- Department of Educational Psychology, The University of Texas at Austin, Austin, TX, United States
What goes into motivating students to take effective action? Ideally, students are not only motivated to invest time into their studying, but that they use their time in effective and productive ways. In the present study, we surveyed college undergraduates (N = 366) about how they engage in one of their college courses. Specifically, we explored how their motivation-related implicit beliefs (ease and difficulty mindsets, intelligence mindset; Dweck, 2000; Fisher and Oyserman, 2017) interact with perceived course interest and course importance to predict their achievement goal orientation for the course and the quality of their study strategies. We used a person-centered latent profiles analysis approach categorize meaningful profiles of implicit beliefs. Those who were likely to highly endorse motivation-increasing implicit beliefs and who found a course interesting were also more likely to hold mastery-approach goals; the relationship, however, was more complicated for performance-approach and performance-avoidance goals. Implicit beliefs profiles themselves did not directly relate to strategy use, but goal orientation did. In particular, mastery-approach goal orientation was uniquely related to all three of the effective study strategies subscales (e.g., elaborative, standard testing, generative testing). Mastery-avoidance was related to less use of elaborative strategies, and performance-goals were not related to any type strategy use. Perceived course importance was positively related to increased passive and elaborative strategies, but not the standard testing or generative testing strategies. We discuss implications for interventions.
Introduction
What goes into motivating students to take effective action? Research on motivation in the educational domain can be broadly categorized into the theories that relate to domain-general motivation-related implicit beliefs (e.g., interpretations of ease and difficulty, growth mindset) and those that relate to content-specific constructs (e.g., value of a particular task or subject). The existing literature largely focuses on how implicit beliefs and value matter for academics because they affect the ways in which students engage. Beyond just affecting the quantity of study (students’ studying harder and for longer), both domain-general implicit beliefs and task-specific value also affect the quality of study (students’ studying smarter, in deeper, more effective ways). In the present study, we examine how these implicit beliefs and value affect students’ quality of study in two ways: as achievement goals, and as study strategies. We build upon the existing literature in several ways. First, rather than pit one type of implicit belief against another, we consider different types of implicit beliefs collectively by identifying “latent profiles” of beliefs. Second, rather than examining how implicit beliefs and value separately relate to study engagement, we examine them together, finding interactions that may have important implications for intervention. Third, we examine how implicit beliefs, value, and achievement goals relate to students’ use of study strategies, drawing on recent literature from cognitive psychology about different types of strategies.
In the following sections, we first clarify our definition of study quality as achievement goals and study strategies. For each, we describe the reasons for why they are important for academic achievement and how they may be influenced by implicit beliefs and value.
Studying Smarter, Not Just Harder: Achievement Goals and Study Strategies
Particularly in the college context and beyond, learners do not always have more time to study. Rather than focusing on how motivational constructs promote task persistence (studying harder), it is therefore also important to consider how they promote higher quality of study (studying smarter). In the present study, we examine two operationalizations of study quality. The first set of outcomes we are interested in is students’ achievement goal orientations. Achievement goal orientations reflect the qualitative ways in which a student defines a successful learning outcome—are they aiming to just do well on course exams and assignments or are they also aiming to develop their competencies and truly learn the content. As such, achievement goals qualitatively change the target of study effort and are an important precursor for the ways students study. The second set of outcomes that we are interested in is students’ use of specific study strategies (i.e., the qualitative differences in how they engage during study).
Achievement Goals Matter
Achievement goal theory (Ames and Archer, 1988; Elliot, 1999; Elliot and Murayama, 2008; Senko et al., 2011) highlights that there are different types of goals that a person may be oriented toward: performance goals (e.g., getting good grades, doing better than one’s peer) and mastery goals (e.g., developing one’s competence, mastering the to-be-learned content). These two types of goals are not the opposite of one another; individuals may hold both simultaneously. A student might both want to master the content and do well on their exams. But the two types of goals are also distinct: It is possible, for example, that a student will cram to get a good grade on the exam and not care if they forget the content soon afterward. Furthermore, these goals also have a valence: approach (focused on positive success, e.g., striving to get a high grade or learn deeply) or avoidance (focused on avoiding negative failure, e.g., striving to avoid failure, or not learning). This 2 × 2 conceptualization of achievement goals yields four types of goal orientations: mastery-approach, performance-approach, mastery-avoidance, and performance-avoidance.
Achievement goals are an important dependent variable to examine because they matter for academic behavior and outcomes. The avoidance-focused goals (mastery-avoidance and performance-avoidance) both tend to be related to negative outcomes (e.g., anxiety, disengagement; Hulleman et al., 2010). The two approach-focused goals (e.g., mastery-approach and performance-approach goals) share some overlapping outcomes, but also have some distinct outcomes. Both are positively related to academic achievement (although there is some suggestion that performance-approach goals might be more reliably related to academic achievement; Harackiewicz et al., 2002; Hulleman et al., 2010). Performance-approach goals, however, may also be uniquely related to maladaptive behaviors too such as increased anxiety and cheating (Anderman et al., 1998; Midgley et al., 2001; Karabenick, 2003; Murdock et al., 2004). Mastery-approach is thought to be uniquely related to more adaptive behaviors, such as intrinsic motivation, persistence and self-efficacy (Grant and Dweck, 2003; Liem et al., 2008).
Predictors of Achievement Goals
In the present study, we are interested in how implicit beliefs, interest, and value are related to achievement goals. Implicit beliefs shape how we interpret and respond to our experiences, and hence can affect achievement goals. Research on achievement goals has been closely linked to one particular type of implicit belief: belief about the nature of intelligence (Dweck and Leggett, 1988; Dweck, 2000). This research has focused on whether a person believes that intelligence is innate and cannot be changed (fixed mindset) or whether they believe that intelligence can be changed and increased through effort (growth mindset). If one believes that intelligence is fixed, they may become more preoccupied with demonstrating competence (i.e., performance), but if one believes that intelligence can grow, then they are more likely to take on a mastery-focus. Indeed, empirical research finds that growth mindset is related to higher mastery goals and lower performance-avoidance goals (Robins and Pals, 2002; Bråten and Strømsø, 2004; Chen and Pajares, 2010).
Belief about the nature of intelligence, however, is not the only implicit belief that can influence achievement goals. Separate from beliefs about intelligence, people may also directly hold beliefs about how the experience of ease or difficulty during learning is interpreted, and these can have an impact on whether learners are focused. For example, when a learner encounters difficulty in the process of learning, they might take that difficulty as a sign that the odds of success are low (difficulty-as-impossibility), and this may foster an avoidance focus: trying simply not to perform too poorly. In contrast, if the learner interprets that difficulty as a sign that the task is important for them (difficulty-as-importance) and hence that one should lean in and engage more deeply, then this may foster an approach-focus: to do well. Analogous interpretations of ease—ease-as-possibility, ease-as-triviality—are similarly distinct. Indeed, Fisher and Oyserman (2017) found that the motivation-increasing ease and difficulty beliefs (difficulty-as-importance, ease-as-possibility) were related to approach-focused goals and while the motivation-undermining ease and difficulty beliefs (difficulty-as-impossibility, ease-as-triviality) were related to avoidance-focused goals.
Finally, whereas implicit beliefs tend to be relatively domain-general, content-specific factors also play key roles in motivation (e.g., Eccles et al., 1983; Schiefele, 1991). In the present study, we focus on two types of value: interest in course content and perceived importance of a course. Learners with a high individual interest in a topic are more likely to ask questions, process for meaning, be reflective, and engage in the mental work of re-organizing their conceptual understanding (Renninger et al., 2002, 2008). In a similar vein, increasing the perceived importance of a course or topic may also increase motivation and academic outcomes. Both correlational and experimental studies have found that increasing the interest and utility-value of course content (Schiefele, 1991; Hulleman and Harackiewicz, 2009; Harackiewicz and Hulleman, 2010) leads to better academic performance. But studies have also shown that may be important for the quality of study too. There is substantial literature connecting value to goals (Wigfield and Cambria, 2010). Interest, for example, is thought to both give rise to and is deepened by mastery goals (Harackiewicz et al., 2002; Hulleman et al., 2008; Renninger et al., 2008). A qualitative study by Lipstein and Renninger (2007), for example, found that students who with a more developed writing interest set themselves more sophisticated, mastery-oriented goals (as opposed to the more performance-oriented goal of “just get it done”).
Effective Strategies Matter, but Are Not Always Intuitive
Operationalization of deeper learning strategies in the existing education literature has tended to be based on relatively outdated frameworks of learning strategies. In particular, much of the literature has focused on deep versus surface learning strategies (Biggs, 1985; Haggis, 2003). Deep learning strategies focus on understanding meanings and making connections, and include activities like self-explanation and elaboration. Surface strategies are those that focus primarily on memorization of facts. While there is still merit in the deep versus surface distinction, the past three decades of cognitive psychology research has seen a great deal of progress in understanding effective strategies for learning, including identifying other types of strategies as effective, perhaps even more so than the classic deep strategies.
The general understanding that effective strategies are ones that engage learners more deeply into the learning process has not changed. What has changed as a result of empirical research in the past decades, however, is an understanding that effective strategies (see desirable difficulties, Bjork, 1994; Yan et al., 2017) are not always obvious or intuitive (McCabe, 2011; Hartwig and Dunlosky, 2012; Bjork et al., 2013). In a large review of the empirical evidence behind the effectiveness of different study strategies, Dunlosky et al. (2013) rated various study strategies based on whether positive effects of the strategies generalized across learning conditions, student characteristics, materials, and different types of tests. The classic deep strategies, elaboration and self-explanation, were rated as moderate-utility strategies. Another classic deep strategy, summarization, was rated as having low-utility, together with other classic surface strategies, such as rereading and highlighting.
Self-testing, however, was rated as a high-utility strategy. Indeed, one of the most robust findings in cognitive psychology is the benefits of retrieving information from long-term memory—it deepens the learning and makes it more easily recalled in the future (Bjork, 1975; Roediger et al., 2011; Rowland, 2014, and it is often missing from operationalizations of deep learning strategies (e.g., as in the Motivated Strategies for Learning Questionnaire, MSLQ; Pintrich et al., 1991), or combined together with low-utility rereading strategies (e.g., as in the Learning and Study Strategies Inventory, LASSI; Weinstein et al., 1987). Moreover, there are benefits of self-testing even when you cannot retrieve anything. The very act of attempting to generate a response, and hence activating one’s prior knowledge, helps to potentiate subsequent learning: that is, there are benefits to testing oneself even before initial learning (Yan et al., 2014b; Little and Bjork, 2016; Sana et al., 2020). These aspects of retrieval practice tend to be missing from the older formulations of deep learning, or are often combined together with the less effective strategies. Unlike the benefits of elaborative processing, the benefits of retrieval practice are consistently underappreciated, especially when compared to rereading (Roediger and Karpicke, 2006; Kirk-Johnson et al., 2019). In fact, Kirk-Johnson et al. (2019) found that the more effortful participants rated retrieval practice, the less effective they thought the strategy was. These results imply that learners, in general, believe that effortful learning is poor learning, and that these misperceptions of strategy effectiveness are related to their study choices.
In the present study, we broaden the examination of quality of how students engage in their studies (and the relationships to motivation) beyond surface and deep by measuring the use of each of these different types of strategies—the passive strategies (rereading, highlighting, summarizing), the elaboration-related strategies (self-explanation, elaboration), and the self-testing strategies (in its myriad forms). Although it was not reviewed by Dunlosky et al. (2013), we also add another strategy for which empirical research has shown pedagogical benefits: varying the way in which to-be-learned information is studied (McDaniel and Masson, 1985; Mannes and Kintsch, 1987).
Predictors of study strategies
There are good reasons to predict that implicit beliefs should matter for use of effective study strategies, especially for the “desirably difficult” strategies. To the extent that experiences of difficulty are interpreted as being a sign that “I must not be learning” and that experiences of ease are interpreted as well-learned (Koriat, 2008; Kirk-Johnson et al., 2019), learners may judge these effective strategies as being ineffective and vice versa. Given that the metacognitive experience of effective strategies is a key factor in whether people recognize their efficacy and choose to use them, the socio-motivational research on ease and difficulty beliefs, and on naive theories of intelligence, are likely relevant. Evidence currently remains fairly sparse. A number of studies have shown that those with a growth mindset are less likely to be misled by experiences of fluency (Miele and Molden, 2010; Miele et al., 2011), although this work did not directly tie the implicit belief to study strategies. There is some indication that a growth mindset is related to appreciating the benefits of retrieval practice (Yan et al., 2014a) and difficulty-as-impossibility mindset as being related to endorsement of learning misconceptions (e.g., that learning should be easy, that rereading is more effective than testing; Yan and Oyserman, 2017). Hence, in the present study, we examine whether implicit beliefs are related to use of different types of strategies.
Content-specific value may also be related to use of better strategies. Lipstein and Renninger found that more interested students were also more likely to use effective strategies to meet their writing goals; Schiefele (1991) found that interest was related to use of deeper, more elaborative strategies (as measured by the elaboration subscale of the MSLQ). Those who value a course and see it as important for their future job, for example, are more likely to use deeper processing strategies and less likely to use surface processing strategies (Simons et al., 2004). Importantly, however, we do not know if the same patterns arise for the high-utility retrieval-based (self-testing) strategies. In Simons et al. (2004), for instance, their definition of deep strategies included summarization, rereading and underlining text—all strategies that Dunlosky et al. (2013) rated as low utility.
Finally, in the present study, we not only treat achievement goals as a dependent variable, but we also examine whether achievement goals are related to the use of different types of learning strategies. From the prior literature, there is some suggestion that mastery goals may be related to deeper learning and use of deeper learning strategies. Deeper learning strategies are those that lead to better long-term retention, but the benefits of these deeper learning strategies are not always apparent in the short-term. For example, retrieval practice is one of the most robust strategies for long-term memory but it does not always consistently lead to better immediate performance (Roediger and Karpicke, 2006; see Soderstrom and Bjork, 2015, for a review on the distinction between learning and performance). Hence, if the goal is to master learning and to be able to retain it long-term, then deeper strategies that involve more elaborative probes (e.g., actively searching memory for relevant knowledge, drawing connections between content) are important. On the other hand, if the goal is just to perform well, use of surface strategies may suffice—use of surface strategies such as rereading can result in good immediate performance. Moreover, if the goal is to perform well, then learners may be more focused on performing well along whatever criteria the teacher has set, and hence, focusing on studying whatever material the teacher has provided (Senko et al., 2013). This focus on pursuing the teacher-set learning agenda may lead students to use relatively passive strategies, such as going over course notes and readings (rereading, highlighting/underlining, summarizing). Indeed, performance goals do appear to predict use of surface strategies (Senko et al., 2011); however, the evidence for this mastery goal-deep strategy association is mixed (Nolen, 1988; Liem et al., 2008; Senko et al., 2011). However, as we elaborated above, the literature has tended to define deep as the relatively intuitive deep strategies (e.g., elaboration, self-explanation) or sometimes even low-utility strategies (e.g., summarization), and miss out of examining the empirically supported, but counterintuitive deep strategies (e.g., self-testing).
Profiles of Implicit Beliefs
While the fixed and growth intelligence beliefs tend to be conceptualized as opposite ends of a single belief spectrum, the difficulty and ease beliefs are generally considered to be distinct from each other. And although they may share similarities in motivational outcomes, psychometric analyses reveal that intelligence belief is distinct from the difficulty and ease beliefs; the correlations between these five implicit beliefs are small to moderate, indicating orthogonality (Fisher and Oyserman, 2017). However, the proliferation of implicit beliefs in the literature is reminiscent of the parable of the blind men and the elephant: each belief construct represents one part of truth and a better, more complete picture is understood when they are considered simultaneously. Whereas a typical analytic approach might involve entering each of the five implicit beliefs as predictors in a multiple regression, in the present study we take a person-centered approach and use latent profiles analysis (LPA) to examine whether meaningful profiles of the implicit beliefs emerge. The benefit of this approach is that it allows us to consider the implicit beliefs collectively rather than pitting them against each other and examining only the ‘unique variance explained’ by one belief after controlling for the others. Put another way, we were not interested in the explanatory power of any single implicit belief and instead were more interested in the collective “profiles” of implicit beliefs that are represented among students in our sample.
Research Questions
The existing literature has some notable gaps. First, most of the studies linking implicit beliefs to goal orientations have focused primarily on the growth mindset belief, rather than any other belief. The growth mindset belief may represent a very narrow slice of what beliefs influence human behavior. Moreover, there has been little research examining how implicit beliefs and values might interact to predict goal orientations. The present study represents an initial step toward exploring how domain-general and course-specific value might jointly relate to achievement goals. Hence, our first key research question in this study is: Do implicit beliefs and course-specific value relate to achievement goals, and do they interact? (RQ1) This research question is represented by the two solid arrows in Figure 1.
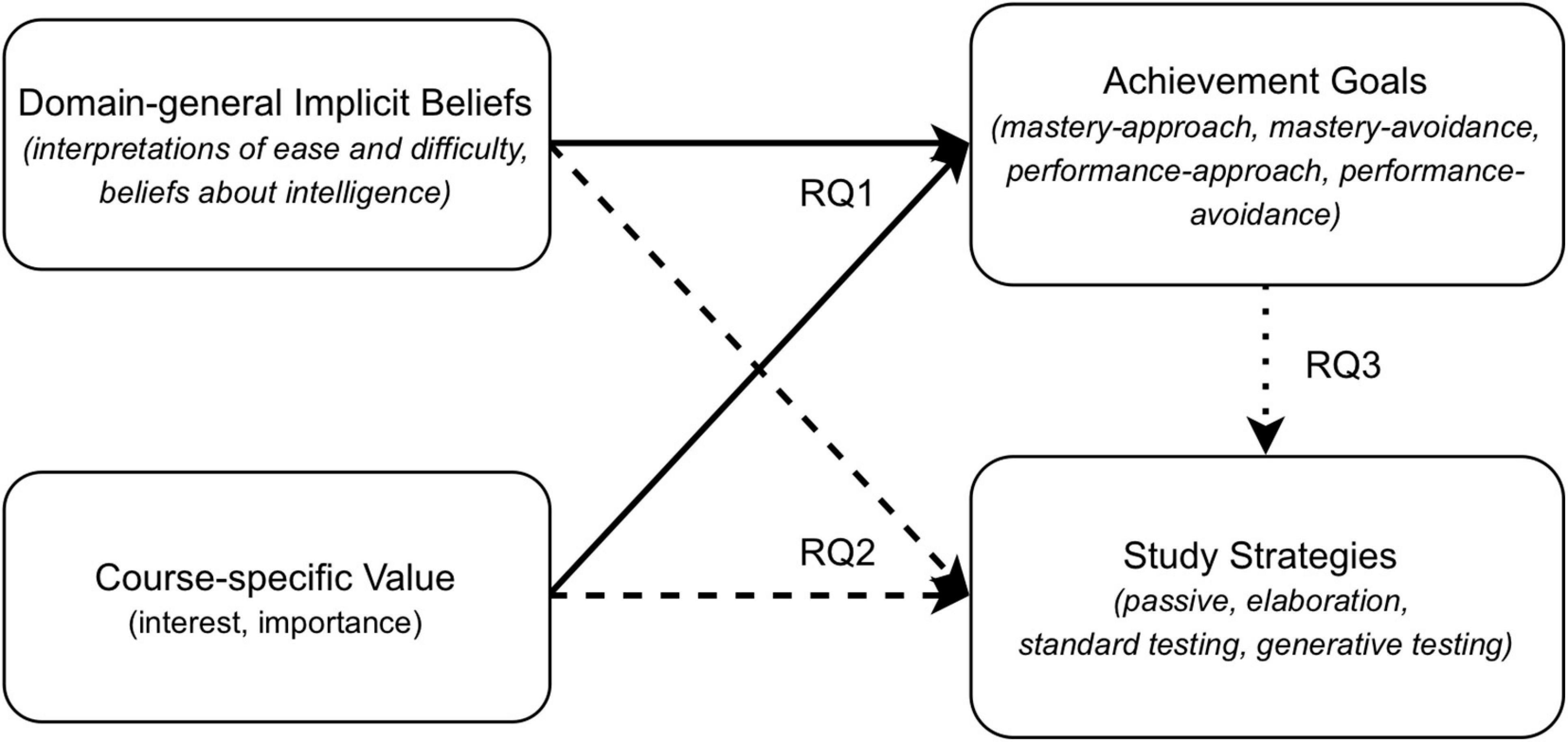
Figure 1. Diagram representing three key research questions. Research Question (RQ) 1 is represented by the solid arrows, RQ2 is represented by the dashed arrows, and RQ3 is represented by the dotted arrow.
Second, the existing literature linking implicit beliefs and value to study strategies has not been updated to include the more recent findings about effective study strategies from cognitive psychology. Our present study draws from the wealth of cognitive psychology research from the past couple of decades to examine how implicit beliefs and values relate to the strategies students engage in their studies. Hence, our second key research question is: Do implicit beliefs and course-specific values relate to study strategies, and do they interact? (RQ2) This research question is represented by the two dashed arrows in Figure 1. Finally, although we treat achievement goals as the outcome variable in RQ1, we recognize that there may also be a relationship between goals (especially mastery-approach) and study strategies, and hence our third key research question is: Do achievement goals relate to study strategies (controlling for implicit beliefs and value)? (RQ3) This research question is represented by the single dotted arrow in Figure 1.
Materials and Methods
Participants
Undergraduate students (N = 458) were recruited from the educational psychology subject pool at a large public southwestern university and compensated with partial course credit. Data from 92 students were excluded because they failed the attention check (one item inserted in the final third of the survey: “For this statement, choose ‘disagree’ to indicate that you are paying attention”), resulting in data from 366 participants (242 females; age range 18–41, mean age = 20.49; 42% White, 22% Hispanic or Latino, 22% Asian, 6% Black, 6% multiracial, 2% other; 25% STEM majors, 25% Social Science majors, 14% Humanities and Arts majors, 36% other; 10% freshman, 24% sophomore, 26% juniors, 40% seniors +). This survey was administered in the second half of the Fall semester, so students had at least 6 weeks of experience in the course that they described.
Materials
Course Value
Course-specific motivation was operationalized as interest in the course and importance of the course. Each variable was measured by a single item: “How interested or uninterested are you in this course” and “How important is this course to you?,” with the responses collected using a slide scale, where 0 = not at all interested/not at all important and 100 = very interested/extremely important.
Study Strategies
The study strategies were asked across two different pages. First, we provided a list of study strategies with brief descriptions. These strategies were largely drawn from the list of study activities reviewed by Dunlosky et al. (2013): rereading, highlighting or underlining, summarizing, elaborating, self-explaining, and varying your learning. Varying your learning was not part of the review by Dunlosky et al. (2013), but was added due to its appearance in reviews of desirably difficult learning strategies (Bjork, 1994; Yan et al., 2017). There were also a few strategies that Dunlosky et al. (2013) included in their review that we did not include: spacing (high-utility) and interleaving (moderate-utility) as these are strategies about how one distributes or sequences study, rather than what one does during a study session itself. We also did not include keyword mnemonics (low-utility) and imagery use for text learning (low-utility), as these two strategies are relatively domain-specific and not as applicable across the broad swath of college courses.
Next, we asked more specific questions about the different ways in which they could have used testing. We included this more detailed question about testing because of the multiple ways in which testing can be used to benefit learning (Roediger et al., 2011). Specifically, we asked about using testing as pre-tests, as a metacognitive check of what you already know, taking provided practice tests, self-testing, and generating test questions. For each strategy, we asked participants to indicate how often they used each study strategy for the course they had described using a 6-pt Likert scale (1 = “never/almost never” to 6 = “almost always/always”).
Achievement Goal Orientation
We measured achievement goal orientation using the questionnaire developed by Elliot and Murayama (2008), which consists of four three-item subscales: mastery-approach (α = 0.87), mastery-avoidance (α = 0.75), performance-approach (α = 0.87), and performance-avoidance (α = 0.86). The presentation order of the twelve items was randomized for each individual. The correlations between the achievement goal orientations are presented in Table 1.
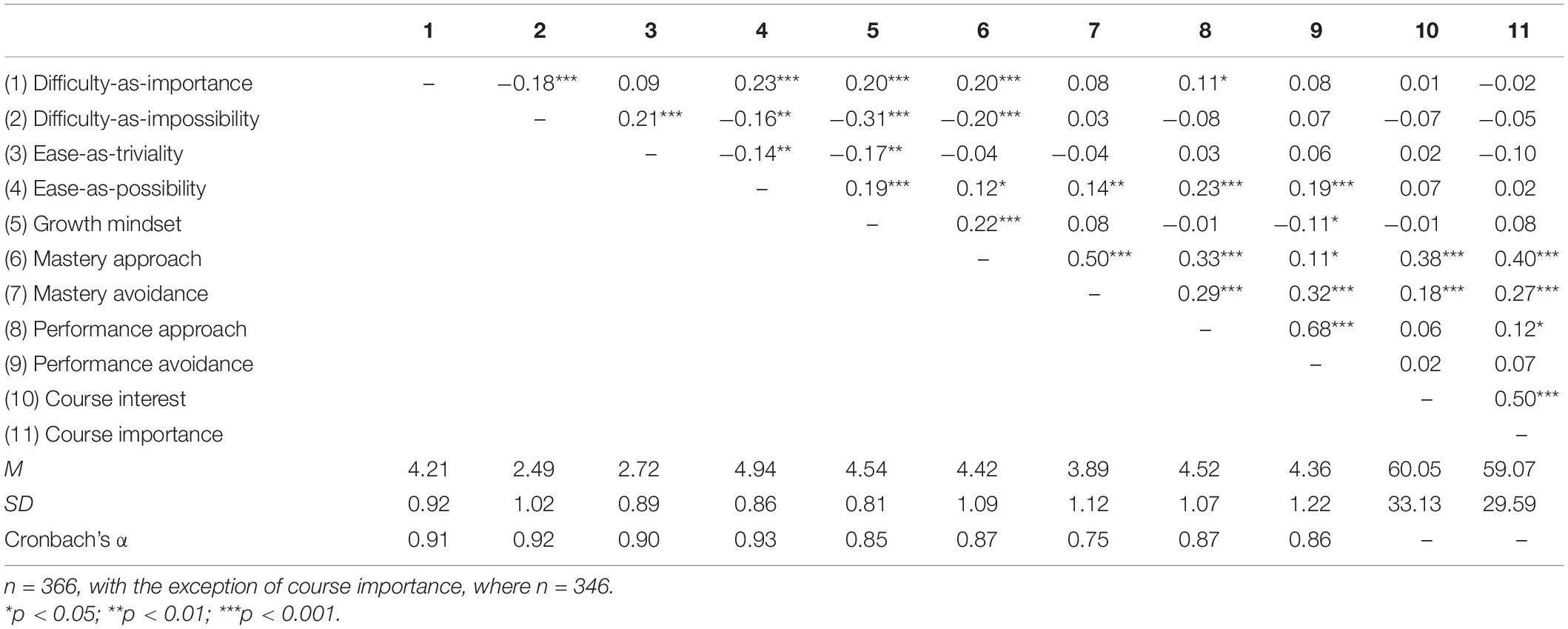
Table 1. Correlation matrix and descriptive statistics of implicit beliefs, achievement goals, and course-specific value (interest and importance).
Implicit Beliefs
We measured five subscales of implicit beliefs: difficulty-as-impossibility (α = 0.92), difficulty-as-importance (α = 0.91), ease-as-possibility (α = 0.93), ease-as-triviality (α = 0.90), and the growth mindset of intelligence (α = 0.85). The first four subscales each consisted of four items and were taken from Fisher and Oyserman (2017). The last subscale consisted of eight items (four fixed mindset, four growth mindset) and were taken from Dweck (2000). Items from each subscale were presented together, but the order of the items within a subscale was randomized, and the order of the subscales was randomized. Responses to the items within each scale were averaged to generate average subscale scores. The correlations between the implicit belief items are presented in Table 1.
Procedure
Participants were randomized to think of either a particularly interesting (n = 196) or uninteresting course (n = 170) that they were currently enrolled in. This manipulation ensured variance in the value of the courses described so that we didn’t have participants choosing only to report on their favorite or most interesting course. To ensure that they had brought to mind details of the course, participants were asked details about the course (e.g., the course title, description). We then measured value directly by asking participants to rate the interest and importance of the course (using 0–100 slider scales). Rather than using the assigned condition as a predictor variable in our analyses, we directly use the interest and importance ratings instead.
The other critical variables were collected in the following order: participants rated how frequently they used different learning strategies in the course. Next, they were asked about their achievement goals for the course and finally, they completed the five domain-general implicit beliefs subscales. To conclude the survey, participants ended by completing a demographics survey.
Results
Data Availability
The data and analysis script are available at https://osf.io/3SYKQ/.
Manipulation Check
First, we checked that our manipulation affected the interest ratings of the courses that participants described. Indeed, we found that those randomized to think of an interesting course (M = 78.27, SD = 22.72) rated their course as being significantly more interesting than those randomized to think of an uninteresting course (M = 39.04, SD = 30.77), Welch’s t(307.10) = 13.70, p < 0.001. Those randomized to think of an interesting course were also more likely to rate their course as being more important (M = 67.55, SD = 25.69) than those randomized to think of an uninteresting course (M = 49.34, SD = 30.83), Welch’s t(312.60) = 5.92, p < 0.001. Interest and importance were positively correlated, r = 0.50, p < 0.001, but not redundant.
Are There Meaningful Profiles of Implicit Beliefs?
The descriptive statistics and the zero-order correlations between the five implicit belief scales is presented in Table 1. As can be seen from the table, all of the correlations are small to moderate, ranging from |r| = 0.09 to 0.31, indicating orthogonality. That is, the implicit beliefs cannot be considered redundant and cannot be simply combined to form a single scale. Hence, in the present paper, we take a person-centered approach rather than an item-centered one: to explore whether there were meaningful profiles of beliefs, latent profile analysis (LPA) was conducted using the tidyLPA package (Rosenberg et al., 2018) in R.
Latent Profile Analysis Model Selection
Latent profile analysis is a method that identifies clusters or profiles of individuals based on responses to a series of indicators (here, we are using implicit beliefs). In standard LPA, two sets of parameters are estimated: the number of profiles in the population, and the means (and variances and covariances) within each profile. The process is a data-driven one. We first explored one to nine latent profiles of implicit beliefs and specified the variance-covariance matrices across profiles to have: (1) equal variance, covariance fixed to zero (EV/FC); (2) varying variance, covariance fixed to zero (VV/FC); (3) equal variance, equal covariance (EV/EC); (4) varying variance, varying covariance (VV/VC), hence there were 36 potential solutions. Solutions with more than three profiles were unable to be estimated using the VV/FC and VV/VC parameter specifications (see Supplementary Table 1 for the fit indices–AIC, AWE, BIC, CLC, KIC–for each solution).
To evaluate the various fit indices and to select the best fitting solution, we used three different approaches to converge upon a solution. First, we used an Analytic Hierarchy Process (Akogul and Erisoglu, 2017) that takes into account Akaike’s Information Criterion (AIC; Akaike, 1973, 1987), Approximate Weight of Evidence (AWE; Banfield and Raftery, 1993), Bayesian Information Criterion (BIC; Schwarz, 1978), Classification Likelihood Criterion (CLC; Biernacki and Govaert, 1997), and Kullback Information Criterion (KIC; Cavanaugh, 1999). The Analytic Hierarchy Process compares a weighted composite of the information criteria for each alternative solution. This analysis is part of the tidyLPA package in R. Both the Analytic Hierarchy Process and BIC suggested that the three-profile solution with varying variance across profiles and covariance fixed to zero (VV/FC) was the best fitting solution.
The second approach we took was to compare a series of solutions with likelihood-based tests to examine the number of profiles and parameter specifications. Lo-Mendell-Rubin adjusted likelihood ratio tests (adjusted LMR; Lo et al., 2001) were used to compare solutions with different numbers of profiles: the 2-, 3-, and 4-profile solutions with VV/FC parameter specification. The three-profile solution significantly improved model fit from the two-profile solution: adjusted LMR(11) = 86.40, p < 0.001. The four-profile solution was unable to be estimated, so no adjusted LMR test was conducted. χ2 difference tests were used to compare the three nested three-profile solutions with EV/FC, VV/FV, and VV/VC parameter specifications. The VV/FC model significantly improved model fit from the EV/FC model: χ2(10) = 123.40, p < 0.001. The VV/VC model also significantly improved model fit from the VV/FC solution: χ2(30) = 76.50, p < 0.001. However, as both the Analytic Hierarchy Process and BIC favored the VV/FC model over the VV/VC model, we choose the former, more parsimonious set of parameter specification.
The Analytic Hierarchy Process and likelihood-based tests all rely on fit indices, but it is also important to evaluate solutions on classification diagnostics and interpretability. Hence, the third approach we took was to visually inspect the Entropy, mean posterior probabilities, and profile sizes for each solution (see Supplementary Table 1). What we found was that the mean posterior probabilities across the models were all very similar. The three-profile VV/FC solution and 2- and 3-profile VV/VC solutions all showed a reasonable spread of participants across profiles; the VV/VC solutions, however, were less parsimonious (more parameters estimated) than the VV/FC solution. Although some of the other solutions may have had higher Entropy scores, they also had much more uneven profiles, or profiles with very few participants.
LPA Final Three-Profile Model
To sum, we selected the three-profile solution with VV/FC parameter specification. Table 2 shows the classification table for this solution, with mean posterior probabilities of participants assigned to a given profile. Mean posterior probabilities of participants assigned to a profile other than their designated profile ranged from 0.04 to 0.12, indicating minimal overlap between profiles.
The mean endorsement of each implicit belief scale by profile is presented in Figure 2. One profile (n = 94) was those who were relatively low in their endorsement of the growth mindset, difficulty-as-importance and ease-as-possibility, and relatively high in difficulty-as-impossibility and ease-as-triviality. These responses reflect a belief that one need not engage with either difficult or easy tasks. We refer to this group as motivation-undermining beliefs. Another profile (n = 126) consisted of participants who were relatively high in their endorsement of the growth mindset, difficulty-as-importance and ease-as-possibility, and relatively low in difficulty-as-impossibility and ease-as-triviality. These responses reflect a belief that both experience of ease and difficulty should be motivating. We refer to this profile as motivation-increasing beliefs. The third group (n = 146) fell in between the other two groups. We refer to this profile as neutral beliefs. In all subsequent analyses, we used implicit belief profiles as a categorical variable with the motivation-undermining profile as the reference profile.
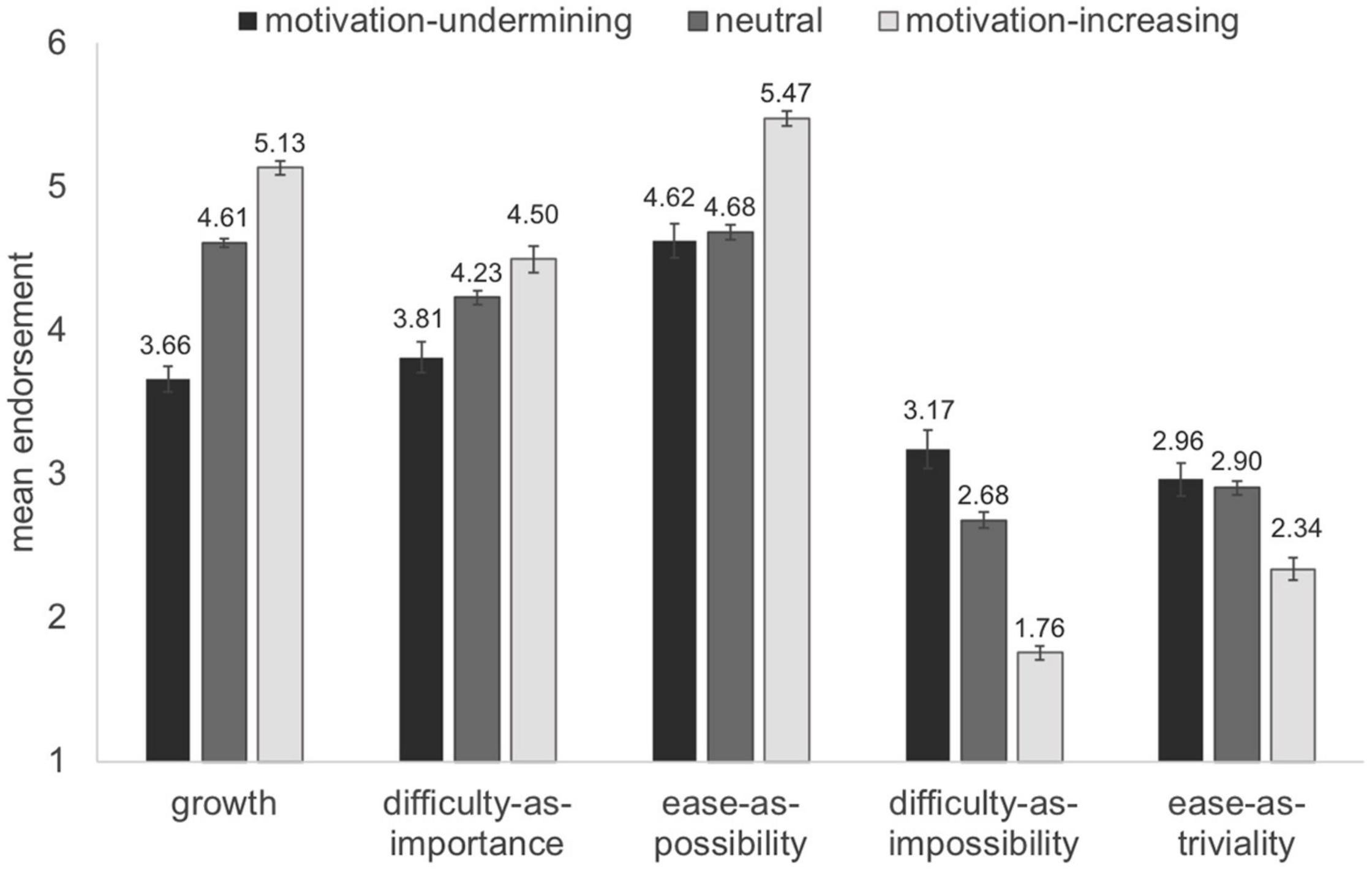
Figure 2. Three latent profiles of implicit beliefs. Error bars represent standard error of the mean.
RQ 1: Are Implicit Beliefs and Value Related to Achievement Goals?
What predicts achievement goal orientations? The descriptive statistics for the achievement goals and the zero-order correlations with other variables are presented in Table 1. As these zero-order correlations show, all four goal orientations were positively related to each other, but there are different patterns of relationship with the implicit beliefs and course-specific value constructs.
To answer our critical question, however, we ran hierarchical linear regressions for each achievement goal orientation: At step 1, we examine whether belief profile predicts endorsement of each goal orientation, and at step 2, we examine whether the relationships between implicit belief profile and achievement goals are moderated by course importance or interest1. The reference profile was the motivation-undermining profile (and this the case in all reported regression analyses).
The regression summaries are presented in Table 3. In general, those with motivation-increasing implicit beliefs were positively related to mastery-approach goals and this held even after controlling for interest and importance. Interest also mattered for mastery-approach goals—the more interested a participant was in their course, the higher their mastery-approach goals. Importance, however, was not related to mastery-approach, and no variable was significantly related to mastery-avoidance goals.
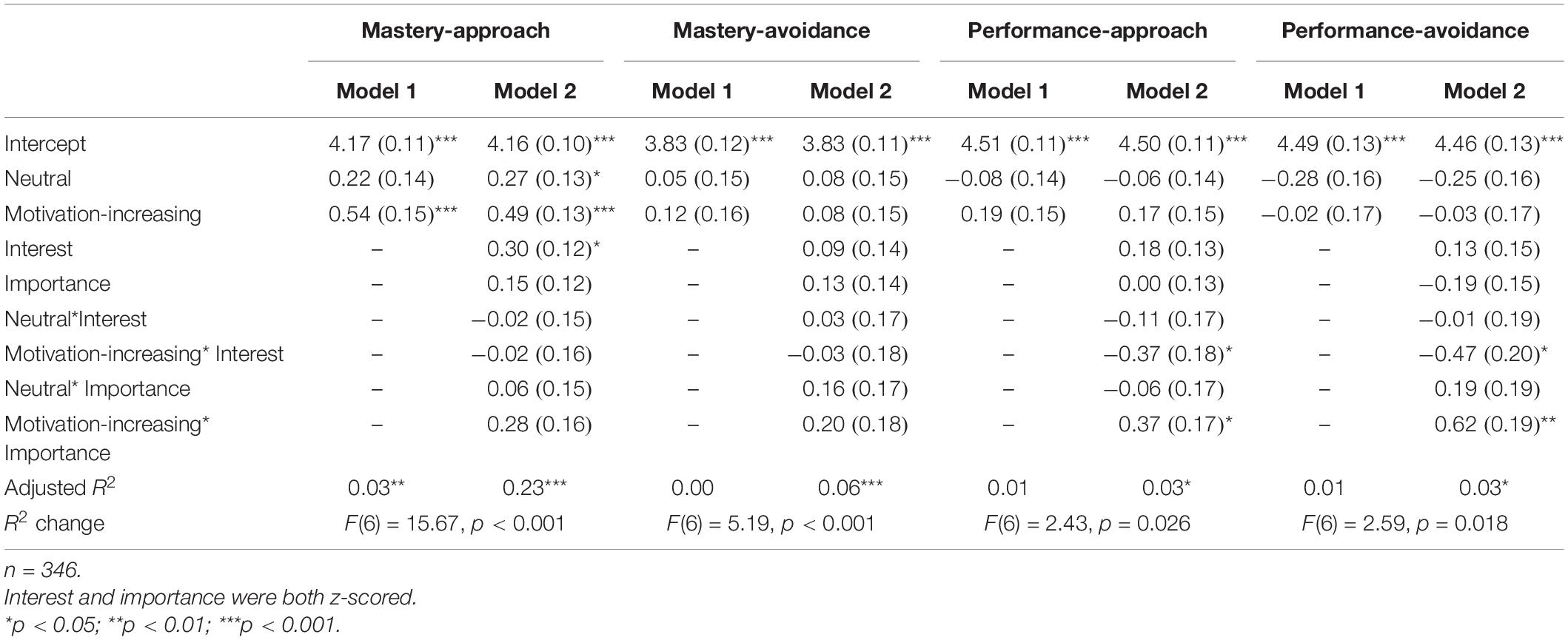
Table 3. Summary of regression coefficients, predicting achievement goals from implicit belief profiles, course interest, and course importance.
There were few direct relationships with the performance goals. In general, implicit belief profiles were not related to performance goals (with the exception of a negative relationship between neutral implicit beliefs and performance-avoidance, which was no longer significant once interest and importance were controlled for). There were however, significant interactions between profile and importance and between profile and interest. These interactions are depicted in Figure 3. Two patterns emerged: for those in the motivation-increasing beliefs group, course importance is positively related to performance-approach and performance-avoidance goals, and course interest is negatively related to performance-approach and performance-avoidance goals. For those in the other two groups, their performance goals were not sensitive to course importance and course interest, or in some cases, the pattern was even reversed.
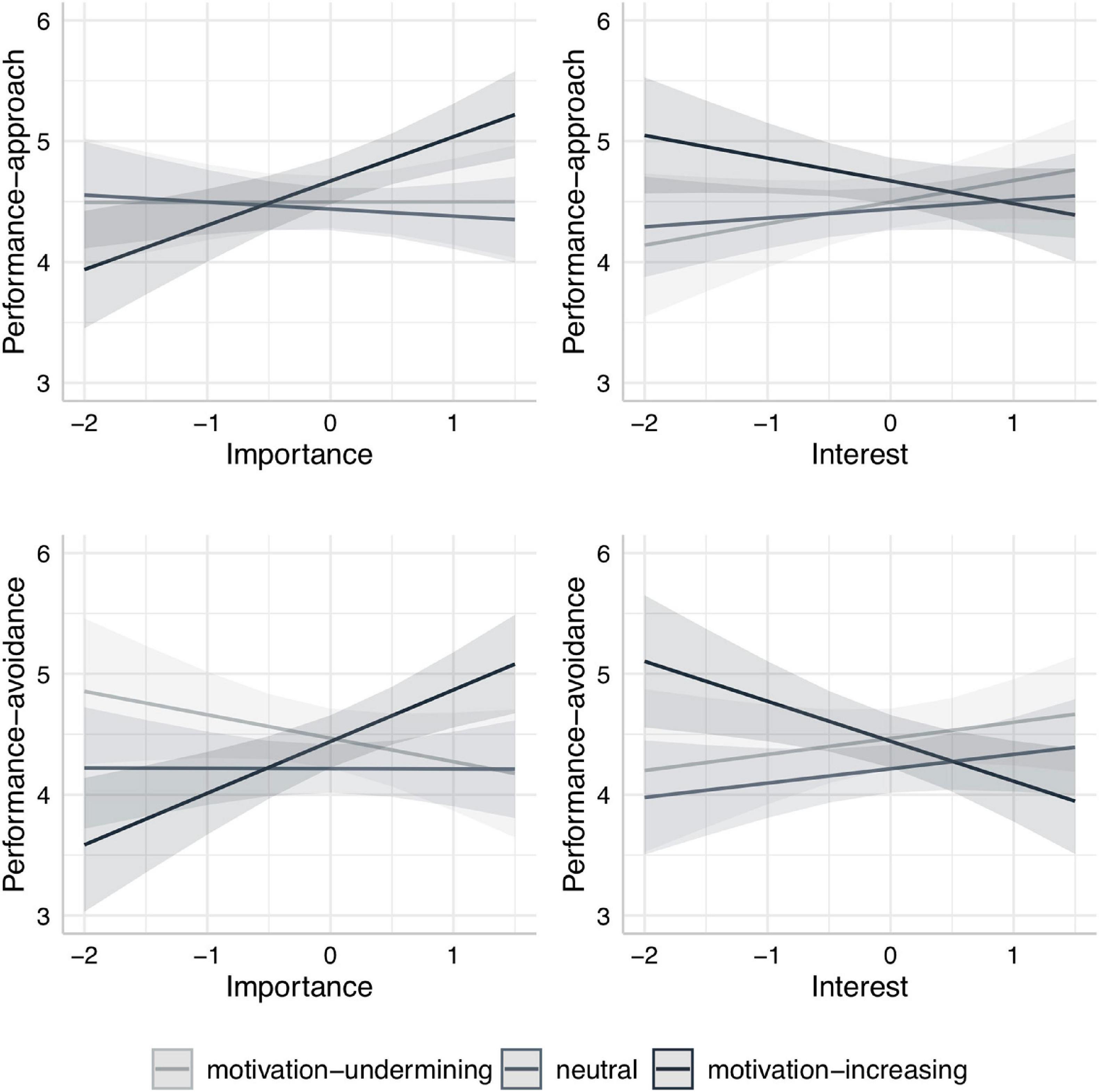
Figure 3. Implicit belief profiles interact with importance and interest to predict performance-approach and performance-avoidance goals. Importance and interest values are standardized; performance-approach and performance-avoidance values have been left in their original units.
RQ 2: Are Implicit Beliefs and Value Related to Study Strategies?
Four Strategy Subscales: Passive, Elaborative, Standard-Testing, and Generative-Testing
The description of each study strategy as it was presented to the participants and then average rated frequency of use are presented in Table 4. In general, there was a good amount of variation in the strategies that participants reported using. Given the large number of study strategies, we first used an exploratory factor analysis approach to examine whether the strategies could be grouped into factors. A parallel analysis and examination of a scree plot suggested a four-factor solution. We chose maximum likelihood with an oblique rotation as our exploratory factor analytic model. The oblique rotation allowed for correlations among factors. This solution showed excellent fit, TLI = 0.993, RMSEA = 0.018 (90% CI: 0, 0.047). The rotated factors and factor loadings are presented in Table 5. The factor correlations are presented in the Supplementary Table 2; the correlations were all small to moderate (r = 0.15 to r = 0.48). For each factor, we averaged the use frequency of the relevant strategies to create four strategy subscale scores. The average subscale scores are found at the bottom of Table 5.
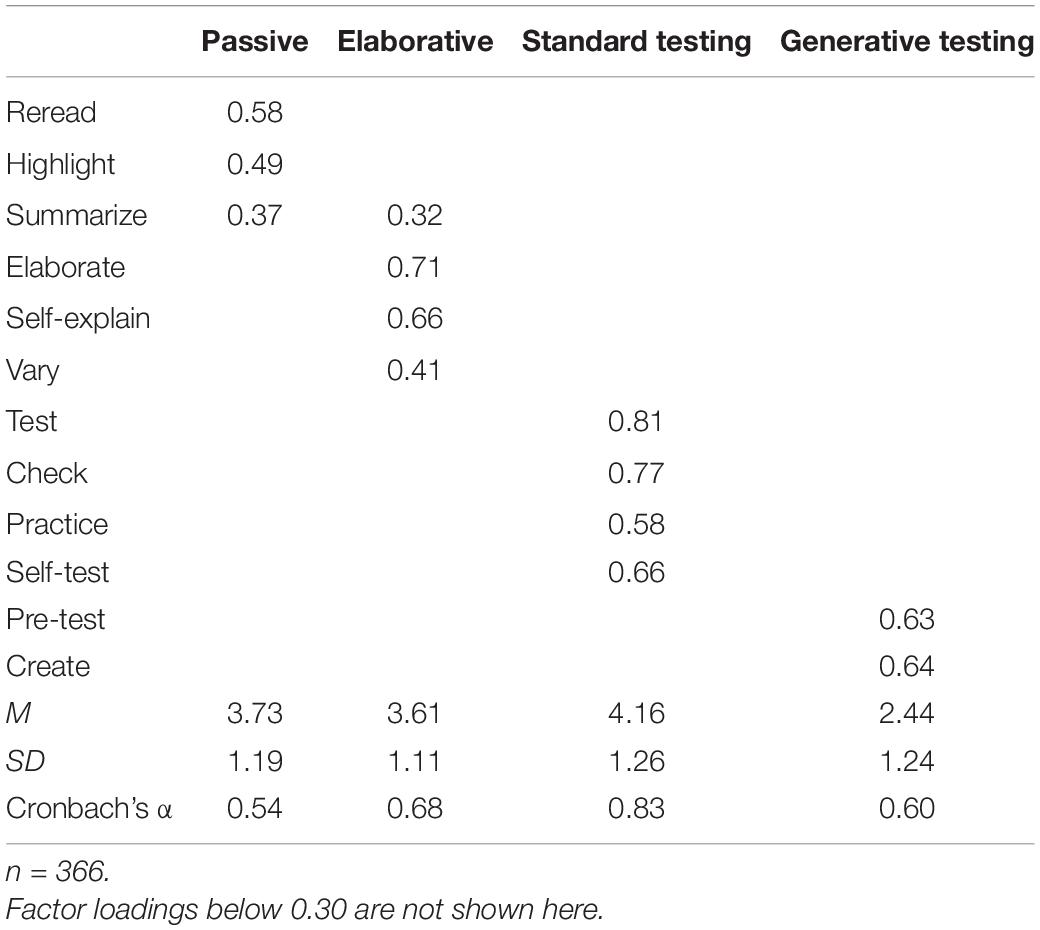
Table 5. Factor loadings of the study strategies (Top) and descriptive statistics for the four study strategy factors (Bottom).
The passive strategies subscale consisted of the strategies that were rated by Dunlosky et al. (2013) as low-utility: highlighting, summarizing, and rereading. The elaborative strategies subscale consisted of the two moderate-utility strategies—elaboration and self-explanation—as well as variation. The remaining two subscales reflect different uses of testing: one reflects the most common uses of testing, and included our generic item about testing (test), as well as the more specific forms about self-testing (self-test), taking practice-tests (practice), and using tests to check what one knows (check); the other reflects more generative uses of testing–using them as pre-tests (pre-test), and creating your own test questions (create). The standard testing subscale had the highest frequency of use ratings whereas the generative testing subscale had the lowest. The average frequency of use ratings for the passive and elaborative strategies subscales were just above the midpoint of the 6 point ratings scale, representing moderate usage.
What Predicts Strategy Use?
More importantly, we asked whether students in the different implicit belief profile groups used different types of strategies, and whether this relationship is moderated by course interest and importance. Again, we examine these patterns for each strategy using hierarchical regression models. At step 1, we predicted the use of each strategy subscale from belief profile. At step 2, we added in two interactions, examining how course interest and course importance each interacted with belief profile. At step 1, we did not find that the implicit belief profiles differed on any strategy subscale. At step 2, adding course interest and course importance to the model did not explain any additional variance for use of the standard testing strategies or for the generative testing strategies. The regression summary statistics for these two strategy subscales are found in the Supplementary Table 3.
Including the interactions with course interest and importance did, however, explain significantly more variance for the elaborative strategies and for passive strategies. A summary of the regression statistics is presented in Table 6. The results show that importance mattered—the more important the student rated the course, the more likely they were to engage in elaborative and passive strategies. There was an interaction between profile and importance for the use of passive strategies: Whereas importance was positively related to use of passive strategies for both the motivation-increasing and motivation-undermining beliefs groups, it was unrelated to passive strategy use for the neutral beliefs group.
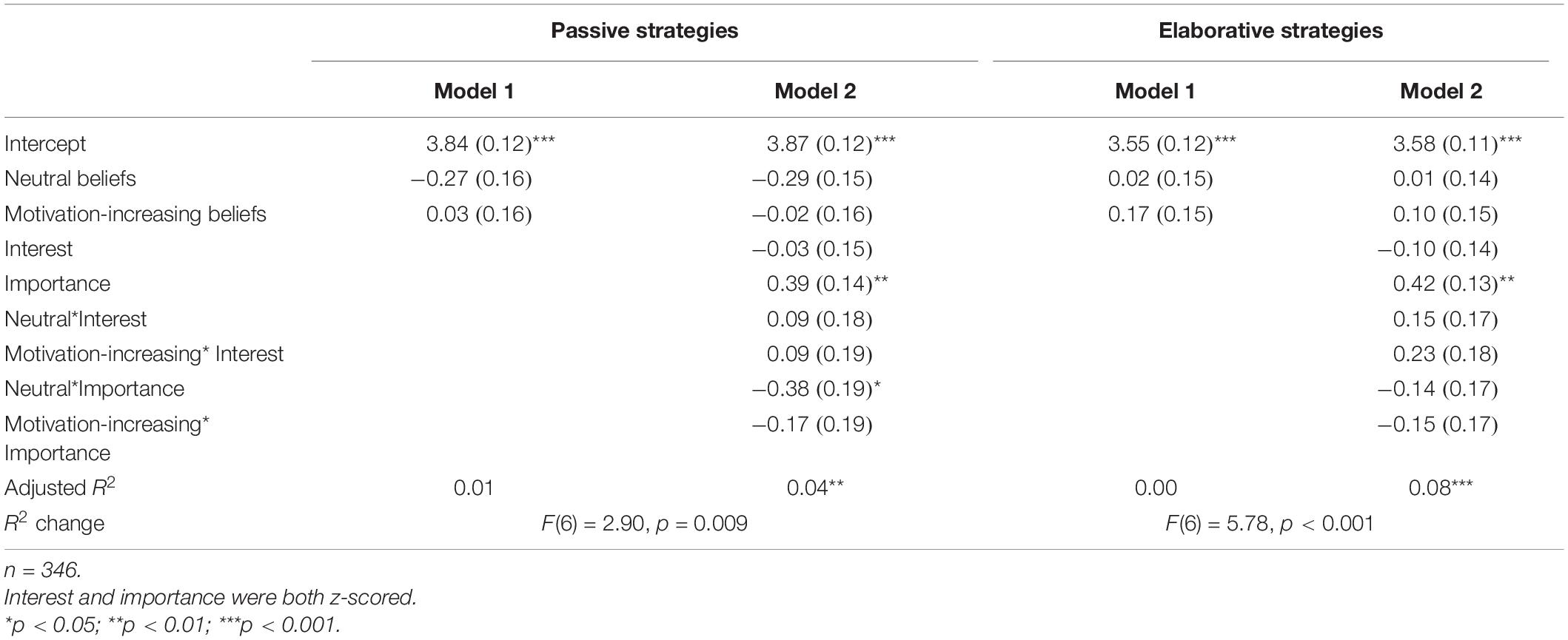
Table 6. Summary of regression coefficients, predicting study strategy use from implicit belief profiles, course interest, and course importance.
RQ 3: Are Achievement Goals Related to Study Strategies?
Finally, we asked whether the three types of achievement goals were related to study strategy use, controlling for implicit beliefs, course importance, and course value. We conducted four multiple regression analyses, one for each strategy subscale. The results are summarized in Table 7. Mastery-approach goals were positively related to the use of elaborative strategies and both types of testing strategies; they were not related to use of passive strategies. Mastery-avoidance goals were negatively related to the use of elaborative strategies, but did not relate to the other strategies. Neither of the performance goals were related to the use of any strategies.
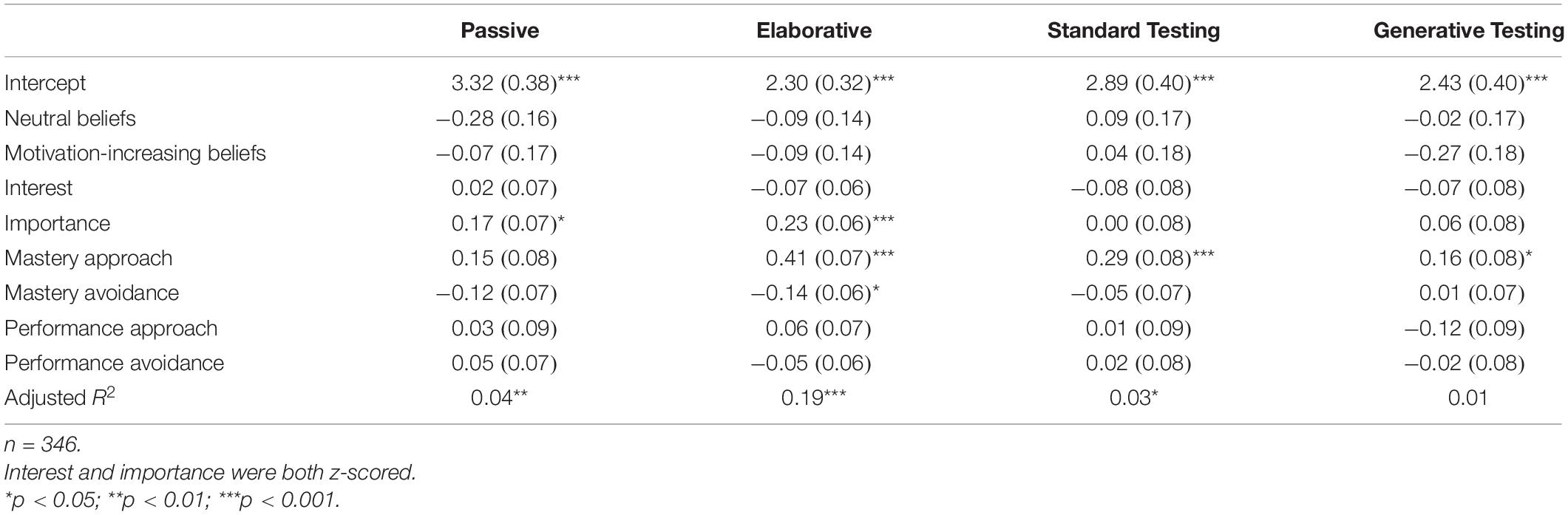
Table 7. Summary of regression coefficients, predicting study strategy use from achievement goals, controlling for implicit belief profiles, course interest, and course importance.
Discussion
In the present study we examined how motivation-related implicit beliefs and course value (interest and importance) relate not to quantity of study (e.g., study effort, persistence), but to the quality of study—the achievement goals that students hold and the study strategies that they engage. We selected a set of implicit beliefs that are likely related to academic experiences, and which have been validated in the motivational literature: difficulty-as-importance, difficulty-as-impossibility, ease-as-possibility, ease-as-triviality (Fisher and Oyserman, 2017), and the growth intelligence mindset (Dweck and Yeager, 2019). Difficulty-as-importance, ease-as-possibility, and the growth intelligence mindset are motivation-increasing—they compel individuals to engage more with a task. Ease-as-triviality and difficulty-as-impossibility as motivation-undermining—they compel individuals to give up and desist. Rather than examining each individual implicit belief separately, we used LPA to reveal profiles of these beliefs, finding three distinct profiles–one that was motivation-increasing, one that was motivation-undermining, and one that fell somewhat in between the two, which we refer to as ‘neutral.’
We examined the quality of student efforts in two ways. First, we looked at achievement goal orientations that students held for a particular course. Second, we looked at the specific study strategies that students reported using for that same course. Although both types of variables relate to the quality of student effort, we found that they were predicted by different variables.
What Factors Are Associated With Achievement Goals?
Previous literature finds that the motivation-undermining difficulty-as-impossibility, and ease-as-triviality are positively related to avoidance goals and that growth mindset is negatively related to performance-avoidance goals (Bråten and Strømsø, 2004; Chen and Pajares, 2010; Fisher and Oyserman, 2017). In our study, we did not find that the implicit belief profiles differed. In part, this might be due to the fact that the LPA approach reduces power by categorizing the beliefs. However, we did find interesting interactions: Interest and importance were related to performance goals, but only for those in the motivation-increasing beliefs group. Moreover, whereas the existing literature tends to find similar patterns for interest and utility-value, we found that interest and importance were related to performance in opposite directions: The more important a course was (controlling for interest), the more those in the motivation-increasing beliefs group were performance-oriented. The more interesting a course was (controlling for importance), the less those in the motivation-increasing beliefs group were performance-oriented. One interpretation of this distinction is that importance in our study may reflect the importance of getting a good grade (an extrinsic motivation, aka performance-goal) whereas interest reflects an intrinsic motivation where performance is less relevant; the motivation-increasing beliefs hence may simply augment these goal orientations.
Prior literature also finds that difficulty-as-importance and ease-as-possibility are positively related to approach goals, difficulty-as-impossibility as negatively related to approach goals, and that growth mindset and value are positively related to mastery-approach (Bråten and Strømsø, 2004; Hulleman et al., 2008; Renninger et al., 2008; Chen and Pajares, 2010; Fisher and Oyserman, 2017). In line with these past findings, we found that both interest and motivation-increasing beliefs were related to having higher mastery-approach goals. Although the zero-order correlations showed that importance was related to mastery-approach goals, this relationship disappeared once interest was controlled for. One interpretation is that participants interpreted the meaning of “importance” as being about performance. Alternatively, it may point to the possibility that the positive effects of increasing perceived importance (e.g., utility-value) are mediated by increased interest.
What Factors Are Associated With the Use of Effective Study Strategies?
Previous studies that suggest implicit beliefs and interest are related to use of deeper, more effective study strategies (Schiefele, 1991; Lipstein and Renninger, 2007; Yan et al., 2014a), but our results did not support predictions based on these prior findings. Instead, we did not find that implicit belief profiles or interest to be related to the use of any of the study strategies subscales.
Only achievement goals and course importance were related to self-reported strategy use. Importance was related only to use of passive and elaborative strategies, but not to either of the self-testing strategy subscales. These results highlight the limitations of increasing value (though both importance and interest were positively correlated with mastery-approach). Above and beyond the other goal orientations and value, mastery-approach goals were uniquely related to increased use of all three of the effective strategies subscales (elaborative strategies, standard testing, and generative testing) and was not related to use of the less effective, passive study strategies. In other words, it uniquely promoted effective strategies, rather than simply promoting all strategies. In fact, mastery-approach was the only goal orientation that was related to the two self-testing strategies—study activities that are directed at retention and maintenance of knowledge. In contrast, mastery-avoidance was related only to reduced use of elaborative strategies. One speculation for this finding is that those trying to avoid revealing to themselves that they have not mastered the knowledge might be less likely attempt self-explanations. One could argue that self-testing should also reveal gaps in one’s own knowledge, but that is only the case if the questions test deeper understanding rather than surface-level rote memorization (e.g., concept-definition multiple-choice questions). Future research could explore how achievement goals are related to the types of test questions that learners seek out and engage with.
In contrast to the prior literature that has linked performance goals to increased use of passive strategies (and in some cases, linked performance-approach with deep strategies, Liem et al., 2008; Vrugt and Oort, 2008), we did not find them to be related to the use of any strategies. One difference between our present study and prior studies may be the definition of passive strategies. Often, the rehearsal subscale of the MSLQ, which focuses on rote repetition (e.g., When I study for this class, I practice saying the material to myself over and over) is used to represent the least effective strategies for learning. Our passive strategies subscale includes popular strategies that might appear to be reasonable but have been empirically shown to be relatively ineffective (rereading, highlighting/underlining, summarization). It would be important for future studies to replicate these findings, but our results suggest that this more nuanced classification of strategies is meaningful for understanding the behavioral correlates of different achievement goals.
Implications for Interventions
These findings have nuanced implications for intervention. This study was exploratory and should not be taken as conclusive, but we describe some of the potential implications that future research might consider.
One implication of the findings is that interventions should target ways of increasing students’ mastery goal orientations, given that mastery-approach goals were uniquely related to use of more effective study strategies. Other research has already shown that mastery-approach goals are associated with better learning outcomes, and the present study contributes that one way in which a mastery goal orientation might lead to better learning outcomes is by shifting students to using more effective study strategies. How should mastery-approach goals be promoted? Our findings suggest that mastery goals may be promoted by both increasing students’ intrinsic interest as well as their motivation-increasing implicit beliefs—the results suggest that interventions that contain a combination of these two aspects may be more effective than either one alone.
Many existing interventions focus on the motivation-increasing implicit beliefs (Oyserman et al., 2006, 2018; Blackwell et al., 2007; Oyserman, 2015; Yeager et al., 2016, 2019). Often the interventions are focused on academic persistence or achievement rather than on quality of engagement. While we did find that motivation-increasing implicit beliefs were related to mastery-approach goal orientation, the lack of a direct effect between these beliefs and use of effective study strategies suggest that attending only to implicit beliefs may not be sufficient to truly increase the quality of study.
Finally, our results also suggest that increasing perceptions of course importance may not be particularly effective, especially if the manipulation of importance does not also increase interest. Controlling for interest, importance was related only to increased performance goals—but this was true only for those in the motivation-increasing beliefs group—and it was not related to mastery goals. Importance was also not consistently related to the use of effective strategies—rather, it was related to increased use of passive strategies, and only one of the three effective strategy subscales. In other words, increasing importance might lead learners to study harder, but not necessarily smarter.
Limitations and Future Directions
A standard word of caution is that this study was a purely correlational, cross-sectional one, which means that the relationships reported in the present paper should not be interpreted as causal. Rather, the analyses conducted here are exploratory and the findings are meant to indicate potentially fruitful new avenues of research. Both longitudinal and experimental follow-up studies may illuminate new insights. For example, it is likely that students’ achievement goals fluctuate across a semester with the ebb and flow of course demands (e.g., midterm examinations, final examinations; Corker et al., 2013; Lee et al., 2017). Rather than only looking at mastery and performance goals in a snapshot of time, it may be interesting to examine how the ebb and flow of goals itself is related to implicit beliefs, interest and importance. One could hypothesize, for example, those with motivation-increasing beliefs might be better able to maintain higher mastery goals all throughout a semester, while those with neutral or motivation-undermining belief might find their mastery goals dropping significantly around midterms or finals.
In the absence of intervention, our results show that the five different implicit beliefs that were measured were only weakly to moderately related to each other. One strength of our LPA approach helped to coalesce these into meaningful profiles, but a weakness of the approach is that it reduces statistical power by categorizing participants into one of three profiles. The LPA approach is also data-driven, which means that future datasets might yield different looking profiles. We view this as a potentially interesting and informative feature of the approach. For example, it may help illuminate what aspects interventions actually affect by examining how the emergent profiles are similar or different, either before and after an intervention, or between an intervention and control group.
Another limitation of the present study is that our course-specific motivation constructs of interest and importance were each only measured with a single item. Though the items themselves are face-valid, the educational literature on value has identified distinctions between different types of interest (e.g., situational and individual sustained interest; Schiefele, 1991; Renninger and Hidi, 2002; Hidi, 2006) and between different types of importance (e.g., utility value, attainment value; Eccles et al., 1983; Wigfield and Eccles, 2000). Our measurement of interest and importance leave it ambiguous as to what particular aspect participants are thinking about.
Conclusion
Nevertheless, the present study contributes to the educational psychology literature in three key ways. First, we provide an example of how various motivation-related implicit beliefs may be considered simultaneously. We take the view that there are many relevant different implicit beliefs that individuals may hold in mind and draw upon. Considering implicit belief profiles, rather than individual beliefs, may hence be a productive way forward in integrating multiple constructs in the existing literature and for identifying learners for different types of interventions. Second, we explore the integration of domain-general and course-specific motivational constructs as they are brought to bear on student engagement. Third, we integrate the social and educational psychology research on student motivation with the cognitive psychology research on effective study strategies, showing both that there are meaningful relationships between motivation and strategy use, but also that the relationship may not be straightforward and that there are still gaps to be filled.
Data Availability Statement
The dataset and analysis script for this study can be found accessed at: https://osf.io/3SYKQ.
Ethics Statement
The studies involving human participants were reviewed and approved by Institutional Review Board (IRB) at the University of Texas at Austin. Written informed consent for participation was not required for this study in accordance with the national legislation and the institutional requirements.
Author Contributions
VY and LW designed and conducted the study together and they analyzed and interpreted the data together. VY primarily drafted the manuscript with assistance from LW. Both authors contributed to the article and approved the submitted version.
Conflict of Interest
The authors declare that the research was conducted in the absence of any commercial or financial relationships that could be construed as a potential conflict of interest.
Supplementary Material
The Supplementary Material for this article can be found online at: https://www.frontiersin.org/articles/10.3389/feduc.2021.643421/full#supplementary-material
Footnote
- ^ Twenty participants did not complete the course importance rating; these twenty participants were hence removed from both steps of the hierarchical linear regression (to enable model comparison), as well as from all subsequent analyses. There were no other missing data.
References
Akaike, H. (1973). “Information theory and an extension of the maximum likelihood principle,” in Second International Symposium on Information Theory, eds B. N. Petrov and B. F. Csaki (Budapest: Academiai Kiado), 267–281.
Akogul, S., and Erisoglu, M. (2017). An approach for determining the number of clusters in a model-based cluster analysis. Entropy 19:452. doi: 10.3390/e19090452
Ames, C., and Archer, J. (1988). Achievement goals in the classroom: students’ learning strategies and motivation processes. J. Educ. Psychol. 80, 260–267. doi: 10.1037/0022-0663.80.3.260
Anderman, E. M., Griesinger, T., and Westerfield, G. (1998). Motivation and cheating during early adolescence. J. Educ. Psychol. 90:84. doi: 10.1037/0022-0663.90.1.84
Banfield, J. D., and Raftery, A. E. (1993). Model-based gaussian and non-gaussian clustering. Biometrics 49, 803–821. doi: 10.2307/2532201
Biernacki, C., and Govaert, G. (1997). Using the classification likelihood to choose the number of clusters. Comput. Sci. Stat. 29, 451–457.
Biggs, J. B. (1985). The role of metalearning in study processes. Br. J. Educ. Psychol. 55, 185–212. doi: 10.1111/j.2044-8279.1985.tb02625.x
Bjork, R. A. (1975). “Retrieval as a memory modifier: an interpretation of negative recency and related phenomena,” in Information Processing and Cognition: the Loyola Symposium, ed. R. L. Solso (Mahwah, NJ: Lawrence Erlbaum), 123–144.
Bjork, R. A. (1994). “Memory and metamemory considerations in the training of human beings,” in Metacognition: Knowing about Knowing, eds J. Metcalfe and A. P. Shimamura (Cambridge, MA: MIT Press), 185–206.
Bjork, R. A., Dunlosky, J., and Kornell, N. (2013). Self-regulated learning: beliefs, techniques, and illusions. Ann. Rev. Psychol. 64, 417–444. doi: 10.1146/annurev-psych-113011-143823
Blackwell, L. S., Trzesniewski, K. H., and Dweck, C. S. (2007). Implicit theories of intelligence predict achievement across an adolescent transition: a longitudinal study and an intervention. Child Dev. 78, 246–263. doi: 10.1111/j.1467-8624.2007.00995.x
Bråten, I., and Strømsø, H. I. (2004). Epistemological beliefs and implicit theories of intelligence as predictors of achievement goals. Contemp. Educ. Psychol. 29, 371–388. doi: 10.1016/j.cedpsych.2003.10.001
Cavanaugh, J. E. (1999). A large-sample model selection criterion based on Kullback’s symmetric divergence. Stat. Probab. Lett. 42, 333–343. doi: 10.1016/S0167-7152(98)00200-4
Chen, J. A., and Pajares, F. (2010). Implicit theories of ability of Grade 6 science students: relation to epistemological beliefs and academic motivation and achievement in science. Contemp. Educ. Psychol. 35, 75–87. doi: 10.1016/j.cedpsych.2009.10.003
Corker, K. S., Donnellan, M. B., and Bowles, R. P. (2013). The development of achievement goals throughout college: modeling stability and change. Pers. Soc. Psychol. Bull. 39, 1404–1417. doi: 10.1177/0146167213494243
Dunlosky, J., Rawson, K. A., Marsh, E. J., Nathan, M. J., and Willingham, D. T. (2013). Improving students’ learning with effective learning techniques: promising directions from cognitive and educational psychology. Psychol. Sci. Public Interest 14, 4–58. doi: 10.1177/1529100612453266
Dweck, C. (2000). Self-Theories: their Role in Motivation, Personality, and Development, Edn 1. Hove: Psychology Press.
Dweck, C. S., and Leggett, E. L. (1988). A social-cognitive approach to motivation and personality. Psychol. Rev. 95, 256–273. doi: 10.1037/0033-295x.95.2.256
Dweck, C. S., and Yeager, D. S. (2019). Mindsets: a view from two eras. Perspect. Psychol. Sci. 14, 481–496. doi: 10.1177/1745691618804166
Eccles, J. S., Adler, T. F., Futterman, R., Goff, S. B., Kaczala, C. M., Meece, J. L., et al. (1983). “Expectancies, values and academic behaviors,” in Achievement and Achievement Motives, ed. J. T. Spence (New York, NY: W. H. Freeman), 75–146.
Elliot, A. J. (1999). Approach and avoidance motivation and achievement goals. Educ. Psychol. 34, 169–189. doi: 10.1207/s15326985ep3403_3
Elliot, A. J., and Murayama, K. (2008). On the measurement of achievement goals: critique, illustration, and application. Journal. Educ. Psychol. 100, 613–628. doi: 10.1037/0022-0663.100.3.613
Fisher, O., and Oyserman, D. (2017). Assessing interpretations of experienced ease and difficulty as motivational constructs. Motiv. Sci. 3, 133–163. doi: 10.1037/mot0000055
Grant, H., and Dweck, C. S. (2003). Clarifying achievement goals and their impact. Journal. Pers. Soc. Psychol. 85:541. doi: 10.1037/0022-3514.85.3.541
Haggis, T. (2003). Constructing images of ourselves? a critical investigation into “approaches to learning” research in higher education. Br. Educ. Res. J. 29, 89–104. doi: 10.1080/0141192032000057401
Harackiewicz, J. M., Barron, K. E., Tauer, J. M., and Elliot, A. J. (2002). Predicting success in college: a longitudinal study of achievement goals and ability measures as predictors of interest and performance from freshman year through graduation. J. Educ. Psychol. 94, 562–575. doi: 10.1037/0022-0663.94.3.562
Harackiewicz, J. M., and Hulleman, C. S. (2010). The importance of interest: the role of achievement goals and task values in promoting the development of interest. Soc. Pers. Psychol. Compass 4, 42–52. doi: 10.1111/j.1751-9004.2009.00207.x
Hartwig, M. K., and Dunlosky, J. (2012). Study strategies of college students: are self-testing and scheduling related to achievement? Psychon. Bull. Rev. 19, 126–134. doi: 10.3758/s13423-011-0181-y
Hidi, S. (2006). Interest: a unique motivational variable. Educ. Res. Rev. 1, 69–82. doi: 10.1016/j.edurev.2006.09.001
Hulleman, C. S., Durik, A. M., Schweigert, S. A., and Harackiewicz, J. M. (2008). Task values, achievement goals, and interest: an integrative analysis. J. Educ. Psychol. 100, 398–416. doi: 10.1037/0022-0663.100.2.398
Hulleman, C. S., and Harackiewicz, J. M. (2009). Promoting interest and performance in high school science classes. Science 326, 1410–1412. doi: 10.1126/science.1177067
Hulleman, C. S., Schrager, S. M., Bodmann, S. M., and Harackiewicz, J. M. (2010). A meta-analytic review of achievement goal measures: different labels for the same constructs or different constructs with similar labels? Psychol. Bull. 136, 422–449. doi: 10.1037/a0018947
Karabenick, S. A. (2003). Seeking help in large college classes: a person-centered approach. Contemp. Educ. Psychol. 28, 37–58. doi: 10.1016/s0361-476x(02)00012-7
Kirk-Johnson, A., Galla, B. M., and Fraundorf, S. H. (2019). Perceiving effort as poor learning: the misinterpreted-effort hypothesis of how experienced effort and perceived learning relate to study strategy choice. Cogn. Psychol. 115:101237. doi: 10.1016/j.cogpsych.2019.101237
Koriat, A. (2008). Easy comes, easy goes? the link between learning and remembering and its exploitation in metacognition. Mem. Cognit. 36, 416–428. doi: 10.3758/MC.36.2.416
Lee, Y., Wormington, S. V., Linnenbrink-Garcia, L., and Roseth, C. J. (2017). A short-term longitudinal study of stability and change in achievement goal profiles. Learn. Individ. Diff. 55, 49–60. doi: 10.1016/j.lindif.2017.02.002
Liem, A. D., Lau, S., and Nie, Y. (2008). The role of self-efficacy, task value, and achievement goals in predicting learning strategies, task disengagement, peer relationship, and achievement outcome. Contemp. Educ. Psychol. 33, 486–512. doi: 10.1016/j.cedpsych.2007.08.001
Lipstein, R. L., and Renninger, K. A. (2007). Interest for writing: how teachers can make a difference. Engl. J. 96, 79–85. doi: 10.2307/30047170
Little, J. L., and Bjork, E. L. (2016). Multiple-choice pretesting potentiates learning of related information. Memory and Cogn. 44, 1085–1101. doi: 10.3758/s13421-016-0621-z
Lo, Y., Mendell, N. R., and Rubin, D. B. (2001). Testing the number of components in a normal mixture. Biometrika 88, 767–778. doi: 10.1093/biomet/88.3.767
Mannes, S. M., and Kintsch, W. (1987). Knowledge organization and text organization. Cogn. Instr. 4, 91–115. doi: 10.1207/s1532690xci0402_2
McCabe, J. (2011). Metacognitive awareness of learning strategies in undergraduates. Mem. Cogn. 39, 462–476. doi: 10.3758/s13421-010-0035-2
McDaniel, M. A., and Masson, M. E. (1985). Altering memory representations through retrieval. J. Exp. Psychol. Learn. Mem. Cogn. 11, 371–385. doi: 10.1037/0278-7393.11.2.371
Midgley, C., Kaplan, A., and Middleton, M. (2001). Performance-approach goals: good for what, for whom, under what circumstances, and at what cost? J. Educ. Psychol. 93, 77–86. doi: 10.1037/0022-0663.93.1.77
Miele, D. B., Finn, B., and Molden, D. C. (2011). Does easily learned mean easily remembered?: it depends on your beliefs about intelligence. Psychol. Sci. 22, 320–324. doi: 10.1177/0956797610397954
Miele, D. B., and Molden, D. C. (2010). Naive theories of intelligence and the role of processing fluency in perceived comprehension. J. Exp. Psychol. Gen. 139, 535–557. doi: 10.1037/a0019745
Murdock, T. B., Miller, A., and Kohlhardt, J. (2004). Effects of classroom context variables on high school students’ judgments of the acceptability and likelihood of cheating. J. Educ. Psychol. 96:765. doi: 10.1037/0022-0663.96.4.765
Nolen, S. B. (1988). Reasons for studying: motivational orientations and study strategies. Cogn. Instr. 5, 269–287. doi: 10.1207/s1532690xci0504_2
Oyserman, D. (2015). Pathways to Success Through Identity-Based Motivation. Oxford: Oxford University Press.
Oyserman, D., Bybee, D., and Terry, K. (2006). Possible selves and academic outcomes: how and when possible selves impel action. J. Pers. Soc. Psychol. 91, 188–204. doi: 10.1037/0022-3514.91.1.188
Oyserman, D., Elmore, K., Novin, S., Fisher, O., and Smith, G. C. (2018). Guiding people to interpret their experienced difficulty as importance highlights their academic possibilities and improves their academic performance. Front. Psychol. 9:781. doi: 10.3389/fpsyg.2018.00781
Pintrich, P. R., Smith, D. A. F., Garcia, T., and McKeachie, W. J. (1991). A Manual for the Use of the Motivated Strategies for Learning Questionnaire (MSLQ). Ann Arbor, MI: The University of Michigan.
Renninger, K. A., Bachrach, J. E., and Posey, S. K. E. (2008). “Learner interest and achievement motivation,” in Social Psychological Perspectives, eds M. L. Martin, S. A. Karabenick, and T. C. Urdan (Derby: Emerald), 461–491. doi: 10.1016/s0749-7423(08)15014-2
Renninger, K. A., Ewen, L., and Lasher, A. K. (2002). Individual interest as context in expository text and mathematical word problems. Learn. Instr. 12, 467–491. doi: 10.1016/S0959-4752(01)00012-3
Renninger, K. A., and Hidi, S. (2002). “Student interest and achievement: developmental issues raised by a case study,” in Development of Achievement Motivation, eds A. Wigfield and J. S. Eccles (Cambridge, MA: Academic Press), 173–195. doi: 10.1016/b978-012750053-9/50009-7
Robins, R. W., and Pals, J. L. (2002). Implicit self-theories in the academic domain: implications for goal orientation, attributions, affect, and self-esteem change. Self Identity 1, 313–336. doi: 10.1080/15298860290106805
Roediger, H. L., and Karpicke, J. D. (2006). Test-enhanced learning: taking memory tests improves long-term retention. Psychol. Sci. 17, 249–255. doi: 10.1111/j.1467-9280.2006.01693.x
Roediger, H. L., Putnam, A. L., and Smith, M. A. (2011). “Chapter one—ten benefits of testing and their applications to educational practice,” in Psychology of Learning and Motivation, eds J. P. Mestre and B. H. Ross (Cambridge, MA: Academic Press).
Rosenberg, J. M., Beymer, P. N., Anderson, D. J., Lissa, C. J. V., and Schmidt, J. A. (2018). tidyLPA: an r package to easily carry out latent profile analysis (LPA) using open-source or commercial software. J. Open Source Softw. 3:978. doi: 10.21105/joss.00978
Rowland, C. A. (2014). The effect of testing versus restudy on retention: a meta-analytic review of the testing effect. Psychol. Bull. 140, 1432–1463. doi: 10.1037/a0037559
Sana, F., Forrin, N. D., Sharma, M., Dubljevic, T., Ho, P., Jalil, E., et al. (2020). Optimizing the efficacy of learning objectives through pretests. CBE—Life Sci. Educ. 19:ar43. doi: 10.1187/cbe.19-11-0257
Schiefele, U. (1991). Interest, learning, and motivation. Educ. Psychol. 26, 299–323. doi: 10.1080/00461520.1991.9653136
Schwarz, G. (1978). Estimating the dimension of a model. Ann. Stat. 6, 461–464. doi: 10.1214/aos/1176344136
Senko, C., Hama, H., and Belmonte, K. (2013). Achievement goals, study strategies, and achievement: A test of the “learning agenda” framework. Learn. Individ. Diff. 24, 1–10. doi: 10.1016/j.lindif.2012.11.003
Senko, C., Hulleman, C. S., and Harackiewicz, J. M. (2011). Achievement goal theory at the crossroads: old controversies, current challenges, and new directions. Educ. Psychol. 46, 26–47. doi: 10.1080/00461520.2011.538646
Simons, J., Dewitte, S., and Lens, W. (2004). The role of different types of instrumentality in motivation, study strategies, and performance: know why you learn, so you’ll know what you learn! Br. J. Educ. Psychol. 74, 343–360. doi: 10.1348/0007099041552314
Soderstrom, N. C., and Bjork, R. A. (2015). Learning versus performance: an integrative review. Perspect. Psychol. Sci. 10, 176–199. doi: 10.1177/1745691615569000
Vrugt, A., and Oort, F. J. (2008). Metacognition, achievement goals, study strategies and academic achievement: pathways to achievement. Metacogn. Learn. 3, 123–146. doi: 10.1007/s11409-008-9022-4
Weinstein, C. E., Palmer, D., and Schulte, A. C. (1987). Learning and Study Strategies Inventory (LASSI). Clearwater, FL: H & H Publishing.
Wigfield, A., and Cambria, J. (2010). Students’ achievement values, goal orientations, and interest: definitions, development, and relations to achievement outcomes. Dev. Rev. 30, 1–35. doi: 10.1016/j.dr.2009.12.001
Wigfield, A., and Eccles, J. S. (2000). Expectancy-value theory of achievement motivation. Contemp. Educ. Psychol. 25, 68–81. doi: 10.1006/ceps.1999.1015
Yan, V. X., Clark, C. M., and Bjork, R. A. (2017). “Memory and metamemory considerations in the instruction of human beings revisited: implications for optimizing online learning,” in from the Laboratory to the Classroom: Translating Science of Learning for Teachers, eds J. C. Horvath, J. M. Lodge, and J. Hattie (Abingdon-on-Thames: Routledge/Taylor & Francis Group), 61–78.
Yan, V. X., and Oyserman, D. (2017). Linking mindsets with toolsets: interpretations of experienced difficulty matter for knowing how to learn. Poster Presented at the 58th Annual Scientific Meeting of the Psychonomic Society, Vancouver, Canada.
Yan, V. X., Thai, K. P., and Bjork, R. A. (2014a). Habits and beliefs that guide self-regulated learning: do they vary with mindset? J. Appl. Res.Mem. Cogn. 3, 140–152. doi: 10.1016/j.jarmac.2014.04.003
Yan, V. X., Yu, Y., Garcia, M. A., and Bjork, R. A. (2014b). Why does guessing incorrectly enhance, rather than impair, retention? Mem. Cogn. 42, 1373–1383. doi: 10.3758/s13421-014-0454-6
Yeager, D. S., Hanselman, P., Walton, G. M., Murray, J. S., Crosnoe, R., Muller, C., et al. (2019). A national experiment reveals where a growth mindset improves achievement. Nature 573, 364–369. doi: 10.1038/s41586-019-1466-y
Keywords: implicit beliefs, achievement goals, value, study strategies, interest
Citation: Yan VX and Wang L (2021) What Predicts Quality of Learners’ Study Efforts? Implicit Beliefs and Interest Are Related to Mastery Goals but Not to Use of Effective Study Strategies. Front. Educ. 6:643421. doi: 10.3389/feduc.2021.643421
Received: 18 December 2020; Accepted: 16 March 2021;
Published: 08 April 2021.
Edited by:
Yves Karlen, University of Applied Sciences and Arts Northwestern Switzerland, SwitzerlandReviewed by:
Martin Daumiller, University of Augsburg, GermanyJennifer M. Johnson, Temple University, United States
Copyright © 2021 Yan and Wang. This is an open-access article distributed under the terms of the Creative Commons Attribution License (CC BY). The use, distribution or reproduction in other forums is permitted, provided the original author(s) and the copyright owner(s) are credited and that the original publication in this journal is cited, in accordance with accepted academic practice. No use, distribution or reproduction is permitted which does not comply with these terms.
*Correspondence: Veronica X. Yan, dmVyb25pY2F5YW5AYXVzdGluLnV0ZXhhcy5lZHU=