- Guangdong Provincial Key Laboratory of Deep Earth Sciences and Geothermal Energy Exploitation and Utilization, Institute of Deep Earth Sciences and Green Energy, College of Civil and Transportation Engineering, Shenzhen University, Shenzhen, China
Geothermal energy is a kind of renewable energy with the characteristics of stability, zero carbon emissions, less land occupation, and localization. It has enormous development potential in the transition from traditional fossil energy to renewable energy, especially in Guangdong, geothermal resources are also abundant. However, the existing resource potential evaluation is relatively broad, and the uncertainty of the distribution of geothermal resources is not considered. Therefore, accurately and scientifically assessing the potential of geothermal resources is currently a research focus, Monte Carlo simulation is an ideal solution to quantitatively measure the distribution of geothermal resources through probability distributions. In this study, based on the volume method, considering the uncertainty of geothermal resource distribution parameters, Monte Carlo simulation was introduced, and the triangular distribution and uniform distribution model were used to simulate the input parameters of geothermal fields, and the potential of uplifted mountain geothermal resources in this area was evaluated. The results show that the fracture-banded reservoir geothermal resources are 5.648–5.867 × 1016 kJ (mean 5.743 × 1016 kJ), the karst-layered reservoir geothermal resources are 5.089–5.536 × 1015 kJ (mean 5.328 × 1015 kJ), finally the uplifted mountain geothermal resources potential of Guangdong are 6.176–6.399 × 1016 kJ (mean 6.275 × 1016 kJ). It quantitatively shows that the existing uplifted mountainous geothermal resources potential in Guangdong Province is enormous, the total amount of uplifted mountainous geothermal resources is equal to 2.11–2.18 × 105 Ten thousand tons of standard coal (mean 2.14 × 105 Ten thousand tons of standard coal).
1 Introduction
Geothermal energy is a kind of natural renewable energy from the inside of the Earth, mainly from the original heat accumulated when the planet was born and the decay of radioactive isotopes such as uranium, thorium, and potassium in the mantle and crust (Liu, et al., 2023). The heat and energy of the Earth’s interior are transported to the Earth’s surface by thermal convection in the mantle and core, which eventually drives the plate tectonics, continental drift, and major orogeny in geological timescale (i.e., over tens of million years), as well as volcanic eruptions and earthquakes in the timescale of human activities (Yoshida and Santosh, 2020). The Earth is a huge thermal reservoir, the inner core temperature at the deepest part of the Earth is about 7,000°C, it has been estimated that the total heat available within the upper 5 km of the Earth’s subsurface is about 140×106 EJ (Trumpy, et al., 2015). Driven by the temperature difference, heat flows continuously from the deep part of the Earth to the surface, and dissipates in the atmosphere and hydrosphere in the form of terrestrial heat flow, the total heat flow is huge, about 1.4×103 EJ yr-1 (Yoshida and Santosh, 2020). Therefore, geothermal energy has not only an enormous potential in the resources reserve, but has some unique superiority such as local energy, good stability, zero carbon emissions, and less land occupation (Alqahtani, et al., 2023b), compared with other renewable energy, like hydropower, solar power, wind energy, and bio-fuels.
The area of Guangdong is one of the most economically vigorous and most densely populous, as well as the most industrialized areas with high energy demand in China. The utilization of geothermal energy can help to decrease its dependence on conventional fossil fuel, reduce its greenhouse gas emissions and improve Guangdong’s energy transition towards China’s pledge to carbon peak by 2030 and carbon neutrality by 2060 (Zhong, et al., 2023). The whole province of Guangdong has over 300 hot springs with abundant medium and deep geothermal resources (Song, et al., 2005), which ranks third in the whole country, making it an ideal area for the exploitation of geothermal resources. It can also be found that the density of hot springs in Guangdong is an anomaly higher than other areas in China except for Yunnan and Tibet province, the fracture-banded reservoir geothermal resources are also plenteous as Figure 1. Shows. And there is sketchy geothermal resources evaluation that has been conducted in Guangdong, either as a part of the hot dry rock or geothermal resources evaluation of the whole of China (Wang, et al., 2012; Jiang, et al., 2016; Wang, et al., 2017) or only assessment of geothermal resources potential in the Guangdong-Hong Kong-Macao Greater Bay Area (Xie, et al., 2019a). Therefore, it is necessary to assess the potential of geothermal resources in Guangdong more accurately and comprehensively.
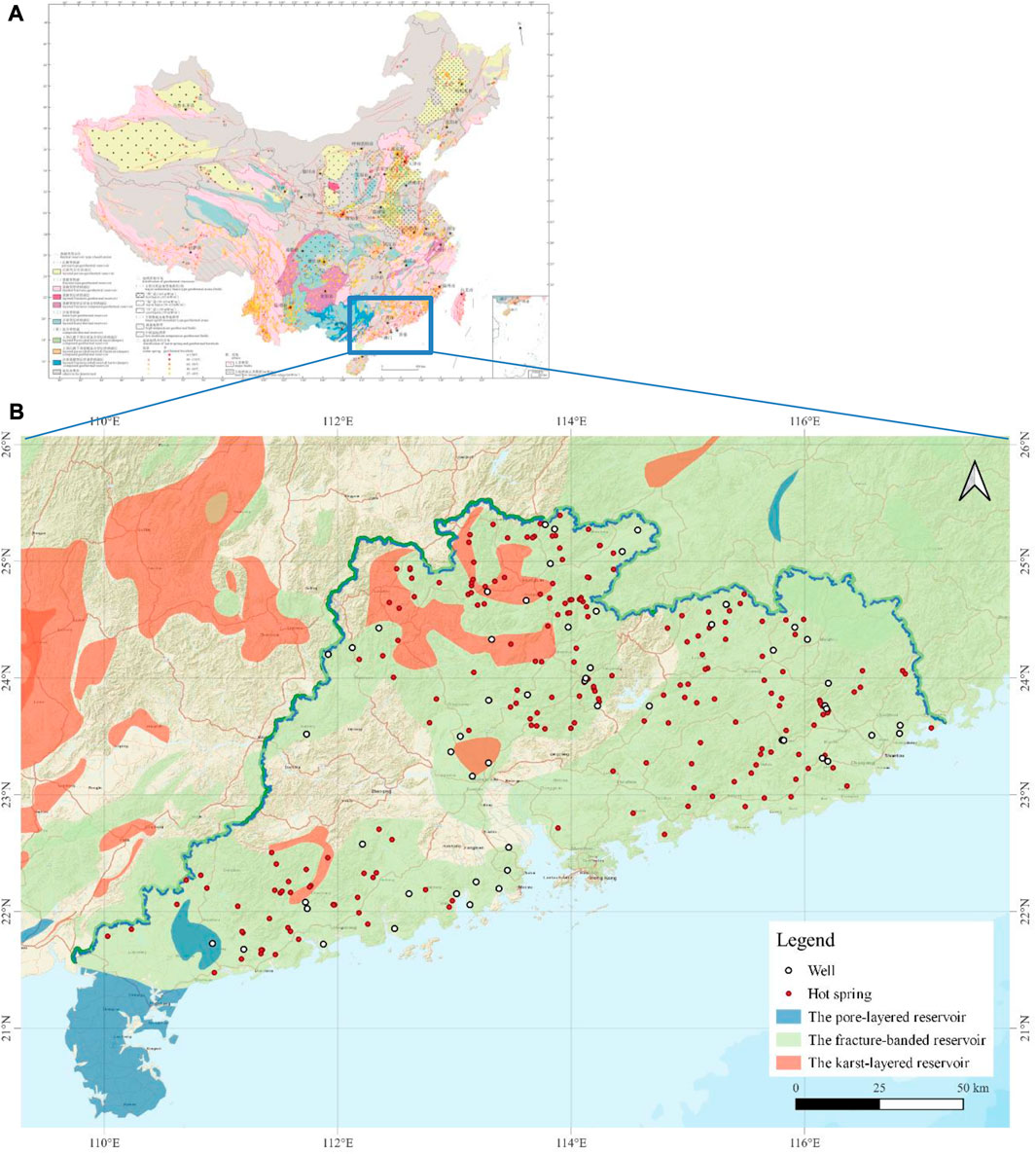
FIGURE 1. Distribution of geothermal resources in Guangdong province. (A) Distribution of geothermal resources in China (data from Wang et al. (2017)). (B) Distribution of geothermal resources in Guangdong province.
However, there are currently some primary issues with the assessment of geothermal resources potential using the volumetric method in Guangdong, 1) the high uncertainty and uneven distribution of uplifted mountain geothermal resources, 2) the limited availability of geological and geothermal information and data in geothermal resources, 3) the volumetric method disregards parameter uncertainties and instead assigns fixed values to thermal storage geometry and physical property parameters, above all make it difficult to an assessment of geothermal resources reserves (Xi, et al., 2018; Witter, et al., 2019; Whealton, et al., 2020; Yuan, et al., 2022). But Monte Carlo simulations can give better help to reduce the variability and uncertainty associated with geothermal resource reserves estimation (Aravena, et al., 2016b; Trota, et al., 2019; Wang et al., 2021). Monte Carlo simulation is a statistical method, that involves the generation of multiple simulations that reflect the variability and uncertainty in parameters such as rock density, rock porosity, reservoir temperature, area, and thickness, all of those parameters follow the probability distribution such as uniform distribution, triangular distribution, normal distribution and so on.
This study employs the Monte Carlo simulation method to explore the geothermal resources potential of Guangdong province. The study proceeds by introducing the geothermal geologic setting of the research area in Section 2. Followed by an outline of the various geothermal resources assessment methods, with an emphasis on analyzing the advantages and limitations of the volumetric method in Section 3, then describes how the Monte Carlo simulation was applied to uplifted mountain geothermal resources assessment. While Section 4 presents the data required for the simulation, including the probability distribution of density, specific heat capacity, and porosity of the geothermal reservoir, as well as the data of Guangdong geothermal fields. In Section 5, the evaluation results of the geothermal resources reserve based on Monte Carlo simulation are presented, with a breakdown of the fracture-banded reservoir and the karst-layered reservoir, and then the uplifted mountain geothermal resources reserve of Guangdong is summarized. At last, the conclusion of this study is concluded in Section 6.
2 Geothermal geologic setting
Guangdong province is located at the southern edge of the hills along the southeast coast of China, with high terrain in the north and low in the south, mainly medium and low hills, and plains along the coast of the Pearl River and coastline of the Pacific Ocean. The study area has a subtropical monsoon climate, with an annual average temperature of 22°C, an annual average rainfall of 2,300 mm, and a rainy season mainly from annual April to September. Most of discovered geothermal resources belong to hydrothermal type, all of which are low-medium temperature geothermal resources (Ying, et al., 2020). The classification of geothermal resources in the Guangdong province is based on the geomorphological structure characteristics and heat transfer mode and can be categorized into two types: uplifted mountain and sedimentary basin, among which uplifted mountain geothermal resources are widely distributed. Moreover, according to the characteristics of geothermal reservoirs, the geothermal reservoir types of Guangdong can be divided into three types: the pore-layered reservoir, the fracture-banded reservoir, and the karst-layered reservoir, and their distribution areas are shown in Figure 1.
2.1 Geological structure
Guangdong province is located at the intersection of the Eurasian Plate, the Pacific Plate, and the Philippine Plate. In the process of plate movement for a long time, the research area has been subducted by the Pacific plate, resulting in the sinking of the cold ocean lithospheric plate but the rise of hot mantle materials, forming a thermo-tectonic zone (Xie, et al., 2019b). The area has experienced many violent tectonic movements and multi-stage magmatic invasions, such as the Indosinian (late Permian–middle Triassic) orogeny and the Yanshanian (Jurassic–Cretaceous) tectonic-magmatic events (Xi, et al., 2018), resulting in secondary faults or rock mass fragmentation and tensile joint fissures in and near the fault zone, which provides space and channels for the storage and migration of geothermal fluids. At the same time, the tectonic activity of deep and large faults not only promotes the formation of thermal storage space, but also communicates the spatial connection between deep geothermal fluid and shallow thermal storage, and becomes an important heat transfer channel in geothermal fields.
Figure 2 can indicate that the distribution of geothermal fields (hot springs) in Guangdong province is mainly controlled by the deep and large faults structure in the NNE-trending and the NW-trending faults. The deep and large NNE-trending faults provide the heat source that mainly comes from the mantle, and the NW-trending fault provides the drainage conditions for the upwelling of hot springs (Zheng, et al., 2021). We can also find that most of the geothermal fields are distributed in bands along deep fault zones in Figure 2, mainly exposed in and around the deep fault axis and at the intersection of faults, while the rest are mostly distributed between various deep and large faults.
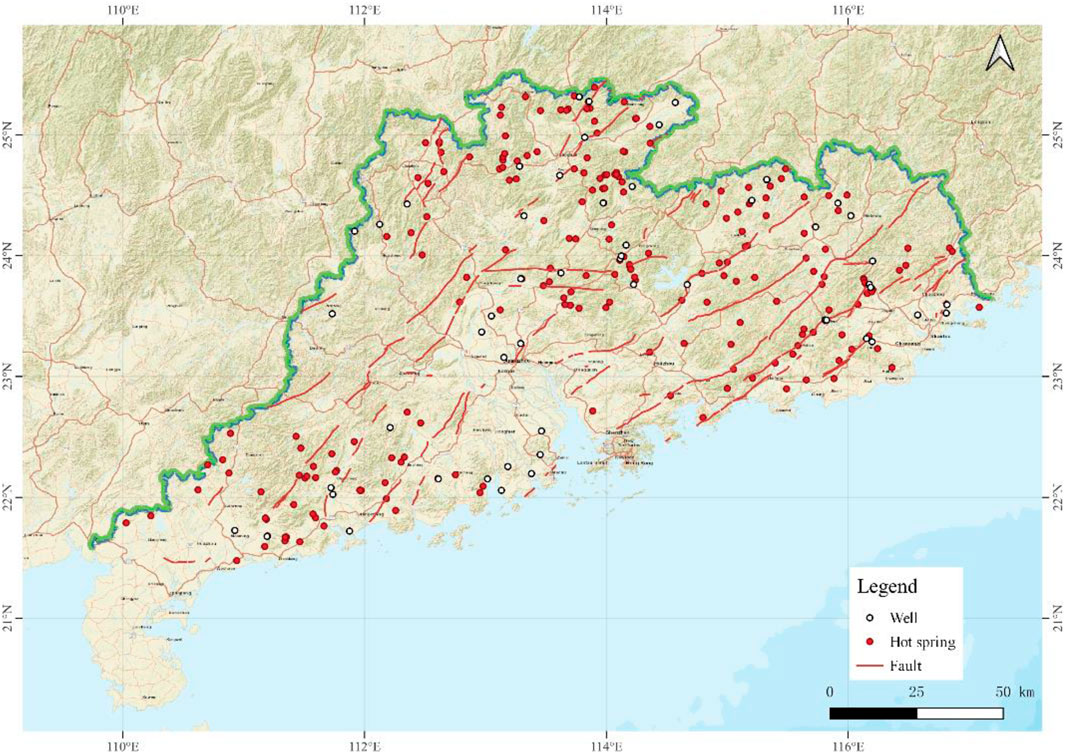
FIGURE 2. Distribution of main faults in Guangdong province (modified after (Wang, et al., 2020)).
In the long period of geological history, the distribution of geothermal fields is not only related to the fragmentation of the original rock mass and the fracture of joints in the contact zone caused by the multi-stage intrusion of magma or the influence of deep and large faults structure but also the geological age of the intrusive rock. Many strong tectonic and magmatic activities occurred in the study area, and the intrusive rock was widely distributed (Figure 3), with acid granite in the Yanshanian period being the most developed (Wang and Shen, 2003). It can also be found that the distribution of geothermal fields is closely related to magma intrusion activity, most of the geothermal fields in the study area are distributed in the outcropping magmatic mass, near late-intrusive dikes or in the contact zone between the rock mass and the surrounding rock.
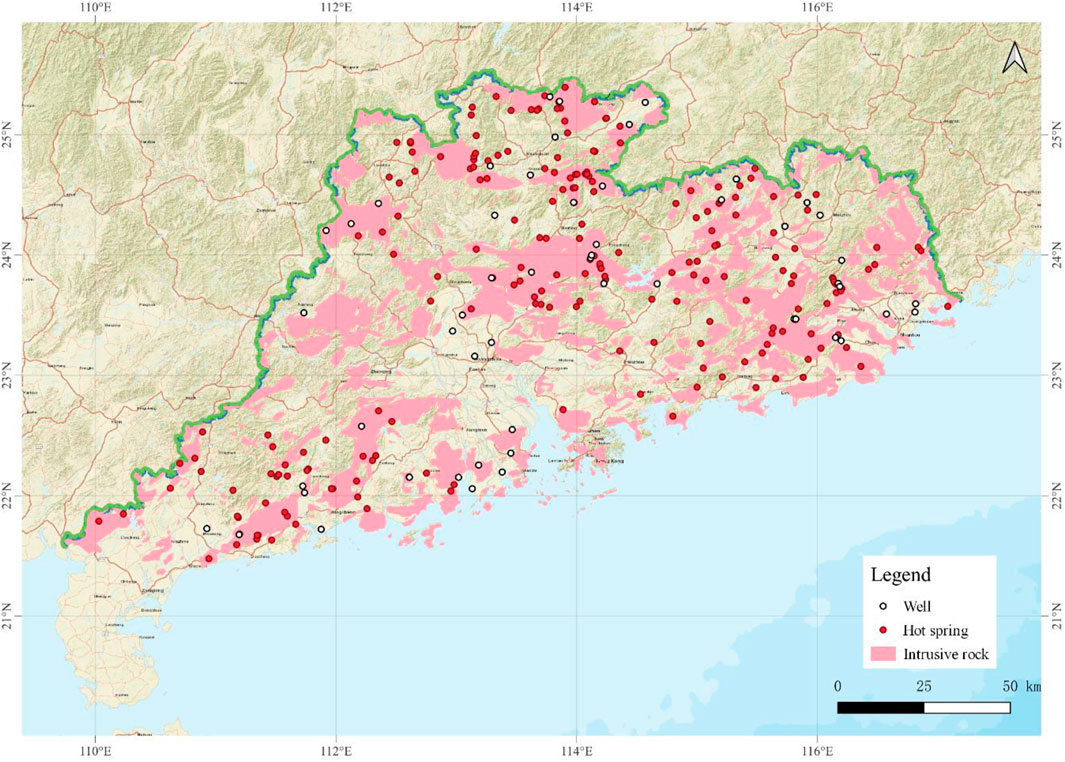
FIGURE 3. Distribution of intrusive rock in Guangdong province (data source: Geological map of Guangdong Province, Hong Kong, and Macao Special Administrative Regions).
2.2 Geothermal background
Terrestrial heat flow is the most direct display of the Earth’s internal heat on the Earth’s surface, which contains a wealth of geological, geophysical, and geodynamic information, and its value is proportional to the geothermal gradient and the thermal conductivity of rocks (Alqahtani, et al., 2023a). It is a key parameter to evaluate the potential of geothermal resources, the southeastern coast, especially in Guangdong province (Figure 4), has a very high geothermal flow background, with an average value of more than 70 mW m-2 (Jiang, et al., 2019). Terrestrial heat flow shows the characteristics of high middle and low north and south. Due to the large number of intrusive rocks distributed along the area of Guangdong, mainly granite in the Yanshanian period, because granite rocks are highly radioactive and their heat generation rate is also high, which provides a direct heat source for crustal heat generation (WENJING, et al., 2016). This also provides an abundant heat source for the geothermal resources background in Guangdong province.
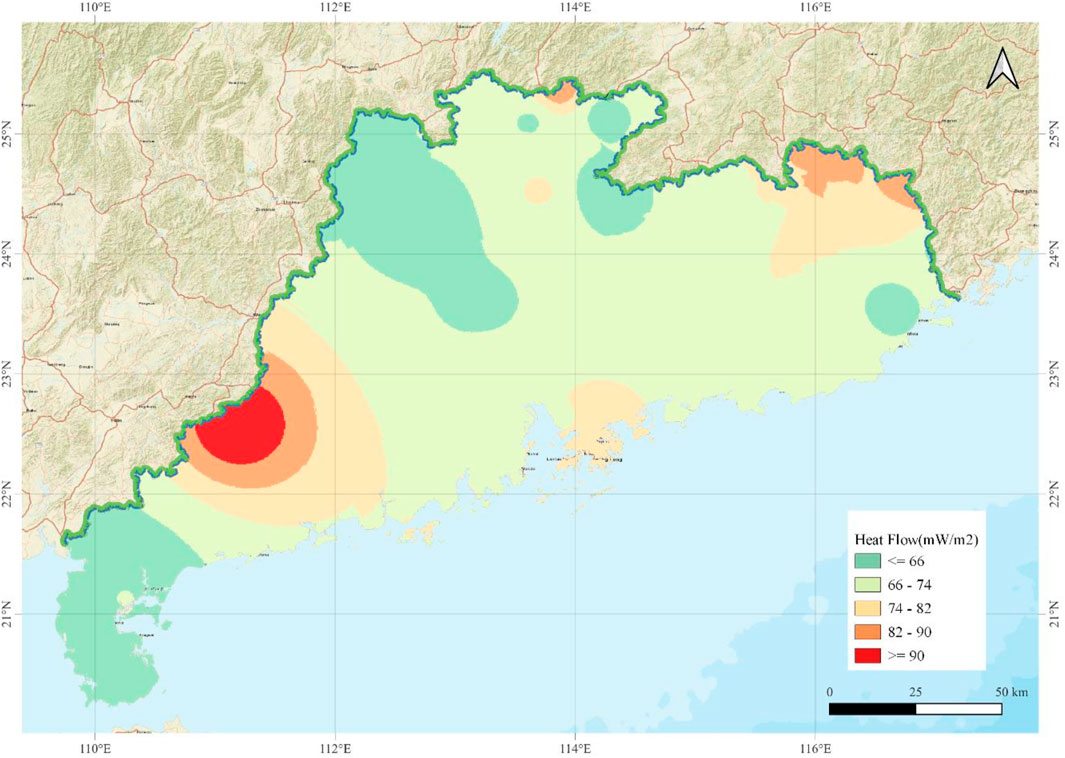
FIGURE 4. Distribution of heat flow in Guangdong province (data source: The Global Geothermal Flow Database recently updated by the International Heat Flow Commission (Fuchs, et al., 2021)).
The geothermal systems of uplifted mountain geothermal resources in Guangdong province are hydrothermal type, which is mainly distributed in and near deep and large fault zones or in the contact zone between magmatic rock mass and surrounding rock. The geothermal system of uplifted mountain geothermal resources has obvious characteristics of fault control, which is closely related to the distribution of granite in the Yanshanian period. The formation mechanism of uplifted mountain geothermal resources in Guangdong province is that atmospheric precipitation, surface water body, or normal temperature groundwater infiltrates into bedrock fractures, and is heated or evaporated by a hot rock during the deep cycle, and it rises and exposes at appropriate locations (such as fault fractures connecting the surface and deep areas) or is revealed by human beings, as shown in Figure 5.
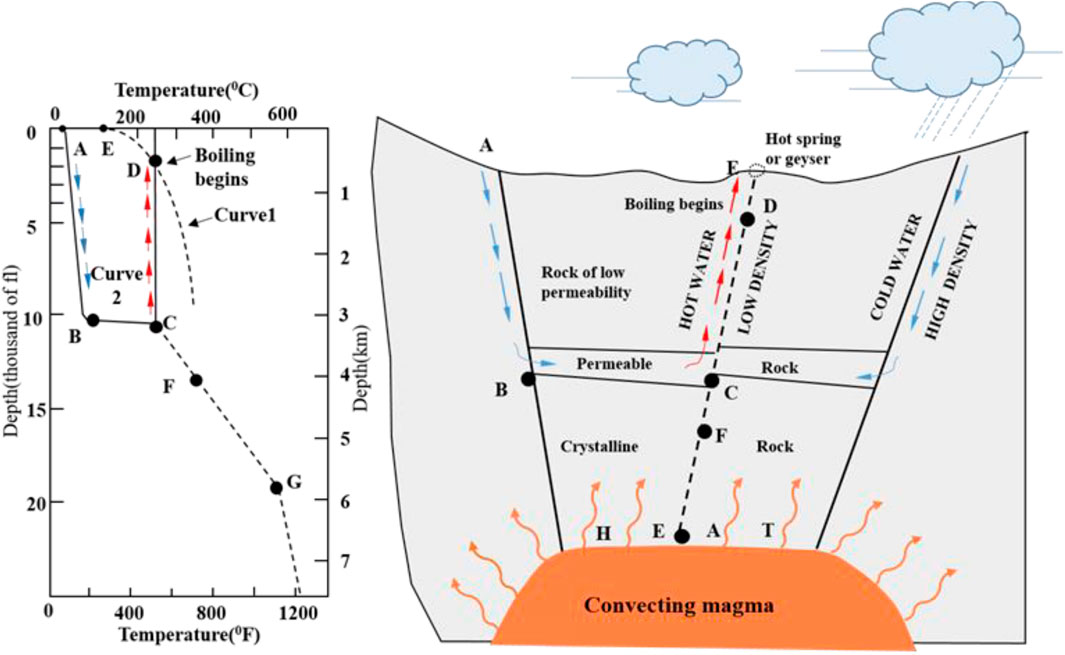
FIGURE 5. The formation schematic diagrams of uplifted mountain geothermal resources (modified after White (White, 1968)).
3 Methodology
Geothermal resources evaluation is one of the earlier tasks of geothermal projects in evaluating the potential of geothermal resources and plays an important role in the decision-making, financing, development, and operation of geothermal resources development and utilization in the next stage. In this investigation, the volume method and Monte Carlo simulation are combined to evaluate the potential of the geothermal resource in Guangdong.
3.1 Evaluation method
Evaluation of geothermal resources has developed from the initial qualitative evaluation to include numerical simulation, analytical solutions, and other quantitative methods, mainly including the surface heat flow method (Muffler and Cataldi, 1978), magma heat budget method (Noguchi, 1970), total good flow method (Huttrer, 1996), dynamic mining method (Kiran, et al., 2022), reservoir volume method (Aravena, et al., 2016a; Palmer-Wilson, et al., 2018; Gao, et al., 2022)and so on, but reservoir volume method is the most widely used. As more and more geothermal fields are discovered and developed, it is increasingly recognized that the exact conditions of many reservoirs are difficult to determine, so Monte Carlo simulation is a good idea to simulate the uncertainty of various parameters, this method mainly combines Monte Carlo simulation and reservoir volume method, which belongs to an improved reservoir volume method (Iglesias and Torres, 2003). Moreover, with the development of computers, numerical simulation of geothermal fields is also used in geothermal resource evaluation, and many calculation methods for geothermal resources are improved based on reservoir volume method (Tian, et al., 2020). Probabilistic evaluation of geothermal resources using design of experiments (DE) and response surface method (RSM) is a more promising technique that can be implemented more easily and quickly (Quinao and Zarrouk, 2018; Pratama, et al., 2020). The general calculation formula of the reservoir volume method is as follows equations (Eq. 1).
Where Qh (J) represents the total geothermal resources stored in water and rock,
3.2 Monte Carlo simulation
Monte Carlo simulation is one of the oldest and most widely used statistical procedures for making inferences based on a small sample under various conditions (Sortino, et al., 2010). It uses diverse probability distribution, which is close to the real distribution of input variables, to imitate the variability and uncertainty of a given model with different input variables. Monte Carlo simulation has been used in geothermal resources potential calculation (Palmer-Wilson, et al., 2018; Sutopo, et al., 2019; Wang, et al., 2019; Wang et al., 2021), geothermal power generation capacity (Quinao and Zarrouk, 2018; Miranda, et al., 2020; Ciriaco, et al., 2022), and techno-economic assessment of geothermal resources (Miranda, et al., 2021; Frey, et al., 2023), and it is based on the reservoir volume method, comprehensively considering the random characteristics of each input variables, using repetitive iteration of random variable sampling sequences which follow different probability distributions, such as uniform, triangular, Gaussian, and pert distribution. At last, the probability distribution and cumulative frequency distributions of geothermal resources potential can be provided.
4 Geothermal features data and other input variables
4.1 The density, porosity, and specific heat capacity of geothermal reservoir rock
Uplifted mountain geothermal resources in Guangdong can be divided into two types according to the geothermal reservoir, one is the most widely distributed the fracture-banded reservoir, and the other is the relatively concentrated distribution of the karst-layered reservoir. However, the medium rock that constitutes the fracture-banded reservoir is mainly intrusive rock bodies formed by magmatic activity, including the Caledonian, the variscan, the Indosinian, the Yanshanian, the Himalayan, and some clastic rock layers of Cretaceous and the tertiary sedimentary rocks, etc., it is diversity. And the medium rock that constitutes the karst-layered reservoir is mainly carbonate rock, including sandstone, limestone, mudstone, and so on. Therefore, according to the uncertainty of, the specific heat capacity and porosity of geothermal reservoir rock, the density (ρr), specific heat capacity (Cr), and porosity ϕ) of geothermal reservoir rock in Guangdong are given a triangular distribution model. The characteristic value of several different reservoir lithologies has been collected from existing literature. The results are shown in Table 1.
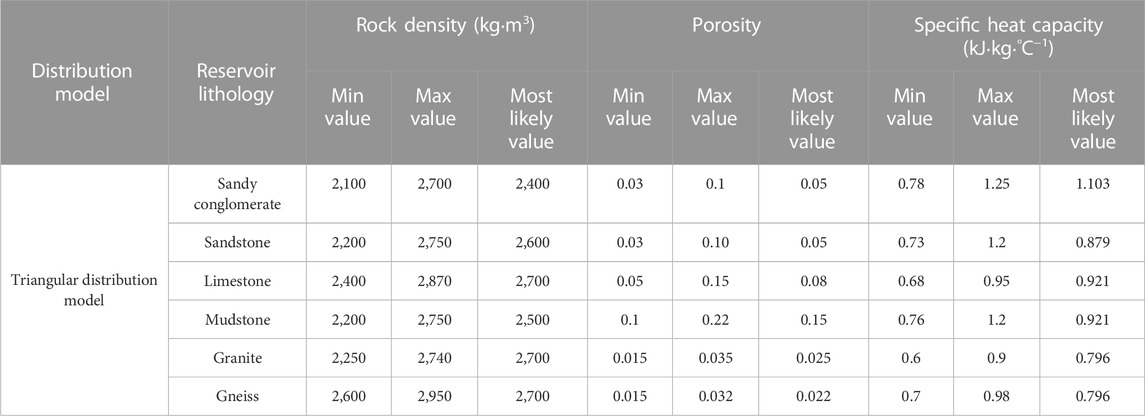
TABLE 1. The characteristic value of the density, specific heat capacity, and porosity in Reservoir lithology.
4.2 The features of geothermal fields
According to the 4th Geological Brigade of Guangdong Geological Bureau (Zeng, 2015; Wang, et al., 2017), geothermal fields including hot springs and geothermal wells have been successfully discovered and drilled in Guangdong. Tables 2, 3. Provides details on the uplifted mountain geothermal resources, including 315 geothermal fields in Guangdong province, such as the geothermal reservoir type, hot water temperature, hot water density (
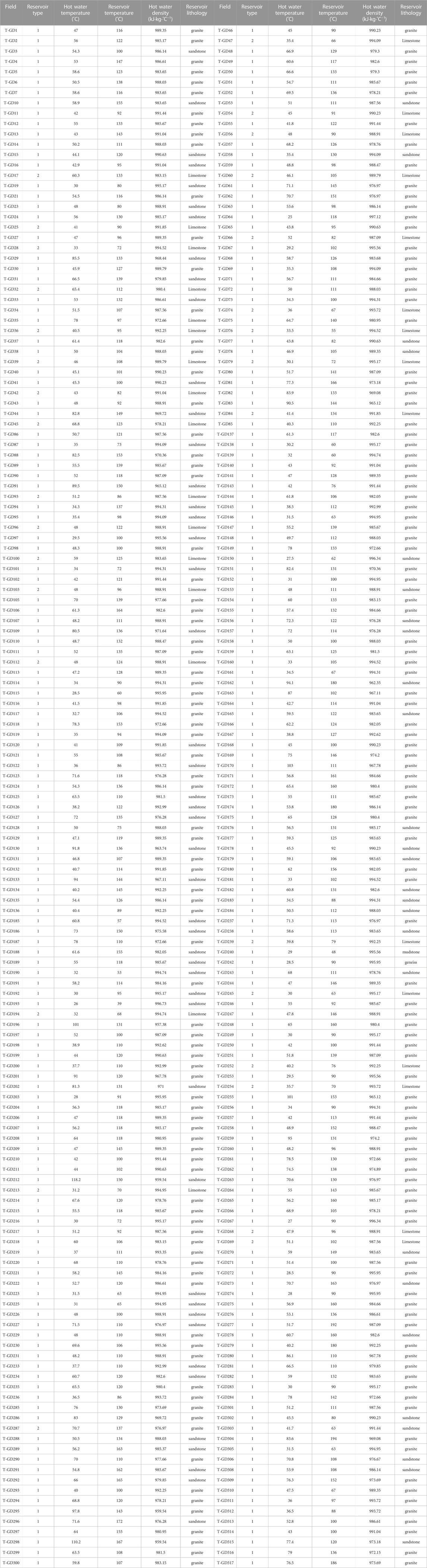
TABLE 2. Geothermal fields data of uplifted mountain in Guangdong province (revised from The 4th Geological Brigade of Guangdong Geological Bureau (Zeng, 2015), and also referenced by ((Wang, et al., 2017)), number 1 in the Reservoir column of the table represents the fracture-banded reservoir, and number 2 represents the karst-layered reservoir).
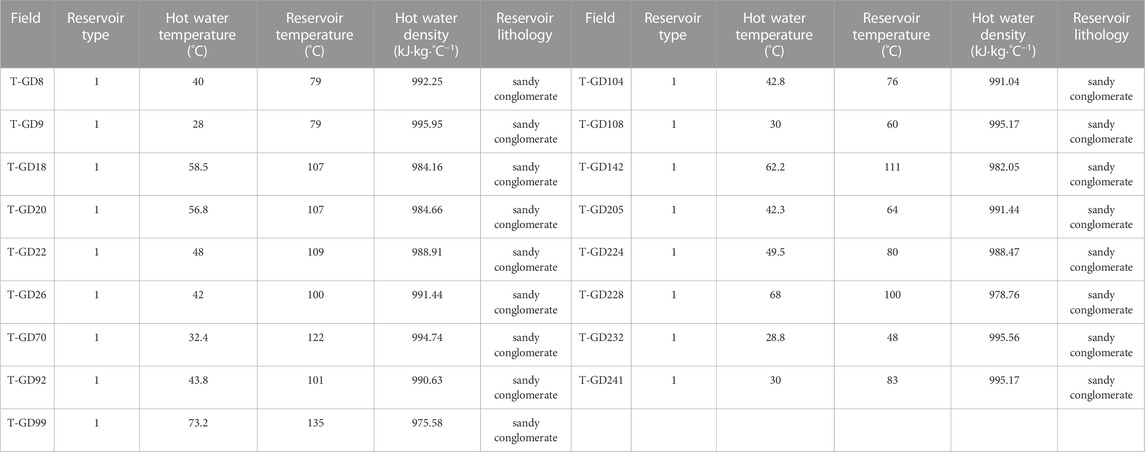
TABLE 3. Geothermal fields data of uplifted mountain in Guangdong province (revised from The 4th Geological Brigade of Guangdong Geological Bureau (Zeng, 2015), and also referenced by ((Wang, et al., 2017)), number 1 in the Reservoir column of the table represents the fracture-banded reservoir, and number 2 represents the karst-layered reservoir).
4.3 Surface temperature
The surface temperature data of Guangdong province comes from the Climatic Research Unit gridded Time Series (CRU TS) climate dataset, CRU TS is one of the most widely used climate datasets in the world, produced by the UK’s National Centre for Atmospheric Science (NCAS), and provides monthly data with 0.5° resolution covering the land surface from 1901 to 2020. The CRU TS dataset has 10 sets of data based on near-surface measurements: temperature, precipitation, humidity, frost days, cloud cover, and potential transpiration (Harris, et al., 2020). To obtain the surface temperature of the whole Guangdong province, this research extracts the global monthly temperature data of this dataset from 2011 to 2020 and then calculates the average temperature of Guangdong Province, Hong Kong, and Macao as shown in Figure 6. The average surface temperature (
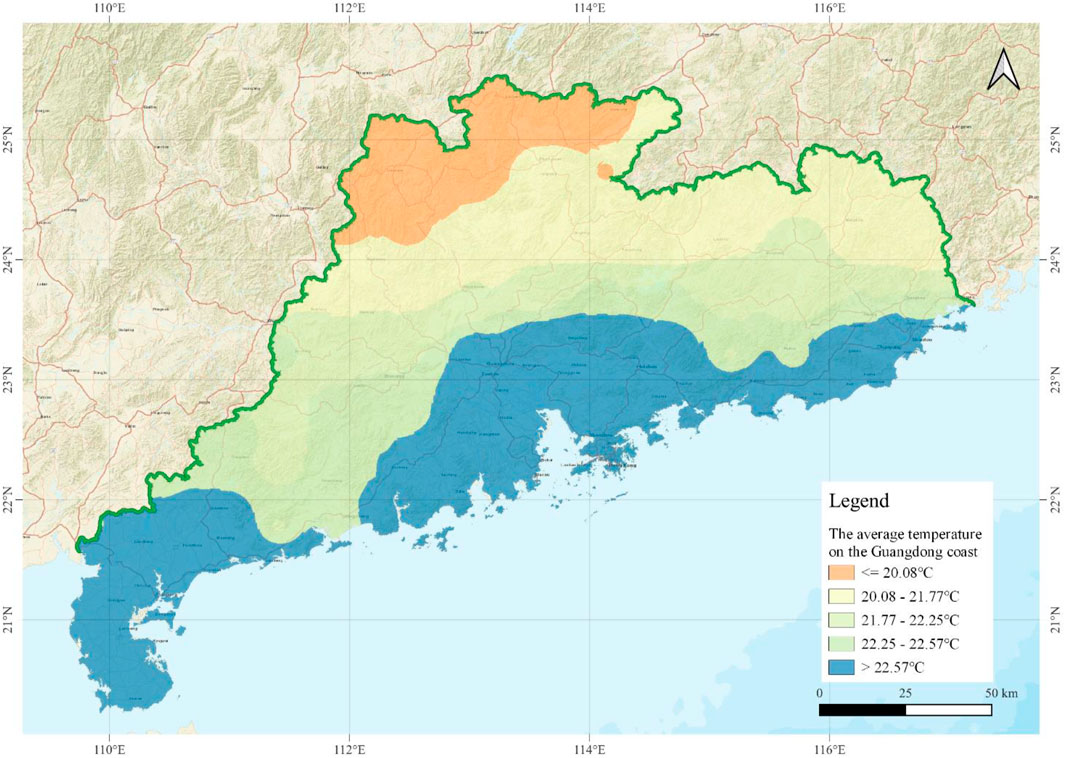
FIGURE 6. Distribution of average temperature in Guangdong province from 2011 to 2020 (obtained from the CRU TS climate dataset (Harris, et al., 2020)).
5 Result
5.1 Simulation of the fracture-banded reservoir geothermal resources
To describe the uncertainty and diversity of input parameters, the density, specific heat capacity, and porosity in reservoir lithology are given to the triangle distribution model as shown in Table 1. The specific heat capacity of hot water and the volume of the geothermal reservoir are given to a uniform distribution model. The Montel Carlo simulation iteration is set as 2000 times. The Monte Carlo simulation results are shown in Figure 7.
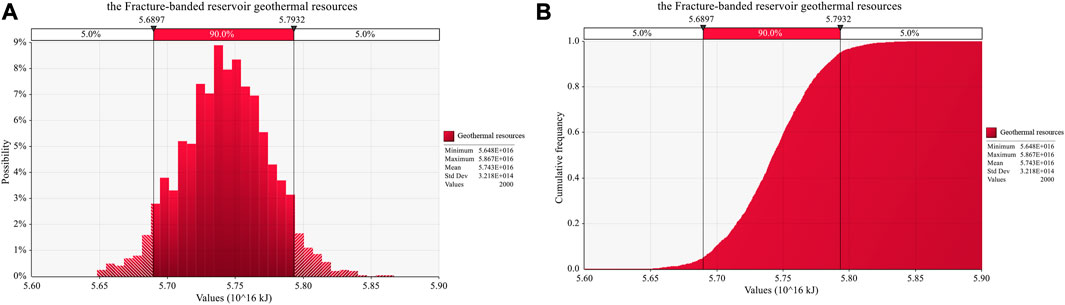
FIGURE 7. Simulation results for the fracture-banded reservoir geothermal resources in Guangdong province. (A) Possibility distribution figure of the fracture-banded reservoir geothermal resources; (B) Cumulative frequency figure of the fracture-banded reservoir geothermal resources).
Figure 7A. indicates that the geothermal resources reserve value of the fracture-banded reservoir in Guangdong province changed from 5.648×1016 kJ to 5.867×1016 kJ with a mean value of (5.743×1016 kJ). And the highest probability exceeds 8.5% with geothermal resources reserves value of approximately 5.743×1016 kJ. Figure 7B presents that a 90% probability of geothermal resources reserves value ranges from 5.6897×1016 kJ to 5.7932×1016 kJ.
5.2 Simulation of the karst-layered reservoir geothermal resources
As for the karst-layered reservoir geothermal resources, the Monte Carlo simulation results are shown in Figure 8 with 2000 times iterations. Figure 8A. indicates that the geothermal resources reserve value of the karst-layered reservoir in Guangdong province changed from 5.089×1015 kJ to 5.536×1015 kJ with a mean value of (5.328×1015 kJ). And the highest probability approaches 8% with geothermal resources reserves value of approximately 5.345×1015 kJ. Figure 8B. Presents that a 90% probability of geothermal resources reserves value ranges from 5.2126×1015 kJ to 5.4457×1015 kJ.
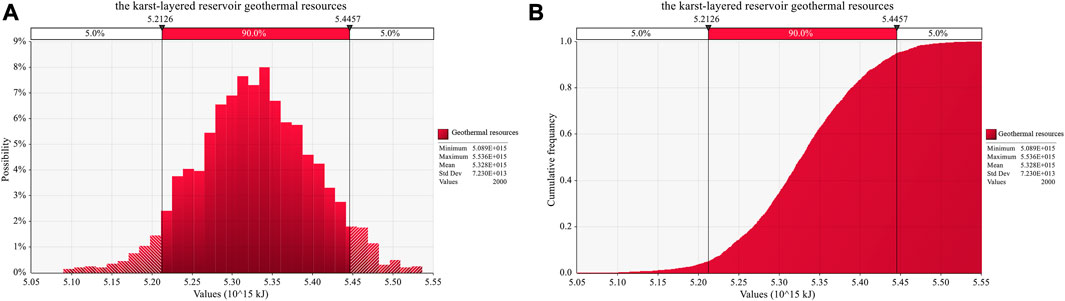
FIGURE 8. Simulation results for the karst-layered reservoir geothermal resources in Guangdong province. (A) Possibility distribution figure of the karst-layered reservoir geothermal resources; (B) Cumulative frequency figure of the karst-layered reservoir geothermal resources).
5.3 Monte Carlo simulation of uplifted mountain geothermal resources in Guangdong province
The finding suggests that uplifted mountain geothermal resources along the Guangdong province are abundant, the geothermal resources reserves value are shown in Figure 9 with 2000 times Monte Carlo simulation iteration. Figure 9A. indicates that uplifted mountain geothermal resources in Guangdong province are estimated to change from 6.176×1016 kJ to 6.399×1016 kJ with a mean value of (6.275×1016 kJ), and the highest probability exceeds 8% with geothermal resources reserves value of approximately 6.28×1016 kJ. Figure 9B. Presents that a 90% probability of uplifted mountain geothermal resources reserves value ranges from 6.2215×1016 kJ to 6.3286×1016 kJ.
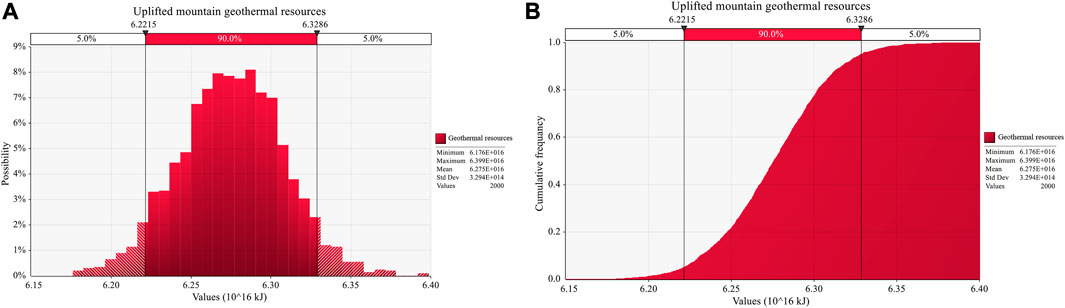
FIGURE 9. Simulation results for uplifted mountain geothermal resources in Guangdong province. (A) Possibility distribution figure of uplifted mountain geothermal resources; (B) Cumulative frequency figure of uplifted mountain geothermal resources).
We can also find that the existing uplifted mountainous geothermal resources potential in Guangdong Province is enormous, and the main geothermal reservoir type of geothermal resources is the fracture-banded reservoir supplemented by the karst-layered reservoir, the geothermal resources reserves of the fracture-banded reservoir are about 10 times that of the karst-layered reservoir. The total amount of uplifted mountainous geothermal resources is equal to 2.11–2.18×105 Ten thousand tons of standard coal (mean 2.14×105 Ten thousand tons of standard coal).
6 Conclusion
This investigation utilized the volume method as its foundation and accounted for the uncertainty of geothermal reservoir parameters by implementing Monte Carlo simulation. Using both triangular and uniform distribution models to simulate input parameters of geothermal fields in Guangdong province, the study successfully evaluated the potential of uplifted mountain geothermal resources within the area. The findings highlight that the fracture-banded reservoir geothermal resources are estimated to range from 5.648–5.867×1016 kJ (mean 5.743×1016 kJ), while the karst-layered reservoir geothermal resources are approximated to be between 5.089–5.536×1015 kJ (mean 5.328×1015 kJ). Ultimately, these results suggest that there is significant potential for uplifted mountain geothermal resources along the Guangdong province, with estimates ranging from 6.176–6.399×1016 kJ (mean 6.256×1016 kJ). It also shows that uplifted mountain geothermal resources are very rich in Guangdong, and the main type of geothermal reservoir is the fracture-banded reservoir supplemented by the karst-layered reservoir. The geothermal resource reserves of the fracture-banded reservoir are about 10 times that of the karst-layered reservoir. The total amount of uplifted mountainous geothermal resources is equal to 2.11–2.18×105 Ten thousand tons of standard coal (mean 2.14×105 Ten thousand tons of standard coal). Guangdong province’s geothermal resources can provide a new direction for the transformation of Guangdong’s energy structure from fossil energy to renewable energy.
Data availability statement
The original contributions presented in the study are included in the article/Supplementary material, further inquiries can be directed to the corresponding author.
Author contributions
YL: conceptualization, methodology, investigation resources, formal analysis, writing–original Draft; XL: data curation, supervision; JL conceptualization, investigation, supervision, writing review and editing. All authors contributed to the article and approved the submitted version.
Funding
This study was financially supported by the National Natural Science Foundation of China (Grant. Nos 52104209, 52174083, 52172625, and U22A20166).
Conflict of interest
The authors declare that the research was conducted in the absence of any commercial or financial relationships that could be construed as a potential conflict of interest.
Publisher’s note
All claims expressed in this article are solely those of the authors and do not necessarily represent those of their affiliated organizations, or those of the publisher, the editors and the reviewers. Any product that may be evaluated in this article, or claim that may be made by its manufacturer, is not guaranteed or endorsed by the publisher.
References
Alqahtani, F., Aboud, E., Ehsan, M., Naseer, Z., Abdulfarraj, M., Abdelwahed, M. F., et al. (2023a). Geothermal exploration using remote sensing, surface temperature, and geophysical data in lunayyir volcanic field, Saudi arabia. Sustainability 15, 7645. doi:10.3390/su15097645
Alqahtani, F., Ehsan, M., Aboud, E., Abdulfarraj, M., and El-Masry, N. (2023b). Integrated approach using petrophysical, gravity, and magnetic data to evaluate the geothermal resources at the Rahat Volcanic Field, Saudi Arabia. Front. Earth Sc-Switz 11, 11. doi:10.3389/feart.2023.1135635
Aravena, D., Munoz, M., Morata, D., Lahsen, A., Angel Parada, M., and Dobson, P. (2016a). Assessment of high enthalpy geothermal resources and promising areas of Chile. Geothermics 59, 1–13. doi:10.1016/j.geothermics.2015.09.001
Aravena, D., Munoz, M., Morata, D., Lahsen, A., Parada, M. A., and Dobson, P. (2016b). Assessment of high enthalpy geothermal resources and promising areas of Chile. Geothermics 59, 1–13. doi:10.1016/j.geothermics.2015.09.001
Ciriaco, A. E., Uribe, M. H., Zarrouk, S. J., Downward, T., Omagbon, J. B., Austria, J. J. C., et al. (2022). Probabilistic geothermal resource assessment using experimental design and response surface methodology: The Leyte geothermal production field. Geothermics 103, 102426. doi:10.1016/j.geothermics.2022.102426
Frey, M., van der Vaart, J., Baer, K., Bossennec, C., Calcagno, P., Dezayes, C., et al. (2023). Techno-economic assessment of geothermal resources in the variscan basement of the northern upper rhine graben. Nat. Resour. Res. 32, 213–234. doi:10.1007/s11053-022-10138-4
Fuchs, S., Norden, B., Artemieva, I., Chiozzi, P., Dedecek, P., Demezhko, D., et al. (2021). The global heat flow Database: Release. doi:10.5880/fidgeo.2021.014
Gao, X., Li, T., Zhang, Y., Kong, X., and Meng, N. (2022). A review of simulation models of heat extraction for a geothermal reservoir in an enhanced geothermal system. Energies 15, 7148. doi:10.3390/en15197148
Harris, I., Osborn, T. J., Jones, P., and Lister, D. (2020). Version 4 of the CRU TS monthly high-resolution gridded multivariate climate dataset. Sci. Data 7, 109. doi:10.1038/s41597-020-0453-3
Huttrer, G. W. (1996). The status of world geothermal power production 1990–1994. Geothermics 25, 165–187. doi:10.1016/0375-6505(95)00041-0
Iglesias, E. R., and Torres, R. J. (2003). Low-to medium-temperature geothermal reserves in Mexico: A first assessment. Geothermics 32, 711–719. doi:10.1016/j.geothermics.2003.07.002
Jiang, G., Li, W., Rao, S., Shi, Y., Tang, X., Zhu, C., et al. (2016). Heat flow, depth-temperature, and assessment of the enhanced geothermal system (EGS) resource base of continental China. Environ. Earth Sci. 75, 1432. doi:10.1007/s12665-016-6238-5
Jiang, G. Z., Hu, S. B., Shi, Y. Z., Zhang, C., Wang, Z. T., and Hu, D. (2019). Terrestrial heat flow of continental China: Updated dataset and tectonic implications. Tectonophysics 753, 36–48. doi:10.1016/j.tecto.2019.01.006
Kiran, R., Dansena, P., Salehi, S., and Rajak, V. K. (2022). Application of machine learning and well log attributes in geothermal drilling. Geothermics 101, 102355. doi:10.1016/j.geothermics.2022.102355
Liu, K., Zhang, Y., He, Q., Zhang, S., Jia, W., He, X., et al. (2023). Characteristics of thermophysical parameters in the Wugongshan area of South China and their insights for geothermal genesis. Front. Environ. Sci. 11, 11. doi:10.3389/fenvs.2023.1112143
Miranda, M. M., Raymond, J., and Dezayes, C. (2020). Uncertainty and risk evaluation of deep geothermal energy source for heat production and electricity generation in remote northern Regions. Energies 13, 4221. doi:10.3390/en13164221
Miranda, M., Raymond, J., Willis-Richards, J., and Dezayes, C. (2021). Are engineered geothermal energy systems a viable solution for arctic off-grid communities? A techno-economic study. Water 13, 3526. doi:10.3390/w13243526
Moxiang, C., and Jiyang, W. (1994). Geothermal resources in China- Formation characteristics and potential evaluation. Beijing: Beijing Science Press.
Muffler, P., and Cataldi, R. (1978). Methods for regional assessment of geothermal resources. Geothermics 7, 53–89. doi:10.1016/0375-6505(78)90002-0
Noguchi, T. (1970). An attempted evaluation of geothermal energy in Japan. Geothermics 2, 474–477. doi:10.1016/0375-6505(70)90046-5
Palmer-Wilson, K., Banks, J., Walsh, W., and Robertson, B. (2018). Sedimentary basin geothermal favourability mapping and power generation assessments. Renew. Energy 127, 1087–1100. doi:10.1016/j.renene.2018.04.078
Pratama, H. B., Supijo, M. C., and Sutopo, (2020). Experimental design and response surface method in geothermal energy: A comprehensive study in probabilistic resource assessment. Geothermics 87, 101869. doi:10.1016/j.geothermics.2020.101869
Quinao, J. J. D., and Zarrouk, S. J. (2018). Geothermal resource assessment using experimental design and response surface methods: The ngatamariki geothermal field, New Zealand. Renew. Energy 116, 324–334. doi:10.1016/j.renene.2017.09.084
Song, G., Zhang, B., Wang, X., Gong, J., Chan, D., Bernett, J., et al. (2005). Indoor radon levels in selected hot spring hotels in Guangdong, China. Sci. Total Environ. 339, 63–70. doi:10.1016/j.scitotenv.2004.06.026
Sortino, F., van der Meer, R., Plantinga, A., and Kuan, B. (2010). “Chapter 3 - beyond the sortino ratio,” in The sortino framework for constructing portfolios. Editor F. Sortino (Boston: Elsevier), 23–52.
Sutopo,, , Prabata, W., and Pratama, H. B. (2019). The development study of Karaha-Talaga Bodas geothermal field using numerical simulation. Geotherm. Energy 7, 21. doi:10.1186/s40517-019-0139-2
Tian, B. Q., Kong, Y. L., Gong, Y. L., Ye, C. T., Pang, Z. H., Wang, J. Y., et al. (2020). An improved volumetric method of geothermal resources assessment for shallow ground combining geophysical data. Renew. Energy 145, 2306–2315. doi:10.1016/j.renene.2019.08.005
Trota, A., Ferreira, P., Gomes, L., Cabral, J., and Kallberg, P. (2019). Power production estimates from geothermal resources by means of small-size compact climeon heat power converters: Case studies from Portugal (sete cidades, azores and longroiva spa, mainland). Energies 12, 2838. doi:10.3390/en12142838
Trumpy, E., Donato, A., Gianelli, G., Gola, G., Minissale, A., Montanari, D., et al. (2015). Data integration and favourability maps for exploring geothermal systems in Sicily, southern Italy. Geothermics 56, 1–16. doi:10.1016/j.geothermics.2015.03.004
Wang, D., Shen, W., and Wei, S. (2003). Accumulation and transformation of atmospheric mercury in soil. Earth Sci. Front. 10, 209–214. doi:10.1016/S0048-9697(02)00569-7
Wang, G., Liu, Y., Zhu, X., and Zhang, W. (2020). The status and development trend of geothermal resources in China. Earth Sci. Front. 27, 1–9. doi:10.13745/j.esf.2020.1.1
Wang, G., Zhang, W., Liang, J., Lin, W., Liu, Z., and Wang, W. (2017). Evaluation of geothermal resources potential in China. Acta Geosci. Sin. 38, 449–459.
Wang, J., Hu, S., Pang, Z., He, L., Zhao, P., Zhu, C., et al. (2012). Estimate of geothermal resources potential for hot dry rock in the continental area of China. Sci. Technol. Rev. 30, 25–31. doi:10.3981/j.issn.1000-7857.2012.32.003
Wang, Y., Wang, L., Bai, Y., Wang, Z., Hu, J., Hu, D., et al. (2021). Assessment of geothermal resources in the north jiangsu basin, east China, using Monte Carlo simulation. Energies 14, 259. doi:10.3390/en14020259
Wang, Z., Jiang, G., Zhang, C., Tang, X., and Hu, S. (2019). Estimating geothermal resources in Bohai Bay Basin, eastern China, using Monte Carlo simulation. Environ. Earth Sci. 78, 355. doi:10.1007/s12665-019-8352-7
Wenjing, L., Haonan, G., Guiling, W., and Feng, M. (2016). Occurrence prospect of HDR and target site selection study in southeastern of China. Acta Geol. Sin. 90, 2043–2058.
Whealton, C. A., Stedinger, J. R., Smith, J. D., Jordan, T. E., Horowitz, F. G., and Richards, M. C. (2020). Multi-criteria spatial screening and uncertainty analysis applied to direct-use geothermal projects. Int. J. Geogr. Inf. Sci. 34, 2053–2076. doi:10.1080/13658816.2020.1765247
White, D. E. (1968). Hydrology, activity, and heat flow of the Steamboat Springs thermal system. Washington, D.C. Washoe County, Nevada.
Witter, J. B., Trainor-Guitton, W. J., and Siler, D. L. (2019). Uncertainty and risk evaluation during the exploration stage of geothermal development: A review. Geothermics 78, 233–242. doi:10.1016/j.geothermics.2018.12.011
Xi, Y., Wang, G., Liu, S., Zhao, Y., and Hu, X. (2018). The formation of a geothermal anomaly and extensional structures in Guangdong, China: Evidence from gravity analyses. Geothermics 72, 225–231. doi:10.1016/j.geothermics.2017.11.009
Xie, H., Yang, Z., and Deng, J. (2019a). Assessment of geothermal resource potential in the Guangdong-Hong Kong-Macao greater Bay area. Adv. Eng. Sci. 51, 1–8.
Xie, H., Yang, Z., and Deng, J. (2019b). Assessment of geothermal resource potential in the Guangdong-Hong Kong-Macao greater Bay area. Gongcheng Kexue Yu Jishu/Advanced Eng. Sci. 51, 1–8. doi:10.15961/j.jsuese.201900026
Ying, Z., Jun, L., and Jian-yun, F. (2020). Characteristics of geothermal reservoirs and utilization of geothermal resources in the southeastern coastal areas of China. J - J. Groundw. Sci. Eng. 8, 134. doi:10.19637/j.cnki.2305-7068.2020.02.005
Yoshida, M., and Santosh, M. (2020). Energetics of the solid earth: An integrated perspective. Energy Geosci. 1, 28–35. doi:10.1016/j.engeos.2020.04.001
Yuan, J., Xu, F., and Zheng, T. (2022). The genesis of saline geothermal groundwater in the coastal area of Guangdong Province: Insight from hydrochemical and isotopic analysis. J. Hydrol. 605, 127345. doi:10.1016/j.jhydrol.2021.127345
Zeng, S. (2015). Investigation and evaluation of geothermal resources in Guangdong Province and zoning report. Zhanjiang: The 4th Geological Brigade of Guangdong Geological Bureau.
Zheng, H., Luo, J., Zhang, Y., Feng, J., Zeng, Y., and Wang, M. (2021). Geological characteristics and distribution of granite geothermal reservoir in southeast coastal areas in China. Front. Earth Sc-Switz 9, 683696. doi:10.3389/feart.2021.683696
Keywords: resources assessment, geothermal resources, Monte Carlo simulation, volume method, uplifted mountain
Citation: Li Y, Long X and Lu J (2023) Evaluation of geothermal resources potential in the uplifted mountain of Guangdong province using the Monte Carlo simulation. Front. Earth Sci. 11:1233026. doi: 10.3389/feart.2023.1233026
Received: 01 June 2023; Accepted: 24 July 2023;
Published: 03 August 2023.
Edited by:
Haibing Shao, Helmholtz Association of German Research Centres (HZ), GermanyReviewed by:
Muhsan Ehsan, Bahria University, PakistanXiaoji Shang, China University of Mining and Technology, China
Copyright © 2023 Li, Long and Lu. This is an open-access article distributed under the terms of the Creative Commons Attribution License (CC BY). The use, distribution or reproduction in other forums is permitted, provided the original author(s) and the copyright owner(s) are credited and that the original publication in this journal is cited, in accordance with accepted academic practice. No use, distribution or reproduction is permitted which does not comply with these terms.
*Correspondence: Jun Lu, anVubHVAc3p1LmVkdS5jbg==