- 1Sadovsky Institute for Dynamics of Geospheres, Russian Academy of Sciences, Moscow, Russia
- 2Department of Theoretical and Experimental Physics of Geosystems, Moscow Institute of Physics and Technology, Dolgoprudny, Russia
- 3Department of Robotic Systems and Mechatronics, Bauman Moscow State Technical University, Moscow, Russia
Tectonic faults show rheological heterogeneity in interfaces, and the spectrum of their sliding regimes span a continuum from the slow-slip events to dynamic ruptures. The heterogeneity of the fault interface is crucial for the mechanics of faulting. By using the earthquake source locations, the complex structure of a fault interface can be reproduced at a resolution down to 50–100 m. Here, we use a declustered seismic catalog of Northern California to investigate structures of 11 segments of San Andreas, Calaveras, and Hayward faults. The cumulative length of all the segments is about 500 km. All the selected segments belong to subvertical strike–slip faults. A noticeable localization of sources near the fault cores is observed for all segments. The projection of earthquake sources to the fault plane shows severe inhomogeneity. Topologically dense clusters (seismogenic patches (SPs)) can be detected in fault planes. The longer the observation are, the more distinct are the clusters. The SPs usually cover about 10%–20% of the fault interface area. It is in the vicinity of SPs that earthquakes of magnitudes above 5 are usually initiated. The Voronoi tessellation is used to determine the orderliness of SPs. Distributions of areas of Voronoi cells of all the SPs obey the lognormal law, and the value of Voronoi entropy of 1.6–1.9 prevails. The findings show the informativeness of the background seismicity in revealing the heterogenous structure of a tectonic fault interface.
Introduction
Earthquake source localization is one of the fundamental problems of seismology and continues to attract considerable attention. In 1910, H.F. Reid provided arguments that earthquakes are linked to faults in the Earth’s crust (Reid, 1910). The localization of earthquake hypocenters in fault zones and tectonic junctions manifests most evidently if the accuracy of their location is high enough (Waldhauser and Schaff, 2008). Earthquake epicenters trace tectonic faults, while the dynamics of seismicity allows judging about fault slip behavior (Valoroso et al., 2014; Vorobieva et al., 2016; Ross et al., 2020).
Earthquake rupture dynamics is controlled by the processes taking place in fault zones. Direct studies of exhumed faults are the main source of information about the fault zone structure. At all scales, fault planes are rough (Candella et al., 2012). Because of the roughness of fault edge surfaces, specific contact areas can be detected in fault interfaces, the so-called asperities, which concentrate tectonic stresses (Lay et al., 1982). Strong asperities form when granular mineral phases such as quartz, feldspar, pyroxene, olivine, calcite, and dolomite predominate. Their static strength is consistent with the Coulomb criterion under Byerlee’s friction law, 0.6 <μ < 0.85 (Collettini et al., 2019). To generate a dynamic rupture, the asperity must be velocity-weakening, which means that the resistance to shear decreases as the sliding velocity grows (Dieterich, 1978; Barbot, 2019). Thus, the asperity will be statically strong and dynamically weak. The area around an asperity is rather unloaded and with a high probability displays velocity strengthening (Scholz and Campos, 2012; Collettini et al., 2019), that is, frictional strength increases as the sliding velocity increases. The rheological heterogeneity of the fault interface can be traced at different scales (Fageneng, 2011; Collettini et al., 2019).
Sizes of asperities can be accessed via seismological methods by determining the slip distribution at the fault plane from the inversion of body waveforms. It is suggested that asperities correspond to the areas with maximum slip amplitude. The slip distribution may either have a single maximum or several slip maxima distributed over the fault plane (Zeng, Anderson, 2000; Yamanaka and Kikuchi, 2004). The slip distribution with several maxima may be interpreted as a complex rupture. In strong earthquakes, the co-seismic ruptures, starting at one of the asperities, can “pass” through the conditionally stable segment and trigger other asperities. The characteristic size of asperity is about 1.5–3 times less than the length of the co-seismic rupture (Kocharyan and Kishkina, 2021). The characteristic sizes of asperities become apparent in the spectra of emitted seismic waves as well (Gusev, 2013).
Geodetic measurements are one more source of information about the location and size of asperities. The degree of interseismic coupling (the ratio of the interseismic slip rate to the plate-convergence velocity (Ruff, Kanamori, 1983)) is determined by resting on the GPS data. Inside the asperity, when the fault is fully locked, the coupling is 1.0. Areas where the average coupling can reach values as low as 0.4 could, therefore, be associated with areas of velocity strengthening behavior—able to slow down or stop rupture propagation (Métois et al., 2016). At creeping segments, where no strong earthquakes occur, the coupling is small. High coupling (inside the asperity) occurs in zones subjected to high normal stresses with a switch to low coupling occurring abruptly as the normal stress decreases below a critical value (Scholz and Campos, 2012).
Probably, the structure of a fault interface remains invariable at least for several decades (Ide, 2019). As fault rupture starting at one of the asperities can “pass” through the conditionally stable segment and trigger other asperities, one cannot judge about the regularities of the emergence of different slip modes (fast dynamic rupture, slow slip event, and aseismic creep (Peng and Gomberg, 2010; Fageneng and Beall, 2021) without understanding the orderliness of asperities. Beyond all doubts, asperities have complex spatial structures. It is suggested that asperities have self-similar structures with the coefficient of self-similarity from 1.4 to 2.5 (Seno, 2003; Mykulyak, 2018; Kocharyan and Kishkina, 2021). But, both the slip distribution patterns obtained in the inversion of body waves and the seismic coupling distribution obtained in geodetic measurements cannot precisely trace the boundary between an asperity with velocity weakening and the surrounding relatively stable area with velocity strengthening. The high accuracy of a weak earthquake source location allows considering seismic catalogs as new sources of information about the structure of fault zones and about the asperity orderliness (Kocharyan and Ostapchuk, 2022). While a single seismic event points to a possible area of asperity localization, an ensemble of events allows judging about asperity orderliness.
Here, we concentrate on the spatial distribution of earthquakes over the fault interface. Before analyzing the seismicity over space, all dependent events, including the main shocks, were removed from the seismic catalog. We applied the method of topological filtering to obtain topologically dense clusters of seismic sources whose areas of localization were interpreted as seismogenic patches (SPs). SPs can be marked out in the fault interface. The structural features of SPs were investigated using Voronoi tessellation.
Seismic Data
We examine spatial structuredness of the fault interface along the San Andreas fault system using the hypocenter information of Double-difference Earthquake Catalog for Northern California. The seismic catalog being analyzed includes 513,474 events from 1984 to 2011. The locations of earthquakes presented in the catalog are shown in Figure 1. The catalog is based on 1.7 billion CC differential time measurements with the correlation coefficients Cf > = 0.7 from all correlated pairs of events that are separated by less than 5 km (Schaff and Waldhauser, 2005). The accuracy of local earthquake locations over horizontal, and depth is less 40 m. The magnitude of completeness Mc is equal to 1.1 (Wiemer, 2001), and its Gutenberg–Richter distribution is as follows (Gutenberg and Richter, 1944):
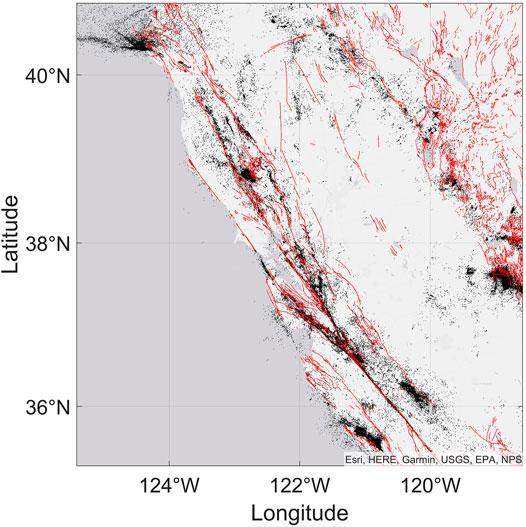
FIGURE 1. Map of seismicity and tectonic faults. Presented are earthquakes (dots) recorded by the Northern California Seismic System (NCSS) between January 1984 and December 2011. Red lines indicate the mapped surface fault traces.
Earthquake localization clearly traces the architecture of fault system. Eleven segments of the well-defined subvertical strike–slip faults were selected for detailed analysis (Table 1; Figure 1). Each selected segment comprises one zone of fault core (Fagereng and Sibson, 2010; McKay et al., 2021). The cumulative length of the selected segments is about 500 km, and 11 earthquakes with ML ≥ 5 belong to the selected segments from 1984 to 2011.
The hypocentral delineation shows that the main portion of earthquakes is concentrated in the vicinity of a single plane which should be interpreted as the fault plane (Figure 2). The layer including 95% of sources localized within 5 km from the fault plane was defined as the seismogenic thickness (Table 1).
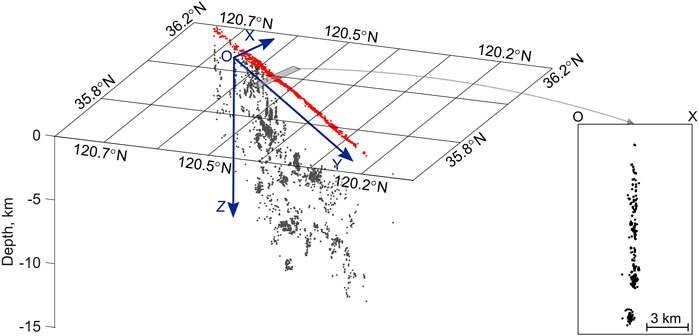
FIGURE 2. Localization of earthquake foci. 3D localization of earthquakes for segment no. 6 (Table 1). Red dots show localization of earthquake epicenters. The inset corresponds to the gray rectangle and shows the fault-normal 3D cross section 4 km long. oXYZ is the coordinate system linked to the fault: OY–direction of fault strike, OX–direction perpendicular to fault strike, and OZ–direction of fault dip.
Considering the segments with narrow seismogenic thickness, it seems reasonable further on to detect the structural properties of the interface in the coordinate system linked to the fault plane (Figure 2). OY is the distance along fault strike, OX is the distance perpendicular to fault strike, and OZ is the distance along fault dip. However, the difference of coordinates along the OX direction can be neglected because the seismogenic thickness is less (Table 1). Figure 3 presents localization of earthquake hypocenters over the fault plane for the segment no. 9. One can detect the areas of point concentration and areas with numerous scattered points. The spatial distribution tends the aggregated type. The distribution of distances between the earthquake foci shows the presence of a single maximum. The characteristic distances between the earthquake foci for all the segments are shown in Table 1.
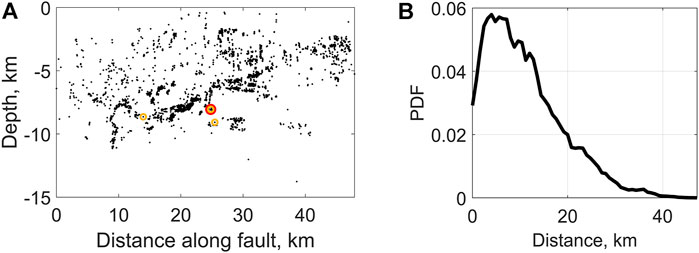
FIGURE 3. Fault plane view of earthquake localizations for segment no. 9 (Table 1). (A) Projection of earthquake foci on the fault plane (yellow dots are the earthquakes with ML ≥ 5 and red circle is the 1984 Morgan Hill earthquake M6.2); (B) Probability density function of the distance between each pair of earthquake foci on the fault plane. Single maximum of the distance distribution is observed, and the mode is 6 km.
Methods
Algorithm of Declustering the Earthquake Catalog
It is well known that foreshocks and aftershocks group in space and time. Declustering of the earthquake catalog (withdrawal of clustered events) is necessary for the analysis of timeless features of the fault structure. To identify the background and clustered populations of seismicity, we follow the method first proposed by Baiesi and Paczuski (2004). It is based on the calculation of nearest neighbor distance in time–space–magnitude domains. For each pair of earthquakes {i, j}, we compute the proximity function (Zaliapin et al., 2008):
where
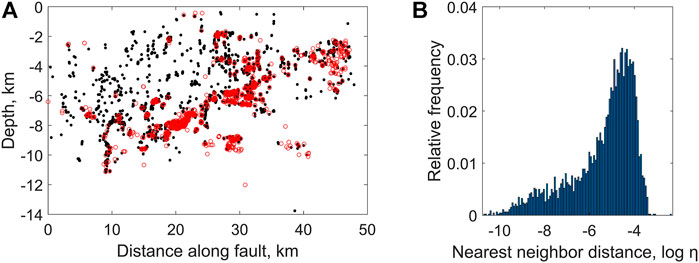
FIGURE 4. Two modes of seismicity (segment no. 9, Table 1). (A) Localizations of the background (dark) and clustered (red) modes of seismicity; (B) Relative frequency histogram of the nearest-neighbor distance η.
Algorithm of Seismic Source Topological Filtering
The spatial distribution of the background seismicity shows a severe inhomogeneity. Several areas of event grouping can be detected, where the stronger it manifests, the longer is the monitoring duration. The Discrete Perfect Sets (DPS) algorithm of topological filtering was applied to reveal dense clusters (Agayan et al., 2014; Dzeboev et al., 2021). The DPS is aimed at isolating subsets with a given density level α in a finite set of Euclidean space. The DPS cuts out isolated objects (subset H) and concatenates rest of the objects in dense clusters (subset B) from the set W of recognition objects of seismic sources:
The result of DPS algorithm is as follows:
where q and β are the free parameters of the algorithm.
The parameter q determines the localization radius rq(W), which is calculated as the power mean of all nontrivial pairwise distances D(W) in a set of recognition objects W:
For a specified radius of location r, the density of an arbitrary subset
The determination of density rests on a fuzzy comparison (Gvishiani et al., 2008). The measure of comparison of the densities PW(W) of set W in all its points
The parameter β in Eq. 4 defines the maximality of density and
It follows from Eq. 5 that
The dense sets
Detection of Seismogenic Patches
Detection of SPs was performed depending on the analysis of the catalog of background seismicity. We rely on the fact that SPs emerge in the zones of most intensive interaction of the fault edges; consequently, it should manifest in the background seismicity. After the DPS filtration of the declustered catalog, a dense set
• Localization of earthquakes in the interface plane shows that along with a pronounced grouping of events, the specific distances between them can be detected (Figure 3). These specific distances are characteristic of all selected segments, and their values are given in Table 1. Condition I: if SPs form in an interface containing dense clusters of seismic sources, the specific distance between the clusters should correspond to the specific distance between all the sources that are localized in the fault segment under consideration.
• The number of SPs that could form in the fault plane, generally, should be defined by the geometry of fault edges, and particularly, by their long-wavelength roughness (Selvadurai and Glaser, 2017; Kocharyan et al., 2022). However, under high heterogeneity of the fault interface, some SPs can turn to be “invisible”, for example, due to velocity strengthening of the interface. Condition II: the SP configuration which best represents the current state of knowledge about the heterogeneity of the fault interface is the one with the largest number of SPs.
The two abovementioned conditions allow to unambiguously define the parameters q and β and, consequently, unambiguously detect the dense clusters of seismic sources
Results
Figure 5 presents the example for detecting SPs. SPs with areas from 0.03 to 56 km2 are detected. Patch boundaries of an ellipse form and the most probable value of flattening is 0.6. Two to 11 SPs can be detected in a separate segment, and the areal density of SPs varies from 0.02 to 0.13 km−2 (Table 1).
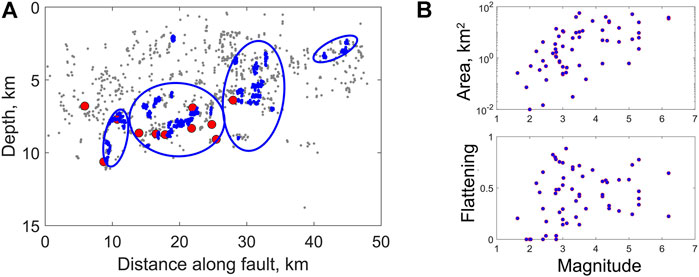
FIGURE 5. Seismogenic patches. (A) Localization of SPs in the interface of fault segment no. 9 (Table 1). Boundaries of SPs are represented by blue ellipses. Gray dots mark the background seismicity, blue dots correspond to dense clusters, and red dots represent earthquakes with ML ≥ 4. (B) Plots of SP area and SP flattening vs. magnitude of the largest earthquake localized inside or in the vicinity of the SP.
As SPs are detected over the declustered catalog, the question arises: «Do sources of earthquakes with ML ≥ 5 get into SPs?" Figure 6 shows the variation of distances from the earthquake sources to the boundary of the nearest SP. One can see that nine of 11 earthquakes with ML ≥ 5 are localized inside or in the vicinity [distance is less than one earthquake source radius (Brune, 1970)] of SPs. For earthquakes with ML ≥ 4, 60% of the events localize inside or in the vicinity of SPs.
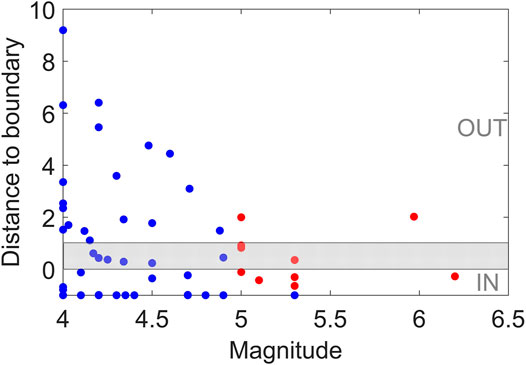
FIGURE 6. Attribution of earthquakes with ML ≥ 4 to SPs. Distance from the earthquake focus to the SP boundary. Distance is normalized by earthquake radius. Distance “0” corresponds to the ellipse boundary. Gray area shows localization near the boundary.
Fault asperities are complex structured objects. Earthquakes are initiated by slippage in a distinct domain in the vicinity of velocity-weakening asperities (Collettini et al., 2019, Barbot, 2019; Kocharyan, 2021). Thus, the distribution of earthquake sources over an SP reveals localizations of some small-scale asperities inside this SP and allows judging about the structural peculiarities of the large-scale asperity [akin to building up a diffraction pattern from individual electrons by recording single one detection events diffracting through a double-slit (Bach et al., 2013)]. Voronoi tessellation is applied to characterize the distribution of background seismic sources from dense clusters
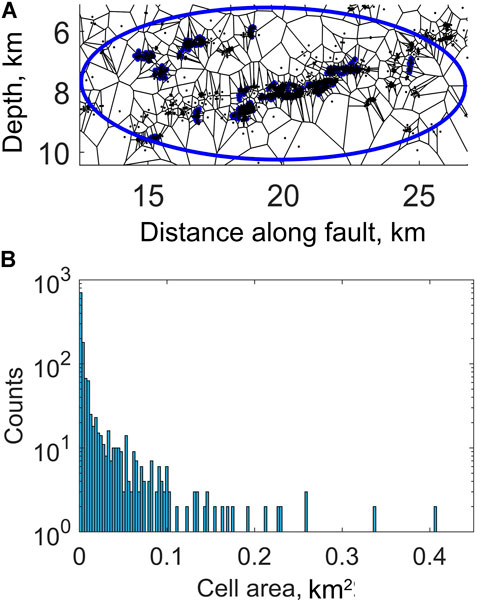
FIGURE 7. Example of Voronoi tessellation of SPs (segment no. 9, Table 1). Voronoi tessellation (A) and distribution of Voronoi cell areas (B) of SPs in the interface of a fault.
If RLR is positive, then the power-law distribution is mathematically a better fit, while a negative value indicates that the alternative distribution is a better fit. We reveal that the log-normal distribution provides a statistically better fit for 75% of all the SPs of all the segments. The remaining SPs cannot be reliably statistically fitted by any of the three (power, log-normal, or exponential) distributions.
The Voronoi entropy is used to quantify the orderliness of the Voronoi tessellation (Bormashenko et al., 2018). The Voronoi entropy is defined as follows:
where Pi is the fraction of cells with n sides for a given Voronoi tessellation. One can see that the entropy varies in a wide range from 1.2 to 1.9 (Table 1). There is no difference between the SPs with earthquakes ML ≥ 4 and SPs with earthquakes ML ≤ 4. The Voronoi entropy becomes zero for a perfectly ordered structure consisting of a single type of cells. For a fully random 2D distribution of points, the value of SVor is 1.71 (Bormashenko et al., 2018).
Discussion
Earthquake source localizations provide essential information about the crustal fault architecture (Kocharyan et al., 2010; Valoroso et al., 2014), while space distribution of dense earthquake clusters highlights the geometric features and rheological heterogeneity of the fault interface.
During a long-term evolution, asperities are subjected to crushing, cataclasis, and frictional wearing, which lead to asperity fragmentation. The log-normal distribution is characteristic of rock fragmentation in blasting and of particle-size distribution in cataclasis (Phillips and Williams, 2021). This can be explained by the fact that the probability of asperity fracturing is independent of asperity size and history (Epstein, 1947). Voronoi mosaic highlights the fragmentation of SPs. The areas of Voronoi cells are distributed according to the log-normal law; hence, the fragmentation of SPs should obey the log-normal law too.
While at the initial stages of fault evolution, an intensive crushing of asperities takes place, a frictional sliding along with flattened asperities goes on in mature faults (Yang et al., 2001). The seismogenic thickness of a mature fault corresponds to the effective thickness of the fractured zone, which is controlled by the waviness of the fault surface (Kocharyan et al., 2010). The inclination of a large-scale asperity at the edge of a mature fault is about
Relation (12) can also link the specific distance between SPs and seismogenic thickness (Table 1). It means that the specific distance corresponds to the large-scale wavelength, and the localization of SPs is predetermined by the waviness of fault edges. SPs must be associated with the fault large-scale asperities. At the same time, fault roughness should control the orderliness at a lower scale. Since SPs are topologically dense clusters, areas of SP localization are characterized by relatively lower roughness.
Two conditions should be true for the slip instability to form. First, the fault segment should display velocity weakening, which means that the resistance to shear decreases as the sliding velocity increases (Dieterich, 1978). Second, the rheologic stiffness (kc) of the fault segment should be higher than the stiffness of the host rock (K). The fault slip mode and earthquake radiation efficiency are governed by the ratio of kc/K. The higher the ratio kc/K is, the higher is the earthquake radiation efficiency (Leeman et al., 2016; Kocharyan et al., 2017). The stiffness of the host rock is (Scholz, 2002) as follows:
where G is the shear modulus of the enclosing massif, η ∼ 1 is the shape factor, and L is the characteristic fault size correlating with the earthquake magnitude. The rheologic stiffness (kc) is determined as
where (b–a) are the frictional rate parameters, ((b–a) > 0—the segment is velocity-weakening, (b–a) < 0—the segment is velocity-strengthening), σn is the normal stress, and Dc is the critical slip distance (Leeman et al., 2016). Spatial variations in the kc/K ratio occur mainly due to changes in rheological stiffness.
The fault roughness controls the critical slip distance Dc (Dieterich, 1979). Laboratory studies have pointed out that Dc decreases with the decreasing fault roughness, and small Dc favors unstable slip. Since asperities are characterized by relatively lower roughness, the fault segments containing asperities are frictionally more unstable ones.
Single crustal fault, tens of kilometers long, can be characterized by both weak fault patches with velocity strengthening ((b–a) < 0) and strong fault patches with velocity weakening ((b–a) > 0) (Collettini et al., 2019). Formation of a frictionally heterogenous structure is determined by metasomatic processes that accompany the long-term evolution of a fault. In rather unloaded areas, an active fluid input occurs and crustal and mantle fluid exerts a chemical role, facilitating the replacement of strong with weak mineral phases (Collettini et al., 2019; Kocharyan, 2021). The frictional strength of the unloaded areas decreases, and the frictional parameter (b–a) decreases. In the stressed zones (in asperities), fluid-assisted reaction of softening is not efficient, and crustal deformation is achieved predominantly by fragmentation and grain-size reduction (Kocharyan, 2021). The frictional strength remains high and obeys the Byerlee’s law, while asperity remains velocity-weakening. As a rule, weak patches with velocity-strengthening show predominantly aseismic creep (Chen, Lapusta, 2009), while strong patches (asperities) determine the seismicity features of the fault segment.
Conclusion
We have presented a new approach to the analysis of seismicity clustering, revealing the structural features of fault interfaces. For the first time, the seismicity pattern is associated with the frictional heterogeneity of the fault interface. Spatial clustering of background seismicity is controlled by the geometric features and frictional heterogeneity of the fault interface. Topologically dense clusters (SPs) can be detected in the fault planes, which are associated with strong velocity-weakening asperities. It is in the vicinity of SPs that earthquakes of magnitudes greater than 5 are usually initiated.
Data Availability Statement
Publicly available datasets were analyzed in this study. This data can be found here: https://www.ldeo.columbia.edu/∼felixw/NCAeqDD/.
Author Contributions
AO was responsible for research conceptualization, organization of the work, and writing the first draft of the article. GK contributed to research conceptualization and problem statement. MP preprocessed the data, declustered the seismic catalog, and prepared figures. VP programed the algorithm and prepared figures. All authors were involved in editing the final version of the manuscript.
Funding
This work was partially supported by the Russian Science Foundation according to the research project No. 20-77-10087 for AO and MP. VP and GK acknowledge Program of IDG RAS No. 122032900178-7.
Conflict of Interest
The authors declare that the research was conducted in the absence of any commercial or financial relationships that could be construed as a potential conflict of interest.
Publisher’s Note
All claims expressed in this article are solely those of the authors and do not necessarily represent those of their affiliated organizations, or those of the publisher, the editors, and the reviewers. Any product that may be evaluated in this article, or claim that may be made by its manufacturer, is not guaranteed or endorsed by the publisher.
References
Agayan, S. M., Bogoutdinov, S. R., and Dobrovolsky, M. N. (2014). Discrete Perfect Sets and Their Application in Cluster Analysis. Cybern. Syst. Anal. 50, 176–190. doi:10.1007/s10559-014-9605-9
Bach, R., Pope, D., Liou, S.-H., Batelaan, H., and Nebraska, U. (2013). Controlled Double-Slit Electron Diffraction. New J. Phys. 15 (3), 033018. doi:10.1088/1367-2630/15/3/033018
Baiesi, M., and Paczuski, M. (2004). Scale-free Networks of Earthquakes and Aftershocks. Phys. Rev. E 69, 066106. doi:10.1103/PhysRevE.69.066106
Barbot, S. (2019). Slow-slip, Slow Earthquakes, Period-Two Cycles, Full and Partial Ruptures, and Deterministic Chaos in a Single Asperity Fault. Tectonophysics 768, 228171. doi:10.1016/j.tecto.2019.228171
Bormashenko, E., Frenkel, M., Vilk, A., Legchenkova, I., Fedorets, A., Aktaev, N., et al. (2018). Characterization of Self-Assembled 2D Patterns with Voronoi Entropy. Entropy 20, 956. doi:10.3390/e20120956
Brune, J. N. (1970). Tectonic Stress and the Spectra of Seismic Shear Waves from Earthquakes. J. Geophys. Res. 75, 4997–5009. doi:10.1029/JB075i026p04997
Candela, T., Renard, F., Klinger, Y., Mair, K., Schmittbuhl, J., and Brodsky, E. E. (2012). Roughness of Fault Surfaces over Nine Decades of Length Scales. J. Geophys. Res. 117, a–n. doi:10.1029/2011JB009041
Chen, T., and Lapusta, N. (2009). Scaling of Small Repeating Earthquakes Explained by Interaction of Seismic and Aseismic Slip in a Rate and State Fault Model. J. Geophys. Res. 114, B01311. doi:10.1029/2008JB005749
Clauset, A., Shalizi, C. R., and Newman, M. E. J. (2009). Power-law Distributions in Empirical Data. SIAM Rev. 51 (4), 661–703. doi:10.1137/070710111
Collettini, C., Tesei, T., Scuderi, M. M., Carpenter, B. M., and Viti, C. (2019). Beyond Byerlee Friction, Weak Faults and Implications for Slip Behavior. Earth Planet. Sci. Lett. 519, 245–263. doi:10.1016/j.epsl.2019.05.011
Dieterich, J. H. (1979). Modeling of Rock Friction: 1. Experimental Results and Constitutive Equations. J. Geophys. Res. 84, 2161–2168. doi:10.1029/jb084ib05p02161
Dieterich, J. H. (1978). Time-dependent Friction and the Mechanics of Stick-Slip. PAGEOPH 116, 790–806. doi:10.1007/bf00876539
Dzeboev, B. A., Gvishiani, A. D., Agayan, S. M., Belov, I. O., Karapetyan, J. K., Dzeranov, B. V., et al. (2021). System-Analytical Method of Earthquake-Prone Areas Recognition. Appl. Sci. 11, 7972. doi:10.3390/app11177972
Epstein, B. (1947). The Mathematical Description of Certain Breakage Mechanisms Leading to the Logarithmico-Normal Distribution. J. Frankl. Inst. 244 (6), 471–477. doi:10.1016/0016-0032(47)90465-1
Fagereng, Å., and Beall, A. (2021). Is Complex Fault Zone Behaviour a Reflection of Rheological Heterogeneity? Phil. Trans. R. Soc. A 379, 20190421. doi:10.1098/rsta.2019.0421
Fagereng, Å. (2011). Frequency-size Distribution of Competent Lenses in a Block-In-Matrix Mélange: Imposed Length Scales of Brittle Deformation? J. Geophys. Res. 116, B05302. doi:10.1029/2010JB007775
Fagereng, Å., and Sibson, R. H. (2010). Mélange Rheology and Seismic Style. Geology 38 (8), 751–754. doi:10.1130/G30868.1
Gusev, A. A. (2013). High-Frequency Radiation from an Earthquake Fault: A Review and a Hypothesis of Fractal Rupture Front Geometry. Pure Appl. Geophys. 170, 65–93. doi:10.1007/s00024-012-0455-y
Gutenberg, R., and Richter, C. F. (1994). Frequency of Earthquakes in California. Bull. Seismol. Soc. Am. 34, 185
Gvishiani, A. D., Agayan, S. M., Bogoutdinov, S. R., Zlotnicki, J., and Bonnin, J. (2008). Mathematical Methods of Geoinformatics. III. Fuzzy Comparisons and Recognition of Anomalies in Time Series. Cybern. Syst. Anal. 44 (3), 309–323. doi:10.1007/s10559-008-9009-9
Ide, S. (2019). Frequent Observations of Identical Onsets of Large and Small Earthquakes. Nature 573, 112–116. doi:10.1038/s41586-019-1508-5
Kocharyan, G. G., and Spivak, A. A. (2003). Dynamics of Rock Deformation. Moscow: Akademkniga. (in Russian).
Kocharyan, G. G., Kishkina, S. B., and Ostapchuk, A. A. (2010). Seismic Picture of a Fault Zone. What Can Be Gained from the Analysis of Fine Patterns of Spatial Distribution of Weak Earthquake Centers? Geodyn. Tectonophys. 1 (4), 419–440. doi:10.5800/gt-2010-1-4-0027
Kocharyan, G. G., and Kishkina, S. B. (2021). The Physical Mesomechanics of the Earthquake Source. Phys. Mesomech. 24, 343–356. doi:10.1134/s1029959921040019
Kocharyan, G. G., Novikov, V. A., Ostapchuk, A. A., and Pavlov, D. V. (2017). A Study of Different Fault Slip Modes Governed by the Gouge Material Composition in Laboratory Experiments. Geophys. J. Int. 208 (1), 521–528. doi:10.1093/gji/ggw409
Kocharyan, G. G. (2021). Nucleation and Evolution of Sliding in Continental Fault Zones under the Action of Natural and Man-Made Factors: A State-Of-The-Art Review. Izv. Phys. Solid Earth 57, 439–473. doi:10.1134/S1069351321040066
Kocharyan, G., and Ostapchuk, A. (2022). The Mesostructure of the Slip Interface of a Tectonic Fault. Phys. Mesomech. 2022, 4
Lay, T., Kanamori, H., and Ruff, L. (1982). The Asperity Model and the Nature of Large Subduction Zone Earthquakes. Earthq. Pred. Res. 1, 3
Leeman, J. R., Saffer, D. M., Scuderi, M. M., and Marone, C. (2016). Laboratory Observations of Slow Earthquakes and the Spectrum of Tectonic Fault Slip Modes. Nat. Commun. 7, 11104. doi:10.1038/ncomms11104
McKay, L., Lunn, R. J., Shipton, Z. K., Pytharouli, S., and Roberts, J. J. (2021). Do intraplate and Plate Boundary Fault Systems Evolve in a Similar Way with Repeated Slip Events? Earth Planet. Sci. Lett. 559 (116), 116757. doi:10.1016/j.epsl.2021.116757
Métois, M., Vigny, C., and Socquet, A. (2016). Interseismic Coupling, Megathrust Earthquakes and Seismic Swarms along the Chilean Subduction Zone (38°-18°S). Pure Appl. Geophys. 173, 1431–1449. doi:10.1007/s00024-016-1280-5
Mykulyak, S. V. (2018). Hierarchical Block Model for Earthquakes. Phys. Rev. E 97, 062130. doi:10.1103/PhysRevE.97.062130
Peng, Z., and Gomberg, J. (2010). An Integrated Perspective of the Continuum between Earthquakes and Slow-Slip Phenomena. Nat. Geosci. 3, 599–607. doi:10.1038/ngeo940
Phillips, N. J., and Williams, R. T. (2021). To D or Not to D? Re-evaluating Particle-Size Distributions in Natural and Experimental Fault Rocks. Earth Planet. Sci. Lett. 553, 116635. doi:10.1016/j.epsl.2020.116635
Reid, H. F. (1910). The Mechanics of the Earthquake. The California Earthquake of April 18, 1906. Report of the State Investigation Commission. Washington, DC: Carnegie Institution of Washington.
Ross, Z. E., Cochran, E. S., Trugman, D. T., and Smith, J. D. (2020). 3D Fault Architecture Controls the Dynamism of Earthquake Swarms. Science 368 (6497), 1357–1361. doi:10.1126/science.abb0779
Ruff, L., and Kanamori, H. (1983). Seismic Coupling and Uncoupling at Subduction Zones. Tectonophysics 99 (2–4), 99–117. doi:10.1016/0040-1951(83)90097-5
Schaff, D. P., and Waldhauser, F. (2005). Waveform Cross-Correlation-Based Differential Travel-Time Measurements at the Northern California Seismic Network. Bull. Seismol. Soc. Am. 95, 2446–2461. doi:10.1785/0120040221
Schoenberg, F. P., Barr, C., and Seo, J. (2009). The Distribution of Voronoi Cells Generated by Southern California Earthquake Epicenters. Environmetrics 20 (2), 159–171. doi:10.1002/env.917
Scholz, C. H. (2002). The Mechanics of Earthquakes and Faulting. Cambridge: Cambridge University Press.
Scholz, C. H., and Campos, J. (2012). The Seismic Coupling of Subduction Zones Revisited. J. Geophys. Res. 117, B05310. doi:10.1029/2011JB009003
Selvadurai, P. A., and Glaser, S. D. (2017). Asperity Generation and its Relationship to Seismicity on a Planar Fault: a Laboratory Simulation. Geophys. J. Int. 208, 1009–1025. doi:10.1093/gji/ggw439
Seno, T. (2003). Fractal Asperities, Invasion of Barriers, and Interplate Earthquakes. Earth Planet Sp. 55, 649–665. doi:10.1186/BF03352472
Shebalin, P., and Narteau, C. (2017). Depth Dependent Stress Revealed by Aftershocks. Nat. Commun. 8, 1317. doi:10.1038/s41467-017-01446-y
Tsuboi, C. (1956). Earthquake Energy, Earthquake Volume, Aftershock Area, and Strength of the Earth's Crust. J. Phys. Earth 4, 63–66. doi:10.4294/jpe1952.4.63
Valoroso, L., Chiaraluce, L., and Collettini, C. (2014). Earthquakes and Fault Zone Structure. Geology 42 (4), 343–346. doi:10.1130/G35071.1
Vorobieva, I., Shebalin, P., and Narteau, C. (2016). Break of Slope in Earthquake Size Distribution and Creep Rate along the San Andreas Fault System. Geophys. Res. Lett. 43, 6869–6875. doi:10.1002/2016GL069636
Voronoi, G. (1908). Nouvelles applications des paramètres continus à la théorie des formes quadratiques Deuxième mémoire. Recherches sur les parallélloèdres primitifs. Reine Angew. Math. 1908, 198–287. doi:10.1515/crll.1908.134.198
Waldhauser, F., and Schaff, D. P. (2008). Large-scale Relocation of Two Decades of Northern California Seismicity Using Cross-Correlation and Double-Difference Methods. J. Geophys. Res. 113, B08311. doi:10.1029/2007JB005479
Wiemer, S. (2001). A Software Package to Analyze Seismicity: ZMAP. Seismol. Res. Lett. 72 (3), 373–382. doi:10.1785/gssrl.72.3.373
Yamanaka, Y., and Kikuchi, M. (2004). Asperity Map along the Subduction Zone in Northeastern Japan Inferred from Regional Seismic Data. J. Geophys. Res. 109, B07307. doi:10.1029/2003JB002683
Yang, Z. Y., Di, C. C., and Yen, K. C. (2001). The Effect of Asperity Order on the Roughness of Rock Joints. Int. J. Rock Mech. Min. Sci. 38, 745–752. doi:10.1016/S1365-1609(01)00032-6
Zaliapin, I., and Ben-Zion, Y. (2013). Earthquake Clusters in Southern California I: Identification and Stability. J. Geophys. Res. Solid Earth 118, 2847–2864. doi:10.1002/jgrb.50179
Zaliapin, I., Gabrielov, A., Keilis-Borok, V., and Wong, H. (2008). Clustering Analysis of Seismicity and Aftershock Identification. Phys. Rev. Lett. 101 (1), 018501. doi:10.1103/PhysRevLett.101.018501
Keywords: earthquake localization, seismic catalog, tectonic asperity, topological filtering, Voronoi tessellation, San Andreas fault
Citation: Ostapchuk A, Polyatykin V, Popov M and Kocharyan G (2022) Seismogenic Patches in a Tectonic Fault Interface. Front. Earth Sci. 10:904814. doi: 10.3389/feart.2022.904814
Received: 25 March 2022; Accepted: 19 April 2022;
Published: 24 May 2022.
Edited by:
Peter Shebalin, Institute of Earthquake Prediction Theory and Mathematical Geophysics (RAS), RussiaReviewed by:
Alexey Lyubushin, Institute of Physics of the Earth (RAS), RussiaEleftheria Papadimitriou, Aristotle University of Thessaloniki, Greece
Copyright © 2022 Ostapchuk, Polyatykin, Popov and Kocharyan. This is an open-access article distributed under the terms of the Creative Commons Attribution License (CC BY). The use, distribution or reproduction in other forums is permitted, provided the original author(s) and the copyright owner(s) are credited and that the original publication in this journal is cited, in accordance with accepted academic practice. No use, distribution or reproduction is permitted which does not comply with these terms.
*Correspondence: Aleksey Ostapchuk, ostapchuk.aa@phystech.edu, ostapchuk.aa@idg.ras.ru