- Department of Biology Education, University of Kassel, Kassel, Germany
Inquiry-based learning can be considered a critical component of science education in which students can assess their understanding of scientific concepts and scientific reasoning skills while actively constructing new knowledge through different types of activity levels (Klahr and Dunbar, 1988; Bell et al., 2005; Hmelo-Silver et al., 2007; Mayer, 2007). However, engaging in inquiry activities can be cognitively demanding for students, especially those with low prior knowledge of scientific reasoning skills (reasoning ability). Learning new information when preexisting schemata are absent entails more interacting elements and thus imposes a high working memory load, resulting in lower long-term learning effects (Paas and van Merriënboer, 1994; Kirschner et al., 2006). Borrowing knowledge from others via video modeling examples before carrying out an inquiry task provides learners with more working memory capacity to focus on problem-solving strategies and construct useful cognitive schemata for solving subsequent (virtual) inquiry tasks (Kant et al., 2017). The goal of the present study (N = 174 6/7th graders) is to investigate the benefits of combining example-based learning with physical, hands-on investigations in inquiry-based learning for acquiring scientific reasoning skills. The study followed a 2 (video modeling example vs. no example) × 2 (guided vs. structured inquiry) × 2 (retention interval: immediate vs. delayed) mixed-factorial design. In addition, the students' need for cognition (Preckel, 2014), cognitive abilities (Heller and Perleth, 2000) (intrinsic, extraneous, and germane) cognitive load (Cierniak et al., 2009) and performance success were measured. Although the results of an intermediate test after the first manipulation were higher among students who watched a video modeling example (d = 0.97), combining video modeling examples with inquiry was not found to benefit performance success. Furthermore, regardless of manipulation, all students achieved equal results on an assessment immediately following the inquiry task. Only in the long run did a video modeling example prove to be advantageous for guided inquiry (ηp2 = 0.023). A video modeling example turned out to be a crucial prerequisite for the long-term effectiveness of guided inquiry because it helped create stable problem-solving schemata; however, the long-term retention of structured inquiry did not rely on a video modeling example.
Introduction
Scientific reasoning is an essential component of science education standards in many countries (OECD, 2007; National Research Council, 2013). Two distinct teaching approaches have been employed to foster scientific reasoning skills in school that appear contradictory at first glance: inquiry-based learning (see section Inquiry-Based Learning) and example-based learning (see section The Relevance and Effectiveness of Example-Based Learning).
In inquiry-based learning, learners actively construct knowledge by investigating scientific phenomena (Klahr and Dunbar, 1988; Hmelo-Silver et al., 2007; Mayer, 2007). Although meta-analyses have revealed (relatively modest) benefits of inquiry-based learning in science (Furtak et al., 2012), other studies have revealed an overload of working memory capacity (e.g., Kirschner et al., 2006). High levels of inquiry, such as open inquiry, are highly cognitively demanding and can overstrain working memory resources, particularly among novice students.
In contrast, in example-based learning, students simply receive an example illustrating how a specific model can be used to solve a scientific problem. This approach is rooted in the notion that learners are more likely to focus on crucial aspects and procedures when they observe examples containing helpful strategies before encountering problems they must solve themselves. However, passively studying examples to reduce the cognitive load might create illusions of understanding, which might in turn inhibit the learning process (Baars et al., 2018) or even result in the expertise reversal effect (Kalyuga et al., 2003) when learners' level of expertise is already high (see section The Roles of Cognitive Load and Prior Knowledge). Thus, along with their many advantages, both approaches have limitations that can be explained with reference to cognitive load theory (see section The Roles of Cognitive Load and Prior Knowledge).
According to van Gog et al. (2011), the provision of an example before a problem-solving task is more effective than problem-solving alone. Kirschner et al. (2006) recommend the use of worked examples as effective methods for guided learning. However, only a few studies have analyzed the effect of example-based learning on a special form of problem-solving, inquiry-based learning (Mulder et al., 2014; Kant et al., 2017). The present study investigates the need for video modeling examples (combining features of modeling examples and worked examples, Leahy and Sweller, 2011) prior to participation in two different levels of inquiry involving less (guided inquiry) or more guidance (structured inquiry). In addition to the effect of the combination of video modeling examples and inquiry on short-term retention (immediate performance), the potential long-term benefit (7 days after the inquiry task) is particularly interesting.
Inquiry-Based Learning
Previous research has found that inquiry-based learning can be more effective than direct instruction (Alfieri et al., 2011). In inquiry-based scientific investigations, students solve authentic scientific problems (e.g., investigating the impact of light on the growth of plants) in a collaborative form of learning in which they apply both content-related knowledge and methodological skills (inquiry skills/scientific reasoning skills). After generating hypotheses and planning appropriate experiments, students actively conduct these experiments and analyze the results to answer their scientific questions (Klahr and Dunbar, 1988; Klahr, 2000; Mayer and Ziemek, 2006; Mayer, 2007). The degree of activity or open-endedness in both the methodological and content phases is associated with students' autonomy and the amount of instructional support or teacher input (Table 1). In open inquiry, the students themselves manage their learning process, like real scientists (Bell et al., 2005). They independently formulate research questions, design and conduct investigations, and analyze their results. At the second highest level, guided inquiry, students investigate a teacher-provided question using an experimental plan they develop themselves. They also conduct the investigations and interpret their results with teacher guidance and support (e.g., scaffolding and feedback). In structured inquiry, both the research question and an appropriate experimental plan are provided by the teacher, but students are asked to generate their own explanations for the results they obtain. In verification inquiry, students are provided with the maximum level of guidance and instructional support; they merely conduct the experiment to verify already known results. Thus, at a low activity level, students primarily passively receive instructions, whereas a high activity level involves many different prompts for students to generate new knowledge and thus a maximum level of student output. Based on the results of a meta-analysis by Lazonder and Harmsen (2016), students must be adequately supported to achieve higher performance success (d = 0.71, 95% CI [0.52, 0.90]) and learning outcomes (d = 0.50, 95% CI [0.37, 0.62]) and to increase learners' involvement in learning/learning activities (d = 0.66, 95% CI [0.44, 0.88]). Guidance and support are needed to compensate for learners' low prior knowledge or poor scientific reasoning skills. Therefore, guided and structured inquiry are the most common, powerful and effective inquiry levels used in practice (Hmelo-Silver et al., 2007).
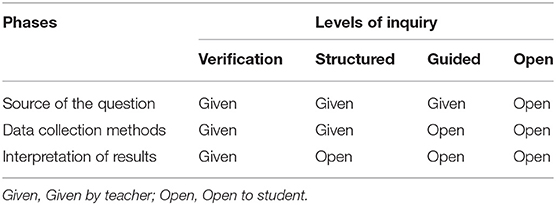
Table 1. Levels of inquiry (Abrams et al., 2008) adapted from Schwab (1962) and Colburn (2000).
The inquiry level can vary both with respect to the content phases, which convey domain-specific concepts, and the methodological phases, which promote scientific reasoning skills. A focus on scientific reasoning is a key recommendation of international science education standards (OECD, 2007; National Research Council, 2013) to promote students' understanding of scientific and technical issues in our society and their active participation in society. Scientific reasoning involves hypothesizing, planning, experimenting, evaluating and communicating the results of investigations (National Research Council, 2013). Insights into the basic rules of unconfounded evidence and their value are a crucial element of the inquiry process and scientific reasoning (Chen and Klahr, 1999; Kuhn and Dean, 2005). This essential scientific reasoning skill has a critical contribution to science education and is known as the control of variables strategy (CVS) (Linn et al., 1981; Chen and Klahr, 1999). It refers to one's ability to plan a controlled experiment by holding exogenous variables constant and examining one or more factor(s) of interest. The application of this strategy substantially curtails the number of options available from the experiment space, which consists of all experiments that could potentially be performed (Klahr and Dunbar, 1988). Moreover, the use of this strategy requires an ability to differentiate between confounded and unconfounded experiments in order to evaluate the evidence for and against scientific propositions (Zimmerman et al., 1998). Debate and controversy exist regarding the most effective approach to use in teaching CVS. In some studies, learners are allowed to obtain more knowledge about a system's function through unguided exploration, as is typical in open inquiry, leading to higher learning outcomes (Vollmeyer and Burns, 1996), while other studies show that unguided discovery methods are less effective in teaching CVS (Klahr and Nigam, 2004; Alfieri et al., 2011). Furthermore, the principles of unconfounded evidence are not learned automatically; explicit practice is needed (Sneider et al., 1984; Schwichow et al., 2016).
Regardless of the inquiry level at which investigations are conducted, inquiry-based learning is characterized by active engagement. Nevertheless, dynamic, effortful active learning techniques, such as generating knowledge in a hands-on inquiry-based learning environment, require a considerable investment of cognitive effort and time, as they are characterized by a high degree of complexity (Clark and Linn, 2003). Generation requirements such as those found in authentic learning settings impede learning, as their greater open-endedness correlates with a higher cognitive burden (Kirschner et al., 2006; Chen et al., 2016). Receiving instructional guidance via examples on how to solve an inquiry task can reduce the degree of complexity and result in better performance than solving problems without any examples (e.g., Aleven, 2002; McLaren et al., 2008; van Gog et al., 2009), a learning approach referred to as example-based learning. According to the borrowing and reorganizing principle, highly structured problem-solving strategies are best learned from other people (Sweller and Sweller, 2006). This approach prevents learners from overstraining their cognitive resources with incorrect problem-solving strategies (Sweller and Sweller, 2006).
The Relevance and Effectiveness of Example-Based Learning
Example-based learning distinguishes between two forms of examples (van Gog and Rummel, 2010; Renkl, 2014): worked examples (Sweller and Cooper, 1985; Cooper and Sweller, 1987; Sweller et al., 1998; Schwonke et al., 2009), in which each step of the procedure used to solve a problem is explained in a text-based manner, and modeling examples (Bandura, 1977, 1986; Collins et al., 1989), in which a model demonstrates and/or explains how to complete a problem-solving task. Worked examples are effective in promoting problem-solving strategies and integrating new with prior knowledge (Roth et al., 1999). They are one of the most time-efficient, effective and widely used instructional learning strategies, particularly in the initial stages of skill acquisition (vanLehn, 1996; Salden et al., 2010). Experiments have repeatedly demonstrated the worked example effect (e.g., Renkl, 1997; Atkinson et al., 2000; Sweller et al., 2011), mainly in fields such as algebra (Sweller and Cooper, 1985) and computer programming (Kalyuga et al., 2001)—domains that are clearly defined, well-structured (mostly iterative), and can be investigated in laboratory studies. More recently, positive effects have also been observed on scientific reasoning (Mulder et al., 2014; Kant et al., 2017). The basic structure of a worked example typically includes three crucial components: (1) examining the key problem to raise awareness of the problem to be solved, (2) explaining the procedure for solving the problem through the completion of a certain number of steps in a specific order to promote the construction of appropriate schemata, and (3) describing the final solution to the problem (Renkl, 1997). After completing all three steps, learners are asked to solve a similar problem on their own to enhance the automation of their problem-solving skills and ensure transfer (Atkinson et al., 2000).
The effect of worked examples is rooted in cognitive load theory (see section The Roles of Cognitive Load and Prior Knowledge). Worked examples provide learners with full guidance concerning the key steps required to solve a problem, thus automatically drawing learners' attention to relevant aspects that form a basis for subsequent problem-solving. These examples allow appropriate cognitive schemata to be developed (Crippen and Earl, 2007; Schworm and Renkl, 2007) before learners are confronted with actual problem-solving demands and information. Sweller and Cooper (1985) claim that worked examples lead to better learning of solution procedures. While studying problems with detailed solutions provides learners with a basic understanding of domain-specific principles, the conventional problem-solving method focuses on searching for processes rather than on aspects crucial to the acquisition of cognitive schemata (Sweller and Cooper, 1985).
A main difference between worked examples and modeling examples concerns attentional focus (Hoogerheide et al., 2014). Modeling examples provide learners with the opportunity to observe a model solving a task without explicitly focusing on relevant aspects or dividing the procedure into individual steps. This approach requires learners to selectively focus on the most critical elements of the demonstrated behavior. The observed information is actively organized and integrated with the learner's prior knowledge during a constructive process. However, the nature of learners' cognitive representations and the level at which they possess the component skills determines whether learners are able to effectively apply the observed strategies (Bandura, 1986). Previously, modeling examples have mainly been used to convey (psycho) motor skills (e.g., Blandin et al., 1999) and skills with low levels of structure (e.g., Braaksma et al., 2002; Zimmerman and Kitsantas, 2002: writing; Rummel and Spada, 2005; Rummel et al., 2009: collaboration). However, over the last few years, new variants of modeling examples have been established in online learning environments that combine features of both worked and modeling examples. For instance, the steps of a problem-solving procedure are shown or/and illustrated on a model's computer screen while a non-visible model explains the relevant actions (e.g., McLaren et al., 2008; van Gog et al., 2009, 2014; Leahy and Sweller, 2011). These new formats (known as “video modeling examples”) combine the advantages of both forms of examples. They employ the audiovisual method of modeling examples and the structured, step-wise procedure of worked examples. By structuring the problem-solving procedure into separate steps and dispensing with a visible model, learners' attention can be focused on task performance and not distracted by task-irrelevant information, e.g., other people's faces, gestures, clothes, and movement (see van Gog et al., 2014). The replacement of written text of worked examples with spoken text leads to a division of information processing into two working memory systems (Baddeley, 1986). Learners direct their visual attention to the images while simultaneously listening to the explanation of the non-visible model. According to the modality effect (Mousavi et al., 1995; Mayer and Moreno, 1998; Kühl et al., 2011), this strategy helps reduce the working memory load (Ginns, 2005; Leahy and Sweller, 2011; Sweller et al., 2011). In addition, learners' attention can be guided to the most relevant elements by highlighting, coloring and zooming in on important aspects.
The Roles of Cognitive Load and Prior Knowledge
An unguided problem provides no indication of which elements should be considered, in contrast to a worked example. Therefore, the study of worked examples reduces the number of elements that must be processed by the working memory (Chen et al., 2016). Since the cognitive architecture is restricted by the working memory capacity, element interactivity—or the degree of complexity of learning content within the framework of cognitive load theory that depends on the learner's prior knowledge (Sweller, 2011; Chen et al., 2016), may not exceed a certain amount if the goal is to promote effective learning. A higher level of element interactivity requires a greater working memory capacity, resulting in a high intrinsic cognitive load. Approaches that guide learners in the right direction removes the need to employ trial and error strategies (Renkl, 2014). Thus, learners can apply their full working memory capacity to construct a problem-solving schema to use in future problem-solving tasks (Cooper and Sweller, 1987). According to the information store principle, knowledge borrowed from others (i.e., instructors) can be reorganized and transferred to long-term memory for storage (Sweller and Sweller, 2006).
The way instructional material is presented also affects working memory, which is referred to as extraneous cognitive load. Both high intrinsic and high extraneous cognitive load might restrict long-term learning outcomes (e.g., Klahr and Nigam, 2004; Kirschner et al., 2006). This influence should be considered when deciding on an appropriate level of instructional guidance. In particular, learners with little expertise or little prior knowledge in the relevant content domain do not benefit from being confronted with too much information and opportunities for active participation at one time. Providing those learners with more instructional guidance before a problem-solving task (in the form of an example) and/or during the task (e.g., via guided or structured inquiry) can reduce mental exertion, thus ensuring that learners' cognitive resources are focused on the most relevant aspects (Sweller et al., 2011; Chen et al., 2016). This approach in turn increases the germane cognitive load, which promotes learners' understanding and the transfer of newly acquired knowledge to long-term memory (Paas and van Merriënboer, 1994; van Merriënboer and Sweller, 2005). On the other hand, the long-term retention and transfer of acquired skills were recently shown to only be achieved through active knowledge construction/generation (Bjork and Bjork, 2014), and thus require high levels of inquiry.
Indeed, an investigation of the active generation of scientific reasoning skills revealed a long-term benefit when a high level of generation success was ensured during inquiry (Kaiser et al., 2018). Students who (successfully) generated plans for scientific investigations (scientific reasoning skills) were at an advantage compared to a matched group that simply followed provided instructions. This phenomenon is referred to as the generation effect (Jacoby, 1978; Slamecka and Graf, 1978). It arises when items are better remembered when they are generated rather than simply read. It is considered an indication that active knowledge construction leads to a higher level of retention than passive observation. On the one hand, direct instruction that completely explains the underlying principles and procedures promotes effective learning, particularly for novel information with high element interactivity—as is usually the case in structured inquiry (Kirschner et al., 2006). On the other hand, the generation effect indicates that active knowledge construction leads to higher retention than passive observation, which favors guided inquiry. However, only a few studies have reported a positive generation effect on complex educationally relevant science material (e.g., Foos et al., 1994; Richland et al., 2007; Kaiser et al., 2018). As shown in the study by Foos et al. (1994), the effect is masked in applied settings because overall test performance is examined instead of performance on (successfully) generated items alone. A generation effect does not exist for non-generated items and is only observed for (successfully) generated items (Foos et al., 1994). Thus, the effectiveness of active generation in an authentic and complex learning environment, such as inquiry-based learning, relies on high generation success during the inquiry session, which in turn depends on prior knowledge (Kaiser et al., 2018). According to Kaiser et al. (2018), immediate performance (success) and the retention of scientific reasoning skills in guided inquiry are primarily influenced by prior knowledge provided through video modeling examples. Thus, learners who aquire a certain amount of (prior) knowledge via a video modeling example are more likely to profit from active generation.
Little research has been conducted on complex curriculum-based material and the impact of prior knowledge on active generation. Most previous studies on the generation effect have considered rather simple material (e.g., synonyms and rhymes) in controlled laboratory settings. They have mainly included non-curricular material for which no preexisting knowledge is required. Moreover, the studies that have examined the influence of prior knowledge by employing educationally relevant material tend to focus on mathematics. For instance, the study by Rittle-Johnson and Kmicikewycz analyzed the effect of prior knowledge on generating or reading answers to multiplication problems. Third graders with low levels of prior knowledge profited from self-generating answers to the problems. These students had better performance on the post-test and retention test than their peers subjected to the reading condition, even on problems they had not practiced (Rittle-Johnson and Kmicikewycz, 2008). Thus, learners' prior knowledge and intuitions often contravene new knowledge (Bransford et al., 2000). In contrast, the effect of active generation tends to be much more muted for the retrieval of unfamiliar material, such as nonwords, or new material, such as unfamiliar sentences from textbooks or experimental plans (Payne et al., 1986; McDaniel et al., 1988; Lutz et al., 2003; Kaiser et al., 2018). Therefore, the generation effect only applies to information rooted in preexisting knowledge (Gardiner and Hampton, 1985; Nairne and Widner, 1987). The results reported by Chen et al. (2016) confirm these findings and explain the discrepancy with the findings described by Rittle-Johnson and Kmicikewycz (2008) by showing that the generation effect only occurs for material with low element interactivity. Element interactivity, in turn, depends not only on the complexity of the material but also on learners' prior knowledge. Learners with a low level of prior knowledge have more problems generating correct information and procedures when faced with highly complex material, resulting in poor performance compared to high-knowledge learners (e.g., Siegler, 1991; Shrager and Siegler, 1998). Learners with a higher level of relevant prior knowledge face a lower element interactivity and require less guidance to successfully solve a problem due to the low intrinsic cognitive load (Sweller, 1994). In contrast, a high intrinsic cognitive load must be reduced to prevent the learner from exceeding his/her working memory limits. However, reducing cognitive load is unnecessary or even counterproductive when the intrinsic cognitive load of the relevant content is low due to the learner's high level of expertise (Chen et al., 2016). High-knowledge learners even tend to face disadvantages above a certain level of guidance and receipt of Supplementary Information—known as the expertise reversal effect (Kalyuga et al., 2003). Thus, the role of guidance in teaching remains an important and controversial issue in instructional theory (Craig, 1956; Ausubel, 1964; Shulman and Keisler, 1966; Mayer, 2004; Kirschner et al., 2006). Mulder et al. (2014) found that heuristic worked examples (Hilbert et al., 2008; Hilbert and Renkl, 2009) enhanced students' performance success but did not result in higher post-test scores. However, they recommended further research on the delayed effects of worked examples in the area of inquiry-based learning, consistent with the findings reported by Hübner et al. (2010) of a worked example effect on a delayed transfer task using strategies for writing learning journals. Kant et al. (2017) observed higher learning outcomes for students who watched a video modeling example before solving an inquiry task than for students who were provided with an example after the inquiry task. The authors compared four groups (example-example, example-inquiry task, inquiry task-example, and inquiry task-inquiry task) with regard to their learning outcomes, perceived difficulty and mental effort, judgments of learning, and monitoring accuracy in a simulation-based inquiry learning environment. The learners in the example groups were provided with a video modeling example in which two models solved an inquiry task—the same task the learners were required to solve on their own in the control condition. Studies on the necessity of combining example-based learning with different levels of inquiry-based learning for the acquisition of scientific reasoning skills are still outstanding. Overall, long-term investigations are lacking.
Research Questions
The present study aims to investigate the necessity of a video modeling example for the development of scientific reasoning skills, determine the extent to which different inquiry levels (guided and structured inquiry) benefit from example-based learning, and identify the role of learners' cognitive load in the long-term retention of scientific reasoning skills. An experiment with students in Grades 6 and 7 was conducted that compared the active generation of scientific reasoning skills in guided inquiry to an inquiry task in which learners simply read instructions on experimental design (structured inquiry) with or without a video modeling example to achieve these aims.
Consistent with recent findings reported by Kant et al. (2017) and Chen et al. (2016), we expected that watching a video modeling example of a method to solve a scientific problem by following the inquiry cycle and using the CVS would positively affect learning outcomes in guided but not structured inquiry (H1). We further expected an interaction between the inquiry level and the presence or absence of a video modeling example such that watching a video modeling example would be more effective when combined with generating answers (in guided inquiry) than reading answers (in structured inquiry), particularly in the long term (Hübner et al., 2010) (H2). Furthermore, we hypothesized that the perceived cognitive load during the learning process would differ across the four conditions (video modeling example vs. no example x guided vs. structured inquiry). According to Kirschner et al. (2006), sturctured inquiry with a video modeling example should result in the lowest cognitive load, while guided inquiry without a video modeling example should result in the highest load on working memory capacity. In contrast, guided inquiry with a video modeling example should reduce learners' intrinsic and extraneous cognitive load, increase the germane load, and promote the learning process (H3). Generation success has been reported to be a reliable predictor of learning outcomes (Foos et al., 1994; Kaiser et al., 2018). Based on these findings, we assumed that students would achieve higher performance during guided inquiry when a video modeling example is provided (H4).
Methods
Participants
We conducted an a priori power analysis using G*Power (Software G*Power; Faul et al., 2007) with a significance level of α = 0.05, a medium effect size of f = 0.25 and a desired power of 0.8; the results indicated a recommended sample size of N = 179. Two hundred and fifteen German students in Grades 6 and 7 from 9 classes in five different schools participated in the present study. A total of 174 students (M = 12.05 years, SD = 0.629) completed all tasks and the first and second post-test. Forty-one students were excluded due to illness or failure to consent to data usage. All data were collected and analyzed anonymously. A subsample of this dataset was already used by Kaiser et al. (2018) to analyze the role of generating scientific reasoning skills in inquiry-based learning in a 2 × 2-mixed-factorial design. In the present study, we used the total sample in an extended 2 × 2 × 2-mixed-factorial design and with (partially) different test instruments. Thus, new data were analyzed. Since the goal of our study was to analyze whether an example is actually needed to achieve a long-term benefit from inquiry-based learning, the control condition was not provided with any form of example. We based our design on the study by Mulder et al. (2014), who also withheld access to worked examples among students in the control condition.
Participants in all classes were randomly assigned to one of two inquiry conditions: guided inquiry, n = 68 with a video modeling example and n = 22 without an example; or structured inquiry, n = 64 with a video modeling example and n = 20 without an example.
The limited number of participants assigned to the control conditions was based on decisions by the participating classes. Classes were able to choose between an additional computer-based introduction to inquiry-based learning in the form of a video modeling example 1 week before completing the experimental unit or a short briefing (without an explicit example) on the same day the experimental was conducted. Most classes selected the extended version. However, students' level of experience in inquiry-based learning was not the reason for their decision. All students had the same low level of expertise.
Research Design
The study used a 2 (video modeling example vs. no example) × 2 (guided vs. structured inquiry) × 2 (retention interval: immediate vs. delayed) mixed-factorial design. Two levels of inquiry, guided inquiry (GI) vs. structured inquiry (SI) and with (+VME) vs. without a video modeling example (-VME), served as the independent variables. As dependent variables, scientific reasoning skills were tested at two different measurement points: post-test performance immediately after the intervention and a follow-up test 1 week later. This approach allowed us to compare the learning and transfer effects on the CVS resulting from guided or structured inquiry with or without a worked example in the short- and long-term. The tests were constructed by applying an equating facet design to control for item difficulty and avoid unanticipated test effects (see section Scientific Reasoning).
Materials
Learning Content
The students were to learn procedures and strategies for holding variables constant (CVS), as well as the fundamental scientific reasoning skills of hypothesizing (searching the hypothesis space), experimenting (testing hypotheses), and evaluating evidence. The learning environment consisted of two different student experiments: a virtual experiment with a computer-based learning program and a real experiment in an inquiry-based student lab. Both experiments analyzed the concept of behavioral adaptations among animals living in and around a pond.
Video modeling example
In the first session, all students briefly discussed the purpose and intent of scientific inquiry with a specially trained instructor, who subsequently introduced them to the topic of “animals of the pond.” Afterwards, one group of the students was taught the CVS in a uniform computer-based introductory session in the new format of a video modeling example (+VME), which combines the benefits of worked examples and modeling examples (see section The Relevance and Effectiveness of Example-Based Learning). The session was designed to develop the students' scientific thinking and understanding of the reason for holding all variables constant across experimental conditions while varying the one variable being investigated. After a short introduction to the discipline-specific methods employed by scientists, a virtual professor (“Professor Plankton”) familiarized the students with the inquiry cycle and the learning content of the unit (the concept of behavioral adaptations among animals living in and around a pond) by guiding them through eight video units corresponding to the steps of an illustrative experiment about dragonfly (Anisoptera) larvae hunting their prey: phenomenon, research question, hypotheses, plan, investigation, analysis, interpretation, and discussion. The example of dragonfly larvae hunting their prey was used to introduce the students to the crucial phases of scientific inquiry: (1) formulating research questions, (2) inferring one or more hypotheses, (3) planning and conducting an experiment, and (4) analyzing the experiment (describing the data, interpreting the data, and critically evaluating the methods used). The students were shown the steps of the procedure on the Professor's computer screen while a non-visible speaker explained the Professor's actions. Hence, the students were able to study the example in a step-by-step procedure by directing their visual attention to the images while simultaneously listening to an explanation by a non-visible model (see the Supplementary Material: Screenshots VME).
Inquiry tasks
In the laboratory sessions, all students completed a scientific experiment using the CVS entitled “The Mystery of Water Fleas' Migration” (Meier and Wulff, 2014), which focused on the daily vertical migration of water fleas (Daphnia magna). This phenomenon was related to the initial example in the learning program, as it also involves a biological adaptation, or structural or behavioral changes that help an organism survive in its environment. Biological adaptation is considered a core disciplinary concept in leading science standards (National Research Council, 2013), which none of the participating classes had covered previously in class.
The module aimed to teach scientific thinking and scientific reasoning skills via guided experimentation. All students received a research workbook (see Supplementary Material: Research Workbooks in Kaiser et al., 2018) to support the students' learning process and provide guidance across all phases of the inquiry cycle (hypothesis generation, designing and conducting an experiment, and interpreting the results). Students in the “Guided Inquiry” (GI) condition received 13 short prompts that helped them plan an appropriate experiment by identifying the independent and dependent variables, control variables (see Supplementary Material: Example Inquiry task), and confounding variables (short answer tasks), as well as a cloze (consisting of 130 words and 15 prompts) that asked them to retrieve information about the CVS immediately following the experimental session. The students in the “Structured Inquiry” (SI) condition received research workbooks with direct instructions for conducting an experiment instead of generation prompts, and a reading text rather than a cloze at the end.
The content of the research workbooks was structured in a similar manner across conditions to ensure comparability. All prompts and feedback material in the GI condition were derived from the text material in the SI condition. Moreover, the students were provided the same amount of time for cognitive processing.
Instruments
Three assessment time points were integrated into the experimental design: the first test was administered prior to the inquiry task or after the video modeling example, the second was administered after the inquiry task, and a final test was administered after a retention interval of 1 week. In addition to scientific reasoning skills (see section Scientific Reasoning), the students' success in generation (see section Learners' Performance Success in Guided Inquiry) and perceived cognitive load (Cierniak et al., 2009) (see section Learners' Cognitive Load) were assessed during the experimental task. Data on the students' demographics; grades in biology, math, and German; need for cognition (Preckel, 2014) and cognitive abilities Heller and Perleth, 2000 (see section Learners' Prerequisites) were collected at each of the three assessment time points. All measurements were paper-based.
Scientific Reasoning
Three questionnaires assessing the acquisition and retention of scientific reasoning skills were developed to evaluate the learning outcomes. After conducting statistical item analyses, the final assessment tests consisted of 6 to 10 items, both single choice and open-ended (Janoschek, 2009; Hof, 2011; Wellnitz and Mayer, 2016; modified). All single-choice items had four possible answer options. In contrast to Kaiser et al. (2018), we also tested the students' inquiry skills in an open-ended format, which allowed us to examine higher levels of competence in inquiry skills.
Immediately after the video modeling example or immediately before the inquiry session, depending on the condition, students completed an intermediate assessment test consisting of six items to identify individual differences in scientific reasoning skills. The assessment test comprised four open-ended items and two single-choice items. Item difficulty was appropriate (p = 0.56), a moderate level of difficulty, and the test indicates an acceptable level of reliability (α = 0.60) for comparing groups (Lienert and Raatz, 1998). Furthermore, the discrimination parameters were all above rit > 0.30.
The following scientific reasoning tests were completed 10 min after the inquiry task and 1 week later (five single-choice items and four or five open-ended items, respectively) (Figure 1). All tests required students to demonstrate their understanding of CVS. They were either asked to select the appropriate design from a set of confounded and unconfounded experiments, amend a confounded experiment, or identify the independent and dependent variables in an unconfounded experiment. We incorporated anchor items into the two post-tests to ensure comparability and provide a baseline for an equating analysis. The construction of the anchor items was based on an equating facet design with three dimensions to ensure systematic variation (Table 2). Each anchor item provided a uniform description of an experimental design (task context) in each post-test, followed by a prompt to either complete Task (1), (2), or (3) in one or two task formats (single choice and/or open-ended item). The use of the same task context ensured the comparability of the two post-tests and sought to focus students' attention on inquiry skills rather than distracting them with excess content-related information. The three different tasks invited students to evaluate the quality of others' research—to identify the independent and dependent variable (searching the hypothesis space), select an appropriate experimental design (testing a hypothesis) or evaluate appropriate measurements (analyze scientific evidence). One of six task contexts was allocated to each task. In addition, some anchor items encompassed two different task formats: single choice (SC) and open-ended (O) counterpart items. Thus, two to six versions of each task context appeared in the test, with varying variables to be defined (see the Supplementary Material: Example Anchor Item). Three task contexts were used in all three tests, while five contexts were used in post-tests 1 and 2 only. Thus, students were tested with 19 (3 × 3 + 5 × 2) anchor items referring to the same scientific knowledge construct and skills across the three measurement points.
Item difficulty, internal consistency, and discrimination parameters were analyzed for post-tests 1 and 2. Item difficulty was appropriate (p = 0.50–0.58) and the tests were reliable (α = 0.70–0.72) for comparing groups (Lienert and Raatz, 1998). Furthermore, the discrimination parameters were all above rit > 0.30.
Learners' Cognitive Load
The students' perceived cognitive load was assessed under all conditions immediately after the inquiry session. Since the main focus of the study was the learning outcomes (see section Scientific Reasoning) and student performance (see section Learners' Performance Success in Guided Inquiry), we sought to keep the questionnaire brief to avoid overtaxing our sample of young learners and decreasing their motivation. The instrument comprised five items (after excluding one) to which the students responded on a six-point Likert scale (ranging from 1 = low to 6 = high) (α = 0.66, rit > 0.20, Cierniak et al., 2009, modified). Cierniak et al. (2009) used this instrument to analyze how different cognitive load types mediate the split attention effect (e.g., Chandler and Sweller, 1991, 1992, 1996) in a learning environment with biological content that included complex figures with accompanying texts. Their measure was chosen because their learning environment was similar to our environment and their questionnaire was shorter than more frequently used scales, such as the scale used by Leppink et al. (2014). In our study, intrinsic and extraneous cognitive load were measured with 4 items, for IL: (1) “How difficult was it for you to understand the experiment?” and (2) “How difficult was it for you to work like a research scientist?,” and for EL: (3) “How difficult was it for you to work with the research workbook?” and (4) “How difficult was it for you to understand the work instructions in the research workbook?.” A single item was used to assess germane load, GL: (5) “How strongly did you concentrate while learning today?.” One item “How much effort did you need to invest into learning today?,” was excluded due to insufficient item properties (see the Supplementary Material: Questionnaire for Cognitive Load in Kaiser et al., 2018). Items (1), (3), and (5) were adopted from Cierniak et al. (2009); items (2) and (4) were new Supplementary Items.
Learners' Abilities
Learners' prerequisites
Two questionnaires with good validity (NFC: p = 3.58, α = 0.89, rit > 0.30; CA: p = 0.48, α = 0.91, rit > 0.30) were included in the study design to assess students' prerequisites, namely the need for cognition (Preckel, 2014) and cognitive abilities (Heller and Perleth, 2000). The questionnaire for the need for cognition comprised 19 items, with responses indicated on a five-point Likert scale. The Questionnaire for Cognitive Abilities for 6th Graders measured the students' figural inductive reasoning skills by asking them to identify figural analogies (KFT 4-12+ R, Subtest N, Heller and Perleth, 2000). It comprised 24 items (after excluding one). Each item had five answer options and only one correct answer. The students were tasked with answering as many items as they could within 9 min (see the Supplementary Material: Questionnaire for Cognitive Abilities in Kaiser et al., 2018).
Learners' performance success in guided inquiry
We further collected qualitative data in the form of all student responses to the generation prompts in the students' research workbooks under the inquiry condition, including the students' proposed experimental designs, discussions of research methodology and the final cloze. This made it possible to confirm the effect of the treatment and examine the role of generation success in short-term and long-term retention. The data were coded on a scale with a potential range of 0 to 33 points. The following components of the experimental design were assessed (each on a 0–2-point scale): identifying the independent and dependent variables; designing a controlled experiment in which one independent variable is varied and all other relevant variables are held constant, thus controlling for potential biases and confounding factors; and specifying the measurement time points and number of animals (water fleas) in the experiment. With respect to the methodological discussion, the following factors were evaluated (also on a 0–2-point scales): ensuring equal control conditions and describing its importance, using an LED light and more than 10 water fleas and describing their importance, avoiding external confounders (light pollution, bumping into the desk, and noise) and describing its importance, and the necessity and duration of a habituation period for the water fleas (for further information, see the Supplementary Material: Coding scheme in Kaiser et al., 2018).
Interrater reliability was calculated using the Kappa statistic to evaluate the consistency of the two independent raters. The Kappa value was 0.94 (p < 0.001), indicating almost perfect agreement (Landis and Koch, 1977).
The research workbooks and the complete coding scheme are published in the study by Kaiser et al. (2018).
Procedure
The experiment consisted of three phases: an introductory video modeling example with a subsequent intermediate test (see section Computer-Based Introduction via a Video Modeling Example), an inquiry-based learning session with a subsequent post-test (see section Inquiry Task), and a second post-test. One hundred and thirty-two students engaged in all three sessions (+VME), which were scheduled over 3 weeks. The other 45 students did not participate in the first computer-based session (-VME).
Computer-Based Introduction via a Video Modeling Example
The first session required ~60 min to complete and was performed at school. A group of students (+VME) received guided instruction in a computer-based learning environment and then individually worked through a brief learning session on computers. Each student had a headset that allowed them to explore the learning program, which consisted of videos and short reading passages, at their own pace. A video modeling example familiarized the participants with fundamental scientific reasoning skills. A virtual figure called Professor Plankton led the students through the learning program. The students were introduced to all experimental phases and the specific terminology associated with them. This instruction lasted 30 min. Immediately afterwards, the students completed a paper-based intermediate assessment test, which sought to identify individual differences in scientific reasoning skills. The students required an average of ~25 min to complete the test; a time limit was not established. The students who did not work through the computer program (-VME) were asked to complete the assessment test items immediately before the inquiry task (in the second session).
We also collected data on the students' demographics, cognitive abilities, need for cognition, and grades in math, German and biology. All students were also asked to indicate whether they had previously attended an inquiry course in our student lab. Students who attended this course were excluded from the calculations. Students were clearly informed that the learning program was in preparation for a subsequent inquiry module at the university.
Inquiry Task
The inquiry module, a scientific experiment on water fleas' vertical migration, took place 1 week after the computer-based introduction. It was conducted in an inquiry-based learning environment in a university lab tailored to work with school students.
During this learning phase, individual students in each class were randomly assigned to the two conditions [guided (GI) vs. structured inquiry (SI)] and separated into small groups (up to five students). They received instruction from trained supervisors. Thus, the students in each group knew one another before the start of the inquiry activity. Intermixing students across classes was not feasible because we only had access to one student lab, a limited number of experimental materials, rooms and supervisors were available, and for other organizational reasons. The supervisors received scripts with detailed information about each inquiry phase to assist them in providing uniform guidance to all groups during the inquiry activity. Supervisors at both inquiry levels were prohibited from answering questions on scientific reasoning to ensure that we collected accurate data on students' inquiry skills. The key difference between the two inquiry levels was the amount of information and instructional support provided; however, the total instructional time remained the same across conditions. The students in each condition were allowed ~180 min to complete the inquiry task in two separate rooms after receiving uniform (general) instructions from their supervisor. Each task was assigned a certain maximum duration (see the Supplementary Material: Research Workbook in Kaiser et al., 2018).
The main differences between the conditions are listed below. Students in the SI condition were provided with a detailed experimental plan and a discussion of the method that would be used, whereas students in the GI condition were required to actively generate their own experimental plan and discuss the data they collected using the inquiry skills acquired in the introductory section. They first generated information individually by identifying independent and dependent variables and jotting down ideas for experimental procedures (scientific reasoning skills: inferring hypotheses, aspects: independent variable and dependent variable; Arnold et al., 2014) (individual work). After discussing their preliminary ideas with one another, the students in each group worked together to develop a detailed experimental plan that operationalized the dependent variable, appropriately varied the independent variable, identified and controlled for biases and confounders, and specified the measurement intervals and number of measurement points (scientific reasoning skills: planning experiments, aspects: independent variable, dependent variable, confounding/nuisance variables, measurement points, and repeated measures; Arnold et al., 2014) (team work). The second phase proceeded in the same manner. First, the students individually analyzed the biases for which they had controlled in the experiment by completing a corresponding checklist (see Example 3) (individual work); then, they discussed their data in groups (team work). The students followed the same procedure and used the same terminology presented in the video modeling example.
As students have been shown to perform better during inquiry when provided more specific guidance (Johnson and Lawson, 1998; Borek et al., 2009; Lazonder and Harmsen, 2016), the students received corrective feedback from their supervisor after both phases to ensure that the students had access to a sufficient amount of information. However, the information the supervisors were permitted to provide was limited to the material defined in a workbook of instructions (see the Supplementary Material: Workbook of Instructions for Generation Group in Kaiser et al., 2018), which all supervisors were required to use. Supervisors provided the students with correct responses or instructed them on how to supplement and/or revise their proposed experimental plans to help the students dismiss incorrect ideas and identify new ideas by following the provided cues. In contrast, students in the SI condition were explicitly informed about which variables to investigate and were provided a series of prescribed steps to follow, similar to a recipe. Instead of completing a checklist and discussing bias after the experiment, the students were simply informed about possible confounders that may have influenced the dependent variable.
Apart from these differences, the procedure was identical under all conditions. Students in both groups completed the physical hands-on activities involved in conducting the experiment, because practice is necessary for learners to develop an understanding of the principles of unconfounded evidence (Sneider et al., 1984; Schwichow et al., 2016). Moreover, no students were asked to generate any content-related information. Thus, they stuck to appropriate interpretations of their experimental data.
Immediately after the inquiry-based learning session, students in all treatment groups completed the same questionnaire about cognitive load, followed by an assessment test measuring scientific reasoning skills (with five SC and four open-ended items). Students were not informed in advance that they would be taking these tests to prevent them from studying for the tests and to increase the probability that post-test scores would reflect knowledge acquired during the experiment. One week later, all students completed a second, comparable post-test with five SC and five open-ended items. The students required an average of ~30 min to complete each test; again, no time limits were imposed.
Data Analysis
We conducted statistical analyses from the paradigm of classical test theory using SPSS software to identify differences between groups and among students with different abilities, as well as to detect the influence of students' characteristics on their learning outcomes.
All results were significant at the 0.05 level unless indicated otherwise. Pairwise comparisons were Bonferroni-corrected to the 0.05 level. The partial eta squared () value is reported as an effect size measure for all ANOVAs, while Cohen's d is reported as an effect size measure for all t-tests.
Results
No significant differences were observed between conditions in students' demographic data, grades, need for cognition or cognitive abilities, indicating that randomization was successful. Additionally significant differences were not observed between the classes that participated in the computer-based introduction and classes that did not, with the sole exception of biology grades. The Bonferroni-adjusted post-hoc analysis revealed that students in the SI+VME condition achieved better grades in biology than students in the SI-VME condition (0.59, 95% CI [1.09, 0.09], p = 0.011). However, biology grades did not significantly affect the learning outcomes.
We also monitored the data for CVS experts, or students who answered all items on the intermediate test correctly without receiving a video modeling example. However, no such experts who might have distorted the results were identified.
Descriptive results for the learners' performance in all test sessions are shown in Table 3.
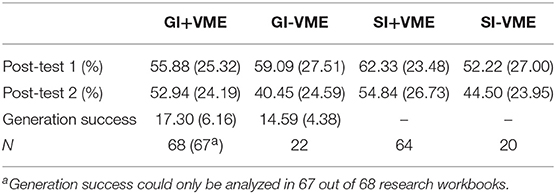
Table 3. Means and standard deviations (in parentheses) of performance assessed in post tests 1 and 2.
Learning Outcome—Video Modeling Example vs. No Example in Guided or Structured Inquiry on Short- and Long-Term Retention (H1) and (H2)
The results were analyzed using a 2 (video modeling example vs. no example) x 2 (guided vs. structured inquiry) x 2 (retention interval: immediate vs. delayed) ANOVA with repeated measures. This model yielded a significant main effect of time, F(1,170) = 18.54, p < 0.001, ηp2 = 0.098, but no main effect of inquiry level, F(1,170) = 0.68, p = 0.412, and only a marginal significant effect of the use of a video modeling example, F(1,170) = 3.32, p = 0.070. Hence, students achieved higher results immediately after the inquiry task than 1 week later. Furthermore, we detected a significant interaction between the retention interval and receipt of a video modeling example, F(1,170) = 4.58, p = 0.034, ηp2 = 0.026. The interaction between the retention interval and level of inquiry was not significant, F(1,170) = 0.06, p = 0.807, nor was the interaction between inquiry level and use of a video modeling example, F(1,170) = 0.48, p = 0.488. However, a significant three-way interaction was observed between the retention interval, receipt of a video modeling example and level of inquiry, F(1,170) = 3.96, p = 0.048, ηp2 = 0.023. Thus, the usefulness of a video modeling examples depends on the level of inquiry and the measurement time point. Therefore, subsequent ANOVAs, post-hoc tests, t-tests and multilevel analyses were performed.
Consistent with our expectations, the results of the intermediate test after the first manipulation (video modeling example vs. no example) were higher among students who watched a video modeling example, t(172) = 5.48, p < 0.001, d = 0.97 (Figure 2A).
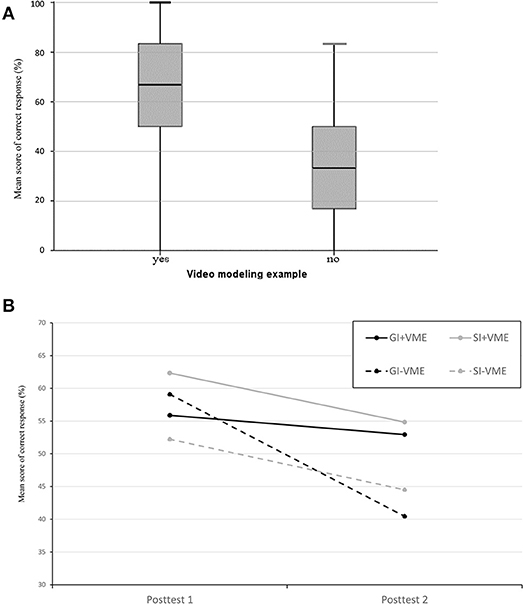
Figure 2. (A) Mean score of correct responses by treatment 1 (video modeling example vs. no video modeling example) in percentage. (B) Mean score of correct responses by treatments and time in percentage.
All students achieved equal results on the assessment immediately after the subsequent inquiry task, regardless of the manipulation. Students who watched a video modeling example before solving a guided or structured inquiry task only outperformed students who did not receive an example in the delayed tests, MD = 0.11, SE = 0.05, 95% CI [0.03,0.20], p = 0.011. No differences were observed at any time point between the levels of inquiry.
Post-hoc analyses (Bonferroni-corrected) of interaction effects revealed that the retention of scientific reasoning skills significantly decreased between the two measurement points in the GI-VME, MD = 0.19, SE = 0.05, 95% CI [0.09, 0.28], p < 0.001, and SI+VME groups, MD = 0.08, SE = 0.03, 95% CI [0.02, 0.13], p = 0.007, but remained stable in the GI+VME and SI-VME groups (Figure 2B). Furthermore, students who watched a video modeling example before solving a guided inquiry task (GI+VME) achieved higher learning outcomes in the second assessment test than students who did not receive an example before solving the same inquiry task (GI-VME), P2: MD = 0.13, SE = 0.06, 95% CI [0.03, 0.25], p = 0.045. No differences were observed in the results of both assessment tests for paired comparisons of structured inquiry (SI) (Figure 2B).
In addition, multilevel analyses were conducted with the R packages lme4 (Bates et al., 2015), lmerTest and lsmeans (Lenth, 2016) in the R environment, version 3.4.4 (R Core Team, 2018) to determine differences between the inquiry levels and between groups provided with or without an example while controlling for class effects. The presence of a video modeling example (VME, no example) and the level of inquiry (guided inquiry or structured inquiry) were the independent variables; the dependent variable was scores on the two tests measuring students' achievement (P1 and P2). We controlled for classes to remove variation in the dependent variable resulting from class effects. Again, no significant differences were observed in the assessment performed immediately after inquiry, P1: β = 0.029 (SE = 0.035), and in the subsequent assessment measure, P2: β = 0.019 (SE = 0.034) between the treatments when controlling for class effects. However, the GI-VME group still produced the worst descriptive results for Post-test 2 compared to all other treatments.
Students' Cognitive Load (H3)
In univariate and multivariate analyses of variance, we did not observe a main effect of the video modeling example on overall cognitive load, and only marginally significant differences in germane load, F(1,170) = 2.91, p = 0.090. However, main effects of the inquiry level on overall cognitive load, F(1,170) = 5.52, p = 0.020, and extraneous load, F(1,170) = 8.09, p = 0.005, were observed. Overall cognitive load was lower in the SI+VME group (MSI+ = 1.94, SD = 0.50) than in the GI+VME group (MGI+ = 2.26, SD = 0.69), MD = 0.315, SE = 0.11, 95% CI [0.02, −0.61], p = 0.028, although both groups were exposed to the introductory video modeling example.
Pairwise comparisons of the two conditions (GI+VME vs. SI+VME; Bonferroni-corrected) revealed that this effect was due to an increased extraneous load caused by generation in guided inquiry, MD = 0.52, SE = 0.13, 95% CI [0.17, 0.86], p = 0.001. Only marginally significant differences were observed in the intrinsic load: MD = 0.32, SE = 0.13, 95% CI [−0.66, 0.019], p = 0.076, and no significant differences were observed in the germane load. Pairwise comparisons revealed no differences between the two inquiry levels when a video modeling example was not presented (GI-VME vs. SI-VME; Bonferroni-corrected): MSI− = 2.17, SD = 0.71; MGI− = 2.38, SD = 0.75.
Furthermore, detailed analyses of the two guided and structured inquiry conditions (GI+VME vs. GI-VME, SI+VME vs. SI-VME) revealed no significant differences in any of the three types of cognitive load. However, the GI-VME group exhibited the worst descriptive results for germane load (Figure 3).
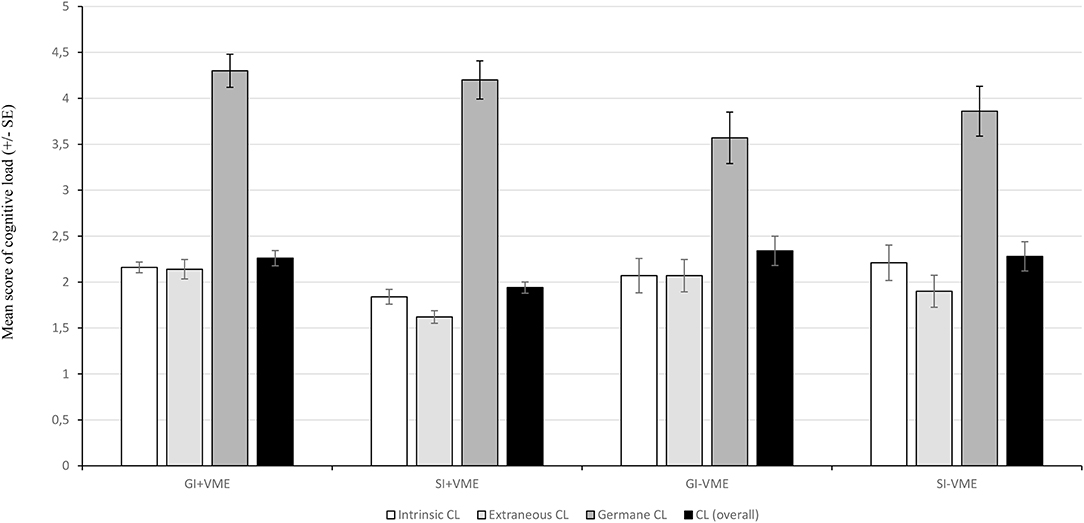
Figure 3. Mean score of (intrinsic, extraneous, germane, overall) cognitive load by treatment (GI+VME, SI+VME, GI-VME, SI-VME).
Students' Performance Success (H4)
The students' experimental plans and methodological discussions were investigated to assess how much information each individual student in the GI group was able to successfully generate and at what frequency (total score = 33). Thus, this assessment represented an analysis of the role of generation success.
No significant benefits of combining video modeling examples with guided inquiry were observed with respect to generation success, t(87) = 1.91, p = 0.060, although a clear descriptive difference was observed (Table 3).
Discussion
The goal of the present study was to investigate the necessity of combining example-based learning with different levels of inquiry-based learning for the acquisition of scientific reasoning skills. Therefore, we analyzed the benefit of (a) presenting vs. (b) omitting a video modeling example before (1) an inquiry activity involving the generation of scientific reasoning skills (guided inquiry) vs. (2) an inquiry activity that had students simply read instructions for an experimental plan and an appropriate methodological discussion (structured inquiry). A computer-based learning program that contained a video modeling example of how to investigate an authentic scientific research question by following the inquiry cycle was developed for the purpose of the study as preparation for the subsequent inquiry task. Effects on the learning process, short-term and long-term learning outcomes in terms of scientific reasoning skills, and crucial prerequisites for effectiveness, such as performance success and perceived cognitive load, were measured.
Hypotheses (H1) and (H2) were partially verified, as watching a video modeling example of how to solve a scientific problem by following the inquiry cycle and using the CVS positively affected learning outcomes in guided, but not structured, inquiry (H1), particularly in the long term (H2). A significant decrease in retention was observed over a period of 1 week for guided inquiry when a video modeling example was not provided. However, the expected worked example effect for guided inquiry after a 1-week delay was not significant.
Consistent with our expectations, structured inquiry with a video modeling example resulted in the lowest cognitive load. However, in contrast to our hypothesis (H3), the provision of a video modeling example did not significantly reduce learners' intrinsic and extraneous cognitive load or increase germane load in guided inquiry.
Regardless of the treatment, students obtained equal results on assessments after and during the inquiry task (performance). Therefore, our hypothesis (H4) was not confirmed. Since the results of an intermediate test were higher among students who watched a video modeling example, the lack of differences between conditions during and after inquiry might be related to the fact that the inquiry task was designed in such a way that all students—regardless of whether they had been provided with a video modeling example—were able to plan, conduct and analyze a scientific experiment using the CVS.
Guided vs. Structured Inquiry
Consistent with the findings reported by Kaiser et al., extraneous load was significantly higher in the structured inquiry group (with a video modeling example) compared to the guided inquiry group (with a video modeling example). Nevertheless, both levels of inquiry were equally effective. No generation effect was observed after a 1-week delay. Students in the structured inquiry group still had higher performance in terms of absolute numbers. In contrast to Kaiser et al., we only identified a descriptive, insignificant short-term disadvantage among students who actively generated information in guided inquiry. A potential explanation for this finding is that our short-term assessment used both open-ended items and single choice items, whereas Kaiser et al. only used a closed response format. According to Hirshman and Bjork (1988), a generation advantage or disadvantage is sensitive to different types of memory tests (recognition, cued recall, and free recall). Solving a generation task with an open-ended format in the inquiry-based learning environment may increase performance on open-ended retention test items. Conversely, students who passively receive information about the experimental plan and methodological discussion in structured inquiry may have an advantage in a recognition format (e.g., single choice items) (transfer appropriate processing, Morris et al., 1977). Therefore, an equal number of single choice and open-ended items was essential to ensure a fair comparison of both conditions. Furthermore, answering open-ended questions is a more demanding process for students, but enabled us to evaluate higher levels of competence in scientific inquiry (Mayer et al., 2008), which requires further analysis. Finally, although all students performed significantly better on single choice questions than open-ended questions, the difference between the two formats was indeed higher in the structured inquiry group.
We expected that students who engaged in guided inquiry, which required them to actively adopt the CVS, after watching a video modeling example would exhibit a lower forgetting rate than students who engaged in structured inquiry. In fact, students who had engaged in guided inquiry with a video modeling example exhibited the same performance on both tests, while retention significantly decreased among students who had engaged in structured inquiry. Based on these results, guided inquiry is potentially more effective in teaching students CVS in terms of memory and knowledge sustainability (storage strength, Bjork and Bjork, 1992). However, further research controlling for generation success (Kaiser et al., 2018) is needed to confirm a long-lasting effect.
Guided Inquiry
Watching a video modeling example before completing an inquiry task was beneficial for students who were later asked to actively generate their own experimental design using the CVS, since retention in this treatment group did not decrease within a week. These results confirm our first two hypotheses (H1 and H2), and are somewhat consistent with the findings reported by Kant et al. (2017) and Chen et al. (2016). However, a worked example effect did not arise. In contrast to the results presented by Kant and colleagues, in which a clear worked example effect was immediately observed for video modeling examples on virtual inquiry learning, video modeling examples only affect long-term retention in guided inquiry in the present study. When a video modeling example was omitted, retention significantly decreased over a period of 1 week. Our finding of a long-term advantage of watching a video modeling example for guided inquiry is consistent with the findings reported by Hübner et al. (2010) and Chen et al. (2016), who revealed the long-term effectiveness of worked examples.
According to our results, a video modeling example enabled students to borrow information from the non-visible model by utilizing the strategies discussed and applied in the video modeling example (Bandura, 1986). The video modeling example helped students focus on relevant aspects and procedures to acquire new cognitive schemata for planning and discussing scientific investigations during guided inquiry. These findings support the notion that learning through modeling is more than just simple imitation (Bandura, 1986). Reliance on observed strategies when solving a less structured inquiry task enabled the students to increase their working memory capacity during inquiry and helped foster their storage strength (Bjork and Bjork, 1992) for the observed strategies for up to 1 week. Thus, the generated information from the inquiry session was permanently integrated into the cognitive schemata acquired from the video modeling example, whereas new information generated during guided inquiry did not result in the same linkages with preexisting knowledge and thus did not exhibit the same storage strength in the absence of a video modeling example. Consistent with these results, participants who received a video modeling example before guided inquiry reported a higher germane cognitive load during inquiry than students who were not provided with an example. However, the difference was only marginally significant (H3). Nevertheless, since the retention of students who were provided with a video modeling example before guided inquiry did not decrease, a single video modeling example appears to be sufficient to guide students' attention to appropriate cognitive schemata, which fosters the long-term learning of inquiry skills (Scheiter et al., 2004; Crippen and Earl, 2007; Schworm and Renkl, 2007; Sweller et al., 2011; Chen et al., 2016). Unexpectedly and in contrast to the results from the study by Kant and colleagues on video modeling examples in virtual inquiry, one example did not appear to be sufficient to significantly reduce the intrinsic and extraneous load. A single example might be insufficient to significantly reduce the cognitive load in physical, hands-on investigations. However, the lack of significant differences might also have been due to insufficient power for small effects (post-hoc power analysis: a significance level of a = 0.05 and a small effect size of d = 0.2 yielded a power of 0.2) and the fact that the test for cognitive load exhibited only an acceptable level of reliability (α = 0.66) for comparing groups (Lienert and Raatz, 1998). Consequently, the results should be interpreted with caution.
Structured Inquiry
Students who watched a video modeling example before engaging in a structured inquiry task reported the lowest level of cognitive load. Consequently, participants who had received a video modeling example perceived the inquiry tasks as less cognitively demanding than students who did not watch an example or students who were provided with less instructional guidance during inquiry. However, the use of the borrowing and reorganizing principle to reduce the cognitive load and thus free more working memory capacity to focus on problem-solving strategies and construct useful cognitive schemata for solving the subsequent inquiry task (Sweller and Sweller, 2006) did not improve learning outcomes in structured inquiry. The students who completed a structured inquiry task achieved equal results, regardless of whether they were provided with a video modeling example. Additional guidance in the form of a video modeling example appears to have no long-term effect on inquiry tasks that are already strongly guided via direct instructions, as is typically the case in structured inquiry (Chen et al., 2016). A learner with a higher level of prior knowledge will perceive a lower element interactivity and require less guidance to solve a problem (Sweller, 1994; Chen et al., 2016). According to Chen and colleagues, the worked example effect only arises when element interactivity is high, resulting in a high intrinsic cognitive load. If the intrinsic cognitive load is already low, control of the extraneous cognitive load using worked examples is unnecessary because the total cognitive load does not threaten to overload the working memory capacity (element interactivity effect, Chen et al., 2016). Nevertheless, we did not observe an expertise reversal effect (Kalyuga et al., 2003). Based on these findings, solving an inquiry task at a low level of inquiry after watching a video modeling example is still challenging for students because, first, the video modeling example and the inquiry task (reading task) in structured inquiry were non-redundant. The strategies and procedures illustrated in the example were required to be applied to a completely new experiment. These conditions might have simultaneously challenged and motivated the students. Second, working memory is already taxed by physical, hands-on investigations (physical lab experiences), which require students to work with information with high element interactivity (Chen et al., 2016) and use a complex hypothetico-deductive procedure.
Further Limitations
Moreover, the following limitations must be considered when drawing conclusions from the experiment. First, the long-term disadvantage observed for the subsample of students who were not provided with a video modeling example might simply result from their spending less time with the learning material. Future research should compare groups of students who merely study an example of how to solve a practice problem vs. actually solve a practice problem for the same amount of time to control for this limitation. Second, the intermediate assessment test and the test for cognitive load exhibited only an acceptable level of reliability (α = 0.60 and α = 0.66) for comparing groups (Lienert and Raatz, 1998). Consequently, the results should be interpreted with caution. Moreover, the subsample was too small for a detailed analysis. Due to the resulting small power, we were unable to apply techniques such as pathway analyses of the four individual conditions. An investigation designed to assess which and to what extent learner characteristics (cognitive load, NFC, KFT, grades, and generation success) affect the short-term and long-term retention of each treatment group would be interesting. Thus, replications are required. Furthermore, randomization within each class was confined to the second manipulation (inquiry level), while the first manipulation was conducted between classes. We were unable to intermix students within classes with respect to the first manipulation for organizational reasons. Third, the students participated in a physical inquiry-based lab experiment in all four conditions. These settings provide an authentic picture of scientific practice and support the application of authentic scientific procedures. On the other hand, higher authenticity is always sensitive to interferences and accompanied by a greater cognitive burden. The application of newly acquired inquiry skills and correct handling and manipulation of physical equipment might be very challenging for students. Moreover, authentic experimental settings include a large number of features that can cause a higher extraneous cognitive load and distractions, as students may focus on insignificant aspects. Hence, due to the reliance on physical experiments, the extraneous cognitive load was high in this study and might have obscured small differences between the treatments. Future research should analyze how to further reduce the extraneous cognitive load, particularly in guided inquiry, since structured inquiry (with a video modeling example) proved to be the least cognitively demanding condition. Consistent with the theory of transfer appropriate processing, the use of the same (digital) medium in both sessions—a learning program with a video modeling example in the introductory session and an accompanying digital scaffold for the hands-on inquiry-based learning environment instead of a human supervisor—might be beneficial.
Implications
In terms of the theoretical implications, this study broadens the research base on video modeling examples and the generation effect, as well as the unresolved didactic question of whether direct instruction or discovery-based methods deliver better learning outcomes and retention to a certain extent (Dean and Kuhn, 2007; Furtak et al., 2012).
In contrast to our expectations and recent findings on the generation effect (e.g., Chen et al., 2016), guided inquiry did not prove to be more beneficial than structured inquiry. As long as guided inquiry was preceded by a video modeling example, both levels of inquiry were equally effective. Consistent with recent studies on example-based learning (van Gog et al., 2011; Leppink et al., 2014; Kant et al., 2017), students who watched a video modeling example in the present study benefitted from being provided with an indication of which elements should be considered when solving an inquiry task. They achieved the same performance results after a period of 1 week had elapsed, while retention was significantly decreased when a video modeling example was not provided in guided inquiry. Thus, a video modeling example affected how much mental effort students were able to invest in solving the inquiry task and promoted the integration of generated information into the cognitive schemata acquired from the example.
Generation in guided inquiry-based learning leads to better long-term learning outcomes when the germane cognitive load is increased through the use of a video modeling example. However, ultimately, higher learning outcomes are influenced either by providing a video modeling example or by directly providing a higher level of instructional guidance during inquiry.
Conclusions
Sufficient knowledge serves as a foundation for long-term retention by providing anchors to assimilate new information into preexisting cognitive schemata and facilitating retrieval. Guided inquiry does not automatically promote deeper learning and retention. Video modeling examples are required to provide a sufficient foundation in terms of scientific reasoning skills and increase working memory capacity. Ultimately, video modeling examples are effective for long-term learning gains in guided inquiry when teaching scientific reasoning skills in inquiry-based learning. In structured inquiry, they but have no significant benefit for long-term retention. But at least they can reduce the cognitive load.
Data Availability Statement
The raw data supporting the conclusions of this manuscript and Supplementary Material (computer-based introductory session, research workbooks, and instruments) are available to any qualified researcher: https://osf.io/uvrwn/?view_only=4c8a7819fcae451a8ed9cdaef63f06f1.
Ethics Statement
No ethics approval was required for the present study according to national guidelines as well as the University of Kassel's own guidelines. The study was conducted in accordance with the recommendations of the University of Kassel's ethics committee and with the approval of the Ministry of Education and Cultural Affairs, Hesse, Germany (Hessisches Kultusministerium) (cf. Education Act of Hesse, section 84). The parents of all participants gave written informed consent in accordance with the Declaration of Helsinki.
Author Contributions
JM developed the basic idea for the present study and supervised the project. He also took the lead on project administration and funding acquisition. IK developed the study material and was responsible for the data collection, conducted the analyses, and drafted the manuscript in consultation with JM. All authors contributed to the final version of the manuscript.
Funding
This project was funded by the LOEWE Excellence Programme: Desirable Difficulties in Learning from the Hessian Ministry for Science and the Arts.
Conflict of Interest
The authors declare that the research was conducted in the absence of any commercial or financial relationships that could be construed as a potential conflict of interest.
Acknowledgments
We gratefully acknowledge the work of all supervisors in the laboratory sessions, all participating classes and teachers, and our colleagues who put us in contact with the participating schools.
Supplementary Material
The Supplementary Material for this article can be found online at: https://www.frontiersin.org/articles/10.3389/feduc.2019.00104/full#supplementary-material
References
Abrams, E., Southerland, S. A., and Evans, C. (2008). “Inquiry in the classroom: identifying necessary components of a useful definition,” in Inquiry in the Science Classroom: Challenges and Opportunities, eds E. Abrams, S. Southerland, and P. Silva (Charlotte, NC: Information Age Publishing), 11–42.
Aleven, V. (2002). An effective metacognitive strategy: learning by doing and explaining with a computer-based Cognitive Tutor. Cogn. Sci. 26, 147–179. doi: 10.1207/s15516709cog2602_1
Alfieri, L., Brooks, P. J., Aldrich, N. J., and Tenenbaum, H. R. (2011). Does discovery-based instruction enhance learning? J. Educ. Psychol. 103, 1–18. doi: 10.1037/a0021017
Arnold, J. C., Kremer, K., and Mayer, J. (2014). Understanding students' experiments—what kind of support do they need in inquiry tasks? Int. J. Sci. Educ. 36, 2719–2749. doi: 10.1080/09500693.2014.930209
Atkinson, R. K., Derry, S. J., Renkl, A., and Wortham, D. (2000). Learning from examples: instructional principles from the worked examples research. Rev. Educ. Res. 70, 181–214. doi: 10.3102/00346543070002181
Ausubel, D. P. (1964). The transition from concrete to abstract cognitive functioning: theoretical issues and implications for education. J. Res. Sci. Teach. 2, 261–266. doi: 10.1002/tea.3660020324
Baars, M., van Gog, T., de Bruin, A., and Paas, F. (2018). Accuracy of primary school children's immediate and delayed judgments of learning about problem-solving tasks. Stud. Educ. Evaluat. 58, 51–59. doi: 10.1016/j.stueduc.2018.05.010
Baddeley, A. (1986). Oxford Psychology Series, No. 11. Working Memory. New York, NY: Clarendon Press/Oxford University Press.
Bandura, A. (1977). Self-efficacy: toward a unifying theory of behavioral change. Psychol. Rev. 84, 191–215. doi: 10.1037/0033-295X.84.2.191
Bandura, A. (1986). Social Foundations of Thought and Action: A Social Cognitive Theory. Englewood Cliffs, NJ: Prentice-Hall.
Bates, D., Mächler, M., Bolker, B., and Walker, S. (2015). Fitting linear mixed-effects models using lme4. J. Stat. Softw. 67, 1–48. doi: 10.18637/jss.v067.i01
Bell, R. L., Smetana, L., and Binns, I. (2005). Simplifying inquiry instruction. Sci. Teach. 72, 30–33.
Bjork, E. L., and Bjork, R. A. (2014). “Making things hard on yourself, but in a good way: Creating desirable difficulties to enhance learning,” in Psychology and the Real World: Essays Illustrating Fundamental Contributions to Society, 2nd Edn, eds M. A. Gernsbacher and J. Pomerantz (New York, NY: Worth), 59–68.
Bjork, R. A., and Bjork, E. L. (1992). “A new theory of disuse and an old theory of stimulus fluctuation,” in From Learning Processes to Cognitive Processes: Essays in Honor of William K. Estes, Vol. 2, eds A. Healy, S. Kosslyn, and R. Shiffrin (Hillsdale, NJ: Erlbaum), 35–67.
Blandin, Y., Lhuisset, L., and Proteau, L. (1999). Cognitive processes underlying observational learning of motor skills. Q. J. Exp. Psychol. Hum. Exp. Psychol. 52 A, 957–979. doi: 10.1080/713755856
Borek, A., McLaren, B. M., Karabinos, M., and Yaron, D. (2009). “How much assistance is helpful to students in discovery learning?” in Learning in the Synergy of Multiple Disciplines. EC-TEL 2009. Lecture Notes in Computer Science, Vol. 5794, eds U. Cress, V. Dimitrova, and M. Specht (Berlin; Heidelberg: Springer).
Braaksma, M., Rijlaarsdam, G., and Bergh, H. (2002). Observational learning and the effects of model-observer similarity. J. Educ. Psychol. 94, 405–415. doi: 10.1037//0022-0663.94.2.405
Bransford, J. D., Brown, A. L., and Cocking, R. R. (2000). How People Learn. Washington, DC: National Academies Press.
Chandler, P., and Sweller, J. (1991). Cognitive load theory and the format of instruction. Cogn. Instr. 8, 293–240. doi: 10.1207/s1532690xci0804_2
Chandler, P., and Sweller, J. (1992). The split-attention effect as a factor in the design of instruction. Br. J. Educ. Psychol. 62:233–246. doi: 10.1111/j.2044-8279.1992.tb01017.x
Chandler, P., and Sweller, J. (1996). Cognitive load while learning to use a computer program. Appl. Cogn. Psychol. 10, 151–170. doi: 10.1002/(SICI)1099-0720(199604)10:2<151::AID-ACP380>3.0.CO;2-U
Chen, O., Kalyuga, S., and Sweller, J. (2016). Relations between the worked example and generation effects on immediate and delayed tests. Learn. Instr. 45, 20–30. doi: 10.1016/j.learninstruc.2016.06.007
Chen, Z., and Klahr, D. (1999). All other things being equal: acquisition and transfer of the control of variables strategy. Child Dev. 70, 1098–1120. doi: 10.1111/1467-8624.00081
Cierniak, G., Scheiter, K., and Gerjets, P. (2009). Explaining the split-attention effect: Is the reduction of extraneous cognitive load accompanied by an increase in germane cognitive load? Comput. Human Behav. 25, 315–324. doi: 10.1016/j.chb.2008.12.020
Clark, D., and Linn, M. C. (2003). Designing for knowledge integration. The impact of instructional time. J. Learn. Sci. 12, 451–493. doi: 10.1207/S15327809JLS1204_1
Collins, A., Brown, J. S., and Newman, S. E. (1989). “Cognitive apprenticeship: Teaching the crafts of reading, writing, and mathematics,” in Knowing, Learning, and Instruction: Essays in Honor of Robert Glaser, ed L. B. Resnick (Hillsdale, NJ: Lawrence Erlbaum Associates), 453–494.
Cooper, G., and Sweller, J. (1987). Effects of schema acquisition and rule automation on mathematical problem-solving transfer. J. Educ. Psychol. 79, 347–362. doi: 10.1037/0022-0663.79.4.347
Craig, R. (1956). Directed versus independent discovery of established relations. J. Educ. Psychol. 47, 223–235. doi: 10.1037/h0046768
Crippen, K. J., and Earl, B. L. (2007). The impact of web-based worked examples and self-explanation on performance, problem solving, and self-efficacy. Comput. Educ. 49, 809–821. doi: 10.1016/j.compedu.2005.11.018
Dean, D. Jr., and Kuhn, D. (2007). Direct instruction vs. discovery: the long view. Sci. Ed. 91, 384–397. doi: 10.1002/sce.20194
Faul, F., Erdfelder, E., Lang, A.-G., and Buchner, A. (2007). G*Power 3: a flexible statistical power analysis program for the social, behavioral, and biomedical sciences. Behav. Res. Methods 39, 175–191. doi: 10.3758/BF03193146
Foos, P. W., Mora, J. J., and Tkacz, S. (1994). Student study techniques and the generation effect. J. Educ. Psychol. 86, 567–576. doi: 10.1037/0022-0663.86.4.567
Furtak, E. M., Seidel, T., Iverson, H., and Briggs, D. C. (2012). Experimental and quasi-experimental studies of inquiry-based science teaching. Rev. Educ. Res. 82, 300–329. doi: 10.3102/0034654312457206
Gardiner, J. M., and Hampton, J. A. (1985). Semantic memory and the generation effect: some tests of the lexical activation hypothesis. J. Exp. Psychol. Learn. Mem. Cogn. 11, 732–741. doi: 10.1037//0278-7393.11.1-4.732
Ginns, P. (2005). Meta-analysis of the modality effect. Learn. Instr. 15, 313–331. doi: 10.1016/j.learninstruc.2005.07.001
Heller, K. A., and Perleth, C. (2000). Kognitiver Fähigkeits-Test (Rev.) für 5.-12. Klassen (KFT 5-12+ R). Göttingen: Beltz-Testgesellschaft.
Hilbert, T. S., and Renkl, A. (2009). Learning how to use a computer-based concept-mapping tool: Self-explaining examples helps. Comput. Human Behav. 25, 267–274. doi: 10.1016/j.chb.2008.12.006
Hilbert, T. S., Renkl, A., Kessler, S., and Reiss, K. (2008). Learning to prove in geometry: learning from heuristic examples and how it can be supported. Learn. Instr. 18, 54–65. doi: 10.1016/j.learninstruc.2006.10.008
Hirshman, E., and Bjork, R. A. (1988). The generation effect: support for a two-factor theory. J. Exp. Psychol. Learn. Mem. Cogn. 14, 484–494. doi: 10.1037//0278-7393.14.3.484
Hmelo-Silver, C. E., Duncan, R. G., and Chinn, C. A. (2007). Scaffolding and achievement in problem-based and inquiry learning: a response to Kirschner, Sweller, and Clark (2006). Educ. Psychol. 42, 99–107. doi: 10.1080/00461520701263368
Hof, S. (2011). Wissenschaftsmethodischer kompetenzerwerb durch forschendes lernen: entwicklung und evaluation einer interventionsstudie. Ph.D. thesis, Universität Kassel, Kassel, Germany.
Hoogerheide, V., Loyens, S. M. M., and van Gog, T. (2014). Effects of creating video-based modeling examples on learning and transfer. Learn. Instr. 33, 108–119. doi: 10.1016/j.learninstruc.2014.04.005
Hübner, S., Nückles, M., and Renkl, A. (2010). Writing learning journals: instructional support to overcome learning-strategy deficits. Learn. Instr. 20, 18–29. doi: 10.1016/j.learninstruc.2008.12.001
Jacoby, L. L. (1978). On interpreting the effects of repetition: Solving a problem versus remembering a solution. J. Verbal Learn. Verbal Behav. 17, 649–667. doi: 10.1016/S0022-5371(78)90393-6
Janoschek, K. (2009). Empirische studie zum kumulativen kompetenzaufbau des experimentierens mit lebenden tieren (asseln). Ph.D. thesis, Universtät Wien, Wien, Austria.
Johnson, M. A., and Lawson, A. E. (1998). What are the relative effects of reasoning ability and prior knowledge on biology achievement in expository and inquiry classes? J. Res. Sci. Teach. 35, 89–103. doi: 10.1002/(SICI)1098-2736(199801)35:1<89::AID-TEA6>3.0.CO;2-J
Kaiser, I., Mayer, J., and Malai, D. (2018). Self-generation in the context of inquiry-based learning. Front. Psychol. 9:2440. doi: 10.3389/fpsyg.2018.02440
Kalyuga, S., Ayres, P., Chandler, P., and Sweller, J. (2003). The expertise reversal effect. Educ. Psychol. 38, 23–31. doi: 10.1207/S15326985EP3801_4
Kalyuga, S., Chandler, P., Tuovinen, J., and Sweller, J. (2001). When problem solving is superior to studying worked examples. J. Educ. Psychol. 93, 579–588. doi: 10.1037/0022-0663.93.3.579
Kant, J. M., Scheiter, K., and Oschatz, K. (2017). How to sequence video modeling examples and inquiry tasks to foster scientific reasoning. Learn. Instr. 52, 46–58. doi: 10.1016/j.learninstruc.2017.04.005
Kirschner, P. A., Sweller, J., and Clark, R. E. (2006). Why minimal guidance during instruction does not work: an analysis of the failure of constructivist, discovery, problem-based, experiential, and inquiry-based teaching. Educ. Psychol. 41, 75–86. doi: 10.1207/s15326985ep4102_1
Klahr, D. (2000). Exploring Science: The Cognition and Development of Discovery Processes. Cambridge, MA: MIT Press.
Klahr, D., and Dunbar, K. (1988). Dual space search during scientific reasoning. Cogn. Sci. 12, 1–48. doi: 10.1207/s15516709cog1201_1
Klahr, D., and Nigam, M. (2004). The equivalence of learning paths in early science instruction: effect of direct instruction and discovery learning. Psychol. Sci. 15, 661–667. doi: 10.1111/j.0956-7976.2004.00737.x
Kühl, T., Scheiter, K., Gerjets, P., and Edelmann, J. (2011). The influence of text modality on learning with static and dynamic visualizations. Comput. Human Behav. 27, 29–35. doi: 10.1016/j.chb.2010.05.008
Kuhn, D., and Dean, D. (2005). Is developing scientific thinking all about learning to control variables? Psychol. Sci. 16, 866–870. doi: 10.1111/j.1467-9280.2005.01628.x
Landis, J. R., and Koch, G. G. (1977). The measurement of observer agreement for categorical data. Biometrics 33:159. doi: 10.2307/2529310
Lazonder, A. W., and Harmsen, R. (2016). Meta-analysis of inquiry-based learning. Rev. Educ. Res. 86, 681–718. doi: 10.3102/0034654315627366
Leahy, W., and Sweller, J. (2011). Cognitive load theory, modality of presentation and the transient information effect. Appl. Cogn. Psychol. 25, 943–951. doi: 10.1002/acp.1787
Lenth, R. V. (2016). Least-squares means: the R package lsmeans. J. Stat. Softw. 69:1–33. doi: 10.18637/jss.v069.i01
Leppink, J., Paas, F., van Gog, T., van der Vleuten, C. P. M., and van Merriënboer, J. J. G. (2014). Effects of pairs of problems and examples on task performance and different types of cognitive load. Learn. Instr. 30, 32–42. doi: 10.1016/j.learninstruc.2013.12.001
Linn, M. C., Pulos, S., and Gans, A. (1981). Correlates of formal reasoning: content and problem effects. J. Res. Sci. Teach. 18, 435–447. doi: 10.1002/tea.3660180507
Lutz, J., Briggs, A., and Cain, K. (2003). An examination of the value of the generation effect for learning new material. J. Gen. Psychol. 130, 171–188. doi: 10.1080/00221300309601283
Mayer, J. (2007). “Erkenntnisgewinnung als wissenschaftliches Problemlösen,” in Theorien in der Biologiedidaktischen Forschung: Ein Handbuch für Lehramtsstudenten und Doktoranden, eds D. Krüger and H. Vogt (Berlin: Springer), 177–186.
Mayer, J., Grube, C., and Möller, A. (2008). “Kompetenzmodell naturwissenschaftlicher erkenntnisgewinnung [Competence model for scientific inquiry],” in Lehr- und Lernforschung in der Biologiedidaktik, eds U. Harms and A. Sandmann (Innsbruck: Studien Verlag), 63–79.
Mayer, J., and Ziemek, H. P. (2006). Offenes experimentieren. forschendes lernen im biologieunterricht. Unterr. Biol. 317, 4–12.
Mayer, R. (2004). Should there be a three-strikes rule against pure discovery learning? The case for guided methods of instruction. Am. Psychol. 59, 14–19. doi: 10.1037/0003-066X.59.1.14
Mayer, R. E., and Moreno, R. (1998). A split-attention effect in multimedia learning: evidence for dual processing systems in working memory. J. Educ. Psychol. 90, 312–320. doi: 10.1037/0022-0663.90.2.312
McDaniel, M. A., Waddill, P. J., and Einstein, G. O. (1988). A contextual account of the generation effect: a three-factor theory. J. Mem. Lang. 27, 521–536. doi: 10.1016/0749-596X(88)90023-X
McLaren, B. M., Lim, S., and Koedinger, K. R. (2008). “When and how often should worked examples be given to students? New results and a summary of the current state of research,” in Proceedings of the 30th Annual Conference of the Cognitive Science Society, eds B. C. Love, K. McRae, and V. M. Sloutsky (Austin, TX: Cognitive Science Society), 2176–2181.
Meier, M., and Wulff, C. (2014). “Daphnia magna as the ultimate classroom organism: Implementing scientific investigations into school practice,” in Daphnia: Biology and Mathematics Perspectives, ed M. O. El-Doma (New York, NY: Nova Science Publishers Inc.), 225–244.
Morris, C. D., Bransford, J. D., and Franks, J. J. (1977). Levels of processing versus transfer appropriate processing. J. Verbal Learn. Verbal Behav. 16, 519–533. doi: 10.1016/S0022-5371(77)80016-9
Mousavi, S. Y., Low, R., and Sweller, J. (1995). Reducing cognitive load by mixing auditory and visual presentation modes. J. Educ. Psychol. 87, 319–334. doi: 10.1037/0022-0663.87.2.319
Mulder, Y. G., Lazonder, A. W., and de Jong, T. (2014). Using heuristic worked examples to promote inquiry-based learning. Learn. Instr. 29, 56–64. doi: 10.1016/j.learninstruc.2013.08.001
Nairne, J. S., and Widner, R. L. (1987). Generation effects with nonwords: the role of test appropriateness. J. Exp. Psychol. Learn. Mem. Cogn. 13, 164–171. doi: 10.1037/0278-7393.13.1.164
National Research Council (2013). Next Generation Science Standards. Washington, DC: National Academies Press.
OECD (2007). PISA 2006: Science Competencies for Tomorrow's World. Volume 1: Analysis. Paris: OECD. Retrieved from http://www.nbbmuseum.be/doc/seminar2010/nl/bibliografie/opleiding/analysis.pdf. doi: 10.1787/9789264040014-en
Paas, F., and van Merriënboer, J. (1994). Variability of worked examples and transfer of geometrical problem solving skills: a cognitive-load approach. J. Educ. Psychol. 86, 122–133. doi: 10.1037/0022-0663.86.1.122
Payne, D. G., Neely, J. H., and Burns, D. J. (1986). The generation effect: Further tests of the lexical activation hypothesis. Mem. Cogn. 14, 246–252. doi: 10.3758/BF03197700
Preckel, F. (2014). Assessing need for cognition in early adolescence. Eur. J. Psychol. Assess. 30, 65–72. doi: 10.1027/1015-5759/a000170
R Core Team (2018). R: A Language and Environment for Statistical Computing. Vienna: R Foundation for Statistical Computing. Available online at: http://www.R-project.org/
Renkl, A. (1997). Learning from worked-out examples: a study on individual differences. Cogn. Sci., 21, 1–29. doi: 10.1207/s15516709cog2101_1
Renkl, A. (2014). “Learning from worked examples: how to prepare students for meaningful problem solving,” in Applying Science of Learning in Education: Infusing Psychological Science into the Curriculum, eds V. A. Benassi, C. E. Overson, and C. M. Hakala (Washington, DC: Society for the Teaching of Psychology), 118–130.
Richland, L. E., Linn, M. C., and Bjork, R. A. (2007). “Cognition and instruction: Bridging laboratory and classroom settings,” in Handbook of Applied Cognition, 2nd Edn., eds F. Durso, R., Nickerson, S., Dumais, S. Lewandowsky, and T. Perfect (West Sussex: John Wiley & Sons Ltd.), 555–583.
Rittle-Johnson, B., and Kmicikewycz, A. O. (2008). When generating answers benefits arithmetic skill: the importance of prior knowledge. J. Exp. Child Psychol. 101, 75–81. doi: 10.1016/j.jecp.2008.03.001
Roth, W.-M., Bowen, G. M., and McGinn, M. K. (1999). Differences in graph-related practices between high school biology textbooks and scientific ecology journals. J. Res. Sci. Teach. 36, 977–1019. doi: 10.1002/(SICI)1098-2736(199911)36:9<977::AID-TEA3>3.0.CO;2-V
Rummel, N., and Spada, H. (2005). Learning to collaborate: an instructional approach to promoting collaborative problem solving in computer-mediated settings. J. Learn. Sci. 14, 201–241. doi: 10.1207/s15327809jls1402_2
Rummel, N., Spada, H., and Hauser, S. (2009). Learning to collaborate while being scripted or by observing a model. Comput. Support. Learn. 4, 69–92. doi: 10.1007/s11412-008-9054-4
Salden, R. J. C. M., Koedinger, K. R., Renkl, A., Aleven, V., and McLaren, B. M. (2010). Accounting for beneficial effects of worked examples in tutored problem solving. Educ. Psychol. Rev. 22, 379–392. doi: 10.1007/s10648-010-9143-6
Scheiter, K., Gerjets, P., and Schuh, J. (2004). “The impact of example comparisons on schema acquisition: do learners really need multiple examples?,” in Proceedings of the 6th International Conference on Learning Sciences, eds Y. B. Kafai, W. A. Sandova, N. Enyedy, A. S. Nixon, and F. Herrera (Mahwah, NJ: Erlbaum), 457–464. Retrieved from http://dl.acm.org/citation.cfm?id=1149182
Schwab, J. (1962). “The teaching of science as enquiry”, in The Teaching of Science, eds J. Schwab and P. Brandwein (Cambridge, MA: Harvard University Press), 1–103.
Schwichow, M., Croker, S., Zimmerman, C., Höffler, T., and Härtig, H. (2016). Teaching the control-of-variables strategy: a meta-analysis. Dev. Rev. 39, 37–63. doi: 10.1016/j.dr.2015.12.001
Schwonke, R., Renkl, A., Krieg, C., Wittwer, J., Aleven, V., and Salden, R. (2009). The worked-example effect: not an artefact of lousy control conditions. Comput. Human Behav. 25, 258–266. doi: 10.1016/j.chb.2008.12.011
Schworm, S., and Renkl, A. (2007). Learning argumentation skills through the use of prompts for self-explaining examples. J. Educ. Psychol. 99, 285–296. doi: 10.1037/0022-0663.99.2.285
Shrager, J., and Siegler, R. S. (1998). SCADS: a model of children's strategy choices and strategy discoveries. Psychol. Sci. 9, 405–410. doi: 10.1111/1467-9280.00076
Shulman, L., and Keisler, E. (1966). Learning by Discovery: A Critical Appraisal. Chicago: Rand McNally.
Siegler, R. S. (1991). Strategy choice and strategy discovery. Learn. Instr. 1, 89–102. doi: 10.1016/0959-4752(91)90020-9
Slamecka, N. J., and Graf, P. (1978). The generation effect. Delineation of a phenomenon. J. Exp. Psychol. Hum. Learn. Mem. 4, 592–604. doi: 10.1037/0278-7393.4.6.592
Sneider, C., Kurlich, K., Pulos, S., and Friedman, A. (1984). Learning to control variables with model rockets: a neo-piagetian study of learning in field settings. Sci. Ed. 68, 465–486. doi: 10.1002/sce.3730680410
Sweller, J. (1994). Cognitive load theory, learning difficulty, and instructional design. Learn. Instr. 4, 295–312. doi: 10.1016/0959-4752(94)90003-5
Sweller, J. (2011). “Cognitive load theory,” in The Psychology of Learning and Motivation: Cognition in Education, Vol. 55, eds J. P. Mestre and B. H. Ross (San Diego, CA: Elsevier Academic Press), 37–76. doi: 10.1016/B978-0-12-387691-1.00002-8
Sweller, J., and Cooper, G. A. (1985). The use of worked examples as a substitute for problem solving in learning algebra. Cogn. Instr. 2, 59–89. doi: 10.1207/s1532690xci0201_3
Sweller, J., and Sweller, S. (2006). Natural information processing systems. Evol. Psychol. 4, 434–458. doi: 10.1177/147470490600400135
Sweller, J., Van Merrienboer, J. J. G., and Paas, F. (1998). Cognitive architecture and instructional design. Educ. Psychol. Rev. 10. 251–296. doi: 10.1023/A:1022193728205
van Gog, T., Jarodzka, H., Scheiter, K., Gerjets, P., and Paas, F. (2009). Attention guidance during example study via the model's eye movements. Comput. Human Behav. 25, 785–791. doi: 10.1016/j.chb.2009.02.007
van Gog, T., Kester, L., and Paas, F. (2011). Effects of worked examples, example-problem, and problem-example pairs on novices' learning. Contemp. Educ. Psychol. 36, 212–218. doi: 10.1016/j.cedpsych.2010.10.004
van Gog, T., and Rummel, N. (2010). Example-based learning: integrating cognitive and social-cognitive research perspectives. Educ. Psychol. Rev. 22. 155–174. doi: 10.1007/s10648-010-9134-7
van Gog, T., Verveer, I., and Verveer, L. (2014). Learning from video modeling examples: effects of seeing the human model's face. Comput. Educ. 72, 323–327. doi: 10.1016/j.compedu.2013.12.004
van Merriënboer, J. J. G., and Sweller, J. (2005). Cognitive load theory and complex learning: recent developments and future directions. Educ. Psychol. Rev. 17, 147–177. doi: 10.1007/s10648-005-3951-0
vanLehn, K. (1996). Cognitive skill acquisition. Annu. Rev. Psychol. 47, 513–539. doi: 10.1146/annurev.psych.47.1.513
Vollmeyer, R., and Burns, B. D. (1996). Hypotheseninstruktion und zielspezifität: bedingungen, die das erlernen und kontrollieren eines komplexen systems beeinflussen. Z. Exp. Psychol. 43, 657–683.
Wellnitz, N., and Mayer, J. (2016). “Methoden der erkenntnisgewinnung im biologieunterricht,” in Biologiedidaktische Forschung: Schwerpunkte und Forschungsstände, eds A. Sandmann and P. Schmiemann (Berlin: Logos Verlag Berlin), 61–82.
Zimmerman, B. J., and Kitsantas, A. (2002). Acquiring writing revision and self-regulatory skill through observation and emulation. J. Educ. Psychol. 94, 660–668. doi: 10.1037/0022-0663.94.4.660
Keywords: inquiry(-based) learning, example-based learning, scientific reasoning skills, control of variables strategy, video modeling example, prior knowledge, cognitive load
Citation: Kaiser I and Mayer J (2019) The Long-Term Benefit of Video Modeling Examples for Guided Inquiry. Front. Educ. 4:104. doi: 10.3389/feduc.2019.00104
Received: 15 February 2019; Accepted: 11 September 2019;
Published: 01 October 2019.
Edited by:
Mark Lattery, University of Wisconsin–Oshkosh, United StatesReviewed by:
Ruomeng Zhao, LinkedIn, United StatesVincent Hoogerheide, Utrecht University, Netherlands
Copyright © 2019 Kaiser and Mayer. This is an open-access article distributed under the terms of the Creative Commons Attribution License (CC BY). The use, distribution or reproduction in other forums is permitted, provided the original author(s) and the copyright owner(s) are credited and that the original publication in this journal is cited, in accordance with accepted academic practice. No use, distribution or reproduction is permitted which does not comply with these terms.
*Correspondence: Irina Kaiser, aS5rYWlzZXJAdW5pLWthc3NlbC5kZQ==