Effects of Gender on Basic Numerical and Arithmetic Skills: Pilot Data From Third to Ninth Grade for a Large-Scale Online Dyscalculia Screener
- 1Faculty of Technology, University of Turku, Turku, Finland
- 2Division of Social and Health Services, Helsinki, Finland
- 3Faculty of Educational Sciences, University of Helsinki, Helsinki, Finland
- 4Open University, University of Helsinki, Helsinki, Finland
- 5Department of Applied Educational Science, Umeå University, Umeå, Sweden
- 6Faculty of Education and Welfare Studies, Åbo Akademi University, Turku, Finland
In this study, we analyzed the development and effects of gender on basic number skills from third to ninth grade in Finland. Because the international comparison studies have shown slightly different developmental trends in mathematical attainment for different language groups in Finland, we added the language of education as a variable in our analysis. Participants were 4,265 students from third to ninth grade in Finland, representing students in two national languages (Finnish, n = 2,833, and Swedish, n = 1,432). Confirmatory factor analyses showed that the subtasks in the dyscalculia screener formed two separate factors, namely, number-processing skills and arithmetic fluency. We found a linear development trend across age cohorts in both the factors. Reliability and validity evidence of the measures supported the use of these tasks in the whole age group from 9 to15 years. In this sample, there was an increasing gender difference in favor of girls and Swedish-speaking students by grade levels in number-processing skills. At the same time, boys showed a better performance and a larger variance in tasks measuring arithmetic fluency. The results indicate that the gender ratio within the group with mathematical learning disabilities depends directly on tasks used to measure their basic number skills.
Introduction
The easy access to the internet and computer technology is changing the way we assess mathematical learning disabilities (MLD). There is a long history of using computerized tasks to assess numerical skills in research. In addition, many international and national assessments, such as OECD PISA studies, are nowadays conducted online. However, a transformation of this research into practical diagnostic tools for clinical educational psychology is still in its infancy (Conole and Warburton, 2005; Räsänen et al., 2015; Molnár andCsapó, 2019; Räsänen et al., 2019).
It has been shown repeatedly that basic number skills form the foundations for learning more complex mathematical skills (Butterworth, 2005; Jordan et al., 2009; Li et al., 2018), and early numerical skills predict later achievement in mathematics (Zhang et al., 2017; Blume et al., 2021). Furthermore, research has shown that weak basic numerical skills form the core deficit in MLD in groups of younger and older students (De Smedt et al., 2013; Zhang et al., 2017). Therefore, assessment of basic numerical skills should be part of every clinical evaluation of MLD.
However, there is not much information on how the basic number skills develop during the school years. Halberda et al. (2012) showed that the fastest development phase in ANS (approximate number system) is between 11 to 16 years of age, not at a younger age range, as expected from such a fundamental skill. ANS, which is typically measured with nonsymbolic number comparison tasks, has a small but significant correlation with mathematical skills in all age groups. However, this skill does not seem to be a reliable task to differentiate children with and without MLD in groups under ten years of age (De Smedt et al., 2013). Brankaer et al. (2017) found that students’ symbolic number comparison skills improved during the whole primary school (grades 1–6) and were consistently related to students’ math performance in all grades.
There are no commonly agreed models of how the basic number skills should be defined or categorized and what tasks should be implemented into a clinical test battery. Aunio and Räsänen (2016) suggested that the core set of skills that should be measured could be clustered into four groups: number sense, counting skills, arithmetic, and understanding mathematical relations. This division did get some support from a factor analytic study with one test battery (Hellstrand et al., 2020). However, this model has not been replicated with other test batteries. Reigosa-Crespo et al. (2012), who screened MLD from over eleven thousand children from second to ninth grade with a computerized test battery, divided their tasks into two groups, namely, basic numerical skills (enumeration and number comparison) and arithmetic fluency. However, the division of tasks into these categories was not based on data analysis. What is clear is that even the basic numerical processing is made up of many different components with different developmental trajectories and relationships to arithmetic achievement (Lyons et al., 2014).
Gender
The international comparison studies on mathematical attainment have shown significant differences in mathematical performances between countries, educational cultures, types of schools, socioeconomic groups, and genders (e.g., OECD, 2019; Mullis et al., 2020). To look at the gender differences in mathematical skills, Reilly et al. (2017) analyzed the results of 45 countries from the 2011 Trends in Mathematics and Science Study (TIMSS). They found small- to medium-sized gender differences for most individual nations with a substantial variation (d =−0.60 to +0.31). The direction varies, and there seem to be no global gender differences, but gender differences seem to be immutable. These international comparison studies of attainment focus on a variety of mathematical skills, mainly concentrating on curriculum-based contents of more complex mathematics and its different applications learned following the curricular plans of the local school systems. Therefore, it is not surprising that there are significant differences between educational cultures, socioeconomic groups, and genders in mathematical skills. However, the differences in more complex skills do not directly tell us if there are differences in basic numerical skills. Surprisingly, only a few studies on the effects of cultural factors, such as language, and only slightly more about gender effects on basic numerical skills have been published.
Stereotypes that girls lack mathematical ability persist and are widely held by parents and teachers (Hyde et al., 2008). Many studies aim to find explanations for this “male advantage.” Typically, in addition to gender stereotypes, explanations for early-grade gender differences have been searched from domain-general and domain-specific cognitive variables. For example, van Tetering et al. (2019) showed that boys outperformed girls in mathematics in most grade levels within children from 7 to 12 years old. At the same time, boys also showed a better performance in spatial mental rotation skills. The authors concluded that their results “suggest that interventions that stimulate the development of spatial skills may facilitate mathematical achievements, especially of young girls” (see also Rosselli et al., 2009). Similarly, Royer et al. (1999) showed in a series of analyses that arithmetic, favoring boys, could explain the gender differences in more complex math performance.
If, for example, boys would outperform girls also in basic number skills, this would lend support to the stereotype that boys have an early cognitive advantage (such as spatial skills or arithmetic fluency) that would explain the differences in more complex mathematical skills later on. However, if there would not be differences between girls and boys on basic number skills, it would suggest that both genders are equally equipped to acquire more complex math skills (Bakker et al., 2019; Hutchison et al., 2019). The reversed results favoring girls might reflect a cognitive advantage supporting girls. For example, Wei et al. (2012) found in a study with 8- to 11-year-old Chinese children that verbal fluency explained the girls’ better arithmetic skills. The gender differences might also mean that the fundamental number skills are strongly malleable to cultural effects. The relationship between basic number skills and more complex mathematical skills may be more reciprocal than expected. The gender differences in basic number skills could also reflect how mathematical skills develop in general within each educational culture.
There is an extensive number of studies on gender differences in school-related mathematical skills. Anastasi (1958) showed that boys outperform girls in mathematics during the elementary school years with some exceptions. For example, girls excelled in computational fluency, while boys performed better on more cognitively demanding tasks such as problem-solving. The early research reviews reported consistent gender differences in mathematical achievement (Fennema, 1974; Halpern, 1986). In the 1990s, Hyde et al., 1990 showed in their extensive meta-analysis of 100 studies (over 3 million subjects) that the gender gap in mathematical achievement had diminished over time, and the recent studies have shown that in developed countries, the genders show an equal aptitude for mathematics (Hyde et al., 2008; Lindberg et al., 2010).
Recently in some OECD countries, there has been a trend that females have started to outperform males at most levels of education and are better represented in universities (OECD, 2015). In some countries, such as Finland, where we conducted this study, girls have also started to outperform boys in school mathematics at the upper grades. However, the gender gaps favoring males have persisted, for example, in average income, employment in prestigious occupations, and leadership roles (CEDA, 2013; Goldin, 2014). Likewise, even though the gender gap in educational achievements would have narrowed or even reversed, the differences, in favor of men, have remained in self-concept and self-promotion (Parker et al., 2018). These last-mentioned noncognitive factors may affect the career choices to STEM disciplines (O’Dea et al., 2018).
Cross-cultural studies have shown that even though there would be differences in school mathematics, there would be no systematic gender differences in basic numerical or calculation skills in younger age groups (Geary et al., 1996; Aunio et al., 2006). Geary, with his colleagues, tested children from kindergarten through third grade from China and the United States using single-digit addition and found no gender effects on the accuracy of performance in either country. Shen et al. (2016) compared arithmetic skills of 7 year-olds in three countries finding that the gender differences varied from one country to another. In simple arithmetic tasks, the gender differences were visible in the strategies but not in the accuracy. In more complex tasks, the gender effect varied by country, reflecting that the educational context may play a role in gender differences in mathematics (Shen et al., 2016).
Hutchison et al. (2019) were the first to publish a systematic large-scale study on gender differences at school-age in tasks measuring basic number skills. They studied 6- to 13-year-old children (grades 1–6) with a large battery of tasks in seven different primary schools in Netherlands. The tasks to measure the basic number skills were similar to those typically used in studies aiming to grasp the fundamental features of MLD (Bartelet et al., 2014; Lyons et al., 2014). They summarized their results to “provide strong evidence of gender similarities on the majority of basic numerical tasks measured, suggesting that a male advantage in foundational numerical skills is the exception rather than the rule.” Moreover, they concluded that this is strong support for the idea that boys and girls are equally equipped with basic numerical competencies and should be equally capable of acquiring complex mathematical skills. Kersey et al. (2018) came to the same conclusion in their large-scale analysis of gender differences. They used different datasets of basic numerical skills collected in different studies of children from 6 months to 8 year-olds.
The older studies that reported gender differences in tasks measuring basic number skills had very mixed results. Krinzinger et al. (2012) studied children at primary school and found that there was a gender difference favoring boys on single- and especially on multi-digit number comparison, while another study (Wei et al., 2012) found an opposite result with a similar task and an eight times larger sample (N = 1,156). Rosselli et al. (2009) did not find any gender differences in their analysis on a number comparison, reading numbers, writing numbers, and ordering numbers in a sample of 526 7–16 year-olds.
Like mentioned earlier, the male advantage in mathematical skills has often been connected to spatial skills (van Tetering et al., 2019). There is strong evidence of male advantage in some aspects of spatial cognition (Halpern et al., 2007; Levine et al., 2016). Spatial skills have been shown to explain mathematical skills (Resnick et al., 2019), as well as the development of mathematical skills (Zhang et al., 2017). Therefore, it is not a surprise to find male advantage in numerical tasks that are based on spatial representations of numbers, such as the SNARC effect (Spatial Numerical Association of Response Codes) and number line estimation tasks. Boys seem to show a larger SNARC effect (Bull et al., 2013), and their estimations are more accurate in a number line estimation task (Thompson and Opfer, 2008; Gunderson et al., 2012; Bull et al., 2013; Reinert et al., 2016). When moving away from spatial numerical tasks to symbolic tasks, the picture of gender differences or similarities becomes blurry. Bull, Cleland, and Mitchell (2013) studied an adult sample. They found that males were faster in discriminating between two numbers and that only females displayed a numerical distance effect (logarithmic vs. linear representation). They suggested that males would have a more accurate representation of number/magnitude, which helps them discriminate between numbers closer to each other. However, studies with children have not replicated this finding with similar types of number comparison tasks (Wei et al., 2012; Krinzinger et al., 2012; Lyons et al., 2015). One factor that may explain the differences between studies is the large variety in the tasks used to assess the gender differences. Another confounding factor is the difference in the age groups of the studies. The studies that showed conflicting results focused mainly on children between the ages of 6–10 years.
Hutchison et al. (2019) analyzed gender differences in a study with children from first to sixth grade (7–13 year-olds, N = 1,463). Their test battery consisted of two numerical comparison tasks (symbolic and nonsymbolic), two matching tasks (visual and auditory), number line estimation, numerical ordering, counting, and two arithmetic tasks (addition/subtraction, multiplication/division). The only systematic gender effect found was in a number line estimation task, where the effect was strong at the early grades but disappeared at the sixth grade. The gender similarity was a systematic finding in their study.
Thus far, Reigosa-Crespo et al. (2012) had the most extensive sample to measure basic numerical and arithmetic skills. They screened over eleven thousand children from second to ninth grade. Unfortunately, they did not report the gender differences directly, but only the ratios within the low- and high-performing groups in the whole sample. They divided their tasks into two subskills: basic numerical skills (enumeration and number comparison) and arithmetic fluency. They found a higher prevalence of boys than girls at the lower end of efficiency in the basic numerical skills. Boys were two times more likely to have a deficit in basic numerical skills compared to girls. In addition, there were four times more boys than girls in the group, which had a deficit both in basic numerical skills and arithmetical fluency. They did not find differences between genders at the higher end of efficiency in enumeration or number comparison tasks but failed to report the results on their arithmetic tasks.
Variance Ratio
While most of the studies on basic number skills find only small or nonexisting differences in means between the genders, in the context of assessing MLD, the differences in variance may be more critical because the differences in variance affect the ends of the skill distribution. There is a long history of analyzing gender differences in variance of cognitive and academic skills (Maccoby andJacklin, 1974; Feingold, 1992). Feingold (1992) summarized that males were more variable than females in quantitative ability and spatial visualization, while there were no differences in variance in verbal tests, short-term memory, abstract reasoning, and perceptual speed. Nowell and Hedges (1998), in their extensive analysis on the datasets of mathematical attainment in the US national assessment, showed that the variance ratio (VR, male variance/female variance) had not changed in mathematics from 1978 to 1994, constantly showing a larger variance for males (1.05–1.42 in mathematics in their report). While the gender gap in means seems to be closing, the variance ratio has been more stable. In the latest studies, while the gender difference in means is no longer significant, the larger male variance in mathematical skills is still found. However, the difference is not so extent that it could alone explain the overrepresentation of males in the STEM field (O’Dea et al., 2018). Interestingly, Penner and Paret (2008) showed that differences in the variance exist already at preschool age.
Therefore, even though the gender similarity hypothesis in basic numerical skills (Bakker et al., 2019; Hutchison et al., 2019) would be systematically replicated and confirmed, the differences in variance could still produce significant gender differences within the extremes. However, until now, also these results have been very mixed at the lower end of the distribution. While Reigosa-Crespo et al. (2012) reported up to four times more boys having MLD than girls, only some studies have agreed on this (Badian, 1983; Ramaa andGowramma, 2002; Barbaresi et al., 2005). Some studies have shown an equal number of genders in the group of MLD (Lewis et al., 1994; Mazzocco and Myers, 2003; Koumoula et al., 2004; Devine et al., 2013), while some studies have shown the reverse gender difference, i.e., a larger number of girls than boys with MLD (Shalev et al., 2000; Dirks et al., 2008). There is a need to look at this question at a task level whether the differences in variances are systematically similar from one task to another.
Culture and Language
Our dataset was collected in Finland. The closest comparison to our dataset where similar measures were used is Hutchison’s (2019) study conducted in Netherlands. Finland and Netherlands have both been high-performing countries in international mathematical comparison studies. There have not been significant differences in how girls and boys perform in school mathematics in these countries. For example, in the latest TIMSS study of 14–15 year-olds, in Netherlands, boys were a nonsignificant +12 points better than girls. At the same time, in Finland, there were no significant gender differences in mathematics at this age, but girls’ average was slightly above those of the boys.
Finland has two official languages, Finnish and Swedish. The Finnish-speaking schools used to perform slightly better than the Swedish-speaking schools (Kupari et al., 2012). Even though in Finland, there are no significant socioeconomic or educational differences between the schools in the system of free public education (Kupiainen et al., 2009). All children in Finland participate in the same public education offered by similarly university-trained teachers, and they all follow exactly the same national curriculum framework.
There has been a shift in mathematical attainment between the genders and between the two language groups in Finland during the last 2 decades. Today, girls perform better than boys, and the Swedish-speaking minority performs better than the Finnish-speaking majority. In the latest TIMSS 2018 study, in the fourth-grade sample, there were no differences in mathematics between the language groups nor genders (Vettenranta et al., 2020a). However, in the eighth grade, the Swedish-speaking sample was slightly better in mathematics, especially the Swedish-speaking girls (Vettenranta et al., 2020b). The trends of improvement of girls’ performance levels compared to that of boys’ and the improvement of the Swedish-speaking minority compared to the Finnish-speaking majority are also visible in the PISA data (Figure 1). In PISA data, the main effect producing these trends in Finland has been that Swedish-speaking girls are the only group that has not shown a similar constant decline in their math performance as the other groups (OECD 2015; OECD 2019).
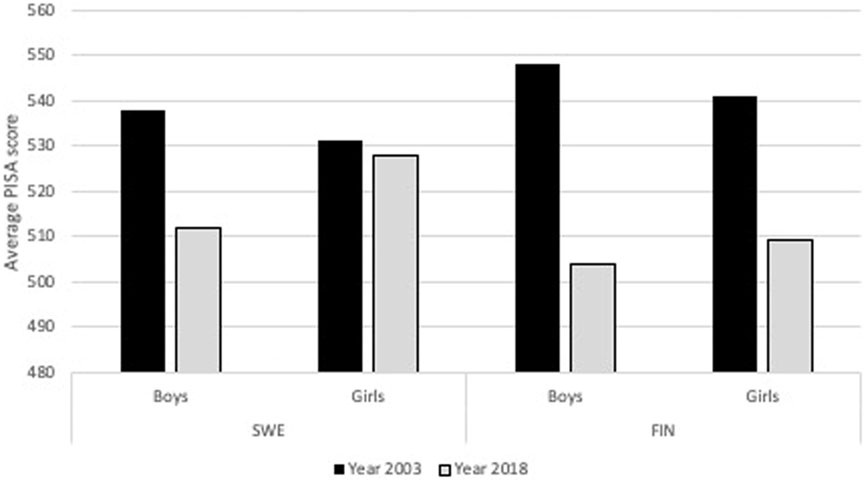
FIGURE 1. The average mathematics attainment in PISA studies of the Finnish and Swedish-speaking samples in Finland in 2003 and 2018 (Source: OECD).
Summary
In this study, we analyzed the effects of gender in basic number skills from third to ninth grade to add one educational culture, Finland, to the small number of studies looking at the gender differences in basic numerical skills. Because the international comparison studies have shown slightly different developmental trends in mathematical attainment for different language groups in Finland, we added the education language in the school as a variable into our analysis. There are two main reasons, one theoretical and one practical, why we are interested in the gender differences and the effects of the language group when we assess the basic number skills. First, the previous studies have shown very mixed results indicating no systematic differences between the genders. The most systematic study until now indicates that gender similarity is the rule and the differences an exception (Hutchison et al., 2019). However, another possible explanation for the mixed results is that there could be a reciprocal relationship between basic number skills and school mathematics. The gender differences and gender similarities in basic number skills may reflect the results of the curriculum-based assessments. Therefore, we would find increasing gender differences favoring Swedish-speaking girls in the older age groups, as has been the trend in the international and national achievement studies.
Second, a practical reason for this analysis is that our data collecting was part of a process to develop an online test battery for clinical use. This study is our first pilot to test both the online technology in practice and investigate the suitability of the tasks for the test battery for screening mathematical learning difficulties. Systematic and significant differences due to gender or language would mean that we should take these differences into account in the forthcoming standardization process of our test. Any differences in means or variation would affect the gender ratio of those diagnosed as having MLD. Substantial differences in some tasks would require us to consider providing different norms for different subgroups. From the clinical perspective, the gender differences in the extremes are even more important than the differences in means. Therefore, we also report here the gender ratios in the extreme values. The previous studies on MLD have shown all three possibilities in the gender ratios. More information is needed to see how the different tasks affect the ratio of males vs. females in the extremes. Therefore, our results will also function as information for others who aim to develop standardized test batteries for screening MLD.
To reliably compare different groups in basic numerical skills, we first need to ensure that our measure 1) shows adequate reliability, 2) structural validity, and 3) measurement invariance across groups (Finnish vs. Swedish; boys vs. girls). Hence, the analyses start with establishing reliability and validity evidence of our test battery. Second, we will look at the trends of the gender differences at different grade levels controlling for the language of instruction. Last, we will look at the variance and the gender ratio in the low- and high-performing extremes.
Materials and Methods
This study is part of a larger FUNA (Functional Numeracy Assessment) project to develop a test battery to assess basic numerical and mathematical skills (see http://oppimisanalytiikka.fi/funa). These data are from a subproject to develop a screening test battery for mathematical learning disabilities (dyscalculia). When ready, the FUNA dyscalculia battery (FUNA-DB) will consist of seven tasks measuring basic number processing and arithmetic skills. The test battery runs on an online educational platform offered to schools in Finland by the Center of Learning Analytics at the University of Turku. The system can offer the contents on an internet browser and collect all user interactions and their timings for further analysis. The system works on all operating systems and machines (computers, tablets, and mobile devices) (more information about the platform in English, see http://eduten.com).
Participants
We collected the data for this pilot study with the help of voluntary teachers and schools. Three methods were used to find volunteers: First, we held three two-day teacher training on dyscalculia, one in North, one in Central, and one in South Finland. The aim of the teacher training offered was to encourage teachers to participate in the data collecting. The teacher training consisted of two days of lectures about dyscalculia (neuropsychology and intervention methods, instructions on how to conduct the assessment and how to interpret the test results), and an assessment of classes of pupils at the schools of the participating teachers using FUNA-DB. Second, we searched for additional voluntary teachers via an advertisement in a newsletter that reaches almost all schools in Finland. Third, we took direct contacts to schools to add the number of schools to the Swedish-speaking sample.
The pupils participated in the study anonymously. The teacher informed the number of girls and boys, their grade levels, and the language of the school to our research assistant. The assistant generated an equal number of random logins/passwords that contained a hidden code for gender, grade, and language. The teacher gave these codes to the children based on their gender and grade levels. These three variables were the only pieces of information that were obtained from the children. Each teacher received feedback from the performance of each of their pupil who participated in the study. The teacher received the stanine scores based on the results of the total sample at each grade level. No other feedback or rewards were given.
The study was conducted as a collaboration with schools from tens of municipalities. Research permission and ethical approval were applied from the local educational research committee of each municipality separately. A research permit was obtained, and the participating pupils’ parents were informed about the study following the instructions and policy of each municipal school authority.
The total sample size was 4,265 pupils from third to ninth grade in two national languages (Finnish, n = 2,833, and Swedish, n = 1,432) in Finland. In Table 1, there is a summary of the number of pupils broken by grade, gender, and language.
The Tasks and the Assessments
In this pilot study, data were collected using seven tasks. However, due to an experimenter error, only six of those tasks are used in this analysis, namely, Number comparison, Digit dot matching, Number series, Single-digit addition, Single-digit subtraction, and Multi-digit calculations (addition/subtraction). In the Number series and Multi-digit Calculation tasks, there were five different parallel versions of the task, which were randomly allocated to the subjects. It means that in the same classrooms, the subjects did slightly different versions of the test batteries.
The teachers were given word-by-word instructions on how to conduct the assessments. After login the pupils were able to proceed in their own speed with the tasks without further instructions from the teacher or other interruptions. Each task started with instructions and had a practice task with 4–5 practice items before that actual task.
The median reaction times and accuracy by grade, gender, and language are presented in the supplementary materials.
Number Comparison
Two single-digit two Arabic numbers were presented on the screen, and the subject was asked to press as soon as possible the button (or key if using a computer) on the same side where the larger of the two numbers was. Each subject was shown a total of 52 items, of which ten were removed from the score calculation (items containing either 1 or 9). The remaining 42 items consisted of pairs of numbers from two to eight. The presentation order of the number pairs for each subject was fully randomized. The score used in the analysis was an efficiency score (the median reaction time of the correct responses divided by the percentage of correct responses). Split-half reliability of the task was Spearman-Brown = 0.924, Guttman split-half = 0.845.
Digit Dot Matching Task
In this equivalence task, the subjects were asked to press as fast as they could one of the two buttons (“same” or “different” or one of the two keys if using a computer) based on the equivalence of the quantities presented in the stimuli. There was an Arabic number on the left side and a randomly organized dot pattern on the right side. The matching pairs (all numbers from 1 to 9) were presented twice, and the remaining nonmatching items were divided into small-difference (e.g., 3 vs. 4) and large-difference items (e.g., 3 vs. 8). A total of 42 items were presented. The score used in the analysis was an efficiency score (the median reaction time of the correct responses divided by the percentage of correct responses). Split-half reliability of the task was Spearman-Brown = 0.756, Guttman split-half = 0.756.
Number Series
A total of 20 series of numbers were presented in order of difficulty. In each item, there were four numbers, and the subject was asked to continue the series based on the rule that the four numbers formed. There were five parallel versions of the series, each containing five same anchor items. The maximum time to solve the problems was 5 min. The score used in the analysis was an efficiency score (the median reaction time of the correct responses divided by the percentage of correct responses). Split-half reliability of the task was Spearman-Brown = 0.803, Guttman split-half = 0.707.
Single-Digit Addition
All 81 single-digit number combinations from 1 to 9 were presented to the subject as an addition (e.g., 3 + 4 = _) in a quasi-random order. There was a digital number pad on the screen which the subject could use to type in the answer (also, the number keys on a computer keyboard could be used). The subjects were instructed to answer as many items as they could during the 2 min time limit. During the last 15 s of the task, there appeared a warning about the ending of the response time. The score was the number of correct items in 2 min. Split-half reliability of the task was Spearman-Brown = 0.995, Guttman split-half = 0.995.
Single-Digit Subtraction
The reverse of the single-digit addition task was presented as subtractions (e.g., 7–3 = _; the answer of the addition task as the minuend). All 81 number combinations were presented in a quasi-random order to the subject. There was a digital number pad on the screen which the subject could use to type in the answer (also, the number keys on a computer keyboard could be used). The subjects were instructed to answer as many items as they could during the 2 min time limit. During the last 15 s of the task, there appeared a warning about the ending of the response time. The score was the number of correct items in 2 min. Split-half reliability of the task was Spearman-Brown = 0.993, Guttman split-half = 0.993.
Multi-Digit Addition and Subtraction
Five different series of addition and subtraction tasks were created from two-to four-digit numbers (e.g., 20 + 50 = _, 320–80 = _) in order of difficulty (i.e., the number of steps required to calculate the answer). Each item in the parallel versions was created to have a matching pair in the other series. Twenty out of the 80 items were anchor items across the series. The subjects were instructed to answer as fast as possible. The score was the number of correct items in 3 min. During the last 15 s of the task, there appeared a warning about the ending of the response time. Split-half reliability of the task was Spearman-Brown = 0.993, Guttman split-half = 0.992.
Statistical Analysis
The analyses were conducted with the SPSS (version 26) and Mplus (version 8.4) statistical software. The factor structure of the FUNA-DB was explored utilizing confirmatory factor analysis (CFA). More specifically, a one-factor model that assumes that all tasks load on an overall basic numerical skills factor was compared to a two-factor model consisting of a number processing factor (number comparison, digit-dot matching) and an arithmetic fluency factor (Number Series, Single-digit Addition, Single-digit Subtraction, Multi-digit Calculations). Measurement invariance was tested with multigroup CFA. In multigroup CFA, a series of nested models are fitted to the data where the endpoints are the least restrictive model with no invariance constraints and the most restrictive model where all parameters are forced to equality across groups (Bollen, 1989). In all analyses, we used the Full information maximum likelihood (FIML) that uses all available data as the estimator. We used chi-square (X2), the Comparative Fit Index (CFI), the Tucker-Lewis Index (TLI), and the Root Mean Square Error of Approximation (RMSEA) as model-fit indicators. The CFI and TLI vary along a 0-to-1 continuum, and values greater than 0.90 and 0.95 typically reflect acceptable and excellent fit to the data, respectively. RMSEA values of less than 0.05 and 0.08 reflect a close fit and a reasonable fit to the data, respectively (Marsh, Hau, and Wen, 2004). To compare nested models, we looked at the change in CFI and RMSEA (Chen, 2007). According to Chen (2007), support for the more parsimonious model requires a change in CFI (ΔCFI) of less than 0.01 or a change in RMSEA (ΔRMSEA) of less than 0.015. We used CFA with covariates to investigate the combined effect of sex, language, and grade levels on basic number skills.
To calculate the variance ratios, we used the standard scores by grade levels and then summed up the results over the grade levels. The variance ratio was calculated by dividing the male standard deviation with the female standard deviation. A larger value indicates a larger male variance.
To estimate the ratio of males and females at the ends of the distribution, low and high performers, we transformed the standard scores into Stanines (standard nine). We used the lowest and highest stanine values (1, 9) as low- and high-performance criteria. This procedure leads to groups of approximately four percentiles at both ends of the distribution.
Results
Outliers and Reliability
In the tasks where the item reaction time was used to calculate the score (Number Comparison, Digit Dot Matching, Number Series), we used three steps to clean the data. First, based on eyeballing the data, extremely long response times were deleted manually as they would have had a large impact on the mean and standard deviation of the items (e.g., there were few cases where for an unknown reason the subject had stopped answering and the response to an item was over a minute). After this, values above three standard deviations of the mean were excluded. Similarly, values under 350 ms were considered unrealistic response times and were excluded from the analyses.
The second step was to clean the cases based on accuracy. In Number Comparison and Digit Dot Matching tasks, cases with the number of correct answers within the binomial probability of guessing (p<0.05; less than 65% correct) were removed from the analysis.
The Number Series task and the three calculation tasks had an open answer field; therefore, a different procedure to remove cases was used. Cases with less than two correct answers were removed from further analysis because we could not confirm that the subject would have tried to answer the items. The reliability of the tasks was investigated with the Spearman-Brown and Guttman split-half coefficients (split-half reliability), where a value over 0.7 indicates adequate internal consistency. The descriptives are presented in Table 2. More detailed information about the performances by gender and language groups is presented in Supplementary Material.
FUNA-DB Factor Structure
The analyses started with an investigation of the factor structure of the FUNA-DB measure. First, a one-factor model where all subtasks were set to load on a basic numerical skills factor was fitted to the data, χ29) = 1,638.174, p<0.001; CFI = 0.875; TLI = 0.791; RMSEA = 0.206. This model did not fit the data very well, and modification indices indicated that the Number Comparison and Digit Dot Matching might form a separate number-processing factor while Number Series, Single-digit Addition, Single-digit Subtraction, and Multi-digit Calculations would load on a separate factor. Hence, a two-factor model with a number-processing factor and an arithmetic fluency factor was fitted to the data. This model showed good model fit and was superior compared to the one-factor model, Δχ2(1) = 1,409.114, p<0.001; ΔCFI = 0.11; ΔRMSEA = 0.13; χ2(8) = 229.060, p<0.001; CFI = 0.983; TLI = 0.968; RMSEA = 0.081.
Measurement Invariance Across Test Version, Gender, Language Group, and Grade Level
After finding the optimal factor structure, our analyses continued with multigroup CFAs to test for measurement invariance across test versions, gender, language group, and grade.
The configural model, which assumes the same factor structure but allows the factor loadings and indicator intercepts to vary across groups, was set as the baseline model in the multigroup CFAs. This model was then compared to a metric invariance (equal factor loadings) and a scalar invariance (equal factor loadings and intercepts) model. Scalar invariance was supported for test version, gender, and language group, ΔCFI<0.01; ΔRMSEA<0.015 (Table 3). Concerning the grade level, the metric model showed a worse model fit than the configural model in terms of ΔCFI = 0.017 but not according to ΔRMSEA<0.015. The scalar model also showed a worse model fit than the metric model in terms of ΔCFI = 0.027 but not according to ΔRMSEA<0.015. Likewise, the scalar model also showed an adequate model fit (Table 3). Therefore these results indicated that FUNA-DB factor scores could be compared across grades. When looking at the factor means and variances, there was a clear association with the grade level. The factor means increased with the grade level for both the number-processing factor and arithmetic fluency factor, indicating that older students had both higher number processing skills and arithmetic fluency. The variance in number-processing skills decreased when the grade level increased. The opposite pattern emerged in arithmetic fluency. It indicates that individual differences were smaller in number-processing skills and larger in arithmetic fluency in older students compared to younger students.
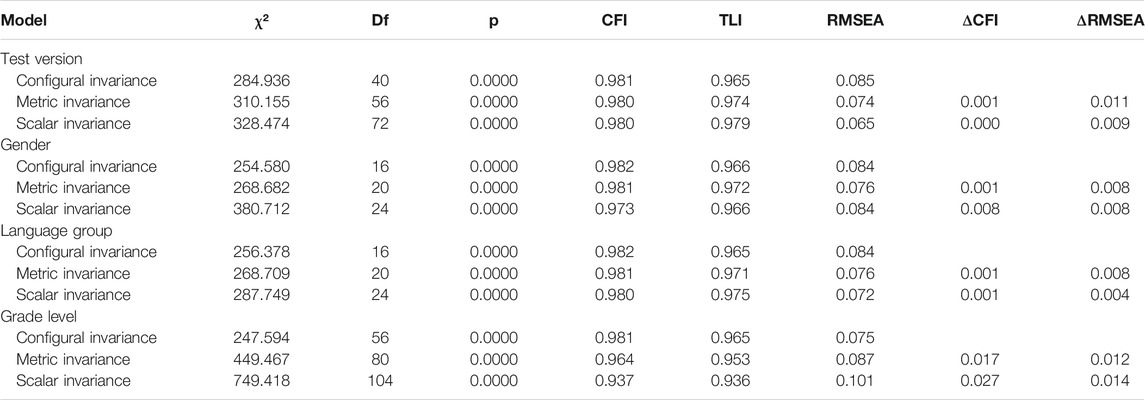
TABLE 3. Summary of goodness of fit for all models used in establishing measurement invariance across test version, gender, language group, and grade level for the dyscalculia screener (FUNA-DB).
Relating FUNA-DB Factor Scores to Gender, Language Group, and Grade Level
Next, having established measurement invariance, the FUNA-DB number-processing factor and arithmetic fluency factor were regressed on the gender, language group, and grade level, χ2(20) = 476.077, p<0.001; CFI = 0.970; TLI = 0.950; RMSEA = 0.073. This model explained 37.1% of the variance in the number-processing factor and 25.9% of the variance in the arithmetic fluency factor. Girls had better number-processing skills (β = 0.06) while boys had higher arithmetic fluency (β =-0.09). Likewise, the Swedish-speaking students had better number-processing skills (β = 0.11) and arithmetic fluency (β = 0.08). As expected, the grade level had the strongest relations to the number-processing factor (β = 0.63) and arithmetic fluency factor (β = 0.51), indicating that older students had higher scores in number-processing and arithmetic fluency tasks.
To probe for possible interaction effects between the gender, language group, and grade level, a model including interaction terms was fitted to the data, χ2(32) = 498.873, p<0.001; CFI = 0.969; TLI = 0.951; RMSEA = 0.059 (Figure 2). This model explained 37.7% of the variance in the number-processing factor and 26.0% of the variance in the arithmetic fluency factor. Gender and language groups were no longer significant predictors of number processing, but the interaction gender x grade level (β = 0.15) and language group x grade level (β = 0.20) were significant. As shown in Figure 3A, the gender difference in favor of girls increased by the grade level. Likewise, the difference between language groups in favor of Swedish-speaking students increased by the grade level (Figure 3B). Concerning arithmetic fluency, the gender and grade level were the only significant predictors, not the interaction effects.
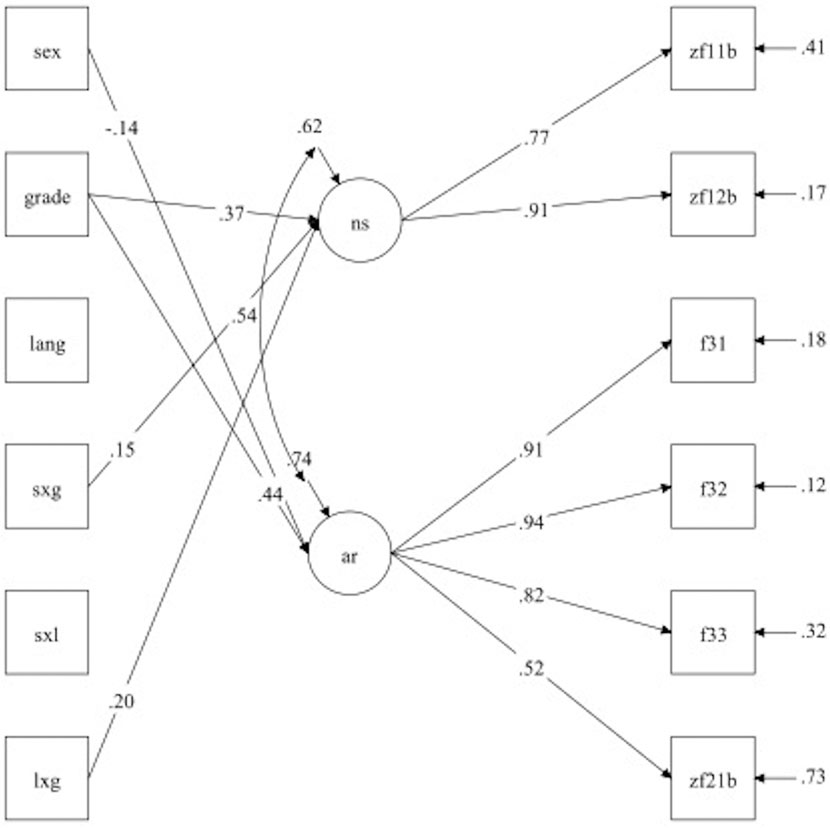
FIGURE 2. Predicting number processing and arithmetic skills with gender, language group, and grade level. Note. ns = number processing; ar = arithmetic skills; sex = gender; grade = grade level; lang = language group; sxg = sex x grade level; sxl = sex x language group; lxg = language group x grade level; zf11b = number comparison; zf12b = dot enumeration; f31 = single-digit addition; f32 = single-digit subtraction; f33 = multi-digt addition and subtraction; zf21b = arithmetic reasoning.
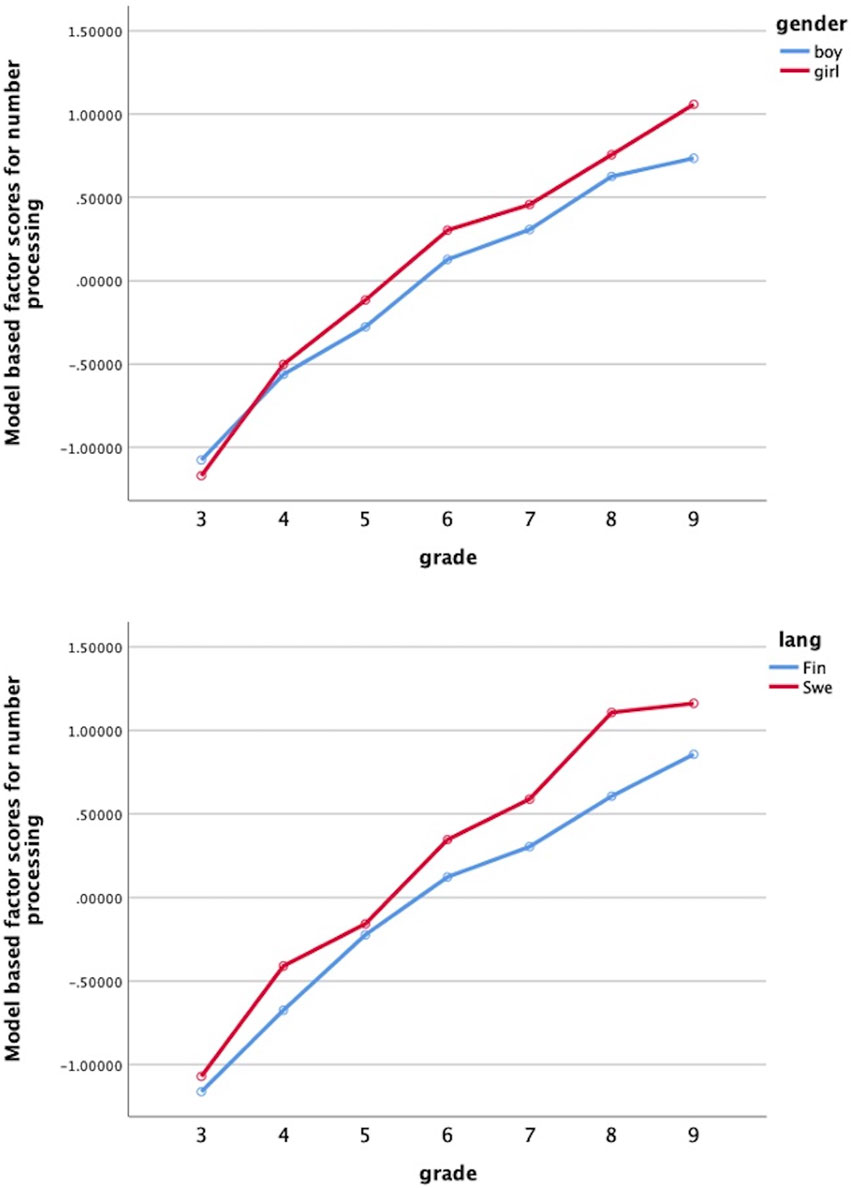
FIGURE 3. (A) The two-way interaction between gender and grade level on number processing. lang = language group, (B) The two-way interaction between language group and grade level on number processing. Note. lang = language group; Fin = Finnish-speaking students; Swe = Swedish-speaking students.
Variance Ratio and Gender Differences in the Groups With Extreme Values
We calculated standard scores for each grade level separately to analyze the means, variance, and variance ratio. The standardized means and variances are presented in Table 4.
There were systematic differences in arithmetic fluency tasks between the genders. First, boys performed better than girls (all p< 0.001), even though the effect size of this difference was small. Second, boys had a larger variance than girls, indicated by the variance ratios above VR>1.10 in all arithmetic fluency tasks (variance ratios for each task at each grade level are presented in the Supplementary Material).
The number-processing tasks behaved differently. In both the number comparison task and the digit-dot equivalence matching task, there was no systematic gender difference in the variance ratio. In the number comparison task, there was a small difference in average performance favoring boys (p. = 005), but the effect size of this difference was extremely small. The digit-dot equivalence matching task was the only task where girls performed better than boys (p< 0.001) (Table 4).
Last we looked at the gender ratios in the extreme groups. The groups were formed using the extreme Stanine groups 1 and 9, each compromising about 4 percent from the end of the distribution. In all tasks measuring Arithmetic fluency, we can find more boys than girls in the groups or very low performing as well as very high-performing pupils (Table 5), replicating the “male variance hypothesis” (all Chi-squared <0.05). However, the number-processing tasks behaved differently. In the Number Comparison task, we find more girls than boys in the group of low performers, and in the digit-dot matching task, there are more girls in the upper end of the skill distribution (all Chi-squared <0.05). Adding language into the subgrouping did not affect the results.
Discussion
The present study is the first to investigate both gender and language differences at the same time in basic number skills in a large sample and with a large age range of school-aged children. Our results showed a linear development trend in basic number skills from third to ninth grade (9–15 years old in Finland). The tasks we had selected into the test battery FUNA-DB displayed good reliability and validity evidence across grade levels. A two-factor model built from number-processing skills and arithmetic fluency was found to be invariant across test versions, gender, language groups, and grade levels, and all subtasks displayed good split-half reliability.
A two-factor model suited the data better than a one-factor of numerical skills. The subtasks Number Comparison and Digit-dot Matching loaded on a number-processing factor, and the arithmetic subtasks including a numerical reasoning task (Number series) loaded on an arithmetic fluency factor. This finding is in line with existing developmental models of mathematical skills (e.g., Krajewski and Schneider, 2009; Aunio andRäsänen, 2016; Braeuning et al., 2020) that differentiates between arithmetic skills and more basic number-processing skills.
Furthermore, these basic skills are critical indicators for mathematical learning difficulties in both younger and older children (De Smedt et al., 2013; Zhang et al., 2017). The fact that our measure was found invariant across grade levels (grades 3–9) lends support to the view that students with MLD, regardless of the grade level, have problems with these basic numerical skills. Moreover, this finding and the reliability evidence indicate that the tasks selected for the assessment can be used to evaluate basic number skills across grade levels from 3 to 9.
We found that both these basic number skills showed a linear developmental trend across cohorts from grade 3 to grade 9. Concerning arithmetic fluency, this is expected as students use and train these skills during regular math classes. The age-related improvements in number-processing skills from grade 3 to grade 9 extend the finding of Brankaer et al. (2017). They observed similar changes in their numerical magnitude comparison measure from grade 1 to grade 6. It could imply two things. First, it might mean that the precision of the neurocognitive system for numerical representations matures at least till the late teenage years. Similar results have been reported in the same age range concerning the development of nonsymbolic magnitude comparison (Halberda et al., 2012). Second, it could indicate that the relationship between number processing and more advanced mathematics content might be more reciprocal than previously expected. The relationship would not be unidirectional where more advanced mathematical skills are built on basic number skills, but that practice on curriculum-based mathematics would also affect your fluency in very basic number processing leading to linear development in basic skills from early years at least to the upper primary grades.
The observed increase in variance with the grade level has also been shown in previous studies (Aunio et al., 2004; Zhang et al., 2017). An increase in variance from one grade level to another means that the difference between low- and high-performing students increases from one year to another. This kind of “Matthew effect” has been often discussed in mathematics. However, our results showed that this effect is at least partly task-dependent phenomena. We did not find a similar increase in number processing as was found in arithmetic fluency.
The second focus of our study was on looking at the gender effects on the developmental trends in basic number skills using large cross-sectional data. In our results, gender was differentially related to number-processing skills and arithmetic fluency. In number-processing skills, there was an increasing difference between genders favoring girls and Swedish-speaking pupils. Therefore, our results with tasks measuring number processing were more in accordance with the results of the mathematical achievement studies (Figure 1).
A systematic, but weak language-effect has been found in dual-digit comparison tasks (Nuerk et al., 2005). Moeller et al. (2015) showed that in dual-digit Arabic number comparison task, there is a systematic effect how the verbal structure of naming the numbers affects processing them. Finnish and Swedish share the same decade-unit structure in their verbal number system. Likewise, Pletzer et al. (2013) have shown a small gender difference in dual-digit comparison tasks, based on gender differences in global/local strategies. Adding a dual-digit comparison task into our battery would make it stronger to identify these effects in studies with multiple languages, and especially between languages with different structures of verbal number systems (e.g. Finnish vs. German). However, our number-processing tasks used only one-digit numbers. The Swedish number words are slightly shorter than Finnish words, but if that would have produced an effect, then there should have been a systematic difference from the early grades. We found a systematically increasing difference between the language groups in basic number processing, supporting our speculation that the language differences here reflect more cultural than cognitive effects.
However, the arithmetic fluency factor showed a different trend. Irrespective of grade and language, boys performed systematically better irrespective of the task measuring arithmetic fluency. We could not replicate Hutchison et al. (2019) results that gender similarity would be the dominating feature of the basic number skills. We conclude that both task-dependent and culture-dependent factors are affecting the gender similarities and differences.
The question of the reciprocal relationship between different basic number skills is interesting. A recent longitudinal study from first to sixth grade by Vanbinst et al. (2019) found that arithmetic skills predicted symbolic numerical magnitude processing longitudinally. Despite relatively high intercorrelation, these two types of factors showed different developmental trends in our cross-sectional study. A longitudinal approach is needed to confirm that the gender and language-dependent trends found in our study are not only a reflection of this specific moment of measurement. We must remember that in studies on curriculum-based mathematics, the results on gender differences have changed dramatically from 1 decade to another.
The previous studies with smaller sets of numerical tasks, smaller range of age groups, and smaller samples have shown mixed results concerning the gender differences or gender similarities. The recent studies of Bakker et al. (2019) and Hutchison et al. (2019) claimed that there would be no gender differences in basic number skills. Our question was if we can replicate their results in a different educational culture and with a wider age range, or if their and our results would reflect more the results typically found in the curriculum-based math achievement studies. Their study was conducted in the Netherlands, where there are no significant gender differences in mathematical skills at school age. Our study was conducted in Finland, where there has been a recent trend toward girls and especially Swedish-speaking girls performing better in mathematics than the other groups. Interestingly, only the number-processing factor seemed to follow similar trends as the more curriculum-based mathematical assessments. More direct studies are needed to assess the extent of reciprocity between the development of basic number skills and mathematical skills.
Like the developmental trends, the boy/girl variance ratios and ratios of girls vs. boys at the end of the distributions differed in the two factors. The tasks in the Arithmetic fluency factor followed the typical “male variance hypothesis,” showing larger variance for boys than for girls. These values are very close to those presented by Nowell and Hedges (1998) in their analysis of gender variance from the dataset extending fifty years back. Our study is in line with the findings that even though the differences in means between the genders have mostly vanished during the last decades, the differences in variances have not. However, we found that this is also task-dependent because we did not find gender differences in variance in basic number-processing tasks (Number Comparison and Digit-dot Matching task).
Last we looked at gender differences in extremes via analyzing the gender ratios in the groups of low and high performers. We defined a pupil as a high or low performer if they belonged to the lowest or highest Stanine (standard nine) group. That means approximately four percent from both ends of the distribution. Reigosa-Crespo et al. (2012), in their large sample with similar skill factors (number processing and arithmetic), found a different ratio of boys and girls in low-performing pupils in tasks measuring number-processing skills (Number Comparison and Digit-dot Matching tasks in our study). In their study, there was twice the number of low-performing boys as girls, but they did not find any gender differences in the group of high performers. Our results did not fully replicate those results. In five out of six tasks, we found a significant overrepresentation of boys in the group of high performers (stanine class 9). Only in the Digit-dot Matching equivalence tasks, more girls showed high performance than boys. Similarly to Reigosa-Crespo’s study, there were significantly more boys at the lower end of the distribution (stanine class 1) in four of the six tasks in our sample. However, in our study, the gender difference in the low-performing group was not as marked as among the high performers. As an exception, there were more girls than boys in the group of low performers in the number comparison task.
Boys were overrepresented at both ends of the distribution in most of our tasks. It was especially clear in arithmetic tasks. Depending on the arithmetic task, there were 1.09–1.73 times more boys than girls in low performers and 1.75–2.86 times more boys in the high-performing group. These numbers are close to those Nowell and Hedges (1998) reported from NAEP and other sizeable national level samples from the United States.
Limitations and Implications for Research and Practice
Although our measure displayed reliability and validity evidence, several limitations need to be considered when interpreting the results. First, our findings are based on cross-sectional data, and therefore we could not investigate the test–retest reliability of our measure. One important criterion for MLD is persistent low performance in mathematics (Mazzocco and Räsänen, 2013). With a longitudinal design, we could investigate the stability of MLD status with our measure. Second, we did not include other measures of mathematical skills to establish convergent validity. It would also have allowed us to see if the same children would be identified as at-risk for MLD with different math measures. Even when considering these shortcomings, our study adds to the literature by showing that it is possible to measure basic numerical skills with the same tasks across a broad age span. There seems to be a linear developmental trend in basic numerical skills from grade 3 to grade 9. Future longitudinal studies are needed to see if our results on increasing gender differences in number processing can be replicated in our and other educational cultures and if the relationships within and between basic number skills and curriculum-based math skills are reciprocal, as our data indicate.
We can only speculate on why we found an increase in girl advantage in number-processing skills by grade levels as our cross-sectional data did not allow for predictions over the grade level. One possible explanation could be that because 15-year-old girls in Finland outperform boys in curriculum-based mathematics (TIMMS 2018), this advantage would positively affect number processing (see Vanbinst et al., 2019 for a similar mechanism concerning arithmetic and basic number processing). This explanation would also fit the increasing advantage for Swedish-speaking pupils in number processing compared to Finnish-speaking students (TIMMS, 2018). However, our results with tasks measuring arithmetic fluency did not support the reciprocal development hypothesis. The results of the arithmetic fluency tasks were more in accordance with the theories of “male advantage” and “male variance hypothesis.” Additional studies are needed to analyze if domain-general cognitive factors (spatial skills, verbal fluency) could partly explain the differences in results from one task to another.
There are several practical implications from our study. The validity information and the linear developmental trend indicate that it would be possible to use the same measure as a screener across several grade levels. This is important if we want to measure the development of the pupils objectively from one grade level to another. This kind of measure makes it easier for educators to conduct systematic screening for students at-risk for MLD and follow their development. The measure might also be suitable to assess the effects of interventions for students with special needs in mathematics education. Future studies will show how well these tasks suit repeated measurements in the context of intervention effectiveness studies.
Several findings in our study were task-dependent: the trends of development, gender differences, and the gender ratios among the low and high performers. Even though this kind of findings makes it difficult to build one theoretically meaningful interpretation of the results, it informs the researchers of numerical cognition about a crucial detail: individual and group differences may be hidden if we use summary scores of multiple variables. Developmental and cognitive factors and effects from educational practices and cultural factors may differently affect different numerical tasks. More studies analyzing the development of skills in basic number processing with different types of tasks are needed.
Finally, our study showed that even within a very homogenous and equality-nurturing culture such as Finland, we can find effects from gender and language. The language effect is fascinating because the tasks used only Arabic numbers, mathematical symbols, and dot patterns as stimuli. Luckily, the online format of the test battery allows us to build collaboration for cross-cultural studies between different countries and educational cultures easily.
The validity and reliability data of the pilot study indicate that we have good grounds to continue the development of the online FUNA-DB battery to be used as a tool to detect individual differences in basic number skills in the age group from 9 to 15 years. Future studies will show how well the battery suits differentiating low performance from specific learning disabilities (Mazzocco andRäsänen, 2013) and whether our tasks are sensitive enough to detect intervention effectiveness. The pilot study results encourage us to continue to construct assessment tools that can build a bridge between empirical research and educational practice.
Data Availability Statement
The raw data supporting the conclusions of this article will be made available by the authors, without undue reservation.
Ethics Statement
The studies involving human participants were reviewed and approved by the Municipal research committees. Written informed consent from the participants’ legal guardian/next of kin was not required to participate in this study in accordance with the national legislation and the institutional requirements.
Author Contributions
PR and JK contributed equally to the task development, designing the tasks and the data collecting, data analysis and writing of the manuscript, PA, AL, AH, and EV participated in project work and writing the manuscript, JF participated in the data analysis, TR and M-JL participated in the task design, building the online assessments and building the datasets.
Funding
This research was supported by a grant from the Swedish Cultural Foundation in Finland (140884) to the research project.
Conflict of Interest
The authors declare that the research was conducted in the absence of any commercial or financial relationships that could be construed as a potential conflict of interest.
Supplementary Material
The Supplementary Material for this article can be found online at: https://www.frontiersin.org/articles/10.3389/feduc.2021.683672/full#supplementary-material
References
Aunio, P., Ee, J., Lim, S. E. A., Hautamäki, J., Luit, V., and Johannes, E. H. (2004). Young Children’s Number Sense in Finland, Hong Kong and Singapore. Int. J. Early Years Educ. 12, 196–216. doi:10.1080/0966976042000268681
Aunio, P., Niemivirta, M., Hautamäki, J., Van Luit, J. E., Shi, J., and Zhang, M. (2006). Young Children’s Number Sense in China and Finland. Scand. J. Educ. Res. 50 (5), 483–502. doi:10.1080/00313830600953576
Aunio, P., and Räsänen, P. (2016). Core Numerical Skills for Learning Mathematics in Children Aged Five to Eight Years – a Working Model for Educators. Eur. Early Child. Educ. Res. J. 24 (5), 684–704. doi:10.1080/1350293X.2014.996424
Badian, N. A. (1983). Dyscalculia and Nonverbal Disorders of Learning. Prog. Learn. disabilities 5, 235–264.
Bakker, M., Torbeyns, J., Wijns, N., Verschaffel, L., and De Smedt, B. (2019). Gender equality in 4‐to 5‐year‐old Preschoolers’Early Numerical Competencies. Develop. Sci. 22 (1), e12718. doi:10.1111/desc.12718
Barbaresi, W. J., Katusic, S. K., Colligan, R. C., Weaver, A. L., and Jacobsen, S. J. (2005). Math Learning Disorder: Incidence in a Population-Based Birth Cohort, 1976–82, Rochester, Minn. Ambul. Pediatr. 5 (5), 281–289. doi:10.1367/A04-209R.1
Bartelet, D., Ansari, D., Vaessen, A., and Blomert, L. (2014). Cognitive Subtypes of Mathematics Learning Difficulties in Primary Education. Res. Develop. Disabilities 35, 657–670. doi:10.1016/j.ridd.2013.12.010
Blume, F., Dresler, T., Gawrilow, C., Ehlis, A. C., Goellner, R., and Moeller, K. (2021). Examining the Relevance of Basic Numerical Skills for Mathematical Achievement in Secondary School Using a Within-Task Assessment Approach. Acta Psychologica 215, 103289. doi:10.1016/j.actpsy.2021.103289
Braeuning, D., Ribner, A., Moeller, K., and Blair, C. (2020). The Multifactorial Nature of Early Numeracy and its Stability. Front. Psychol. 11, 518981. doi:10.3389/fpsyg.2020.518981
Brankaer, C., Ghesquiére, P., and De Smedt, B. (2017). Symbolic Magnitude Processing in Elementary School Children: A Group-Administered Paper-And-Pencil Measure (SYMPTest). Behav. Res. Methods 49, 1361–1373. doi:10.3758/s13428-016-0792-3
Bull, R., Cleland, A. A., and Mitchell, T. (2013). Gender Differences in the Spatial Representation of Number. J. Exp. Psychol. Gen. 142, 181–192. doi:10.1037/a0028387
Butterworth, B. (2005). The Development of Arithmetical Abilities. J.Child Psychol.Psychiatry 46 (1), 3–18. doi:10.1111/j.1469-7610.2004.00374.x
Committee for Economic Development of Australia [CEDA] (2013). Women in Leadership: Understanding the Gender gap. CEDA, Available at: .http://adminpanel.ceda.com.au/folders/Service/Files/Documents/15355∼cedawiljune%202013final.pdf
Conole, G., and Warburton, B. (2005). A Review of Computer-Assisted Assessment. ALT-J 13 (1), 17–31. doi:10.1080/0968776042000339772
De Smedt, B., Noël, M. P., Gilmore, C., and Ansari, D. (2013). How Do Symbolic and Non-symbolic Numerical Magnitude Processing Skills Relate to Individual Differences in Children’s Mathematical Skills? AReview of Evidence from Brain and Behavior. Trends Neurosci. Educ. 2 (2), 48–55. doi:10.1016/j.tine.2013.06.001
Devine, A., Soltész, F., Nobes, A., Goswami, U., and Szűcs, D. (2013). Gender Differences in Developmental Dyscalculia Depend on Diagnostic Criteria. Learn. Instruction 27, 31–39. doi:10.1016/j.learninstruc.2013.02.004
Dirks, E., Spyer, G., van Lieshout, E. C., and de Sonneville, L. (2008). Prevalence of Combined reading and Arithmetic Disabilities. J.Learn. Disabilities 41 (5), 460–473. doi:10.1177/0022219408321128
Feingold, A. (1992). Sex Differences in Variability in Intellectual Abilities: A New Look at an Old Controversy. Rev. Educ. Res. 62 (1), 61–84. doi:10.3102/00346543062001061
Fennema, E. (1974). Mathematics Learning and the Genders. J. Res. Maths. Educ. 5, 126–129. doi:10.2307/748949
Geary, D. C., Bow‐Thomas, C. C., Liu, F., and Siegler, R. S. (1996). Development of Arithmetical Competencies in Chinese and American Children: Influence of Age, Language, and Schooling. Child. Develop. 67 (5), 2022–2044. doi:10.1111/j.1467-8624.1996.tb01841.x
Goldin, C. (2014). A Grand Gender Convergence: Its Last Chapter. Am. Econ. Rev. 104 (4), 1091–1119. doi:10.1257/aer.104.4.1091
Gunderson, E. A., Ramirez, G., Beilock, S. L., and Levine, S. C. (2012). The Relation between Spatial Skill and Early Number Knowledge: The Role of the Linear Number-Line. Develop. Psychol. 48, 1229. doi:10.1037/a0027433
Halberda, J., Ly, R., Wilmer, J. B., Naiman, D. Q., and Germine, L. (2012). Number Sense across the Lifespan as Revealed by aMassive Internet-Based Sample. Proc. Natl. Acad. Sci. 109 (28), 11116–11120. doi:10.1073/pnas.1200196109
Halpern, D. F., Benbow, C. P., Geary, D. C., Gur, R. C., Hyde, J. S., and Gernsbacher, M. A. (2007). The Science of Gender Differences in Science and Mathematics. Psychol. Sci. Public Interest 8, 1–51. doi:10.1111/j.1529-1006.2007.00032.x
Hellstrand, H., Korhonen, J., Räsänen, P., Linnanmäki, K., and Aunio, P. (2020). Reliability and Validity Evidence of the Early Numeracy Test for Identifying Children at Risk for Mathematical Learning Difficulties. Int. J. Educ. Res. 102, 101580. doi:10.1016/j.ijer.2020.101580
Hutchison, J. E., Lyons, I. M., and Ansari, D. (2019). More Similar ThanDifferent: Gender Differences in Children’s Basic Numerical Skills Are the Exception Not the Rule. Child. Develop. 90 (1), e66–e79. doi:10.1111/cdev.13044
Hyde, J. S., Fennema, E., and Lamon, S. J. (1990). Gender differences in mathematics performance: a meta-analysis. Psychol. Bull. 107 (2), 139–155. doi:10.1037/0033-2909.107.2.139
Hyde, J. S., Lindberg, S. M., Linn, M. C., Ellis, A. B., and Williams, C. C. (2008). Gender Similarities Characterize Math Performance. Science 321, 494–495. doi:10.1126/science.1160364
Jordan, N. C., Kaplan, D., Ramineni, C., and Locuniak, M. N. (2009). Early Math Matters: Kindergarten Number Competence and Later Mathematics Outcomes. Develop. Psychol. 45 (3), 850–867. doi:10.1037/a0014939
Kersey, A. J., Braham, E. J., Csumitta, K. D., Libertus, M. E., and Cantlon, J. F. (2018). No Intrinsic Gender Differences in Children’s Earliest Numerical Abilities. npj Sci. Learn. 3 (1), 1–10. doi:10.1038/s41539-018-0028-7
Koumoula, A., Tsironi, V., Stamouli, V., Bardani, I., Siapati, S., Graham, A., et al. (2004). An Epidemiological Study of Number Processing and Mental Calculation in Greek School children. J. Learn. Disabilities 37 (5), 377–388. doi:10.1177/00222194040370050201
Krajewski, K., and Schneider, W. (2009). Early Development of Quantity to Number-word Linkage as a Precursor of Mathematical School Achievement and Mathematical Difficulties: Findings from a Four-Year Longitudinal Study. Learn. Instruction 19, 513–526. doi:10.1016/j.learninstruc.2008.10.002
Krinzinger, H., Wood, G., and Willmes, K. (2012). What Accounts for Individual and Gender Differences in the Multi-Digit Number Processing of Primary School Children? Z. für Psychol. 220 (2), 78–89. doi:10.1027/2151-2604/a000099
Kupari, P., Vettenranta, J., and Nissinen, K. (2012). Oppijalähtöistä PedagogiikkaaEtsimään. KahdeksannenLuokanOppilaidenMatematiikanJaLuonnontieteidenOsaaminen. Koulutuksentutkimuslaitos: JyväskylänyliopistopainoAvailable at: https://ktl.jyu.fi/vanhat/julkaisut/julkaisuluettelo/julkaisut/2012/d106.
Kupiainen, S., Hautamäki, J., and Karjalainen, T. (2009). The Finnish Education System and PISA. Helsinki, Finland: Ministry of EducationAvailable at: https://julkaisut.valtioneuvosto.fi/bitstream/handle/10024/75640/opm46.pdf.
Levine, S. C., Foley, A., Lourenco, S., Ehrlich, S., and Ratliff, K. (2016). Gender Differences in Spatial Cognition: Advancing the Conversation. Wiley Interdiscip. Rev. Cogn. Sci. 7, 127–155. doi:10.1002/wcs.1380
Lewis, C., Hitch, G. J., and Walker, P. (1994). The Prevalence of Specific Arithmetic Difficulties and Specific reading Difficulties in 9‐to 10‐year‐old Boys and Girls. J.Child Psychol. Psychiatry 35 (2), 283–292. doi:10.1111/j.1469-7610.1994.tb01162.x
Li, Y., Zhang, M., Chen, Y., Deng, Z., Zhu, X., and Yan, S. (2018). Children’s Non-symbolic and Symbolic Numerical Representations and Their Associations with Mathematical Ability. Front. Psychol. 9, 1035. doi:10.3389/fpsyg.2018.01035
Lindberg, S. M., Hyde, J. S., Petersen, J. L., and Linn, M. C. (2010). New Trends in Gender and Mathematics Performance: AMeta-Analysis. Psychol. Bull. 136, 1123–1135. doi:10.1037/a0021276
Lyons, I. M., Nuerk, H., and Ansari, D. (2015). Rethinking the Implications of Numerical Ratio Effects for Understanding the Development of Representational Precision and Numerical Processing across Formats. J. Exp. Psychol. Gen. 144, 1021–1035. doi:10.1037/xge0000094
Lyons, I. M., Price, G. R., Vaessen, A., Blomert, L., and Ansari, D. (2014). Numerical Predictors of Arithmetic success in Grades 1–6. Develop. Sci. 17, 714–726. doi:10.1111/desc.12152
Maccoby, E. E., and Jacklin, C. N. (1974). The Psychology of Sex Differences. Stanford: Stanford University Press.
Mazzocco, M. M., and Myers, G. F. (2003). Complexities in Identifying and Defining Mathematics Learning Disability in the Primary School-AgeYears. Ann.dyslexia 53 (1), 218–253.
Mazzocco, M. M., and Räsänen, P. (2013). Contributions of Longitudinal Studies to Evolving Definitions and Knowledge of Developmental Dyscalculia.Trends Neuroscience Education 2 (2), 65–73. doi:10.1016/j.tine.2013.05.001
Moeller, K., Shaki, S., Göbel, S. M., and Nuerk, H. C. (2015). Language Influences Number Processing–AQuadrilingualStudy. Cognition 136, 150–155. doi:10.1016/j.cognition.2014.11.003
Molnár, G., and Csapó, B. (2019). “Technology-based Diagnostic Assessments for Identifying Early Mathematical Learning Difficulties,” in In International Handbook of Mathematical Learning Difficulties. Editors A-M. Fritz, V. Haase, and P. Räsänen (Cham: Springer), 683–707.
Mullis, I. V. S., Martin, M. O., Foy, P., Kelly, D. L., and Fishbein, B. (2020). TIMSS 2019 International Results in Mathematics and Science. Retrieved from Boston College, TIMSS & PIRLS International Study Center. Available at: https://timssandpirls.bc.edu/timss2019/international-results/
Nowell, A., and Hedges, L. V. (1998). Trends in Gender Differences in Academic Achievement from 1960 to 1994: An Analysis of Differences in Mean, Variance, and Extreme Scores. Sex roles 39 (1), 21–43. doi:10.1023/A:1018873615316
Nuerk, H. C., Weger, U., and Willmes, K. (2005). Language Effects in Magnitude Comparison: Small, but NotIrrelevant. Brain Lang. 92 (3), 262–277. doi:10.1016/j.bandl.2004.06.107
O’Dea, R. E., Lagisz, M., Jennions, M. D., and Nakagawa, S. (2018). Gender Differences in Individual Variation in Academic Grades Fail to Fit Expected Patterns for STEM. Nat. Commun. 9 (1), 1–8. doi:10.1038/s41467-018-06292-0
OECD (2019). PISA 2018 Results (Volume I): What Students Know and Can Do. Paris: PISA, OECD Publishing. doi:10.1787/5f07c754-en
OECD (2015). PISA the ABC of Gender equality in Education Aptitude, Behaviour, Confidence: Aptitude, Behaviour, Confidence. Paris: OECD Publishing. doi:10.1787/9789264229945-en
Parker, P. D., Van Zanden, B., and Parker, R. B. (2018). Girls GetSmart, Boys Get Smug: Historical Changes in Gender Differences in Math, Literacy, and Academic Social Comparison and Achievement. Learn. Instruction 54, 125–137. doi:10.1016/j.learninstruc.2017.09.002
Penner, A. M., and Paret, M. (2008). Gender Differences in Mathematics Achievement: Exploring the Early Grades and the Extremes. Soc. Sci. Res. 37 (1), 239–253. doi:10.1016/j.ssresearch.2007.06.012
Pletzer, B., Kronbichler, M., Nuerk, H. C., and Kerschbaum, H. (2013). Sex Differences in the Processing of Global vs. Local Stimulus Aspects in a Two-Digit Number Comparison Task–An fMRI Study. PloS one 8 (1), e53824. doi:10.1371/journal.pone.0053824
Ramaa, S., and Gowramma, I. P. (2002). A Systematic Procedure for Identifying and Classifying Children with Dyscalculia Among Primary School Children in India. Dyslexia 8 (2), 67–85. doi:10.1002/dys.214
Räsänen, P. (2015). “Computer-assisted interventions on basic number skills,” in The Oxford Handbook of Mathematical Cognition. Editors R. Cohen Kadosh, and A. Dowker (Oxford University Press), 745–766.
Räsänen, P., Laurillard, D., Käser, T., and von Aster, M. (2019). “Perspectives to Technology- Enhanced Learning and Teaching in Mathematical Learning Difficulties,” in International Handbook of Mathematical Learning Difficulties: From lab to classroom. Editors A. Fritz, V. Haase, and P. Räsänen (Cham: Springer International Publishing), 733–754.
Reigosa-Crespo, V., Valdés-Sosa, M., Butterworth, B., Estévez, N., Rodríguez, M., Santos, E., et al. (2012). Basic Numerical Capacities and Prevalence of Developmental Dyscalculia: the Havana Survey. Develop. Psychol. 48 (1), 123. doi:10.1037/a0025356
Reilly, D., Neumann, D. L., and Andrews, G. (2017). Investigating Gender Differences in Mathematics and Science: Results from the 2011 Trends in Mathematics and Science Survey. Res. Sci. Educ. 49 (1), 25–50. doi:10.1007/s11165-017-9630-6
Reinert, R. M., Huber, S., Nuerk, H. C., and Moeller, K. (2016). Gender Differences in Number-Line Estimation: The Role of Numerical Estimation. Br. J. Psychol. 108 (2), 334–350. doi:10.1111/bjop.12203
Resnick, I., Newcombe, N. S., and Jordan, N. C. (2019). “The Relation between Spatial Reasoning and Mathematical Achievement in Children with Mathematical Learning Difficulties,” in International Handbook of Mathematical Learning Difficulties.Editors A-M. Fritz, V. Haase, and P. Räsänen (Cham: Springer), 423–435.
Rosselli, M., Ardila, A., Matute, E., and Inozemtseva, O. (2009). Gender Differences and Cognitive Correlates of Mathematical Skills in School-AgedChildren. Child. Neuropsychol. 15, 216–231. doi:10.1080/09297040802195205
Royer, J. M., Tronsky, L. N., Chan, Y., Jackson, S. J., and Marchant, H. (1999). Math-fact Retrieval as the Cognitive Mechanism Underlying Gender Differences in Math TestPerformance. Contemp. Educ. Psychol. 24 (3), 181–266. doi:10.1006/ceps.1999.1004
Shalev, R. S., Auerbach, J., Manor, O. H. A. D., and Gross-Tsur, V. (2000). Developmental Dyscalculia: Prevalence and Prognosis. Eur. Child Adolesc. Psychiatry 9 (2), S58–S64. doi:10.1007/s007870070009
Shen, C., Vasilyeva, M., and Laski, E. V. (2016). Here, but NotThere: Cross-National Variability of Gender Effects in Arithmetic. J. Exp. Child Psychol. 146, 50–65. doi:10.1016/j.jecp.2016.01.016
Thompson, C. A., and Opfer, J. E. (2008). Costs and Benefits of Representational Change: Effects of Context on Age and Gender Differences in Symbolic Magnitude Estimation. J. Exp. Child Psychol., 101, 20–51.doi:10.1016/j.jecp.2008.02.003
van Tetering, M., van der Donk, M., De Groot, R. H. M., and Jolles, J. (2019). Sex Differences in the Performance of 7–12 Year Olds on aMental Rotation Task and the Relation with Arithmetic Performance. Front. Psychol. 10, 107.doi:10.3389/fpsyg.2019.00107
Vanbinst, K., Ghesquière, P., and De Smedt, B. (2019). Is the Long-Term Association between Symbolic Numerical Magnitude Processing and Arithmetic Bi-directional? J. Numer. Cogn. 5 (3), 358–370. doi:10.5964/jnc.v5i3.202
Vettenranta, J., Hiltunen, J., Kotila, J., Lehtola, P., Nissinen, K., Puhakka, E., et al. (2020a). PerustaidoistaVauhtiaKoulutielle: NeljännenLuokanOppilaidenMatematiikanJaLuonnontieteidenOsaaminen: KansainvälinenTIMSS 2019-tutkimusSuomessa. Jyväskylä: KoulutuksentutkimuslaitosAvailable at: https://jyx.jyu.fi/bitstream/handle/123456789/73016/1/4-TIMSS-2019%20JULKAISU.pdf.
Vettenranta, J., Hiltunen, J., Kotila, J., Lehtola, P., Nissinen, K., Puhakka, E., et al. (2020b). TulevaisuudenAvaintaidotPuntarissa: KahdeksannenLuokanOppilaidenMatematiikanJaLuonnontieteidenOsaaminen: KansainvälinenTIMSS 2019-tutkimusSuomessa. Jyväskylä: KoulutuksentutkimuslaitosAvailable at: https://jyx.jyu.fi/bitstream/handle/123456789/73019/2/8-TIMSS-2019%20JULKAISU_eidt.pdf.
Wei, W., Lu, H., Zhao, H., Chen, C., Dong, Q., and Zhou, X. (2012). Gender Differences in Children’s Arithmetic Performance Are Accounted for by Gender Differences in Language Abilities. Psychol. Sci. 23, 320–330. doi:10.1177/0956797611427168
Keywords: learning disabilities, number sense, arithmetic fluency, language, gender differences, mathematics, variance ratio, basic number skills
Citation: Räsänen P, Aunio P, Laine A, Hakkarainen A, Väisänen E, Finell J, Rajala T, Laakso M-J and Korhonen J (2021) Effects of Gender on Basic Numerical and Arithmetic Skills: Pilot Data From Third to Ninth Grade for a Large-Scale Online Dyscalculia Screener. Front. Educ. 6:683672. doi: 10.3389/feduc.2021.683672
Received: 21 March 2021; Accepted: 28 May 2021;
Published: 19 July 2021.
Edited by:
Korbinian Moeller, Loughborough University, United KingdomReviewed by:
Alberto Crescentini, University of Applied Sciences and Arts of Southern Switzerland (SUPSI), SwitzerlandAnies Al-Hroub, American University of Beirut, Lebanon
Copyright © 2021 Räsänen, Aunio, Laine, Hakkarainen, Väisänen, Finell, Rajala, Laakso and Korhonen. This is an open-access article distributed under the terms of the Creative Commons Attribution License (CC BY). The use, distribution or reproduction in other forums is permitted, provided the original author(s) and the copyright owner(s) are credited and that the original publication in this journal is cited, in accordance with accepted academic practice. No use, distribution or reproduction is permitted which does not comply with these terms.
*Correspondence: Pekka Räsänen, pjrasa@utu.fi