- 1Research and Academic Branch, Fourth Military Medical University, Xi’an, China
- 2Department of Military Biomedical Engineering, Fourth Military Medical University, Xi’an, China
Objective: Non-contact penetrating detection and sensing of human beings (vital signs) through nonmetallic obstacles (ruins, wall, and smog) using the ultra-wideband (UWB) bio-radar plays a significant role in various post-disaster rescue operations in national public security events, like earthquake, building collapse, and factory explosion. In practical application scenarios, the narrowband radio frequency interference (RFI) and surrounding movement interference (SMI) are the two most common and major types of environmental interference (EI), which would cause serious effects on the penetrating detection performance of the UWB bio-radar.
Methods: Therefore, through establishing a quantitative and controllable experiment system and combining a proposed quantitative evaluation method, this paper quantitatively investigates and evaluates the influencing characteristics and laws of these two interferences on human vital sign detection using the UWB bio-radar. In the quantitative experiments, two key environmental spatial parameters (interference distance and angle) of interference sources and two kinds of system parameters (main lobe width and time window) of the UWB bio-radar are considered.
Results: Numerous experiments at different interference positions and corresponding statistical results demonstrated that both the RFI and SMI have a high negative correlation with the interference distance. Meanwhile, the SMI, which is highly related to the interference angle, could be screened or isolated by the detecting time window and main lobe boundary of UWB bio-radar, but the RFI could not be detected.
Discussion: This study shows potential in providing transcendental knowledge and guidance for special EI suppression study, and even for the future design, manufacture, and rational use of the UWB bio-radar, facilitating improvement in the practical performance and effectiveness of the UWB bio-radar search and rescue system.
1 Introduction
As a novel concept of radar, the bio-radar is a combination of bio-medical engineering technology and radar technology, which can detect and sense live body actions, such as physiological activities (respiration and heart beating) (Li et al., 2010; Li X. et al., 2013; Li Z. et al., 2013; Lv et al., 2014; Hsieh et al., 2015; Qi et al., 2016a; Wu et al., 2016) and other body movements (walking and waving) (Fioranelli et al., 2015; Kim and Moon, 2016a; Qi et al., 2016b; Nanzer, 2017; Qi et al., 2017; Qi et al., 2020) through some nonmetal obstacles (clothes, ruins, bricks, and treetops). As shown in the working principle diagram of bio-radar in Figure 1A, the bio-radar emits electromagnetic waves to irradiate the moving human body, and the aforementioned micro-motion will generate a specific phase and amplitude modulation on the electromagnetic waves. By demodulating the radar echo, the energy and frequency information on micro-motion can be obtained. The ultra-wideband (UWB) bio-radar could also obtain the position information on the target due to its high distance resolution, and superior penetration ability and stability; hence, it is widely adopted in various penetrating detection scenarios, urban anti-terrorism, clinical vital sign monitoring, and family health monitoring, especially post-disaster search and rescue, as shown in Figure 1B.
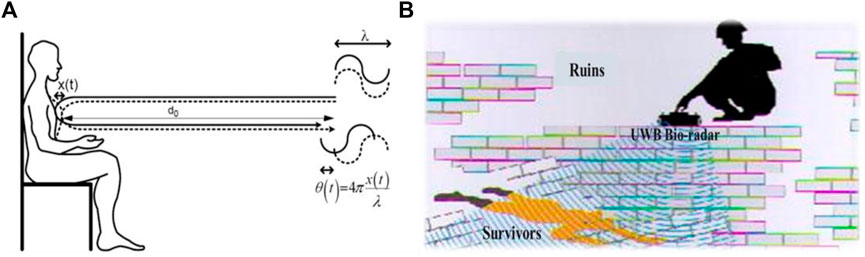
FIGURE 1. (A) Principle diagram of the bio-radar. (B) Search and rescue detection of survivors using the UWB bio-radar.
Most existing studies of the UWB bio-radar were oriented in laboratory conditions or mainly focus on some scientific problems, such as relevant information on vital sign or micro-motion detection for human beings, target positioning and recognition (Deming et al., 2009), and behavior detection (Kim and Moon, 2016b; Qi et al., 2016b; Qi et al., 2019). However, few efforts were allocated to discuss and solve relevant problems affecting the environmental during practical application, such as electromagnetic cluster and movement of leaves and grass at a search and rescue site outdoors, which will seriously curb the bio-radar in reaching its best performance. For instance, during some post-earthquake search–rescue operations in China (like the 2008 Wenchuan Earthquake, 2010 Yushu Earthquake, and 2014 Ludian Earthquake, using the “SJ-3000” and “SJ-6000” UWB bio-radar developed by our group), practical rescue experience and feedback implicate that these on-site environmental interferences (EIs) would significantly affect the detection performance of the instruments. Therefore, conducting a clear exploration of the influencing characteristics and rules of these environmental factors on detecting performance of the bio-radar could provide transcendental knowledge and guidance for special EI suppression study, and even for the future design, manufacture, and rational use of the UWB bio-radar.
EI on the bio-radar in practical application scenarios mainly comes from two aspects. The first kind of interference involves the surrounding movement interference (SMI) in the site environment, such as grass swayed by wind and UWB radar operator movements. The introduced movement interference of the operator could be avoided through training and ruling the operator, but the most common wind-swaying-grass interference is unavoidable, which thus becomes the main source of SMI. Additionally, there are also a variety of sources of electromagnetic interference from the rescue environment, such as mobile phones, global positioning system (GPS) signals, and radios, which become another kind of interference affecting the performance of the UWB radar. Fortunately, most of the interference bands are lower than the radar operating frequency band, so there would be no substantial impact on radar detection performance. Specifically, for the UWB bio-radar, according to long-term search–rescue operation experience and feedback from Wenchuan and Yushu earthquake post-disaster search–rescue work in China, we found that the main electromagnetic interference is narrowband RFI. It mainly comes from the wireless walkie–talkie equipped with a large number of search and rescue organizations operating at different frequency bands for different application types (409∼410 MHz for civil use, 400∼420 MHz for military use, and 350∼390 MHz for economical use). These frequency bands all overlap with the relatively low operating central frequency (lower than 500 MHz in order to guarantee its excellent penetrating detection ability) of most UWB search–rescue radars (such as the “LifeLocator” of the United States with 270 MHz and “SJ-3000” of China with 400/500 MHz).
Currently, some preliminary and general studies mainly focus on RFI and SMI suppression for radar detection, such as proposing frequency-domain filters, median filtering to conduct radio frequency interference (RFI) suppression (Zhao et al., 2013), applying cross-correlation operation on the echoes of the double L-frequency band antennas (Chen et al., 2000), establishing a double continuous wave (CW) radar system with minimum mean square error adaptive filtering (Zhang et al., 2010) to suppress the SMI for the CW radar, and using a singular value decomposition (SVD) method to suppress the SMI introduced by engineering vehicles (Xu et al., 2006). However, almost all these studies consider all kinds of EIs as a whole, instead of analyzing type differences of EIs. Moreover, few studies aim at studying the UWB radar detection performance in special post-disaster search–rescue environments, let alone investigating the influencing effect and characteristics caused by the spatial difference and category difference of interference.
In this paper, we attempt to explore the influencing characteristics and laws of SMI and RFI on the UWB bio-radar based on large amounts of actual experiments. Moreover, we will quantify the influencing characteristics for the UWB bio-radar over two key spatial interference parameters (angle and distance) and two different interferences based on the proposed evaluation criteria, aiming to provide transcendental knowledge and guidance for special environmental interference suppression study, and even for the future design, manufacture, and rational use of the UWB bio-radar, which is a promising aspect to greatly improve the practical performance and effectiveness of a UWB bio-radar search and rescue system.
The rest of this paper is organized as follows: Section 2 introduces the UWB bio-radar experiment system and corresponding data collection; the data preprocessing and interference evaluation methods are introduced in Section 3; Section 4 explores the influencing characteristics and laws of two interferences on the detection performance of the UWB bio-radar, according to corresponding experiment results. Finally, Section 5 concludes the paper.
2 Experimental platform establishment and data collection
This study established a UWB bio-radar detection experiment system and human respiratory simulation system based on the precision linear module, which is exploited to conduct quantitative detection experiments with distinct and controllable surrounding interference.
2.1 UWB bio-radar detection system
The impulse radio UWB (IR-UWB) bio-radar adopted in this study is developed by our group. Its key parameters are shown in Table 1. Its operating center frequency Fc and bandwidth (BW) are both Fc=400 MHz and BW=400 MHz, respectively, so it could guarantee both the ability of penetrating detection and distance resolution. The sampling number Ns=2,048 in the range dimension and frequency fs =64 Hz in the time dimension are sufficient to grasp the simultaneous change of respiration movement. For the IR-UWB radar, its main lobe width is 60°, and it adopts the butterfly antenna with vertical polarization. The detecting time window can be used to determine the effective detecting range scope. For instance, when the upper and lower edges of the detecting time window are set to 40 ns (6 m) and 20 ns (3 m), respectively, the effective UWB radar detection distance ranges from 3–6 m. The experimental scenario is shown in Figure 2.
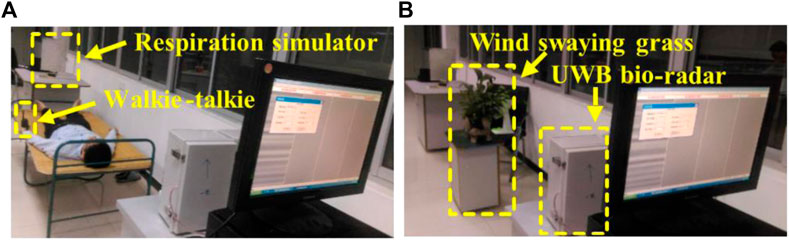
FIGURE 2. UWB bio-radar respiration detection experiment layouts with different interferences. (A) Narrowband RFI (walkie–talkie) and (B) SMI (wind-swaying grass).
2.2 Human respiratory simulation system
In previous studies, volunteers were commonly used as the source of respiratory signals. However, different volunteers will generate different respiration rates and displacements of the chest and abdomen. Even for the same volunteer, posture change, breathing state difference, and human body status change in different experimental scenarios also cause different effects on radar waves. Thus, during this quantitative interference investigation experiment, we developed a human respiratory simulation system based on the precision linear module to generate controllable, quantitative, and identical human respiratory simulation signals, which could exclude the influence of random differences from volunteers on the experiments.
As shown in Figure 2A, a precise human respiration simulator based on a linear guide rail (LGR) could simulate the required regular motion signals by driving the beacon performing reciprocating motion, according to the requirement of a certain amplitude and frequency. With a high load capacity and high-power brushless direct current (DC) motor, the human respiratory simulation system could support a series of quantitative settings of the moving speed and displacement, with an accuracy of speed over 0.1 mm/m, accuracy of displacement greater than 0.1 mm, and displacement scope of 0.1–300 mm. Moreover, 30 cm*40 cm*1 mm (maybe 1 mm more suitable) aluminum is used as the beacon placed in the breathing simulator. It is because the human respiratory backscattering area characteristic curve is most similar to that of aluminum. Additionally, chest and abdomen movement caused by human respiratory motion is approximately 30 cm*40 cm.
2.3 Collection of UWB radar human respiration signals with distinct interferences
In order to collect the two kinds of UWB radar human respiration signals with distinct interferences, as shown in Figure 2, we introduced the representative interference of wind-swaying grass and RFI interference of a wireless walkie–talkie during the respiration detection experiments. There are two key parameters, where ϴ represents the angle between the respiratory simulator and the source of interference, and R represents the distance from the radar to the interference source. The effective detection range determined by the selected time window parameters of the bio-radar is 3–6 m, so we placed the respiratory simulator at the middle position of 4 m (≈26.7 ns for the radar) directing to the radar. During the experiments, the respiratory simulator conducts reciprocating motion with a speed of 2.5 mm/s and a frequency of 0.2 Hz to simulate the respiration movement, which is generally consistent with the characteristics of normal human respiratory thoracic movement.
For the narrowband RFI experiments, as shown in Figure 2A, the BF-6600 multifunctional frequency-modulated (FM) walkie–talkie handled to control the signal transmission by a volunteer lying steadily is applied to introduce the narrowband RFI into the UWB bio-radar respiration signal. Its operating frequency is 400–470 MHz, overlapping the frequency band of the UWB bio-radar, and its duration time is 10 s per set of data. We know that the transmitter of the walkie–talkie would generate the main narrowband RFI when transmitting information, so only the transmitter would be considered the investigated object in the following study, and the receiver would be placed in an unchanged position. The collection time per data is 1 min, and the volunteer controls the walkie–talkie to transmit the interference during 25–40 s. Moreover, for the SMI, as shown in Figure 2B, we used the electric fan (three-level wind and freely rotating mode) blowing the plant to generate the interference during the whole 1-min collection time. The Ethics Committee of the Fourth Military Medical University approved the study, and all the subjects authorized the data release.
Moreover, for both kinds of interferences, the angle and distance between the detecting target and the interference source are two considerable factors when investigating the influencing characteristics and laws of interference. According to the layout of the experimental site, the location distributions of interference sources are as shown in Figure 3, and every kind of interference experiments at every position are repeated 10 times for further analysis and statistics. Specifically, the experiment was not carried out at four locations due to indoor experimental venue size limitations. Meanwhile, the available locations are sufficient to reveal the influence rules of the two kinds of interference.
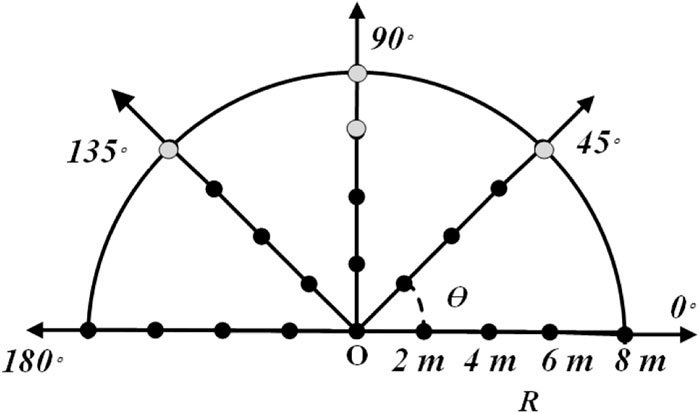
FIGURE 3. Position distribution of the interference source (black solid points represent the effective experiment position).
3 Data processing and interference evaluation
After acquiring the UWB radar respiration echo under interference at all positions, as shown in Figure 3 (
3.1 Preprocessing of the UWB bio-radar echo
In order to analyze the characteristics of the respiratory response from the radar echo better, it is necessary to pre-process the original radar echo to eliminate clutter and noise.
For human movement detection using the UWB radar, different echo delays from movements at different distance points could be acquired. After being amplified and sampled, these echoes would be stored in a two-dimensional matrix
where m and n represent the index of range and time, respectively.
In order to preliminarily eliminate the noise and clutter, pre-processing for the UWB echo mainly includes four steps, as shown in Figure 4:
Step 1: Downsampling in range dimension. The sampling operation is conducted on the 2-D matrix
Step 2: Background removal. The sliding window mean subtraction in the time dimension is exploited to remove the background. In this study, sliding windows are set as 100 points, according to numerous experiment results with an adjusted window length.
Step 3: Adaptive enhancement. A 128-order adaptive filtering algorithm based on the least mean square (LMS) is used to achieve data enhancement.
Step 4: Low-pass filtering. The 121-order finite impulse response (FIR) low-pass filter is applied to remove high-frequency noise and clutter. The cutoff frequency is set to 0.5 Hz, due to the motion frequency of the breathing simulator being 0.2 Hz.
Taking the UWB radar respiration signal with wind-swaying grass interference (
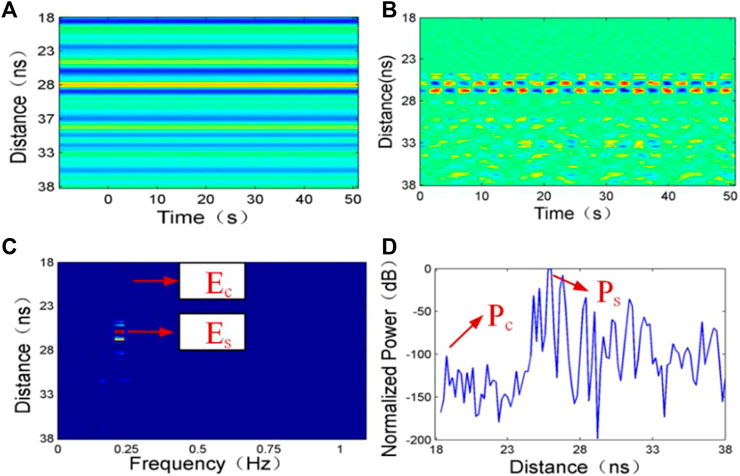
FIGURE 5. UWB radar respiration signal with wind-swaying grass interference (
Moreover, the results will be completely different if there is no environmental interference (motion interference and electromagnetic interference) and radar system noise. For the radar time–distance echo and frequency–distance matrix, there would be only clear respiratory response that persists over time at a distance of 26 ns, and no noise and clutter at any distance positions. Correspondingly, only a strong peak at the target distance position would be observed in the distance–power spectrum, while the surrounding power values remain stable and low.
3.2 Quantitative interference evaluation criteria of SNCR
This paper proposed reliable criteria of SNCR to quantitatively evaluate the influence of different interferences at different spatial locations on the UWB radar respiration response.
Still taking the respiration signal with the wind-swaying grass interference, for example, range–frequency matrices given in Figure 5B are obtained by performing fast Fourier transform (FFT) in the time dimension. Energy peaks obviously appear in these matrices, as shown in Figure 5C. We can observe that the energy peak lies at the position of 26 ns and 0.2 Hz, which is consistent with the position and moving frequency of the respiration simulator, respectively. The energy peak is defined as
4 Results and discussion
4.1 Investigation of the narrowband RFI effect
The RFI experiments were performed at all the interference positions given in Figure 3. All the UWB radar signals were analyzed by the proposed method and categorized to summarize the influencing characteristics and laws of RFI. Some representative experiments of two scenarios at typical positions were conducted and are discussed as follows. For the first scenario, the angle
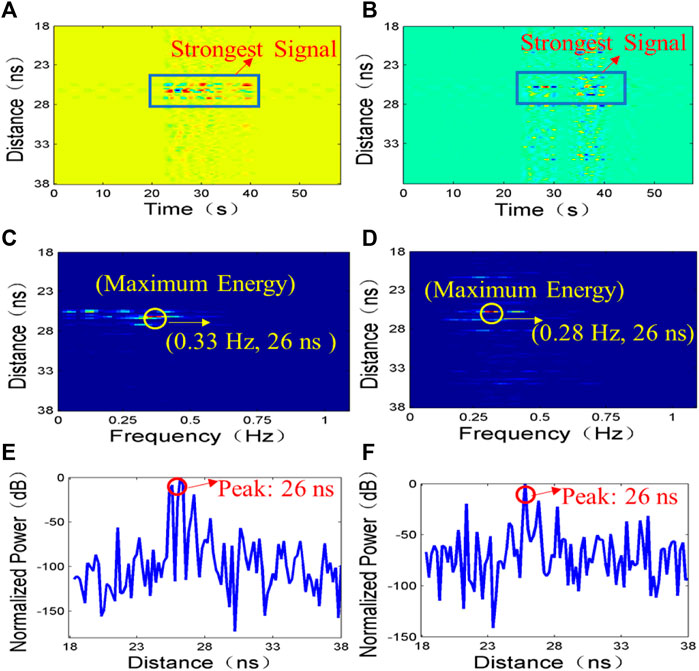
FIGURE 6. Experiment results of the UWB radar respiration signal with narrowband RFI (
For the experimental results (
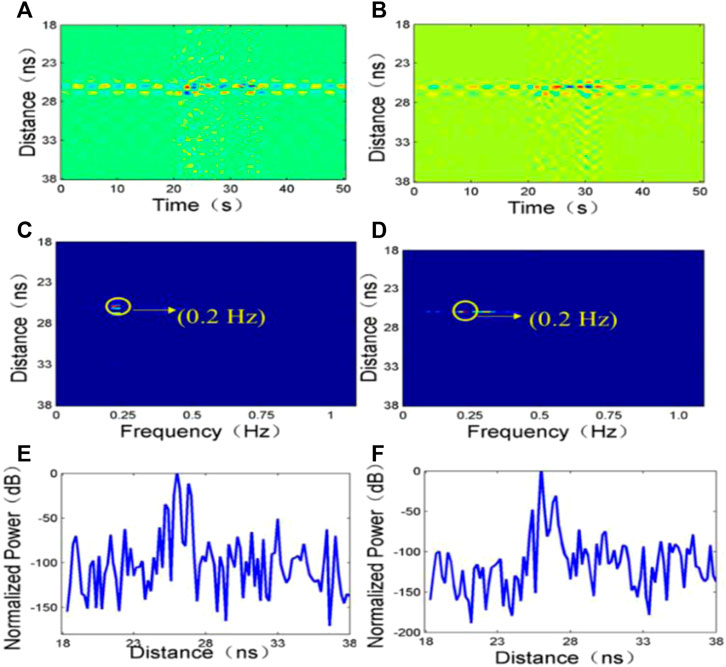
FIGURE 7. Experiment results of the UWB radar respiration signal with narrowband RFI (
For the second scenario,
According to the experimental results (
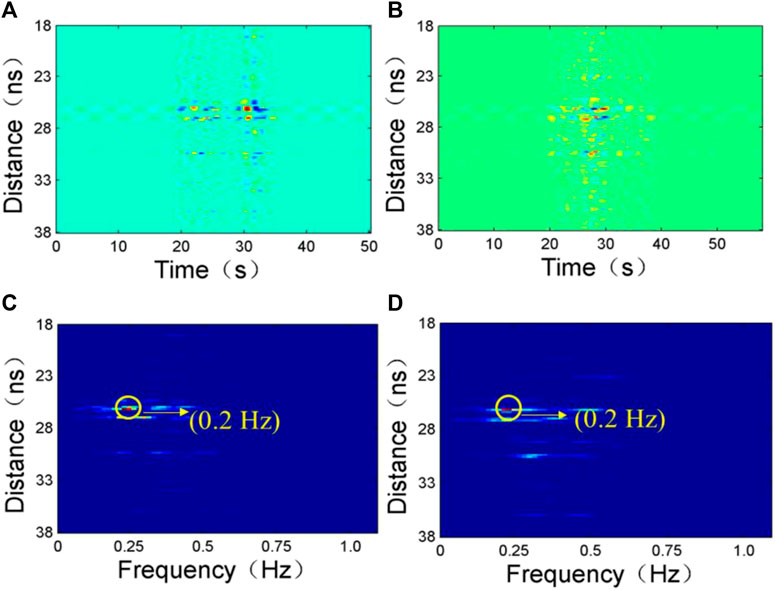
FIGURE 8. Experiment results of the UWB radar respiration signal with narrowband RFI (
All groups of data with narrowband RFI described in Figure 3 were processed according to the aforementioned process. The final average SNCR of 10 repeats of experiments at each specific interference position is applied to evaluate its specific impact. A variable-controlling approach was used to analyze and discuss the influencing characteristics of narrowband RFI over the two key parameters, namely, angle and distance.
According to the experimental results shown in Figure 9, we can observe that 1) RFI would influence and decrease the SNCR of the radar respiration signal significantly within a close distance (R < 2 m) so that the respiration energy peaks cannot be found and even lead to misjudgments; 2) the RFI influencing effect is highly correlated with the interference distance. For all the interference angle curves shown in Figure 9A, the SNCR values exhibit an increasing trend with increasing distance; 3) as shown in Figure 9B, for a fixed interference distance, the angle change usually leads to little interference difference (except an extreme case of
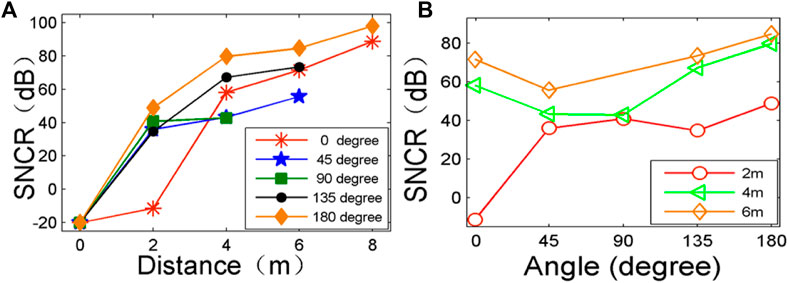
FIGURE 9. SNCR curves of the UWB bio-radar respiration signal with narrowband RFI at different interference positions: (A) fixed distance R and changeable angle θ, and (B) fixed angle θ and changeable distance R.
4.2 Investigation of the surrounding movement interference effect
Similarly, the same method and operation were applied to investigate the influencing characteristics of SMI. Some representative experiments of two scenarios at typical positions are discussed as follows:
For the first scenario of fixed angle θ and changeable distance R, according to the experimental results (
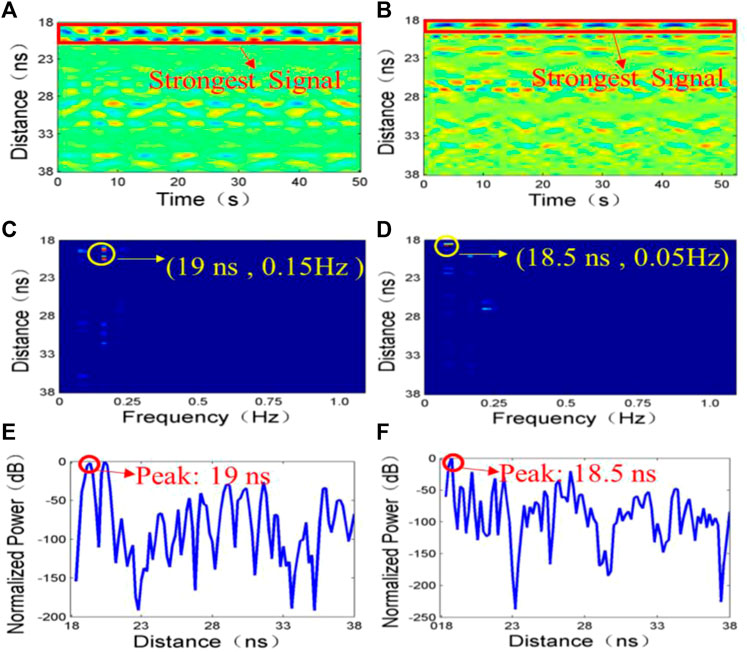
FIGURE 10. Experiment results of the UWB radar respiration signal with SMI (
With R increasing to 4 and 6 m, obvious respiration response, reasonable location feature, and frequency feature of energy peaks can be observed and detected from corresponding radar echoes and D-F matrices, as shown in Figure 11. The final SNCRs generated from the D-P spectrum are 35.63 and 102 dB, implying that the interference influenced the detection performance to some extent under these conditions but did not lead to detection mistakes.
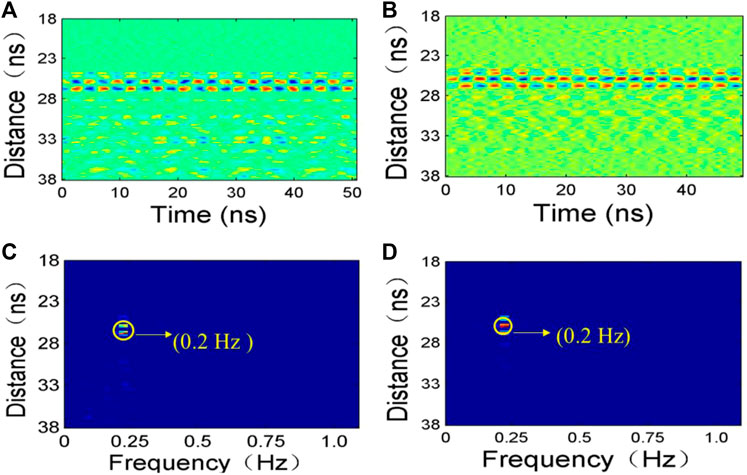
FIGURE 11. Experiment results of the UWB radar respiration signal with SMI (
For the second scenario of fixed R and changeable θ of SMI, according to the experiment results (
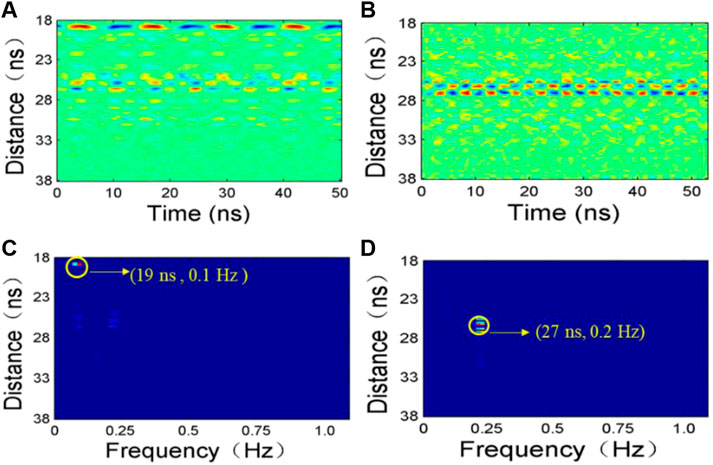
FIGURE 12. Experiment results of the UWB radar respiration signal with SMI (
All groups of data with SMI (wind-swaying grass) were processed based on the same process, and the SNCRs were also analyzed and compared, as shown in Figure 13. Statistical results of SNCRs suggested that 1) the wind-swaying grass interference only generates serious influence on UWB radar respiration within a close distance (<2 m), and it will lead to detection mistakes with negative SNCRs, as shown in Figure 13A; 2) within the range of the radar main lobe, the influencing strength of the wind-swaying grass interference on UWB radar detection is highly related to the interference distance in the detection window. As shown by the curves of
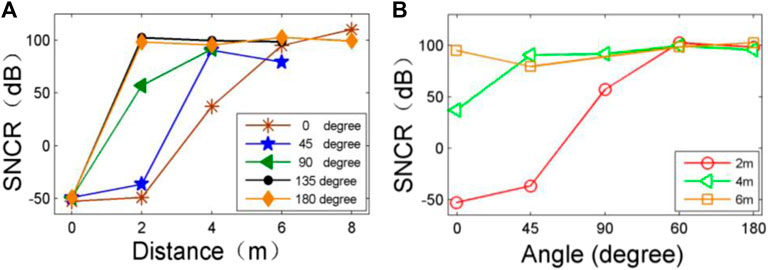
FIGURE 13. SNCR curves of the UWB bio-radar respiration signal with SMI at different interference positions: (A) fixed distance R and changeable angle θ, and (B) fixed angle θ and changeable distance R.
Additionally, although there are also some other electromagnetic interferences in the rescue environment, such as mobile phones, GPS signals, and radios, they may not cause serious interference to this special penetrating detection-oriented bio-radar system characterized by low operating center-frequency (<500 MHz). For the commonly used 3 G and 4 G communication, their frequency mainly centered surrounding 2.1 GHz. The operating frequency of a GPS signal ranges from 1,230 to 1,570 MHz, and the civil radio frequency usually ranges from 70 to 110 MHz. Obviously, all these frequency ranges did not overlap with the bio-radar working frequency band and thus will not lead to serious interference theoretically. Therefore, these kinds of interferences were not considered in the study, and additional investigating experiments were not developed. Even with some influence, their influencing strength would be much weaker than that of the narrowband RFI due to their working signal power.
5 Conclusion
This study attempts to quantitatively investigate the influencing characteristics and laws of two main existing EIs with human respiration detection using the UWB bio-radar in some post-disaster search and rescue sites.
First, this paper established a quantitative and controllable experimental evaluation platform system. It consists of a UWB bio-radar detection experiment system, different interference simulation systems, and the human respiratory simulation system based on the precision linear module. Then, reasonable evaluation criteria of SNCR were especially proposed to analyze and quantify the interference influence level. Based on the aforementioned experimental platform and signal processing method, numerous experiments were performed under different and well-designed experimental layouts. All experiment results were analyzed and summarized, focusing on investigating interference influence characteristics.
For the narrowband RFI, numerous experiment results show that the SNCR of the UWB radar respiration signal within a close distance (R < 2 m) is seriously decreased due to RFI and even leads to misjudgments. However, with the interference distance increasing, the influence effect decreases gradually. Statistical results demonstrated that there is no strong correlation between the interference strength and interference angle. RFI at any specific interference angle exhibits almost the same influence effect on respiration detection as long as the interference is located within the same interference distance, no matter whether it was located in the main lobe or side lobe of the radar. Contrarily, RFI is highly related to the interference distance, and the influencing strength attenuates gradually (SNCR increases) with the interference distance increasing, even when the interference sources have been moved outside the detecting time window. It indicates that the function of the time window of the UWB radar is ineffective to the RFI so that the interference influence cannot be screened or isolated.
The influencing effect of SMI exhibits some distinct characteristics compared with those of RFI. SMI is affected by the main lobe width and the detecting time window of the UWB radar, although the influencing effect is similar within a close interference distance (R < 2 m). Contrary to RFI, the influencing strength of SMI is highly related to the interference angle. The influencing effect decreases and SNCRs increase with the interference angle increasing at any specific interference distance. Once the interference sources were located in the side lobe field at a little far distance (>2 m), it caused almost no influence. Additionally, similar to RFI, the SMI has a strong relation with the interference distance within the detecting time window in the main radar lobe, while the interference attenuates sharply outside the time widow.
In the future, based on these characteristic research results of interference, we will further study corresponding targeted interference suppression technology and conduct the strategy to reduce the interference influence during production, design, and use of the UWB bio-radar, aiming to improving the practical performance and effectiveness of the UWB bio-radar search and rescue system.
Data availability statement
The raw data supporting the conclusion of this article will be made available by the authors, without undue reservation.
Ethics statement
Ethical review and approval was not required for the study on human participants in accordance with the local legislation and institutional requirements. The patients/participants provided their written informed consent to participate in this study.
Author contributions
Conceptualization: HL and JW; methodology: TM and FQ; formal analysis: XY; investigation: TJ; data curation: XY; writing—original draft preparation: FQ; writing—review and editing: FQ; supervision: HL and JW; funding acquisition: JW. All authors contributed to the article and approved the submitted version.
Funding
This work was supported by the National Key R&D Program of China (2021YFC1200104), the National Natural Science Foundation of China (Grant No. 62201578), and the Key Research and Development Program of Shaanxi (grant no. 2022SF-482).
Conflict of interest
The authors declare that the research was conducted in the absence of any commercial or financial relationships that could be construed as a potential conflict of interest.
Publisher’s note
All claims expressed in this article are solely those of the authors and do not necessarily represent those of their affiliated organizations, or those of the publisher, the editors, and the reviewers. Any product that may be evaluated in this article, or claim that may be made by its manufacturer, is not guaranteed or endorsed by the publisher.
References
Chen, K. M., Huang, Y., Zhang, J., and Norman, A. (2000). Microwave life-detection systems for searching human subjects under earthquake rubble or behind barrier. IEEE Trans. bio-medical Eng. 47, 105–114. doi:10.1109/10.817625
Deming, R., Schindler, J., and Perlovsky, L. (2009). Multi-target/multi-sensor tracking using only range and Doppler measurements. IEEE Trans. Aerosp. Electron. Syst. 45, 593–611. doi:10.1109/taes.2009.5089543
Fioranelli, F., Ritchie, M., and Griffiths, H. (2015). Classification of unarmed/armed personnel using the NetRAD multistatic radar for micro-Doppler and singular value decomposition features. IEEE Geoscience Remote Sens. Lett. 12, 1933–1937. doi:10.1109/lgrs.2015.2439393
Hsieh, C., Chiu, Y., Shen, Y., Chu, T., and Huang, Y. (2015). A UWB radar signal processing platform for real-time human respiratory feature extraction based on four-segment linear waveform model. IEEE Trans. Biomed. circuits Syst. 10, 219–230. doi:10.1109/tbcas.2014.2376956
Kim, Y., and Moon, T. (2016a). Human detection and activity classification based on micro-Doppler signatures using deep convolutional neural networks. Hum. Detect. Activity Classif. Based Micro-Doppler Signatures Using Deep Convolutional Neural Netw. 13, 8–12. doi:10.1109/lgrs.2015.2491329
Kim, Y., and Moon, T. (2016b). Human detection and activity classification based on micro-Doppler signatures using deep convolutional neural networks. IEEE geoscience remote Sens. Lett. 13, 8–12. doi:10.1109/lgrs.2015.2491329
Li, C., Ling, J., Li, J., and Lin, J. (2010). Accurate Doppler radar noncontact vital sign detection using the RELAX algorithm. IEEE Trans. Instrum. Meas. 59, 687–695. doi:10.1109/tim.2009.2025986
Li, X., Qiao, D., Li, Y., and Dai, H. (2013a). A novel through-wall respiration detection algorithm using UWB radar. Conf. Proc. IEEE Eng. Med. Biol. Soc. 2013, 1013–1016. doi:10.1109/EMBC.2013.6609675
Li, Z., Li, W., Lv, H., Zhang, Y., Jing, X., and Wang, J. (2013b). A novel method for respiration-like clutter cancellation in life detection by dual-frequency IR-UWB radar. IEEE Trans. Microw. Theory and Tech. 61, 2086–2092. doi:10.1109/tmtt.2013.2247054
Lv, H., Li, W., Li, Z., Zhang, Y., Jiao, T., Xue, H., et al. (2014). Characterization and identification of IR-UWB respiratory-motion response of trapped victims. IEEE Trans. Geoscience Remote Sens. 52, 7195–7204. doi:10.1109/tgrs.2014.2309141
Nanzer, J. A. (2017). A review of microwave wireless techniques for human presence detection and classification. IEEE Trans. Microw. Theory Tech. 65, 1780–1794. doi:10.1109/tmtt.2017.2650909
Qi, F., Li, C., Wang, S., Zhang, H., Wang, J., and Lu, G. (2016a). Contact-free detection of obstructive sleep apnea based on wavelet information entropy spectrum using bio-radar. Entropy 18, 306. doi:10.3390/e18080306
Qi, F., Liang, F., Liu, M., Lv, H., Wang, P., Xue, H., et al. (2019). Position-information-indexed classifier for improved through-wall detection and classification of human activities using UWB bio-radar. IEEE Antennas Wirel. Propag. Lett. 18, 437–441. doi:10.1109/lawp.2019.2893358
Qi, F., Liang, F., Lv, H., Li, C., Chen, F., and Wang, J. (2016b). Detection and classification of finer-grained human activities based on stepped-frequency continuous-wave through-wall radar. Sensors 16, 885. doi:10.3390/s16060885
Qi, F., Lv, H., Liang, F., Li, Z., Yu, X., and Wang, J. (2017). MHHT-based method for analysis of micro-Doppler signatures for human finer-grained activity using through-wall SFCW radar. Remote Sens. 9, 260. doi:10.3390/rs9030260
Qi, F., Lv, H., Wang, J., and Fathy, A. E. (2020). Quantitative evaluation of channel micro-Doppler capacity for MIMO UWB radar human activity signals based on time-frequency signatures. IEEE Trans. Geoscience Remote Sens. 58, 6138–6151. doi:10.1109/tgrs.2020.2974749
Wu, S., Yao, S., Liu, W., Tan, K., Xia, Z., Meng, S., et al. (2016). Study on a novel UWB linear array human respiration model and detection method. IEEE J. Sel. Top. Appl. Earth Observations Remote Sens. 9, 125–140. doi:10.1109/jstars.2016.2519760
Xu, S., Bai, Z., Yang, Q., and Kwak, K. S. (2006). “Singular value decomposition-based algorithm for IEEE 802.11a interference suppression in DS-UWB and TH-PAM UWB systems,” in Proceedings of the Vehicular technology conference, 2006 (Germany: Spring), May 2006 2378–2382.
Zhang, Y., Jiao, T., Jing, X., Xiao, Y. U., and Wang, J. (2010). Study on moving target interference suppression for life detecting radar. Newdelhi: Informatization Research.
Keywords: environmental interference, ultra-wideband bio-radar, respiration detection, interference distance, interference angle
Citation: Ma T, Qi F, Yu X, Jiao T, Lv H and Wang J (2023) Study on the environmental effect on human being detection using the UWB bio-radar. Front. Earth Sci. 11:1158764. doi: 10.3389/feart.2023.1158764
Received: 12 April 2023; Accepted: 21 June 2023;
Published: 03 July 2023.
Edited by:
Puhong Duan, Hunan University, ChinaReviewed by:
Mo Yan, Changsha University of Science and Technology, ChinaZhuojun Xie, Hunan University, China
Copyright © 2023 Ma, Qi, Yu, Jiao, Lv and Wang. This is an open-access article distributed under the terms of the Creative Commons Attribution License (CC BY). The use, distribution or reproduction in other forums is permitted, provided the original author(s) and the copyright owner(s) are credited and that the original publication in this journal is cited, in accordance with accepted academic practice. No use, distribution or reproduction is permitted which does not comply with these terms.
*Correspondence: Hao Lv, Zm1tdWx2aGFvQGZtbXUuZWR1LmNu; Jianqi Wang, d2FuZ2pxQGZtbXUuZWR1LmNu
†These authors have contributed equally to this work