- 1Department of Rehabilitation Sciences, College of Health and Rehabilitation Sciences, Princess Nourah bint Abdulrahman University, Riyadh, Saudi Arabia
- 2Department of Physical Therapy, Faculty of Medical Rehabilitation Sciences, King Abdulaziz University, Jeddah, Saudi Arabia
- 3Department of Rehabilitation Sciences, College of Applied Medical Sciences, King Saud University, Riyadh, Saudi Arabia
This study aims at describing academic self-efficacy in online learning and exploring the determinant factors of learners’ academic self-efficacy. In this cross-sectional survey-based study, 892 respondents voluntarily participated in and completed the survey. Online learning self-efficacy was assessed using an online academic self-efficacy questionnaire. The sociodemographic characteristics showed that 85% of the respondents were female, 21% were medical students, and 13% were postgraduate students. Most respondents reported insufficient self-efficacy for learning and time management, whereas 51% showed good self-efficacy for technology. When considering the study’s field, more medical students reported good self-efficacy scores for learning and technology (36% and 68%, respectively) than non-medical students (33% and 47%, respectively). Time management self-efficacy was mostly reported to be good (47%) among those with a high grade point average. The results showed that educational status, field of study, and learning satisfaction were significant predictors of self-efficacy for learning and technology (p < 0.001). Gender, academic performance, and learning satisfaction were significant predictors of self-efficacy for time management (p < 0.001). Self-efficacy can play a major role in online learning, particularly in circumstances like the sudden transformation of the educational system that has occurred during the COVID-19 pandemic. An effective academic self-efficacy of learning, use of technology, and time management skills should also be emphasized. The value of this research lies in exploring the electronic learning self-efficacy and its predictor among university students. The research findings help us improve students’ academic self-efficacy to enhance the learning environment by planning new studies.
Introduction
Academic self-efficacy is a core element of a successful learning environment (Shen et al., 2013). It is defined as “individuals’ confidence in their ability to successfully perform academic tasks at a designated level” (Schunk, 1991). The construct of self-efficacy was recognized by Bandura in 1997 as “beliefs in one’s capabilities to organize and execute the courses of action required to produce given attainments” (Bandura, 1997). Self-efficacy indicates the way in which individuals feel, think, and perceive motivation, thereby determining their actions and behaviors.
Bandura’s social-cognitive theory recognizes self-efficacy as an element of learning systems that influences students’ development (Bandura, 1986; Zimmerman et al., 1992). Higher academic self-efficacy might be directly linked to students’ coping behavior. Students with higher self-efficacy are more likely to present a persistence in their manner, that is they do not easily despair and always strive to achieve their goals (Adams et al., 2020; Lee et al., 2020; Musa, 2020). Bandura suggests that learners’ self-efficacy might be affected by four different factors: performance accomplishments, vicarious experiences, verbal persuasions, and physiological states (Bandura, 1997). According to some researchers, self-efficacy for online learners might be influenced by previous success with online learning, pre-course training, instructor feedback, and anxiety related to the online learning technology (Bates and Khasawneh, 2007).
Learners’ self-efficacy may have been affected by the sudden transition to electronic learning and use of online educational methods consequential to the COVID-19 outbreak, and the effects may differ between students. This unprecedented transition has caused students in the Kingdom of Saudi Arabia (KSA) to possibly doubt their abilities to accomplish the required online academic tasks. As mentioned above, self-efficacy is crucial to academic learning and performance (Schunk, 1991; Hodges, 2008). Self-efficacy variations could be attributed to different factors, such as age, gender, level of education, field of education, and the students’ educational institute at which the students are receiving education. A previous study investigated students’ self-efficacy components, which are technology self-efficacy, learning self-efficacy, and social interaction self-efficacy (Shen et al., 2013). The author found that students’ gender, level of academic status, and number of virtual courses accounted for the variation in the students’ self-efficacy to complete the course. Academic levels were also found to be strong predictors of students’ self-efficacy in the context of handling digital technological tools (Shen et al., 2013).
The concept of self-efficacy for electronic learning among university students in the KSA, particularly during the COVID-19 pandemic, requires investigation to clarify the factors that may contribute to their electronic learning self-efficacy. The domains of electronic learning self-efficacy (learning, time management, and technology) may have been affected by the sudden changes in the educational system due to the pandemic. In this respect, there is a gap in knowledge regarding Saudi Arabian universities. Therefore, the aim of the current study is twofold: 1) to characterize academic self-efficacy and its domains in the context of online learning and 2) to explore the determinant factors of learners’ academic self-efficacy. The study considers the differences in gender, age, and educational component, among students, as determinant factors. The findings will shed light on the role of self-efficacy in online learning environments, which could be the key to successful learning experiences and performance. Furthermore, the findings may provide strategies for improving online learning methods and outcomes.
Materials and Methods
Study Design and Population
In this cross-sectional survey-based study, an electronic questionnaire was distributed, and data were collected during the COVID-19 lockdown between May and June 2020 in the KSA. Students in the medical field (medicine, applied medical sciences, dental, and pharmacy) and non-medical professions (arts, engineering, computer sciences, and commerce) were included in the study. The survey was generated using an online survey system (SurveyMonkey). The inclusion criterion entailed students who were enrolled in the largest top universities in the KSA.
A convenience sample consisting of 892 students consented to participate in the study. A total of 759 respondents were female (85%), and 133 respondents were male (15%). In terms of specific sample characteristics, 21% of respondents were in the medical field, while 79% were in the non-medical study field. This study was approved by the Institutional Review Board of the Princess Nourah Bint Abdulrahman University, KSA (IRB H-01-R-059). Informed consent was obtained from the respondents prior to participation.
Study Procedure
The online survey was sent to students via email with a link to the description of the study and the survey. The survey took approximately 10 min to complete. To maximize the response rate, students were sent a biweekly reminder and were encouraged to share the link with classmates and colleagues.
Study Instrumentation
The survey used in this study consisted of demographic information and an online learning self-efficacy (OLSES) questionnaire. The survey’s design was adapted from a study on academic self-efficacy which was studied and validated by Zimmerman and Kulikowich (2016). The OLSES questionnaire consists of 22 items related to the three domains of academic self-efficacy: electronic learning (10 items), time management (five items), and technology (seven items) (Zimmerman and Kulikowich, 2016). The items were rated on a 6-point Likert scale, which ranged from “strongly disagree” to “strongly agree.” Participants who answered “agree” or “strongly agree” were deemed to have good self-efficacy. Those who answered “slightly disagree” or “slightly agree” were deemed to have insufficient self-efficacy. Those who answered “disagree” or “strongly disagree” to the items were deemed to have poor self-efficacy. The reliability results of the scale revealed Cronbach’s alphas of 0.89 for the 10-item online learning environment subscale, 0.85 for the five-item time management subscale, and 0.84 for the seven-item technology use subscale.
The electronic learning satisfaction questionnaire of electronic learning was assessed using electronic learning satisfaction questionnaire of electronic learning which was adapted from Wang (2003). It had a Cronbach’s alpha of 0.95, indicating excellent reliability. The satisfaction measurement had 20 items, which were ranked on a 5-point Likert scale, ranging from 1, “strongly disagree” to 5, “strongly agree.”
Statistical Analyses
The Kolmogorov-Smirnov test was performed to evaluate the normality assumption of the data. None of the major outcomes followed a normal distribution; therefore, the data were presented as medians (MED) and interquartile ranges (IQR) for continuous variables and as frequency and percentage (%) for categorical variables. Only complete responses were included in the analysis. This study examined the students’ electronic learning self-efficacy with respect to Saudi Arabia’s education system. The study focused on comparing the difference and examined the moderating effect of factors like gender, field of study, and academic level which may moderate the change in the students’ academic self-efficacy. A chi-squared test and Fisher’s exact test were conducted to compare self-efficacy response scores of poor, insufficient, and good. Ordered logistic regression was used to assess the predictor of self-efficacy domains. Statistical significance was set at a level of p ≤ 0.05. The data were analyzed using Stata version 16 (Stata Corp LP, College Station, TX, United States).
Results
Sociodemographic Characteristics
A total of 892 students voluntarily participated in the study. Approximately 85% were between 18 and 25 years old (Table 1). Twenty-four percent held bachelors’ degree in the medical field and seventy-nine percent were enrolled in non-medical sciences programs. A total of five universities were included in the study, and most of the students were from Princess Nourah Bint Abdulrahman University (404; 45%). Figure 1 shows the percentage of students from each university who completed the survey.
Characterization of Academic Self-Efficacy Domains
Students were asked to report their academic self-efficacy in three domains: learning, time management, and technology use. The descriptive statistical results gathered from the questionnaire on those domains are reported in Figure 2. Most of the students reported insufficient self-efficacy for learning and time management. However, they reported having good perceived self-efficacy for the technology being used in electronic learning. Fisher’s exact test results were used to determine whether the self-efficacy levels related to learning, time management, and technology use differ significantly according to the students’ educational performance as reflected by their grade point average (GPA), learning satisfaction, academic field of study, education status, gender, age, or university (Tables 2–4).
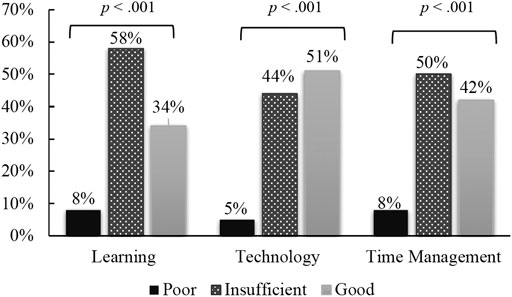
FIGURE 2. Participants’ responses to the electronic learning self-efficacy domains. Fisher’s exact used in the analysis.
Self-efficacy is domain-specific and is influenced by several factors. Since the education system in KSA is gender-segregated, students’ samples were stratified based on education related factors to compare the difference and explore the predictors. As seen in Table 2, students’ learning self-efficacy only differed significantly in terms of their field of the study. The finding showed that 36% of the medical students reported good self-efficacy for learning compared to 33% of the non-medical students. Fifty-eight percent of the non-medical students reported insufficient learning self-efficacy compared to sixty-one percent of the medical field students. There was no significant difference in students’ learning self-efficacy across universities.
We found that technology self-efficacy significantly differed according to the field of study and university (Table 3). Our findings show that most of the medical students (68%) reported good technology self-efficacy compared to the non-medical students (48% of whom reported insufficient technology self-efficacy). The technology self-efficacy of students who perceived themselves to be successful and reported good self-efficacy differed significantly across universities as students at King Abdulaziz University showed the highest percentage (59%), followed by King Saud University (58%), Taibah University (54%), Princess Nourah Bint Abdulrahman University (49%), and Imam Muhammed Bin Saud University (43%).
Students’ self-efficacy related to time management differed significantly based on the students’ academic performance, as reflected by their GPA. Of those who reported higher GPAs, most (47%) had good time management self-efficacy (Table 4). In comparison, students with lower GPAs reported a higher percentage of insufficient time management self-efficacy. As presented in Table 4, the time management self-efficacy responses were significantly different across the universities.
Predictors of Academic Self-Efficacy Domains
Students’ characteristics, including gender, age, academic performance (GPA), field of study (medical/non-medical), educational status, and learning satisfaction, were first tested for the prediction of the learning and technology self-efficacy models. The fit indices for learning and technology self-efficacy models were obtained via ordered logistic regression (the likelihood ratio chi2 = 724, Pseud R2 = 0.27, p < 0.001; the likelihood ratio chi2 = 533, Pseud R2 = 0.20, p < 0.001, respectively). The results indicated that only educational status (bachelor/postgraduate), field of study (medical), and learning satisfaction significantly predicted self-efficacy for learning and technology. According to the data, gender, academic performance (GPA), and learning satisfaction had a significant effect on self-efficacy for time management. The fit indices for time management self-efficacy models showed likelihood ratio chi2 = 461, Pseud R2 = 0.17, p < 0.001 (Table 5).
Discussion
In this study, we investigated three academic self-efficacy domains (i.e., learning, time management, and technology use) of university students in the KSA. We also examined whether these domains were associated with gender, age, educational components (i.e., field of study, educational status, academic performance, and university), or learning satisfaction. Our results indicate that different factors tend to predict each domain of self-efficacy. Educational status (bachelor’s/postgraduate), field of study (medical), and student satisfaction predicted learning and technology self-efficacy significantly. Similarly, gender (female), academic performance (GPA), and learning satisfaction had a significant effect on time management self-efficacy. The students’ time management self-efficacy differed significantly by academic performance: generally, students with excellent GPAs (defined as percentage grade ≥90%) exhibited higher time management self-efficacy than those with good and fair GPAs. However, we also found that time management self-efficacy differed across universities in Saudi Arabia as it was higher among students at Taibah University. Further, medical students had higher self-efficacy scores for learning and technology than non-medical students. These findings are consistent with previous studies (Gore, 2006; Elias and MacDonald, 2007; Hejazi et al., 2009).
The concept of self-efficacy has been widely recognized in several fields, including the academic field of education. Derived from Bandura’s self-efficacy theory, academic self-efficacy reflects an individual’s belief in their ability to accomplish a specific task and to regulate their belief in different educational duties (Bandura, 1997; Schunk and Pajares, 2002; Gore, 2006). Academic self-efficacy of students contributes to learning and may differ based on different essential factors. An educational component may influence self-efficacy related to learning, such as the ability to succeed with electronic learning, handle electronic technology, and apply time management skills.
In the current study, students’ academic fields influenced their self-efficacy, as students who were enrolled in medical programs had higher overall academic self-efficacy than those in non-medical programs. These findings could be attributed to factors, such as program type and instructor support. The universities in KSA adhere to a competitive criterion for students enrolling into the medical programs, which includes a high GPA in the high school, excellent performance in the General Aptitude Test, and success in the interview conducted by the admission committee. In addition, since the language of instruction used in the medical programs is English, students enrolled in the medical programs might comprehend English better compared to students in the non-medical programs. Students’ abilities to speak and understand English might provide them with more resources in electronic learning environments; moreover, it has been found that English proficiency had a positive effect on the academic performance in students who were non-native English speakers (Andrade, 2009; Jimoh and Kenneth, 2016; Rafiu and Nwalo, 2016). Previous studies have found that teachers’ support and motivation can affect students’ self-efficacy and might reshape their learning self-efficacy (Mitchell and DellaMattera, 2010; Kim et al., 2018). A direct negative correlation has been identified between the behavior of the educators in the class and students’ self-efficacy, as negative behavior affects students negatively (Banfield, 2009). This information suggests that teachers’ good behavior toward their students should be encouraged as it influences students positively, sculpting their self-efficacy. However, we did not study instructors’ behaviors and their relationships to our participants; thus, we cannot elaborate on this topic.
Our findings showed that students with higher GPAs tended to exhibit higher self-efficacy. This is in line with the findings of a previous study that compared students with low and high self-efficacy. It was found that students with higher self-efficacy increased their mental effort related to learning by using well-organized learning strategies and managing arduous academic duties (Chemers et al., 2001; Margolis and McCabe, 2003). In comparison, students with lower levels of academic self-efficacy experienced a decline in their academic performance and lacked the commitment to complete academic duties (Bandura et al., 1999). Interestingly, another study found that higher academic self-efficacy correlated to higher academic degrees and vice versa (Wuebbels, 2006), which contradicts our finding that self-efficacy does not differ in terms of educational status, as students with bachelor’s degrees showed similar self-efficacy to postgraduate students.
Technology has created new channels for education. Students’ personal beliefs and perceived ability to master the technological tools used in electronic learning may influence their learning environment. According to Davis, (1989) technology acceptance model, two key factors affect the personal intention to use technology: perceived usefulness and ease of use of the new technology. The bidirectional relationship between the factors stated in the technology acceptance model and students’ technology self-efficacy may be the reason for our findings (Gan and Balakrishnan, 2017).
In searching the existing literature, an inconsistency exists in the relationship between technology self-efficacy and intention to use technology in an electronic learning environment. Some studies have revealed that self-efficacy beliefs related to the use of technology influenced students’ adoption of electronic technology (Chen et al., 2013; Coskun and Mardikyan, 2016; Bakhsh et al., 2017). However, others have identified conflicting findings. Some studies have found no relationship between the intention to use technology and self-efficacy; however, these studies have only investigated educators (Purnomo and Lee, 2013; Mac Callum et al., 2014). Such conflicting findings could be attributed to the difference in population between students and educators and their levels of skill and experience with technology. However, the perceived usefulness of technology has not been assessed in the current study and requires further investigation. The results regarding higher technology self-efficacy among medical students might be explained by educational environments.
In the present study, the lack of difference between learning and technology self-efficacy based on academic performance aligned with the findings of previous studies, which revealed no significant difference in academic self-efficacy based on academic performance (Cho and Shen, 2013; Gębka, 2014). In contrast, the correlation between academic self-efficacy and academic achievement in an online learning environment has been outlined in previous studies (Joo et al., 2000; Kitsantas and Chow, 2007; Yukselturk and Bulut, 2007). Operationalization of academic self-efficacy, timing of measurement, and cultural differences have been proposed as reasons for these differences (Honicke and Broadbent, 2016).
Self-efficacy domains can be predicted by several factors. Our results show that academic level, field of study, and learning satisfaction predicted the self-efficacy domains of learning and technology for students at KSA universities. In addition, the time management domain of self-efficacy was predicted by gender, academic performance, and learning satisfaction of students. Aligned with our findings, learning satisfaction was a predictor of learning self-efficacy (Shen et al., 2013) and technology self-efficacy (Lim, 2001; Artino, 2008). Shen et al., (2013) found that students who had higher learning self-efficacy were more satisfied. Lim (2001) and Artino (2008) reported that students who had higher technology self-efficacy were more satisfied with the online learning experience. In our study, we found that learning satisfaction predicted both learning and technology self-efficacy. The findings might suggest reciprocal relationships between learning and technology self-efficacy and learning satisfaction. These findings should encourage educational policymakers in the KSA to incorporate training sessions in computers and information technology for students with low technology self-efficacy. Improving technology self-efficacy in an online learning environment could help students to increase their learning satisfaction and obtain higher grades (Wang et al., 2013).
Interestingly, our data showed differences among students’ technology self-efficacy based on the enrolled university. We found that respondents in King Abdulaziz University (KAU) reported a higher percentage (59%) of good self-efficacy for technology compared with those in KSU, TU, PNU, and IMSIU (58%, 54%, 49%. 43%; respectively). The differences in self-efficacy-related technology might be attributed to the rank of the university by which the quality of education in each university is being assessed. KAU has gained successful experience and pioneered multiple academic programs regarding the development of higher education in the Kingdom of Saudi Arabia (King Abdulaziz University | World University Rankings | THE, n. d.).. KAU has been ranked first among middle east universities and 42nd on a United States news global world ranking (King Abdulaziz University in Saudi Arabia–United States News Best Global Universities, n. d.), which could explain the better responses in technology self-efficacy of KAU students. Moreover, the diversity of academic programs at KAU may also play a role in the differences among students’ responses. KAU has almost twenty different faculties that may include more than 100 different scientific programs. Such variety may indicate the need for high-tech educational methods and equipment, which the students can efficiently utilize to further their education. It is worth noting that KAU is recognized as a top public university in Jeddah and a prestigious university in KSA. Together, the previously mentioned measures may rationalize the good response of technology self-efficacy among enrolled students compared to others.
In terms of the association between gender and self-efficacy, our findings indicate that gender was not a predictor of technology self-efficacy. Similarly, Wang et al. (2013) reported no difference in technology self-efficacy between males and females, according to data from 256 graduate and undergraduate students. However, Shen et al. (2013) reported that being female was a predictor of technology self-efficacy,which is a result not supported by our data. This could be due to the academic status of our students, as the majority of our students were undergraduate females, while Shen recruited more students from the graduate level, and graduate students might be more experienced in dealing with technology (Shen et al., 2013). We cannot elaborate on the difference between undergraduate and graduate students in terms of experience using technology, as this is beyond the scope of the current study. Students’ academic level could also be a predictor of technology self-efficacy. We found that both undergraduate and graduate academic levels were predictors of technology self-efficacy, while according to Shen et al. (2013), graduate students had higher technology self-efficacy. In addition, presently, technology is widely used by most students, which might increase their technology self-efficacy; however, we could not confirm this statement because we did not collect data regarding students’ experience with technology.
Time management self-efficacy could be a pivotal factor in an online learning environment. We reported that higher GPAs were a predictor of time management self-efficacy. According to a study, college students with high GPAs exhibit good practices in terms of time management (Britton and Tesser, 1991). This might imply that students with higher GPAs can wisely manage and invest their time while studying at college. However, students recruited in this previous study (Britton and Tesser, 1991) were enrolled in-person classes. Thus, a comparison is not appropriate since the current study was conducted via online learning classes.
Additionally, we found that gender predicts time management self-efficacy. Similarly, other studies report that undergraduate female students enrolled in face-to-face classes had better time management skills than their male classmates (Bidjerano, 2005). This could be attributed to gender differences in terms of behaviors and use of learning strategies (Bidjerano, 2005); however, qualitative studies might be conducted in the future to investigate the differences in behaviors of male and female students and whether they use learning strategies differently.
Nevertheless, this study has some limitations. First, the study design was cross-sectional, which limits its ability to show cause-and-effect relationships between the variables. Second, the participants in this study were predominantly female students. Thus, the findings might not be representative of men and cannot be generalized to other postgraduate or male samples with varying demographic backgrounds. Third, we did not investigate a few aspects that might affect the domains of self-efficacy, such as interacting with classmates and instructors during online courses, number of online courses that students have taken, and the quality of course instruction. Fourth, we did not measure students’ prior experience using the technology involved in online courses, which requires general computer skills and skills to deal with various platforms of online learning (Wang et al., 2013). Fifth, we utilized a subjective tool to measure the self-efficacy domains and learning satisfaction, which might have some bias that could affect the survey’s construct validity (Thorndike and Thorndike-Christ, 2010). Sixth, the self-efficacy questionnaire has not been validated on students in KSA; therefore, the results might be affected, and future studies might be needed to create a specific self-efficacy scale for students in Arab countries. Seventh, the universities’ facilities and infrastructures might be different, which might create unequal online learning environments for students since we did not control for these differences. Future research should include behavioral and objective instruments to measure self-efficacy and learning satisfaction to obtain more robust information. Besides, recruiting equal numbers of both male and female students should be encouraged to examine whether self-efficacy domains differ by gender since both genders might respond to the questionnaires differently (Bidjerano, 2005). Finally, this study was conducted during the outbreak of the COVID-19 pandemic where the transition to electronic learning occurred in KSA. In this context, the study was conducted to characterize the students’ academic self-efficacy due to complete transition to electronic learning. However, the psychological effect of the COVID-19 pandemic and social distancing—which may influence the student learning—should be taken into consideration in the next research.
Conclusion
In conclusion, self-efficacy may play a major role in online learning. We found that students in medical fields had higher learning and technology self-efficacy compared with students in non-medical fields. In addition, students with higher GPAs had higher time management self-efficacy. Learning satisfaction was a predictor of all domains of self-efficacy. Being an undergraduate or graduate student and a student in a medical field were predictors of learning and technology self-efficacy, whereas being a female student and having a high GPA were predictors of time management self-efficacy. The results might encourage education policymakers to provide students, particularly those in non-medical fields, with appropriate training to use online learning environment technology. This study’s findings outline the salience of academic self-efficacy and emphasize its significance in the learning environment. In addition, students should be provided with time management workshops to enhance their experience of online learning platforms.
Data Availability Statement
The data that support the findings of this study are available on request from the corresponding author.
Ethics Statement
The studies involving human participants were reviewed and approved by the Institutional Review Board of the Princess Nourah Bint Abdulrahman University, KSA (IRB H-01-R-059). The patients/participants provided their written informed consent to participate in this study.
Author Contributions
All the authors contributed substantially to the manuscript. MA, BB, and AA contributed to the conception and analysis of the study. MA, BB, and AA were involved in preparation of the manuscript and reviewed the manuscript for important intellectual content. All authors revised the final version of the manuscript.
Funding
This research was funded by the Deanship of Scientific Research at Princess Nourah Bint Abdulrahman University through the Fast-track Research Funding Program. The role of the sponsors was in the decision to submit the article for publication, but it had no involvement in the data analysis or collection.
Conflict of Interest
The authors declare that the research was conducted in the absence of any commercial or financial relationships that could be construed as a potential conflict of interest.
Acknowledgments
Author would like to thank Princess Nourah Bint Abdulrahman University in helping to conduct the research project. A special thank for the Deanship of Scientific Research at Princess Nourah Bint Abdulrahman University for funding this project through the Fast-track Research Funding Program, Riyadh, Saudi Arabia.
Supplementary Material
The Supplementary Material for this article can be found online at: https://www.frontiersin.org/articles/10.3389/feduc.2021.614333/full#supplementary-material.
References
Adams, A. M., Wilson, H., Money, J., Palmer-Conn, S., and Fearn, J. (2020). Student engagement with feedback and attainment: the role of academic self-efficacy. Assess. Eval. Higher Educ. 45 (1), 317–329. doi:10.1080/02602938.2019.1640184
Andrade, M. S. (2009). The effects of English language proficiency on adjustment to university life. Int. Multilingual Res. J. 3 (1), 16–34. doi:10.1080/19313150802668249
Artino, A. R. (2008). Motivational beliefs and perceptions of instructional quality: predicting satisfaction with online training. J. Comput. Assist. Learn. 24 (3), 260–270. doi:10.1111/j.1365-2729.2007.00258.x
Bakhsh, M., Mahmood, A., and Sangi, N. A. (2017). Examination of factors influencing students and faculty behavior towards m-learning acceptance. Int. J. Inf. Learn. Technol. 34, 166–188. doi:10.1108/IJILT-08-2016-0028
Bandura, A. (1986). The explanatory and predictive scope of self-efficacy theory. J. Soc. Clin. Psychol. 4 (3), 359–373. doi:10.1521/jscp.1986.4.3.359
Bandura, A. (1997). “The nature and structure of self-efficacy,” in Self-efficacy: the exercise of control (New York, NY: W H Freeman and Company), 37–78.
Banfield, S. R. (2009). How do college/university teacher misbehaviors influence student cognitive learning, academic self-efficacy, motivation, and curiosity?. Dr. dissertation, Graduate thesis: West Virginia University. WV, United States
Bandura, A., Freeman, W. H, and Lightsey, R. (1999). Self-efficacy: The exercise of control. J Cognitive Psychotherapy 13, 158–166. doi:10.1891/0889-8391.13.2.158
Bates, R., and Khasawneh, S. (2007). Self-efficacy and college students’ perceptions and use of online learning systems. Comput. Hum. Behav. 23 (1), 175–191. doi:10.1016/j.chb.2004.04.004
Bidjerano, T. (2005). Gender differences in self-regulated learning. Educ. Technol. Soc. 12 (3), 12–22
Britton, B. K., and Tesser, A. (1991). Effects of time-management practices on college grades. J. Educ. Psychol. 83 (3), 405–410. doi:10.1037/0022-0663.83.3.405
Chemers, M. M., Hu, L., and Garcia, B. F. (2001). Academic self-efficacy and first year college student performance and adjustment. J. Educ. Psychol. 93 (1), 55–64. doi:10.1037/0022-0663.93.1.55
Chen, Y. C., Lin, Y. C., Yeh, R. C., and Lou, S. (2013). Examining factors affecting college students’ intention to use web-based instruction systems: towards an integrated model. Turk. Online J. Educ. Technol. 12 (2), 111–121
Cho, M. H., and Shen, D. (2013). Self-regulation in online learning. Dist. Educ. 34 (3), 290–301. doi:10.1080/01587919.2013.835770
Coskun, M., and Mardikyan, S. (2016). Predictor factors for actual usage of online evaluation and assessment systems: a structural equation model (SEM) study. Egitim Bilim 41 (188), 131–152. doi:10.15390/EB.2016.6579
Davis, F. D. (1989). Perceived usefulness, perceived ease of use, and user acceptance of information technology. MIS Q. 13 (3), 319–340. doi:10.2307/249008
Elias, S. M., and MacDonald, S. (2007). Using past performance, proxy efficacy, and academic self-efficacy to predict college performance. J. Appl. Soc. Psychol. 37 (11), 2518–2531. doi:10.1111/j.1559-1816.2007.00268.x
Gan, C. L., and Balakrishnan, V. (2017). Predicting acceptance of mobile technology for aiding student-lecturer interactions: an empirical study. Australas. J. Educ. Technol. 33 (2), 143–158. doi:10.1080/10447318.2017.1380970
Gębka, B. (2014). Psychological determinants of university students’ academic performance: an empirical study. J. Further Higher Educ. 38 (6), 813–837. doi:10.1080/0309877X.2013.765945
Gore, P. A. (2006). Academic self-efficacy as a predictor of college outcomes: two incremental validity studies. J. Career Assess. 14 (1), 92–115. doi:10.1177/1069072705281367
Hejazi, E., Shahraray, M., Farsinejad, M., and Asgary, A. (2009). Identity styles and academic achievement: mediating role of academic self-efficacy. Soc. Psychol. Educ. 12 (1), 123–135. doi:10.1007/s11218-008-9067-x
Hodges, C. B. (2008). Self-efficacy in the context of online learning environments: a review of the literature and directions for research. Perf. Improv. Q. 20, 7–25. doi:10.1002/piq.20001
Honicke, T., and Broadbent, J. (2016). The influence of academic self-efficacy on academic performance: a systematic review. Educ. Res. Rev. 17, 63–84. doi:10.1016/j.edurev.2015.11.002
Jimoh, R., and Kenneth, I. N. N. (2016). Effect of English Language proficiency on students performance in cataloguing and classification courses in polytechnic-based library schools in polytechnic - based library schools in Nigeria. Int. J. Libr. Inf. Sci. 8 (6), 54–61. doi:10.5897/IJLIS2016.0708
Joo, Y.-J., Bong, M., and Choi, H.-J. (2000). Self-efficacy for self-regulated learning, academic self-efficacy, and Internet self-efficacy in Web-based instruction. Educ. Technol. Res. Dev. 48, 5–17. doi:10.1007/BF02313398
Kim, L. E., Dar-Nimrod, I., and MacCann, C. (2018). Teacher personality and teacher effectiveness in secondary school: personality predicts teacher support and student self-efficacy but not academic achievement. J. Educ. Psychol. 110 (3), 309–323. doi:10.1037/edu0000217
Kitsantas, A., and Chow, A. (2007). College students’ perceived threat and preference for seeking help in traditional, distributed, and distance learning environments. Comput. Educ. 48 (3), 383–395. doi:10.1016/j.compedu.2005.01.008
Lee, D., Watson, S. L., and Watson, W. R. (2020). The relationships between self-efficacy, task value, and self-regulated learning strategies in massive open online courses. Int. Rev. Res. Open Distrib. Learn. 21 (1), 23–39. doi:10.19173/irrodl.v20i5.4389
Lim, C. K. (2001). Computer self-efficacy, academic self-concept, and other predictors of satisfaction and future participation of adult distance learners. Am. J. Dist. Educ. 15 (2), 41–51. doi:10.1080/08923640109527083
Mac Callum, K., Jeffrey, L., and Na, K. (2014). Factors impacting teachers’ adoption of mobile learning. J. Inf. Technol. Educ.: Res. 13, 141–162. doi:10.28945/1970
Margolis, H., and McCabe, P. P. (2003). Self-efficacy: a key to improving the motivation of struggling learners. Prev. Sch. Fail. Altern. Educ. Child. Youth 47 (4), 162–169. doi:10.1080/10459880309603362
Mitchell, S., and DellaMattera, J. (2010). Teacher support and student’s self-efficacy beliefs. J. Contemp. Issues Educ. 5 (2), 24–35. doi:10.20355/C5X30Q
Musa, M. (2020). Academic self-efficacy and academic performance among university undergraduate students: an antecedent to academic success. Eur. J. Educ. Stud. 7 (3), 15. doi:10.5281/zenodo.3756004
Purnomo, S. H., and Lee, Y.-H. (2013). E-learning adoption in the banking workplace in Indonesia: an empirical study. Inf. Dev. 29 (2), 138–153. doi:10.1177/0266666912448258
Rafiu, J., and Nwalo, KIN. (2016). Effect of English Language proficiency on students' performance in cataloguing and classification courses in polytechnic - based library schools in Nigeria. Int. J. Library Information Sci. 8 (6), 54–61.
Schunk, D. H. (1991). Self-efficacy and academic motivation. Educ. Psychol. 26, 207–231. doi:10.1080/00461520.1991.9653133
Schunk, D. H., and Pajares, F. (2002). “The Development of Academic Self-Efficacy,” in Development of Achievement Motivation. Editors A. Wigfield, and J. S. Eccles (San Diego, CA: Academic Press), 15–31. doi:10.1016/B978-012750053-9/50003-6
Shen, D., Cho, M., Tsai, C., and Marra, R. (2013). Unpacking online learning experiences: online learning self-efficacy and learning satisfaction. Internet High Educ. 19, 10–17. doi:10.1016/j.iheduc.2013.04.001
Thorndike, R. M., and Thorndike-Christ, T. M. (2010). Measurement and evaluation in psychology and education. London, UK:Pearson Education Inc, 104–105.
Wang, C.-H., Shannon, D. M., and Ross, M. E. (2013). Students’ characteristics, self-regulated learning, technology self-efficacy, and course outcomes in online learning. Dist. Educ. 34 (3), 302–323. doi:10.1080/01587919.2013.835779
Wang, Y.-S. (2003). Assessment of learner satisfaction with asynchronous electronic learning systems. Inf. Manag. 41 (1), 75–86. doi:10.1016/S0378-7206(03)00028-4
Wuebbels, A. L. (2006). The development of a social emotional and academic self-efficacy curriculum for sixth grade adolescent students. PhD thesis: Saint Louis University. MO, United States.
Yukselturk, E., and Bulut, S. (2007). Predictors for student success in an online course. J. Educ. Technol. Soc. 10 (2), 71–83
Zimmerman, B. J., Bandura, A., and Martinez-Pons, M. (1992). Self-Motivation for academic attainment: the role of self-efficacy beliefs and personal goal setting. Am. Educ. Res. J. 29 (3), 663–676. doi:10.3102/00028312029003663
Keywords: self-efficacy, electronic learning, technology, college students, medical field, learning
Citation: Aldhahi MI, Baattaiah BA and Alqahtani AS (2021) Predictors of Electronic Learning Self-Efficacy: A Cross-Sectional Study in Saudi Arabian Universities. Front. Educ. 6:614333. doi: 10.3389/feduc.2021.614333
Received: 05 October 2020; Accepted: 04 January 2021;
Published: 05 February 2021.
Edited by:
Tom Crick, Swansea University, United KingdomReviewed by:
Dorian Gorgan, Technical University of Cluj-Napoca, RomaniaMohammed Fawzi Farghally, Virginia Tech, United States
Copyright © 2021 Aldhahi, Baattaiah and Alqahtani. This is an open-access article distributed under the terms of the Creative Commons Attribution License (CC BY). The use, distribution or reproduction in other forums is permitted, provided the original author(s) and the copyright owner(s) are credited and that the original publication in this journal is cited, in accordance with accepted academic practice. No use, distribution or reproduction is permitted which does not comply with these terms.
*Correspondence: Monira I. Aldhahi, Mialdhahi@pnu.edu.sa