- 1Department of Pharmaceutical Microbiology, Muhimbili University of Healthy and Allied Sciences, Dar es Salaam, Tanzania
- 2Muhimbili Sickle Cell Program, Department of Haematology and Blood Transfusion, Muhimbili University of Healthy and Allied Sciences, Dar es Salaam, Tanzania
- 3Department of Molecular Biology and Biotechnology, University of Dar es Salaam, Dar es Salaam, Tanzania
- 4Plant Protection Department, Swedish University of Agricultural Sciences, Alnarp, Sweden
- 5Department of Biological Sciences, Dar es Salaam University College of Education, Dar es Salaam, Tanzania
- 6Department of Crop Sciences and Horticulture, Sokoine University of Agriculture, Morogoro, Tanzania
- 7Department of Biochemistry, Mbeya College of Health and Allied Sciences, University of Dar es Salaam, Mbeya, Tanzania
Due to the insufficient human and infrastructure capacity to use novel genomics and bioinformatics technologies, Sub-Saharan Africa countries have not entirely ripped the benefits of these technologies in health and other sectors. The main objective of this study was to map out the interest and capacity for conducting bioinformatics and related research in Tanzania. The survey collected demographic information like age group, experience, seniority level, gender, number of respondents per institution, number of publications, and willingness to join the community of practice. The survey also investigated the capacity of individuals and institutions about computing infrastructure, operating system use, statistical packages in use, the basic Microsoft packages experience, programming language experience, bioinformatics tools and resources usage, and type of analyses performed. Moreover, respondents were surveyed about the challenges they faced in implementing bioinformatics and their willingness to join the bioinformatics community of practice in Tanzania. Out of 84 respondents, 50 (59.5%) were males. More than half of these 44 (52.4%) were between 26–32 years. The majority, 41 (48.8%), were master’s degree holders with at least one publication related to bioinformatics. Eighty (95.2%) were willing to join the bioinformatics network and initiative in Tanzania. The major challenge faced by 22 (26.2%) respondents was the lack of training and skills. The most used resources for bioinformatics analyses were BLAST, PubMed, and GenBank. Most respondents who performed analyses included sequence alignment and phylogenetics, which was reported by 57 (67.9%) and 42 (50%) of the respondents, respectively. The most frequently used statistical software packages were SPSS and R. A quarter of the respondents were conversant with computer programming. Early career and young scientists were the largest groups of responders engaged in bioinformatics research and activities across surveyed institutions in Tanzania. The use of bioinformatics tools for analysis is still low, including basic analysis tools such as BLAST, GenBank, sequence alignment software, Swiss-prot and TrEMBL. There is also poor access to resources and tools for bioinformatics analyses. To address the skills and resources gaps, we recommend various modes of training and capacity building of relevant bioinformatics skills and infrastructure to improve bioinformatics capacity in Tanzania.
Introduction
Recently, the field of genomics has become instrumental in medical research and provision of healthcare diagnosis, understanding prevention, and treatment of several disease conditions (Adedokun et al., 2016; Shoko et al., 2018). This was fuelled by the increased ability to generate data and perform bioinformatics analysis, which has become critical for biomedical scientists (Mulder et al., 2016a), particularly in the face of the continued fall of the cost of data generation and analysis using trending technologies (Mulder et al., 2016a).
Despite the decreasing cost of using bioinformatics technologies for research at the global level, Sub-Saharan Africa (SSA) countries, including Tanzania, face difficulties accessing quality health despite the significant disease burden in these countries (de Martel et al., 2020). The lag in SSA is due to the lack of human and technological capacity to run and interpret such bioinformatics analysis effectively, thus hindering the benefits of applying genomics in medicine and other research areas (Karikari et al., 2015; Adedokun et al., 2016; Mulder et al., 2017). The hurdle in health research also extends into leveraging new technologies such as genomics and bioinformatics to resolve some significant issues such as food insecurity and poverty (Lyantagaye, 2013) by focusing on human health, agriculture and animals production research.
Several initiatives have been established to address the gap. One of the initiatives is the Human Heredity and Health in Africa (H3Africa, 2021a) Pan Africa Bioinformatics Network (H3ABioNet) (Mulder et al., 2017). This African initiative was established to facilitate bioinformatics capacity in the continent and health genomics research (H3Africa, 2021b; Mulder et al., 2016a). H3Africa has successfully mobilized resources and developed researchers’ networks and capacity for various research resources, including biobanks, developing researchers networks, and capacity to analyze genomics data through H3ABioNet (Mulder et al., 2017). Through this network, both human and infrastructure bioinformatics needs have been addressed through training, setting up of standardized bioinformatics analysis workflows, access to expertise in various domains and data harmonization have been put in place in Africa (Mulder et al., 2017).
However, individual countries may not have fully embraced the collaborative efforts to strengthen the bioinformatics capacity. For example, in Tanzania, only three nodes became members of the network. These were initially the University of Dar es Salaam (UDSM), Muhimbili University of Health and Allied Sciences (MUHAS) and the Management and Development for Health (MDH). MUHAS is still an active member since 2012, with a renewed new grant for the year 2017–2022 (H3ABionet, 2021). Tanzania is also a part of other international and regional bioinformatics networks and consortia, including the Eastern Africa Network for Bioinformatics Training (EANBiT) (Hernández-de-Diego et al., 2017) and the African Society for Bioinformatics and Computational Biology (ASBCB) (http://www.asbcb.org/). Several local initiatives are geared towards advancing the capacity to conduct bioinformatics and related research, such as the Tanzania Genome Network, an association of bioinformaticians from public and private research institutions, and the Tanzania Society of Human Genetics (TSHG).
Currently, only the University of Dar es Salaam (UDSM) and Sokoine University of Agriculture (SUA) in Tanzania offer some form of training in Bioinformatics. The UDSM and SUA undergraduate prospectus 2018/2019 include selected programs offering Bioinformatics courses at undergraduate and postgraduate levels (Lyantagaye, 2013). These bioinformatics courses are embedded in other programs and none of the Tanzanian universities offer a pure bioinformatics program at undergraduate or postgraduate levels.
There is a need to document the existing human capacity for conducting bioinformatics-related research and analyses. This will enable effective leveraging of existing resources and strategizing to build sustainable expertise in the country further. There is no documentation on the existing bioinformatics capacity in the country to the best of our knowledge.
This study aimed to evaluate the existing human expertise and capacity to use bioinformatics tools for research in public and private institutions to address this challenge. On the one hand, the documentation is hoped to guide the leveraging of present resources and identify areas for improvement and training. On the other hand, it will also support the H3Africa and H3ABioNet and other projects' efforts to build bioinformatics capacity in Africa. The study findings may help to make recommendations for improvement in bioinformatics training and research in Tanzania, a model that can be emulated in other SSA countries. Training people in Bioinformatics will also provide the critical mass to manage the local resources such as computing infrastructure, data centers and high-performance computers.
Materials and Methods
This is a cross-sectional, explorative, descriptive study among researchers and academics in Tanzania's public and private academic and research institutions. The study employed a self-administered online survey to gather information regarding the baseline status of bioinformatics practices in Tanzania.
An online survey was developed and distributed using REDCap tool (Harris et al., 2009; Harris et al., 2019). The study population included staff from research institutions and academic institutions offering education in health, agriculture and other natural sciences. A survey link was sent to scientists in Tanzania’s academic and research institutions, including research, education, and commercial institutions. The survey was distributed through individual emails, mailing lists in relevant groups such as Tanzania Genome Network (TGN) and institutional mailing lists and social media platforms. The English language was used for the survey since it is the official language of communication in academia and research in Tanzania. The survey was conducted between September 2018 and November 2018.
The survey began with an introduction to the bioinformatics research, an explanation of the study’s objectives and the information expected from the participant. Participants were assured of anonymity and privacy of collected data by reporting it in an aggregated format. Information captured included respondents’ demographics such as employment institution, age group, gender, level of seniority, and area of research. The level of seniority question intended to capture self-perceived positioning of seniority in the profession where 0–50 was the early carrier, 50 was a mid-carrier and 51–100 was a senior. Other questions related to the years of work experience, number of publications in bioinformatics, and the highest level of education attained. The sections that followed investigated access to and knowledge about infrastructure and software tools for bioinformatics analysis. We also asked questions about access to computing facilities and computer operating systems in regular use by the respondents.
We evaluated the skill levels of the selected Microsoft Office tools and selected statistical packages as well as the frequency of use of some basic bioinformatics resources such as PubMed (Ossom Williamson and Minter, 2019), Swiss-prot and TrEMBL—Protein sequence databases (Bairoch, 1996), National Center for Biotechnology Information (NCBI) ’s BLAST search (McGinnis and Madden, 2004), GenBank (Clark et al., 2016), European Bioinformatics Institute (EMBL-EBI) (Li et al., 2015; Madeira et al., 2019), DNA Data Bank of Japan (DDBJ) (Mashima et al., 2017), Entrez Genome Browser, Human Genome Browser from UCSC (Kent et al., 2002), Protein Data Bank (PDB) (Berman, 2000), sequence alignments software such as Muscle (Edgar, 2004), T-coffee (Di Tommaso et al., 2011) and CLC Workbench (a QIAGEN product for DNA, RNA and protein sequence data analysis) (Smith, 2015). Lastly, we asked questions intending to understand frequently analyzed tasks, ranging from sequence alignment, phylogenetic, 16s data analysis, genome-wide association studies (GWAS), internal transcribed spacer (ITS) data analysis, variant calling, genome annotation, RNASeq, proteomics and other tasks as specified.
We also asked questions intended to investigate the participants’ knowledge and type of computer programming languages and the computer database management systems preferred. We sought out to identify the challenges that respondents face in bioinformatics research. The broader problems were re-categorized into electric power and internet, mentorship and research network, computer infrastructure, and training skills.
Finally, we interrogated the participants’ willingness to join the bioinformatics network and initiative in Tanzania under the TGN.
Total Bioinformatics Analysis Knowledge Score
The total analysis score was calculated based on scoring knowledge of nine essential bioinformatics skills. These included sequence alignment, phylogenetics, 16s analysis, GWAS, ITS, variant calling, genome annotation, RNASeq or proteomics. A respondent scored a '1′ for each skill they knew and then a total score was calculated.
Statistical Analysis
The survey responses were exported from REDCap into a comma-separated file for analysis. Analysis of the results was conducted using R (R Development Core Team, 2020) software integrated into R Studio version 1.2.5033.
Descriptive statistics, including frequency tables and bar plots, were used to summarize the responses. The Pearson chi-square test was employed to determine the association between the publication status and knowledge of Bioinformatics analysis tools, taking a p-value < 0.05 as a significant cutoff at a 95% confidence interval.
Ethical Approval and Consent to Participate
Participant’s consent was requested before conducting the survey. The survey was halted if the participants opted not participating in the survey. The Muhimbili University of Health and Allied Sciences (MUHAS) Research Ethics Committee granted a study waiver of informed consent. No identifying information was collected.
Results
Demographic Characteristics of Respondents
A total of 90 respondents from academic and non-academic institutions participated in the survey. Six respondents were removed because they acknowledged that they do not know anything about bioinformatics at the beginning of the survey. The majority of respondents (Table 1) were male participants, 50 (59.5%), while females were only 34 (40.5%). When asked to self-rate their seniority on a scale of 0–100, respondents rated themselves with mean seniority of 39.1 [Interquartile range (IQR) 8.0–53.0]. The mean work experience of the respondents in years was 6.2 (IQR 2.0–8.0). Concerning the participants’ age groups, the majority of respondents, 44 (52.4%), were aged between 26 and 32 years (Table 1). The highest education level attained by most respondents were master's degree holders 41 (45.8%) followed by bachelor degree holders 25 (29.8%) (Table 1).
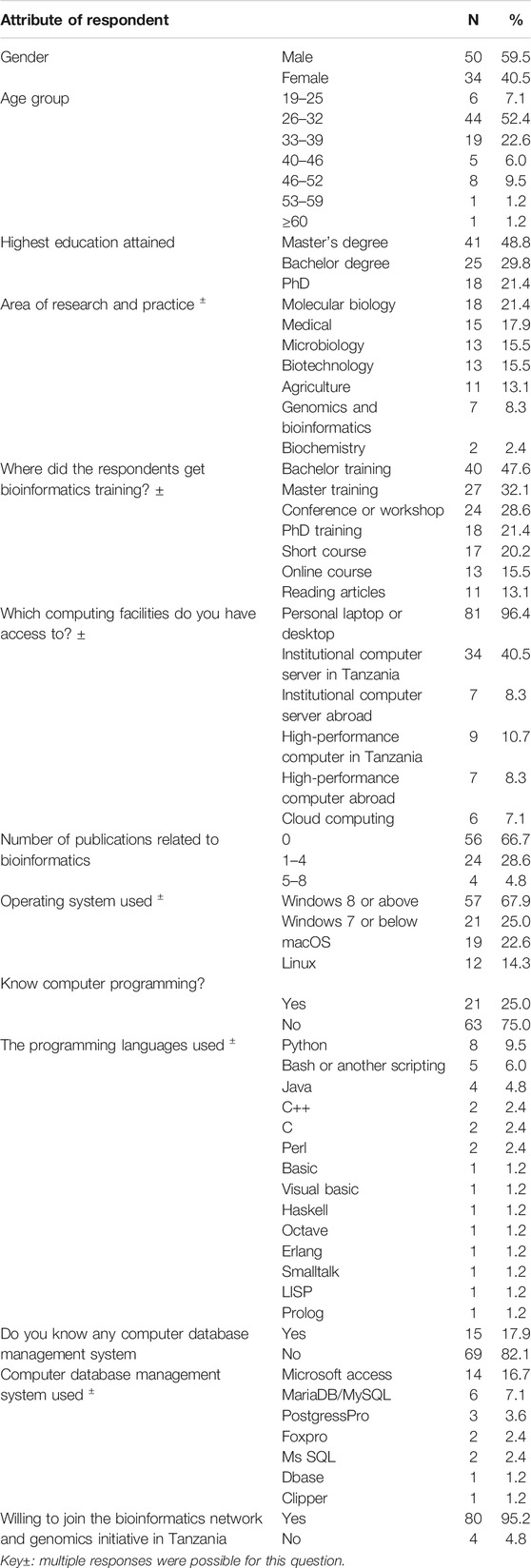
TABLE 1. Demographic attributes of the respondents surveyed about bioinformatics practice in Tanzania (N = 84).
The number of publications related to bioinformatics by the respondents was mainly in the range of 1–4, as reported by 24 (28.57) respondents (Table 1). Altogether, only 28 (33.3%) of the surveyed respondents have at least one publication about bioinformatics. In comparison, 56 (67.7%) did not have any publications in bioinformatics. We did not find any association between the number of publications and the total score of bioinformatics analysis knowledge (Chi-square p-value = 0.360) (Supplementary Table S1).
The area of research or practice for the majority of the respondents was molecular biology 18 (21.4%), followed by 15 (17.9%) from the field of medicine (Table 1).
Most of the respondents reported learning bioinformatics at bachelor 40 (47.6%), followed by master’s training 27 (32.1%) and other sources (Table 1).
Access to Infrastructure for Bioinformatics Analysis
Eighty-one (96.4%) of the respondents used their personal computers (laptops) for bioinformatics work. A small percentage (less than 10%) indicated having access to institutional servers abroad or computer cloud (Table 1). Fifty-seven (67.9%) of these respondents run their computers on Windows 8. Only twelve (14.3%) of these respondents have the Linux operating system on their computer systems (Table 1).
Knowledge and use of computer programming language and database management systems.
Only a quarter of the respondents reported using computer programming language and 15 (17.9%) use a database management system. The most used programming language is Python by 8 (9.5%) of the respondents. The widely used database management systems were Microsoft Access and MariaDB/MySQL, which were used by 14 (16.7%) and 6 (7.1%) of the respondents, respectively (Table 1).
Out of the 84 respondents confirmed to know bioinformatics, 80 (95.2%) (Table 1) were willing and ready to join the bioinformatics network and initiative in Tanzania under the TGN.
The majority of respondents were from research institutions, 50 (59.5%) (Table 2). The respondents were from a total of 33 institutions (Supplementary Table S2).
Challenges Facing Bioinformatics in Tanzania
More than half of the respondents reported one or more problems in Tanzania's bioinformatics practice (Table 3). The majority, 22 (26.2%), reported a lack of training and skills as a significant problem. Only 2 (2.4%) of the respondents reported inadequate electrical power supply and lack of internet access (Table 3). All except one of the 51 respondents faced challenges running bioinformatics analyses use personal computers or laptops. However, even those who did not face challenges, only one out of 24 (Chi-Square p-value = 0.338) used infrastructure other than personal computers or laptops (Supplementary Table S3).
Many challenges were given by participants in the categories shown in Table 3. Here are two examples of challenges stated by the responses that were given as free text. A female respondent replied, “Yes, I face challenges. I used JoinMap (Ooijen, 2021) (a Microsoft-Windows program for the calculation of genetic linkage maps in experimental populations of diploid species) when I was in a (university in the United States) doing DNA sequence alignment, linkage mapping and quantitative trait locus (QTL) analysis which was under (the university in the United States) license. When I came back to a (University in Tanzania). I started facing difficulties because the [University in Tanzania] does not have such a program. In addition, there are only a few individuals working on research involving sequencing at the (University in Tanzania). Due to this problem, I had to send back my data to the (University in the United States) for assistance in performing the analysis instead of doing it by myself in the (University in Tanzania)”. Another male respondent said, “Yes, I face challenges in bioinformatics. We do not have a well-recognized, reputable center for training on bioinformatics in Tanzania. During our studies, the bioinformatics training was merely an overview and a few practical demonstrations. At least we can do partial sequence analyses on data such as sequence alignment and phylogeny. However, extensive proteomics analysis is still a challenge. Besides, whole-genome sequence analysis is a challenge in many institutions in Tanzania.
Nevertheless, the world is moving toward whole-genome approaches. Therefore, Tanzanian experts need to disseminate their knowledge to their global counterparts. For instance, many PhD students plan to undertake whole-genome analysis in their research at the (University in Tanzania). However, almost all of them plan to go to the International Livestock Research Institute (ILRI) in Kenya to train on bioinformatics and perform whole genome sequencing and analysis”.
Usage of Bioinformatics Tools and Genomics and Bioinformatics Analyses Performed by the Respondents
Of the surveyed bioinformatics tools and resources, the seldom-used ones were QIAGEN CLC Main Workbench, where 57 (67.9%) respondents reported that they never used the program. This was followed by the DNA Data Bank of Japan (DDBJ), where 52 (61.9%) never used the resource. The most used resources were BLAST, PubMed and GenBank (Figure 1).
The majority, 57 (67.9%) of the surveyed participants, did perform sequence alignment, followed by 42 (50%) who carried out phylogenetics analysis (Figure 2).
Software Usage of Statistical Package and Microsoft Office Products by Respondents
Regarding statistical software packages, the least use of statistical software packages was reported by 78 (92.9%) in WinBUGS followed by 73 (86.9%) in MedCalc (Figure 3). The frequently used were SPSS and R, where respondents report expert, high and intermediate skills in these tools (Figure 3).
On the one hand, respondents reported more Microsoft Word expertise 27 (32.1%), followed by Microsoft PowerPoint 19 (23.2%). On the other hand, less expertise 1 (1.3%) was noted in Microsoft Access (Figure 4). These numbers self-reporting high skills are slightly higher for with 44 (52.4%) and 38 (46.3%) and 13 (16.3%), in Microsoft Word, Microsoft PowerPoint and Microsoft Access, respectively (Figure 4).
Discussion
This is the first study that assesses the level of bioinformatics capacity in Tanzania to the best of our knowledge. We found out that the majority of the respondents were males, had a master’s degree and were in the age group 26–32 years. The mean work experience of the respondents in years was 6.2, indicating a young group of scientists. The highest education level for most respondents was a master’s degree, followed by a bachelor’s degree. When asked to rate their seniority on a scale of 0–100, the respondents rated themselves with mean seniority of 39.1, further indicating the junior ship’s perception in the area of bioinformatics practice. Only 21.4% were PhD holders; this pool of scientists can mentor the early-career counterparts. Interestingly, most of the respondents' current specialization area was mostly molecular biology. Only a few related their complete research interest in genomics and bioinformatics, suggesting that molecular biology scientists diversify their careers into bioinformatics.
This survey pointed out that the infrastructure and the human capacity to conduct bioinformatics-related research in Tanzania are underdeveloped. Precisely, 96.4% of the respondents perform bioinformatics analysis using personal computers/laptops, with only about 10% having access to advanced infrastructures such as high-performance computers, cloud computing and institutional servers. Although 40.5% of respondents have access to the institutional computer server, these servers are mainly available to provide file and printing services rather than bioinformatics services.
This severely limits the capacity to conduct bioinformatics-related research. It usually involves massive datasets and requires reliable high computing capacity that personal computers cannot afford alone (Johansen Taber et al., 2014). More than 67% of the respondents use Windows operating system (OS), which does not support many genomics and bioinformatics analysis platforms, contrary to only about 14.3% who use the Linux OS that supports a broad range of bioinformatics analysis tools. However, there is a possibility that respondents using Windows use it to run bioinformatics analysis such as phylogenetics with Windows-based software. The same respondents may also use their personal Windows machines to access online-based tools such as BLAST. There are software programs that efficiently run in Windows MEGA (Kumar et al., 2016) and UGENE (Okonechnikov et al., 2012), JALVIEW (Waterhouse et al., 2009) for protein and DNA alignments. In addition, Windows 10 ships with a Windows Subsystem for Linux (WSL), which provides support to run native Linux command-line tools directly on Windows operating system (https://docs.microsoft.com/en-us/windows/wsl/faq). This has allowed running most of the bioinformatics tools directly on Windows. Nevertheless, it is still necessary to know Linux command lines to use this resource. In addition, some Linux-based packages may be hard to run in this environment.
For most respondents, the usage of standard bioinformatics analysis tools was also low; therefore, it comes as no surprise that 66.7% of the respondents had no publication related to bioinformatics. These findings align with Lyantagaye's (2013) review, which noted that the level of bioinformatics research in Tanzania was still in its infancy, lacking investment and underdeveloped infrastructure. The review noted the presence of one modern laboratory at SUA, capable of generating molecular biology and genomics data. The STM-1 SEACOM undersea fiber-optic cable was expected to increase the internet speed bandwidth (Lyantagaye, 2013). The situation is not unique to Tanzania alone. Karikari (2015) noted a low level of bioinformatics capacity in terms of personnel and infrastructure in Ghana, with frequent electrical power failures, unreliable internet connections, and lack of high-speed computing power being significant infrastructural challenges (Karikari, 2015). In Africa, three countries are responsible for a large fraction of the continent’s bioinformatics output; South Africa, Kenya, and Nigeria. The existence of H3ABioNet has, to a large extent, tried to reduce this disparity by empowering other countries in Africa to participate and contribute to bioinformatics (Matovu et al., 2014; Mulder et al., 2016a).
Bioinformatics consists of multidisciplinary fields, including mathematics, computer science, statistics and others. Statistics and programming are among the disciplines that play significant roles in building reproducible methods for biological discovery and validation, especially for complex, high-dimensional data as encountered in genomics. Therefore, assessing the knowledge and level of usage of statistics and programming among the respondents was essential. We found that only a quarter of respondents reported using computer programming language and 17.9% used a database management system. The most used programming language was Python by 8 (9.5%) of the respondents and the database management systems most used were Microsoft Access and MySQL. Both Python and MySQL find wide applications in bioinformatics tools and pipelines (Pasculescu et al., 2014). However, there are a large proportion of respondents without skills in hardcore programming. Short training may help to improve the skills of these researchers. It was also evident that the knowledge and usage of different statistical packages are mainly based on IBM’s SPSS package. On the one hand, many respondents are using R statistical packages. On the other hand, packages like WinBUGS and SAS are rarely used by bioinformatics researchers in Tanzania.
In bioinformatics, it is essential that computational thinking is adopted to increase the pool of hardcore programmers. This will facilitate efficient bioinformatics analyses and communication among scientists, bioinformaticians, and data analysts. To this end, short and long-term training are necessary for computer programming such as Python and R statistical package, among others. Other training should focus on database management. These efforts are essential in Tanzania and other African Scientific communities (Gurwitz et al., 2017). Nevertheless, software like Galaxy (Giardine, 2005) offers a potential advantage for non-programmers. Galaxy training can therefore be handy for biologists who undertake bioinformatics analysis.
Our respondents made high use of Microsoft Office Products, particularly Microsoft Word, Microsoft PowerPoint and Microsoft Excel. Only a few individuals made occasional use of Microsoft Access and Microsoft Outlook, again showing less advanced use of these products. These Microsoft Office Products are not essential for running bioinformatics. However, high reliance on Microsoft Office Products indicates an inclination towards using a Windows-based operating system. In addition, the use of Microsoft Access products may be a step for scientists to begin the use of large databases.
There is a wide range of bioinformatics tools and resources that respondents said they could access, with PubMed, which they use to retrieve scientific literature, which is the most popular. PubMed is widely used by the scientific community, not necessarily by the bioinformatics community. However, the responses about PubMed allow us to gauge its use in comparison with other resources that are widely used in the bioinformatics community. The other frequently used resources in this community were GenBank and some sequence alignment tools, showing good progress as users can access relevant and essential resources. Commercial products such as CLC Workbench (a QIAGEN platform for DNA, RNA and protein sequence data analysis) were limited, probably due to a shortage of funding (Smith, 2015).
More than half of the respondents reported one or more problems they face in relation to bioinformatics practice in Tanzania. The majority of the respondents reported a lack of training and skills as a significant problem. Only a few respondents reported inadequate electrical power supply and lack of internet access as challenges. The reduced cost of internet connectivity and bandwidth improvement has helped other Africa nations improve their bioinformatics infrastructure and capacity (Mulder et al., 2016b). Tanzania has equally benefited from bandwidth improvement, which may be why few respondents cited internet connectivity as a challenge. Capacity building through training and infrastructural support for bioinformatics research remains the major challenge, as noted in other African countries (Karikari, 2015; Karikari et al., 2015; Mulder et al., 2016b; Shoko et al., 2018).
The majority of respondents reported having knowledge of at least two to three bioinformatics skills. The most commonly performed analyses were sequence alignment and phylogenetics. Other methods of analysis, such as GWAS were less commonly used. The most and the least frequent applications may require training modules for long or short-term training to allow scientists to master these critical bioinformatics skills.
In our study, most of the respondents, 40 (47.6%), reported learning bioinformatics at bachelor’s degree level, followed by 27 (32.1%) who learned at the masters’ training and only 18 (21.4%) during PhD training. Conferences and workshops also serve as essential sources of bioinformatics skills for some respondents (28.6%), while a small percentage (15.5%) used online resources to learn bioinformatics skills. These later may have benefitted from the opportunity provided by the H3ABioNet (Gurwitz et al., 2017) in addition to other training opportunities such as those used in other countries (Cattley and Arthur, 2007; Ding et al., 2014; Vincent et al., 2018).
It is possible that most of the surveyed Tanzanian bioinformatics researchers were either trained abroad or learned bioinformatics through postgraduate research projects. Today, no full bioinformatics or computational biology degree program exists in the country. Bioinformatics courses are part of undergraduate and postgraduate degree programs at the University of Dar es Salaam (UDSM) and Sokoine University of Agriculture (SUA). Two undergraduate courses exist at the UDSM according to the UDSM undergraduate prospectus 2018/2019. Besides, seven postgraduate courses also exist at UDSM according to the 2019/2020 postgraduate prospectus. At SUA, three undergraduate and three postgraduate courses are offered (SUA prospectus 2014/15) (Lyantagaye, 2013). Therefore, it is not surprising that most respondents, 16.7 and 14.3% in this study, are from UDSM and SUA, respectively.
There is a long way to go and an opportunity to fill the expertise gap observed in this survey. For starters, Muhimbili University of Health and Allied Sciences (MUHAS) is preparing to start a Master’s of Science in Bioinformatics through collaboration with EANBiT (Eastern Africa Network for Bioinformatics Training) (http://eanbit.icipe.org/). EANBiT has developed a 2-years master’s degree curriculum that has been used in training since 2017 and is expected to be adopted by MUHAS in the foreseeable future (http://eanbit.icipe.org/) (EANBiT). This will be important in establishing a critical mass of expertise in bioinformatics and computational biology in Tanzania. Eventually, it may attract grants, research projects, collaborations, and the development of infrastructure necessary to research in the field.
In terms of curriculum development and training establishment, there are examples to learn from other countries such as India and South Africa (Kulkarni-Kale et al., 2010; Mulder et al., 2016b). In the early days of bioinformatics, the discipline was not embedded in undergraduate curricula in South Africa. To address the gap, students registered for postgraduate degrees in bioinformatics in South African Universities had to start with short formal bioinformatics training before embarking on their studies. Later, the National Bioinformatics Network (NBN) developed joint courses compulsory for NBN-funded students, introducing them to a range of bioinformatics topics, programming and other technical skills (Mulder et al., 2016b). In India, similar initiatives were undertaken by the Biotechnology Information System (BTIS) under the Department of Biotechnology (DBT), Government of India (Ding et al., 2014).
Equally in Tanzania, there is also a need to develop relevant skills by extending undergraduate bioinformatics courses to other universities that offer biomedical, life and computer science courses. Students will be exposed to the field early on and potentially incite their interest. It will also prepare them with basic knowledge and skills for postgraduate research and education specializing in bioinformatics education (Bishop et al., 2015). Besides, we advocate for establishing short programs for professionals who may be constrained by time to do a full-fledged degree. This can go hand in hand with existing programs and infrastructure and collaborate with other organizations in Tanzania, Africa and worldwide. EANBiT, for example, offers a residential training course on bioinformatics for East African students and early career researchers (http://eanbit.icipe.org/content/2018-trainees). Other successful training models were in Sudan (Ahmed et al., 2020).
In the era of digital technologies, bioinformatics capacity in Tanzania could greatly benefit from online learning and has to be prioritized. It is less costly, often self-paced and accessible to many people at the same time. Online learning may be more suitable for professionals who cannot spend time in physical classes. Although a multitude of online learning platforms for bioinformatics exist, relevant organizations and institutions have a critical role in developing an appropriate curriculum and mobilizing resources to facilitate the learning process and ensure that online learning is effective. The duration of vast online courses and resources and providing guidelines to learners is also essential.
Collaborative programs with hybrid virtual-physical models have become especially attractive recently, such as the Courses such as the 3-months Introduction to Bioinformatics (IBT) course offered by H3ABioNet (https://www.h3abionet.org/training/ibt)m) (H3ABionet, 2021). The annual system that has been provided since the year 2016 attracted 364 enrolled participants hosted at 20 institutions across 10 African countries in the inaugural year (Gurwitz et al., 2017). In 2020, the course went utterly online due to physical meeting restrictions caused by the pandemic of COVID-19 but still had over 1,000 participants distributed across 40 classrooms in Africa (H3ABioNet newsletter May 2020: https://spark.adobe.com/page/0OVCv7sPapYfa/). H3ABioNet has also hosted a 16S analysis course since 2019 in a similar manner.
Bioinformatics and computational biology research are expensive to conduct. Establishing collaborations among relevant institutions and stakeholders in Tanzania and with external partners may help develop the necessary infrastructure and conduct research. Collaboration between research institutions, academia, and civil society with similar objectives regarding bioinformatics research catalyzes the field’s rapid growth. The recent establishment of the Tanzania Society of Human Genetics (TSHG) (http://tshg.or.tz/) indicates both the need and interest in furthering this critical biological sub-discipline. This will lead to the development of vital programs and improve the competitiveness of funding. In addition to joining Pan African and global networks, Tanzania needs to plan to improve and offer streamlined bioinformatics services. Initiatives of this nature have worked in other countries such as Australia (Schneider et al., 2019; Tauch and Al-Dilaimi, 2019). To build total capacity in bioinformatics, Tanzania needs to work closely with existing bioinformatics networks to strengthen its capacity through training. The H3ABioNet help desk can help African countries quickly grasp the assistance needed to get going to bioinformatics tasks (Kumuthini et al., 2019). Fostering collaboration in bioinformatics will depend on both scientist-led and Government-led initiatives.
The Government has a pivotal role to play by supporting basic infrastructure for education and training as well as for research and application. The Government also plays a crucial role in promoting human capacity building in bioinformatics and computational biology by ensuring that graduates are recognized by the government scheme and get job opportunities. The collaborative approach will help guarantee the sustainability of the initiatives, training, and infrastructure and research activities. Tanzania can emulate examples from other countries where government funding has facilitated bioinformatics (Mulder et al., 2016b; Schneider et al., 2019; Tauch and Al-Dilaimi, 2019). In South Africa, the bioinformatics leader in Africa, the very early phase of bioinformatics at the South African National Bioinformatics Institute (SANBI) on the University of the Western Cape (UWC) campus was co-funded by the Government through the South Africa's National Research Foundation (NRF) (Mulder et al., 2016b). Tanzania and other African countries need to emulate the funding models of SANBI to improve bioinformatics skills and research in their institutions.
The respondents agreed to participate in the bioinformatics network and genomics initiative in Tanzania. The bioinformatics community needs to work with the Government to support a national forum that brings together bioinformaticians and genomics practitioners to discuss common interest issues. Such a forum can already build on the existing platforms such as TGN and the TSHG to facilitate joint meetings and promote a bioinformatics agenda. Similar National platforms have been shown to help build bioinformatics capacity in South Africa, India and Australia (Kulkarni-Kale et al., 2010; Mulder et al., 2016b; Schneider et al., 2019).
Conclusion
In this study, we found out that the majority of the respondents engaging in bioinformatics research in Tanzania were at the early stages of their careers. Although there is a high level of interest in bioinformatics in Tanzania, a low level of skilled human resources and the lack of infrastructure pertinent to research in the field are limited. The use of bioinformatics tools for data analysis is still low, even for essential analysis tools such as BLAST (McGinnis and Madden, 2004), GenBank (Clark et al., 2016), sequence alignment software, Swiss-prot (Bairoch, 1996) and TrEMBL (Bairoch, 1996). This may be because most respondents also lacked access to basic tools and resources for bioinformatics research.
Investment in human capacity building through undergraduate and postgraduate training and encouraging and promoting digital learning may help improve the situation. Provision of infrastructure, mentorship and networking is needed to improve bioinformatics capacity in Tanzania. We recommend building strong collaborations among Tanzania institutions to promote the effective utilization of shared resources and expertise. Moreover, regional and global network partners and stakeholders may be crucial in developing infrastructure and research activities and ensuring sustainability. Support from the Government by setting the groundwork and funding basic teaching and research infrastructure is also essential to the growth and success of the field. The launch of a community of practice such as the TSHG of the TGN may help continue the Pan-African efforts to promote the use of bioinformatics for the betterment of humankind.
Data Availability Statement
The raw data supporting the conclusion of this article will be made available by the authors, without undue reservation.
Author Contributions
All authors have read and approved the manuscript; RS and AM designed the survey and collected the data; UM and RS performed the statistical analysis and resulted in interpretation; UM, RS, SN, LM, SLL, AM, DM and JM contributed to writing and reviewing the manuscript.
Conflict of Interest
The authors declare that the research was conducted in the absence of any commercial or financial relationships that could be construed as a potential conflict of interest.
Acknowledgments
We wish to thank the respondents who took the time to respond to this survey. This article has been released as a pre-print at https://www.researchsquare.com/article/rs-112131/v1, (Sangeda et al., 2020).
Supplementary Material
The Supplementary Material for this article can be found online at: https://www.frontiersin.org/articles/10.3389/feduc.2021.665313/full#supplementary-material
References
Adedokun, B. O., Olopade, C. O., and Olopade, O. I. (2016). Building Local Capacity for Genomics Research in Africa: Recommendations from Analysis of Publications in Sub-saharan Africa from 2004 to 2013. Glob. Health Action. 9, 31026. doi:10.3402/gha.v9.31026
Ahmed, A. E., Awadallah, A. A., Tagelsir, M., Suliman, M. A., Eltigani, A., Elsafi, H., et al. (2020). Delivering Blended Bioinformatics Training in Resource-Limited Settings: a Case Study on the University of Khartoum H3ABioNet Node. Brief. Bioinform. 21, 719–728. doi:10.1093/bib/bbz004
Bairoch, A. (1996). The SWISS-PROT Protein Sequence Data Bank and its New Supplement TREMBL. Nucleic Acids Res. 24, 21–25. doi:10.1093/nar/24.1.21
Berman, H. M. (2000). The Protein Data Bank. Nucleic Acids Res. 28, 235–242. doi:10.1093/nar/28.1.235
Tastan Bishop, Ö., Adebiyi, E. F., Alzohairy, A. M., Everett, D., Ghedira, K., Ghouila, A., et al. (2015). Bioinformatics Education-Pperspectives and Challenges Out of Africa. Brief. Bioinform. 16, 355–364. doi:10.1093/bib/bbu022
Cattley, S., and Arthur, J. W. (2007). BioManager: the Use of a Bioinformatics Web Application as a Teaching Tool in Undergraduate Bioinformatics Training. Brief. Bioinform. 8, 457–465. doi:10.1093/bib/bbm039
Clark, K., Karsch-Mizrachi, I., Lipman, D. J., Ostell, J., and Sayers, E. W. (2016). GenBank. Nucleic Acids Res. 44, D67–D72. doi:10.1093/nar/gkv1276
de Martel, C., Georges, D., Bray, F., Ferlay, J., and Clifford, G. M. (2020). Global burden of Cancer Attributable to Infections in 2018: a Worldwide Incidence Analysis. Lancet Glob. Heal. 8, e180–e190. doi:10.1016/S2214-109X(19)30488-7
Di Tommaso, P., Moretti, S., Xenarios, I., Orobitg, M., Montanyola, A., Chang, J.-M., et al. (2011). T-coffee: a Web Server for the Multiple Sequence Alignment of Protein and RNA Sequences Using Structural Information and Homology Extension. Nucleic Acids Res. 39, W13–W17. doi:10.1093/nar/gkr245
Ding, Y., Wang, M., He, Y., Ye, A. Y., Yang, X., Liu, F., et al. (2014). "Bioinformatics: Introduction and Methods," a Bilingual Massive Open Online Course (MOOC) as a New Example for Global Bioinformatics Education. Plos Comput. Biol. 10, e1003955. doi:10.1371/journal.pcbi.1003955
Edgar, R. C. (2004). MUSCLE: Multiple Sequence Alignment with High Accuracy and High Throughput. Nucleic Acids Res. 32, 1792–1797. doi:10.1093/nar/gkh340
Giardine, B. (2005). Galaxy: A Platform for Interactive Large-Scale Genome Analysis. Genome Res. 15, 1451–1455. doi:10.1101/gr.4086505
Gurwitz, K. T., Aron, S., Panji, S., Maslamoney, S., Fernandes, P. L., Judge, D. P., et al. (2017). Designing a Course Model for Distance-Based Online Bioinformatics Training in Africa: The H3ABioNet Experience. PLOS Comput. Biol. 13, e1005715. doi:10.1371/journal.pcbi.1005715
H3ABionet, (2021). H3ABioNet - a Pan African Bioinformatics Network for the Human Heredity and Health in Africa (H3Africa) Consortium. Available at: https://www.h3abionet.org/(Accessed May 4, 2021).
H3Africa (2021a). EANBiT Eastern Africa Network for Bioinformatics Training. Available at: http://eanbit.icipe.org/news/eastern-africa-network-%28eanbit%29-sample-%0Aarticle-1 (Accessed July 1, 2020).
H3Africa (2021b). H3Africa Human Heredity for Health Africa. Available at: https://h3africa.org/(Accessed July 1, 2020).
Harris, P. A., Taylor, R., Thielke, R., Payne, J., Gonzalez, N., and Conde, J. G. (2009). Research Electronic Data Capture (REDCap)-A Metadata-Driven Methodology and Workflow Process for Providing Translational Research Informatics Support. J. Biomed. Inform. 42, 377–381. doi:10.1016/j.jbi.2008.08.010
Harris, P. A., Taylor, R., Minor, B. L., Elliott, V., Fernandez, M., O'Neal, L., et al. (2019). The REDCap Consortium: Building an International Community of Software Platform Partners. J. Biomed. Inform. 95, 103208. doi:10.1016/J.JBI.2019.103208
Hernández-de-Diego, R., de Villiers, E. P., Klingström, T., Gourlé, H., Conesa, A., Bongcam-Rudloff, E., et al. (2017). The eBioKit, a Stand-Alone Educational Platform for Bioinformatics. PLOS Comput. Biol. 13, e1005616. doi:10.1371/journal.pcbi.1005616
Johansen Taber, K. A., Dickinson, B. D., and Wilson, M. (2014). The Promise and Challenges of Next-Generation Genome Sequencing for Clinical Care. JAMA Intern. Med. 174, 275. doi:10.1001/jamainternmed.2013.12048
Karikari, T. K., Quansah, E., and Mohamed, W. M. Y. (2015). Developing Expertise in Bioinformatics for Biomedical Research in Africa. Appl. Translational Genomics 6, 31–34. doi:10.1016/j.atg.2015.10.002
Karikari, T. K. (2015). Bioinformatics in Africa: The Rise of Ghana? Plos Comput. Biol. 11, e1004308. doi:10.1371/journal.pcbi.1004308
Kent, W. J., Sugnet, C. W., Furey, T. S., Roskin, K. M., Pringle, T. H., Zahler, A. M., et al. (2002). The Human Genome Browser at UCSC. Genome Res. 12, 996–1006. doi:10.1101/gr.229102
Kulkarni-Kale, U., Sawant, S., and Chavan, V. (2010). Bioinformatics Education in India. Brief. Bioinform. 11, 616–625. doi:10.1093/bib/bbq027
Kumar, S., Stecher, G., and Tamura, K. (2016). MEGA7: Molecular Evolutionary Genetics Analysis Version 7.0 for Bigger Datasets. Mol. Biol. Evol. 33, 1870–1874. doi:10.1093/molbev/msw054
Kumuthini, J., Zass, L., Zass, L., Panji, S., Salifu, S. P., Kayondo, J. K., et al. (2019). The H3ABioNet Helpdesk: an Online Bioinformatics Resource, Enhancing Africa's Capacity for Genomics Research. BMC Bioinformatics 20, 741. doi:10.1186/s12859-019-3322-3
Li, W., Cowley, A., Uludag, M., Gur, T., McWilliam, H., Squizzato, S., et al. (2015). The EMBL-EBI Bioinformatics Web and Programmatic Tools Framework. Nucleic Acids Res. 43, W580–W584. doi:10.1093/nar/gkv279
Lyantagaye, S. (2013). Current Status and Future Perspectives of Bioinformatics in Tanzania. Tanzania J. Sci. 39, 1–11. doi:10.4314/tjs.v39i1
Madeira, F., Park, Y. m., Lee, J., Buso, N., Gur, T., Madhusoodanan, N., et al. (2019). The EMBL-EBI Search and Sequence Analysis Tools APIs in 2019. Nucleic Acids Res. 47, W636–W641. doi:10.1093/nar/gkz268
Mashima, J., Kodama, Y., Fujisawa, T., Katayama, T., Okuda, Y., Kaminuma, E., et al. (2017). DNA Data Bank of Japan. Nucleic Acids Res. 45, D25–D31. doi:10.1093/nar/gkw1001
Matovu, E., Bucheton, B., Chisi, J., Enyaru, J., Hertz-Fowler, C., Koffi, M., et al. (2014). Enabling the Genomic Revolution in Africa. Sci. (80- 344, 1346–1348. doi:10.1126/science.1251546
McGinnis, S., and Madden, T. L. (2004). BLAST: at the Core of a Powerful and Diverse Set of Sequence Analysis Tools. Nucleic Acids Res. 32, W20–W25. doi:10.1093/nar/gkh435
Mulder, N. J., Adebiyi, E., Alami, R., Benkahla, A., Brandful, J., Doumbia, S., et al. (2016a). H3ABioNet, a Sustainable Pan-African Bioinformatics Network for Human Heredity and Health in Africa. Genome Res. 26, 271–277. doi:10.1101/gr.196295.115
Mulder, N. J., Christoffels, A., de Oliveira, T., Gamieldien, J., Hazelhurst, S., Joubert, F., et al. (2016b). The Development of Computational Biology in South Africa: Successes Achieved and Lessons Learnt. PLOS Comput. Biol. 12, e1004395. doi:10.1371/journal.pcbi.1004395
Mulder, N. J., Adebiyi, E., Adebiyi, M., Adeyemi, S., Ahmed, A., Ahmed, R., et al. (2017). Development of Bioinformatics Infrastructure for Genomics Research. gh 12, 91. doi:10.1016/j.gheart.2017.01.005
Okonechnikov, K., Golosova, O., and Fursov, M. (2012). Unipro UGENE: a Unified Bioinformatics Toolkit. Bioinformatics 28, 1166–1167. doi:10.1093/bioinformatics/bts091
Ooijen, J. W. (2021). JoinMap ® 5 Software for the Calculation of Genetic Linkage Maps in Experimental Populations of Diploid Species. Available at: https://www.kyazma.nl/index.php/JoinMap/(Accessed May 4, 2021).
Ossom Williamson, P., and Minter, C. I. J. (2019). Exploring PubMed as a Reliable Resource for Scholarly Communications Services. jmla 107, 16–29. doi:10.5195/JMLA.2019.433
Pasculescu, A., Schoof, E. M., Creixell, P., Zheng, Y., Olhovsky, M., Tian, R., et al. (2014). CoreFlow: A Computational Platform for Integration, Analysis and Modeling of Complex Biological Data. J. Proteomics 100, 167–173. doi:10.1016/j.jprot.2014.01.023
R Development Core Team (2020). R: A Language and Environment for Statistical Computing. Available at: http://www.r-project.org/ (Accessed April 1, 2020).
Sangeda, R. Z., Mwakilili, A. D., Masamu, U., Nkya, S., Mwita, L. A., Massawe, D. P., et al. (2020). Baseline Evaluation of Bioinformatics Capacity in Tanzania. [Epub ahead of print]. doi:10.21203/RS.3.RS-112131/V1
Sangeda, R. Z., Mwakilili, A. D., Masamu, U., Nkya, S., Mwita, L. A., Massawe, D. P., et al. (2021). Dataset and Supplementary Materials for Baseline Evaluation of Bioinformatics Capacity in Tanzania in 2018. Mendeley Data doi:10.17632/t79ddvj48j.1
Schneider, M. V., Griffin, P. C., Tyagi, S., Flannery, M., Dayalan, S., Gladman, S., et al. (2019). Establishing a Distributed National Research Infrastructure Providing Bioinformatics Support to Life Science Researchers in Australia. Brief. Bioinform. 20, 384–389. doi:10.1093/bib/bbx071
Shoko, R., Manasa, J., Maphosa, M., Mbanga, J., Mudziwapasi, R., Nembaware, V., et al. (2018). Strategies and Opportunities for Promoting Bioinformatics in Zimbabwe. PLOS Comput. Biol. 14, e1006480. doi:10.1371/journal.pcbi.1006480
Smith, D. R. (2015). Buying in to Bioinformatics: an Introduction to Commercial Sequence Analysis Software. Brief. Bioinform. 16, 700–709. doi:10.1093/bib/bbu030
Tauch, A., and Al-Dilaimi, A. (2019). Bioinformatics in Germany: toward a National-Level Infrastructure. Brief. Bioinform. 20, 370–374. doi:10.1093/bib/bbx040
Vincent, A. T., Bourbonnais, Y., Brouard, J.-S., Deveau, H., Droit, A., Gagné, S. M., et al. (2018). Implementing a Web-Based Introductory Bioinformatics Course for Non-bioinformaticians that Incorporates Practical Exercises. Biochem. Mol. Biol. Educ. 46, 31–38. doi:10.1002/bmb.21086
Keywords: bioinformatics, Tanzania, Tanzania genome network, Tanzania society of human genomics, bioinformatics education, bioinformatics capacity
Citation: Sangeda RZ, Mwakilili AD, Masamu U, Nkya S, Mwita LA, Massawe DP, Lyantagaye SL and Makani J (2021) A Baseline Evaluation of Bioinformatics Capacity in Tanzania Reveals Areas for Training. Front. Educ. 6:665313. doi: 10.3389/feduc.2021.665313
Received: 07 February 2021; Accepted: 15 June 2021;
Published: 25 June 2021.
Edited by:
Raquel Cardoso de Melo Minardi, Minas Gerais State University, BrazilReviewed by:
Renato Augusto Corrêa Dos Santos, State University of Campinas, BrazilSabrina Silveira, Universidade Federal de Viçosa, Brazil
Copyright © 2021 Sangeda, Mwakilili, Masamu, Nkya, Mwita, Massawe, Lyantagaye and Makani. This is an open-access article distributed under the terms of the Creative Commons Attribution License (CC BY). The use, distribution or reproduction in other forums is permitted, provided the original author(s) and the copyright owner(s) are credited and that the original publication in this journal is cited, in accordance with accepted academic practice. No use, distribution or reproduction is permitted which does not comply with these terms.
*Correspondence: Raphael Zozimus Sangeda, c2FuZ2VkYUBnbWFpbC5jb20=