- Ontario Institute for Studies in Education, University of Toronto, Toronto, ON, Canada
In the early-elementary years, emerging readers experience many emotions due to the high value that is placed on the development of reading skills. If emotions are not regulated using effective learning strategies, they can negatively shape students’ experiences in and perceptions of reading, which can be detrimental to their developing reading skills. This study used automated facial expression monitoring software (FACET) to examine whether emotions affect the types of self-regulated learning (SRL) strategies students use during a narrative storytelling task and whether SRL strategies affect narrative storytelling scores. The participants were 150 emerging readers (Mage = 8, SD = 10.63, 56% girls). Results demonstrated that two emotions were related to SRL strategies; frustration was negatively related to planning and joy was positively related to monitoring. Two SRL strategies were related to narrative storytelling; planning and control were positively related to narrative storytelling scores. There was a significant indirect effect of frustration on storytelling scores through planning, indicating that planning fully mediated the effect of frustration on narrative storytelling scores. This finding suggests that although frustration has a significant negative effect on story comprehension, this relationship operates through decreased use of planning strategies. Future research should investigate pedagogical strategies to manage emotions and increase SRL strategy use during reading and literacy activities.
Introduction
Emotions both positive and negative, have a substantial effect on the cognitive processes that aid and facilitate learning in the classroom (Immordino-Yang and Damasio, 2007). Emotions are woven into many functional aspects of the classroom, as they include feelings about certain subjects, thoughts about ability, motivation to engage with learning materials and the physiological reactions involved in nervousness and anxiety (Scherer, 2009; Scherer and Moors, 2019). The effect of emotions is highly evident in subjects where achievement is strongly valued, such as reading. During the early elementary years, parents and teachers prioritize helping students attain proficiency in reading, as literacy skills are required for learning in the middle and upper elementary years, and proficiency in reading predicts later academic achievement (Cain and Oakhill, 2008). As such, students who are learning to read (i.e., emerging readers) are highly susceptible to experiencing strong emotions while reading. The effect of consistently experiencing pervasive emotions, especially when they are negative, may be detrimental to the development of literacy skills and attitudes toward reading (Grills-Taquechel et al., 2012). Since the early elementary years are a time when academic self-concepts begin to take shape (Dweck, 1999), it is crucial that educators understand the effects of emotions on emerging readers. While previous research has examined how emotions affect learning outcomes, there has been less research on how emotions affect the Self-Regulated Learning (SRL) strategies that emerging readers use.
Self-regulated learning strategies are metacognitive strategies that are used during learning tasks to monitor learning and progress toward a goal (Winne and Hadwin, 1998; Pintrich, 2000). Learners with strong SRL skills effectively manage their own learning through monitoring, planning, and cognitive strategy use (Winne, 2017). SRL strategies are highly functional, as they help students manage emotions and maintain focus in addition to monitoring learning and progress toward goals (Calvo and D’Mello, 2011; D’Mello and Graesser, 2012). Emotions have a significant effect on the SRL strategies that students can access and utilize during learning, as positive emotions are related to the use of advanced SRL strategies such as planning, monitoring, elaboration, and critical thinking (Pekrun, 2017). Negative emotions, on the other hand, are related to the use of shallow SRL strategies such as rote memorization and recall (Pekrun, 2017). Despite the important role emotions play in SRL, very little research examining the relationship between emotions and SRL strategies has been conducted to date (Taub et al., 2019). Additionally, few studies have examined the contribution of individual emotions, such as joy, fear, confusion and frustration, to the use of SRL strategies, as emotions are commonly grouped by valence as either positive or negative (D’Mello and Graesser, 2012; Harley et al., 2015; Jarrell et al., 2017; Pekrun, 2017).
The current study examined the relationship between emotions, SRL strategies, and scores on a narrative storytelling task. Narrative storytelling is a measure of story comprehension and oral language fluency that is commonly used with emerging readers. During a narrative storytelling task the student constructs a narrative from a wordless picture book instead of reading written words. This task allows researchers to analyze story comprehension in young students while their ability to decode written text is emerging, as success on the task does not depend on the student’s reading ability. Narrative storytelling is a stable predictor of many language and reading skills that develop in later years, such as vocabulary, fluency, and reading comprehension (Storch and Whitehurst, 2002; Reese et al., 2010; Babayiğit et al., 2020). Previous research has demonstrated that negative emotions are related to decreases in scores on reading comprehension (Grills-Taquechel et al., 2014) and narrative storytelling (Buono and Woodruff, unpublished). It is not well understood, however, how emotions affect the SRL strategies students use to manage goals and monitor comprehension, and how this relationship affects story comprehension. In this study, students were asked to construct a spontaneous narrative from a wordless picture book and comprehension scores were derived from students’ interpretation of story events and quality of language. The objective of this study was to examine the role of SRL strategies in the relationship between emotions and narrative storytelling scores in emerging readers.
Emotions During Learning
Emotions are an important aspect of the learning process, as they shape students attitudes toward certain subjects and their motivation to learn (Pekrun, 2006). Emotions that emerge in the classroom are a function of appraisals of both the student’s control over the outcome of the task, and value placed on the task (Pekrun, 2006; Pekrun and Perry, 2014). Ongoing appraisals of control and value, which include memories of past successes and failures, and anticipated future outcomes, act as both determinants of emotions, and the objects of emotions (Pekrun et al., 2017). For example, if a student experiences nervousness and fear while reading out-loud in class and makes a mistake in front the class, both the experience of the emotion, fear, and the outcome of making a mistake will influence subsequent appraisals, emotions, and outcomes. Because emotions are deeply woven throughout the learning process (Immordino-Yang and Damasio, 2007), students bring memories of both the emotions and the outcomes into subsequent learning (Pekrun et al., 2017).
In general, positive emotions such as hope, pride, and enjoyment are favorable in the classroom, as they are related to flexible thinking, intrinsic motivation (Pekrun et al., 2002, 2011; D’Mello et al., 2017), and increases in learning outcomes (Knörzer et al., 2016; Jarrell et al., 2017). Negative emotions, such as anxiety, shame, and anger are related to task-irrelevant thinking, extrinsic motivation, preoccupation with failure, and comparison between peers (Meinhardt and Pekrun, 2003; Gable and Harmon-Jones, 2010). The detrimental effect of pervasive negative emotions on students’ self-perceptions, commitment to academics, and academic achievement is long-lasting (Pekrun et al., 2017). When they are not regulated, negative emotions lead to boredom and disengagement (Pekrun et al., 2010; D’Mello and Graesser, 2012) and decreases in learning outcomes (Grills-Taquechel et al., 2014; Sabourin and Lester, 2014; Knörzer et al., 2016). Students who experience highly activating negative emotions, such as frustration, achieve fewer learning gains than students who experience positive emotions, and students who experience boredom and disengagement (Jarrell et al., 2017). Further, students who experience negative emotions while engaged in an academic task are less likely to move out of that negative state than students who experience positive or neutral emotions (Sinclair et al., 2018; Wortha et al., 2019).
Although many studies have found that negative emotions are unfavorable during learning, in samples of older adolescents and adults, research has also found that some negative emotions such as frustration and confusion, can be productive learning emotions (D’Mello, 2013; D’Mello et al., 2014). The model of affective dynamics highlights the important role of cognitive disequilibrium, which is a state of uncertainty that occurs when a student is confronted with an obstacle that impedes a learning goal, and the resulting emotions are confusion or frustration (D’Mello and Graesser, 2012). Such obstacles can occur when information presented within the task contradicts the learner’s understanding, or when there is an impasse between the learner’s knowledge and the information presented within the task. When students engage in extensive questioning and updating comprehension in an attempt to persist through this impasse and resolve confusion, the learning they acquire is deep and long lasting, (D’Mello and Graesser, 2012). Research in the field has supported this assumption, as studies have found that frustration (Taub et al., 2019) and confusion (D’Mello et al., 2014) can lead to stronger understanding of material and higher learning gains, respectively. These varied findings on the relation between some negative emotions and learning suggest that additional research is required to understand the nuances of how individual emotions affect learning outcomes in different subject areas. Additionally, findings on the positive effects of confusion and frustration have not yet been replicated with early elementary students, which suggests that additional research is required with this population of students.
Self-Regulated Learning (SRL)
Self-regulated learning (SRL) is a multidimensional process that includes affective, cognitive, and metacognitive processes (Pintrich, 2000; Winne, 2017), which are systematically oriented toward personal goal achievement (Zimmerman and Schunk, 2011). SRL is a self-directed process through which learners use comprehension monitoring and affect regulation in academic environments such as schools and classrooms to successfully shape academic outcomes. Utilizing SRL strategies requires flexible thinking, monitoring, and updating of both affective states and comprehension of material (Pekrun et al., 2011; D’Mello and Graesser, 2012). In general, SRL strategies help facilitate meaningful learning gains and lead to improvements in academic achievement (Zheng, 2016).
Self-regulated learning is a dynamic, constructive process whereby learners set goals, and then regulate, monitor, and control their thoughts, behavior, and motivation through the process of; (1) planning, (2) monitoring, (3) cognitive control, and (4) reaction and reflection (Pintrich, 2000). During these four phases, learners engage in planning by identifying a target goal that helps guide cognition during a task. Monitoring involves the awareness and updating of different aspects of cognition during the task (e.g., monitoring comprehension, progress toward goals). Cognitive control includes processes that are used to adapt and modify thoughts, such as memory retrieval strategies, reasoning, and problem solving. Reaction and reflection involves learners’ evaluations of their performance on a task (Pintrich, 2000). SRL strategies are used in an ongoing and iterative process that accounts for multiple phases of learning, such as; (1) task definition, (2) goal setting and planning, (3) enactments (i.e., strategy use), and (4) adaptations (e.g., revising previously used strategies) (Winne, 2017). In the first three phases, the learner monitors features such as conditions, operations, products, evaluations, and standards (COPES) in their environment. Students either use cognitive operations, such as study tactics, or change external and internal conditions (e.g., seeking help) to meet their learning goals. In the final stage of the model, learners make changes to the strategies that were previously used to incorporate revised or novel strategies (Winne, 2017). This process allows the learner to determine whether goals have been met or if further adjustments to SRL strategies need to be made to reach the goal.
While there has been extensive research on SRL and learning, very little research has been conducted on the relationship between emotions and SRL strategies, and their joint effect on learning outcomes. Previous research has found that learning gains are related to the use of advanced SRL strategies (Zheng, 2016; Jang et al., 2017), which help learners manage and regulate emotions to maintain focus on the task (Calvo and D’Mello, 2011; D’Mello and Graesser, 2012). Additionally, previous research has demonstrated that students with strong emotion regulation skills both experience less intense negative emotions, and use more metacognitive strategies during learning (Price et al., 2018). Few studies, however, have examined how individual emotions affect the types of SRL strategies students use. Taub et al. (2019) recently found that surprise was related to increases in the accuracy of metacognitive judgments, and frustration was related to increased accuracy during note taking. Additionally, confusion has been related to increases in metacognitive strategies used during learning activities (Taub et al., 2018). While these studies have found that individual emotions affect the types of SRL strategies students use, many studies have not found significant effects of individual emotions on learning outcomes (Price et al., 2018; Taub et al., 2018). These findings suggest that additional research is required to investigate the effects of individual emotions on both SRL strategies and learning outcomes.
The Current Study
The current study examined the relationship between emotions, SRL strategies and narrative storytelling scores in a sample of elementary-aged students. During the early elementary years, reading is a highly prioritized and valued activity. The need for students to become proficient and skilled readers brings intense and highly salient emotions. The early elementary years are also a time in which students become increasingly aware of how their thoughts and emotions interact to influence behavior (Lagattuta et al., 2016), and when their self-concept as a learner forms (Dweck, 1999). As such, it is important to understand how emotions and SRL strategies affect learning outcomes in literacy activities during this time, so educators can help students effectively manage emotions and achieve learning goals.
This study used a narrative storytelling task in which students were asked to formulate a spontaneous narrative using a wordless picture book prompt (Mayer, 1969). Video recordings of the participants facial expressions were collected, and facial expression recognition software coded expressions of emotion (iMotions, 2018). Students’ use of SRL strategies were coded from the videos of the task. As previous research has demonstrated that negative emotions are related to decreases in narrative storytelling scores (Buono and Woodruff, unpublished), the objectives of this study were to expand on previous findings by addressing the following research questions: (1) Are individual emotions related to the use of SRL strategies? (2) Are SRL strategies related to narrative storytelling scores? (3) Do SRL strategies mediate the relationship between emotions and narrative scores? It was hypothesized that the emotions, anger, fear, frustration, and confusion would be related to decreases in the use of SRL strategies, while positive emotions such as joy and surprise would be related to increases in the use of SRL strategies. It was also hypothesized that SRL strategies would be related to increases in narrative storytelling scores. Finally, it was hypothesized that the relationship between emotions and narrative storytelling scores would be mediated by the use of SRL strategies.
Materials and Methods
Participants
The sample consisted of 150 students between the ages of 6 and 9 years old [M = 98.02 months (8 years, 2 months), SD = 10.63], 56% (n = 84) of whom were females. The majority of mothers reported completing a college level professional diploma (37%, n = 55) or a bachelor’s degree (39%, n = 58), and the remainder reported not finishing high school (1.4%, n = 2), completing a high school diploma (9%, n = 14), a master’s degree (9%, n = 14), and a doctorate degree (4%, n = 6).
Measures
Narrative Storytelling Scores were derived using the wordless picture book “Frog where are you?” (Mayer, 1969). Since this book does not contain any words, participants were required to spontaneously formulate a cohesive story about the events depicted in the book. Participants went through a familiarization phase, during which they were allowed to look through all pages of the book to understand the sequence of events. Following the familiarization phase, participants were asked to orally tell a story corresponding to the images on each page of the book.
Narratives were evaluated by two independent raters who were blind to the hypothesis of the study, using a coding scheme adapted from Reilly et al. (2004). This coding scheme produced a storytelling score based on language variables, which included the length of the story, frequency of morphological errors, complex syntax, and story comprehension, which includes the use of evaluative devices, and identification of the theme and individual story components. Discrepancies in codes were resolved through discussion with a third rater. A detailed account of the coding scheme is provided in Supplementary Appendix A.
Emotions were measured using automated facial expression monitoring software, FACET (iMotions, 2018). This software uses the Facial Action Coding System (FACS; Ekman and Friesen, 1978) to automatically code facial expressions of emotions in real time, from the camera of a computer. Musculature movement in the face is measured using movement indicators at 19 Action Units (AU) and scores indicate the degree to which an emotion is present on the face. The software provides scores for 6 academic emotions; anger, fear, frustration and confusion, joy and surprise which were used for the purposes of this analysis. The emotion variables collected using this task are expressed as evidence values, which is a standard integer between −3 and 3 representing the log (base10) likelihood that a human rater would rate the emotion in the same way (iMotions, 2018). For example, a score of 1, 2, and 3 represents the likelihood that 10, 100, or 1,000 human raters would code the emotion in the same way. This software has been validated over many trials, with children and adults (Bartlett et al., 2009).
Self- Regulated Learning (SRL) strategies were coded based on a coding scheme adapted from Pintrich (2000) and Winne (2017). Two independent raters who were blind to the hypothesis of the study watched videos of participants completing the narrative task and coded the frequency of occurrence for each of the following SRL strategies: planning, monitoring, control, and reflection. Any discrepancies in codes between raters were resolved by a third rater. The coding scheme included individual scores for planning, monitoring, control, and reaction and reflection. Planning behaviors involved goal setting, planning time, sequencing events, forethought and activation. Monitoring behaviors included references to monitoring progress and comprehension, check-ins, and seeking clarification. Control included strategies used to adapt and modify thoughts or statements of personal agency, such as memory cues, retrieval strategies, and statements related to agency over the outcome. Reaction and reflection included evaluations of performance or statements of personal affect. A detailed account of the coding scheme is provided in Supplementary Appendix B.
Reading Experience was measured to gauge the quantity and quality of students’ experience with books at home. Parents reported the amount of time their child spends reading at home, how much their child enjoys reading, the child’s perceived reading ability, and the child’s motivation to read. Responses to each item were recorded on a scale from 1 to 5. A reading experience composite score was calculated to account for the combined ratings on these items.
Vocabulary was measured to account for individual differences in receptive vocabulary that could contribute to scores in oral language fluency using the NIH Toolbox Picture Vocabulary Test (TPVT; Gershon et al., 2013). Students were asked to identify one picture from an array of four pictures, which corresponds to a target word presented orally by the researcher.
Procedure
Participants completed all study related activities during school hours, while sitting in front of a computer. Students’ facial expressions were recorded using a webcam, which was used as input for the FACET facial expression analysis software. Prior to completing the narrative task participants completed a warm-up activity in which they were asked to tell a story about a single picture. The purpose of this activity was to familiarize the participant with the procedure of telling a story corresponding to a picture (Reilly et al., 2004). Following the warm-up activity, the researcher began the familiarization phase of the task by introducing the participant to the book and inviting the participant to look through the pages to become familiar with the events in the book before beginning the task. The researcher used the following instruction prompt; “I am going to show you a book about a boy, a dog and a frog. First, I want you to look through the pages. When you are done looking through the pages, I want you to tell me a story to go along with the pictures. You can look through the pages as many times as you want before you tell your story.” If the participant began telling a story without looking at the pictures, the researcher repeated the instruction prompt.
The FACET software extracted the linear orientation of facial muscles at each AU and derived a score for each facial expression using a support vector machine (SVM; iMotions, 2018). Because this software measures musculature movement and this task required the participants to speak, each participant’s video file was cut to exclude footage of the participant speaking. The video frames that were used in the analysis were taken from the time in which the participant was looking at a new page, until they began speaking. This video segmentation was done to eliminate the time when the software would have classified movement in the facial muscles that is required for speech but is not an expression of emotion. The mean of emotion score from each page was collapsed across the narrative task and used in the analysis.
Results
Emotions and SRL Strategies
The mean evidence values for all individual emotions demonstrated that fear was significantly higher than confusion (t = 3.378, SD = 0.503 p = 0.001) and frustration (t = 2.565, SD = 0.422 p = 0.011), but not anger (t = 1.63, SD = 0.428 p = 0.105). The mean evidence value for anger was significantly higher than confusion (t = 2.69, SD = 0.372 p = 0.008) but not frustration (t = 1.43, SD = 0.27 p = 0.154). Joy was significantly higher than confusion (t = 2.47, SD = 0.409 p = 0.011), frustration (t = 2.69, SD = 0.372 p = 0.008) and anger (t = 1.38, SD = 0.368 p = 0.040). Surprise was significantly higher than confusion (t = 1.87, SD = 0.301 p = 0.044) and frustration (t = 1.67, SD = 0.389 p = 0.045). The evidence values for emotions expressed during the task are presented in Figure 1.
The standardized means of SRL strategies used were analyzed individually and illustrated in Figure 2. There were no significant differences in the use of planning, monitoring, control and reflection strategies used (Figure 2). Planning and control were positively correlated (0.22, p = 0.007) such that increases in planning were related to increases in control (Table 1).
Emotions, SRL Strategies and Narratives
The emotions; anger, fear, confusion, and frustration, joy and surprise, and the SRL strategies; planning, monitoring, control, and reflection were included in an initial correlation analysis. The first hypothesis was partially confirmed, as frustration was negatively related to planning and joy was positively related to monitoring. The emotions anger, fear, confusion and surprise were not related to any SRL strategies. The second hypothesis was partially confirmed, as planning and control were positively related to storytelling scores. The SRL strategies reaction and reflection and monitoring were not related to storytelling scores. Correlations between negative emotions, SRL strategies and storytelling scores are presented in Table 1.
Mediated Path Model
A path model with tests of indirect effects was constructed using MPlus version 8 (Muthén and Muthén, 2017) to address the third research question of whether SRL strategies mediated the effect of emotions on storytelling scores. The covariates included in the model were gender, age, vocabulary, maternal education and reading experience. The emotions frustration and joy, and the SRL strategies planning, monitoring and control were included as independent variables. Two indirect effects were tested; the indirect effect of frustration on storytelling scores with planning as a mediator, and the indirect effect of joy on storytelling scores with monitoring as a mediator. Ten thousand bootstrapping samples were used to calculate 95% confidence intervals for the estimates (Preacher and Hayes, 2008). Correlations between all variables and covariates in the model are included in Table 2.
First a model without indirect effects was constructed. The results demonstrated that age and gender significantly predicted storytelling scores, such that older students and girls had higher scores. Vocabulary, maternal education and experience with books were not significantly related to storytelling scores. The SRL strategies planning and control were positively related to storytelling scores, while monitoring was not related to storytelling scores. Joy was positively related to monitoring but not related to storytelling scores. Frustration was negatively related to planning strategies and storytelling scores (path-c). All path coefficients, standard errors, p-values, CIs, and R-squared values are presented in Table 3.
Next, a model with two indirect effects was tested. There was a significant indirect effect of frustration on storytelling scores through planning strategies, which indicates that the effect of frustration on narrative scores was fully mediated through planning. The path-a coefficient between frustration and planning was significant, as was the path-b coefficient between planning and storytelling scores. The effect of frustration on storytelling scores was not significant when planning was included as a mediator in the model. The confidence intervals for the indirect effect did not include zero, which indicates that the relationship between frustration and storytelling scores is fully mediated through the use of planning strategies (Preacher and Hayes, 2008). The indirect effect of joy on storytelling scores through monitoring was not significant (Table 3). Mediation pathways are illustrated in Figure 3.
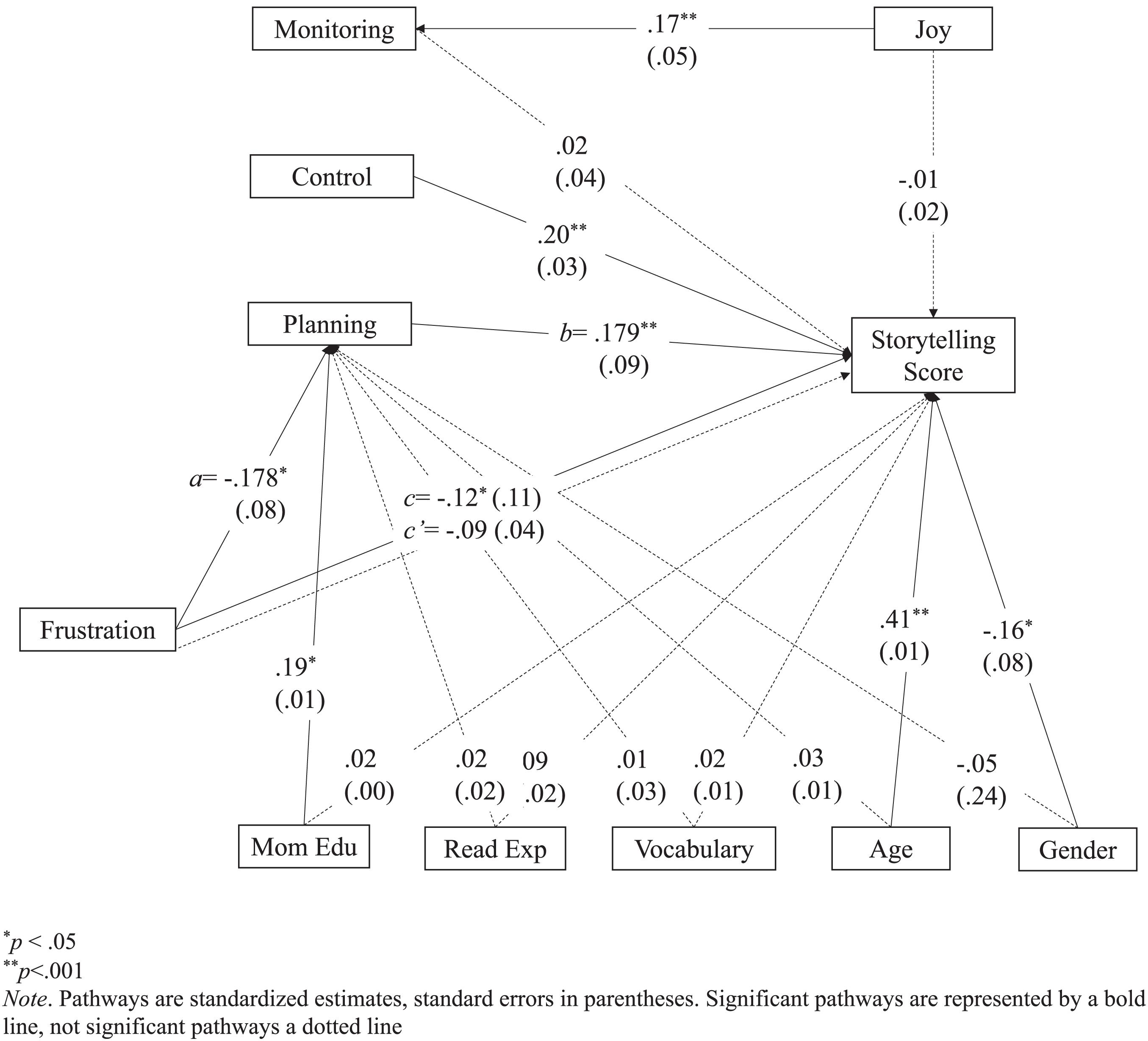
Figure 3. Model with indirect mediated effects. Pathways are standardized estimates, standard errors in parentheses. Significant pathways are represented by a bold line, not significant pathways a dotted line. *p < 0.05, **p < 0.001.
Discussion
In the early elementary years when literacy skills are still emerging, reading elicits strong emotions. While there has been some research on the effect of anxiety on reading (Daley et al., 2014; Grills-Taquechel et al., 2014), the effect of individual emotions, such as anger, fear and frustration has not been thoroughly examined in emerging readers (Graesser and D’Mello, 2012). Additionally, while some research has been conducted on the relationship between emotions and SRL strategies in older students (D’Mello and Graesser, 2012; Price et al., 2018), research on how emotions affect the types of SRL strategies students use, and the subsequent effects on learning outcomes has been limited (Taub et al., 2018; Graesser, 2019; Taub et al., 2019), especially with early elementary students. The objective of this study was to address these gaps in the current literature by examining the relationship between emotions, SRL strategies and scores on a narrative storytelling task in a sample of emerging readers. The results demonstrated that joy was related to increases in monitoring, frustration was related to the use of fewer planning strategies, and both planning and cognitive control were related to increases in storytelling scores. Tests of indirect effects demonstrated that the use of planning strategies fully mediated the relationship between frustration and storytelling scores. These results suggest that SRL strategies are an important mechanism in the relationship between emotions and storytelling scores.
The results of the first research question regarding the relationship between emotions and SRL strategies revealed that joy was related to increases in monitoring strategies, and frustration was related to decreases in the use of planning strategies. The finding that joy is related to increases in monitoring strategies is novel, as no prior studies have demonstrated this effect of an individual emotion on SRL strategies. The effect of joy, however, did not extend to increases in the outcome variable, as joy was not related to increases in storytelling scores. There have been competing findings on the effect of frustration on SRL strategies, as some research has found that frustration is related to boredom and disengagement (D’Mello and Graesser, 2012), while other researchers have found that frustration is related to more favourable SRL strategies (Taub et al., 2019). These results are in line with the former findings, as they suggest that in a sample of emerging readers, frustration negatively affects students’ use of planning strategies.
The hypotheses for the second research question regarding whether SRL strategies are related to storytelling scores were partially confirmed, as the SRL strategies planning and control were related to increases in storytelling scores but monitoring and reflection were not. It is noteworthy that planning and control were also positively related to each other. This result suggests that during a narrative storytelling task, planning and cognitive control could employ similar processes, as planning might scaffold the goals and expectations of the task enough to cue the students to engage in thought modification strategies involved in cognitive control. The general finding that SRL strategies are related to increases in storytelling scores is supported by previous research with older adolescents and undergraduate students, which has demonstrated that using SRL strategies is related to increases in reasoning, and deeper learning (D’Mello and Graesser, 2012; Zheng, 2016; Jang et al., 2017). Although it was expected that monitoring would be related to learning outcomes, it is possible that engaging in planning strategies, such as clarifying the objectives and sub goals of the task, is more important to success on this type of task, since comprehension can be revised and reconstructed based on novel story events. It is also possible that students were engaging in monitoring strategies but did not voice them out loud. Future iterations of this work should include an analysis of whether using SRL prompts during a narrative task demonstrates different effects on story comprehension (Bannert et al., 2015; Hadwin, 2019).
Finally, the results of the third research question revealed that planning fully mediated the effect of frustration on storytelling scores when controlling for age, vocabulary, maternal education, experience with books, and gender. This finding indicates that the negative relationship between frustration and storytelling scores is explained by the use of planning strategies. These results provide support for the model of affective dynamics (D’Mello and Graesser, 2012), as the SRL strategy is the mechanism through which a significant proportion of the relationship between negative emotions and storytelling scores is explained. This finding suggests that when students are engaging in literacy activities, such as spontaneously constructing a narrative or comprehending story events, experiencing frustration is related to decreases in planning strategies, which would help students refocus on the learning goal and succeed on the task. SRL strategies could be a useful tool to help students not only improve learning outcomes, but manage emotions. By focusing attention away from the emotion and onto to the learning goal, SRL strategies can help students who experience anxiety and fear during reading but have difficulty responding to interventions that focus on emotion reframing strategies (Grills-Taquechel et al., 2014). Future research should further examine the directionality of this relationship, and whether SRL strategies can act as a buffer to the relationship between emotions and learning outcomes during literacy tasks.
The results of this study are in line with findings indicating that negative emotions are associated with unfavorable learning outcomes (D’Mello and Graesser, 2012) and the use of less favorable learning strategies (Jang et al., 2017; Price et al., 2018). This finding is also in line with previous research which has found that negative emotions are related to decreases in scores on reading activities (Grills-Taquechel et al., 2012; Daley et al., 2014). This finding could be partially explained by the specific demands of this task, as the instruction prompt is open ended. Frustration due to ambiguity in task demands might have reduced students’ perceptions of control over their success on the task (Pekrun and Perry, 2014). If students became frustrated by the task demands, they may have been less likely to consider the broad goals of the task, and engage in planning strategies to achieve that goal (D’Mello and Graesser, 2012). Additionally, frustration is an emotion that is highly activating (Posner et al., 2005; Pekrun, 2006), and decreases in storytelling scores might have been related to the increases in arousal that often accompany frustration (Daley et al., 2014). It is possible that while previous research has demonstrated that frustration is beneficial to SRL strategy use (Taub et al., 2019), this finding does not generalize to literacy activities, which could require emotions that are less activating to facilitate comprehension (Daley et al., 2014).
Implications
These findings are highly useful to educators and education researchers developing pedagogical classroom strategies for managing emotions. These results support the model of affective dynamics, which asserts that SRL strategies aid in managing and regulating emotions, by focusing on the learning goal, and helping students monitor their progressive learning gains (D’Mello and Graesser, 2012). As the results of this study demonstrated that SRL strategies mediated the effect of frustration on storytelling scores, it is possible that teachers’ efforts in helping students comprehend story events should focus on their use of planning strategies. This means that teachers can focus on explicitly teaching, prompting and providing feedback on using SRL strategies not only as a means of improving learning, but reducing the expression of negative emotions, such as frustration.
These findings significantly contribute to the current literature on emotions, SRL strategies and literacy outcomes, as few studies have examined the relationship between these three variables. While some studies have looked at the relationship between SRL strategies and learning outcomes (D’Mello and Graesser, 2012; Zheng, 2016; Jang et al., 2017), few studies have examined the relationship between emotions and SRL strategies (Price et al., 2018; Taub et al., 2019), and of these studies, fewer have established connections to learning outcomes. This study looked at broad macro-level SRL strategies, such as planning, monitoring and cognitive control (Bannert et al., 2015) instead of investigating the micro-level behaviors (e.g., memory retrieval statements, checking understanding) within these broad strategies (Greene and Azevedo, 2009). While the results at this level of analysis were useful to address these specific research questions, future research should examine micro-level SRL strategies and individual discrete emotions during literacy tasks.
This study used an unobtrusive observational measure of emotional expression with a software that automatically detects facial expressions based on the FACS system (Ekman and Friesen, 1978). This study is novel in its use of automatic FACS processing with elementary aged students during learning activities, as previous research has used automatic detection of emotions to study prosocial behavior, lying and pain expression in children (Larochette et al., 2006; Dys and Malti, 2016; Zanette et al., 2016). Automatic detection of emotions allows researchers to measure the moment-to-moment emotions experienced during an academic task. In education research, measures of emotional traits or typical emotional behavior are commonly used (Pekrun et al., 2011). While these methods hold great value in understanding patterns of emotional behavior during learning, it is equally important to understand the role of individual emotions on learning (D’Mello and Graesser, 2012; Harley et al., 2015). Additional research is needed to more thoroughly understand the applications and limitations of automatic FACS software in young students, however, a strength of this tool is that automatic detection of emotions allows researchers to capture these dynamic processes in real-time.
Limitations and Future Directions
A limitation of this study is that emotions were measured using one automatic detection method. Emotions are considered multi-componential processes and as such, inferences about their effects are strongest when measured using multi-channel methods which incorporate measurement of arousal, expression, and neural activity (Harley et al., 2015; Järvelä and Bannert, 2019). While it can be challenging to maintain a naturalistic setting while collecting multiple channels of physiological data, future research in this field should continue to use automatic detection of emotions with measures of arousal, to investigate the specific contributions of arousal and emotion to learning.
Future directions of this research should expand upon this research by using explicit prompting strategies to aid students in implementing and effectively using SRL strategies to regulate learning (Bannert et al., 2015). Additionally, this research can be used in classroom settings to analyze the impact of regulation and managing emotions. Using multimodal software, the effects of using SRL prompts and strategies can be analyzed in real time, so educators can understand the impact of managing emotions using SRL strategies (Bannert et al., 2015; Molenaar et al., 2019). This research can be used in the classroom to help educators understand the best ways to utilize SRL strategies in the moment to help students stay engaged and motivated during learning. The pedagogical applications of real-time detection of emotions paired with SRL strategy use can have significant value in improving academic achievement, self-efficacy and engagement in learning.
Data Availability Statement
The datasets generated for this study are not readily available because at the time this study was conducted, we did not receive written informed consent to share this data.
Ethics Statement
The studies involving human participants were reviewed and approved by University of Toronto Research Ethics Board (REB). Written informed consent to participate in this study was provided by the participants’ legal guardian/next of kin. Participants provided verbal and written assent to participate in this study.
Author Contributions
SB conducted the data analysis and approximately 60% of the writing of this manuscript. AZ provided assistance and guidance in the data analysis and completed approximately 30% of the writing and the majority of the editing. ML participated in coding and completed approximately 10% of the writing of this manuscript. EW participated in the development of the project, oversaw the coding procedures, and the editing of this manuscript. All authors contributed to the article and approved the submitted version.
Funding
This project was funded by the Social Sciences and Humanities Council of Canada (435-2016-1590) for research in academic emotions, student engagement and online knowledge building.
Conflict of Interest
The authors declare that the research was conducted in the absence of any commercial or financial relationships that could be construed as a potential conflict of interest.
Supplementary Material
The Supplementary Material for this article can be found online at: https://www.frontiersin.org/articles/10.3389/feduc.2020.588043/full#supplementary-material
References
Babayiğit, S., Roulstone, S., and Wren, Y. (2020). Linguistic comprehension and narrative skills predict reading ability: a 9-year longitudinal study. Br. J. Educ. Psychol. doi: 10.1111/bjep.12353 [Epub ahead of print].
Bannert, M., Sonnenberg, C., Mengelkamp, C., and Pieger, E. (2015). Short- and long-term effects of students’ self-directed metacognitive prompts on navigation behavior and learning performance. Comput. Hum. Behav. 52, 293–306. doi: 10.1016/j.chb.2015.05.038
Bartlett, M., Littlewort, G., Vural, E., Whitehill, J., Wu, T., Lee, K., et al. (2009). “Insights on spontaneous facial expressions from automatic expression measurement,” in Dynamic Faces: Insights from Experiments and Computation, eds M. Giese, C. Curio, and H. Bulthoff (Cambridge, MA: MIT Press).
Cain, K., and Oakhill, J. (2008). “Introduction to comprehension development,” in Children’s Comprehension Problems in Oral and Written Language: A Cognitive Perspective, eds K. Cain and J. Oakhill (New York, NY: Guilford Press), 3–40.
Calvo, R., and D’Mello, S. (2011). New Perspectives on Affect and Learning Technologies. Berlin: Springer-Verlag.
Daley, S. G., Willett, J. B., and Fischer, K. W. (2014). Emotional responses during reading: physiological responses predict real-time reading comprehension. J. Educ. Psychol. 106, 132–143. doi: 10.1037/a0033408
D’Mello, S. (2013). A selective meta-analysis on the relative incidence of discrete affective states during learning with technology. J. Educ. Psychol. 105, 1082–1099. doi: 10.1037/a0032674
D’Mello, S., and Graesser, A. (2012). Dynamics of affective states during complex learning. Learn. Instruct. 22, 145–157. doi: 10.1016/j.learninstruc.2011.10.001
D’Mello, S., Kappas, A., and Gratch, J. (2017). The affective computing approach to affect measurement. Emot. Rev. 10, 174–183. doi: 10.1177/1754073917696583
D’Mello, S., Lehman, B., Pekrun, R., and Graesser, A. (2014). Confusion can be beneficial for learning. Learn. Instruct. 29, 153–170. doi: 10.1016/j.learninstruc.2012.05.003
Dweck, C. S. (1999). Self-Theories: Their Role in Motivation Personality and Development. London: Psychology Press.
Dys, S. P., and Malti, T. (2016). It’s a two-way street: automatic and controlled processes in children’s emotional responses to moral transgressions. J. Exper. Child Psychol. 152, 31–40. doi: 10.1016/j.jecp.2016.06.011
Ekman, P., and Friesen, W. V. (1978). Facial Action Coding System: A Technique for the Measurement of Facial Movement. New York, NY: Consulting Psychologists Press.
Gable, P., and Harmon-Jones, E. (2010). The blues broaden, but the nasty narrows: attentional consequences of negative affects low and high in motivational intensity. Psychol. Sci. 21, 211–215. doi: 10.1177/0956797609359622
Gershon, R. C., Slotkin, J., Manly, J. J., Blitz, D. L., Beaumont, J. L., Schnipke, D., et al. (2013). IV. NIH toolbox cognition battery (CB): measuring language (vocabulary comprehension and reading decoding). Monogr. Soc. Res. Child Dev. 78, 49–69. doi: 10.1111/mono.12034
Graesser, A. C. (2019). Emotions are the experiential glue of learning environments in the 21st century. Learn. Instruct. 2019:101212. doi: 10.1016/j.learninstruc.2019.05.009
Graesser, A. C., and D’Mello, S. (2012). Moment-to-moment emotions during reading. Read. Teach. 66, 238–242. doi: 10.1002/TRTR.01121
Greene, J. A., and Azevedo, R. (2009). A macro-level analysis of SRL processes and their relations to the acquisition of a sophisticated mental model of a complex system. Contemp. Educ. Psychol. 34, 18–29. doi: 10.1016/j.cedpsych.2008.05.006
Grills-Taquechel, A. E., Fletcher, J. M., Vaughn, S., Barth, A., Denton, C. A., and Stuebing, K. K. (2014). Anxiety and response to reading intervention among first grade students. Child Youth Care Forum 43, 417–431. doi: 10.1007/s10566-014-9244-3
Grills-Taquechel, A. E., Fletcher, J. M., Vaughn, S. R., and Stuebing, K. K. (2012). Anxiety and reading difficulties in early elementary school: evidence for unidirectional- or bi-directional relations? Child Psychiatr. Hum. Dev. 43, 35–47. doi: 10.1007/s10578-011-0246-1
Hadwin, A. F. (2019). Commentary and future directions: what can multi-modal data reveal about temporal and adaptive processes in self-regulated learning? Learn. Instruct. 2019:101287. doi: 10.1016/j.learninstruc.2019.101287
Harley, J. M., Bouchet, F., Hussain, M. S., Azevedo, R., and Calvo, R. (2015). A multi-componential analysis of emotions during complex learning with an intelligent multi-agent system. Comput. Hum. Behav. 48, 615–625. doi: 10.1016/j.chb.2015.02.013
Immordino-Yang, M. H., and Damasio, A. (2007). We feel, therefore we learn: the relevance of affective and social neuroscience to education. Mind Brain Educ. 1, 3–10. doi: 10.1111/j.1751-228X.2007.00004.x
Jang, E. E., Lajoie, S. P., Wagner, M., Xu, Z., Poitras, E., and Naismith, L. (2017). Person-oriented approaches to profiling learners in technology-rich learning environments for ecological learner modeling. J. Educ. Comput. Res. 55, 552–597. doi: 10.1177/0735633116678995
Jarrell, A., Harley, J. M., Lajoie, S., and Naismith, L. (2017). Success, failure and emotions: examining the relationship between performance feedback and emotions in diagnostic reasoning. Educ. Technol. Res. Dev. 65, 1263–1284. doi: 10.1007/s11423-017-9521-6
Järvelä, S., and Bannert, M. (2019). Temporal and adaptive processes of regulated learning - What can multimodal data tell? Learn. Instruct. 2019:101268. doi: 10.1016/j.learninstruc.2019.101268
Knörzer, L., Brünken, R., and Park, B. (2016). Emotions and multimedia learning: the moderating role of learner characteristics. J. Comput. Assist. Learn. 32, 618–631. doi: 10.1111/jcal.12158
Lagattuta, K. H., Elrod, N. M., and Kramer, H. J. (2016). How do thoughts, emotions, and decisions align? A new way to examine theory of mind during middle childhood and beyond. J. Exper. Child Psychol. 149, 116–133. doi: 10.1016/j.jecp.2016.01.013
Larochette, A. C., Chambers, C. T., and Craig, K. D. (2006). Genuine, suppressed and faked facial expressions of pain in children. Pain 126, 64–71. doi: 10.1016/j.pain.2006.06.013
Meinhardt, J., and Pekrun, R. (2003). Attentional resource allocation to emotional events: an ERP study. Cogn. Emot. 17, 477–500. doi: 10.1080/02699930244000039
Molenaar, I., Horvers, A., and Baker, R. S. (2019). What can moment-by-moment learning curves tell about students’ self-regulated learning? Learn. Instruct. 2019:101206. doi: 10.1016/j.learninstruc.2019.05.003
Pekrun, R. (2006). The control-value theory of achievement emotions: assumptions, corollaries, and implications for educational research and practice. Educ. Psychol. Rev. 18, 315–341. doi: 10.1007/s10648-006-9029-9
Pekrun, R. (2017). Emotion and achievement during adolescence. Child Dev. Perspect. 11, 215–221. doi: 10.1111/cdep.12237
Pekrun, R., Goetz, T., Daniels, L. M., Stupnisky, R. H., and Perry, R. P. (2010). Boredom in achievement settings: exploring control-value antecedents and performance outcomes of a neglected emotion. J. Educ. Psychol. 102, 531–549. doi: 10.1037/a0019243
Pekrun, R., Goetz, T., Frenzel, A. C., Barchfeld, P., and Perry, R. P. (2011). Measuring emotions in students’ learning and performance: the achievement emotions questionnaire (AEQ). Contemp. Educ. Psychol. 36, 36–48. doi: 10.1016/j.cedpsych.2010.10.002
Pekrun, R., Goetz, T., Titz, W., and Perry, R. P. (2002). Academic emotions in students’ self-regulated learning and achievement: a program of qualitative and quantitative research. Educ. Psychol. 37, 91–105. doi: 10.1207/S15326985EP3702_4
Pekrun, R., Lichtenfeld, S., Marsh, H. W., Murayama, K., and Goetz, T. (2017). Achievement emotions and academic performance: longitudinal models of reciprocal effects. Child Dev. 88, 1653–1670. doi: 10.1111/cdev.12704
Pekrun, R., and Perry, R. P. (2014). “Control-value theory of achievement emotions,” in International Handbook of Emotions in Education, eds R. Pekrun and L. Linnenbrink-Garcia (Tennessee: Rutledge).
Pintrich, P. R. (2000). “The role of goal orientation in self regulated learning,” in Handbook of Self Regulation, eds M. Boekaerts, P. Pintrich, and M. Zeidner (Cambridge, MA: Academic Press).
Posner, J., Russell, J. A., and Peterson, B. S. (2005). The circumplex model of affect: an integrative approach to affective neuroscience, cognitive development, and psychopathology. Dev. Psychopathol. 17, 715–734. doi: 10.1017/S0954579405050340
Preacher, K. J., and Hayes, A. F. (2008). Asymptotic and resampling strategies for assessing and comparing indirect effects in multiple mediator models. Behav. Res. Methods 40, 879–891. doi: 10.3758/BRM.40.3.879
Price, M. J., Mudrick, N. V., Taub, M., and Azevedo, R. (2018). “The role of negative emotions and emotion regulation on self-regulated learning with MetaTutor,” in Intelligent Tutoring Systems, eds R. Nkambou, R. Azevedo, and J. Vassileva (Cham: Springer).
Reese, E., Suggate, S., Long, J., and Schaughency, E. (2010). Children’s oral narrative and reading skills in the first 3 years of reading instruction. Rea. Writ. 23, 627–644. doi: 10.1007/s11145-009-9175-9
Reilly, J., Losh, M., Bellugi, U., and Wulfeck, B. (2004). Frog, where are you?” Narratives in children with specific language impairment, early focal brain injury, and Williams syndrome. Brain Lang. 88, 229–247. doi: 10.1016/S0093-934X(03)00101-9
Sabourin, J. L., and Lester, J. C. (2014). Affect and engagement in game-basedlearning environments. IEEE Trans. Affect. Comput. 5, 45–56. doi: 10.1109/T-AFFC.2013.27
Scherer, K. R. (2009). The dynamic architecture of emotion: evidence for the component process model. Cogn. Emot. 23, 1307–1351. doi: 10.1080/02699930902928969
Scherer, K. R., and Moors, A. (2019). The emotion process: event appraisal and component differentiation. Annu. Rev. Psychol. 70, 719–745. doi: 10.1146/annurev-psych-122216-011854
Sinclair, J., Jang, E. E., Azevedo, R., Lau, C., Taub, M., and Mudrick, N. V. (2018). “Changes in emotion and their relationship with learning gains in the context of MetaTutor,” in Intelligent Tutoring Systems. ITS 2018. Lecture Notes in Computer Science, Vol. 10858, eds R. Nkambou, R. Azevedo, and J. Vassileva (Cham: Springer), doi: 10.1007/978-3-319-91464-0_20
Storch, S. A., and Whitehurst, G. J. (2002). Oral language and code-related precursors to reading: evidence from a longitudinal structural model. Dev. Psychol. 38, 934–947. doi: 10.1037/0012-1649.38.6.934
Taub, M., Azevedo, R., Rajendran, R., Cloude, E. B., Biswas, G., and Price, M. J. (2019). How are students’ emotions related to the accuracy of cognitive and metacognitive processes during learning with an intelligent tutoring system? Learn. Instruct. 2019:101200. doi: 10.1016/j.learninstruc.2019.04.001
Taub, M., Mudrick, N. V., Rajendran, R., Dong, Y., Biswas, G., and Azevedo, R. (2018). “How are students’ emotions associated with the accuracy of their note taking and summarizing during learning with ITSs?,” in Intelligent Tutoring Systems. ITS 2018. Lecture Notes in Computer Science, Vol. 10858, eds R. Nkambou, R. Azevedo, and J. Vassileva (Cham: Springer), doi: 10.1007/978-3-319-91464-0_23
Winne, P. H. (2017). “Cognition and metacognition within self-regulated learning,” in Handbook of Self Regulated Learning and Performance, eds P. A. Alexander, D. H. Schunk, and J. A. Greene (Abingdon: Routledge).
Winne, P. H., and Hadwin, A. R. (1998). “Studying as self-regulated learning,” in Metacognition in Educational Theory and Practice, eds D. J. Hacker, J. Dunlosky, and A. Graesser (New Jersey: Lawrence Erlbaum).
Wortha, F., Azevedo, R., Taub, M., and Narciss, S. (2019). Multiple negative emotions during learning with digital learning environments - evidence on their detrimental effect on learning from two methodological approaches. Front. Psychol. 10:2678. doi: 10.3389/fpsyg.2019.02678
Zanette, S., Gao, X., Brunet, M., Bartlett, M. S., and Lee, K. (2016). Automated decoding of facial expressions reveals marked differences in children when telling antisocial versus prosocial lies. J. Exper. Child Psychol. 150, 165–179. doi: 10.1016/j.jecp.2016.05.007
Zheng, L. (2016). The effectiveness of self-regulated learning scaffolds on academic performance in computer-based learning environments: a meta-analysis. Asia Pac. Educ. Rev. 17, 187–202. doi: 10.1007/s12564-016-9426-9
Keywords: emotions, academic emotions, self-regulated learning, planning, frustration, literacy, emerging readers
Citation: Buono S, Zdravkovic A, Lazic M and Woodruff E (2020) The Effect of Emotions on Self-Regulated-Learning (SRL) and Story Comprehension in Emerging Readers. Front. Educ. 5:588043. doi: 10.3389/feduc.2020.588043
Received: 28 July 2020; Accepted: 07 October 2020;
Published: 03 November 2020.
Edited by:
Evely Boruchovitch, State University of Campinas, BrazilReviewed by:
Zhen Wu, Tsinghua University, ChinaDiamanto Filippatou, University of Thessaly, Greece
Copyright © 2020 Buono, Zdravkovic, Lazic and Woodruff. This is an open-access article distributed under the terms of the Creative Commons Attribution License (CC BY). The use, distribution or reproduction in other forums is permitted, provided the original author(s) and the copyright owner(s) are credited and that the original publication in this journal is cited, in accordance with accepted academic practice. No use, distribution or reproduction is permitted which does not comply with these terms.
*Correspondence: Stephanie Buono, c3RlcGhhbmllLmJ1b25vQG1haWwudXRvcm9udG8uY2E=