2), 97–110. doi:10.1080/15230406.2016.1271356
Lin, L., Di, L., Yu, E. G., Tang, J., Shrestha, R., Rahman, M. S., et al. (2017), Extract Flood Duration from Dartmouth Flood Observatory Flood Product. In 2017 6th International Conference on Agro-Geoinformatics, 1–4. doi:10.1109/Agro-Geoinformatics.2017.8047064
Lovari, A., and Bowen, S. A. (2020). Social media in Disaster Communication: A Case Study of Strategies, Barriers, and Ethical Implications. J. Public Aff. 20 (1), e1967. doi:10.1002/pa.1967
Lukyanenko, R., Parsons, J., and Wiersma, Y. F. (2016). Emerging Problems of Data Quality in Citizen Science. Conservation Biol. 30 (3), 447–449. doi:10.1111/cobi.12706
Mahmoud, M. T., Al-Zahrani, M. A., and Sharif, H. O. (2018). Assessment of Global Precipitation Measurement Satellite Products over Saudi Arabia. J. Hydrol. 559, 1–12. doi:10.1016/j.jhydrol.2018.02.015
Maidl, E., and Buchecker, M. (2015). Raising Risk Preparedness by Flood Risk Communication. Nat. Hazards Earth Syst. Sci. 15 (7), 1577–1595. doi:10.5194/nhess-15-1577-2015
Martins, B., Nunes, A., and Lourenço, L. (2019). Spatial Risk Perception Among 9th Grade Students mainland Portugal versus the Metropolitan Area of Porto. Int. Res. Geographical Environ. Edu. 28 (3), 194–210. doi:10.1080/10382046.2018.1561632
Matczak, P., and Hegger, D. L. T. (2020). Flood Risk Governance for More Resilience-Reviewing the Special Issue's Contribution to Existing Insights. Water 12 (8), 2122. doi:10.3390/w12082122
McCallum, I., Liu, W., See, L., Mechler, R., Keating, A., Hochrainer-Stigler, S., et al. (2016). Technologies to Support Community Flood Disaster Risk Reduction. Int. J. Disaster Risk Sci. 7 (2), 198–204. doi:10.1007/s13753-016-0086-5
McCreadie, R., Buntain, C., and Soboroff, I. (2019).TREC Incident Streams: Finding Actionable Information on Social media. In Proceedings of the 16th ISCRAM Conference – València. Spain, 691–705. Available at: https://eprints.gla.ac.uk/183409/7/183409.pdf (Last accessed 1 25, 2022).
McEnery, J., Ingram, J., Duan, Q., Adams, T., and Anderson, L. (2005). Noaa's Advanced Hydrologic Prediction Service. Bull. Amer. Meteorol. Soc. 86 (3), 375–386. doi:10.1175/BAMS-86-3-375
Mees, H., Alexander, M., Gralepois, M., Matczak, P., and Mees, H. (20182018). Typologies of Citizen Co-production in Flood Risk Governance. Environ. Sci. Pol. 89, 330–339. doi:10.1016/j.envsci.2018.08.011
Mees, H., Crabbé, A., Alexander, M., Kaufmann, M., Bruzzone, S., Lévy, L., et al. (2016). Coproducing Flood Risk Management through Citizen Involvement: Insights from Cross-Country Comparison in Europe. E&S 21 (3), 7. doi:10.5751/ES-08500-210307
Michelson, K., and Chang, H. (2019). Spatial Characteristics and Frequency of Citizen-Observed Pluvial Flooding Events in Relation to Storm Size in Portland, Oregon. Urban Clim. 29, 100487. doi:10.1016/j.uclim.2019.100487
Miller, A., and Goidel, R. (2009). News Organizations and Information Gathering during a Natural Disaster: Lessons from Hurricane Katrina. J. Contingencies Crisis Manag. 17 (4), 266–273. doi:10.1111/j.1468-5973.2009.00586.x
Molino, S. (2009). Unifying Flood Information–The Victorian Flood Web portal. In Floodplain Management Authorities Conference, Albury. Available at: https://www.molinostewart.com.au/wp-content/uploads/2009-FMA-Unifying-Flood-Information.pdf (Last accessed 1 25, 2022).Management Authorities Conference, Albury
Mostafiz, R. B., Bushra, N., Rohli, R. V., Friedland, C. J., and Rahim, M. A. (2021a). Present vs. Future Property Losses from a 100-year Coastal Flood: A Case Study of Grand isle, Louisiana. Front. Water 3, 763358. doi:10.3389/frwa.2021.763358
Mostafiz, R. B., Friedland, C. J., Rahman, M. A., Rohli, R. V., Tate, E., Bushra, N., et al. (2021b). Comparison of Neighborhood-Scale, Residential Property Flood-Loss Assessment Methodologies. Front. Environ. Sci., 9, Art. No. 734294, doi:10.3389/fenvs.2021.734294
Mostafiz, R. B., Bushra, N., Friedland, C., and Rohli, R. V. (2021c). Present vs. Future Losses from a 100-Year Flood: A Case Study of Grand Isle, Louisiana. AGU Fall Meeting 2021. AGU.
Mullusky, M., Demargne, J., Welles, E., Wu, L., and Schaake, J. (2003). Hydrologic Applications of Short and Medium Range Ensemble Forecasts in the NWS Advanced Hydrologic Prediction Services (AHPS). in. AMS 16th Conf. Numer. Weather Prediction. Available at: https://ams.confex.com/ams/84Annual/techprogram/paper_72137.htm (Last accessed 1 25, 2022).
National Flood Services (2022). My Flood Quote. Available at: https://myfloodquote.com/ (Last accessed 1 25, 2022).
National Oceanic and Atmospheric Administration (2022). Sea Level Rise Viewer. Available at: https://coast.noaa.gov/slr/# (Last accessed 1 25, 2022).
National Research Council (2006). Toward a New Advanced Hydrologic Prediction Service (AHPS). Washington D.C.: National Academies Press.
National Weather Service (Nws), (2022). Automated Flood Warning Systems. Available at: https://water.weather.gov/afws/faq.php (Last accessed 1 25, 2022).
Nigro, J., Slayback, D., Policelli, F., and Brakenridge, G. R. (2014). NASA/DFO MODIS Near Real-Time (NRT) Global Flood Mapping Product Evaluation of Flood and Permanent Water Detection. Greenbelt, MD. Available at: https://floodmap.modaps.eosdis.nasa.gov//documents/NASAGlobalNRTEvaluationSummary_v4.pdf (Last accessed 1 25, 2022).
Niles, M. T., Emery, B. F., Reagan, A. J., Dodds, P. S., and Danforth, C. M. (2019). Social media Usage Patterns during Natural Hazards. PLoS One, 14(2), Art. No. e0210484. doi:10.1371/journal.pone.0210484
OPIDIN (2022). OPIDIN – Forecast Tool to Predict the Inundations in the Inner Niger Delta. Available at: https://www.opidin.org/en (Last accessed 1 25, 2022).
Oubeidillah, A. A., Kao, S.-C., Ashfaq, M., Naz, B. S., and Tootle, G. (2014). A Large-Scale, High-Resolution Hydrological Model Parameter Data Set for Climate Change Impact Assessment for the Conterminous US. Hydrol. Earth Syst. Sci. 18 (1), 67–84. doi:10.5194/hess-18-67-2014
Pandeya, B., Uprety, M., Paul, J. D., Sharma, R. R., Dugar, S., and Buytaert, W. (2020). Mitigating Flood Risk Using Low‐cost Sensors and Citizen Science: A Proof‐of‐concept Study from Western Nepal. J. Flood Risk Manag., 14(1), Art. No. e12675. doi:10.1111/jfr3.12675
Passerotti, G., Massazza, G., Pezzoli, A., Bigi, V., Zsótér, E., and Rosso, M. (2020). Hydrological Model Application in the Sirba River: Early Warning System and GloFAS Improvements. Water 12 (3), 620. doi:10.3390/w12030620
Paul, J. D., Buytaert, W., Allen, S., Ballesteros‐Cánovas, J. A., Bhusal, J., Cieslik, K., et al. (2018). Citizen Science for Hydrological Risk Reduction and Resilience Building. WIREs Water 5 (1). Art. No. e1262. doi:10.1002/wat2.1262
Pavlidou, T. (2014). Constructing Collectivity: “We” across Languages and Contexts. Amsterdam: Benjamins.
Pennebaker, J. W. (2013). The Secret Life of Pronouns: What Our Words Say about Us. New York: Bloomsbury USA.
Perez-Lugo, M. (2001). The Mass Media and Disaster Awareness in Puerto Rico. Organ. Environ. 14 (1), 55–73. doi:10.1177/1086026601141003
Petersson, L., ten Veldhuis, M.-C., Verhoeven, G., Kapelan, Z., Maholi, I., and Winsemius, H. C. (2020). Community Mapping Supports Comprehensive Urban Flood Modeling for Flood Risk Management in a Data-Scarce Environment. Front. Earth Sci., 8, Art. No. 304. doi:10.3389/feart.2020.00304
Rajeshkannan, C., and Kogilavani, S. V. (2021). Reconstructing Geographical Flood Probability and Analyzed Inundation Flood Mapping on Social media Implementation. Stud. Inform. Control. 30 (1), 29–38. doi:10.24846/v30i1y202103
Ready (2022). Flood. Available at: https://www.ready.gov/floods#content (Last accessed 1 25, 2022).
Reager, J. T., and Famiglietti, J. S.L23402 (2009). Global Terrestrial Water Storage Capacity and Flood Potential Using GRACE. Geophys. Res. Lett. 36 (23). doi:10.1029/2009GL040826
Remember Samu, R., and Bertuğ Akıntuğ, B. (2020). Pre-disaster Planning and Preparedness: Drought and Flood Forecasting and Analysis in Zimbabwe. Wsa 46 (3), 448‒457. doi:10.17159/wsa/2020.v46.i3.8655
Restrepo-Estrada, C., de Andrade, S. C., Abe, N., Fava, M. C., Mendiondo, E. M., and de Albuquerque, J. P. (20182018). Geo-social media as a Proxy for Hydrometeorological Data for Streamflow Estimation and to Improve Flood Monitoring. Comput. Geosciences 111, 148‒158. doi:10.1016/j.cageo.017.10.01010.1016/j.cageo.2017.10.010
Rosser, J. F., Leibovici, D. G., and Jackson, M. J. (2017). Rapid Flood Inundation Mapping Using Social media, Remote Sensing and Topographic Data. Nat. Hazards 87 (1), 103–120. doi:10.1007/s11069-017-2755-0
Salvati, P., Pernice, U., Bianchi, C., Marchesini, I., Fiorucci, F., and Guzzetti, F. (2016). Communication Strategies to Address Geohydrological Risks: The POLARIS Web Initiative in Italy. Nat. Hazards Earth Syst. Sci. 16 (6), 1487–1497. doi:10.5194/nhess-16-1487-2016
Samu, R., and Kentel, A. S. (20182018). An Analysis of the Flood Management and Mitigation Measures in Zimbabwe for a Sustainable Future. Int. J. Disaster Risk Reduction 31, 691–697. doi:10.1016/j.ijdrr.2018.07.013
Santos, C. A., Freire, P. K., Silva, R. M. D., and Akrami, S. A. (2019). Hybrid Wavelet Neural Network Approach for Daily Inflow Forecasting Using Tropical Rainfall Measuring mission Data. J. Hydrologic Eng., 24(2), Art. No. 04018062. doi:10.1061/(ASCE)HE.1943-5584.0001725
Sattaru, J. S., Bhatt, C. M., and Saran, S. (2021). Utilizing Geo-Social media as a Proxy Data for Enhanced Flood Monitoring. J. Indian Soc. Remote Sens 49 (9), 2173–2186. doi:10.1007/s12524-021-01376-9
Schaake, J. C., Hartman, R., Demargne, J., Mullusky, M., Welles, E., Wu, L., et al. (2004). Ensemble Streamflow Prediction by the National Weather Service (NWS) Advanced Hydrologic Prediction Services (AHPS). American Geophysical Union Spring Meeting Abstracts. H33A-01.
Schaake, J. (2003). “Ensemble Approach to Hydrologic Forecasting for the United States Advanced Hydrologic Prediction Services (AHPS),” in EGS-AGU-EUG Joint Assembly, 11679.
Seebauer, S., Ortner, S., Babcicky, P., and Thaler, T. (2019). Bottom‐up Citizen Initiatives as Emergent Actors in Flood Risk Management: Mapping Roles, Relations and Limitations. J. Flood Risk Manag., 12(3), Art. No. e12468. doi:10.1111/jfr3.12468
Senent-Aparicio, J., Blanco-Gómez, P., López-Ballesteros, A., Jimeno-Sáez, P., and Pérez-Sánchez, J. (2021). Evaluating the Potential of GloFAS-ERA5 River Discharge Reanalysis Data for Calibrating the SWAT Model in the Grande San Miguel River Basin (El Salvador). Remote Sensing 13 (16), 3299. doi:10.3390/rs13163299
Sharp, E. N., and Carter, H. (2020). Examination of How Social media Can Inform the Management of Volunteers during a Flood Disaster. J. Flood Risk Manag. 13 (4), e12665. doi:10.1111/jfr3.12665
Shepherd, J., and van Vuuren, K. (2014). The Brisbane Flood: CALD Gatekeepers' Risk Communication Role. Brisbane flood: CALD gatekeepers' Risk Commun. role Disaster Prev. Manag. 23 (4), 469–483. doi:10.1108/DPM-08-2013-0133
Skinner, P. (2022). Louisiana FloodMaps Portal. Available at: https://www.lsuagcenter.com/topics/family_home/home/design_construction/laws%20licenses%20permits/getting%20a%20permit/your%20flood%20zone/flood_maps (Last accessed 1 25, 2022).
Smith, B., and Rodriguez, S. (2017). Spatial Analysis of High-Resolution Radar Rainfall and Citizen-Reported Flash Flood Data in Ultra-urban New York City. Water 9 (10), 736. doi:10.3390/w9100736
Smith, L., Liang, Q., James, P., and Lin, W. (2017). Assessing the Utility of Social media as a Data Source for Flood Risk Management Using a Real-Time Modelling Framework. J. Flood Risk Manage. 10 (3), 370–380. doi:10.1111/jfr3.12154
Spicer, P., Schlichting, D., Huguenard, K., Roche, A. J., and Rickard, L. N. (2021). Sensing Storm Surge: A Framework for Establishing a Citizen Scientist Monitored Water Level Network. Ocean Coastal Manag. 211, 105802. doi:10.1016/j.ocecoaman.2021.105802
Stephenson, J., Vaganay, M., Coon, D., Cameron, R., and Hewitt, N. (2018). The Role of Facebook and Twitter as Organisational Communication Platforms in Relation to Flood Events in Northern Ireland. J. Flood Risk Manag. 11 (3), 339–350. doi:10.1111/jfr3.12329
Sutanudjaja, E., van Beek, R., Winsemius, H., Ward, P., and Bierkens, M. (2017). “New Version of 1 Km Global River Flood hazard Maps for the Next Generation of Aqueduct Global Flood Analyzer,” in EGU General Assembly Conference Abstracts, 9669. Available at: https://ui.adsabs.harvard.edu/abs/2017EGUGA..19.9669S/abstract (Last accessed 1 25, 2022).
Sy, B., Frischknecht, C., Dao, H., Consuegra, D., and Giuliani, G. (2019). Flood hazard Assessment and the Role of Citizen Science. J. Flood Risk Manag., 12(S2), Art. No. e12519. doi:10.1111/jfr3.12519
Takahashi, B., and Tandoc, E. C. (2016). Media Sources, Credibility, and Perceptions of Science: Learning about How People Learn about Science. Public Underst Sci. 25 (6), 674–690. doi:10.1177/0963662515574986
Tapiador, F. J., Turk, F. J., Petersen, W., Hou, A. Y., García-Ortega, E., Machado, L. A. T., et al. (2012). Global Precipitation Measurement: Methods, Datasets and Applications. Atmos. Res. 104-105, 70–97. doi:10.1016/j.atmosres.2011.10.021
Tekeli-Yeşil, S., Dedeoğlu, N., Braun-Fahrlaender, C., and Tanner, M. (20112011). Earthquake Awareness and Perception of Risk Among the Residents of Istanbul. Nat. Hazards 59, 427–446. doi:10.1007/s11069-011-9764-1
Temitope Olanrewaju, A.-s., Ahmad, R., and Hashim, K. F. (2016). Information Exchanges of Social media Evangelists during Flood: A Social Network Analysis. Jurnal Teknologi 78 (9‒3). doi:10.11113/jt.v78.9718
Tian, X., ten Veldhuis, M.-c., Schleiss, M., Bouwens, C., and van de Giesen, N. (20192019). Critical Rainfall Thresholds for Urban Pluvial Flooding Inferred from Citizen Observations. Sci. Total Environ. 689, 258–268. doi:10.1016/j.scitotenv.2019.06.355
United Nations Institute for Training and Research (2022). UNOSAT Flood portal. Available at: http://floods.unosat.org/geoportal/catalog/main/home.page (Last accessed 1 25, 2022).
United States Geological Survey (2022). Flood Inundation Mapper. Available at: https://fim.wim.usgs.gov/fim/ (Last accessed 1 25, 2022).
Usón, T. J., Klonner, C., and Höfle, B. (20162016). Using participatory geographic approaches for urban flood risk in Santiago de Chile: Insights from a governance analysis. Environ. Sci. Pol. 66, 62–72. doi:10.1016/j.envsci.2016.08.002
Valencio, N., and Valencio, A. (2018). Media Coverage of the 'UK Flooding Crisis': a Social Panorama. Disasters 42 (3), 407–431. doi:10.1111/disa.12255
Vieweg, S., Castillo, C., and Imran, M. (2014). “Integrating Social media Communications into the Rapid Assessment of Sudden Onset Disasters,” in International Conference on Social Informatics (Cham: Springer), 444–461. doi:10.1007/978-3-319-13734-6_32
Vigicrues (2022). Vigicrues. Available at: https://www.vigicrues.gouv.fr/ (Last accessed 1 25, 2022).
Virginia Department of Conservation and Recreation (2022). Virginia Flood Risk Information System (VFRIS). Available at: https://consapps.dcr.virginia.gov/VFRIS/ (Last accessed 1 25, 2022).
Vyncke, B., Perko, T., and Van Gorp, B. (2017). Information Sources as Explanatory Variables for the Belgian Health-Related Risk Perception of the Fukushima Nuclear Accident. Risk Anal. 37 (3), 570–582. doi:10.1111/risa.12618
Wahlberg, A. A. F., and Sjoberg, L. (2000). Risk Perception and the media. J. Risk Res. 3 (1), 31–50. doi:10.1080/136698700376699
Wang, K., Lam, N. S. N., Zou, L., and Mihunov, V. (2021). Twitter Use in Hurricane Isaac and its Implications for Disaster Resilience. Ijgi 10 (3), 116. doi:10.3390/ijgi10030116
Ward, P. (2017). “Aqueduct Global Flood Analyzer-Bringing Risk Information to Practice,” in EGU General Assembly Conference Abstracts, 2725. Available at: https://ui.adsabs.harvard.edu/abs/2017EGUGA..19.2725W/abstract (Last accessed 1 25, 2022).
Ward, P. J., Jongman, B., Salamon, P., Simpson, A., Bates, P., De Groeve, T., et al. (2015). Usefulness and Limitations of Global Flood Risk Models. Nat. Clim Change 5 (8), 712–715. doi:10.1038/nclimate2742
Wehn, U., McCarthy, S., Lanfranchi, V., and Tapsell, S. (2015a). Citizen Observatories as Facilitators of Change in Water Governance? Experiences from Three European Cases. Environ. Eng. Manag. J. 14 (9), 2073–2086. doi:10.30638/eemj.2015.222
Wehn, U., Rusca, M., Evers, J., and Lanfranchi, V. (2015b2015). Participation in Flood Risk Management and the Potential of Citizen Observatories: A Governance Analysis. Environ. Sci. Pol. 48, 225–236. doi:10.1016/j.envsci.2014.12.017
World Food Programme (2022). World Food Programme. Available at: https://geonode.wfp.org/adam.html (Last accessed 1 25, 2022).
Wu, H., Adler, R. F., Tian, Y., Huffman, G. J., Li, H., and Wang, J. (2014). Real-time Global Flood Estimation Using Satellite-Based Precipitation and a Coupled Land Surface and Routing Modelflood Estimation Using Satellite-Based Precipitation and a Coupled Land Surface and Routing Model. Water Resour. Res. 50 (3), 2693–2717. doi:10.1002/2013WR014710
Wu, H., Gu, G., Yan, Y., Gao, Z., and Adler, R. F. (2018). Global Flood Monitoring Using Satellite Precipitation and Hydrological Modeling. Mapp. Forecast., 87–113. doi:10.1002/9781119217886.ch6
Wu, H., Kimball, J. S., Zhou, N., Alfieri, L., Luo, L., Du, J., et al. (2019). Evaluation of Real-Time Global Flood Modeling with Satellite Surface Inundation Observations from SMAP. Remote Sensing Environ. 233, 111360. doi:10.1016/j.rse.2019.111360
Yang, D., Yang, A., Qiu, H., Zhou, Y., Herrero, H., Fu, C.-S., et al. (2019). A Citizen-Contributed GIS Approach for Evaluating the Impacts of Land Use on hurricane-Harvey-induced Flooding in Houston Area. Land 8 (2), 25. doi:10.3390/land8020025
Yeo, J., Knox, C. C., and Hu, Q. (2020). Disaster Recovery Communication in the Digital Era: Social media and the 2016 Southern Louisiana Flood. Risk Anal.. doi:10.1111/risa.13652
Yeo, J., Knox, C. C., and Jung, K. (2018). Unveiling Cultures in Emergency Response Communication Networks on Social media: Following the 2016 Louisiana Floods. Qual. Quant 52 (2), 519–535. doi:10.1007/s11135-017-0595-3
Yoo, E., Rand, W., Eftekhar, M., and Rabinovich, E. (2016). Evaluating Information Diffusion Speed and its Determinants in Social media Networks during Humanitarian Crises. J. Operations Manag. 45 (SI), 123–133. doi:10.1016/j.jom.2016.05.007
You, M., and Ju, Y. (2017). A Comprehensive Examination of the Determinants for Food Risk Perception: Focusing on Psychometric Factors, Perceivers' Characteristics, and Media Use. Health Commun. 32 (1), 82–91. doi:10.1080/10410236.2015.1110003
Zade, H., Shah, K., Rangarajan, V., Kshirsagar, P., Imran, M., and Starbird, K. (2018). From Situational Awareness to Actionability. Proc. ACM Hum.-Comput. Interact. 2, 1–18. doi:10.1145/3274464
Keywords: web-based tools, website, flood insurance and cost, flood forecasting and monitoring, flood zone, social media, mass media, citizen science
Citation: Mostafiz RB, Rohli RV, Friedland CJ and Lee Y-C (2022) Actionable Information in Flood Risk Communications and the Potential for New Web-Based Tools for Long-Term Planning for Individuals and Community. Front. Earth Sci. 10:840250. doi: 10.3389/feart.2022.840250
Received: 20 December 2021; Accepted: 07 February 2022;
Published: 28 February 2022.
Edited by:
Anna Scolobig, Université de Genève, SwitzerlandReviewed by:
Pingping Luo, Chang’an University, ChinaMatteo Convertino, DrEng, Tsinghua University, China
Ashraf Dewan, Curtin University, Australia
Copyright © 2022 Mostafiz, Rohli, Friedland and Lee. This is an open-access article distributed under the terms of the Creative Commons Attribution License (CC BY). The use, distribution or reproduction in other forums is permitted, provided the original author(s) and the copyright owner(s) are credited and that the original publication in this journal is cited, in accordance with accepted academic practice. No use, distribution or reproduction is permitted which does not comply with these terms.
*Correspondence: Rubayet Bin Mostafiz, cmJpbm1vMUBsc3UuZWR1