- 1Department of Psychology, UiT The Arctic University of Norway, Tromsø, Norway
- 2Department of Psychology and University Library, UiT The Arctic University of Norway, Tromsø, Norway
The Tromsø Interest Questionnaire (TRIQ) is the first suite of self-report subscales designed for focused investigations on how interest is experienced in relation to Hidi and Renninger’s four-phase model of interest development. In response to the plethora of varied interest measures that already exist in terms of theoretical grounding, form, and tested quality, the TRIQ subscales were designed with a consistent form to measure general interest, situation dependence, positive affect, competence level, competence aspirations, meaningfulness, and self-regulation answered in relation to some object of interest. Two studies testing the subscales’ performance using different objects of interest (self-chosen “object-general,” and prespecified “object-specific”) provide evidence of the subscales’ internal consistency, temporal reliability, and phase-distinguishing validity. Patterns across the two studies demonstrate that the TRIQ is a sufficiently reliable and valid domain-tailorable tool that is particularly effective at distinguishing phase 1 (triggered situational) from phase 4 (well-developed individual) interest. The findings raise interesting questions for further investigation about the distinction and distance between all interest phases, the push-pull factors that influence how interests evolve and additional subscales to add to the suite.
Introduction
We know it when we feel it, that feeling of interest in something. Sometimes the feeling hits us for the first time when we are introduced to that object or event in some attention-grabbing way, and sometimes that feeling is what drives us to pursue the interest more on our own. We know it when we feel it. But do we know it when we measure it?
Interest
Interest catches and holds our attention (Hidi and Baird, 1986) and facilitates emotionally engaged interactions with objects of interest–critical activities for both initiating and sustaining learning over time (e.g., Harackiewicz et al., 2008). As a construct, interest has both emotional and cognitive aspects. Discrete emotions, or affective states, are perceptional and emotional processes that help prime us to focus on particular kinds of stimuli in involuntary physiological, preattentive ways that can vary in intensity and duration, and uniquely influence attention, behavior, and memory of the stimuli involved (Dolan, 2002; Panksepp, 2003; Izard, 2011)—serving, in a sense, as “relevance detectors” (Scherer, 2005).
In the case of interest, the stimuli that catch our attention related to things we have less control over (novelty and uncertainty) set in motion curiosity-driven behaviors to explore them further (Silvia, 2006; Oatley et al., 2019). As a more conscious feeling, interest-related arousal—when cognitively appraised as something pleasant—becomes a driver for the deliberate pursuit of goals related to that particular object of interest (Scherer, 2005; Silvia, 2006), like cooking Thai food or solving math puzzles. Interest’s value lies in how, once triggered, it focuses our attention and orients us toward exploration and persistence in the face of obstacles (like having to find a rare ingredient or seeking harder math puzzles), uniquely fueling stamina and motivation more than other positive emotions such as enjoyment or happiness (Schiefele et al., 1992; Hidi et al., 2004; Thoman et al., 2011).
However, our relationship to each object or event can change over time—either evolving into a more stable interest (from interest to interests, as some describe, e.g., Berlyne, 1949; Silvia, 2006), or devolving into something situation dependent or no longer interesting (Hidi and Renninger, 2006).
Hidi and Renninger (2006) have been the most explicit in capturing this movement in their four-phase model of interest development. The model describes interest as an experience with four distinct developmental phases. These span experiences of triggered situational interest (phase 1), maintained situational interest (phase 2), emerging individual interest (phase 3), and well-developed individual interest (phase 4). The first two phases require more from the environment to initially trigger and maintain an interest (phase 1 being the most situation-dependent and fleeting), while interest in the last two phases is pursued increasingly more independently (phase 4 being the most independently pursued, and most stable in the face of obstacles).
To date, there has been no self-report tool that enables us to adequately test the experiences of Hidi and Renningers 2006 model in a unified manner. Renninger and Hidi (2011) have offered an overview of how interest has been operationalized and measured quantitatively and qualitatively by others, though without specific details of how those tools were developed and how they could ideally be used to test their model.
Measuring Interest
If we were to rely on self-report measures that capture the underlying architecture of interest from Hidi and Renninger’s model, we must ground them in a clear definition of what interest is and put it in a form that is relatively quick and easy for people to describe in ways that are reliable and conceptually valid. Indeed, many have already developed self-report measures with this in mind. However, since our understanding of interest has changed over time, what has been measured in these self-report tools has varied, as has how the veracity of their value has been determined.
We began with a critical analysis of how other self-report measures have been developed in terms of 1) their theoretical grounding, 2) how they measure interest in domain tailorable ways, and 3) the evidence provided about existing measures’ reliability and validity. We found that though the concepts these other tools touch on do overlap with aspects of the four-phase model of interest development, there is considerable variation among them, making none of them perfect matches for testing the full four-phase model—either alone or in combination with each other. Nevertheless, the way these other tools have been designed and tested is valuable to how we designed and tested ours to redress that gap. We therefore begin with an overview of other self-report interest measures and how that informed how we developed and tested the Tromsø Interest Questionnaire (TRIQ) subscales.
Theoretical Bases
Naturally, over time, the bases for items and measures used have evolved along with how our understanding of interest has evolved (Renninger and Hidi, 2016). Preceding Hidi and Renninger’s four-phase model, self-report inventories were grounded in theories such as interest as an affective and dispositional state (Schiefele et al., 1988), the expectancy-value framework (Eccles et al., 1983; Eccles et al., 1993), interest as something that can be triggered, situational, and personal (e.g., Schraw et al., 1995; Ainley et al., 2002), interest as feeling and value (Krapp et al., 1988; Schiefele, 1999), conceptualization of interest as a multidimensional construct related to self-determination theory (Deci, 1992), interest as a part of the Cognitive-Motivational Process Model (Vollmeyer and Rheinberg, 2000), appraisal theory of interest (Silvia, 2006; Silvia, 2010), and self-concept theory (Marsh et al., 2005). Other measures have evolved with theories complementary to the four-phase model, such as work focused on the triggering, feeling, and value of interest (Linnenbrink-Garcia et al., 2010).
A small handful of measures have been designed more deliberately in harmony with the four-phase model. Examples of this work include that of Bathgate et al. (2014) who focused on the situatedness of interest, Ely et al. (2013) who focused on the stability of affect and interest over time, and Rotgans (2015) who focused on positive feelings, value, and the desire to reengage with an object of interest.
Smorgasbord of Existing Measures
Existing measures of interest represent a varied terrain in both content and form that challenge the navigation of long lines of thought. To give a detailed sense of the variation, an overview of many of the commonly used measures is presented in Supplementary Table S1, with a brief overview here.
Of the simplest kinds of measures, participants can indicate their interests categorically with checklists, e.g., Bathgate et al. (2014), or dragging and dropping into categories (Ely et al., 2013). Single ratings of a selected set of objects of interest (such as topics or activities) have been used with 4-, 5-, 7-, and 10-point scales, e.g., Ainley et al. (2002), Alexander et al. (1995), Dawson (2000), and Häussler and Hoffmann (2002).
Scales with multiple ratings for each object of interest have also been developed with as few as 4 and as many as 24 items to rate on 4- to 7-point scales. They represent differential scale items (Silvia, 2010) and single factor scales such as the Individual Interest Questionnaire (IIQ, Rotgans, 2015), the Task Value and Competence Beliefs scales (Eccles et al., 1993), the Study Interest Questionnaire (SIQ) and Cognitive Competence scale (Schiefele et al., 1988), the Situational Interest (SI) measures (Linnenbrink-Garcia et al., 2010), the Situational Interest Scale (SIS, Chen et al., 1999), parts of the Questionnaire on Current Motivation (QCM) (Vollmeyer and Rheinberg, 1998; Vollmeyer and Rheinberg, 2000), Affect and Experience scales (Ely et al., 2013), and others (e.g., Bathgate et al., 2014). Many of these scales are domain tailorable, though some were made specifically for particular domains, such as the Math Class-Specific Interest and Math Domain-Specific Interest measures (Marsh et al., 2005).
In sum, interest has been captured differently in terms of domain focus, the kinds of prompts and items used, the constellations of items used, and the scoring of the items used. Results from these measures are therefore difficult to compare, even for those that are conceptually related and domain tailorable. Additionally, because of the variety of questions and response alternatives, any attempt at combining these measures into a single scale would be cumbersome for respondents, hence the value of replacing these with a single comprehensive, domain-tailorable suite of measures.
What has Been Used to Provide Evidence of Measure Reliability and Validity
Existing measures of interest vary in how much evidence has been provided regarding their reliability and validity. Though some do document the reliability of their interest measures, many do not. Among those that do, scale reliability has been tested and asserted using 1) internal consistency measures (Schiefele et al., 1988; Chen et al., 1999; Vollmeyer and Rheinberg, 2000; Häussler and Hoffmann, 2002; Marsh et al., 2005; Bathgate et al., 2014); 2) test-retest measures with lengths of delay as long as 2 months (Ely et al., 2013) and 3 months (Alexander et al., 1995); 3) confirmatory factor analysis to assert the construct reliability of a latent variable (Rotgans, 2015); 4) tests of multi-group invariance on a scale (e.g., Rotgans, 2015); and 5) Cohen’s D to assert the reliability of a measure for distinguishing low- from high-scoring participants (Chen et al., 1999).
Fewer studies, though, provide evidence of the measure’s validity. Those that do, have done so in several ways. Most commonly, evidence of construct validity has been tested with exploratory and confirmatory factor analyses (e.g., Eccles et al., 1993; Chen et al., 1999; Ainley et al., 2002; Linnenbrink-Garcia et al., 2010), yet other methods have also been used, including measures of face validity (e.g., Schraw et al., 1995), ecological validity (by, for example, relating interest to a classroom activity; Vollmeyer and Rheinberg, 2000), intraclass correlations (e.g., comparing relations between interest appraisals with appraisals of the objects of interest; Silvia, 2010), multi-group or multi-object invariance (e.g., Eccles et al., 1993; Chen et al., 1999; Linnenbrink-Garcia et al., 2010; Rotgans, 2015); predictive validity (Rotgans, 2015); convergent and divergent validity of interest measures correlated with other motivational measures (e.g., Schiefele et al., 1988; Bathgate et al., 2014); and structural equation modeling (e.g., Marsh et al., 2005).
If we want to test and develop a deeper understanding of the lived experience inherent to the multiple phases of interest Hidi and Renninger have defined, we have to find ways to distinguish each phase from the others both conceptually and through people’s reported interest experiences. That can be done by deliberately asserting and testing the unique psychological architecture underlying each phase. However, in our survey of the literature, we have yet to find a measure, or series of measures, that are sufficiently grounded in Hidi and Renninger’s (2006) four-phase model of interest development to do that. If we can redress that, then we might also be able to unlock an even deeper understanding of how interest changes over time and how to better influence these changes. But first, it is time for a single, parsimonious tool with appropriate reliability and theoretically grounded construct validity to explore that (Kane, 2001). We also need a method for developing it so that the tool can be expanded in the future with additional subscales to help us test yet other aspects of that conceptual architecture.
The Posited Architecture of Each Phase
To set the stage for construct validity, our initial intent was to develop a tool that would capture general interest and four central elements of the four-phase model of interest development: situation dependence, positive affect, competence, and meaningfulness (Dahl, 2011; Dahl, 2014). If sufficiently robust, these measures should enable us to test the relationships we hypothesize among those elements at each phase of the model in ways that previous research yet has not done.
Figure 1 summarizes the hypothesized relationships among four core variables from the four-phase model of interest development (Hidi and Renninger, 2006; Ekeland and Dahl, 2016; Dahl et al., 2019). These relationships are not posited to be absolute mean scores on a set scale, but rather relative relationships varying by degree between the core measured elements within and across each phase of interest. In other words, in line with Hidi and Renninger’s model (2006), it is posited that the mean Situation Dependence score will be at its highest point in phase 1 and lowest in phase 4, steadily decreasing how much space for developing the interest is, by phase, situation-dependent. On the other hand, and also in line with the work of Linnenbrink-Garcia et al. (2010) and Schraw et al. (1995), we posit that the mean Positive Affect score in phase 1 will be lowest as a mean score in phase 1 (though higher than Competence and Meaningfulness), and steadily increase through to phase 4. Similarly, in line with Harackiewicz et al. (2008), competence will be lowest as a mean score in phase 1 and steadily increase with a similar slope to Positive Affect through to phase 4, though with a lower mean score at each phase than that of Positive Affect. Furthermore, in the spirit of findings from Bolkan and Griffin (2018), we posit that Meaningfulness mean scores will also be least and the lowest of all the four measured elements in phase 1, and steadily increase toward phase 4, exhibiting the steepest slope of all the increasing variables and highest mean score in phase 4.
The TRIQ
Like the Motivated Strategies for Learning Questionnaire (MSLQ; Pintrich et al., 1993), the TRIQ is being created as a modular suite of short subscales with a consistent form that can be expanded and tailored as needed in a conceptually and methodologically streamlined way to better understand Hidi and Renninger’s developmental model. What we ultimately hope to be able to answer with the TRIQ’s subscales is how the quality of interest varies by interest phase.
Item Bank
Prior to these studies, we created a large bank of items consisting of existing items from other measures, variations on existing items from other measures, and self-composed items. All had face validity in terms of the four key elements of the four-phase model of interest development. We later added items to this work to capture general interest and self-regulation, since reduced situation dependence implies increased demand for self-regulation. Based on principal component analyses of these items (unpublished manuscript), we selected sets of items for each scale that were found to be topically linked by underlying factors that represent situation dependence, positive affect, knowledge, and meaningfulness. From this preliminary work, we were motivated to divide “knowledge” into two variables—Competence Level (a respondent’s assessment of what they currently know about, or are able to do with, the object of interest), and Competence Aspiration (a respondent’s desire to know or be able to do more).
Finally, we wanted to be able to test our measure with students in Norway, and to create a measure that could also be used by the international research community. We therefore produced and tested the measure in both Norwegian and English. A pilot study yielded no significant differences between language forms (Nierenberg et al., 2021).
Evidence of Reliability and Validity
We will provide evidence of reliability in the form of the internal consistency for each subscale using coefficient alpha; and temporal consistency using test-retest reliability with a 1-week delay and intraclass correlations. Evidence of phase-distinct construct validity will be tested with the subscales, by phase, with multivariate analyses of variance (MANOVA) and descriptive discriminant analysis (DDA). The findings will be tested for multi-object invariance of all measures by comparing the results of one group who responded in terms of a self-chosen object of interest (the Object-General group) with the results from another group who responded in terms of a specified object of interest (the Object-Specific group).
Study Design
Two studies were designed to test peoples’ experiences of the two different target objects of interest: 1) self-chosen objects of interest (“Object-General,” referred to as “X”), or 2) an object of interest that they were provided, namely interest in being or becoming an information literate person (“Object-Specific,” referred to as “IL”). The first study was important for identifying phase similarities independent of the objects of interest upon which they were based. The second study was important for testing if those same patterns were consistent when all respondents were focused on one shared object of interest, in essence enabling us to compare the multiobject-invariance nature of the measures from the first study with the more person-invariant test from the second study. We chose IL as our specified object of interest since information literacy is a critical skill both in academic work (Feekery, 2013; Løkse et al., 2017) and in daily life, empowering people to be socially responsible consumers, users, and creators of information (Walton and Cleland, 2017).
In the Object-General questionnaire, participants read a short summary of each phase of the four phases of interest. They were then asked to identify one personal object of interest that fit each interest description. Participants were then randomly divided into even-sized groups by phase based on their birth months. Within their assigned phase, participants answered the scale questions about the self-identified X object of interest that they had listed for that phase. Though the objects of interest were unique to each participant within each phase, the quality of their interest in their object of interest was arguably similar.
In the Object-Specific questionnaire, we asked participants to indicate their phase of interest in a specific, predesignated IL object of interest (in this case, being or becoming an information-literate person, with a clear definition provided). The participants in this round ended up in groups that were more varied in size, as people’s interest in being or becoming an information-literate person naturally varies. Within each phase, however, the object of interest was the same for every participant.
General Methods
This section applies to both the Object-General (X) and Object-Specific (IL) investigations.
Participants and Procedure
Data were collected between February 2019 and April 2020. Respondents answered the survey, twice, with either a time lapse of 1 week (test-retest) or one semester (pretest-posttest) between surveys. Whereas the test-retest group had no intervention, the pretest-posttest group received 2–4 hour of information literacy instruction between the surveys. The distinction between test-retest and pretest-posttest groups is therefore important for all analyses that involve Time 2 (T2) data.
Object-General (X) Participants
Data for the Object-General (X) measure were collected in both 2019 (X19) and 2020 (X20). In the combined T1 X-cohorts there were 335 participants (115 males, 215 females, 5 other). The 2019 participants (n = 86) received the questionnaire once, while the X20 test-retest group (n = 247) received the same questionnaire twice, with a 1-week time interval. Of those, 118 (35 males, 79 females, 1 other, 3 missing) also answered at T2. In both the rounds, ages ranged from 17 to 84 years (mode = 18–24, median = 35–44).
Object-Specific (IL) Participants
Data for the Object-Specific (IL) measure were collected in 2020. Two groups, with varying time intervals between T1 and T2, answered the survey in which participants’ interest in being or becoming information literate (IL) was the specified object of interest. At T1, the two IL groups involved 364 participants all together (129 males, 229 females, 4 other, 2 missing). The age range of this combined group is 18–85 years, with a mean age of 23.1 years, and median and mode age spans of 18–24 years. At T2, the test-retest group analyzed in this study involved 69 participants (29 male, 40 female). The age range of this group is 18–84 years, with a mean age of 32.6 years, a median age span of 25–34 years, and a mode age span of 18–24 years.
The IL “test-retest group” (n = 253 at T1) had only 1 week between surveys. This group consisted of both students and others, in Norway and the United States, who were recruited exclusively for this study. The IL “pretest-posttest group” (n = 111 at T1) had nearly a whole semester, with 2–4 hour of IL-instruction between surveys, because they participated simultaneously in a longitudinal study tracking their IL-development over the semester. This group was comprised solely of first-year undergraduates in a wide range of disciplines in Norway. Both the groups were combined in the T1 analysis, while only the test-retest group was used in the T2 analyses.
Questionnaire Dissemination
The Object-General test-retest group’s survey was distributed to a convenience sampling of Norwegian and English-speaking participants, through email and social media (e.g., mailing lists, FB, and Twitter). To secure heterogeneous age group representation (Etikan et al., 2016), we intentionally identified distribution sources that would enable us to reach a broad age range of participants in both Norway and the United States. Also, participants were encouraged to share the link to the survey with acquaintances. Furthermore, rewards were offered for those participants who answered also the second time. For this phase of our research, convenience sampling was feasible and sufficient, as the psychological architecture of interest development is regarded as a basic, neurologically based human experience (Hidi, 2006; Hidi and Renninger, 2006; Renninger and Hidi, 2011). Likewise, the questionnaire is in its early phase of development and open for additional scrutiny in subsequent work.
All test-retest participants were given the choice of answering in either Norwegian or English, while the pretest-posttest group answered the survey in Norwegian.
Materials
An online interest survey, developed and distributed through Qualtrics, was used to collect the data (see Supplementary Table S2). Following questions about consent and demographics, the survey contained a “phase” section with four paragraphs, pretested for comprehensibility, which summarized the phases of interest derived from Hidi and Renninger’s (2006) four-phase model of interest development. Participants assessed how well each interest phase description matched their target object of interest.
Following the phase section came the “subscale” section that contained a questionnaire comprised of the seven subscales that participants answered with respect to either their self-chosen (Object-General; X) or prespecified (Object-Specific; IL) object of interest. Once their general interest was assessed, the presentation of the remaining subscales was counterbalanced to avoid order effects. At the end of the survey, participants had an opportunity to write comments.
Below are example items from each subscale as presented in the Object-General questionnaire. “X” represented the interest that the respondent had self-identified for that phase. For the corresponding items in the Object-Specific questionnaire, X is replaced by “being or becoming an information literate person.” For example, for the X questionnaire, participants were asked “How interested are you in X” and for the IL questionnaire, participants were asked “How interested are you in being or becoming an information literate person.” Items in the General Interest and Positive Affect subscales utilized Likert scales ranging from 1 (not at all) to 6 (very much), while the other subscales used Likert scales ranging from 1 (not true at all) to 6 (very true).
• General Interest: Three items, modified from Renninger and Hidi (2011), including “How interested are you in X?”
• Situation Dependence: Three items, two self-composed and one adapted from Rotgans (2015), including “I am dependent upon others for maintaining my interest in X.”
• Positive Affect: Four matrix items, modified from Vittersø et al. (2005), including “How little or much do you experience these feelings (pleasure, happiness, interest, engagement) when you think about your interest in X?”
• Competence Levels: Three self-composed items, inspired by Rotgans (2015), including “I am satisfied with what I know about X.”
• Competence Aspiration: Three items, some self-composed and others adapted from Rotgans (2015) and Tracey (2002), including “I want to learn more about X.”
• Meaningfulness: Five items, some self-composed and some adapted from Rakoczy et al. (2005), Renninger and Hidi (2011), and Schiefele and Krapp (1996), including “Having an interest in X is very useful for me.”
• Self-regulation: Six self-composed items, including “I make time to develop my X-related knowledge and skills.”
Analyses
In both the studies, how well the interest phase description matched their experience of their object of interest was assessed. Also, evidence of subscale reliability was determined through tests of internal consistency and temporal consistency.
The internal consistency of the items across all the phases was tested with Cronbach’s alpha for each subscale. Our preferred criterion for reliability was α ≥ 0.80, as this is generally considered good internal consistency (George and Mallery, 2003).
Temporal consistency, by phase and subscale, was tested in two ways: with test-retest analyses and intraclass correlations (ICC). Although interest is by definition somewhat fluid, and test-retest methods are typically used to determine a variable’s stability, a 1-week time interval was chosen as an interval long enough to limit memory of previous answers, yet short enough to limit the amount of interest change. Our reliability criterion for these tests was nonsignificant difference between Time 1 (T1) and Time 2 (T2) responses.
ICC analyses of T1 and T2 scores for each subscale by phase used to determine individual subscale scores indicated good agreement properties (Berchtold, 2016), by indicating whether within-individual scores are statistically similar enough to discriminate between individuals (Aldridge et al., 2017). We used ICC(A,N), a two-way random model with absolute agreement. Our three agreement criteria were that the ICC was positive, moderate to high (ICC ≥ 0.50), and significant (p < 0.05) for each subscale by phase.
In terms of validity, analyses were done using all seven TRIQ subscales (General Interest, Situation Dependence, Positive Affect, Competence Level, Competence Aspiration, Meaningfulness, and Self-Regulation). Phase distinction was tested with MANOVA to determine if there were notable differences in the subscale scores by a person’s level (phase) of interest in their object of interest. Our reliability criterion was a significant main effect for subscale scores by phase, where all subscale scores would increase from phase 1 to 4, apart from Situation Dependence, which would decrease. We had no a priori hypotheses about the size of the distinctions between phases.
Phase discriminant validity was tested with a descriptive discriminant function analysis with phase as the grouping variable, the subscales as the independent variables and prior probabilities set for all phases being equal (Huberty and Hussein, 2003; Warne, 2014; Barton et al., 2016). This enabled us to determine how well the interest phases are related to distinct interest experiences measured with the Time 1 subscales with the benefit of a reduced possibility of Type 1 error (Sherry, 2006), a method superior to multinomial logistic regression given our focus on the validity of the categories and the number of categories being tested (Al-Jazzar, 2012). Our validity criterion was one or more discriminant functions significantly correlated with the subscale scores in ways that distinguish people’s object of interest experience by phase. We also tested whether these distinctions predicted how accurately people’s experiences were categorized better than chance (chance being 25%).
Finally, the pattern of relationships was tested against the relationships posited in Figure 1.
All analyses were done using the statistical package IBM SPSS Statistics 26. In addition to what is reported in the results, the temporal reliability results and discriminant function classification tables are included in Supplementary Table S3.
Study 1: Object-General Interest
Method
Materials
After the descriptions of the four phases of interest (see General methods), respondents were asked to identify, for each of the interest descriptions, one interest they had that matched the description (see “Object-General” in Supplementary Table S3). In addition to rating each self-provided object of interest by how well it matched with the relevant phase interest description (Match), they also rated how difficult it was to think of an appropriate example for each phase (Example-Finding Difficulty).
Based on their month of birth, respondents were then sent to the next part of the questionnaire, where they answered remaining questions in relation to the example they gave for one of the four interest phases, e.g., those born in the first 3 months described their phase 1 triggered situational interest example, those born in the second 3 months described their phase 2 maintained situational interest example, and so forth. What they chose is referred to as their self-chose object of interest “X.”
Procedure
Participants who fully completed the questionnaire used a mean time of 16.9 min (SD = 13.4) on the task. One week after completing the survey, the test-retest participants were sent a link, via e-mail, to the same questionnaire again.
In T2, participants were instructed to “write down which interest X you focused on in the first round. All your answers must be based on that interest again,” though we did not ask which interest phase description that interest now matched. Unfortunately, due to a programming error in Qualtrics, the test-retest time lag for participants in phase four was 1 month instead of 1 week. These both have implications discussed in the results.
Results
What Kinds of Things did People Report Being Interested In?
The kinds of interests people answered their questions about varied from creative activity (29%, for example dance, photography, handiwork, art, food, music, writing), physical activity (20%, for example working out, cycling, skiing, scuba diving), intellectual activity (17%, for example computer programming, science, history), friluftsliv outdoor life (9%, for example fishing, hiking, hunting), and team sports (8%, for example soccer, handball). The remaining categories (5% or less each, included gardening, general maintenance, entertainment, social engagement, games, flying, and being social).
Phase Description and Interest Match
To understand how well each interest phase description worked for stimulating object of interest examples and how well participant experience of those objects of interests fit with the interest phase description, mean scores were calculated for each.
For the Example-finding difficulty scores (n = 130), the T1 means, standard deviations, and standard error of the mean were the following: phase 1, M = 3.63, SD = 1.51, SE = 0.13; phase 2, M = 2.69, SD = 1.41, SE = 0.12; phase 3, M = 2.29, SD = 1.38, SE = 0.12; and phase 4, M = 1.79, SD = 1.25, SE = 0.11. Since higher scores indicated greater difficulty, these values suggest that it was progressively easier for participants to come up with examples for successively higher phases of interest (p < 0.01 for all paired t-test comparisons).
For the Match scores (n = 130), the T1 means, standard deviations, and standard error of the mean were the following: phase 1, M = 4.42, SD = 1.18, SE = 0.10; phase 2, M = 4.70 SD = 1.03, SE = 0.09; phase 3, M = 4.99 SD = 1.07, SE = 0.10; phase 4, M = 5.25 SD = 1.15, SE = 0.10. A MANOVA of match by phase did not reveal any significant differences by phase, F(12, 375, 000) = 0.280.83, ns, Pillai’s trace = 0.027, η2 = 0.165. This suggests that participants were likely thoughtful about choosing their examples to match the descriptions as best they could, providing a reasonable foundation for the next phase-based analyses.
Evidence of Reliability
Internal consistency: Cronbach’s alpha. The internal consistency of subscale items, measured with Cronbach’s alpha (α), was calculated for each subscale using mean scores from T1. All but one subscale met our α ≥ 0.80 criterion: General Interest (α = 0.87), Situation Dependence (α = 0.84), Positive Affect (α = 0.81), Competence Levels (α = 0.76), Competence Aspiration (α = 0.91), Meaningfulness (α = 0.89), and Self-regulation (α = 0.90).
Paired sample t-tests by phase. This test compared each subscale’s test and retest scores for participants who completed both T1 and T2 questionnaires (see Supplementary Table S3). Our reliability criterion was fulfilled for phases 1-3, exhibiting nonsignificant difference over time. Phase 4, however, showed significantly reduced means at T2 for four of the seven subscales (p < 0.05 for General Interest, Competence Aspiration, Meaningfulness, and Self-regulation), and therefore only partially fulfilled this reliability criterion.
Intraclass correlation by phase. This test correlated T1 and T2 results for each subscale to determine the degree to which participants in the same phase score similarly both times. Our three reliability criteria were fulfilled for phases 1-3 for all subscales; the ICC was positive, moderate to high (ICC ≥ 0.50), and significant (p < 0.05) (see Supplementary Table S3). However, only two subscales had significant correlations for phase 4 (Competence Level, 0.48**, and Self-regulation, 0.38*), yet neither of these ICC’s were ≥0.50. Our reliability criteria were therefore only partially fulfilled for phase 4.
Evidence of Validity
Phase distinction MANOVA. A MANOVA run with T1 subscale scores by phase showed a main effect for phase, F(21, 717, 00) = 6.10, p < 0.001, Pillai’s Trace = 0.455, η2 = 0.15 (see Table 1). All but Situation Dependence showed significant increases by phase (all p < 0.001). Within the subscales with distinct changes, there were nevertheless some nonsignificant differences between phases. Least significant difference post hoc tests showed nonsignificant differences between phases for the following subscales: General Interest, phases 2 and 3; Positive Affect, phases 3 and 4; Competence Level, phases 1 and 2 and phases 2 and 3; Competence Aspiration, phases 2, 3, and 4; Meaningfulness, phases 2, 3, and 4; Self-regulation, phases 2 and 3 and phases 3 and 4. Overall, then, for interest in X, the subscale scores provided the clearest distinction between Phases 1 and 4, and the least distinction between neighboring Phases 2 and 3, and 3 and 4.
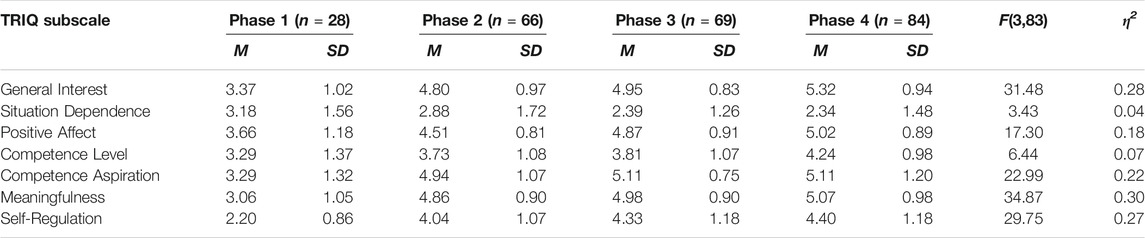
TABLE 1. Means, standard deviations, and multiple analysis of variance between-group effects for TRIQ object-general (X) subscales by interest phase.
Discriminant function analysis. For this analysis, the seven subscales were used to determine if participants’ experience of interest qualitatively differed in meaningful ways by phase. Indeed, results show that the phases are associated with significantly distinct experiences.
Three discriminant functions were calculated. Function 1 had an effect size of 33.99% and Function 2 had an effect size of 8.41% (see Table 2). The first two discriminant functions accounted for 81% and 14% of the between-phase variability. Since the test of Function 3 was not significant, it is not included in the remainder of these analyses.
Standardized discriminant function coefficients and structure coefficients were examined to determine how the subscale variables contributed to the differences between phases (see Table 3). As the squared, pooled, within-group correlations between the subscales and canonical discriminant functions (rs2) indicate, for Function 1, all of the variables aside from Situation Dependence significantly contributed to the group differences (p < 0.05), though Competence Level much less so. For Function 2, only Competence Level was substantially responsible for phase differences.
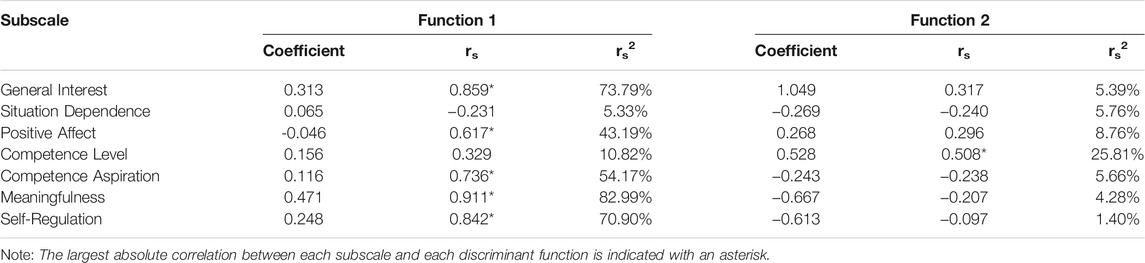
TABLE 3. Standardized discriminant function and structure coefficients for the four phases of interest in X.
As seen in Figure 2, the group centroids for each phase indicate that Function 1 maximally distinguishes phase 1 from 4, and Function 2 maximally distinguishes phase 2 from 4. The Function 1 and 2 centroids are for phase 1, −1.938 and 0.184; phase 2, 0.042 and −0.327; phase 3, 0.203 and −0.210; and phase 4, 0.447 and 0.368. This suggests that phase 1 is most distinct in relation to Function 1, particularly and in this order of effect (see Table 3), with less experience of Meaningfulness, General Interest, Self-regulation, Competence Aspiration, and Positive Affect. In terms of Function 2, phase 4 is slightly more distinct with a higher level experience of competence.
Based on the subscale hit rate for each self-reported interest phase, 51.5% of the original grouped cases were correctly classified in T1 by Function 1. As seen in Supplementary Table S3, the classifications were most distinct for phases 1 (75%) and 4 (58%), and less so for phases 2 (33%) and 3 (29%).
Object-General Discussion
An advantage of this study is that the phase groups were designed to be relatively equal in size, though with varied objects of interest. As hoped, the match between how interested participants were in their self-chosen objects of interest and the interest phase descriptions was comparable across all phases. Furthermore, Cronbach’s alpha indicated that all subscales showed strong internal consistency. Six subscales met our criterion of α ≥ 0.80, and the seventh was only slightly below.
For the test-retest procedures, we recognize that given the number of t-test comparisons and varying sample sizes, this could increase likelihood of Type II errors. We therefore assess the overall pattern of results and give due attention to variation. First, the notable consistency with our predictions was promising. The additional ICC results that were also reasonably consistent with our predictions for phases 1-3 are a positive, corroborative point. However, variations in the data indicate more shifting than presumed, particularly for phase 4 interests. Most likely, this is because of the error in the data collection which gave T1 phase 4 respondents a greater lag time before responding to the T2 retest. This observed instability over time is as likely a result of the fluid nature of interest as the properties of the questionnaire itself—a point corroborated by the low intraclass correlations, though this remains to be verified with T1-T2 t-test comparisons. Since we did not ask which phase people were in with their object of interest at T2, we interpret the results for all phases with some caution. Note: to correct for the T2 phase presumption in subsequent research, we specifically asked for which phase participants in the object-specific study would classify their object of interest in at both T1 and T2.
The MANOVA and descriptive discriminant function analyses based on T1 data show significant differences in subscale scores by phase. Meaningfulness, General Interest and Self-regulation are most distinct across phases, followed by Competence Aspiration and Positive Affect. Given how distinctly General Interest contributes to this finding, we suggest using the General Interest subscale together with the other TRIQ subscales in future interest development research to account for other general aspects of interest not yet captured by the other variables. Given how much additional variance is accounted for by the other subscales, though, we tentatively suggest not using General Interest by itself when interest is the primary variable of study.
Based on the two discriminant functions, all of the variables, aside from Situation Dependence, were responsible for group differences captured by Function 1, though Competence Level contributed substantially to more phase differences accounted for by Function 2. Furthermore, correct phase classification was above chance in all cases, particularly for phases 1 and 4. Altogether, this indicates that the combined subscales offer a moderately good, albeit uneven, indicator of how distinctly, and in which ways, each phase of interest is experienced.
That all the subscales combined worked best in distinguishing T1 phases 1 and 4 could be a result of the quality of the phase descriptions, the subscales themselves, or the fluid nature of interest development. In general, the greater ease people reported for finding later phase interest examples may indicate not only a deeper interest, but also greater metacognitive awareness of such interests. This may also have influenced the smaller number of phase 1 participants even though equally many people were randomly invited to each phase group. This is a matter to keep in mind in future collection and interpretation of self-report results.
In the end, the multi-object invariance related to the internal consistency of all subscales and the temporal stability of all subscales across phases 1, 2, and 3 is promising for TRIQ’s research value. So, too, is the clear distinction of the T1 interest experience of phases 1 and 4. Nevertheless, the temporal stability of phase 4 remains to be properly tested.
Study 2: Object-Specific
Method
Materials
In addition to the TRIQ focused on measuring students’ interest in being or becoming information literate (see the General Methods section), the pretest-posttest group completed a 21-item multiple choice test measuring the students’ knowledge of key aspects of information literacy, at both T1 and T2 (see Nierenberg et al., 2021). The presentation of these two questionnaires was evenly counterbalanced to limit possible order effects.
Procedure
The object-specific interest in being or becoming information literate (IL) test-retest group was recruited via social media and e-mail, using the same procedure as the object-general test-retest group. Participants used a mean of 14.5 min (SD = 10.8) to fill out the T1 survey. Participants who completed the initial interest survey received a retest 1 week later via e-mail.
The pretest-posttest group was recruited from multiple disciplines at several higher education (HE) institutions in Norway where IL instruction was offered. The survey was distributed via the students’ learning management system (LMS). A pretest (T1) was distributed at the beginning of the semester, before IL instruction, and an identical posttest (T2) was distributed 10–16 weeks later in the semester, after 2–4 hour of IL instruction. Rewards were provided to those who completed the posttest. Participants used a mean of 27.6 min (SD = 14.2) to fill out the T1 survey with both the interest questionnaire and ILtest.
Object-Specific Results
Phase Description and Interest Match
Mean scores were again calculated for Match. In this case, match indicated how well participants’ experience of their interest in being or becoming information literate fit with each interest phase description.
For phases 1-4 (n = 354), the T1 means and SDs for the phase description that matched their level of interest were as follows: phase 1, M = 4.37 (1.06); phase 2, M = 4.79 (0.83); phase 3, M = 4.84 (1.04); phase 4, M = 5.37 (0.78). These values show that the matches between participants’ experiences of interest in being or becoming an IL person and the phase descriptions they identified as closest to that experience were moderate to strong for all phases (on the 1-6 scale)—though least for phase 1 and most for phase 4. A multivariate analysis of variance of match by phase was significant, F(12,1047) = 55.83, p < 0.001, Pillai’s trace = 1.17, η2 = 0.39. Post hoc tests indicated that the Match score was significantly distinct, and consistently higher for phase 4 than for the other three phase descriptions. This provides a reasonable foundation for the next phase-based analyses.
Evidence of Reliability
Internal consistency: Cronbach’s alpha. The internal consistency of subscales in Study 2’s object-specific study was calculated using mean T1 subscale scores, with the same criterion as Study 1 of α ≥ 0.80. All subscales met this condition: General Interest (α = 0.84), Situation Dependence (α = 0.80), Positive Affect (α = 0.87), Competence Level (α = 0.89), Competence Aspiration (α = 0.91), Meaningfulness (α = 0.93), and Self-regulation (α = 0.88).
Temporal consistency. To be considered reliable, each subscale should meet the four criteria detailed in the tests below. However, as motivated by the object-general study, the findings in Table 4 indicate that during the week between the test and retest, some people’s interest in being or becoming information literate shifted, mostly to a neighboring level, and mostly to a higher level of interest.
In light of the interests in phases 1 and 2 being more situational and in phases 3 and 4 as more personal, we note the following. For the shifters who classified their interest as being in phase 2 at T1, many reported a more personal interest in IL at the T2 retest. For those who classified their interest as being in phase 3 at T1, the interest was more stable, with an equally small number of shifters experiencing an increase to a more personal interest level and a decrease to a more situational interest level. The T1 phase 4 shifters experienced a decrease in interest, but still mostly within a level of personal interest.
The test-retest analyses capture the nature of an interest experienced within a particular phase. The subsequent analyses are therefore linked to the interests that were constant at T1 and T2 (n = 44; bold in Table 4). Note that there are therefore no temporal results reported for phase 1—already a small group of one, and arguably the most tenuous of all the phases.
Paired sample t-tests by phase. This test compared subscale T1 and T2 data from the test-retest group. Our reliability criterion was fulfilled for phases 2-4, exhibiting consistent nonsignificant difference over time (see Supplementary Table S3). All test-retest scores for all scales were nonsignificant with only two exceptions: phase 3 and phase 4 Meaningfulness scores increased significantly over the week.
Intraclass correlation by phase. The same three ICC-criteria as in Study 1 were used to interpret correlations in Study 2, namely that ICC should be positive, moderate to high, and significant. All of the criteria were met for General Interest, Positive Affect, and Competence Aspiration (see Supplementary Table S3). In terms of the criteria met with the other scales, for Situation Dependence, phase 3 met all criteria, phase 4 met two, and phase 2 met one (though correlations for both phases 4 and 2 nevertheless moderate and therefore close). For Competence Level, phase 4 met all, phase 2 met two (though with a larger sample, the correlation would have been significant), and phase 3 met one. For Meaningfulness, phase 4 met two, phase 3 met one, and phase 2 did not meet any of the criteria. Finally, for Self-regulation, phase 4 met all criteria, phase 3 met one (though the correlation was nevertheless moderate and therefore close), and phase 2 met none. Meaningfulness showed the greatest variation from T1 to T2 even though, overall, mean Meaningfulness scores increased from one phase to the next with relatively low variance.
Evidence of Validity
Phase distinction MANOVA. A MANOVA run with T1 test-retest and pretest-posttest subscale scores by phase showed a main effect for phase, F(21,996) = 8.63, p < 0.001, Pillai’s Trace = 0.462, η2 = 0.15 (see Table 5). All subscales changed as predicted—all significantly increasing by phase, aside from Situation Dependence, which significantly decreased by phase. Within the subscales, least significant difference post hoc tests showed that almost all scores significantly differed from each other by phase in the predicted direction (p < 0.01 for all and p < 0.001 for most), with the following exceptions: 1) for Situation Dependence, neither phases 1 and 2 nor phases 3 and 4 were significantly distinct, and for phases 1 and 3, p < 0.05; 2) for Competence Level, phase 1 and 2 p < 0.05; and finally, 3) for Competence Aspirations, phases 3 and 4 were not significantly distinct.
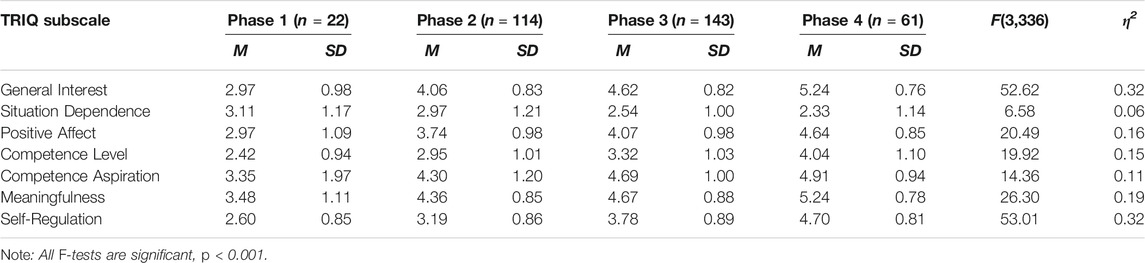
TABLE 5. Means, standard deviations, and multiple analysis of variance between-group effects for TRIQ object-specific (IL) subscales by interest phase.
Discriminant function analysis. For this analysis, all the subscales were used to determine if the phase of interest that T1 participants in both the test-retest and pretest-posttest groups were experientially distinct.
Three discriminant functions were calculated, and showed a moderately high correlation with Function 1, with an effect size of 39.82%, and low canonical correlation with Function 2 (see Table 6). The first two discriminant functions accounted for 91 and 7% of the between-phase variability. The test of function 3 was not significant and therefore not considered in the remainder of these analyses.
Standardized discriminant function coefficients and structure coefficients were examined to determine how the subscale variables contributed to the differences between phases (see Table 7). As the squared, pooled, within-group correlations between the subscales and canonical discriminant functions (rs2) indicate, for Function 1, all of the variables aside from Situation Dependence significantly contributed to the group differences (p < 0.05), though, in this case Competence Aspiration a bit less so. For Function 2, Competence Aspiration was primarily responsible for group differences. Note that, compared to the object-general analyses, the same variables were significantly associated with Functions 1 and 2. However, in the object-specific analyses, Competence Level and Aspiration traded which function they significantly correlated with.
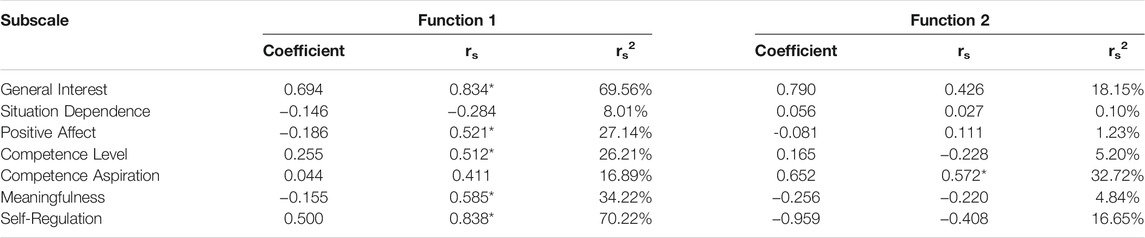
TABLE 7. Standardized discriminant function and structure coefficients for the four phases of interest in IL.
Results showed that people were correctly classified by their subscale scores into their selected phase well beyond chance. Based on the subscale hit rates for each T1 self-reported interest phase, 49.4% of the original grouped cases were correctly classified by Function 1 in T1 (Wilks’ Lambda = 0.56). The results of the discriminant function analyses are found in Supplementary Table S3.
As seen in Figure 3, the group centroids for each phase indicate that Function 1 maximally distinguishes phase 1 from 4, and Function 2 maximally distinguishes phase 1 from phases 2 and 3. The Function 1 and 2 centroids are for phase 1, −1.773 and −0.622; phase 2, −0.632 and 0.100; phase 3, 0.230 and 0.132; and phase 4, 1.282 and −0.273. This suggests that phase 1 is distinct in relation to Function 1, particularly and in this order of effect (see Table 7), in terms of lower General Interest and Self-regulation, and then Meaningfulness, Positive Affect, and Competence Level. In terms of Function 2, Phases 1 and 4 are distinguished by lower Competence Aspiration than phases 2 and 3.
Object-Specific Discussion
While an advantage in this study is that the object of interest was the same for all participants, a disadvantage is that the number of participants in each phase varied, though larger participant groups helped compensate for that deficit.
The test-retest findings in Study 2 indicate, again, that interests have a fluid quality. We were able to catch people’s changes in interest by asking people at T1 and T2 which phase description best described their interest in being or becoming information literate. The results indicated that even over the course of a week, interests can (d)evolve. Table 4 indicates that regarding IL, the participants in this study tended to remain in or move into phases of individual interest more than situational interest. We therefore suggest that participants both indicate their general phase of interest and fill out the TRIQ subscales when documenting the quality of their interest over time (which we had not done in the object-general study).
Though the ICC analyses showed the greatest consistency in participants’ General Interest, Positive Affect, and Competence Aspiration over time, where the subscales did show some variation, they varied least in phase 4, then in phase 3, and finally in phase 2. This pattern fits with what one might predict about fluidity from the more established nature of individual interests (phases 3 and 4 being more stable, as expected, though unable to test in the object-general study because of the phase 4 snafu) over situational interests (phases 1 and 2 being more fluid) in the four-phase model. This also addresses the question we were unable to answer about Phase 4 in the object-general study because of the longer T2 delay.
Based on two discriminant functions, all of the variables aside from Situation Dependence were responsible for group differences captured by Function 1, though Competence Aspiration contributed substantially to more group differences accounted for by Function 2. Actual interest experiences and interest description matches were strongest for phases 1 and 4, though in all the cases, the discriminant function analysis demonstrated phase prediction above chance. Again, this indicates that the combined subscales offer a moderately good, albeit uneven, indicator of how distinctly, and in which ways, each phase of interest is experienced. Furthermore, the combined strength of all the subscales reinforces the value of including the General Interest subscale to capture aspects of interest otherwise not measured by the other TRIQ subscales, and vice versa when interest phase or development is the primary object of the study.
Object-General and Object-Specific Variable Relationships
When we compare the relationships among the core variables in the original hypotheses illustrated in Figure 1 with both the object-general and object-specific results represented in Figures 4A,B, the patterns of means (and confidence intervals, CI) are notably similar, and corroborate the findings from the MANOVA and descriptive discriminant analyses.
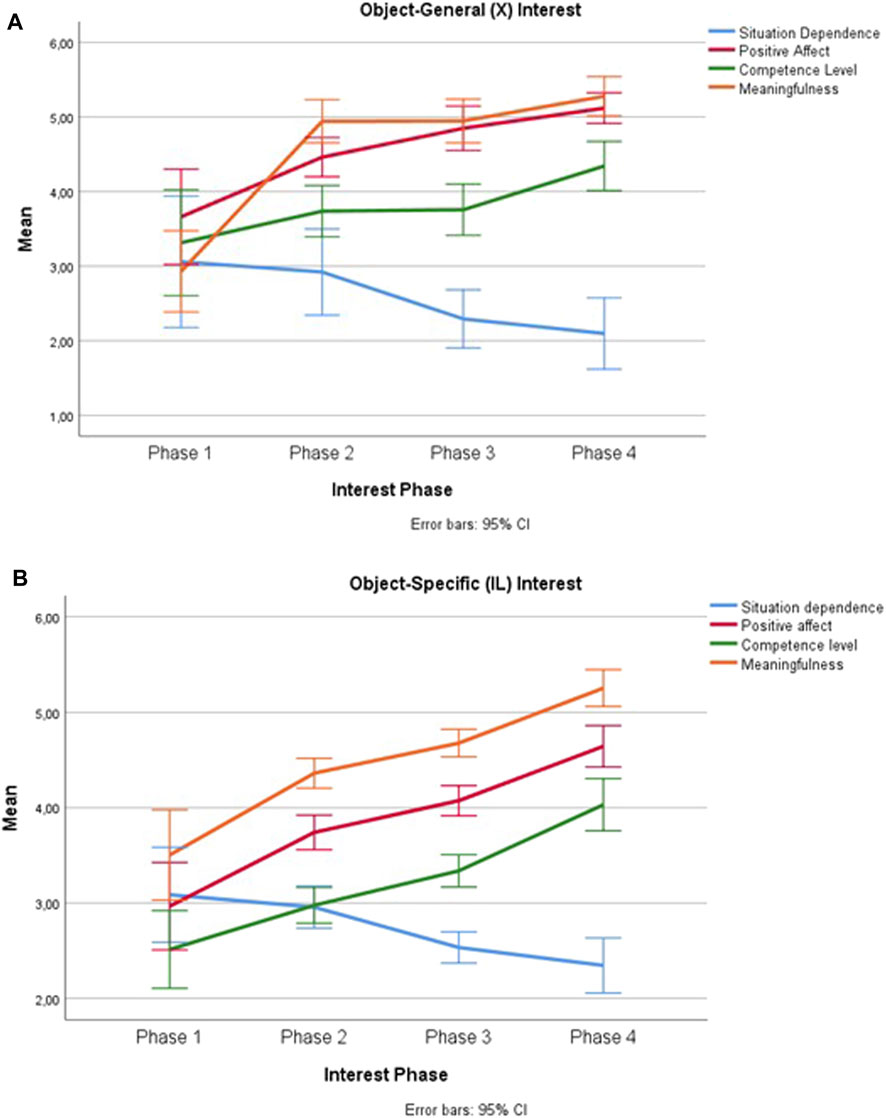
FIGURE 4. Mean TRIQ subscale scores for object-general and object-specific interests by phase (for comparison with original hypotheses). (A) Object-general (X) relationships between key interest variables. (B) Object-specific (IL) relationships between key interest variables.
In absolute terms, the original variables we set out to study, in both the object-general and object-specific analyses, indicated, as predicted, that Positive Affect, Competence Level, and Meaningfulness all increase significantly and in distinct ways by phase. However, Situation Dependence varied less by phase than predicted. Also, the Meaningfulness score deviated from our phase 1 prediction in the object-specific study by being higher than Positive Affect and Competence Level. This is perhaps an artifact of that particular object of interest (IL) in as probed among participants from academic settings. The degree to which a triggered interest can be immediately meaningful to people may be more situation-dependent than we had hypothesized. This warrants further research.
Meanwhile, as already reported, the variables of General Interest, Competence Aspiration, and Self-regulation that were added after the original hypotheses were posited also increased significantly by phase, as one would also expect.
That these patterns were quite similar in both studies, and that most of the subscales correlated significantly with the first discriminant function, suggests that the subscales capture important aspects of interest in a coherent and arguably domain-tailorable way, providing compelling evidence for construct validity (Kane, 2001). Accordingly, we suggest that TRIQ can be used in future research to study general and specified interests, though the interaction between specified interests and context may offer additional, important information about how an interest devolves.
Overall Discussion
Have we designed a tool that can help us study the four different phases of interest described by Hidi and Renninger (2006) in reliable and valid ways?
We have designed subscales that are uniform in design and have documented their internal consistency and temporal reliability. In terms of inferences we can make about the subscales’ construct validity relevance, as originally posited, evidence indicates qualitatively different experiences in predictable ways in terms of each phase’s absolute and relative subscale score means and slopes.
What this evidence does not indicate, however, is equal distance among the variables by phase, suggesting that the difference between them is less defined, at least by the key variables we focused on, than asserted in the four-phase model. However, the General Interest scale captures differences between the phases that have yet to be distinguished, so understanding more about what the General Interest measure captures and explicitly distinguishing any additional underlying factors with their own subscales may help define the phases more distinctly. For example, we suggest the TRIQ suite of subscales be supplemented with a subscale to measure the desire to reengage (Rotgans, 2015) and perhaps a general self-efficacy measure for problem-solving—perhaps an important factor for realizing the desire to pursue an interest further on one’s own (e.g., Chen et al., 2001).
Limitations and Suggestions for Future Research
We found that the TRIQ subscales did not reveal equally distinct interest experiences for all interest phases. The blurrier distinction between phases 2 and 3 and phases 3 and 4, may suggest that either our tools are not sharp enough to discern these distinctions, or the psychological distance between them may actually be less than the psychological distance between phase 1 and all the rest. The next step would be to determine if 1) that is an artifact of people describing interests from the past or present, or 2) if there are additional variables that ought to be tested and included in the TRIQ to better distinguish people’s phase 2-4 interest experiences.
Also, our work was originally motivated by the nature of general interest and the four phases of interest as they related to situation dependence, positive affect, knowledge, and meaningfulness. However, in preparing the items for our measures, we ended up replacing knowledge with two more distinct knowledge measures (Competence Level and Competence Aspiration) and added a Self-regulation measure. These additions accounted for additional variance and are worthy of closer scrutiny.
Additionally, recruiting and retaining sufficient numbers of participants for repeated measures work poses unique challenges, hence our need to recruit multiple samples of participants over time. This is worth keeping in mind in the design of future subscale contributions to the TRIQ suite of measures.
Finally, in the interest of better understanding how interest (d)evolves, it would be useful to employ the TRIQ to test what moves people from one phase to the next and if there are border-distinct push or pull factors between phases. For example, what is the impact of relevance (where meaningfulness and competence intersect) or resonance (where meaningfulness and positive affect intersect) on one’s draw to a higher or lower phase? How might other questions and methods supplement TRIQ findings to help us understand those relationships even better? For example, by evaluating the probabilities of being associated with one of two neighboring categories using multinomial logistic regression (DeRose, 1991; Al-Jazzar, 2012) or by elucidating the experience of particular phases of interest through qualitative interviews (Renninger and Hidi, 2011)?
Conclusion
The object-general study allowed us to test how stable the TRIQ scales were, by phase, across various domains, while the object-specific study allowed us to retest the veracity of the object-general findings in an object-specific way. The similarities between the two studies’ findings offer compelling evidence that the TRIQ suite of subscales are reliable, theoretically valid, and can therefore be useful for studying varying and phase-distinct experiences of interest commensurate with Hidi and Renninger’s (2006) four-phase model of interest development. We encourage the development of more subscales to add to the TRIQ suite, adding variable measures that use the same basic form, and tested similarly for reliability and validity.
As for interest, we still know it when we feel it. Can we now get to know it even better with this new way of measuring it? It is a TRIQ question.
Data Availability Statement
The original contributions presented in the study are included in the article/Supplementary Material, further inquiries can be directed to the corresponding author.
Ethics Statement
Ethical review and approval was not required for the study on human participants in accordance with the local legislation and institutional requirements. The patients/participants provided their written informed consent to participate in this study.
Author Contributions
TID was primarily responsible for the conception and the design of study 1, while TID and EN contributed equally to the conception and design of study 2. TID and EN organized the databases for both studies. EN primarily organized and performed the descriptive analyses while TID organized and performed the inferential statistical analyses. TID and EN both wrote sections of the manuscript, and TID completed the first full draft. Both authors contributed to manuscript revision, read, and approved the submitted version.
Funding
This work was initially funded by The Research Council of Norway for the project Service Innovation and Tourist Experiences in the High North 195306/I40 (2009-2017). The publication of this article is funded by UiT The Arctic University of Norway.
Conflict of Interest
The authors declare that the research was conducted in the absence of any commercial or financial relationships that could be construed as a potential conflict of interest.
Publisher’s Note
All claims expressed in this article are solely those of the authors and do not necessarily represent those of their affiliated organizations, or those of the publisher, the editors and the reviewers. Any product that may be evaluated in this article, or claim that may be made by its manufacturer, is not guaranteed or endorsed by the publisher.
Acknowledgments
The authors would like to thank the Norwegian Research Council for funding the Northern InSights project 195306/i40 that helped initiate this work. They would also like to thank UiT students Ingvild Lappegård, Karine Thorheim Nilsen, Torstein de Besche, Tilde Ingebrigtsen and Jesper Kraugerud, along with early contributors Kjærsti Thorsteinsen and Tony Pedersen. Their involvement in the project along the way was critical to its healthy start and final completion.
Supplementary Material
The Supplementary Material for this article can be found online at: https://www.frontiersin.org/articles/10.3389/feduc.2021.716543/full#supplementary-material
References
Ainley, M., Hidi, S., and Berndorff, D. (2002). Interest, Learning, and the Psychological Processes That Mediate Their Relationship. J. Educ. Psychol. 94 (3), 545–561. doi:10.1037/0022-0663.94.3.545
Al-Jazzar, M. (2012). A Comparative Study between Linear Discriminant Analysis and Multinomial Logistic Regression in Classification and Predictive Modeling. Gaza: Master's thesis, Al Azhar University Gaza.
Aldridge, V. K., Dovey, T. M., and Wade, A. (2017). Assessing Test-Retest Reliability of Psychological Measures. Eur. Psychol. 22, 207–218. doi:10.1027/1016-9040/a000298
Alexander, P. A., Jetton, T. L., and Kulikowich, J. M. (1995). Interrelationship of Knowledge, Interest, and Recall: Assessing a Model of Domain Learning. J. Educ. Psychol. 87 (4), 559–575. doi:10.1037/0022-0663.87.4.559
Barton, M., Yeatts, P. E., Henson, R. K., and Martin, S. B. (2016). Moving Beyond Univariate Post-hoc Testing in Exercise Science: A Primer on Descriptive Discriminate Analysis. Res. Q. Exerc. Sport. 87 (4), 365–375. doi:10.1080/02701367.2016.1213352
Bathgate, M. E., Schunn, C. D., and Correnti, R. (2014). Children's Motivation Toward Science Across Contexts, Manner of Interaction, and Topic. Sci. Ed. 98 (2), 189–215. doi:10.1002/sce.21095
Berchtold, A. (2016). Test-retest: Agreement or Reliability? Methodological Innov. 9, 205979911667287. doi:10.1177/2059799116672875
Berlyne, D. E. (1949). Interest as a Psychological Concept. Br. J. Psychol. Gen. Sect. 39 (4), 184–195. doi:10.1111/j.2044-8295.1949.tb00219.x
Bolkan, S., and Griffin, D. J. (2018). Catch and Hold: Instructional Interventions and Their Differential Impact on Student Interest, Attention, and Autonomous Motivation. Commun. Education. 67 (3), 269–286. doi:10.1080/03634523.2018.1465193
Chen, A., Darst, P. W., and Pangrazi, R. P. (1999). What Constitutes Situational Interest? Validating a Construct in Physical Education. Meas. Phys. Education Exerc. Sci. 3 (3), 157–XXX. doi:10.1207/s15327841mpee0303_3
Chen, G., Gully, S. M., and Eden, D. (2001). Validation of a New General Self-Efficacy Scale. Organizational Res. Methods. 4 (1), 62–83. doi:10.1177/109442810141004
Dahl, T. I., Lappegård, I., Thorheim, K., and Nierenberg, E. (2019). “Developing a Measure of Interest: Where to Next From This fork in the Road?,” in 20th Norwegian Social Psychology and Community Psychology Conference Tromsø, Norway.
Dahl, T. I. (2011). “Moving People: A Conceptual Framework for Capturing Important Learning From High North Experiences,” in Opplevelser I Nord/Northern InSights. Svolvær, Norway.
Dahl, T. I. (2014). “Moving People: A Conceptual Framework for Understanding How Visitor Experiences Can Be Enhanced by Mindful Attention to Interest,” in Creating Experience Value in Tourism. Editors N.K. Prebensen, J.S. Chen, and M. Uysal (Boston, MA: CABI), 79–94. doi:10.1079/9781780643489.0079
Dawson, C. (2000). Upper Primary Boys' and Girls' Interests in Science: Have They Changed Since 1980? Int. J. Sci. Education. 22 (6), 557–570. doi:10.1080/095006900289660
Deci, E. L. (1992). “The Relation of Interest to the Motivation of Behavior: A Self-Determination Theory Perspective,” in The Role of Interest in Learning and Development. Editors K.A. Renninger, S. Hidi, and A. Krapp (Hillsdale, NJ, USA: Lawrence Erlbaum), 43–69.
DeRose, D. (1991). Comparing Classification Models From Multinomial Logistic Regression and Multiple Discriminant Analysis. Ann Arbor, MI: University Microfilms International.
Dolan, R. J. (2002). Emotion, Cognition, and Behavior. Science. 298 (5596), 1191–1194. doi:10.1126/science.1076358
Eccles, J., Wigfield, A., Harold, R. D., and Blumenfeld, P. (1993). Age and Gender Differences in Children's Self- and Task Perceptions During Elementary School. Child. Dev. 64 (3), 830–847. doi:10.2307/113122110.1111/j.1467-8624.1993.tb02946.x
Eccles, J., Adler, T. F., Futterman, R., Goff, S. B., Kaczala, C. M., Meece, J., et al. (1983). “Expectancies, Values, and Academic Behaviors,” in Achievement and Achievement Motives. Editor J. T. Spence (San Francisco: Freeman), 75–146.
Ekeland, C. B., and Dahl, T. I. (2016). Hunting the Light in the High Arctic: Interest Development Among English Tourists Aboard the Coastal Steamer Hurtigruten. Tourism Cult. Commun. 16 (1-2), 33–58. doi:10.3727/109830416X14655571061719
Ely, R., Ainley, M., and Pearce, J. (2013). More Than Enjoyment: Identifying the Positive Affect Component of Interest That Supports Student Engagement and Achievement. Middle Grades Res. J. 8 (1), 13–32.
Etikan, I., Musa, S. A., and Alkassim, R. S. (2016). Comparison of Convenience Sampling and Purposive Sampling. Ajtas. 5 (1), 1–4. doi:10.11648/j.ajtas.20160501.11
Feekery, A. J. (2013). Conversation and Change: Integrating Information Literacy to Support Learning in the New Zealand Tertiary Context: A Thesis Presented for Partial Fulfilment of the Requirements for the Degree of Doctor of Philosophy at Massey University. Manawatu: Massey University.
George, D., and Mallery, P. (2003). SPSS for Windows Step by Step: A Simple Guide and Reference, 11.0 Update. Boston, MA, USA: Allyn & Bacon.
Harackiewicz, J. M., Durik, A. M., Barron, K. E., Linnenbrink-Garcia, L., and Tauer, J. M. (2008). The Role of Achievement Goals in the Development of Interest: Reciprocal Relations Between Achievement Goals, Interest, and Performance. J. Educ. Psychol. 100 (1), 105–122. doi:10.1037/0022-0663.100.1.105
Häussler, P., and Hoffmann, L. (2002). An Intervention Study to Enhance Girls' Interest, Self-Concept, and Achievement in Physics Classes. J. Res. Sci. Teach. 39 (9), 870–888. doi:10.1002/tea.10048
Hidi, S., and Baird, W. (1986). Interestingness-A Neglected Variable in Discourse Processing. Cogn. Sci. 10 (2), 179–194. doi:10.1016/S0364-0213(86)80003-9
Hidi, S. (2006). Interest: A Unique Motivational Variable. Educ. Res. Rev. 1 (2), 69–82. doi:10.1016/j.edurev.2006.09.001
Hidi, S., Renninger, K. A., and Krapp, A. (2004). “Interest, a Motivational Variable that Combines Affective and Cognitive Functioning,” in He Educational Psychology Series. Motivation, Emotion, and Cognition: Integrative Perspectives on Intellectual Functioning and Development. Editors D. Y. Dai, and R. J. Sternberg (Lawrence Erlbaum Associates Publishers), 89–115. doi:10.4324/9781410610515-11
Hidi, S., and Renninger, K. A. (2006). The Four-Phase Model of Interest Development. Educ. Psychol. 41 (2), 111–127. doi:10.1207/s15326985ep4102_4
Huberty, C. J., and Hussein, M. H. (2003). Some Problems in Reporting Use of Discriminant Analyses. J. Exp. Education. 71 (2), 177–192. doi:10.1080/00220970309602062
Izard, C. E. (2011). Forms and Functions of Emotions: Matters of Emotion-Cognition Interactions. Emot. Rev. 3 (4), 371–378. doi:10.1177/1754073911410737
Kane, M. T. (2001). Current Concerns in Validity Theory. J. Educ. Meas. 38 (4), 319–342. doi:10.1111/j.1745-3984.2001.tb01130.x
Krapp, A., Schiefele, U., and Winteler, A. (1988). Studieninteresse und fachbezogene Wissensstruktur. Psychol. Erziehung Unterricht. 35, 106–118.
Linnenbrink-Garcia, L., Durik, A. M., Conley, A. M., Barron, K. E., Tauer, J. M., Karabenick, S. A., et al. (2010). Measuring Situational Interest in Academic Domains. Educ. Psychol. Meas. 70 (4), 647–671. doi:10.1177/0013164409355699
Løkse, M., Låg, T., Solberg, M., Andreassen, H. N., and Stenersen, M. (2017). Teaching Information Literacy in Higher Education: Effective Teaching and Active Learning. Cambridge, MA: Chandos Publishing.
Marsh, H. W., Trautwein, U., Lüdtke, O., Köller, O., and Baumert, J. (2005). Academic Self-Concept, Interest, Grades, and Standardized Test Scores: Reciprocal Effects Models of Causal Ordering. Child. Dev. 76 (2), 397–416. doi:10.1111/j.1467-8624.2005.00853.x
Nierenberg, E., Låg, T., and Dahl, T. I. (2021). Knowing and Doing. Jil. 15 (2), 78. doi:10.11645/15.2.2795
Oatley, K., Keltner, D., and Jenkins, J. M. (2019). Understanding Emotions. Hoboken, NJ: John Wiley & Sons.
Panksepp, J. (2003). Neuroscience. Feeling the Pain of Social Loss. Science. 302 (5643), 237–239. doi:10.1126/science.1091062
Pintrich, P. R., Smith, D. A. F., Garcia, T., and McKeachie, W. J. (1993). Reliability and Predictive Validity of the Motivated Strategies for Learning Questionnaire (MSLQ). Educ. Psychol. Meas. 53 (3), 801–813. doi:10.1177/0013164493053003024
Rakoczy, K., Buff, A., and Lipowsky, F. (2005). “Dokumentation der Erhebungs-und Auswertungsinstrumente zur schweizerisch-deutschen Videostudie,” in Unterrichtsqualität, Lernverhalten und mathematisches Verständnis". 1. Befragungsinstrumente (Frankfurt, Main: GFPF ua).
Renninger, K. A., and Hidi, S. (2011). Revisiting the Conceptualization, Measurement, and Generation of Interest. Educ. Psychol. 46 (3), 168–184. doi:10.1080/00461520.2011.587723
Renninger, K. A., and Hidi, S. (2016). The Power of Interest for Motivation and Engagement. New York: Routledge.
Rotgans, J. I. (2015). Validation Study of a General Subject-Matter Interest Measure: The Individual Interest Questionnaire (IIQ). Health Professions Education. 1 (1), 67–75. doi:10.1016/j.hpe.2015.11.009
Scherer, K. R. (2005). What Are Emotions? and How Can They Be Measured?. Soc. Sci. Inf. 44 (4), 695–729. doi:10.1177/0539018405058216
Schiefele, U. (1999). Interest and Learning from Text. Scientific Stud. Reading. 3 (3), 257–279. doi:10.1207/s1532799xssr0303_4
Schiefele, U., and Krapp, A. (1996). Topic Interest and Free Recall of Expository Text. Learn. Individual Differences. 8 (2), 141–160. doi:10.1016/S1041-6080(96)90030-8
Schiefele, U., Krapp, A., and Winteler, A. (1992). “Interest as a Predictor of Academic Achievement: A Meta-Analysis of Research,” in The Role of Interest in Learning and Development. Editors K.A. Renninger, S. Hidi, and A. Krapp (Mahwah, NJ, USA: Lawrence Erlbaum Associates), 183–212.
Schiefele, U., Krapp, A., and Winteler, A. (1988). Conceptualization and Measurement of Interest. In Annual Meeting of the American Educational Research Association. New Orleans, LA, USA.
Schraw, G., Bruning, R., and Svoboda, C. (1995). Sources of Situational Interest. J. Reading Behav. 27 (1), 1–17. doi:10.1080/10862969509547866
Sherry, A. (2006). Discriminant Analysis in Counseling Psychology Research. Couns. Psychol. 34 (5), 661–683. doi:10.1177/0011000006287103
Silvia, P. J. (2010). Confusion and Interest: The Role of Knowledge Emotions in Aesthetic Experience. Psychol. Aesthetics, Creativity, Arts. 4 (2), 75–80. doi:10.1037/a0017081
Thoman, D. B., Smith, J. L., and Silvia, P. J. (2011). The Resource Replenishment Function of Interest. Soc. Psychol. Personal. Sci. 2 (6), 592–599. doi:10.1177/1948550611402521
Tracey, T. J. G. (2002). Development of Interests and Competency Beliefs: A 1-year Longitudinal Study of Fifth- to Eighth-Grade Students Using the ICA-R and Structural Equation Modeling. J. Couns. Psychol. 49 (2), 148–163. doi:10.1037/0022-0167.49.2.148
Vittersø, J., Biswas-Diener, R., and Diener, E. (2005). The Divergent Meanings of Life Satisfaction: Item Response Modeling of the Satisfaction With Life Scale in Greenland and Norway. Soc. Indic Res. 74 (2), 327–348. doi:10.1007/s11205-004-4644-7
Vollmeyer, R., and Rheinberg, F. (1998). Motivationale Einflüsse auf Erwerb und Anwendung von Wissen in Einem Computersimulierten System [Motivational influences on the Acquisition and Application of Knowledge in a Simulated System]. Z. für Pädagogische Psychol. 12 (1), 11–23.
Vollmeyer, R., and Rheinberg, F. (2000). Does Motivation Affect Performance via Persistence? Learn. Instruction. 10 (4), 293–309. doi:10.1016/S0959-4752(99)00031-6
Walton, G., and Cleland, J. (2017). Information Literacy. Jd. 73 (4), 582–594. doi:10.1108/JD-04-2015-0048
Keywords: interest, information literacy, four-phase model of interest development, reliability, validity, scale construction
Citation: Dahl TI and Nierenberg E (2021) Here’s the TRIQ: The Tromsø Interest Questionnaire Based on the Four-Phase Model of Interest Development. Front. Educ. 6:716543. doi: 10.3389/feduc.2021.716543
Received: 28 May 2021; Accepted: 21 September 2021;
Published: 08 November 2021.
Edited by:
Ramón Chacón-Cuberos, University of Granada, SpainReviewed by:
Michael Schurig, Technical University Dortmund, GermanyMariana Mármol, University of Granada, Spain
Copyright © 2021 Dahl and Nierenberg. This is an open-access article distributed under the terms of the Creative Commons Attribution License (CC BY). The use, distribution or reproduction in other forums is permitted, provided the original author(s) and the copyright owner(s) are credited and that the original publication in this journal is cited, in accordance with accepted academic practice. No use, distribution or reproduction is permitted which does not comply with these terms.
*Correspondence: Tove I. Dahl, dG92ZS5kYWhsQHVpdC5ubw==