- 1Bremer Institut fur Produktion und Logistik (BIBA), Bremen, Germany
- 2Faculty of Production Engineering, University of Bremen, Bremen, Germany
In the industrial work context, Augmented Reality (AR) can support work processes and employees’ cognitive relief through the location-specific and context-related superimposition of real objects with virtual information. The AR-based support of industrial work processes ranges over product development, manufacturing, assembly, maintenance, and training. In all these areas, numerous location-based AR support functions are being prototypically implemented, aiming to improve work efficiency, communication in mobile work situations, or employee qualification in the work process. In contrast to the increasing number of developed AR solutions in recent years, there is no widespread use of these solutions in industrial practice. AR systems’ successful introduction is closely related to user acceptance, which has not been comprehensively considered over the system development process. In addition to improving AR hardware ergonomic features, usability or user interface design play an essential role in user acceptance. Particularly in the context of employee qualification, increasing employee engagement can be named as a success factor. Previous user studies of industrial AR systems only include individual user acceptance aspects. The use of game elements has not been widely addressed in connection with manual tasks in production environments, including AR-based assistance systems. This paper aims to examine user acceptance of industrial AR systems and the relevant factors for investigating user acceptance, e.g., ease of use or enjoyment, based on a systematic literature review. An analysis of existing review articles on industrial AR systems elaborates the current state of the art and identifies the research gap. This review of 109 scientific articles from 2011 to 2020 provides an overview of the current state of research on the inclusion of user acceptance in industrial AR systems. The identified papers from the scientific databases, Scopus, Web of Science, IEEE Xplore Digital Library, ACM Digital Library, and Science Direct, are evaluated for their relevance and selected for further analysis based on inclusion and exclusion criteria, e.g., year of publication. This review presents the current challenges regarding user acceptance of industrial AR systems and future possibilities for the comprehensive integration of user acceptance factors into the development, evaluation, and implementation process.
1 Introduction
In his 1997 survey on AR, Azuma (1997) published one of the most common definitions of AR, which does not limit itself to the use of specific AR hardware classes, such as data glasses. According to Azuma (1997), the following three characteristics define an AR system: the combination of reality with virtual content, the interaction with virtual objects in real-time, and the three-dimensional representation. Following Azuma’s definition, Fite-Georgel (2011), defines industrial AR as the use of AR technology to support an industrial process. In this context, photo-based overlays have proven useful and are therefore intentionally included in this definition.
Compared to the paper-based provision of information in the work environment, AR can reduce employees’ cognitive load by providing context-sensitive virtual information (Gattullo et al., 2020). In the industrial work context, AR can contribute to technical facts’ communication by the location-accurate overlay of real objects with virtual information and instructions. Industrial AR systems support assembly tasks’ execution and the context-sensitive provision of information in the work process. Besides, AR provides data visualization and interaction with this data in the function of a human-machine interface. Typical industrial applications include indoor navigation and support for maintenance, quality control, or materials management (Fraga-Lamas et al., 2018). User support through AR systems includes planning, design, ergonomic assessment, provision of operational support, or training (Jetter et al., 2018).
Across all use cases, industrial AR systems’ support of work processes involves the possibility for employee training in the work environment. Examples of AR use in employee training include providing step-by-step instructions on a specific piece of equipment or machine, providing instructions depending on the employee’s level of knowledge, or passing knowledge from experienced employees to less experienced employees by integrating this knowledge into AR solutions (Fraga-Lamas et al., 2018). With the qualification and support of employees in the work process, the use of gamification offers an opportunity to increase employee motivation. However, in the context of AR systems, no standards have yet emerged for the use and design of game elements. Further research into user acceptance of the systems used and their design for the work environment is highly relevant here (Bright and Ponis, 2021).
The number of AR applications for the industrial application context has increased significantly in recent years. The increasing number of AR solutions is counterbalanced because the developed AR solutions are still not widely used in industrial practice (Palmarini et al., 2018). This is due to the complex requirements for industrial AR solutions, which, depending on the use case, can be assigned to the following areas: users, technology, work environment, guidelines, and laws, or economic factors (Lorenz et al., 2018; Gattullo et al., 2020). From the user’s perspective, acceptance of AR systems, in particular, is crucial for successful adoption (Egger and Masood, 2020). According to Dey et al. (2018), increasing usability and user experience leads to higher user acceptance of AR systems. Furthermore, in an industrial context, ergonomic factors of the AR hardware used (Egger and Masood, 2020), the perceived efficiency of the AR solution compared to the current work process (Syberfeldt et al., 2016), the design of the user interfaces (Stoltz et al., 2017) or the cognitive load of the users during system use (Egger and Masood, 2020) were found to have an impact on user acceptance. However, user acceptance has not yet been comprehensively studied in the context of industrial AR systems (Egger and Masood, 2020).
This paper aims to present the current status of user acceptance aspects in industrial AR applications. Based on the reviewed studies, the authors examine challenges associated with the investigation of user acceptance, the assignment to the phases of the development process, the industrial application fields, and the methods used. Conducting a systematic literature review ensures the repeatability of this study. The methodology for the systematic literature review described by Booth et al. (2016), was used to conduct the review. Palmarini et al. (2018), and Egger and Masood (2020), already applied this methodology for their reviews in the field of industrial AR systems, which ensures the rigorous scientific approach of this review. The review addresses developers who deal with industrial AR systems and scientists who deal with the investigation of user acceptance in different fields of application. With this review, the authors aim to give the readers an insight into the consideration of user acceptance in the context of industrial AR systems. Furthermore, the authors want to work out for the scientific target group how future user studies on the acceptance of these systems can be designed and which areas have not been investigated so far.
The remainder of the paper organizes as follows. Section 2 elaborates on user acceptance aspects in previous reviews in industrial AR systems and defines the concept of user acceptance and related methods. The following section presents the underlying methodology in detail and highlights the individual steps of the review. In Section 4, the authors present the current state of the art and subsequently derive future opportunities for integrating aspects of user acceptance into an industrial AR applications’ development process. The paper concludes with a summary and an outlook on future research directions.
2 Background
2.1 Augmented Reality
In this section, the authors present the consideration of user acceptance aspects in widely cited and recent review papers in the context of industrial AR and elaborate on the research gap for this review.
Nee et al. (2012), conducted a comprehensive review of AR applications in product design and manufacturing. The authors analyze AR hardware and software, various AR solutions in a manufacturing context, and their technical implementation. Human factors and interaction issues in this context are examined, in addition to the related technical challenges to AR systems. Nee et al. (2012) identify the development of user-friendly human-machine interfaces as a focus for future research in this area. In their view, user acceptance also plays an important role, closely related to the user interface (Nee et al., 2012).
Dey et al. (2018), conducted a comprehensive review of usability studies from 2005 to 2014, which deals with the conducted user studies of industrial AR applications. In addition to gathering performance-related metrics, these user studies focus on workload. The authors state that improvements in user experience and usability positively influence user acceptance of AR solutions. A formal user study on usability or user experience was conducted in less than 10% of all papers examined (Dey et al., 2018).
In their paper, Fraga-Lamas et al. (2018), analyze the concept of industrial AR and its applications. The authors mainly address the field of shipbuilding. Among the essential aspects for the successful development of an industrial AR application, the authors consider user perception in terms of ergonomic and aesthetic factors of the application and the user interface design in an intuitive and user-friendly way (Fraga-Lamas et al., 2018).
In their review on the use of AR solutions in the industrial application context, Bottani and Vignali (2019), identified the main application fields, described the technical implementations, and highlighted the possible uses of AR technology. They identified several studies on user acceptance, including Rapaccini et al. (2014), of a collaborative AR solution for service technicians using the Technology Acceptance Model. The authors state that in addition to the well-investigated application areas of assembly, maintenance, product design, and training/learning, the future potential lies in the closer investigation of the areas of safety, ergonomics, and remote collaboration (Bottani and Vignali, 2019).
In their review, Boboc et al. (2020), examine the application of AR, the characteristics of existing systems, and the benefits and challenges of AR use in the automotive industry. The authors note that ergonomics, human-technology interaction, human factors, user acceptance, and ethical issues increasingly play a role but do not provide more detail in their study (Boboc et al., 2020).
Egger and Masood (2020), analyze the state of research, current challenges, and future research directions for AR systems in manufacturing in their systematic review. Among the future research topics in industrial AR, the authors include the computing power of mobile AR systems, ergonomic aspects of AR hardware, and increasing the flexibility and robustness of software solutions. Furthermore, Egger and Masood (2020), identify user involvement to increase acceptance, organizational integration in terms of processes and hierarchies, and the corporate environment to adopt and implement new technologies as future research topics. The authors note that user acceptance of AR in an industrial context has not been extensively studied (Egger and Masood, 2020).
From the reviews presented, it can be deduced that user acceptance initially played a subordinate role in developing industrial AR systems due to a large number of new technical challenges. With the increasing technological maturity of industrial AR solutions, several studies aim for user-centered development. None of the existing reviews has a focus on examining user acceptance. In parallel with the development of technical prototypes of AR-based systems in the industrial work context, the possibilities for increasing employee motivation and the associated increase in engagement are being investigated. In this context, a significant improvement can be achieved by integrating gamification approaches. The research in this field is closely related to the investigation of user acceptance of the game elements used. Up to now, there has been no standardized procedure for the integration and design of gamification in the context of industrial applications, especially with regard to manual work activities (Ulmer et al., 2020). In order to increase user acceptance of AR-based applications, insights can be gained from the use of game environments that can possibly be transferred to the design of industrial AR systems (Söbke et al., 2017). However, to date, user acceptance and its integration into the development and evaluation of industrial AR solutions have not been comprehensively investigated.
2.2 User Acceptance
The introduction of new technologies and their productive use in the work context closely links to employees’ acceptance and use of these solutions (Venkatesh et al., 2003). Along with the analysis of why employees accept new technologies comes the possibility to improve the design, evaluation, and prediction of user behavior of information systems (Taherdoost, 2018). Numerous studies on individual acceptance of new information technologies have produced many models and approaches with individual acceptance by intention or use of the system, success in adoption within the organization, or task technology fit, among others, as dependent variables to determine user acceptance (Venkatesh et al., 2003).
Due to its widespread use, numerous studies, and adaptations to different industrial use cases, Davis’ Technology Acceptance Model (Davis, 1989) is considered one of the dominant models for studying user acceptance (Marangunić and Granić, 2015; Taherdoost, 2018). This model emerges from the Theory of Reasoned Action (Fishbein and Ajzen, 1975) and the Theory of Planned Behavior (Ajzen, 1985). Over the past decades, Davis’ model has been modified and extended, as, in TAM Extension (Jackson et al., 1997), TAM 2 (Venkatesh and Davis, 2000), TAM 3 (Venkatesh and Bala, 2008). Moreover, the basic constructs of TAM have been integrated into other models, such as the Unified Theory of Acceptance and Use of Technology (UTAUT) (Venkatesh et al., 2003), UTAUT 2 (Venkatesh et al., 2012), Extended IS Success Model (Sabherwal et al., 2006). The core constructs of Davis’ TAM model are “perceived usefulness” and “perceived ease of use,” which describe the degree of perceived improvement in the performance of the work task and the degree to which the user assumes that the system will be used without effort (Davis, 1989). Since the introduction of the model, the primary constructs have not changed; new factors have been identified that impact the technology acceptance in the particular application area (Marangunić and Granić, 2015).
3 Methodology
This review follows the methodology of Booth et al. (2016) that they present in their book on “systematic approaches to a successful literature review.” This methodology was also applied in the reviews of Palmarini et al. (2018); Egger and Masood (2020); and Gattullo et al. (2020) from the field of industrial AR. Therefore, we selected Booth et al. (2016) as a methodological basis, as many reviews on industrial AR have been conducted without a fundamental methodology. The seven steps of the methodology - Planning, Defining the Scope, Searching, Assessing, Synthesizing, Analyzing, and Writing - ensure this review’s reproducibility and are illustrated in Figure 1 in the context of this review.
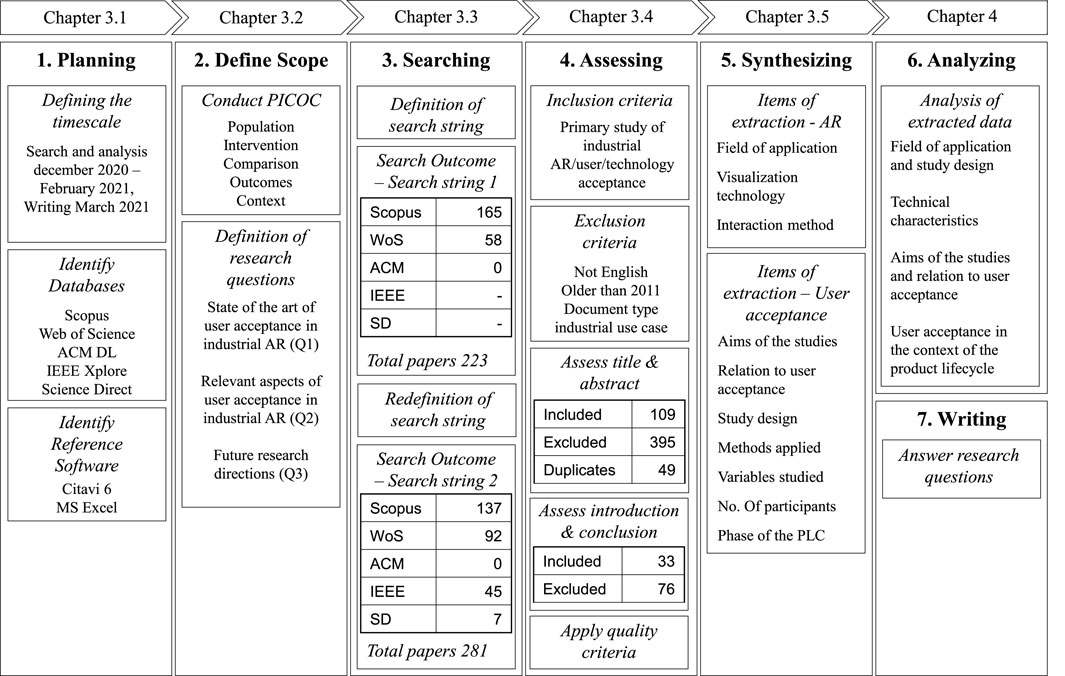
FIGURE 1. Systematic literature review based on the methodology of Booth et al. (2016).
3.1 Planning
The planning phase includes defining a time frame for conducting the review, identifying the databases, and selecting and using the software for analysis and literature management. Furthermore, we used the planning phase, following the methodology of Booth et al. (2016), for an initial literature search identifying previous review papers and their relation to user acceptance.
Booth et al. (2016) present a procedure for the systematic search of the literature according to the following five steps: 1) initial search of the literature: scoping search, 2) conduct search, 3) bibliography search, 4) verification, and 5) documentation. An initial literature search is proposed in the first phase of this methodology to get an impression of the topic and the scientific literature published on it. The results of this search are presented in Section 2.1. In addition, this search considered other reviews from the topic area of AR that were included based on the methodology used for the review (Palmarini et al., 2018) or the current findings on a continuously evolving technology (Gattullo et al., 2020). We considered the findings of key papers of industrial AR systems for the structure of this review, e.g., regarding the selection of databases and the timespan of the papers reviewed. Furthermore, we were able to derive key search terms from the existing reviews. We present the further steps of the systematic search in the context of this review in Section 3.3.
The search and analysis of scientific articles spanned December 2020-February 2021, with the seventh phase of “writing” completed in March 2021. We selected five Databases for the search of the scientific articles. These databases were selected based on the current reviews from Gattullo et al. (2020) and Boboc et al. (2020). The selection of the databases is further based on the technological reference of these databases:
• Scopus (www.scopus.com)
• Web of Science (www.webofknowledge.com)
• ACM Digital library (https://dl.acm.org/)
• IEEE Xplore Digital library (www.ieeexplore.ieee.org)
• Science Direct (www.sciencedirect.com)
We used Citavi 6 to manage the scientific sources and MS Excel additionally to analyze the sources further. Furthermore, we used MS Word and the Citavi-plugin to insert the sources.
3.2 Defining the Scope
For a systematic literature review, defining the scope and the associated formulation of research questions represents the basis of a systematic approach (Booth et al., 2016). In this review, the formal basis for formulating the research questions is the PICOC framework (Petticrew and Roberts, 2006).
The elements of the PICOC related to this review are as follows:
• Population: peer-reviewed publications on AR applications in industrial environments containing an investigation of user acceptance/technology acceptance aspects
• Intervention: collect evidence on the role of user acceptance in the conception, development, and evaluation of industrial AR; identify aspects of AR-based systems that require user acceptance
• Comparison: this does not apply because no alternative technologies or concepts are investigated
• Outcomes: state of the art of user acceptance studies and relevant aspects of user acceptance in industrial AR, approaches for a comprehensive investigation of user acceptance in the context of industrial AR
• Context: usage of AR in industrial environments
From this, we derive the following research questions for this review:
• Q1: What is the state of the art of industrial AR systems in the context of investigating user acceptance?
• Q2: What are the relevant aspects of user acceptance in the context of industrial AR systems?
• Q3: How can user acceptance be included in future studies of industrial AR?
3.3 Searching
We defined the search terms based on previous reviews of industrial AR to ensure the most comprehensive integration of all relevant contributions in this review. This corresponds to the methodological literature search according to Booth et al. (2016) as described in Section 3.1.
The execution of the search addresses the second step of the systematic literature search according to the selected methodology. In this step, we searched all previously selected databases according to the defined search criteria. The restriction of the search to the search fields “title,” “abstract,” and “keywords” follows existing reviews in the field of industrial AR (Palmarini et al., 2018; Egger and Masood, 2020). We assume that by following this procedure, we have found a representative sample of papers. Through the continuous documentation of the search and analysis results, we determined that many publications were assigned to other application fields or represented duplicates (see also the detailed representation of the literature assessment in Figure 2). The search was not modified and performed in the same way for all databases. We searched the metadata for the IEEE Xplore database because this database does not allow searching by title, abstract, and keywords. In the third step of the systematic search, we searched the bibliographies of the included papers for other relevant papers. This procedure did not immediately identify any other relevant papers but confirmed the relevance of papers already identified in the search. Based on these results, we decided in the validation step that no adjustment of the search strategies was necessary since many relevant papers are already included in the search from the authors’ point of view. The authors validated the search strategy and the identified literature by discussing the search strings and results with an expert in the field of technology acceptance of industrial applications. By performing a full-text search or considering other databases, the search results can be extended. This review limits the search to “title,” “abstract,” and “keywords.”
After defining the search string, we performed a separate search in all databases mentioned in step 1. The search string for this review is composed as follows:
Part 1 - Reference to the technology
“Augmented Reality” OR “Mixed Reality.”
Various authors have already used this combination of search terms to search for articles on AR prototypes (Gattullo et al., 2020).
Part 2: Reference to industrial applications
(“Manufact*” OR “Production” OR “Industr*” OR “industrial application*” OR “logistic*”) OR (“maintenance” OR “training” OR “quality” OR “shopfloor” OR “warehouse” OR “assembly”)
This part of the search string was adopted from Egger and Masood (2020), who derived their search from the search strings of previous review papers on industrial AR (Carmigniani et al., 2011; Nee et al., 2012).
Part 3: Reference to user evaluation
“user evaluation*” OR “user study*” OR “feedback” OR “experiment*” OR “pilot study” OR (“participant” AND “study”) OR (“participant” AND “experiment”) OR (“subject” AND “study”) OR (“subject” AND “experiment”).
We adapted this part of the search string from Dey et al. (2018), which relied on the earlier review by Dünser et al. (2008) to identify user studies in AR.
Part 4: Reference to user acceptance
“technology acceptance” OR “user acceptance” OR “usefulness” OR “ease of use” OR “enjoyment.”
We added this part of the search string based on Davis (1989), and Marangunić and Granić (2015), to reference user acceptance.
The search of each database returned the following results for this search string (see Table 1). A total of 223 papers were identified. The search was limited to journal articles, conference papers, and reviews.
The length of this search string did not allow searches in the IEEE Xplore and Science Direct databases. Therefore, we decided to perform another search with a simplified search string, searching in all databases. For this search, we simplified the existing search string as follows:
(“augmented reality” OR “mixed reality”) AND (“industrial” OR “industry”) AND (“technology acceptance” OR “user acceptance” OR “usefulness” OR “ease of use” OR “enjoyment”).
With this search string, a total of 281 titles could be found (see Table 2). We considered both search results in the subsequent steps of the assessment. The removal of duplicates was conducted in the assessment of titles and abstracts. Accordingly, we completed the entire search process with a total number of 504 titles. The search in the databases for both search strings we performed on December 8th, 2020.
3.4 Assessing
To evaluate the relevance of the sources identified by the search in the bibliographic databases for answering the research questions, we established inclusion and exclusion criteria. These criteria were part of the search settings when narrowing down the search in the databases and are subsequently applied by the authors first to the papers’ titles and abstracts and second to the papers’ introductions and conclusions. The criteria were developed following the reviews of Palmarini et al. (2018) and Egger and Masood (2020) and adapted as follows:
Inclusion Criteria
• Primary study that represents the use of AR in an industrial context
• Primary study including aspects of user or technology acceptance
Exclusion Criteria
• Not in the English language
• Older than 2011. This limitation is related to previous reviews in the field of industrial AR by Nee et al. (2012), Egger and Masood (2020). These reviews address user acceptance aspects as presented in Section 2.1. Furthermore, the selected timespan addresses the technological advancements in the field of AR. Mainly related to technological leaps of AR hardware in recent years, e.g., handhelds and head-mounted displays
• Does not fall into the document types of article, conference paper, or review
• Not related to an industrial use case
By searching the five bibliographic databases, a total of 504 papers were identified. We evaluated these papers for relevance to this review based on the title and abstract in the next step. For this purpose, we used the inclusion and exclusion criteria defined previously. In this step, we excluded only papers from the further evaluation that undoubtedly did not meet one of the criteria, such as the restriction to an industrial application. Numerous contributions were attributable, e.g., to medical applications and thus had no industrial application context.
In this step, to reduce further effort, duplicates were identified and sorted out according to the presented procedure in Figure 2. We then reviewed the introduction and conclusion of the remaining 109 papers to the criteria and identified 33 papers for further analysis. In carrying out this evaluation, the authors achieved a detailed assessment by analyzing further parts of the paper to fulfill the criteria after reviewing the introduction and conclusion, if applicable. We analyzed the papers to a level of detail that allowed an explicit inclusion or exclusion for further analysis, always starting with the introduction and conclusion. Despite all care taken in the assessment process, the authors are aware of the subjectivity of the evaluation. Nevertheless, we believe that our systematic approach based on the defined criteria has enabled a comprehensible evaluation of the papers up to this point.
For further assessment, we evaluated the identified 33 papers according to the following quality criteria, which are based on the quality criteria from Palmarini et al. (2018):
• Document is clear
• Methodology is well exposed and detailed
• Results are provided
• Relevance to research question 1: state of the art industrial AR systems in the context of user acceptance
• Relevance to research question 2: relevant aspects of industrial AR systems in terms of acceptance
• Relevance to research question 3: inclusion of user acceptance in future studies
Papers were scored 1 for completely meeting a criterion, 0.5 for partially meeting a criterion, and 0 for not meeting a criterion. For the evaluation, all 33 contributions were read and then evaluated. None of the contributions was excluded from further analysis on the basis of this subjective evaluation. The resulting evaluation of the papers was used as an orientation for the subsequent analysis, particularly with regard to the relevance of the papers to answer the research questions.
3.5 Synthesizing and Analyzing
To answer the research questions, we synthesized and analyzed the 33 remaining contributions in the next step. The development of usable AR systems is dependent on technical functionalities. Nonetheless, considering user requirements in the development is crucial for acceptance (Egger and Masood, 2020). The technical characteristics of industrial AR solutions are therefore a prerequisite for the acceptance of industrial assistance systems. For this purpose, we elaborated the technical characteristics of the contributions in the following categories. Specifically, we consider the field of application, the visualization technology, and the interaction method.
In addition, we extracted data related to user acceptance from the contributions. These are the aim of the study and the relation to user acceptance. Further data comprises the number of study participants, the phase of the study regarding the product lifecycle (PLC), the applied methods and models in this context, the results of the studies, and the resulting challenges and limitations.
3.5.1 AR-Based Data Analysis
In this section, we present the items on technological issues concerning the AR implementation that we were able to identify for each of the selected categories during the analysis. In the context of this paper, the industrial application field and the technical characteristics of the AR system concerning the user are particularly relevant. The components of an AR system include a sensor system, a tracking system, a computing unit, a visualization technology, and a user interface (Wang et al., 2016; Masood and Egger, 2019). Regarding the definition of Azuma (1997), for the perspective correct alignment of the virtual objects to reality, the position of the users to the natural environment has to be determined first. Subsequently, this position must be continuously updated with the user’s movement. The sensor system and the tracking system serve for this (Billinghurst et al., 2014). The computing unit processes the resulting data. This paper primarily addresses the interaction of the AR system with the user and the associated user acceptance of the AR system in the industrial application context. Therefore, the field of application and the components of the AR system that are in direct user contact are analyzed. These are the respective visualization technologies and the interaction methods. The interaction methods are dependent on the AR hardware used. Depending on the hardware selection as two- or three-dimensional interaction becomes possible. The requirements of the industrial use case, such as mobility, freedom of movement, or infrastructure, have a significant influence on the design of the AR system (Syberfeldt et al., 2017). For these reasons, the characteristics of an AR system that apply to the user play an essential role in designing user-friendly AR systems. The content analysis follows in the results and discussion section.
Field of Application
The following fields of application were identified in the contributions:
• Maintenance
• Training
• Assembly
• Order picking
• Other
We have assigned the contributions to one of the above areas according to their respective content focus. Some contributions consider different or several use cases. These will be discussed in more detail in the following analysis.
Visualization Technology
We have divided the visualization technologies into the following categories:
• Handheld
• Head-Mounted Display (HMD)
• Spatial AR
• Screen
The handheld category includes all end devices from the class of smartphones or tablets. HMDs include data glasses by various manufacturers, regardless of whether the virtual content is displayed binocularly or monocularly. Spatial AR addresses projection-based systems that are used mainly at assembly workstations. Some of the contributions utilize more than one technology in the context of the conducted studies; other contributions, especially the review papers, cannot be assigned to any of the technologies.
Interaction method
In the context of augmented reality, the interaction between humans and technology is strongly dependent on the hardware used. The different hardware classes offer certain interaction methods, such as hand gesture-based control, which is strongly linked to the use of HMDs. The following interaction methods could be identified in the 33 contributions:
• Object-based
• Gesture-based
• Voice-based
• Touch-based
• Projection-based
The total number of interaction methods does not correspond to the number of contributions since many of the hardware types used provide different interaction methods, or the studies considered use more than one visualization technology. As in the case of visualization technologies, some papers do not present an AR solution and are therefore not addressed in this category.
3.5.2 User Acceptance-Based Data Extraction
In the context of user acceptance, we first classified the objectives of the identified studies in terms of user acceptance. In this step, we were able to assign the contributions to the following categories:
• Consideration of user acceptance in the context of user studies
• Integration of user acceptance factors in the evaluation
• Adaptation of existing models and theories for predicting or evaluating user acceptance
User studies were conducted in 28 of the 33 identified contributions. For these contributions, we recorded the methods used to conduct the user study, the methods and theories applied from the field of user acceptance, the variables studied in relation to user acceptance, other factors considered in these studies, and the number of study participants. The results of this analysis are presented and discussed in detail in the following section.
To conclude the analysis, we examined the contributions regarding the phase of the study in relation to the PLC. This provides an assessment of the technological maturity of the solutions under consideration throughout the development process. In this context, the evaluation of a prototype is widely used. However, the methods also allow evaluation in the early stages of the development process (Dix et al., 2004). To address this question in our context, we have assigned the 28 contributions conducting user studies to the phases of the PLC according to Stark (2020):
• Ideation: Generation of ideas in the minds of the developers
• Definition: Creation of a detailed description of the product
• Realization: At the end of this phase, the product exists in its final form
• Use: The customer uses the product
• Disposal: The product is no longer used and is sent for recycling
The assignment of the contributions to the phases of the PLC is conducted in the following section. We discuss the results regarding the research questions.
4 Results and Discussion
In this section, we present the results and answer the research questions. The section begins with a categorization of the contributions as well as the user studies conducted. The studies’ objectives follow the presentation of the technical characteristics of the studied contributions in terms of investigating user acceptance. From these results, we can answer the first research question. Section 4.3 analyzes the methods used to conduct user studies and the factors studied concerning user acceptance. These results allow us to answer the second research question. Finally, we present the user studies in the context of the PLC. In conjunction with the results and limitations of the studies, we can answer the third research question.
4.1 Field of Application and Study Design
In Table 3, we assigned the papers to the fields of application described in Section 3.5.1. The study design represents the conducted user studies. Here, we could identify surveys, laboratory studies, field studies, or combined laboratory and field studies. No user studies were conducted in the four identified review papers. The paper by Quandt et al. (2018), which we have assigned to the field of maintenance, does not include a user study either. The paper examines industrial AR systems’ requirements based on the literature and two case studies. Supplementary Table S1 shows the exact assignment of the analyzed papers to the fields of applications, the type of publication, and the information on the study design.
4.1.1 Maintenance
Out of the 33 papers reviewed, we assigned eight papers to the maintenance application domain. Maintenance activities include maintenance of cranes in heavy industry (Aromaa et al., 2016), maintenance in automotive production on engines and other components (Jetter et al., 2018), or maintenance of printing machines in mobile service operations (Rapaccini et al., 2014). The studies in the field of maintenance are evenly distributed in terms of study design, with half of the AR systems tested in the field under realistic conditions.
4.1.2 Training
In the training application field, we identified eight contributions. We assigned three of these eight contributions to assembly processes training (Loch et al., 2016; Werrlich et al., 2017; Daling et al., 2020). Two of the contributions present AR-based systems for training building evacuation (Catal et al., 2019; Stigall and Sharma, 2019). Two contributions investigate systems for training maintenance processes (Helin et al., 2018; Scott et al., 2020). Beckmann et al., 2019, describe different activities in learning on-site in the heating, ventilation, and air conditioning industry. The user studies in this area were mostly conducted in the laboratory on prototype AR systems. The study by Scott et al. (2020), uses a survey to investigate technology readiness and AR and VR technology acceptance. In these studies that address user training, no game elements are directly addressed, or the use of gamification is specifically mentioned.
4.1.3 Assembly
The five contributions assigned to assembly deal with assembly assistance in the work process (Kim and Lee, 2016; Alves et al., 2019; Bosch et al., 2020; Masood and Egger, 2020; Min et al., 2020). In terms of content, these contributions extend to examining different forms of interaction (Kim and Lee, 2016) or AR-based instructions versus other forms of information preparation (Min et al., 2020). Four user studies took place in the lab, and two of the applications were tested in a real-world work environment.
4.1.4 Order Picking
In the area of order picking, we identified two contributions. The paper by Minow et al. (2020), compares two types of employee support in the picking process in a virtual environment. The contribution by Murauer (2019), uses an employee survey to investigate the acceptance for the introduction of innovations on the shop floor.
4.1.5 Other
In the category other, we have summarized all other application areas. These include indoor navigation in hazardous areas (Arntz et al., 2020), the construction sector, and the associated digitization of construction sites (Edirisinghe, 2019; Elshafey et al., 2020), or the support of electronics manufacturing with AR smart glasses (Terhoeven et al., 2018). Contributions to which no user study could be assigned include review papers that address the use of industrial AR systems in different application fields. The review by Belletier et al. (2019), addresses cognitive, social, and organizational psychology in the context of wearable cognitive systems. Boboc et al. (2020), address the state of the art of AR research in the automotive industry. The review by Bottani and Vignali (2019), addresses recent AR studies with a focus on manufacturing. These reviews were analyzed in terms of contributions to user acceptance.
4.2 Technical Characteristics
We introduced the items identified in each case in Section 3.5.1. The assignment of the technical characteristics to the individual papers is presented in the Supplementary Table S1.
4.2.1 Vizualization Technology
For the area of visualization technology, we identified eleven contributions with AR solutions on a handheld device, i.e., tablet or smartphone. Another 14 industrial AR systems were tested on a head-mounted display. The three spatial AR systems were implemented on a projector, while two of the applications were developed for a screen. A total of 30 AR devices were used in 24 identified user studies. Some of the studies were comparative, using different AR hardware types to support a work task (Aromaa et al., 2016; Helin et al., 2018; Alves et al., 2019; Daling et al., 2020; Serras et al., 2020). Some studies did not use AR hardware because they were conducted early in the product development process. We discuss these in more detail in Section 4.4.
4.2.2 Interaction Method
The interaction method is closely related to the AR hardware used, as shown earlier in Section 3.5.1. Different interaction methods can be used for each hardware type, so the number of 31 interaction forms does not correspond to the number of contributions studied. Seven of the eight identified contributions that use gesture-based control (see Figure 3) can be attributed to the use of AR data glasses (Aromaa et al., 2016; Werrlich et al., 2017; Helin et al., 2018; Quandt et al., 2018; Lee et al., 2020; Masood and Egger, 2020; Park et al., 2020). Of the eleven papers that use touch interaction, four are attributed to the use of a smartphone (Aromaa et al., 2016; Aromaa et al., 2018a; Stigall and Sharma, 2019; Min et al., 2020) and five to the use of a tablet (Kim and Lee, 2016; Aromaa et al., 2018b; Jetter et al., 2018; Catal et al., 2019; Stigall and Sharma, 2019; Daling et al., 2020). Stigall and Sharma, 2019 used both a smartphone and a tablet. Another form of interaction includes direct interaction with virtual objects, which was described in five papers (Quandt et al., 2018; Alves et al., 2019; Min et al., 2020; Minow et al., 2020; Park et al., 2020).
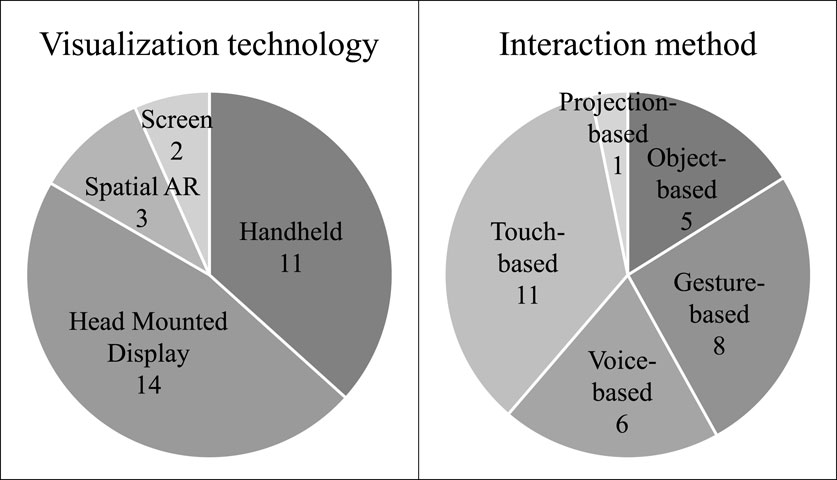
FIGURE 3. Analysis results of the contributions regarding visualization technology, interaction method.
4.3 Aims of the Studies and Relation to User Acceptance
We divided the 33 contributions into the three categories mentioned in Section 3.5.2 concerning user acceptance. These categories distinguish the consideration of user acceptance aspects in the context of user studies, the integration of user acceptance constructs in the evaluation of the systems, and the adaption of existing user acceptance models and theories.
4.3.1 Consideration of User Acceptance Aspects in User Studies
In 19 of the 33 contributions, we examined aspects of user acceptance in user studies. A distinction must be made between user studies that focus on measuring performance metrics and studies in which the models are applied to investigate user acceptance. In the following, we discuss these differences based on the factors examined. A detailed analysis of the methods used and variables examined in these studies can be found in Supplementary Table S2. Of these 19 studies, the authors used questionnaires in all user studies to obtain users’ opinions about the AR solutions tested. The studies by Alves et al. (2019), Arntz et al. (2020), and Serras et al. (2020), examined the perceived Ease of Use. Alves et al. (2019) focus on investigating performance measures in their user studies, e.g., workload, time for completion. Besides, the authors refer to the investigation of Ease of Use, which is not methodically investigated. Arntz et al. (2020) primarily investigate flow, immersion, and usability. Ease of Use is part of the applied questionnaire on the AR Applications Attitude Scale (ARAAS). Serras et al. (2020) focus on usability in their user study. The examination of Ease of Use is part of the applied usability study. In these studies, the focus is not on investigating user acceptance. User acceptance is a partial aspect of user studies primarily designed for performance but is not investigated with corresponding methods.
The studies by Aromaa et al. (2016), Aromaa et al. (2018a), Aromaa et al. (2018b), Bosch et al. (2020), Minow et al. (2020), Stigall and Sharma (2019), and Terhoeven et al. (2018), refer to Davis, 1989s TAM model for designing user questionnaires. In these studies, the factors perceived Usefulness and Ease of Use are examined. Bosch et al. (2020), does not examine either factor despite referencing the TAM model. The study by Aromaa et al. (2016), only examines the Ease of Use.
Catal et al. (2019), cite the TAM and TAM 2 models as references for their study and examine the factors perceived Usefulness and Ease of Use. Daling et al. (2020), refer to TAM 3 and include media self-efficacy and perceived enjoyment in their study in addition to the two previously mentioned factors. Lee et al. (2020), refer to TAM and UTAUT and investigate user satisfaction and behavioral intention in addition to the two factors of TAM. Loch et al. (2016), incorporate items from TAM 3 into their user questionnaire. These include perceived Ease of Use, perceived external control, and perceived enjoyment. Min et al. (2020), integrate items from UTAUT into their questionnaire. In this study, the factors performance expectancy, effort expectancy, attitude toward using, and behavioral intention to use are addressed.
In the studies by Helin et al. (2018), Masood and Egger (2020), and Park et al. (2020), user acceptance is mentioned as a factor to be investigated. These studies do not include any of the standard models in their study and do not systematically examine the factors associated with user acceptance. Most of the studies mentioned in this category examine other factors in their user studies, such as task completion time (Kim and Lee, 2016; Loch et al., 2016; Alves et al., 2019; Catal et al., 2019; Bosch et al., 2020; Masood and Egger, 2020; Min et al., 2020; Park et al., 2020), perceived usability (Aromaa et al., 2016; Helin et al., 2018; Terhoeven et al., 2018; Stigall and Sharma, 2019; Arntz et al., 2020; Bosch et al., 2020; Min et al., 2020; Minow et al., 2020; Park et al., 2020; Serras et al., 2020), or perceived workload (Loch et al., 2016; Alves et al., 2019; Bosch et al., 2020; Daling et al., 2020; Masood and Egger, 2020; Park et al., 2020).
4.3.2 Integration of User Acceptance Factors in AR System Design, Development, and Evaluation
We assigned eight papers to our second category that deal with integrating user acceptance factors in the design, development, and evaluation of AR systems. These studies investigate user acceptance factors in this context but do not include them in a user study. Beckmann et al. (2019), present an evaluation concept for on-site learning using AR and VR technologies. In this concept, the authors have integrated elements of TAM, which should be considered in the evaluation of such applications, besides usability, VR sickness, or presence.
The review by Belletier et al. (2019), investigates person-job fit in the context of wearable cognitive systems and proposes both the Job Characteristics Model and the TAM for investigation. By considering the factors of perceived Usefulness and perceived Ease of Use, the authors expect to improve user acceptance in developing and studying cognitive assistance systems in an industrial work environment.
In their review, Boboc et al. (2020), considered the state of research of AR-based systems in the automotive industry. The authors have mentioned some contributions regarding user acceptance, such as Jetter et al. (2018), or Egger and Masood (2020). Besides these contributions, which we also included in this review, the authors found that user acceptance does not significantly impact the reviewed studies.
Bottani and Vignali (2019), focus on AR studies in industry, especially in manufacturing. In their review, they note that only 18 of 67 technical papers contain a user study. For the papers with a user acceptance study, Bottani and Vignali (2019), elaborated that Ease of Use is mainly addressed regarding user acceptance and often studied together with performance measures, such as the time saved compared to other solutions or reducing the error rate.
Edirisinghe (2019), reviews the smart construction site of the future. The author addresses the importance of user acceptance as a critical factor. However, very few studies are identified that already address technology’s evaluation from the social or human perspective. Therefore, the author calls for a more intensive involvement of users in defining requirements and the development of technical assistance systems in the future.
In their paper, Masood and Egger (2019), examined the determining factors for the success of industrial AR systems. In their study, the authors surveyed 84 participants using the technology, organization, and environment model to examine the adoption and implementation of innovations in the context of AR. The study results show the importance of including organizational factors in predicting the adoption of new technologies, besides a functional technical implementation. In their qualitative analysis of the study results, Masood and Egger (2019), identify user acceptance, visibility of information, efficiency improvement, ergonomics, and usability as the most crucial success factors of industrial AR systems.
In the contribution of Quandt et al. (2018), general requirements for the development of industrial AR systems are identified and evaluated and extended based on two use cases. The authors suggest the consideration of user acceptance factors in future studies.
The paper by Werrlich et al. (2017), performs a demand analysis for AR-based training of engine assembly and maintenance in the automotive industry. The authors raise requirements for increasing the acceptance of such training assistance systems.
4.3.3 Adaption of User Acceptance Models and Theories for Industrial AR Systems
In six papers, which we assigned to our third category, we could find aspects of adaptation of existing models and theories for predicting or evaluating user acceptance in the context of industrial AR systems. Due to the direct reference of models and theories of user acceptance to industrial AR systems, we relate these approaches to the respective technical characteristics of these studies.
Elshafey et al. (2020), investigate in their paper the acceptance and adoption of AR in the context of Building Information Modelling. In a user survey based on the constructs of TAM 3, the authors identify the relevant factors of the model in relation to their use case. Their result presents the following constructs as relevant as a result of factor analysis: perceived Ease of Use, perceived Usefulness, perceived enjoyment, image, computer anxiety, job relevance, voluntariness, behavioral intention, result demonstrability, and perception of external control. This use case is not based on a concrete technical implementation of an AR system. Therefore, no connection to visualization technology and the associated interaction concepts can be made in this case.
In Jetter et al. (2018), the authors first identified KPIs for AR use in automotive maintenance. KPIs are related to TAM, and the authors identify the relevant constructs of user acceptance for this study in a questionnaire-based study. By testing the adapted model on a market-ready AR solution to support maintenance processes, the authors were able to verify the relationships between the selected TAM constructs for the chosen application context. In this case, the technical implementation of the AR system took place on a handheld (tablet) with a touch-based interaction.
The contribution of Murauer (2019), aims at increasing the acceptance of innovations in order picking by involving employees on the shop floor. For this purpose, the authors address the Diffusion of Innovation theory and interview fourteen employees regarding their requirements for AR-based systems. The results of this study show that user involvement in the adoption of new technologies has been low, and the inclusion of user requirements should be sought at an early stage of development to increase adoption. In this paper, the acceptance study refers to the use of an HMD. The study takes place before implementing an assistance system. Therefore, there is no reference to interaction forms.
Rapaccini et al. (2014), investigate the acceptance of a mobile collaborative AR system for field services maintenance based on a field study. For this purpose, the authors conducted a user survey among 65 service technicians based on the field study results with a system prototype related to TAM. The authors were able to find that behavioral intentions to use AR in field services were mostly affected by the perception of Usefulness, and only indirectly impacted by the perceived Ease of Use. According to the authors, extending the TAM-based study to include task-technology fit aspects may further increase predictive accuracy for technology use. In this paper, the AR system was implemented on an HMD. Users cannot interact with the system; the HMD is used as a pure display device in this case. The control is done remotely via a desktop computer.
Scott et al. (2020), conduct a management-level survey of 15 companies to determine the technology readiness and acceptance of AR and VR technologies to support maintenance training. This survey bases on the constructs of the UTAUT. The developed model aims to determine the contextual and organizational factors for unlocking the potential of visualization technologies for small and medium enterprises. This study did not include a technical implementation of an AR system. Therefore, no insights can be gained concerning the technical characteristics.
In their paper, Wild et al. (2017), use experts to develop an adapted model of technology acceptance for wearables and AR in the aviation and space sector. For this purpose, 15 experts first weight constructs from various user acceptance models, such as UTAUT 2 or TAM 3. From the large number of factors considered, the authors select 20 constructs from the results of this expert survey for a user questionnaire that includes the current trends for this area and contains constructs such as interoperability, learnability, and privacy as well as hedonic quality, among others. The study by Wild et al. (2017) does not include a technical implementation of an AR system. Therefore, no insights can be gained for the use of visualization technology and interaction method.
4.3.4 Summary of Interim Results in Relation to Research Questions 1 and 2
From the results presented, we have answered the first two research questions of this paper. The first question deals with the state of research regarding the investigation of user acceptance in the context of industrial AR systems. For this question, we were able to present how application contexts address user acceptance so far and what kind of studies have been conducted (see Section 4.1). Furthermore, we described the technical characteristics of these solutions in Section 4.2 that show the technical implementation of the investigated industrial AR systems. Finally, in this section, we were able to elaborate that most studies integrate aspects of user acceptance into user studies that include the collection of performance metrics, usability, or workload, among others. A smaller number of papers deal with the adaptation of technology acceptance models to introducing AR-based systems in different industrial application fields. Depending on the use case, studies address different factors of user acceptance. In four of the six identified papers, the studies on user acceptance were conducted independently of implementing an AR system. In three of the studies, the acceptance study refers to a specific visualization technology, and only one of the AR systems allows interaction by users. The identified link to the use of gamification (see Section 2.1) and the potential increase in user engagement and accentuation were not addressed in the papers studied.
The second research question addresses relevant aspects related to the study of user acceptance in the context of industrial AR systems. Numerous identified papers invoke Davis’s TAM and the two central constructs of this model, “perceived Usefulness” and “perceived Ease of Use.” These studies predominantly include questionnaires that elaborate on these constructs on user studies of AR prototypes. Some studies investigate the adaptation from the models and theories of user acceptance to industrial AR systems implementation and include additional constructs in the investigations. These model adaptations have been very few and limited to specific industrial use cases so far.
4.4 User Acceptance in the Context of the Product Lifecycle
In Table 4, we have assigned the studies to the phases of the PLC according to Stark (2020). Besides, we have sorted the papers according to the year of publication. In total, we were able to assign 29 of the 33 papers. The individual assignment of the analyzed papers to the PLC phases is presented in Supplementary Table S1. We excluded the review papers from this analysis.
4.4.1 Papers Assigned to the Realization Phase
From Table 4, we can observe a strong focus on the “realization” phase. All papers assigned to this phase deal with user testing of AR prototypes in laboratory or field tests. The studies investigate user acceptance factors in questionnaires to users, usually completed after the user tests. The results of comparative user studies establish user preference for the tested AR solution over other assistance technologies (Loch et al., 2016; Lee et al., 2020) or different AR implementation variants (Alves et al., 2019; Arntz et al., 2020). Other studies find positive user acceptance (Aromaa et al., 2016; Catal et al., 2019), good Ease of Use (Rapaccini et al., 2014; Aromaa et al., 2018a; Daling et al., 2020; Serras et al., 2020) of the tested solution. Some studies do not present substantial findings on user acceptance but announce further research in this direction (Werrlich et al., 2017; Min et al., 2020; Minow et al., 2020; Park et al., 2020). Rapaccini et al. (2014) found in their study that the behavioral intention to use the AR system in field service depends more on the perceived Usefulness than on the Ease of Use. Regarding user acceptance factors, Loch et al. (2016), concluded that there is a significant effect of Ease of Use on use intention and a strong effect of perceived enjoyment on use intention.
In addition to the studies’ results related to user acceptance, the studies identified challenges and limitations. Alves et al., 2019; Arntz et al., 2020). Other studies find positive user acceptance (Aromaa et al., 2016; Catal et al., 2019), good Ease of Use (Rapaccini et al., 2014; Aromaa et al., 2018a; Daling et al., 2020; Serras et al., 2020) of the tested solution. Some studies do not present substantial findings on user acceptance but announce further research in this direction (Werrlich et al., 2017; Min et al., 2020; Minow et al., 2020; Park et al., 2020). Rapaccini et al. (2014) found in their study that the behavioral intention to use the AR system in field service depends more on the perceived Usefulness than on the Ease of Use. Regarding user acceptance factors, Loch et al. (2016), concluded that there is a significant effect of Ease of Use on use intention and a strong effect of perceived enjoyment on use intention.
In addition to the studies’ results related to user acceptance, the studies identified challenges and limitations. Several studies identify the lack of ergonomics of the hardware used, especially in the context of smart glasses (Rapaccini et al., 2014; Daling et al., 2020; Masood and Egger, 2020). Furthermore, the studies mention physical discomfort (Lee et al., 2020; Masood and Egger, 2020) or the limited field of view as limitations in the hardware context (Alves et al., 2019; Masood and Egger, 2020). Aromaa et al. (2018a), express the following additional concerns by users about the introduction of AR-based systems in an industrial work environment: attitude toward new technology, lack of knowledge to use the system, lack of added value, language barriers, robustness in harsh environments, system complexity, location accuracy, system integration, and connectivity. Some authors state the transferability and generality of the study results as a limitation. Alves et al. (2019) find that the task’s complexity in their study does not correspond to that of an actual task in the industrial environment. Other authors point out that further studies need to be conducted in a real working environment (Loch et al., 2016; Arntz et al., 2020), or their study considered only one specific use case (Jetter et al., 2018). Therefore, some authors suggest increasing the studies’ validity by integrating further models (Rapaccini et al., 2014; Jetter et al., 2018).
4.4.2 Papers Assigned to the Definition Phase
We assigned eight studies to the “definition” phase of the PLC. Of these eight studies, five focus on the requirements for industrial AR systems in the respective application context (Werrlich et al., 2017; Quandt et al., 2018; Terhoeven et al., 2018; Murauer, 2019; Scott et al., 2020). The results of these studies highlight the importance of including user requirements and user participation in the development process for user acceptance of industrial AR systems. Terhoeven et al. (2018) identified age effects associated with a lower expectation of older employees’ perceived Usefulness. The other three papers assigned to this phase adapt models and theories of user acceptance to a specific use case to assess AR technology’s acceptance and adoption in this domain.
Wild et al. (2017), examine items from the UTAUT, TAM3, UTAUT2, and TPB models for the use of wearable technology and AR in aviation, medicine, and space. The result is a questionnaire with the 20 most relevant items for this field. This study bases on the assumption that models of technology acceptance need to be adapted to current trends. The results of this study can be summarized as follows: the authors added constructs on interoperability, learnability, and privacy to the questionnaire; hedonic quality and performance gains are important for users; there is a demand for usability testing on issues concerning increase in productivity, precision, and live feedback; the users show a lack of concern for privacy issues.
Masood and Egger (2019), use the TOE (technology, organization, and environment model) to investigate the determining factors influencing industrial AR systems’ implementation success. User acceptance, visibility of information, efficiency improvement, ergonomics, and user interface usability are the most crucial factors identified in their qualitative analysis. The authors see the technical implementation as the basis for the AR systems’ success, but the organizational aspects and the users must not be neglected.
Elshafey et al. (2020), adapt the TAM 3 for the application field construction and derive the following results. They find that perceived Usefulness is rated higher than Ease of Use regarding the intention to use the system and that perceived enjoyment directly affects Ease of Use. Further results show that job relevance has a direct influence on perceived Usefulness, and voluntariness has a direct influence on Ease of Use and Usefulness. This study’s limitation lies in the limited scope and sample size of the study, which makes results not generally applicable.
4.4.3 Summary of Interim Results in Relation to Research Question 3
From Table 4, we can infer that most of the identified studies refer only to one phase of the PLC. Only individual studies have a focus that spans multiple phases of the PLC. Rapaccini et al. (2014), test a prototype of an AR system in a field study. From the field study analysis, the authors conducted a broad user study based on the TAM. Jetter et al., 2018, investigate the relationship of KPIs for the maintenance in automotive production with user acceptance constructs. On this basis, the authors conduct a TAM-based user study. Overall, we can conclude that many of the studies do not continue. Most of the studies were published in the last 3 years due to the chosen period under consideration. However, we could also not identify continuing studies for older studies, as announced in many papers. In our view, this effect is related to the short innovation cycles of AR hardware. Of the 20 studies assigned to the realization phase, one study (Helin et al., 2018) was conducted in 2018, and six studies (Arntz et al., 2020; Daling et al., 2020; Lee et al., 2020; Masood and Egger, 2020; Park et al., 2020; Serras et al., 2020) were conducted in 2020 using Microsoft HoloLens. Due to the second version of these AR smartglasses, we do not expect these studies to continue. Despite the predominant high acceptance of the tested AR systems by users, there is no widespread adoption of AR technology in the industrial application fields. We assume that this is related to the lack of acceptance of the solutions in the companies and the lack of maturity of the available AR hardware so far.
Based on the results presented in this section, we can answer the third research question: How can user acceptance be included in future studies of industrial AR systems? The numerous studies in different industrial application fields with prototypes of AR-based systems show that user acceptance is increasingly seen as an essential factor for AR systems’ success. In these studies, the TAM application is predominant so far, other models are also mentioned, and individual factors are integrated into user questionnaires. The studies collect their findings on user acceptance factors besides other quantitative and qualitative data, such as task completion times or user experience factors. Some studies have looked at specific use cases and adapted models of user acceptance according to the requirements of these fields of application. Due to the chosen focus, these do not show general validity so far. Overall, we can state that the research community recognized the fundamental need to investigate user acceptance in the context of industrial AR systems. The selection of relevant factors is closely related to the corresponding use case. A generally applicable procedure does not yet exist.
5 Conclusion and Outlook
In this systematic review on user acceptance in the context of industrial AR systems, we identified contributions to the areas of the state of research, the state of integration of specific factors of user acceptance, and the future integration of user acceptance in the development and evaluation of industrial AR systems. This paper presents the addressed application fields and the study design of the 33 contributions. We assigned most contributions to the application fields of maintenance, training, and assembly and found that the study design mainly includes laboratory studies of system prototypes. Most of the field studies in a realistic work environment occur in the area of maintenance; some field studies occur in the assembly area. All other domains refrain from field studies of their AR systems. The user tests under laboratory conditions, which mainly occur with non-specialist personnel, confirm the results of other reviews, which also found few user tests of industrial AR systems under realistic conditions (Dey et al., 2018).
The systems’ technical characteristics show an almost even split between handhelds and HMDs in terms of the hardware used. Especially for assembly workstations, projection-based solutions are implemented. The interaction is closely related to the selected hardware. Some studies investigate different AR design options, e.g., Arntz, compared with other technical implementations compared, e.g., Minow. Only two of the considered studies investigate an interaction concept. However, in these contributions, the user acceptance’s systematic recording is not in focus (Kim and Lee, 2016; Serras et al., 2020). In the studies presented, which apply the models and theories of user acceptance to a concrete use case (Section 4.3), we found that only two of these six studies are directly related to an implemented AR system. Therefore, we see a need in the future to link these use-case-related studies to the design of an AR system. This way, insights into accepting different visualization technologies and interaction methods in concrete industrial use cases can be gained.
In 19 of the 33 investigated contributions, user acceptance is included in user studies that survey different factors, e.g., user experience, workload. In eight of the 33 contributions, the consideration of user acceptance took place in a larger context. These contributions address the general inclusion of user acceptance factors in the context of different industrial use cases. In this context, the authors note that user acceptance has not been considered in many studies to date and suggest integrating it into future studies. In six of the 33 studies, the authors adopt models and theories of technology acceptance to specific use cases. These studies build on the TAM, TAM3, Diffusion of Innovation, UTAUT, and UTAUT 2 models. The results of these studies are limited in terms of general transferability since there is a direct reference to a specific use case in each case.
We can make factual statements about the factors taken into account, particularly the questionnaire-based evaluation studies conducted. The authors predominantly integrate TAM constructs, perceived Ease of Use, and Usefulness into their studies. The adapted models address further factors, e.g., enjoyment, behavioral intention. The investigation of these factors’ general effectiveness on user acceptance for industrial AR systems in a broader application context has yet to be conducted.
From the assignment of the investigated contributions to the PLC phases, we gained the insight that only a few studies can be assigned to more than one phase of the PLC. Furthermore, studies generally do not continue after a user study has been conducted to test a prototype, regardless of positive user feedback. We suspect that this is mainly related to the short innovation cycles of AR hardware.
The systematic performance of the review ensures reproducibility. This review follows the approach developed by Booth et al. (2016) that has been applied by other reviews in the AR field (Palmarini et al., 2018; Egger and Masood, 2020) that were named in Section 3.1 as the foundation for this review. Consideration of previous reviews in the topic area during the planning phase of this review (see Section 3.1) resulted in the limitations of the review to the five selected databases and the selected period of reviewed papers. With the use of a systematic approach and the care taken in the search and evaluation of the identified articles, the authors assume that they have found a representative sample of literature. Potential limitations of the literature search and assessment are described in Section 3. The authors intentionally limited the time span of the review to a period starting in 2011 due to the rapid technological advancements in the field of AR. Due to the focus on industrial use cases, we selected appropriate databases for the search. By including other databases, such as Google Scholar https://scholar.google.com/or Ebsco (https://www.ebscohost.com/), and expanding the time span, the findings of this review can be extended. Another approach to extend the review results is to expand the search to the entire text body.
In addition, during the research, we observed that a large number of reviews have emerged in the topic area of industrial AR since 2018, as there are a large number of research studies on the topic, which are related to the current AR hardware, especially HMDs. Assuming continued high momentum of new AR hardware, many more relevant results will emerge in the following years.
To further investigate user acceptance, the studies must be continued, and further insights gained. Studies in the natural application field with real end-users can provide further insights into user acceptance of AR systems in the industrial work context. In this context, the further development of AR hardware also plays a role. So far, the ergonomics of the hardware, especially the HMD, is not yet ideal. From our perspective, further research is needed that investigates the combination of factors that allow a holistic evaluation of the use of industrial AR systems in a reasonable effort to conduct the study. The interaction between humans and technology plays an important role. In this context, user acceptance has been studied only very sporadically so far. We see a focus on future research in this area in order to be able to exploit the potential of AR technology in industrial use.
In the future, existing studies can be used as a starting point for conducting studies to investigate user acceptance in the context of industrial AR systems. The studies presented in this review take exciting approaches that can be applied to the respective industrial use cases. Jetter et al. (2018) combine the recording and analysis of performance measures, e.g., task execution times and workload, with TAM constructs. The authors combine user studies on systems performance in the industrial work context with studies on user acceptance. Other authors, e.g., Elshafey et al. (2020), Rapaccini et al. (2014), have investigated the relevance of aspects of user acceptance for a specific industrial use case and conducted user studies on technology acceptance in their application domain. Scott et al. (2020) and Wild et al. (2017) use the approach of having the constructs of different models of user acceptance first evaluated by experts on the management level for relevance to the particular use case and then applied in a corresponding study. The findings of these existing studies should be integrated. User studies could capture and analyze the relevant performance measures and user acceptance factors relevant for the specific use case. Another research direction is the transfer of the findings of existing studies to other industrial use cases. Based on the analyzed body of literature, the authors conclude that no standardized procedure has yet emerged for investigating user acceptance factors for industrial AR systems but that the approaches presented here provide a reasonable basis for further studies.
In addition, we see the need to transfer the approaches for the design and use of AR technology from other application areas, where AR is already successfully used, to the industrial use of AR. Games, in particular, can serve as a template, as they already reach many users and offer insight into which aspects play a decisive role in user acceptance of such applications. For example, Söbke et al. (2017) were able to find out from the example of the AR game Ingress that the adaptation of the game to the user’s abilities or the possibilities for social interaction plays a vital role for the user acceptance. Especially concerning the intuitive design and the ease of interaction between humans and the AR system, we want to gain insights into the design of industrial assistance systems, e.g., from the study of Harborth and Pape (2017), on user acceptance of Pokémon Go. In this context, incorporating gamification elements is also promising, as they can increase user acceptance of AR applications, as described in Section 2.1. This review could show that the integration of gamification elements in the context of industrial AR applications has received little or no attention so far. This gap should be closed by developing industrial AR systems including gamification elements and by studying the effects on user acceptance.
Author Contributions
Conceptualization, MQ and MF; methodology, MQ; formal analysis, MQ; investigation, MQ; resources, MQ; data curation, MQ; writing-original draft preparation, MQ; writing-review and editing, MF and MQ; visualization, MQ; supervision, MF.
Conflict of Interest
The authors declare that the research was conducted in the absence of any commercial or financial relationships that could be construed as a potential conflict of interest.
Publisher’s Note
All claims expressed in this article are solely those of the authors and do not necessarily represent those of their affiliated organizations, or those of the publisher, the editors and the reviewers. Any product that may be evaluated in this article, or claim that may be made by its manufacturer, is not guaranteed or endorsed by the publisher.
Acknowledgments
The authors would like to thank the German Federal Ministry of Economic Affairs and Energy (BMWi) for their support within the project “compARe – Optimization of the maintenance of wind turbines by using image processing methods on mobile augmented reality devices” (grant number 03EE3034A). Further thanks are directed to the central research funding of the University of Bremen, which made it possible for Mr. Moritz Quandt to spend a research period at the KTH Royal Insitute of Technology in Södertälje, Sweden, through the BremenIDEA program. This stay at the department for Sustainable Production Development contributed significantly to the realization of this paper.
Supplementary Material
The Supplementary Material for this article can be found online at: https://www.frontiersin.org/articles/10.3389/feduc.2021.700760/full#supplementary-material
References
Ajzen, I. (1985). “From Intentions to Actions: A Theory of Planned Behavior,” in Action Control. Editors J. Kuhl,, and J. Beckmann (Berlin, Heidelberg: Springer Berlin Heidelberg), 11–39. doi:10.1007/978-3-642-69746-3_2
Alves, J., Marques, B., Oliveira, M., Araujo, T., Dias, P., and Santos, B. S. (2019). “Comparing Spatial and Mobile Augmented Reality for Guiding Assembling Procedures with Task Validation,” in 2019 IEEE International Conference on Autonomous Robot Systems and Competitions (ICARSC), Porto, Portugal, April 24–26, 2019 (IEEE), 1–6.
Arntz, A., Keßler, D., Borgert, N., Zengeler, N., Jansen, M., Handmann, U., et al. (2020). “Navigating a Heavy Industry Environment Using Augmented Reality - A Comparison of Two Indoor Navigation Designs,” in Virtual, Augmented and Mixed Reality. Industrial and Everyday Life Applications. Editors J. Y. C. Chen,, and G. Fragomeni (Cham: Springer International Publishing), 3–18. doi:10.1007/978-3-030-49698-2_1
Aromaa, S., Aaltonen, I., Kaasinen, E., Elo, J., and Parkkinen, I. (2016). “Use of Wearable and Augmented Reality Technologies in Industrial Maintenance Work,” in Proceedings of the 20th International Academic Mindtrek Conference, Tampere, Finland, October17–18, 2016 (New York, NY, USA: ACM), 235–242.
Aromaa, S., Väätänen, A., Hakkarainen, M., and Kaasinen, E. (2018a). “User Experience and User Acceptance of an Augmented Reality Based Knowledge-Sharing Solution in Industrial Maintenance Work,” in Advances in Usability and User Experience: Proceedings of the AHFE 2017 International Conference on Usability and User Experience, July 17-21, 2017, the Westin Bonaventure Hotel, Los Angeles, California, USA. Editors T. Z. Ahram,, and C. Falcão (Cham, Switzerland: Springer), 145–156. doi:10.1007/978-3-319-60492-3_14
Aromaa, S., Väätänen, A., Kaasinen, E., Uimonen, M., and Siltanen, S. (2018b). “Human Factors and Ergonomics Evaluation of a Tablet Based Augmented Reality System in Maintenance Work,” in Proceedings of the 22nd International Academic Mindtrek Conference, Tampere, Finland, October10–11, 2018 (New York, NY, USA: ACM), 118–125. doi:10.1145/3275116.3275125
Azuma, R. T. (1997). A Survey of Augmented Reality. Presence: Teleoperators. Virtual Environ. 6, 355–385. doi:10.1162/pres.1997.6.4.355
Beckmann, J., Menke, K., and Weber, P. (2019). “Holistic Evaluation of AR/VR-Trainings in the ARSuL-Project,” in INTED2019 Proceedings, Valencia, Spain, March 11–13, 2019. Editors L. Gómez Chova, A. López Martínez, and I. Candel Torres (Valencia, Spain: IATED), 4317–4327. doi:10.21125/inted.2019.1079
Belletier, C., Charkhabi, M., Pires de Andrade Silva, G., Ametepe, K., Lutz, M., and Izaute, M. (2019). Wearable Cognitive Assistants in a Factory Setting: a Critical Review of a Promising Way of Enhancing Cognitive Performance and Well-Being. Cogn. Tech. Work. 23, 103–116. doi:10.1007/s10111-019-00610-2
Billinghurst, M., Clark, A., and Lee, G. (2015). A Survey of Augmented Reality. FNT in Human-Computer Interaction 8, 73–272. doi:10.1561/1100000049
Boboc, R. G., Gîrbacia, F., and Butilă, E. V. (2020). The Application of Augmented Reality in the Automotive Industry: A Systematic Literature Review. Appl. Sci. 10, 4259. doi:10.3390/app10124259
Booth, A., Sutton, A., and Papaioannou, D. (2016). Systematic Approaches to a Successful Literature Review. Los Angeles, London, New Delhi: SAGE.
Bosch, T., van Rhijn, G., Krause, F., Könemann, R., Wilschut, E. S., and Looze, M. de. (2020). “Spatial Augmented Reality,” in PETRA 2020: The 13th ACM International Conference on PErvasive Technologies Related to Assistive Environments. virtual conference : conference proceedings, Corfu Greece, June 30-July 3, 2020. Editor F. Makedon (New York, NY, United States: Association for Computing Machinery), 1–7.
Bottani, E., and Vignali, G. (2019). Augmented Reality Technology in the Manufacturing Industry: A Review of the Last Decade. IISE Trans. 51, 284–310. doi:10.1080/24725854.2018.1493244
Bright, A. G., and Ponis, S. T. (2021). Introducing Gamification in the AR-Enhanced Order Picking Process: A Proposed Approach. Logistics 5, 14. doi:10.3390/logistics5010014
Carmigniani, J., Furht, B., Anisetti, M., Ceravolo, P., Damiani, E., and Ivkovic, M. (2011). Augmented Reality Technologies, Systems and Applications. Multimed Tools Appl. 51, 341–377. doi:10.1007/s11042-010-0660-6
Catal, C., Akbulut, A., Tunali, B., Ulug, E., and Ozturk, E. (2019). Evaluation of Augmented Reality Technology for the Design of an Evacuation Training Game. Virtual Reality 24, 359–368. doi:10.1007/s10055-019-00410-z
Daling, L., Abdelrazeq, A., Sauerborn, C., and Hees, F. (2020). “A Comparative Study of Augmented Reality Assistant Tools in Assembly,” in Advances In Usability And User Experience. Editors T. Ahram,, and C. Falcão (Cham: Springer International Publishing), 755–767. doi:10.1007/978-3-030-19135-1_74
Davis, F. D. (1989). Perceived Usefulness, Perceived Ease of Use, and User Acceptance of Information Technology. MIS Q. 13, 319. doi:10.2307/249008
Dey, A., Billinghurst, M., Lindeman, R. W., and Swan, J. E. (2018). A Systematic Review of 10 Years of Augmented Reality Usability Studies: 2005 to 2014. Front. Robot. AI 5, 1–28. doi:10.3389/frobt.2018.00037
Dix, A., Finlay, J., Abowd, G. D., and Beale, R. (2004). Human-computer Interaction. Harlow: Pearson Education.
Dünser, A., Grasset, R., and Billinghurst, M. (2008). A Survey of Evaluation Techniques Used in Augmented Reality Studies. Technical Report. Christchurch: HIT Lab NZ. doi:10.1145/1508044.1508049
Edirisinghe, R. (2019). Digital Skin of the Construction Site. ECAM 26, 184–223. doi:10.1108/ECAM-04-2017-0066
Egger, J., and Masood, T. (2020). Augmented Reality in Support of Intelligent Manufacturing - A Systematic Literature Review. Comput. Ind. Eng. 140, 106195. doi:10.1016/j.cie.2019.106195
Elshafey, A., Saar, C. C., Aminudin, E. B., Gheisari, M., and Usmani, A. (2020). Technology Acceptance Model for Augmented Reality and Building Information Modeling Integration in the Construction Industry. ITcon 25, 161–172. doi:10.36680/j.itcon.2020.010
Fishbein, M., and Ajzen, I. (1975). Belief, Attitude, Intention and Behaviour: An Introduction to Theory and Research. Reading, Massachusetts: Addison-Wesley.
Fite-Georgel, P. (2011). “Is There a Reality in Industrial Augmented Reality,” in 10th IEEE International Symposium on Mixed and Augmented Reality (ISMAR), 2011, Basel, Switzerland, 26-29 Oct. 2011 (Piscataway, NJ: IEEE), 26201–29210.
Fraga-Lamas, P., Fernandez-Carames, T. M., Blanco-Novoa, O., and Vilar-Montesinos, M. A. (2018). A Review on Industrial Augmented Reality Systems for the Industry 4.0 Shipyard. IEEE Access 6, 13358–13375. doi:10.1109/ACCESS.2018.2808326
Gattullo, M., Evangelista, A., Uva, A. E., Fiorentino, M., and Gabbard, J. (2020). What, How, and Why Are Visual Assets Used in Industrial Augmented Reality? A Systematic Review and Classification in Maintenance, Assembly, and Training (From 1997 to 2019). IEEE Trans. Vis. Comput. Graphics 6, 1. doi:10.1109/TVCG.2020.3014614
Harborth, D., and Pape, S. (2017). “Exploring the Hype: Investigating Technology Acceptance Factors of Pokémon Go,” in 2017 IEEE International Symposium on Mixed and Augmented Reality (ISMAR), Nantes, France, October 9–13, 2017 (IEEE), 155–168.
Helin, K., Kuula, T., Vizzi, C., Karjalainen, J., and Vovk, A. (2018). User Experience of Augmented Reality System for Astronaut's Manual Work Support. Front. Robot. AI 5, 106. doi:10.3389/frobt.2018.00106
Jackson, C. M., Chow, S., and Leitch, R. A. (1997). Toward an Understanding of the Behavioral Intention to Use an Information System. Decis. Sci. 28, 357–389. doi:10.1111/j.1540-5915.1997.tb01315.x
Jetter, J., Eimecke, J., and Rese, A. (2018). Augmented Reality Tools for Industrial Applications: What Are Potential Key Performance Indicators and Who Benefits? Comput. Hum. Behav. 87, 18–33. doi:10.1016/j.chb.2018.04.054
Kim, M., and Lee, J. Y. (2016). Touch and Hand Gesture-Based Interactions for Directly Manipulating 3D Virtual Objects in mobile Augmented Reality. Multimed Tools Appl. 75, 16529–16550. doi:10.1007/s11042-016-3355-9
Lee, J. G., Seo, J., Abbas, A., and Choi, M. (2020). End-Users' Augmented Reality Utilization for Architectural Design Review. Appl. Sci. 10, 5363. doi:10.3390/app10155363
Loch, F., Quint, F., and Brishtel, I. (2016). “Comparing Video and Augmented Reality Assistance in Manual Assembly,” in 2016 12th International Conference on Intelligent Environments (IE), London, UK, September 14–16, 2016 (IEEE), 147–150.
Lorenz, M., Knopp, S., and Klimant, P. (2018). “Industrial Augmented Reality: Requirements for an Augmented Reality Maintenance Worker Support System,” in Adjunct proceedings 2018 IEEE International Symposium on Mixed and Augmented Reality, Munich, Germany, Oct. 16 2018 to Oct. 20 2018 (Piscataway, NJ: IEEE), 151–153. doi:10.1109/ismar-adjunct.2018.00055
Marangunić, N., and Granić, A. (2015). Technology Acceptance Model: a Literature Review from 1986 to 2013. Univ. Access Inf. Soc. 14, 81–95. doi:10.1007/s10209-014-0348-1
Masood, T., and Egger, J. (2020). Adopting Augmented Reality in the Age of Industrial Digitalisation. Comput. Industry 115, 103112. doi:10.1016/j.compind.2019.07.002
Masood, T., and Egger, J. (2019). Augmented Reality in Support of Industry 4.0-Implementation Challenges and success Factors. Robotics and Computer-Integrated Manufacturing 58, 181–195. doi:10.1016/j.rcim.2019.02.003
Min, J. S., Kwak, G., and Hwang, W. (2020). Comparison Among Types of Assembly Manuals Based on Paper, Video and Augmented Reality. ICIC Express Lett. 14, 303–310. doi:10.24507/icicel.14.03.303
Minow, A., Stüring, S., and Böckelmann, I. (2020). “Mental Effort and Usability of Assistance Systems in Manual Assembly - A Comparison of Pick-To-Light and AR Contours through VR Simulation,” in HCI International 2020 - Posters. HCII 2020. Communications in Computer and Information Science. Editors C. Stephanidis, and M. Antona (Cham: Springer) 1224, 455–461. doi:10.1007/978-3-030-50726-8_60
Murauer, N. (2019). “Designing a User-Centered Approach to Improve Acceptance of Innovations on the Shop Floor Using Rogers' 'Diffusion of Innovations',” in Proceedings of the 20th Congress of the International Ergonomics Association (IEA 2018). Editors S. Bagnara, R. Tartaglia, S. Albolino, T. Alexander, and Y. Fujita (Cham: Springer International Publishing), 3–10. doi:10.1007/978-3-319-96068-5_1
Nee, A. Y. C., Ong, S. K., Chryssolouris, G., and Mourtzis, D. (2012). Augmented Reality Applications in Design and Manufacturing. CIRP Ann. 61, 657–679. doi:10.1016/j.cirp.2012.05.010
Palmarini, R., Erkoyuncu, J. A., Roy, R., and Torabmostaedi, H. (2018). A Systematic Review of Augmented Reality Applications in Maintenance. Robot. Comput. Integr. Manuf. 49, 215–228. doi:10.1016/j.rcim.2017.06.002
Park, K.-B., Kim, M., Choi, S. H., and Lee, J. Y. (2020). Deep Learning-Based Smart Task Assistance in Wearable Augmented Reality. Robot. Comput. Integr. Manuf. 63, 101887. doi:10.1016/j.rcim.2019.101887
Petticrew, M., and Roberts, H. (2006). Systematic Reviews in the Social Sciences: A Practical Guide. Malden: Blackwell.
Quandt, M., Knoke, B., Gorldt, C., Freitag, M., and Thoben, K.-D. (2018). General Requirements for Industrial Augmented Reality Applications. Proced. CIRP 72, 1130–1135. doi:10.1016/j.procir.2018.03.061
Rapaccini, M., Porcelli, I., Espíndola, D. B., and Pereira, C. E. (2014). Evaluating the Use of mobile Collaborative Augmented Reality within Field Service Networks: the Case of Océ Italia - Canon Group. Prod. Manufacturing Res. 2, 738–755. doi:10.1080/21693277.2014.943430
Sabherwal, R., Jeyaraj, A., and Chowa, C. (2006). Information System Success: Individual and Organizational Determinants. Manage. Sci. 52, 1849–1864. doi:10.1287/mnsc.1060.0583
Scott, H., Baglee, D., O', R., Brien, N. A., and Potts, R. (2020). An Investigation of Acceptance and E-Readiness for the Application of Virtual Reality and Augmented Reality Technologies to Maintenance Training in the Manufacturing Industry. IJMMS 13, 39. doi:10.1504/IJMMS.2020.108310
Serras, M., García-Sardiña, L., Simões, B., Álvarez, H., and Arambarri, J. (2020). Dialogue Enhanced Extended Reality: Interactive System for the Operator 4.0. Appl. Sci. 10, 3960. doi:10.3390/app10113960
Söbke, H., Baalsrud Hauge, J., and Stefan, I. A. (2017). Prime Example Ingress Reframing the Pervasive Game Design Framework (PGDF). IJSG 4, 39–58. doi:10.17083/ijsg.v4i2.182
Stark, J. (2020). Product Lifecycle Management. (Volume 1). Cham: Springer International Publishing.
Stigall, J., and Sharma, S. (2019). Evaluation of Mobile Augmented Reality Application for Building Evacuation. In Proceedings of 28th International Conference on Software Engineering and Data Engineering, SEDE 2019, San Diego, CA, September 30–October 2, 2019. Editors F. C. Harris, S. Dascalu, S. Sharma, and R. Wu (Manchester, United Kingdom: EasyChair), 109–118. doi:10.29007/7jch
Stoltz, M.-H., Giannikas, V., McFarlane, D., Strachan, J., Um, J., and Srinivasan, R. (2017). Augmented Reality in Warehouse Operations: Opportunities and Barriers. IFAC-PapersOnLine 50, 12979–12984. doi:10.1016/j.ifacol.2017.08.1807
Syberfeldt, A., Danielsson, O., and Gustavsson, P. (2017). Augmented Reality Smart Glasses in the Smart Factory: Product Evaluation Guidelines and Review of Available Products. IEEE Access 5, 9118–9130. doi:10.1109/ACCESS.2017.2703952
Syberfeldt, A., Holm, M., Danielsson, O., Wang, L., and Brewster, R. L. (2016). Support Systems on the Industrial Shop-Floors of the Future - Operators' Perspective on Augmented Reality. Proced. CIRP 44, 108–113. doi:10.1016/j.procir.2016.02.017
Taherdoost, H. (2018). A Review of Technology Acceptance and Adoption Models and Theories. Proced. Manufacturing 22, 960–967. doi:10.1016/j.promfg.2018.03.137
Terhoeven, J., Schiefelbein, F.-P., and Wischniewski, S. (2018). User Expectations on Smart Glasses as Work Assistance in Electronics Manufacturing. Proced. CIRP 72, 1028–1032. doi:10.1016/j.procir.2018.03.060
Ulmer, J., Braun, S., Cheng, C.-T., Dowey, S., and Wollert, J. (2020). Human-Centered Gamification Framework for Manufacturing Systems. Proced. CIRP 93, 670–675. doi:10.1016/j.procir.2020.04.076
Venkatesh, V., Morris, M. G., Davis, G. B., and Davis, F. D. (2003). User Acceptance of Information Technology: Toward a Unified View. MIS Q. 27, 425. doi:10.2307/30036540
Venkatesh, V., Thong, J. Y. L., and Xu, X. (2012). Consumer Acceptance and Use of Information Technology: Extending the Unified Theory of Acceptance and Use of Technology. MIS Q. 36, 157. doi:10.2307/41410412
Venkatesh, V., and Bala, H. (2008). Technology Acceptance Model 3 and a Research Agenda on Interventions. Decis. Sci. 39, 273–315. doi:10.1111/j.1540-5915.2008.00192.x
Venkatesh, V., and Davis, F. D. (2000). A Theoretical Extension of the Technology Acceptance Model: Four Longitudinal Field Studies. Manage. Sci. 46, 186–204. doi:10.1287/mnsc.46.2.186.11926
Wang, X., Ong, S. K., and Nee, A. Y. C. (2016). A Comprehensive Survey of Augmented Reality Assembly Research. Adv. Manuf. 4, 1–22. doi:10.1007/s40436-015-0131-4
Werrlich, S., Nitsche, K., and Notni, G. (2017). “Demand Analysis for an Augmented Reality Based Assembly Training,” in Proceedings of the 10th International Conference on PErvasive Technologies Related to Assistive Environments, Rhodes, Greece, June 21–23, 2017 (New York, NY, USA: ACM), 416–422. doi:10.1145/3056540.3076190
Wild, F., Klemke, R., Lefrere, P., Fominykh, M., and Kuula, T. (2017). “Technology Acceptance of Augmented Reality and Wearable Technologies,” in Immersive Learning Research Network: Third International Conference, iLRN 2017, Coimbra, Portugal, June 26-29, 2017 : Proceedings. Editors D. Beck, C. Allison, L. Morgado, J. Pirker, F. Khosmood, and J. Richter (Cham: Springer), 129–141. doi:10.1007/978-3-319-60633-0_11
Keywords: augmented reality, AR, user acceptance, technology acceptance, TAM, systematic literature review
Citation: Quandt M and Freitag M (2021) A Systematic Review of User Acceptance in Industrial Augmented Reality. Front. Educ. 6:700760. doi: 10.3389/feduc.2021.700760
Received: 26 April 2021; Accepted: 15 November 2021;
Published: 20 December 2021.
Edited by:
Heinrich Söbke, Bauhaus-Universität Weimar, GermanyReviewed by:
Mark Billinghurst, University of South Australia, AustraliaTina Haase, Fraunhofer Institute for Factory Operation and Automation (IFF), Germany
Copyright © 2021 Quandt and Freitag. This is an open-access article distributed under the terms of the Creative Commons Attribution License (CC BY). The use, distribution or reproduction in other forums is permitted, provided the original author(s) and the copyright owner(s) are credited and that the original publication in this journal is cited, in accordance with accepted academic practice. No use, distribution or reproduction is permitted which does not comply with these terms.
*Correspondence: Moritz Quandt, cXVhQGJpYmEudW5pLWJyZW1lbi5kZSYjeDAyMDBhOw==