Predicting Visuospatial and Verbal Working Memory by Individual Differences in E-Learning Activities
- 1Department of Applied Educational Science, Umeå University, Umeå, Sweden
- 2Department of Computer Science and Media Technology, Linnaeus University, Växjö, Sweden
- 3Hypocampus AB, Gothenburg, Sweden
E-learning is being considered as a widely recognized option to traditional learning environments, allowing for highly tailor-made adaptive learning paths with the goal to maximize learning outcomes. However, for being able to create personalized e-learning systems, it is important to identify relevant student prerequisites that are related learning success. One aspect crucial for all kind of learning that is relatively unstudied in relation to e-learning is working memory (WM), conceptualized as the ability to maintain and manipulate incoming information before it decays. The aim of the present study was to examine how individual differences in online activities is related to visuospatial- and verbal WM performance. Our sample consisted of 98 participants studying on an e-learning platform. We extracted 18 relevant features of online activities tapping on Quiz accuracy, Study activity, Within-session activity, and Repetitive behavior. Using best subset multiple regression analyses, the results showed that individual differences in online activities significantly predicted verbal WM performance (p < 0.001, = 0.166), but not visuospatial WM performance (p = 0.058, = 0.065). The obtained results contribute to the existing research of WM in e-learning environments, and further suggest that individual differences in verbal WM performance can be predicted by how students interact on e-learning platforms.
Introduction
As a result of digitalization, more and more educational content has been transferred from teacher-centered teaching to online-based learning platforms. Such learning platforms, often called e-learning, can be defined as individualized instructions distributed over public or private computer networks (Manochehr, 2006). Thus, compared to traditional desktop-teaching, e-learning platforms have the potential to register all kind of online activities, allowing researchers, and teachers to observe learners' behaviors throughout the learning process and with the use of data mining and computerized algorithms, to quickly identify and analyze trends in big datasets (Truong, 2016). However, the digitalized nature of e-learning results in less face-to-face interaction with teachers or instructors, reducing the possibility to detect needs for changes in instructional and individual scaffolding. It is therefore vital that e-learning platforms can be tailored according to learners' individual needs and prerequisites. Accordingly, both explanatory studies using traditional statistical techniques (Coldwell et al., 2008; Dawson et al., 2008; Macfadyen and Dawson, 2010; Yu and Jo, 2014; Zacharis, 2015) as well as predictive analytics studies using machine learning- and neural networks (Lykourentzou et al., 2009; Hu et al., 2014; Sayed and Baker, 2015; Costa et al., 2017; Shelton et al., 2017) have been conducted to gain better insights in online learning activities with the aim to optimize and tailor e-learning platforms according to students' individual needs (e.g., Thalmann, 2014; Truong, 2016).
While most of the previous studies have been focusing on the direct relationship between online activities on e-learning platforms and learning outcomes, very few studies have studied the inter-individual differences in cognitive profiles, and how these differences can be predicted by online activity. It is therefore important to identify relevant user activity features that predicts WM, having the potential to act as substitute measures of cognitive capacity without the necessity to test every single student using the platform with time-consuming and tedious cognitive assessments. A cognitive component possessing a pivotal role in all kind of learning is working memory (WM). WM refers to the ability to maintain, access, and manipulate incoming information (Baddeley, 2000; Oberauer et al., 2003), thus serving as the mental workspace for ongoing cognitive activities. Several theories concerning the function of WM has been put forth (e.g., Baddeley and Hitch, 1974; Ericsson and Kintsch, 1995; Cowan, 2001). In this study, the seminal multicomponent proposed model by Baddeley and Hitch (1974) will serve as the theoretical reference. The multicomponent model suggest that WM comprise two autonomous slave systems, the phonological loop and the visospatial sketchpad, along with a central executive that interacts with the two slave systems (Baddeley and Hitch, 1974), and the more recently added episodic buffer (Baddeley, 2000). The phonological loop with its subvocal rehearsal and phonological storage components is most directly relevant for remembering verbal information and learning, whereas the visuospatial sketchpad is responsible for remembering spatial and visual images and visuospatial learning. The functioning of the two domain-specific slave systems are typically measured with simple span tasks, prompting participants to memorize increasingly long strings of items followed by an immediate recall without further processing (Van de Weijer-Bergsma et al., 2015). Given that most of the content on e-learning platforms are presented in textual form, particularly verbal WM plays a pivotal role to facilitate the to-be-learned materials in long-term memory (Gathercole and Alloway, 2008). However, visuospatial WM is relevant for linguistic processing as well. It has been suggested that visuospatial WM, particularly among those with higher-level reading skills, allows for ortographic processing, and thus a more efficient long-term memory encoding of regular and irregular patterns of words (Badian, 2005; Ehri, 2005; Pham and Hasson, 2014).
Another theoretical framework related to e-learning, and partly building upon the multicomponent model of WM, is the cognitive load theory (Sweller, 1988). The cognitive load theory suggests that learning can be best explained by an information-processing model of human cognition, in which novel memoranda needs to be processed by WM before it can be manifested in long-term memory (Van Merriënboer and Sweller, 2005; Sweller, 2010). As WM has a limited capacity, the cognitive load theory pinpoints the importance of reducing unnecessary WM loads, allowing students' to direct their WM capacity to merely learning-related processes (Anmarkrud et al., 2019). In other words, if the WM capacity threshold is exceeded, it could result to a cognitive overload, and thereby to inhibited learning outcomes (Kalyuga, 2011).
Several studies have examined how low vs. high WM individuals differ in traditional classroom learning activities. It has, for instance, been shown that low WM individuals have poorer performances in school subjects such as arithmetics (Gathercole and Pickering, 2001; Swanson and Beebe-Frankenberger, 2004; Raghubar et al., 2010), and reading comprehension (Daneman and Carpenter, 1980; Turner and Engle, 1989). It has also been found that those with poorer WM performance tend to perform worse in quiz tasks assessed immediately following the learning phase (Wiklund-Hörnqvist et al., 2014; Agarwal et al., 2017; Bertilsson et al., 2017), including poorer performances in final course exams (Turner and Engle, 1989; Aronen et al., 2005; Cowan et al., 2005). As for the more specific differences in learning activities, WM has shown to predict how frequently the learner goes back and repeat earlier learned materials such that those with poorer WM tend to go back and read earlier material more frequently as compared to those with better WM performance (Rosen and Engle, 1997; Kemper et al., 2004). It has also been shown that WM is highly involved in how much time the learner is spending on a particular quiz: learners with poor WM spend more time on reading the to-be-learned materials compared to high WM individuals (Engle et al., 1992; Calvo, 2001; Kemper and Liu, 2007).
Although it has been extensively investigated how learning activities and WM performance are interrelated in lab- and classroom-based settings, only a few studies have investigated how individual differences in learning activities predicts WM performance on online-based e-learning platforms (Huai, 2000; Banas and Sanchez, 2012; Rouet et al., 2012; Skuballa et al., 2012). In a Ph.D. thesis by Huai (2000), the author examined whether serial vs. holistic learning style, and linear vs. non-linear navigational patterns in an e-learning course was related to WM performance. Here, the results showed that students with higher WM performance were more prone to focus on a linear navigation of the to-be-learned materials whereas those with lower WM performance followed the course material in a more non-linear fashion. In a study by Rouet et al. (2012), the authors examined the impact WM had on students' incidental learning when faced with simple hierarchical hypertextual documents on a website. The results showed that individuals with low WM performance tended to lose track of the structure in the to-be-learned materials as they navigated deeper in the text structure. Skuballa et al. (2012) investigated whether low vs. high WM individuals were more prone to utilize externally provided (a) visual cues or (b) verbal instructions when practicing with an online-based audiovisual animation sequence for 5 minutes. Employing a pre-posttest design, the results on the learning outcomes showed that low WM individuals tended to benefit more of visual cues whereas high WM individuals tended to benefit more of verbal information. Banas and Sanchez (2012) probed for the impact WM had on individual differences in online activities among participants that read a web-based text about plant taxonomy, while simultaneously completing a secondary search task tapping on simple factual quizzes. The results showed that participants with better WM performance improved in their implicit understanding of the relationships underlying the to-be-learned materials, whereas those with poorer WM performance did not.
In summary, WM is linked to a multitude of features related to learning success (Daneman and Carpenter, 1980; Daneman and Merikle, 1996; Rosen and Engle, 1997; Aronen et al., 2005; Cowan et al., 2005), and the scarce evidence in the context of e-learning indicates that WM affects aspects such as how linearly the student follows the to-be-learned materials (Huai, 2000), and which kind of cues on the platform the student use for memorization (Skuballa et al., 2012). This suggest that WM constitute a significant factor for profiling and personalizing e-learning platforms (Kalyuga and Sweller, 2005; Tsianos et al., 2010; Banas and Sanchez, 2012). Hence, being able to identify relevant user activity features that predicts WM without the burden of testing every single student using the platform is an important step toward more personalized e-learning platforms. Considering the few studies on individual differences and e-learning, more research is needed to gain deeper insights regarding the relationship between online activities and how it can be used to predict students' WM performance.
The aim of the present study was to examine whether individual differences in online e-learning activities would be predictive of visuospatial and verbal WM performance. More specifically, we sought to identify which online activity features that are most strongly related to WM, and to untangle whether online activity is differently related to WM performance depending on the subcomponent which is analyzed (i.e., visuospatial and verbal subcomponent). The data for this study stems from an interactive e-learning platform in Sweden titled Hypocampus (https://www.hypocampus.se). To date, approximately 15,000 students use Hypocampus as a platform for carrying out university courses, with most of the users consisting of medical students. The e-learning platform provides the students with compressed course materials that are highly relevant for the to-be-completed courses at their universities. In other words, instead of completing the course by reading textual literature from the books, the content of the course is transferred to the interactive e-learning platform. Hypocampus also provides a high degree of learner control, with a broad array of courses covering different topics in medicine that students can complete non-linearly in their own pace (i.e., they can choose to jump back and forth from a course to another), thus resembling typical massive open online platforms (MOOCs; Kaplan and Haenlein, 2016). Hence, in line with previous MOOC studies (Brinton and Chiang, 2015; Kloft et al., 2015), we focused on extracting only features stemming from clickstream data in the present study. Hypocampus has also implemented an evidence-based strategy purported to facilitate learning, namely retrieval practice (for a review, see Dunlosky et al., 2013). Retrieval practice prompts students to deliberately recall the to-be-learned materials, thereby serving as so-called self-testing of what one has learned. This strategy is implemented at the end of each learning section in either multiple-choice format (i.e., a question followed by four alternatives out of which one is correct) or in open-ended format (i.e., a question followed by an empty box where the student type his/her answer).
Altogether 18 online activity features were extracted from the e-learning platform which were based on existing work (e.g., Jovanovic et al., 2012; Tortorella et al., 2015; Zacharis, 2015). Specifically, we divided these features into four broader subdomains of online activity, namely Quiz accuracy, Study activity, Within-session activity, and Repetitive behavior. Quiz accuracy pertains to features capturing how accurately one has been responding on quizzes related to the to-be-learned materials. As quiz accuracy measures have been linked to WM performance in previous work (Wiklund-Hörnqvist et al., 2014; Bertilsson et al., 2017), we deemed it important to extract such features for the present study as well. Study activity refers to features capturing how active the student has been on the platform (e.g., time spent in the system, the number of reading sessions, number of quizzes taken) whereas Within-session activity refers to features capturing student activities within a session (e.g., average reading- and quiz times per session). Both of these subdomains were motivated to be included in the present study, as prior evidence stemming from behavioral and eye-tracking studies shows that those with poorer WM performance tend to spend more time on the to-be-learned materials as compared to those with better WM performance (Engle et al., 1992; Calvo, 2001; Kemper and Liu, 2007). This raises the intriguing question whether a similar pattern could be observed in the context of e-learning. Lastly, the subdomain Repetitive behavior relates to features that taxes how often, and how much individuals repeat previously learned materials. The reason for including measures of repetitive behavior was also theoratically motivated; results from previous studies shows that high WM individuals tend to repeat less compared with low WM individuals (Rosen and Engle, 1997; Kemper et al., 2004).
For capturing WM, we administered a Block span tapping on visuospatial WM (e.g., Vandierendonck et al., 2004) and a Digit span tapping on verbal WM (D'Amico and Guarnera, 2005). In the light of the theoretical framework of WM (Baddeley and Hitch, 1974; Baddeley, 2000, 2003), we hypothesized that both subcomponents of WM would be related to individual differences in online activities. However, as the majority of the content on the e-learning platform involves reading, we expected that the Digit span would show stronger relationships with online activity since they both rely heavily on verbal WM. This assumption was based on the theoretical framework of WM (Baddeley and Hitch, 1974) and evidence from cross-sectional- and longitudinal studies, showing that verbal WM tasks typically are more sensitive for predicting academic achievements with verbal content as compared with visuospatial WM tasks (e.g., Pazzaglia et al., 2008; Gropper and Tannock, 2009; Van de Weijer-Bergsma et al., 2015).
Lastly, one should point out that there has been relatively mixed approaches in previous studies as whether WM performance has been regressed on online activities (Pazzaglia et al., 2008; Banas and Sanchez, 2012) or vice versa (Huai, 2000). In the present study, we used best subset multiple regression analyses where the online activity features served as features for predicting the subcomponents of WM (note that the subcomponents were analyzed in separate models). If we would identify online activity features that shows strong associations with WM performance, they could serve as proxy estimates of WM performance, paving way for a fast screening of the students' cognitive profiles, while simultaneously circumventing the burden of assessing each students' WM capacity which is both cognitively demanding and time-consuming from the students' perspective. Thus, this choice of direction in the present study (i.e., online activity features as predictors of WM) was justified (for a discussion, see Chang et al., 2015).
Methods
Hypocampus
As mentioned earlier, Hypocampus is a web-based e-learning platform primarily used by ca. 15000 medical students. Every day that a student uses the platform a vast amount of interactional data is generated. This data is collected using JavaScript methods available in the user's browser and stored in the backend system in a database. Hypocampus offers a high degree of learner control in a wide range of courses in medicine which the students' can complete non-linearly, while also having the possibility to study in their own pace and complete the courses and learning sections in the order of their own choice. Moreover, for reducing extraneous cognitive load, the to-be-learned materials is presented in an easily and understandable way at the platform with clear headings, sub-headings, and additional features such as highlighting the most important information, including tables that summarizes the learning section with bullet points. The platform also provides images and video clips as supporting tools for facilitating the to-be-learned materials (note, however, that the images and video clips occurs on the platform only occasionally, and thus to a much lesser extent than the written materials).
Participants and Procedure
The participants in the present study consisted of students who were studying on an e-learning platform (titled Hypocampus) to prepare themselves for the actual exam at the university. Most of the enrolled participants were medical students studying either at the Umeå University, Karolinska Institute, Gothenburg University, Lund University, Uppsala University, Örebro University, or Linköping University. The study was approved by the regional vetting committee (2017/517-31), Sweden, and informed consent was obtained from all participants. Moreover, as this was the first data collection conducted on Hypocampus, we did not gather any demographical data from the participants (i.e., we considered it important to maintain maximum anonymity of the students)1. Furthermore, we did not state any prior inclusion criteria for being an eligible participant for the present study.
For capturing individual differences in WM, we invited a sub-sample of 1,000 randomly selected students at Hypocampus to complete the test session consisting of four cognitive tasks tapping on working memory and episodic memory (duration ca. 1 h). The test session was administered online using an in-house developed web-based test platform by sending a link to the participants via their email who completed the experiment on a computer of their choosing (e.g., Röhlcke et al., 2018). Of the 1,000 invited participants, 272 of them completed the test session to the end. However, prior to data collection, we did not set any threshold for being an eligible participant with respect to study activity. Thus, we noticed afterwards that the test takers were highly varying in terms of how much they had been spent studying at Hypocampus. For leveling out those who only was visiting the platform from those that actually used the platform for studying, we first excluded participants that had been active only once during the first 100 days since registering themselves on the system (i.e., only one login session). Moreover, we identified two additional exclusion criteria deemed adequate for eliminate non-active participants: those that had been completing >10 separate study sessions, and those that had completed >50 quizzes. Together, these cut-off criteria resulted in a final sample size of 98 eligible participants.
Feature Extraction
As depicted in Table 1, 18 features were extracted from the online e-learning platform in order to untangle individual differences in online activity. The extracted features were based on existing work, and could broadly be classified into four separate categories as follows: Quiz accuracy, Study activity, Within-session activity, and Repetitive behavior (see below for more detailed information of the features belonged to each category).
Quiz Accuracy
This category encompassed three different measures of quiz accuracy: quiz mc accuracy, quiz txt accuracy, and quiz both accuracy. As previously mentioned, participants were prompted with optional quizzes following each learning session at Hypocampus following the principles of retrieval practice (Dunlosky et al., 2013) which could be either in multiple-choice format or open-ended format. In the multiple choice quizzes (titled quiz mc accuracy), participants were asked about specific information concerning the learning section followed by four alternatives out of which one was correct. Correctly recalled quiz responses were logged as “True” whereas incorrectly recalled quiz responses were logged as “False.” In the open-ended quiz format, participants were prompted with a quiz in a similar fashion as in the multiple-choice quizzes (titled as quiz txt accuracy). However, instead of being prompted with four alternatives, they were now asked to respond to the quiz in a written format by typing down their response in an empty box. Following the response, the system showed the correct answer. The scoring of the responses were self-corrected, meaning that the participants were to tick either on a red box with a text stating Read more (corresponding to an incorrectly recalled quiz and marked as False in the log file) or a green box with a text stating I knew this (corresponding to a correctly recalled quiz and marked as True in the log file). Due to the differences in the scoring procedures, we extracted one feature encompassing the proportion of correct responses in the multiple-choice quizzes, and one feature encompassing the proportion of correct responses in the open ended quizzes. We also summed together the proportion of correct responses from both quizzes, titled quiz both accuracy. We calculated the proportion scores (i.e., quiz accuracies) for each participant using the following formula:
Study Activity
The study activity features captured different measures of how often the participants have been using the platform during the first 100 days of studying. The feature reading sessions consist of the total amount of times the participant have logged in to his/her account and read some materials on the platform, whereas quiz sessions tallies the number of times the participants have logged in to his/her account and responded to one or more quizzes. Quizzes refer to how many quizzes the participant has responded to in total, whereas video clips depicts how many times participants have been clicking on video-clips. The features, quiz time, reading time, answer time, and review time are different measures of the total amount of time the participants have been reading specific sections of the platform (see Table 1 for more information).
Within-Session Activity
We extracted four time features tapping on individual differences in reading activity within a session: reading time avg, answer time avg, review time avg, and quiz time avg. All these features were calculated by averaging the reading time stemming from all reading sessions for each student. Specifically, reading time avg captured the average reading time a student spent on reading the to-be-learned materials per session, answer time avg captured the average time a student spent on reading the question before responding to the quizzes per session, and review time avg captured the average time a student spent on reviewing the correct answers following a quiz per session. The quiz time avg sums up the answer time avg and review time avg.
Repetitive Behavior
Repetition of previously learned materials have shown to be related to WM performance (Rosen and Engle, 1997; Kemper et al., 2004). Thus, we extracted three features tapping on repetitive behavior: repetitions avg, repetitions prop, and repetitions count. Generally, these features measured individual differences in how often the participants go back and repeat previously answered quizzes. The repetitions avg measures the average number of repetitions made in a learning session, the repetitions prop is the proportion of repetitions in relation to the total amount of completed quizzes, and the repetitions count is the number of times a student went back and repeated earlier completed quizzes.
Working Memory Tasks
The WM tasks included in the study comprised two different Simple span tasks, the Block span task and the Digit span task, both which are assumed to predominantly tap on WM storage (Conway et al., 2005). In simple span tasks, lists of stimulus items with varying length are to be reproduced while maintaining the order of presentation. Simple span tasks have been used extensively in the literature, and they are part of common standardized neuropsychological and IQ tests (Wechsler, 1997).
Block Span
For capturing participants' visuospatial WM, we administered a Block span. Here, 16 blue visuospatial locations were displayed on the computer screen, arranged in a 4 × 4, with one random location flashing in red color (display time 1,000 ms, interstimulus display time 1,000 ms) each time when a response was required from the participants. In each trial, the participants were prompted to memorize the sequence of locations displayed in red, and finally recall the sequence in the correct serial order with the computer mouse. All participants started with three trials of a span length of 2 (i.e., two spatial locations that had to be recalled in the correct serial order). The span length increased with +1 until the participants failed to repeat two sequences at any particular span length. As the dependent variable, we used the maximum reached span length.
Digit Span
We also administered a Digit span purported to tap on verbal WM. In this task, numbers ranging from 1 to 9 occurred on the computer screen in a random order, with each digit being displayed for 1,000 ms (interstimulus display time 500 ms). The participants were asked to respond by recalling the digits in the correct serial order by pressing the corresponding digit on the keyboard. Span lengths increased from two to a maximum of ten digits in length, with three trials for each span length. The task continued until the participants failed to repeat two sets at any given span length. As in the Block span, the maximum reached span length served as the dependent variable in the analyses for the Digit span.
Data Preprocessing
In the final dataset extracted from the e-learning platform (n = 98), some of the variables had missing data. Two participants had not responded to a single multiple-choice quiz (i.e., quiz mc accuracy) and two of the participants had not repeated a single quiz during their studies on the platform. Moreover, eight participants had missing data in the Digit span. We also observed 7 multivariate outliers using the Mahalanobis distance value χ2 table (p < 0.001; Tabachnick and Fidell, 2007) and replaced the values stemming from these participants as missing. When screening for possible univariate outliers (scores on any online activity feature that deviated more than 3.5 SD from the z-standardized group mean were defined as univariate outliers), one extreme value was noticed in the following variables and were replaced with missing values: reading sessions, quiz sessions, video clips, reading time, review time, quiz time, reading time avg, quiz time avg, answer time avg, repetitionsprop, and repetitions count. Moreover, two univariate outliers were observed in the feature repetitions avg, and likewise, replaced as missing. All missing values pertaining to the screening procedures explained above were imputed using multivariate imputations by chained equations (MICE) (van Buuren and Groothuis-Oudshoorn, 2011), allowing us to include all 98 participants in the analyses.
Results
We used Python (version 3.7) for feature extraction, and R (Version 3.5.2; R Core Team, 2016) for data preprocessing- and analyses. Table 2 depicts descriptive statistics for the extracted online activity features and the WM tasks, whereas correlations, and adjusted r-squared values between the WM tasks and the online activity features can be found in Appendix A in Supplementary Materials.
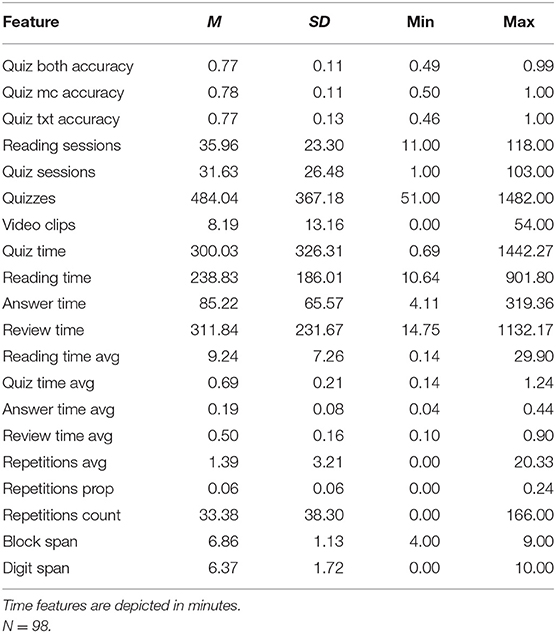
Table 2. Descriptive statistics for the extracted online activity features and the working memory tasks.
Best Subset Regression Analysis
For predicting WM performance, we employed best subset multiple regression analyses (e.g., James et al., 2013), allowing one to identify a subset of the p predictors assumed to be most related to the dependent variable. All possible combinations of the independent variables were used to create several models that predicted WM performance, and these models were examined and compared with each other by evaluating the adjusted r-squared values (). To reduce issues with multicollinearity (i.e., high intercorrelations between the predictor features), we excluded one of those variable pairs that correlated highly with each other (cut-off r > 0.80). The features excluded from the analyses were as follows: quiz both acc, answer time, quiz time, review time, answer time avg, review time avg. Thus, altogether 12 features were fed into the best subset multiple regression analyses. As the number of participants per predictor in regression analyses have been recommended to consist of at least 15 participants (e.g., Stevens, 2002), we fitted the best subset regression models with the largest subset size limited to six features [i.e., six predictors yields 16 participants per feature with respect to our sample size (n = 98)].
Block Span
From the best subset obtained on the Block span, it was found that a combination of six independent features best predicted the dependent variable. These four features were quiz txt accuracy, reading sessions, quiz sessions, reading time avg, repetitions avg, and repetitions prop. An analysis of standard residuals was carried out, showing that the data contained no outliers (Std. Residual Min = −2.48, Std. Residual Max = 2.08). The assumption of collinearity indicated that multicollinearity was not a concern (Tolerance range 0.641—0.942, VIF range 1.06−1.56). The data met the assumption of independent errors (Durbin-Watson value = 2.02). The histogram of standardized residuals revealed that the data comprised of approximately normally distributed errors. Also the normal Q-Q plot of standardized residuals showed that the points were relatively diagonally aligned (see Appendix B1 in Supplementary Materials for visual illustrations of the assumptions).
The combination of these features showed a statistically non-significant relationship between visuospatial WM and online activity [F(7, 91) = 2.126, p = 0.058], with an adjusted R2 of 0.065. A closer examination on the single coefficients (for a summary table, see Table 3) showed that no feature was significantly related to visuospatial WM performance (all p > 0.05).
Digit Span
The best subset obtained on the Digit span showed that a combination of six independent variables possessed the best predictive power on the dependent variable. The six features were as follows: quiz mc accuracy, reading sessions, quizzes, reading time reading time avg, and repetitions avg. An analysis of standard residuals was carried out, showing that the data contained one outlier participant (Std. Residual < −3.29). Thus, we excluded this outlier from the analysis, after which all standardized residuals was in an acceptable range (−2.72−2.08). The assumption of collinearity indicated that multicollinearity was not a concern (Tolerance range 0.171−0.949, VIF range 1.05−5.84) and the data met the assumption of independent errors (Durbin-Watson value = 2.24). The histogram of standardized residuals revealed that the data comprised of approximately normally distributed errors, and the normal Q-Q plot of standardized residuals showed that the points were relatively diagonally aligned (see also Appendix B2 in Supplementary Materials for visual illustrations of the assumptions).
The results from the best subset regression showed that the six online activity features explained 16.7 % ( = 0.166) of the variance in the dependent variable, and the regression equation was statistically significant [F(7, 90) = 4.195, p < 0.001]. A closer inspection of the coefficients (for a summary, see Table 4) showed that quiz mc accuracy [t(97) = 2.513, p = 0.014], and quizzes [t(97) = 2.690, p = 0.009] were positively associated with verbal WM, suggesting that those with higher proportion of correctly recalled multiple-choice quizzes and those completing more quizzes, performed better in the Digit span. Moreover, we found statistically significant negative relationships between verbal WM and reading sessions [t(97) = −2.446, p = 0.016], reading time avg [t(97) = −2.159, p = 0.034], and repetitions avg [t(97) = −3.725, p < 0.001]. This suggests that those completing more unique reading sessions and those spending more time on reading the materials tend to have lower verbal WM performance. The results also indicate that those who, on average, repeat more previously learned material during a reading session tend to perform worse in the Digit span.
Discussion
The relationship between e-learning online activities and learning outcomes has been extensively tested, but one relatively overlooked component in the context of e-learning is working memory (WM) and how individual differences in this crucial cognitive ability can be predicted by differences in online activities between students. This study aimed to examine whether individual differences in online activities on an e-learning platform would be predictive of visuospatial and verbal working memory (WM) performance. Based on previous evidence (Rogers and Monsell, 1995; Pazzaglia et al., 2008; Gropper and Tannock, 2009; Van de Weijer-Bergsma et al., 2015), and the theoretical framework of WM (Baddeley and Hitch, 1974; Baddeley et al., 1998; Baddeley, 2000), we hypothesized that particularly the verbal WM subcomponent would be related to individual differences in online activities. Being able to identify relevant user activity features that predicts WM and its subcomponents without the necessity to test every single student is an important first step towards personalized e-learning platforms according to students' cognitive profiles.
The results showed that online activities were predictive of verbal WM but not visuospatial WM, explaining nearly 17% of the performance in the former subcomponent, and only about 5% of the performance in the latter subcomponent. The relatively low correlation between the Block span and the Digit span (r = 0.287, p = 0.004) might also explain why the size of shared variance with the online activity features differed between tasks. As such, our results are in line with previous studies, showing that tasks tapping on verbal WM typically are more sensitive for predicting academic achievements with textual content compared to tasks tapping on visuospatial WM (Rogers and Monsell, 1995; Pazzaglia et al., 2008; Gropper and Tannock, 2009; Van de Weijer-Bergsma et al., 2015). As the majority of the content on the e-learning platform involves reading, it appears feasible to assume that the online activities extracted from the e-learning platform is more strongly linked to the Digit span as both the task and the to-be-learned relies heavily on processing components tapping on verbal WM (cf. Gropper and Tannock, 2009).
In the present study, we found several single features that significantly predicted verbal WM performance. One of those features was multiple-choice quizzes, indicating that individuals with higher quiz accuracies performed better in the Digit span (Wiklund-Hörnqvist et al., 2014; Bertilsson et al., 2017). Reflecting this finding to the multicomponent model of WM (Baddeley and Hitch, 1974), one could speculate whether this association mirrors differences in how effectively the subvocal rehearsal and the phonological loop is utilized, both when reading the to-be-learned materials, as well as when performing the WM task. Indeed, it has been shown that strategies such as rehearsal is adopted more often among individuals with high WM performance (McNamara and Scott, 2001; Turley-Ames and Whitfield, 2003) and this strategy might be equally effective when reading the course materials, thus reflected in improved accuracy in the quizzes.
As regards the predictors tapping on Study activity, we found that those completing less reading sessions across the first 100 days of studying tended to perform better in the Digit span as compared to those with more reading sessions. However, conversely to the aforementioned relationship, we observed a positive relationship between the amount of quizzes taken and verbal WM, indicating that those completing more quiz sessions performed better in the Digit span. The quizzes administered on the e-learning platform were of optional nature and primarily applied from the framework of retrieval practice; a strategy shown to have positive effects on the learning outcomes (for reviews, see Kornell et al., 2011; Rowland, 2014). As it has been suggested that individuals with better WM functioning are more prone to spontaneously employ efficient strategies in their studies (Brewer and Unsworth, 2012), it appears logical to assume that the high WM individuals in the present study were more likely to utilize the optional quizzes offered at the platform.
We also observed a statistically significant negative relationship between the average time spent on reading the to-be-learned materials and verbal WM. More specifically, those with poorer verbal WM tended to spend more time on reading the material within a learning session as compared to those with better verbal WM which aligns well with previous evidence from behavioral- and eye-tracking studies (Engle et al., 1992; Calvo, 2001; Kemper and Liu, 2007). Lastly, we found a significant relationship between repetitive behavior and verbal WM, such that those with poorer verbal WM performance tended to repeat previously completed quizzes to a greater extent than those with better verbal WM performance. This finding is also in line with previous studies examining the relationship between re-reading and WM performance (Rosen and Engle, 1997; Kemper et al., 2004). Furthermore, reflecting these findings to the cognitive load theory (Sweller, 1988), the repetitive behavior observed among low WM individuals might stem from inhibited learning due to cognitive overload in the limited capacity of WM (Kalyuga, 2011; Leppink et al., 2013), thus manifested in an increased repetition.
When examining whether visuospatial WM (i.e., Block span) was predicted by student online activity, the results showed a statistically non-significant relationship. Furthermore, a closer look on the single coefficients showed that none of the online acitvity features were significantly related to visuospatial WM performance. Thus, the results stands in stark contrast to the other model where the Digit span served as the dependent variable, showing several online activity features tha was related to verbal WM. This raises the intriguing question regarding the mechanisms underlying these differences. There could be many reasons for the discrepancy, but perhaps the most prominent one might be a result of the separate subcomponents of WM these tasks are taxing (Baddeley and Hitch, 1974; Baddeley, 2000). Moreover, our results are generally in line with evidence from cross-sectional- and longitudinal studies, showing that verbal WM tasks tend to discriminate better than visuospatial WM tasks in several reading-related activities such as educational attainment (Gropper and Tannock, 2009), math performance (Van de Weijer-Bergsma et al., 2015), and even how accurately students' are memorizing to-be-learned materials on e-learning platforms (Pazzaglia et al., 2008). Thus, it is not overly surprising that visuospatial WM, as compared with verbal WM, was less related to online activities in the present study.
Some shortcomings in the present study should be pointed out. Firstly, our sample size was small, encompassing only 98 students. Thus, the results should be interpret cautiously, and rather serve as a preliminary framework for how to examine the relationship between e-learning behavior and cognition. Second, the purpose of the study was explanatory rather than predictive (for a discussion, see Yarkoni and Westfall, 2017), and thus we refrained from predicting WM performance by training a model using a part of the dataset and testing the model on untrained labels (or alternatively applying k-fold cross-validation). Such an approach would indeed have reduced the risk of overfitting, and increased the generalizability of our results (James et al., 2013). Third, we are aware of the fact that the study lacks information of relevant demographical data (e.g., age, gender, and education) of the participants. This is an evident shortcoming, indicating that we cannot generalize our results to the general population.
Conclusions and Future Work
The present study set out to test the relationship between e-learning online activities and individual differences in visuospatial and verbal WM performance. The results showed that online activities predicted verbal WM performance but not visuospatial WM performance. Predictors significantly related to verbal WM performance in the present study were accuracy in recalled quizzes (multiple-choice), the amount of reading sessions, completed quizzes, average reading time per learning session, and repetition of previously learned materials. The obtained results contributes to the existing research of e-learning and WM, and further suggest that individual differences in the verbal WM subcomponent is related to how students interact on e-learning platforms. Moreover, our results insinuates that online activity alone can predict WM performance to a substantial degree (R2Adjusted = 0.166). However, future prediction research with larger sample sizes, more heterogeneous samples and with a greater number of relevant features is needed for untangling the complex interplay between WM and online activities. If successful, this would allow for a fast screening of the students' cognitive profiles captured by individual differences in online activities, and a circumvention of the demanding and time-consuming WM assessments. Another recommendation for future research is to take into account individual differences in WM performance together with a broad array of other relevant background factors (e.g, personality, learning style, motivation) for creating a e-learning system that personalizes learning according to students' prerequisites.
Data Availability Statement
Datasets and scripts for feature extraction- and analyses can be received upon request.
Ethics Statement
The studies involving human participants were reviewed and approved by the regional vetting committee (2017/517-31). The patients/participants provided their written informed consent to participate in this study.
Author Contributions
DF developed the study concept and all authors contributed to the study design. Feature extraction- and data preprocessing was conducted by DF who also performed the data analysis and interpretation together with BJ. DF drafted the manuscript. All co-authors provided critical revisions and approved the final version of the manuscript for submission.
Funding
This work was funded by the Swedish Research Council (2014-2099).
Conflict of Interest
The authors declare that the research was conducted in the absence of any commercial or financial relationships that could be construed as a potential conflict of interest.
Acknowledgments
We wish to thank Hypocampus AB for providing the researchers with data from the e-learning platform.
Supplementary Material
The Supplementary Material for this article can be found online at: https://www.frontiersin.org/articles/10.3389/feduc.2020.00022/full#supplementary-material
Footnotes
1. ^However, in a subsequent study where we gathered demographical data, descriptive analyses showed that the participants mean age was 30.70 (SD = 8.48), out of which 52% were females.
References
Agarwal, P. K., Finley, J. R., Rose, N. S., and Roediger, H. L. (2017). Benefits from retrieval practice are greater for students with lower working memory capacity. Memory 25, 764–771. doi: 10.1080/09658211.2016.1220579
Anmarkrud, Ø., Andresen, A., and Bråten, I. (2019). Cognitive load and working memory in multimedia learning: conceptual and measurement issues. Educ. Psychol. 54, 61–83. doi: 10.1080/00461520.2018.1554484
Aronen, E. T., Vuontela, V., Steenari, M. R., Salmi, J., and Carlson, S. (2005). Working memory, psychiatric symptoms, and academic performance at school. Neurobiol. Learn. Mem. 83, 33–42. doi: 10.1016/j.nlm.2004.06.010
Baddeley, A. (2000). The episodic buffer: a new component of working memory? Trends Cogn. Sci. 4, 417–423. doi: 10.1016/S1364-6613(00)01538-2
Baddeley, A. (2003). Working memory and language: an overview. J. Commun. Disord. 36, 189–208. doi: 10.1016/S0021-9924(03)00019-4
Baddeley, A., Gathercole, S., and Papagno, C. (1998). The phonological loop as a language learning device. Psychol. Rev. 105, 158–173. doi: 10.1037/0033-295X.105.1.158
Baddeley, A., and Hitch, G. (1974). Working memory. Psychol. Learn. Motiv. 8, 47–89. doi: 10.1016/S0079-7421(08)60452-1
Badian, N. A. (2005). Does a visual-orthographic deficit contribute to reading disability? Ann. Dyslexia 55, 28–52. doi: 10.1007/s11881-005-0003-x
Banas, S., and Sanchez, C. A. (2012). Working memory capacity and learning underlying conceptual relationships across multiple documents. Appl. Cogn. Psychol. 26, 594–600. doi: 10.1002/acp.2834
Bertilsson, F., Wiklund-Hörnqvist, C., Stenlund, T., and Jonsson, B. (2017). The testing effect and its relation to working memory capacity and personality characteristics. J. Cogn. Educ. Psychol. 16, 241–259. doi: 10.1891/1945-8959.16.3.241
Brewer, G. A., and Unsworth, N. (2012). Individual differences in the effects of retrieval from long-term memory. J. Mem. Lang. 66, 407–415. doi: 10.1016/j.jml.2011.12.009
Brinton, C. G., and Chiang, M. (2015). “MOOC performance prediction via clickstream data and social learning networks,” in IEEE Conference on Computer Communications (INFOCOM), (Kowloon), 2299–2307. doi: 10.1109/INFOCOM.2015.7218617
Calvo, M. G. (2001). Working memory and inferences: evidence from eye fixations during reading. Memory 9, 4–6. doi: 10.1080/09658210143000083
Chang, T. W., Kurcz, J., El-Bishouty, M. M., and Kinshuk Graf, S. (2015). “Adaptive and personalized learning based on students' cognitive characteristics,” in Ubiquitous Learning Environments and Technologies. Lecture Notes in Educational Technology, ed H. R. Kinshuk (Berlin: Springer). doi: 10.1007/978-3-662-44659-1_5
Coldwell, J., Craig, A., Paterson, T., and Mustard, J. (2008). Online students: relationships between participation, demographics and academic performance. Electron. J. E-Learn. 6, 19–30.
Conway, A. R. A., Kane, M. J., Bunting, M. F., Hambrick, D. Z., Wilhelm, O., and Engle, R. W. (2005). Working memory span tasks: a methodological review and user's guide. Psychon. Bull. Rev. 12, 769–786. doi: 10.3758/BF03196772
Costa, E. B., Fonseca, B., Santana, M. A., de Araújo, F. F., and Rego, J. (2017). Evaluating the effectiveness of educational data mining techniques for early prediction of students' academic failure in introductory programming courses. Comput. Human Behav. 73, 247–256. doi: 10.1016/j.chb.2017.01.047
Cowan, N. (2001). The magical number 4 in short-term memory: a reconsideration of mental storage capacity. Behav. Brain Sci. 24, 87–114. doi: 10.1017/S0140525X01003922
Cowan, N., Elliott, E. M., Saults, J. S., Morey, C. C., Mattox, S., Hismjatullina, A., et al. (2005). On the capacity of attention: its estimation and its role in working memory and cognitive aptitudes. Cogn. Psychol. 51, 42–100. doi: 10.1016/j.cogpsych.2004.12.001
D'Amico, A., and Guarnera, M. (2005). Exploring working memory in children with low arithmetical achievement. Learn. Individ. Differ. 15, 189–202. doi: 10.1016/j.lindif.2005.01.002
Daneman, M., and Carpenter, P. A. (1980). Individual differences in working memory and reading. J. Verbal Learning Verbal Behav. 19, 450–466. doi: 10.1016/S0022-5371(80)90312-6
Daneman, M., and Merikle, P. M. (1996). Working memory and language comprehension: a meta-analysis. Psychon. Bull. Rev. 3, 422–433. doi: 10.3758/BF03214546
Dawson, S., McWilliam, E., and Tan, J. (2008). “Teaching smarter: how mining ICT data can inform and improve learning and teaching practice,” in Annual Conference of the Australasian Society for Computers in Learning in Tertiary Education, (Melbourne, VIC), 221–230.
Dunlosky, J., Rawson, K. A., Marsh, E. J., Nathan, M. J., and Willingham, D. T. (2013). Improving students' learning with effective learning techniques: promising directions from cognitive and educational psychology. Psychol. Sci. Public Interest 14, 4–58. doi: 10.1177/1529100612453266
Ehri, L. C. (2005). Learning to read words: theory, findings, and issues. Sci. Stud. Reading 9, 167–188. doi: 10.1207/s1532799xssr0902_4
Engle, R. W., Cantor, J., and Carullo, J. J. (1992). Individual differences in working memory and comprehension: a test of four hypotheses. J. Exp. Psychol. Learn. Memory Cogn. 18, 972–992. doi: 10.1037/0278-7393.18.5.972
Ericsson, K. A., and Kintsch, W. (1995). Long-term working memory. Psychol. Rev. 102, 211–245. doi: 10.1037/0033-295X.102.2.211
Gathercole, S. E., and Alloway, T. P. (2008). Working Memory and Learning: a Practical Guide for Teachers. London, England: Sage Press.
Gathercole, S. E., and Pickering, S. (2001). Working memory deficits in children with special educational needs. Br. J. Spec. Educ. 28, 89–97. doi: 10.1111/1467-8527.00225
Gropper, R. J., and Tannock, R. (2009). A pilot study of working memory and academic achievement in college students with ADHD. J. Atten. Disord. 12, 574–581. doi: 10.1177/1087054708320390
Hu, Y. H., Lo, C. L., and Shih, S. P. (2014). Developing early warning systems to predict students' online learning performance. Comput. Human Behav. 36, 469–478. doi: 10.1016/j.chb.2014.04.002
Huai, H. (2000). Cognitive Style and Memory Capacity: Effects of Concept Mapping as a Learning Method. Netherlands: Twente University.
James, G., Witten, D., Hastie, T., and Tibshirani, R. (2013). An Introduction to Statistical Learning. New York, NY: Springer. doi: 10.1007/978-1-4614-7138-7
Jovanovic, M., Vukicevic, M., Milovanovic, M., and Minovic, M. (2012). Using data mining on student behavior and cognitive style data for improving e-learning systems: a case study. Int. J. Comput. Intell. Systems 5, 597–610. doi: 10.1080/18756891.2012.696923
Kalyuga, S. (2011). Cognitive load theory: how many types of load does it really need? Educ. Psychol. Rev. 23, 1–19. doi: 10.1007/s10648-010-9150-7
Kalyuga, S., and Sweller, J. (2005). Rapid dynamic assessment of expertise to improve the efficiency of adaptive e-learning. Educ. Technol. Res. Dev. 53, 83–93. doi: 10.1007/BF02504800
Kaplan, A. M., and Haenlein, M. (2016). Higher education and the digital revolution: about MOOCs, SPOCs, social media, and the cookie monster. Bus. Horiz. 59, 441–450. doi: 10.1016/j.bushor.2016.03.008
Kemper, S., Crow, A., and Kemtes, K. (2004). Eye-fixation patterns of high-and low-span young and older adults: down the garden path and back again. Psychol. Aging 19, 157–170. doi: 10.1037/0882-7974.19.1.157
Kemper, S., and Liu, C.-J. (2007). Eye movements of young and older adults during reading. Psychol. Aging 22, 84–93. doi: 10.1037/0882-7974.22.1.84
Kloft, M., Stiehler, F., Zheng, Z., and Pinkwart, N. (2015). “Predicting MOOC dropout over weeks using machine learning methods,” in Proceedings of the EMNLP 2014 Workshop on Analysis of Large Scale Social Interaction in MOOCs, 60–65. doi: 10.3115/v1/W14-4111
Kornell, N., Bjork, R. A., and Garcia, M. A. (2011). Why tests appear to prevent forgetting: a distribution-based bifurcation model. J. Mem. Lang. 65, 85–97. doi: 10.1016/j.jml.2011.04.002
Leppink, J., Paas, F., Van der Vleuten, C. P. M., Van Gog, T., and Van Merriënboer, J. J. G. (2013). Development of an instrument for measuring different types of cognitive load. Behav. Res. Methods 45, 1058–1072. doi: 10.3758/s13428-013-0334-1
Lykourentzou, I., Giannoukos, I., Mpardis, G., Nikolopoulos, V., and Loumos, V. (2009). Early and dynamic student achievement prediction in E-learning courses using neural networks. J. Am. Soci. Inf. Sci. Technol. 60, 372–380. doi: 10.1002/asi.20970
Macfadyen, L. P., and Dawson, S. (2010). Mining LMS data to develop an “early warning system” for educators: a proof of concept. Comput. Educ. 54, 74–79. doi: 10.1016/j.compedu.2009.09.008
Manochehr, N.-N. (2006). The influence of learning styles on learners in e-learning environments: an empirical study. Comput. High. Educ. Econ. Rev. 18, 10–14.
McNamara, D. S., and Scott, J. L. (2001). Working memory capacity and strategy use. Mem. Cognit. 29, 10–17. doi: 10.3758/BF03195736
Oberauer, K., Süß, H.-M., Wilhelm, O., and Wittman, W. W. (2003). The multiple faces of working memory: storage, processing, supervision, and coordination. Intelligence 31, 167–193. doi: 10.1016/S0160-2896(02)00115-0
Pazzaglia, F., Toso, C., and Cacciamani, S. (2008). The specific involvement of verbal and visuospatial working memory in hypermedia learning. Br. J. Educ. Technol. 1, 110–124. doi: 10.1111/j.1467-8535.2007.00741.x
Pham, A. V., and Hasson, R. M. (2014). Verbal and visuospatial working memory as predictors of children's reading ability. Arch. Clin. Neuropsychol. 29, 467–477. doi: 10.1093/arclin/acu024
R Core Team (2016). R: A Language and Environment for Statistical Computing. Vienna: R Foundation for Statistical Computing Vienna Austria.
Raghubar, K. P., Barnes, M. A., and Hecht, S. A. (2010). Working memory and mathematics: a review of developmental, individual difference, and cognitive approaches. Learn. Ind. Diff. 20, 110–122. doi: 10.1016/j.lindif.2009.10.005
Rogers, R. D., and Monsell, S. (1995). Costs of a predictible switch between simple cognitive tasks. J. Exp. Psychol. Gen. 124, 207–231. doi: 10.1037/0096-3445.124.2.207
Röhlcke, S., Bäcklund, C., Sörman, D. E., and Jonsson, B. (2018). Time on task matters most in video game expertise. PLoS ONE 13:e0206555. doi: 10.1371/journal.pone.0206555
Rosen, V. M., and Engle, R. W. (1997). The role of working memory capacity in retrieval. J. Exp. Psychol. Gen. 126, 211–227. doi: 10.1037/0096-3445.126.3.211
Rouet, J. F., Vörös, Z., and Pléh, C. (2012). Incidental learning of links during navigation: the role of visuo-spatial capacity. Behav. Inf. Technol. 31, 71–81. doi: 10.1080/0144929X.2011.604103
Rowland, C. A. (2014). The effect of testing versus restudy on retention: a meta-analytic review of the testing effect. Psychol. Bull. 140, 1432–1463. doi: 10.1037/a0037559
Sayed, M., and Baker, F. (2015). E-learning optimization using supervised artificial neural-network. J. Softw. Eng. Appl. 8, 26–34. doi: 10.4236/jsea.2015.81004
Shelton, B. E., Hung, J. L., and Lowenthal, P. R. (2017). Predicting student success by modeling student interaction in asynchronous online courses. Distance Educ. 38, 59–69. doi: 10.1080/01587919.2017.1299562
Skuballa, I. T., Schwonke, R., and Renkl, A. (2012). Learning from narrated animations with different support procedures: working memory capacity matters. Appl. Cogn. Psychol. 26, 840–847. doi: 10.1002/acp.2884
Stevens, J. (2002). Applied Multivariate Statistics for the Social Sciences (4th ed.). Mahwah, NJ: Lawrence Erlbaum Associates.
Swanson, H. L., and Beebe-Frankenberger, M. (2004). The relationship between working memory and mathematical problem solving in children at risk and not at risk for serious math difficulties. J. Educ. Psychol. 96, 471–491. doi: 10.1037/0022-0663.96.3.471
Sweller, J. (1988). Cognitive load during problem solving: effects on learning. Cogn. Sci. 12, 257–285. doi: 10.1207/s15516709cog1202_4
Sweller, J. (2010). Element interactivity and intrinsic, extraneous, and germane cognitive load. Educ. Psychol. Rev. 22, 123–138. doi: 10.1007/s10648-010-9128-5
Tabachnick, B. G., and Fidell, L. S. (2007). Using Multivariate Statistics (5th ed.). Boston: Pearson/Allyn & Bacon.
Thalmann, S. (2014). Adaptation criteria for the personalised delivery of learning materials: a multi-stage empirical investigation. Australas. J. Educ. Technol. 30, 45–60. doi: 10.14742/ajet.235
Tortorella, R. A. W., Hobbs, D., Kurcz, J., Bernard, J., Baldiris, S., Chang, T.-W., et al. (2015). “Improving learning based on the identification of working memory capacity, adaptive context systems, collaborative and learning analytics,” in Proceedings of Science and Technology Innovations, 39–55.
Truong, H. M. (2016). Integrating learning styles and adaptive e-learning system: current developments, problems and opportunities. Comput. Human Behav. 55, 1185–1193. doi: 10.1016/j.chb.2015.02.014
Tsianos, N., Panagiotis, G., Lekkas, Z., Mourlas, C., and Samaras, G. (2010). “Working memory span and e-learning: the effect of personalization techniques on learners' performance,” in International Conference on User Modeling, Adaptation, and Personalization (Berlin: Springer), 66–74.
Turley-Ames, K. J., and Whitfield, M. M. (2003). Strategy training and working memory task performance. J. Mem. Lang. 49, 446–468. doi: 10.1016/S0749-596X(03)00095-0
Turner, M. L., and Engle, R. W. (1989). Is working memory capacity task dependent? J. Mem. Lang. 28, 127–154. doi: 10.1016/0749-596X(89)90040-5
van Buuren, S., and Groothuis-Oudshoorn, K. (2011). Mice: multivariate imputation by chained equations in R. J. Stat. Softw. 45, 1–67. doi: 10.18637/jss.v045.i03
Van de Weijer-Bergsma, E., Kroesbergen, E. H., and Van Luit, J. E. H. (2015). Verbal and visual-spatial working memory and mathematical ability in different domains throughout primary school. Memory Cogn. 43, 367–378. doi: 10.3758/s13421-014-0480-4
Van Merriënboer, J. J. G., and Sweller, J. (2005). Cognitive load theory and complex learning: recent developments and future directions. Educ. Psychol. Rev. 17, 147–177. doi: 10.1007/s10648-005-3951-0
Vandierendonck, A., Kemps, E., Fastame, M. C., and Szmalec, A. (2004). Working memory components of the corsi blocks task. Br. J. Psychol. 95, 57–79. doi: 10.1348/000712604322779460
Wechsler, D. (1997). Wechsler Memory Scale (Third Ed.). San Antonio, TX: The Psychological Corporation.
Wiklund-Hörnqvist, C., Jonsson, B., and Nyberg, L. (2014). Strengthening concept learning by repeated testing. Scand. J. Psychol. 55, 10–16. doi: 10.1111/sjop.12093
Yarkoni, T., and Westfall, J. (2017). Choosing prediction over explanation in psychology: lessons from machine learning. Perspect. Psychol. Sci. 12, 1100–1122. doi: 10.1177/1745691617693393
Yu, T., and Jo, I.-H. (2014). “Educational technology approach toward learning analytics: relationship between student online behavior and learning performance in higher education,” in Proceedings of the 4th International Conference on Learning Analytics And Knowledge - LAK '14, (Indianapolis, IN), 269–270. doi: 10.1145/2567574.2567594
Keywords: working memory, e-learning, online activities, cognition, retrieval practice
Citation: Fellman D, Lincke A, Berge E and Jonsson B (2020) Predicting Visuospatial and Verbal Working Memory by Individual Differences in E-Learning Activities. Front. Educ. 5:22. doi: 10.3389/feduc.2020.00022
Received: 27 August 2019; Accepted: 27 February 2020;
Published: 17 March 2020.
Edited by:
Tom Crick, Swansea University, United KingdomReviewed by:
Henrik Danielsson, Linköping University, SwedenJosé Manuel Reales, National University of Distance Education (UNED), Spain
Copyright © 2020 Fellman, Lincke, Berge and Jonsson. This is an open-access article distributed under the terms of the Creative Commons Attribution License (CC BY). The use, distribution or reproduction in other forums is permitted, provided the original author(s) and the copyright owner(s) are credited and that the original publication in this journal is cited, in accordance with accepted academic practice. No use, distribution or reproduction is permitted which does not comply with these terms.
*Correspondence: Daniel Fellman, daniel.fellman@umu.se