- 1School of Dentistry, University of Liverpool, Liverpool, United Kingdom
- 2School of Psychology, University of Wolverhampton, Wolverhampton, United Kingdom
Research attention has shifted from feedback delivery mechanisms to supporting learners to receive feedback well (Winstone et al., 2017a). Recognizing feedback and the action necessary to take the next steps are vital to self-regulated performance (Zimmerman, 2000; Panadero, 2017). Evaluative judgments supporting such mechanisms are vital forces that promote academic endeavor and lifelong learning (Ajjawi et al., 2018). Measuring such mechanisms is well-developed in occupational settings (Boudrias et al., 2014). Understanding how these relate to self-regulated learning gains in Higher Education (HE) is less well-understood (Forsythe and Jellicoe, 2018). Here we refined a measure of feedback integration from the occupational research domain (Boudrias et al., 2014) and investigate its application to HE. Two groups of psychology undergraduates endorsed perspectives associated with feedback. The measure examines characteristics associated with feedback including message valence, source credibility, interventions that provide challenge, feedback acceptance, awareness, motivational intentions, and the desire to make behavioral changes and undertake development activities following feedback. Of these suggested characteristics, exploratory factor analysis revealed that undergraduate learners endorsed credible source challenge, acceptance of feedback, awareness from feedback, motivational intentions and the desire to take behavioral changes and participate in development activities formed a single factor. The structure of the instrument and hypothesized paths between derived factors was confirmed using latent variable structural equation modeling. Both models achieved mostly good, and at least acceptable fit, endorsing the robustness of the measure in HE learners. These finding increase understanding of HE learner's relationship with feedback. Here, acceptance of feedback predicts the extent to which learners found the source of feedback credible. Credible source challenge in turn predicts awareness resulting from feedback. Subsequently, awareness predicts motivations to act. These promising results, whilst cross-sectional, also have implications for programmes. Further research employing this instrument is necessary to understand changes in learner attitudes in developing beneficial self-regulated skills that support both programmes of study and graduates in their careers.
Introduction
Providing feedback that assesses learner performance relative to goals or objectives is proposed as a necessary process in optimizing performance. Universities have spent significant resources in their attempts to improve student satisfaction in relation to assessment and feedback. For example, increasing learner assessment literacy through the use of rubrics is thought to make available the tacit knowledge that academics often carry around in their heads. However, most of these interventions have had very little impact on student satisfaction with assessment and feedback and National Student Survey scores on this area remain relatively stable (and low) across the HE sector (Evans et al., 2018).
Providing feedback may not be sufficient. Developing learner's skills in integrating feedback by evaluating and making judgments about the courses of action necessary for progression appears to be a necessary additional step (Nicol and Macfarlane-Dick, 2006; Ajjawi et al., 2018). Feedback enables adjustment by informing learners where they are against a desired standard of performance. This negative feedback loop is suggested to promote self-regulation in the workplace by enabling goal confirmation or revision (Locke and Latham, 1990; Diefendorff and Lord, 2008; Lord et al., 2010). Within the learning domain, the evaluations that learners make following performance, for example in response to feedback, is suggested to be a central mechanism in self-regulated learning (Zimmerman, 2000; Panadero et al., 2017). Once these skills are developed, researchers propose that learners can become self-directed (Van Merriënboer and Kirschner, 2017).
Theoretical Background
In its broadest sense, feedback is reported to hold two fundamental roles, it acts as a mediator of “in-flight” performance or as a moderator of subsequent performance, by upregulating or downregulating subsequent goals (Ashford and De Stobbeleir, 2013). Here, we focus primarily on the idea of post-performance feedback and its role in changing future performance as this mirrors much of the HE assessment landscape. Hattie and Timperley propose for feedback to have an effect that three evaluations must be considered “Where am I going? (What are the goals?), How am I going? (What progress is being made toward the goal?), and Where to next? (What activities need to be undertaken to make better progress?)” (Hattie and Timperley, 2007, p. 86). These evaluations support an ipsative self-regulatory approach, connecting previous and future learning, particularly working toward a known performance standard (Brookhart, 2018). Within this approach, there is an inherent assumption that learners possess the necessary skills and motivations to engage in feedback, in an objective and dispassionate manner (Joughin et al., 2018) that support self-regulated approaches to performance indicated by Lord and colleagues (Lord et al., 2010).
Given the importance of feedback, the mechanisms for delivering effective feedback to HE learners has been the focus of research for some time (Nicol and Macfarlane-Dick, 2006). Whilst it seems clear that feedback can have an effect on performance, a wide range of effects have been reported, depending on the types of feedback mechanisms utilized (Hattie and Timperley, 2007). A medium to large effect of feedback on performance has been reported by some researchers (Hattie et al., 1996). However, one third of feedback interventions are reported to have a deleterious effect on performance (Kluger and DeNisi, 1996). Research attention on delivery has led some to suggest that HE learners are typecast as passive recipients in feedback discussions (Evans, 2013). Some authors report that neither party is said to understand who owns feedback, nor do they report being satisfied with it (Hughes, 2011). There are some suggestions that even if learners acknowledge the utility of feedback, managing barriers is no easy task (Forsythe and Johnson, 2017). This evidence suggests that complexity in the feedback environment leads to lack of receptivity.
Despite the research focus on delivery mechanisms in HE, and learners understanding which of these mechanisms serve them best, fostering greater awareness and receptivity to feedback remains problematic (Winstone et al., 2016, 2017a). Learners are reported to seek feedback that increases positive feeling but pragmatically is reported to have little effect in terms of future performance (Hattie and Timperley, 2007). Recent evidence indicates that students are aware of and in many cases value useful feedback that provides challenge (Winstone et al., 2016; Forsythe and Jellicoe, 2018). However, learners often fail to engage in adaptive evaluations of this information. It is suggested that these relate to learner heuristics and biases (Joughin et al., 2018), and associated barriers (Winstone et al., 2017b). Recent evidence supports the idea of adaptive or defensive evaluations made by learners during appraisal which has the power to undermine decision making relating to feedback (Forsythe and Johnson, 2017; Van Merriënboer and Kirschner, 2017; Panadero et al., 2018). These are typified by dual processing theories of decision making (Kahneman and Tversky, 1979, 1984; Stanovich and West, 2000; Kahneman, 2011). In the first of these dual perspectives, described as system one thinking, reactive judgments are made quickly and rely on rules of thumb. In this mode of thinking, Joughin et al. (2018) indicate that learners may opt not to engage in the deliberate and resource intensive cognitive appraisals that are necessary to optimizing gains from learning. Such evaluations typify system two thinking, the second to these perspectives. In addition to stunting engagement, heuristics and biases are proposed to inflate learner evaluations of their work and the confidence they have in it (Peverly et al., 2003). This suggests that developing analytical and deliberate evaluative judgment processes supports more realistic levels of confidence. Learners may not be in possession of the resources necessary to engage in such deliberative appraisals, as these might be aversive prompt anxiety. In this frame of thinking it is suggested that learners look to invalid cues that typify system one thinking (Van Merriënboer and Kirschner, 2017). To optimize gains in learning taking an objective, and deliberate approach to feedback is necessary. A number of personal and relationship barriers must be negotiated to engage with feedback in an adaptive manner (Winstone et al., 2017b).
Fostering an environment that encourages positive dialogue is a pillar of good feedback practice (Nicol and Macfarlane-Dick, 2006). Recent evidence suggests that student engagement in such dialogue is challenged when learners see themselves as consumers (Bunce et al., 2017). Researchers have suggested, for example, that instructors modeling feedback response provide an enlightening scaffold for learners, particularly where structural barriers exist, such as learner remoteness from instructors (Carless and Boud, 2018). Characteristics of the feedback message and the context in which messages are transmitted by the sender and absorbed by the recipient has the power to enable or restrict action. Amongst others, these perspectives are reported to lead to differential patterns in perceptions of confidence, competence, motivation and effort which have downstream effects on performance (Pitt and Norton, 2017). Recent research indicates that feedback that provides challenge and strategy are highly endorsed by learners (Winstone et al., 2016; Forsythe and Johnson, 2017; Forsythe and Jellicoe, 2018).
Within the HE context, several barriers are reported. Barriers relate to lack of awareness of the feedback process; poor knowledge of associated strategies and opportunities for development; lacking agency and associated self-regulatory strategies; and low engagement and volition with addressing the issues raised in feedback. Managing these barriers is suggested to be an important step in a move toward encouraging learner receptivity to feedback (Winstone et al., 2017a). Transforming this narrative from a passive to an active process is suggested to be best considered as a partnership (Evans, 2013), and others have suggested working in co-operation with learners to co-construct goals from feedback (Farrell et al., 2019). The extent to which feedback is used for development, relates to a complex mix of characteristics, including those associated with the message under consideration, inter-personal relationships and intra-personal factors (Hattie and Timperley, 2007; Stone and Heen, 2015). Although evidence is mixed, message characteristics include whether the feedback message has positive or negative valence, and also relate to whether the recipient believes that it has face-validity (Evans, 2013). Interpersonal relationships, between the source of the feedback and the recipient are thought to be crucial in creating a suitable environment (Winstone et al., 2017a). Boudrias et al. (2014) posit that where the feedback source is trustworthy, greater acceptance and awareness is promoted by feedback. Intrapersonal factors, including personality, motivations and emotions also foster a dynamic self-regulatory environment (Evans, 2013). These ideas are supported both within approaches to self-regulated learning and recent models of feedback integration.
A receiving focus in relation to feedback is supported in Winstone et al. (2017a) recent SAGE framework. The SAGE framework promotes strategies that aim to increase the learner's ability to “Self-assess,” possess greater “Assessment Literacy,” employ “Goal-setting and self-regulatory strategies,” and develop “Engagement and motivational strategies.” In this way, developing learner's abilities to judge the quality of their work and to make necessary adjustments must be a key outcome for educators, and students, in particular if they are transition to be effective lifelong learners (Ajjawi et al., 2018). These abilities that support learning are also suggested to be fundamental to self-regulated performance in the workplace (Diefendorff and Lord, 2008; Lord et al., 2010). As such, academics seeking to promote incremental learning gains require appropriate diagnostic skills to make appropriate recommendations to foster change, where students require metacognitive abilities associated with self-assessment and self-management to enable them to optimize their chances of success (Evans, 2013).
However derived, engaging students in the development of adaptive knowledge, skills and attitudes that underpins hard won gains in learning is crucial, in particular if learners are to develop the ability to manage themselves during the courses of their studies and into employment (Forsythe and Jellicoe, 2018). A recent qualitative report indicates that learners in HE, even when approaching graduation, do not possess the emotional repertoire to manage and act upon feedback and are not enabled in doing so (O'Donovan et al., 2016; Pitt and Norton, 2017). Indications are that current assessment approaches do not enable learners to engage in development in the manner expected by employers (The Confederation of British Industry, 2016).
Within the occupational domain, Boudrias et al. (2014) developed a measure of feedback integration for candidates exposed to individual psychological assessment feedback following evaluation at an assessment center. Based on earlier such measures (see for example Kudisch, 1996) a revised measure was proposed that aimed to examine whether candidates in occupational settings who were exposed to developmental feedback would be motivated toward taking developmental actions and adopt behavioral changes resulting from feedback. Boudrias et al. (2014) postulated a causal path where characteristics associated with feedback including valence of the message, its face validity, the credibility of the source and challenge were associated with greater acceptance of feedback and awareness of changes. In turn, acceptance and awareness were proposed to relate to greater motivational intention, which was hypothesized to lead to increased behavioral and developmental changes. Observations from 97 candidates were taken on two separate occasions, separated by a 3 months interval, with 178 observations taken in total. Boudrias et al. (2014) describe a model that had excellent fit to the data. Findings indicate that awareness and its direct and indirect antecedents led to motivational intention, but acceptance did not. In this model, motivational intentions were more strongly associated in turn with behavioral change than taking developmental action. Authors suggest that these results are consistent with the Theory of Planned Behavior (Ajzen, 1996). For example, candidates evaluations are that they hold greater volition changing their own behaviors change, whereas engaging in developmental activities relies on external developmental opportunities becoming available. Sample size considerations, the self-report nature of the instrument and low reliability relating to valence of the message, limit these findings somewhat. The participant pool (n = 97) used in this study may have resulted in imprecise estimates. Generally a sample size of 200 is considered optimal (Kenny, 2015). Low power meant that Boudrias et al. (2014) were unable to examine the latent factor structure, relying instead on Cronbach's alpha. It has been argued that such metrics do not provide adequate evidence of construct validity (Flake et al., 2017; Flake and Fried, 2019). Whilst validity also relates to theoretical consideration of measures, examining the factor structure of any such measure is recommended for reliable and valid prediction.
Despite the noted limitations in Boudrias et al. (2014) model, these results provide an interesting perspective suggesting that increased awareness led to greater integration of feedback and a desire to act in accordance with feedback messages received. Whilst focused in occupational settings, Boudrias and colleagues findings could contribute important understandings in relation to undergraduates' evaluations and integration of feedback. Feedback that is more specific is postulated to lead to greater levels of performance striving (Ashford and De Stobbeleir, 2013). This is because specificity leads to greater awareness and ability to interpret the feedback in terms of the learner's future progress. This follows work suggesting that integration or recipience of feedback (see for example Nicol and Macfarlane-Dick, 2006; Winstone et al., 2017a) is an under-represented area of research and will support greater understanding of how evaluations support self-regulation during learning (Panadero et al., 2017). It has been proposed that learners can develop evaluative judgments by being engaged in formative assessment that encourages self-regulated learning (Panadero et al., 2018). In this approach, learners must understand how a piece of work is related to its context, develop the expertise that is necessary to understand the qualities and standards against which it is being judged and how these relate to assessment criteria. These evaluations align with the three considerations proposed by Hattie and Timperley (2007). Measuring learner endorsement of behaviors associated with feedback and its integration would provide a useful means of indicating whether students were prepared to make the incremental gains in learning necessary for development. Domain specific refinements are necessary to secure its applicability in terms of undergraduate learning and development. Refinements would also support the self-awareness component of the SAGE model of feedback integration (Winstone et al., 2017a). If such measurement instruments demonstrate utility in learner's self-regulated approach to learning, then it follows that employing these in diagnosis and intervention will be informative. Given the suggestion that undergraduate learners, as they move toward greater independence, often find self-regulation challenging (Zimmerman and Paulsen, 1995). Such a supportive mechanism will help to address a gap in current knowledge.
Current Study
Drawing on these suggestions, the first aim of the current study is to explore the factor structure of a modified feedback integration measure that has been utilized in occupational domains (Boudrias et al., 2014) and to translate it from occupational environments into academic endeavors. There has been some limited use of a modified version of this survey instrument within academic settings (e.g., Forsythe and Johnson, 2017; Forsythe and Jellicoe, 2018), which have provided an interesting pattern of results. However, as indicated, there has been no thorough and systematic investigation of the measurement properties of the scale within student populations. Further, we have no knowledge of a similar instrument that can either be used by educators to target interventions in the manner intended by Winstone et al. (2017a). Boudrias et al. (2014) measure appears to have a strong theoretical foundation. Whilst there are synergies between the experiences of those in the workplace and HE, the extent to which the suggested factors replicate and measure knowledge, skills and attitudes related to feedback in HE learners is not necessarily assured. Boudrias et al. (2014) measure was confirmed with a relatively small participant pool, and as a result, before this is used, further examining the nature of this measurement appears to be warranted. Further, such a measure appears to have utility as part of a self-directed approach to promote understanding in learners and address deficits in relation to feedback. As a result of the identified issues with the previous exploration of the factors, and the modifications necessary for an academic audience, a data driven analysis approach was used in the first instance as a route to providing a measurement structure that is definitive for a tertiary academic audience.
Research Aims and Hypotheses
Addressing the issues above, the first research aim was to determine a data driven approach to understanding feedback integration in tertiary academic audience based on a modification of a measure provided by Boudrias et al. (2014). The second aim of the current study is first to confirm the derived factor structure of the FLS, determined as part of exploratory analysis in aim one. Simultaneously, a tentative unidirectional path between the factors identified during exploratory analysis will be examined. Taking account of the directional model proposed by Boudrias et al. (2014) four paths were hypothesized, addressing the five derived factors. The first hypothesized path proposes that acceptance of feedback will predict credible source challenge. A second hypothesized path predicts that credible source challenge will predict awareness from feedback. Awareness from feedback will in turn predict motivational intentions, is the third hypothesized path. The final fourth path hypothesizes that motivational intention will predict behavioral changes and developmental activities.
Methodology
Participants
Two pools of participants were recruited to examine cognitive and behavioral factors associated with integration of feedback in learning. A first convenience sample of 353 first and second-year psychology undergraduate students were recruited to participate in the current study. Two sources of opportunity recruitment were used. In the first of these a convenience sample of 163 second year undergraduate participants were recruited. The first recruitment opportunity was time-limited and did not generate a large enough sample for exploratory analysis. As a result, a further sample of first year students (n = 190) were recruited using an experimental participation scheme (EPS) in return for nominal course credit. Twelve cases were excluded from the first sample and three from the second, as they failed to respond to all survey items. Following exclusion, participants were Mage = 19.54, SDage = 2.98. Eighty six percent of participants were female, mirroring the profile seen in samples recruited from these populations. Following inspection of these data, seventeen cases were excluded based on an inspection of Mahalanobis distance cut-off criteria (Kline, 2015). The remaining 321 complete cases were used in Exploratory Factor Analysis (EFA).
A second convenience sample of 402 second year students registered on a half year psychology module were requested to participate as part of a wider data collection process. Forty-six responses were excluded in the second sample where participants failed to respond to one or more of the items. Following exclusions, participants were Mage = 20.31; SDage = 3.64. Matching the first sample, 86% of participants were female. The remaining 356 complete cases were used in latent variable structural equation modeling (LVSEM).
Design
The current study employed a cross-sectional design and structural equation modeling (SEM) to explore two pools of responses to determine the factor structure of the FLS. A data driven approach using EFA was used to explore the first sample. The second sample was used in a LVSEM approach to simultaneously confirm the psychometric properties of the scale and to test a linear hypothetical path through the factors derived in EFA.
Measures
The Feedback in Learning Scale
A 34-item measure examined perspectives supporting integration of feedback in learning. Items were derived from an existing measure of feedback integration typically used in occupational research (Boudrias et al., 2014). Fit measures were at least adequate, although these were derived with relatively low participant pool. The original measure developed within an occupational setting suggests a nine-factor structure. The original measure referred to candidate integration of feedback following attendance at a specific assessment center occasion, minor modifications were made recognize the different context of the measure. To illustrate, one item “I have changed my less-efficient behaviors discussed during the feedback session” was modified to “… behaviors described in the feedback I received” supervisor is replaced by tutor to reflect the academic context. Further items from Boudrias et al. (2014) measure relating to the workplace are modified to situate the measure in higher education, an example of this relates to assessment face validity. Of these, message valence relates to the extent to the feedback received is positive or aversive; face validity can be interpreted as the idea that participants endorse the relatedness of the feedback to themselves and their future careers; source credibility relates to the person assessing the work can be relied upon to provide an accurate assessment of work; and challenge interventions, which speak to the idea that the assessor's feedback provides a catalyst for change. Here, due to its poor performance in a previous examination, and the challenge of meaningfully operationalizing face validity, we decided to discard these items from the analysis (Forsythe and Jellicoe, 2018). Five remaining factors relating to integration of feedback were also assessed. These relate to feedback acceptance, or whether the student recognizes that the feedback they receive relates to them; awareness from feedback, such that the learner will have a greater understanding of their strengths and limitations; and motivational intentions, which relate to the desire to take action, perhaps as a result these earlier factors. Finally, two outcome measures indicate the extent to which students are likely to make behavioral changes and undertake developmental actions as a result of the feedback they receive. Participant ratings of the FLS were endorsed using a 6-point response format; with a value of 1–6 (1, Strongly Disagree; 6, Strongly Agree). Higher scores relate to endorsement of each factor. As a result, reverse scoring ensured that inter-item correlations remained positive.
Procedure
Participants completed the survey online via a hyperlink directing participants to the Qualtrics (2018) online surveying platform. Participants read a participant information sheet and indicated consent to participate in the study. Participants were informed of the benign nature of the study, and that there were no anticipated risks or rewards associated with participation. In the second part of the study, related to a pedagogical project, students were furnished with automated reports, which summed scores associated with the factors they had endorsed. These automated individual feedback reports were designed to debrief participants by prompting individual reflection and greater self-awareness. Interpretive support was made available for students. This study was carried out in accordance with the recommendations of the British Psychological Society. The protocol was approved by the University of Liverpool Ethics Committee. All subjects gave written informed consent in accordance with the Declaration of Helsinki.
Data Analyses
All analyses were conducted in an R environment (R Core Team, 2013) using Jupyter notebook architecture (Kluyver et al., 2016). Data files are available as Supplementary Materials.
Analysis Checks and Data Preparation (Samples 1 and 2)
FLS items were assessed for normality. In relation to the first sample, employed in EFA, sampling adequacy was assessed using the Kaiser-Myer-Olkin (KMO) statistic. Bartlett's test of sphericity was used to assess whether inter-item correlations were sufficiently large to continue with EFA. For the second sample, data were examined for multivariate normality. As Mardia's Kurtosis test was violated (Crede and Harms, 2019; Gana and Broc, 2019) maximum likelihood estimation package with robust standard errors and Satorra-Bentler scaled test statistics (Satorra and Bentler, 2010) were obtained to correct for this violation using the MLM procedure in lavaan (Rosseel, 2012). As a result, 356 observations were employed in a latent variable structural equation modeling approach to assess a hybrid confirmatory factor analysis and path analytic approach.
Approach to Structural Equation Modeling
Model fit was assessed using the Normed X2 statistic (X2/df) (Ullman, 2001), the Tucker Lewis Index, Comparative Fit Index (TLI; CFI; Bentler, 1990; Hu and Bentler, 1999) the Root Mean Square Error of Approximation (RMSEA; MacCallum et al., 1996), and Standardized Root Mean Square Residual (SRMR; Hu and Bentler, 1999). Normed X2/df <2 (Ullman, 2001), and TLI and CFI above 0.90 (Bentler, 1990) are considered acceptable. RMSEA values indicate a good- (<0.05), fair- (>0.05, <0.08), mediocre- (>0.08, <0.10), and poor-fit (>0.10), respectively (MacCallum et al., 1996). SRMR <0.08 are deemed a good fit (Hu and Bentler, 1999).
Exploratory Factor Analysis (Sample 1)
The psych package (Revelle, 2016), was used for EFA purposes. To identify an initial factor solution, those factors reporting eigenvalues >0.70 (Jolliffe, 1972) and visual inspection of scree plots were used to confirm retained items (Cattell, 1966). This was selected as the Kaiser criterion (i.e., retaining eigenvalues > 1) is = not always considered an optimal cut-off threshold when determining factors to retain (Costello and Osborne, 2005). As factors were expected to correlate, an oblique rotation was employed (Vogt and Johnson, 2011). At each iteration, items were removed where factor loadings were <0.40 (Costello and Osborne, 2005).
Internal Consistency and Descriptive Statistics (Samples 1 and 2)
Internal consistency of the FLS was assessed using the Cronbach's alpha. A lower bound estimate of α = 0.70 was considered acceptable (Nunnally and Bernstein, 1994). The psych package (Revelle, 2016) was used to calculate mean scores and reliabilities for each of the identified factors.
Latent Variable Structural Equation Modeling (Sample 2)
The lavaan package (Rosseel, 2012) was used to perform LVSEM this sought to confirm the factor solution identified from EFA. In addition, and simultaneously in this measurement approach, we hypothesized paths between the latent variables following a consideration of the solution derived from EFA and considering the measurement model hypothesized by Boudrias et al. (2014). Items were free to load onto related latent factors and no restrictions were place on them. Following initial modeling, model fit was improved by adding covariance between error terms. These adjustments followed consideration of modification indices and theory.
Results
Analysis Checks and Participant Characteristics
With the exception of one variable across both samples, skewness and kurtosis values were between −2 and 2. Whilst there is lack of clarity in the literature, skewness and kurtosis values were below “rules of thumb” indicated by Kline (2015), with skewness ≤3, and Kurtosis ≤10. In all cases, such values were well below these thresholds.
Exploratory Factor Analysis (Sample 1)
The KMO statistic for the model was above the 0.50 threshold (KMO = 0.90) and Bartlett's test of sphericity was significant (p < 0.001). Participant characteristics for sample one and two are reported in Table 1.
Three iterations were sufficient to derive simple factor structure. In the first iteration, EFA with an oblique (oblimin) using a maximum likelihood approach, visual inspection of the scree plot and the 0.70 eigenvalue criteria revealed a five-factor solution. However, an unclear factor structure was indicated. Six items reported factor loadings less than the suggested 0.4 criteria. Following the removal of these items, and using the same cut-off criteria, a second iteration revealed a five-factor solution. In this iteration however, simple structure was not achieved, with one further item failing to load on to the five derived factors. Following the removal of this single item, a third and final iteration of the same EFA procedure was undertaken. A five-factor structure converged during the final iteration with 27 individual items retained. Eigenvalues for the respective factors were 7.95, 2.03, 1.12, 0.99, and 0.71. Factor one, made up of eleven items, referencing credible source challenge, for example “the staff who assessed me are outstanding in their capacity to gain my confidence,” accounted for 17% of the total variance in the model. Five items loaded on the second factor accounting for 9% of the total variance. This factor represents one's desire to make behavioral changes and developmental actions resulting from feedback, for example, “following feedback I have searched for developmental activities in line with competencies described during the feedback.” Three items loaded on to factor three, feedback acceptance, an example item includes “I believe the feedback I received depicts me accurately.” This factor again accounted for 9% of the total variance in the model. Four items make up the fourth factor, motivational intentions, an illustrative example suggests “I am motivated to develop myself in the direction of the feedback I received.” This fourth factor accounted for 8% of the variance in the model. Finally, the fifth factor, accounted for 6% of the variance in the model, addressing awareness from feedback; “I am more aware of the strengths that I can draw on from my studies” an indicative item supporting this factor. Item factor loadings are provided in Table 2. As a total, the factors cumulatively explained 49% of the variance in the model. The full 27 item FLS and scoring instructions are provided in Appendix of the current chapter. See Figure 1 for a diagramme depicting the fitted exploratory model. The final iteration indicated an acceptable to good fit to the data (see Figure 1), Normed X2 (X2/df) = 1.11, RMSEA (90% CI) = 0.06 (0.050–0.065), CFI = 0.939, TLI = 0.904, SRMR = 0.03.
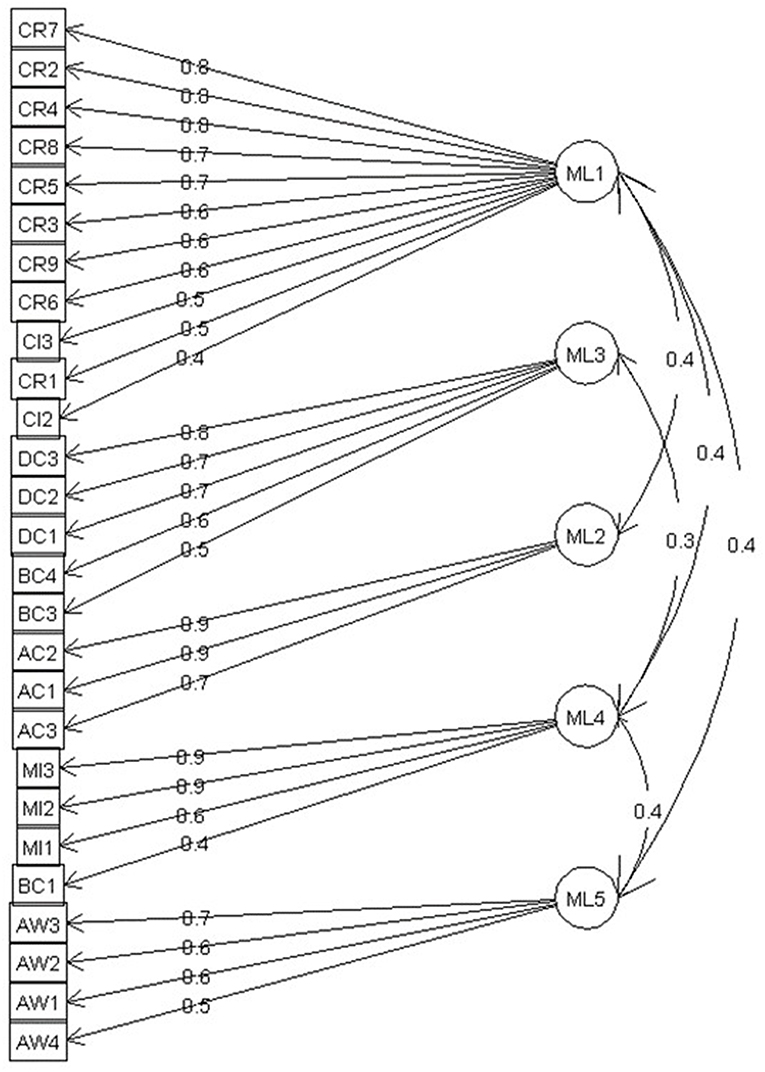
Figure 1. Factor model of FLS with standardized factor loadings represented on unidirectional arrows. Factors in this figure are represented by the following key. ML1, Credible Source Challenge; ML2, Feedback Acceptance; ML3, Behavioral Changes and Developmental Actions; ML4, Motivational Intentions; and ML5, Awareness from Feedback. See Appendix for a detailed key to items.
Internal Consistency and Descriptive Statistics
Subscale scores for both samples in relation to the FLS are reported in Table 3 together with internal consistency, reported using Cronbach's alpha. Inter-item correlations are displayed in Figures 2, 3 for samples 1 and 2, respectively.
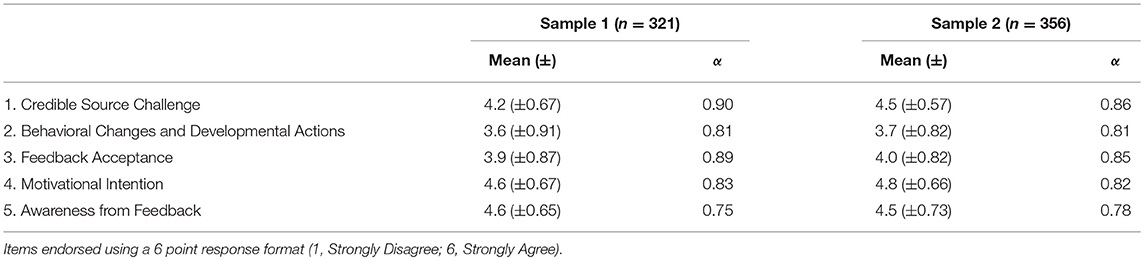
Table 3. Descriptive statistics (where values are means and standard deviation ±) and internal consistency (Cronbach's alpha) for the FLS.
Latent Variable Structural Equation Modeling (Sample 2)
Model Specification
A measurement model was specified that simultaneously confirmed the latent factor structure and examined a unidirectional path through identified factors. In the model specified, from twenty-seven items identified in EFA, eleven were free to load on to the latent factor Credible Source Challenge; five items were free to load on to the latent factor Behavior and Development Change; four items each were free to load on Feedback Acceptance and Motivational Intention, respectively; and the remaining three items were free to load on latent factor Awareness from Feedback. Following consideration of the factor structure, four tentative paths were specified at the latent variable level. These were that feedback acceptance predicts credible source challenge. In turn credible source challenge predicts greater awareness from feedback. Subsequently, it was predicted that awareness from feedback will predict motivational intentions. Our final prediction was that motivational intentions will predict the endorsement of behavioral changes and developmental actions resulting from feedback.
The initial iteration did not achieve acceptable fit to the data without modifications. Following inspection of modification indices, a number of items were allowed to covary due to conceptual congruity, see Appendix for key to items. These include MI2 with MI3, both concern motivations to develop in line with feedback (cov = 0.531, p < 0.001). BC3 with BC4, both items are concerned with seeking out developmental plans (cov = 0.483, p < 0.001); CI2 with CI3, which concern positive challenge interventions (cov = 0.407, p < 0.001). AW2 with AW3 were correlated as they relate to greater self-knowledge and reaction (cov = 0.445, p = 0.001). Standardized factor loadings are presented in Table 2 and indicate that items reflected the underlying latent variable (p < 0.001).
Confirming the Latent Factor Structure
Factors and related items identified in data driven analysis were confirmed in the LVSEM model. Table 2 includes a summary of factors and related item loadings. Table 4 and Figure 4 indicate the associations in the LVSEM of the hypothesized paths between latent variables. In addition, summary factor scores and internal consistency coefficients are reported in Table 3.
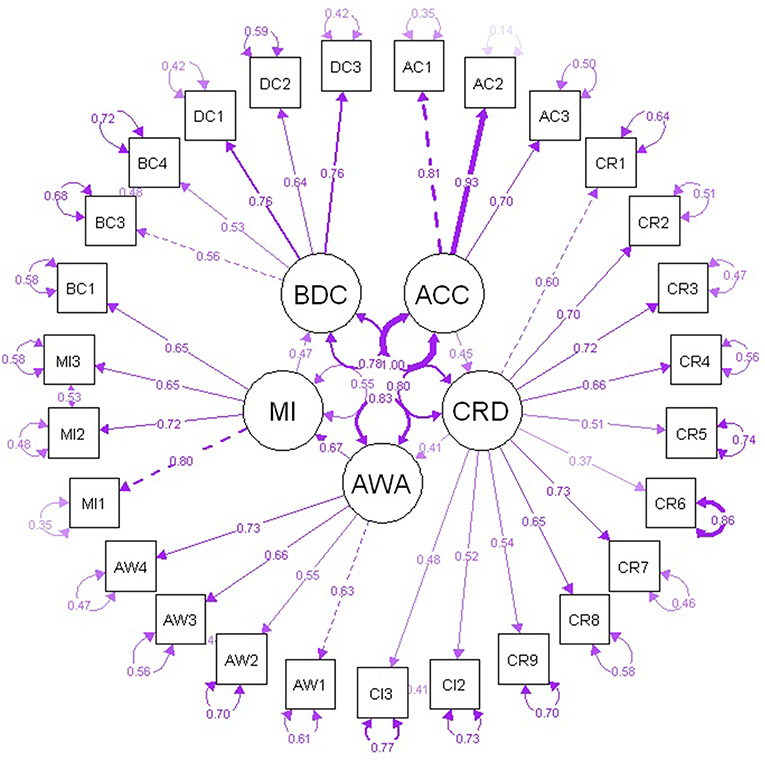
Figure 4. Latent variable structural equation model of FLS with standardized factor loadings (reported on unidirectional arrows), error terms (circled values), and covariances (reported on bidirectional arrows). Factors in this figure are represented by the following key: ACC, Feedback Acceptance; CRD, Credible Source Challenge; AWA, Awareness from Feedback; MI, Motivational Intentions; and BDC, Behavioral Changes and Developmental Actions. See Appendix for a detailed key to items.
Testing Hypothesized Paths
All the paths specified in the model were significant (ps < 0.001). In relation to the first path, feedback acceptance positively predicted credible source challenge (β = 0.45) explaining 21% of the variance in the outcome; a second path found that credible source challenge positively predicted learners awareness from feedback (β = 0.41) explaining 17% of the variance in the outcome; a third positive path found awareness from feedback predicted motivational intention (β = 0.67) explaining 45% of the variance in the outcome; and the final path explained 22% of the variance in behavioral changes and development actions when regressed on motivational intention (β = 0.47).
Following modifications, the final model achieved an acceptable to good fit to the data (see Figure 4). Robust fit statistics using the (Satorra and Bentler, 2010) adjustment with a scaling factor of 1.288 were as follows, normed X2 (X2/df) = 1.59, RMSEA (90% CI) = 0.041 (0.035–0.046), CFI = 0.934, robust TLI = 0.927, SRMR = 0.066. For comparison purposes, unscaled maximum likelihood fit measures were again acceptable or good, and are as follows, normed X2 (X2/df) = 2.05, RMSEA (90% CI) = 0.054 (.048–0.060), CFI = 0.913, TLI = 0.903, SRMR = 0.066.
Consideration of Alternate Latent Variable Structural Equation Models
Three alternate models were explored. In the first such model [A1], the first specified path predicted that credible source challenge led to awareness from feedback. In turn, awareness from feedback was allowed to predict acceptance of feedback. A subsequent path was specified from acceptance to motivational intentions. In the second model [A2], credible source challenge was allowed to predict both awareness from feedback and acceptance of feedback. Both factors in turn predicted motivational intentions. A third model [A3] took a similar linear approach to the hypothesized model; however, in this approach credible source challenge was allowed to predict acceptance of feedback, transposing the order in the hypothesized model. Next, acceptance led to awareness, and then in turn to motivational intentions. In each of alternative models, as with the hypothesized model, behavioral change and developmental action predicted by motivational intentions was specified as the final path.
Remaining constant in all models, modification indices suggested the specification of four covariances between item error terms. Two additional modifications were suggested to the first alternate model. The first of these suggested a path between acceptance of feedback to credible source challenge. The second modification suggested a path from awareness from feedback to motivational intentions. These paths reintroduced the suggested directional paths from the hypothesized model. For comparison purposes, fit measures for each model are presented in Table 5. Whilst fit measures were equivalent or worse when compared to the hypothesized, it was noted that none were superior.
Summary
The current investigation examined the factor structure of a modified measure of feedback integration. Analyses explored and confirmed five latent factors associated with feedback integration by HE learners. Alongside the confirmatory analysis, a hypothesis driven model was reported at least marginally superior fit to alternative models explored. This indicated a directional path through each of the derived five factors. Acceptance of feedback led to credible source challenge, in turn predicting awareness from feedback. Greater awareness subsequently predicted motivational intentions. Finally, motives predicted action. Both models achieved at least acceptable fit to the data, and the paths between factors represented unique proportions of the variance in the model.
Discussion
The current study refined and validated a measure, drawn from the occupational domain, examining the nature of feedback integration in undergraduate learners. A first data driven derived a feedback in learning scale with a five factor structure. The first factor, credible source challenge, addresses the credibility of the source providing feedback and the challenge they provide. Behavioral change and developmental actions, the second factor, represents the learner's desire to take action following feedback. Next, acceptance from feedback considers whether the feedback received is acknowledged by the learner. The penultimate factor represents the motivational intentions in response to feedback. The final fifth factor relates to awareness from feedback, specifically whether learners were more aware of their strengths and weaknesses following feedback. Except where noted, findings largely support factors derived by Boudrias et al. (2014). However, a message valence factor, the extent to which previous feedback was positive or negative, was discarded during exploratory analysis.
A latent variable structural equation modeling approach, was used to address a second research aim. First, the latent factor structure identified in the first exploratory investigation was confirmed. Conjointly, four hypothesized paths were proposed between each of the five latent factors following a consideration of theory and the model indicated by Boudrias et al. (2014). Our hypotheses were that learner acceptance of feedback would predict the learners view that the source of feedback provided credible challenge. Subsequently, we proposed that this trust in the source of feedback would predict awareness in learners. In turn, our third hypothesized path indicated that the level of awareness would predict learners motivational intentions in respect of feedback. The final, fourth path hypothesized that behavioral changes and development actions in response to feedback would be predicted by motivational intentions. Supporting our suggestions, significant associations were seen for all hypothesized paths with medium to large effects seen across all paths. Necessary minor modifications saw the hypothesized model achieve at least acceptable, and mostly good, fit. In both samples, the suggested factors demonstrated acceptable internal reliability. Endorsement of factors was consistent across both samples. Learners reported moderate to high levels of awareness from feedback and motivational intentions, followed by trust in the source of feedback. More variability and lower scores were exhibited in relation to acceptance and lowest in relation to behavior change and developmental actions. These findings indicate that learners feel more aware and motivated once they have engaged in feedback, they are less sure of how to change or access development activities securing the next steps. This supports the pattern of results found by Boudrias et al. (2014) who suggested that development activities and behavioral change may not always be perceived to be under the control of those receiving feedback. As research indicates undergraduate learners appear to be hostage to poor strategy and planning processes (Winstone et al., 2017b), supporting learners with pedagogies that promote the identification of appropriate strategies, such as planning future action may be critical to integrating feedback (Winstone et al., 2019). Instructors that provide appropriately challenging feedback are critical to this process (Forsythe and Johnson, 2017; Carless and Boud, 2018). Developing approaches that extend beyond immediate learning which support learners into employment would appear an appropriate aim if learners are to become effective graduates (Ajjawi et al., 2018; Carless, 2019).
Although one model is reported in the current study, alternate explanatory models were examined following good practice (Crede and Harms, 2019). Whilst some indication of equivalence fit measures was observed between models, none of the models examined were superior to the hypothesized model. The hypothesized model is parsimonious and aligns well with Boudrias et al. (2014) previous findings. Nevertheless, future research should consider that alternate models may be plausible. Data for the current study are open and as a result developments in theory may give rise to further testing, as recommended by Crede and Harms (2019).
Findings from the current study speak to five factors associated with feedback integration in tertiary learning. These findings are particularly noteworthy as they highlight the importance of raising learner awareness of strengths and challenges as a central role for intervention. Awareness from feedback is seen to relate directly to learners' motivational intentions, which accounted for the greatest proportion of the variance in the path model. Learner motives led to behavioral changes and developmental actions endorsed by learners following feedback. Further, these findings suggest that learners may seek out additional feedback and action plans from credible sources of information. This understanding may come from a credible source, such as a tutor or a trusted peer. The relationships seen in the current study appear to address the three considerations highlighted by Hattie and Timperley (2007). These suggest that to integrate feedback learners need to understand where and how they are going, together with an evaluation necessary to operationalize awareness in to action (Ajjawi et al., 2018). Although learners endorsed motivated intentions and actions, being motivated to carry out an action may not necessarily lead to the desired action during goal striving (Gollwitzer, 1999). However, in models of self-regulation (Zimmerman, 2000), adaptive evaluations and the resulting motivations following task performance are suggested to lead to the setting of more challenging and specific subsequent goals. Although this is untested in the current study, feedback data from a trusted, reliable source only has utility if it is acted upon. In some undergraduates learners self-regulatory skills are not well-developed (Zimmerman and Paulsen, 1995), it is further suggested that the learners ability to control the course of action may increasingly be compromised (Duckworth et al., 2019). These results indicate that tertiary learners equipped with greater awareness subsequently hold greater motivational intentions. In turn, these motivations are associated with subsequent intention to take action.
Supporting recent theoretical models, factors including the increasing self-awareness, goal setting, and engagement and motivation are also established as central forces in recent models of feedback recipience (Winstone et al., 2017a,b). This addresses the idea of motivational intention in the current model. Although goal setting is not addressed directly in the current approach, HE learners appear to possess a sense of where they are going in their endorsement of behavioral changes and developmental actions resulting from feedback. Goal setting and volitional action have been endorsed as a central pillar of the SAGE model of feedback integration (Winstone et al., 2017a). Evidence to support the importance of this assertion is somewhat limited, as noted by the authors. Goal setting has previously been highlighted as a possible intervention route, for example to promote learner response to feedback (Evans, 2013), and as a route to bolstering agentic beliefs, such as self-efficacy (Richardson et al., 2012; Morisano, 2013). Despite there being a prima facie case to support the role of goal setting, this remains a fruitful area for investigation; as a result, we highlight the need for further research in this area. As indicated, the findings of the current study appear to align well with models of self-regulated learning which suggest reciprocal causality between planning, action and evaluation (Zimmerman, 2000; Panadero, 2017). These also align well with workplace models of self-regulation (Lord et al., 2010). Increasing awareness, may lead to greater motivation, which in turn may lead to improved planning processes in a virtuous cycle.
Using the measure developed and validated here for diagnosis and intervention will prove useful as a cost effective route to identifying and addressing maladaptive behaviors. For example, the FLS is a tool that facilitates identification of learners with lower levels of acceptance, trust, awareness, motivational intent, and desire to act in response to feedback. Following identification, addressing suboptimal feedback behaviors using appropriate pedagogies appears to be an effective mechanism to assist learners in developing the evaluative judgments that are necessary to optimize learning (Winstone et al., 2019). The ability to be able to accept feedback, in particular how this is associated with the ability to trust the source of challenge and feedback, was endorsed in the feedback measure. These relationships have previously been discussed in terms of modeling feedback behaviors and building improved relationships, which are often perceived as distant (Evans, 2013; Pitt and Norton, 2017; Carless and Boud, 2018). The SAGE model also highlights the importance of interpersonal characteristics as a route to proactive feedback response (Winstone et al., 2017a). The emergence of five key factors in the FLS operationalize an economic model of feedback integration that appears to assist in understanding student responses to feedback.
Despite providing a parsimonious model of feedback integration, the current study has its limitations. The model of feedback integration reported here represents one model of feedback integration, it is possible that any number of other hypothetical models may account for the data just as well, and possibly better. Although, this approach aligns well with theories of self-regulated learning (Zimmerman, 2000; Panadero, 2017), we are not aware of similar measures that can be used to measure perceptions and changes in attitudes and behaviors over time. A strength of the approach is that having modified the original measure, many of the items and similar latent factors were retained. In addition, similar paths are seen. This suggests a common approach between the domains in integrating feedback, which will benefit HE learners when they enter the graduate workforce. We increased statistical power across both samples, when compared to Boudrias et al. (2014) original measure. This allowed for latent variable estimation, which was not possible in the source measure, and potentially provides a more a more robust model in this investigation. These results are however derived from two separate samples of psychology students within the same tertiary education setting. This, and the gender imbalance, may limit the results. As a result, examining this measure in other disciplines, with other samples of students, will further establish its utility as a measure of feedback integration within HE learning. We attempted to broaden the participant base, by recruiting from undergraduate learners at different stages of their undergraduate career, albeit these were drawn from the same setting and course. Finally, findings here are based on two cross-sectional samples of data, whilst tentative casual paths were specified in the second model, only longitudinal or experimental research can support suggested regression paths seen in the path model.
Conclusion
In summary, the current investigation indicates that the FLS represents a valid and reliable measure of feedback integration behaviors in undergraduate learners. Three aligned practical implications of the FLS are suggested. Firstly, the measure may assist in identifying active components associated with feedback integration in undergraduate learners. Using the FLS for identification of behaviors and change over time, as a meaningful mechanism for capturing gains in learning provides a useful tool to promote further research. In addition, using the FLS as part of interventions and pedagogies to raise learner self-awareness may support learners to take the steps necessary to evaluate and make necessary changes to optimize learning. Future research is necessary to validate the FLS as reliable tool in other tertiary settings to determine if the measure has utility beyond the current setting and domain of learning. However, these ideas are consistent with theory (Zimmerman, 2000; Panadero et al., 2017), and have important implications for practice by providing an supplementary tool to encourage integration of feedback in HE learners (Nicol and Macfarlane-Dick, 2006; Evans, 2013; Winstone et al., 2017a).
Data Availability
All datasets generated for this study are included in the manuscript and/or the Supplementary Files.
Ethics Statement
This study was carried out in accordance with the recommendations of The British Psychological Society, with written informed consent from all subjects. All subjects gave written informed consent in accordance with the Declaration of Helsinki. The protocol was approved by the Institute of Psychology, Health and Society Research Ethics Committee of The University of Liverpool.
Author Contributions
MJ designed the study, analyzed the data, and led the writing of the article. AF supervised, helped in designing the study, and aided in writing the article.
Funding
On acceptance, publications fees will be funded by the University of Liverpool institutional fund for open access payments.
Conflict of Interest Statement
The authors declare that the research was conducted in the absence of any commercial or financial relationships that could be construed as a potential conflict of interest.
Supplementary Material
The Supplementary Material for this article can be found online at: https://www.frontiersin.org/articles/10.3389/feduc.2019.00084/full#supplementary-material
References
Ajjawi, R., Tai, J., Dawson, P., and Boud, D. (2018). “Conceptualising evaluative judgement for sustainable assessment in higher education,” in Developing Evaluative Judgement in Higher Education: Assessment for Knowing and Producing Quality Work, eds D. Boud, R. Ajjawi, P. Dawson, and J. Tai (Abingdon: Routledge), 23–33. doi: 10.4324/9781315109251-2
Ajzen, I. (1996). “The social psychology of decision making,” in Social Psychology: Handbook of Basic Principles, eds E. T. Higgins and A. W. Kruglanski (New York, NY: Guilford Press, 297–325.
Ashford, S. J., and De Stobbeleir, K. E. M. (2013). “Feedback, goal-setting, and task performance revisited,” in New Developments in Goal-Setting and Task Performance, eds E. A. Locke and G. P. Latham (Hove: Routledge, 51–64.
Bentler, P. M. (1990). Comparative fit indexes in structural models. Psychol. Bull. 107:238. doi: 10.1037/0033-2909.107.2.238
Boudrias, J.-S., Bernaud, J.-L., and Plunier, P. (2014). Candidates' integration of individual psychological assessment feedback. J. Manag. Psychol. 29, 341–359. doi: 10.1108/JMP-01-2012-0016
Brookhart, S. M. (2018). Appropriate criteria: key to effective rubrics. Front. Educ. 3:22. doi: 10.3389/feduc.2018.00022
Bunce, L., Baird, A., and Jones, S. E. (2017). The student-as-consumer approach in higher education and its effects on academic performance. Stud. High. Educ. 42, 1958–1978. doi: 10.1080/03075079.2015.1127908
Carless, D. (2019). Feedback loops and the longer-term: towards feedback spirals. Assess. Eval. High. Educ. 44, 705–714. doi: 10.1080/02602938.2018.1531108
Carless, D., and Boud, D. (2018). The development of student feedback literacy: enabling uptake of feedback. Assess. Eval. High. Educ. 43, 1315–1325. doi: 10.1080/02602938.2018.1463354
Cattell, R. B. (1966). The scree test for the number of factors. Multivar. Behav. Res. 1, 245–276. doi: 10.1207/s15327906mbr0102_10
Costello, A. B., and Osborne, J. W. (2005). Best practices in exploratory factor analysis: four recommendations for getting the most from your analysis. Pract. Assess. Res. Eval. 10, 1–9. Available online at: http://pareonline.net/getvn.asp?v=10&n=7
Crede, M., and Harms, P. (2019). Questionable research practices when using confirmatory factor analysis. J. Manag. Psychol. 34:15. doi: 10.1108/JMP-06-2018-0272
Diefendorff, J. M., and Lord, R. G. (2008). “Goal-striving and self-regulation processes,” in Work Motivation: Past, Present, and Future, eds R. Kanfer, G. Chen, and R. D. Pritchard (New York, NY: Routledge, 151–196.
Duckworth, A. L., Taxer, J. L., Eskreis-Winkler, L., Galla, B. M., and Gross, J. J. (2019). Self-control and academic achievement. Annu. Rev. Psychol. 70, 373–399. doi: 10.1146/annurev-psych-010418-103230
Evans, C. (2013). Making sense of assessment feedback in higher education. Rev. Educ. Res. 83, 70–120. doi: 10.3102/0034654312474350
Evans, C., Howson, C. K., and Forsythe, A. (2018). Making sense of learning gain in higher education. High. Educ. Pedag. 3, 1–45. doi: 10.1080/23752696.2018.1508360
Farrell, L., Bourgeois-Law, G., Buydens, S., and Regher, G. (2019). Your goals, my goals, our goals: the complexity of coconstructing goals with learners in medical education. Teach. Learn. Med. 31, 370–377. doi: 10.1080/10401334.2019.1576526
Flake, J. K., and Fried, E. I. (2019). Measurement Schmeasurement: Questionable Measurement Practices and How to Avoid Them. doi: 10.31234/osf.io/hs7wm
Flake, J. K., Pek, J., and Hehman, E. (2017). Construct validation in social and personality research: current practice and recommendations. Soc. Psychol. Pers. Sci. 8, 370–378. doi: 10.1177/1948550617693063
Forsythe, A., and Jellicoe, M. (2018). Predicting gainful learning in Higher Education; a goal-orientation approach. High. Educ. Pedag. 3, 82–96. doi: 10.1080/23752696.2018.1435298
Forsythe, A., and Johnson, S. (2017). Thanks, but no-thanks for the feedback. Assess. Eval. High. Educ. 42, 850–859. doi: 10.1080/02602938.2016.1202190
Gana, K., and Broc, G. (2019). Structural Equation Modeling With Lavaan. Hoboken, NJ: John Wiley & Sons. doi: 10.1002/9781119579038
Gollwitzer, P. M. (1999). Implementation intentions: strong effects of simple plans. Am. Psychol. 54:493. doi: 10.1037/0003-066X.54.7.493
Hattie, J., Biggs, J., and Purdie, N. (1996). Effects of learning skills interventions on student learning: a meta-analysis. Rev. Educ. Res. 66, 99–136. doi: 10.3102/00346543066002099
Hattie, J., and Timperley, H. (2007). The power of feedback. Rev. Educ. Res. 77, 81–112. doi: 10.3102/003465430298487
Hu, L., and Bentler, P. M. (1999). Cutoff criteria for fit indexes in covariance structure analysis: conventional criteria versus new alternatives. Struct. Equat. Model. Multidiscipl. J. 6, 1–55. doi: 10.1080/10705519909540118
Hughes, G. (2011). Towards a personal best: a case for introducing ipsative assessment in higher education. Stud. High. Educ. 36, 353–367. doi: 10.1080/03075079.2010.486859
Jolliffe, I. T. (1972). Discarding variables in a principal component analysis. I: artificial data. Appl. Stat. 21, 160–173. doi: 10.2307/2346488
Joughin, G., Boud, D., and Dawson, P. (2018). Threats to student evaluative judgement and their management. High. Educ. Res. Dev. 38, 537–549. doi: 10.1080/07294360.2018.1544227
Kahneman, D., and Tversky, A. (1979). Prospect theory: an analysis of decision under risk. Econometrica 47, 263–291. doi: 10.2307/1914185
Kahneman, D., and Tversky, A. (1984). Choices, values, and frames. Am. Psychol. 39, 341–350. doi: 10.1037/0003-066X.39.4.341
Kenny, D. A. (2015). Measuring Model Fit. Retrieved from: http://www.davidakenny.net/cm/fit.htm (accessed January 16, 2019).
Kline, R. B. (2015). Principles and Practice of Structural Equation Modeling, 4th Edn. New York, NY: Guilford Publications.
Kluger, A. N., and DeNisi, A. (1996). The effects of feedback interventions on performance: a historical review, a meta-analysis, and a preliminary feedback intervention theory. Psychol. Bull. 119:254. doi: 10.1037/0033-2909.119.2.254
Kluyver, T., Ragan-Kelley, B., Pandeacuterez, F., Granger, B., Bussonnier, M., Jonathan, F., and Jupyter Development Team (2016). “Jupyter notebooks; a publishing format for reproducible computational workflows,” in Positioning and Power in Academic Publishing: Players, Agents and Agendas, eds F. Loizides and B. Schmidt (Amsterdam: IOS Press, 87–90.
Kudisch, J. D. (1996). Factors related to participant's acceptance of developmental assessment center feedback (Doctoral thesis). University of Tennessee, Knoxville, TN, United States.
Locke, E. A., and Latham, G. P. (1990). A Theory of Goal Setting & Task Performance. Englewood Cliffs, NJ: Prentice-Hall, Inc.
Lord, R. G., Diefendorff, J. M., Schmidt, A. M., and Hall, R. J. (2010). Self-regulation at work. Annu. Rev. Psychol. 61, 543–568. doi: 10.1146/annurev.psych.093008.100314
MacCallum, R. C., Browne, M. W., and Sugawara, H. M. (1996). Power analysis and determination of sample size for covariance structure modeling. Psychol. Methods 1:130. doi: 10.1037/1082-989X.1.2.130
Morisano, D. (2013). “Goal setting in the academic arena,” in New Developments in Goal Setting and Task Performance, eds E. A. Locke and G. P. Latham (Hove: Routledge, 495–506.
Nicol, D. J., and Macfarlane-Dick, D. (2006). Formative assessment and self-regulated learning: a model and seven principles of good feedback practice. Stud. High. Educ. 31, 199–218. doi: 10.1080/03075070600572090
Nunnally, J. C., and Bernstein, I. H. (1994). Psychometric Theory (McGraw-Hill Series in Psychology), Vol. 3. New York, NY: McGraw-Hill New York.
O'Donovan, B., Rust, C., and Price, M. (2016). A scholarly approach to solving the feedback dilemma in practice. Assess. Eval. High. Educ. 41, 938–949. doi: 10.1080/02602938.2015.1052774
Panadero, E. (2017). A review of self-regulated learning: six models and four directions for research. Front. Psychol. 8:422. doi: 10.3389/fpsyg.2017.00422
Panadero, E., Broadbent, J., Boud, D., and Lodge, J. M. (2018). Using formative assessment to influence self-and co-regulated learning: the role of evaluative judgement. Eur. J. Psychol. Educ. 34, 535–557. doi: 10.1007/s10212-018-0407-8
Panadero, E., Jonsson, A., and Botella, J. (2017). Effects of self-assessment on self-regulated learning and self-efficacy: four meta-analyses. Educ. Res. Rev. 22, 74–98. doi: 10.1016/j.edurev.2017.08.004
Peverly, S. T., Brobst, K. E., Graham, M., and Shaw, R. (2003). College adults are not good at self-regulation: a study on the relationship of self-regulation, note taking, and test taking. J. Educ. Psychol. 95, 335–346. doi: 10.1037/0022-0663.95.2.335
Pitt, E., and Norton, L. (2017). ‘Now that's the feedback I want!' Students' reactions to feedback on graded work and what they do with it. Assess. Eval. High. Educ. 42, 499–516. doi: 10.1080/02602938.2016.1142500
Qualtrics (2018). Qualtrics. Retrieved from: https://www.qualtrics.com
R Core Team (2013). R: A Language and Environment for Statistical Computing. Vienna: R Foundation for Statistical Computing.
Revelle, W. (2016). psych: Procedures for Personality and Psychological Research (Version Version R package version 1.6.4.). Retrieved from: http://cran.r-project.org/web/packages/psych/
Richardson, M., Abraham, C., and Bond, R. (2012). Psychological correlates of university students' academic performance: a systematic review and meta-analysis. Psychol. Bull. 138:353. doi: 10.1037/a0026838
Rosseel, Y. (2012). Lavaan: An R package for structural equation modeling and more. Version 0.5–12 (BETA). J. Stat. Softw. 48, 1–36. doi: 10.18637/jss.v048.i02
Satorra, A., and Bentler, P. M. (2010). Ensuring positiveness of the scaled difference chi-square test statistic. Psychometrika 75, 243–248. doi: 10.1007/s11336-009-9135-y
Stanovich, K. E., and West, R. F. (2000). Individual differences in reasoning: implications for the rationality debate? Behav. Brain Sci. 23, 645–665. doi: 10.1017/S0140525X00003435
Stone, D., and Heen, S. (2015). Thanks for the Feedback: The Science and Art of Receiving Feedback Well (Even When It Is Off Base, Unfair, Poorly Delivered, and Frankly, You're Not in the Mood). New York, NY: Penguin.
The Confederation of British Industry (2016). The Right Combination: CBI/Pearson Education and Skills Survey 2016. London: The Confederation of British Industry.
Ullman, S. (2001). “Structural equation modeling,” in Using Multivariate Statistics, Vol. 4, eds B. G. Tabachnick and L. S. Fidell (Needham Heights, MA: Allyn and Bacon, 653–771.
Van Merriënboer, J. J., and Kirschner, P. A. (2017). Ten Steps to Complex Learning: A Systematic Approach to Four-Component Instructional Design, 3rd Edn. New York, NY: Routledge. doi: 10.4324/9781315113210
Vogt, W. P., and Johnson, B. (2011). Dictionary of Statistics & Methodology: A Nontechnical Guide for the Social Sciences, 4th Edn. Thousand Oaks, CA: Sage.
Winstone, N. E., Mathlin, G., and Nash, R. A. (2019). Building feedback literacy: students' perceptions of the developing engagement with feedback toolkit. Front. Educ. 4:39. doi: 10.3389/feduc.2019.00039
Winstone, N. E., Nash, R. A., Parker, M., and Rowntree, J. (2017a). Supporting learners' agentic engagement with feedback: a systematic review and a taxonomy of recipience processes. Educ. Psychol. 52, 17–37. doi: 10.1080/00461520.2016.1207538
Winstone, N. E., Nash, R. A., Rowntree, J., and Menezes, R. (2016). What do students want most from written feedback information? Distinguishing necessities from luxuries using a budgeting methodology. Assess. Eval. High. Educ. 41, 1237–1253. doi: 10.1080/02602938.2015.1075956
Winstone, N. E., Nash, R. A., Rowntree, J., and Parker, M. (2017b). “It'd be useful, but I wouldn't use it”: barriers to university students' feedback seeking and recipience. Stud. High. Educ. 42, 2026–2041. doi: 10.1080/03075079.2015.1130032
Zimmerman, B. J. (2000). “Attaining self-regulation: a social cognitive perspective,” in Handbook of Self-Regulation, eds M. Boekaerts and P. R. Pintrich (San Diego, CA: Academic Press), 13–39. doi: 10.1016/B978-012109890-2/50031-7
Zimmerman, B. J., and Paulsen, A. S. (1995). Self-monitoring during collegiate studying: an invaluable tool for academic self-regulation. New Direct. Teach. Learn. 1995, 13–27. doi: 10.1002/tl.37219956305
Appendix
Keywords: feedback integration, higher education, self regulated learning, learning gain, scale development and psychometric evaluation
Citation: Jellicoe M and Forsythe A (2019) The Development and Validation of the Feedback in Learning Scale (FLS). Front. Educ. 4:84. doi: 10.3389/feduc.2019.00084
Received: 12 March 2019; Accepted: 29 July 2019;
Published: 14 August 2019.
Edited by:
Anastasiya A. Lipnevich, The City University of New York, United StatesReviewed by:
Wei Shin Leong, Nanyang Technological University, SingaporeJana Groß Ophoff, University of Tübingen, Germany
Copyright © 2019 Jellicoe and Forsythe. This is an open-access article distributed under the terms of the Creative Commons Attribution License (CC BY). The use, distribution or reproduction in other forums is permitted, provided the original author(s) and the copyright owner(s) are credited and that the original publication in this journal is cited, in accordance with accepted academic practice. No use, distribution or reproduction is permitted which does not comply with these terms.
*Correspondence: Mark Jellicoe, bWFyay5qZWxsaWNvZUBsaXZlcnBvb2wuYWMudWs=