- 1School of Education, Culture and Communication, Mälardalen University, Västerås, Sweden
- 2NCM, University of Gothenburg, Göteborg, Sweden
We reassess the relation between students’ socioeconomic status (SES) and their achievement by treating SES as multidimensional instead of unidimensional. We use data from almost 600,000 students in 77 countries participating in the 2018 PISA assessment of student achievement in math, science, and reading. The composite measure of SES that PISA uses can be broken down into six component variables that we here use as simultaneous predictors of achievement. This analysis yields several new insights. First, in the typical society, two predictors (books at home and parents’ highest occupational status) clearly outperform the rest. Second, a new composite measure based only on these two components often reveals substantially larger achievement gaps than those reported by PISA. Third, the analysis revealed remarkable differences between societies in the relation between achievement and wealth possessions. In most societies, the independent effect of wealth possessions on student achievement was zero or even slightly negative—but in the least developed societies it was strongly positive. These findings have implications for how SES achievement gaps should be measured and interpreted.
Introduction
For more than half a century, socioeconomic status (SES) has been recognized as a major influence on student achievement (White, 1982; Coleman et al., 1966; Sirin, 2005; Harwell et al., 2017). In this body of research, SES is typically treated as a unidimensional predictor that can be operationalized in many different ways, such as by parents’ educational attainment, parents’ occupations, or the family’s economic resources, or by a composite of these factors (Cowan et al., 2012). Our research question here is what we can learn by instead treating SES as a multidimensional predictor of achievement.
Definitions of SES typically refer to an individual’s or a family’s position on a hierarchical social structure based on their control and/or access to resources like wealth, prestige, power, and social and cultural capital (Mueller and Parcel, 1981; Willms and Tramonte, 2019). The dominant perspective in this research area is that different socioeconomic factors are not important in their own right. Key socioeconomic factors such as parents’ income and occupations are treated merely as different indicators of the presumed unidimensional hierarchical social structure that influences the achievement of students. In this perspective, the only relevant comparison between different socioeconomic is which of them serves best as an indicator of SES and the literature offers a range of views on this issue (e.g., Blau and Duncan, 1967; Lienet al., 2001; Schulz, 2005; Marks, 2011; Jerrim et al., 2019). A common view is that a composite of several socioeconomic factors is preferred, both to reflect how the concept is defined and to reduce measurement error (Cowan et al., 2012). This is the view taken by the international large-scale assessment PISA, which uses a composite measure based on parental educational attainment, parental occupational status, and home possessions (Avvisati, 2020).
For the big picture about the relation between SES and student achievement, it does not really matter how SES is operationalized. A positive relation with achievement tends to be found whether SES is represented by a single indicator or a composite measure based on several indicators, although the effect size may vary across different operationalizations (White, 1982; Sirin, 2005; Harwell et al., 2017). However, important information may be lost when SES is treated as unidimensional. Our view is that SES should instead be regarded as a multidimensional predictor of achievement with different socioeconomic measures to be used as multiple separate variables. While similar views have been expressed in passing by others (e.g., Harwell et al., 2017; Willms and Tramonte, 2019), it is difficult to find any studies that have actually used multiple separate socioeconomic measures. Harwell and colleagues note that to do so would be at odds with recent recommendations. To defend our view, we must therefore examine the basis for these recommendations.
The NCES Report on the Measurement of Socioeconomic Status
The recommendations referred to by Harwell et al. (2017) are given in a report from a panel of experts convened by the National Center for Education Statistics in the United States (Cowan et al., 2012). The panel was tasked with providing recommendations concerning the definition and measurement of socioeconomic status. Their report explicitly declares an instrumental view of SES: “Researchers and policy makers are interested in SES as a contextual variable to study educational equity and fairness issues, as a covariate with achievement to examine the effects of other variables such as class size or school governance policies, and as a matching variable to ensure the equivalence of treatment and control groups in educational intervention studies” (Cowan et al., 2012, p. 7). The report’s argument against treating SES as multiple separate variables is that doing so could lead to potentially conflicting results for different variables, thereby complicating interpretation. Instead, the report recommends the use of a composite variable to combine information from multiple variables while “avoiding conflicting stories about relationships to achievement” (Cowan et al., 2012, p. 22). In line with the report’s instrumental view of SES, this argument amounts to a preference for simplicity over complexity. However, to the extent that a phenomenon is in fact complex, a simplistic approach may stand in the way of deeper understanding.
Advantages of Treating SES as a Multidimensional Predictor of Achievement
Treating SES as multidimensional instead of unidimensional has several advantages. One advantage is the possibility of gaining a more detailed understanding of the phenomenon. Conspicuously absent in most empirical research on the link between SES and student achievement is any detailed consideration of what mechanisms cause this link in the first place. Yet, detailed knowledge of the pathways through which a socioeconomic advantage turns into an achievement advantage should be of great value to researchers and policy makers interested in leveling the playing field. Potential mechanisms suggested in the literature include genetic transfer of skills across generations, nonfinancial inputs into children’s development (e.g., reading stories and helping with homework), monetary inputs into children’s development (e.g., tuition fees and paying for private tuition), and the negative effects of high stress levels caused by economic hardship (Jerrim and Macmillan, 2015; Rözer and van de Werfhorst, 2019). Note that none of these mechanisms refer to an abstract social hierarchy. Rather it seems that what matters is a variety of more concrete things like genes, skills, money, time, etc. It should therefore be possible to gain a richer understanding by disentangling the separate effects of these things. For instance, consider the possession of wealth. People may acquire wealth in various ways, not all of them related to long education or a high-status occupation. To the extent that wealth has a direct effect on student achievement (the aforementioned “monetary input” pathway), it should be largely independent of where the money comes from and thus separable from other effects. By including parents’ wealth, education, and occupation as simultaneous separate predictors of achievement we can examine whether wealth in fact has any independent effect.
A second advantage of treating SES as multidimensional arises when estimating the total amount of variation in achievement that is accounted for by socioeconomic factors. This can be thought of as the strength of the relation between SES and achievement, or simply the “socioeconomic achievement gap.” Much research has focused on the size of the achievement gap and meta-studies find very different estimates across studies (White, 1982; Sirin, 2005; Harwell et al., 2017). In particular, there are strong indications that different choices of SES components yield different estimates of the achievement gap (Sirin, 2005). If different socioeconomic factors have independent effects on achievement, the use of any single factor will necessarily underestimate the total amount of variation in achievement that is accounted for by socioeconomic factors. An advantage of treating SES as multidimensional is that it helps avoid such underestimation.
Underestimation of the total effect of SES on achievement could also be achieved by use of a composite SES measure that is constructed through an optimal choice of component weights. A third advantage of using SES components as multiple separate predictors is that the results of such analyses provide the optimal weights for a composite measure to avoid underestimation of the SES effect. Extant composite measures are usually constructed based on other principles (Avvisati, 2020), hence do not avoid the underestimation problem.
Finally, estimations of the effect of SES on achievement vary considerably in magnitude across different societies and tend to be lower in developing countries (e.g., OECD, 2018; Kim et al., 2019). This cross-societal variation in SES effects is something of a puzzle, because it is not accounted for by factors such as the number of teaching hours, reduced class size, and teacher quality (Strietholt et al., 2019; Rözer and van de Werfhorst, 2019). However, the cross-societal variation in SES effects may be mysterious in part because it is not well-defined. Prior research suggests that the relative relevance of different socioeconomic factors in a society depends on its development level (Kim et al., 2019). It is therefore possible that country differences in the effect of SES on achievement look completely different if SES is operationalized by, say, wealth or parents’ occupational status. A fourth advantage of treating SES as a multidimensional predictor, specifically in a multi-society study, is that it enables an examination of how different SES measures interact with societal factors. Such analyses could provide crucial insights into the reasons why SES achievement gaps vary with the development level of countries.
Aims of the Current Study
Above we discussed four potential advantages of treating SES as a multidimensional predictor of achievement. The current study aims at empirically demonstrating these advantages using data from the international large-scale assessment PISA. By estimating the independent effects on achievement of the different components of SES, separately in each participating society, we 1) examine which components tend to have the largest independent effects, 2) assess how much the composite measure underestimates the SES achievement gap compared to multiple components, 3) propose an alternative composite measure with more desirable properties, and 4) examine how the independent SES component effects vary with the development level of countries.
Methods
PISA is an international assessment of 15-year-old students’ achievement in math, reading, and science, conducted by the Organization for Economic Cooperation and Development (OECD). PISA uses a representative sample of students from each participating country, with sample sizes usually around 5,000 per country but sometimes substantially larger, see the official report for details on the sampling strategy (OECD, 2019). In the present study we use data from 2018, the most recent wave of PISA for which data is available at this time. We use data from 72 participating countries plus 5 additional participating entities (Hong Kong, Taiwan, Macau, Beijing-Shanghai-Jiangsu-Zhejiang, and Baku in Azerbaijan), for a total of 77 societies with almost 600,000 participating students, generally born in 2002. See Supplementary Table S1 for the full list of societies and sample sizes.
Achievement Measures
Full descriptions of the math, science, and reading skills assessed in PISA are available in the official report (OECD, 2019). To assess a broader range of topics at the country level, PISA only tests each student on a subset of the complete tests. Based on their test results, 10 “plausible values” are imputed for students’ achievement in each domain. Special software is available for analyses of plausible values, see below.
Measures of Socioeconomic Status
To obtain multiple measures of SES we started with PISA′ composite measure and decomposed using subdivisions provided by PISA. As described below, this yielded six component measures: parents’ highest occupational status, parents’ highest educational level, wealth possessions, cultural possessions, home educational resources, and books at home. Below we describe these measures (with the name of the variable in the PISA dataset within parentheses). For further details, see Chapter 16 of the PISA 2018 Technical Report,1 especially table 16.4 in that chapter.
PISA’s Composite Measure of Socioeconomic Status
PISA offers the ESCS composite measure of socioeconomic status. It is based on three variables—parents’ highest occupational status, parents’ highest educational level, and home possessions—which are standardized and then averaged to an index. Home possessions are based on a set of 25 items which PISA subdivides further into four variables: wealth possessions, cultural possessions, home educational resources, and the number of books at home.
Parents’ Highest Occupational Status
PISA asked open-ended questions to students on the occupations of their mother and father. Responses were coded and mapped to an international socioeconomic index of occupational status (Ganzeboom, 2010). HISEI is the higher score of either parent or the only available parent’s score.
Parents’ Highest Educational Level
PISA asked students about the educational level of their mother and father, ranging from primary education to post-graduate education. PAREDINT is the higher level of either parent, transformed into years of education based on an international standard.
Wealth Possessions
The wealth possessions variable is based on 12 items, such as possession of cars and a room of your own. There are also some country-specific wealth items.
Cultural Possessions (CULTPOSS)
The cultural possessions variable is based on 5 items relating to literature, art, and music.
Home Educational Resources
The home educational resources variable is based on 7 items relating to studies at home, such as a desk to study at, a computer to use, a dictionary, etc.
Books at Home (ST013Q01TA)
Students were asked to estimate the number of books at home on a six-step scale (1 = “0–10 books,” 2 = “11–25 books,” 3 = “26–100 books,” 4 = “101–200 books,” 5 = “201–500 books,” 6 = “More than 500 books”). Note that the books at home variable is sometimes used on its own as a single-item measure of SES (e.g., Blömeke et al., 2016; Eriksson et al., 2019).
Analysis
We used the IDB Analyzer provided by the International Association for the Evaluation of Educational Achievement (IEA, 2017). The IDB Analyzer creates SPSS syntax to analyze PISA data in such a way that standard errors correctly reflect the complex design of the study (e.g., the use of plausible values). Using the IDB Analyzer we calculated the correlations, multiple linear regressions, and quartile means described below.
Results
Intercorrelations of SES Components
Table 1 reports descriptive statistics for within-society mean values, standard deviations, and intercorrelations among the six SES components. Note that the correlations between different SES components were not very strong. Averaged across societies, the largest correlations were well below 0.50 and most correlations were below 0.30. In other words, different SES components are not very closely related to each other. There is therefore good reason to conceive of them as distinct dimensions and to examine their independent effects on student achievement.

TABLE 1. Mean (SD) within-society mean values, standard deviations, and Pearson intercorrelations among SES components in 77 societies.
Six SES Components’ Independent Effects on Student Achievement
In each society we performed multiple linear regression analyses of student achievement in three different domains, using all six SES components as predictors. For comparability across SES components, we focus on the standardized coefficients. These coefficients estimate the standardized increase in achievement from an increase of the predictor by one standard deviation.
Domain Generality of SES Component Effects on Achievement
Figure 1 shows the mean value of the coefficient (i.e., averaged across societies) for each SES component, separately for the three academic domains. Note that the pattern of results was almost identical across different academic domains. For example, the effect of books at home was as large in the domains of science and math as in the domain of reading. We may therefore disregard domain in analyses. For each SES component we averaged the effect across the three domains (Cronbach’s α > 0.98). Descriptive statistics of these average effect measures are reported in Table 2.
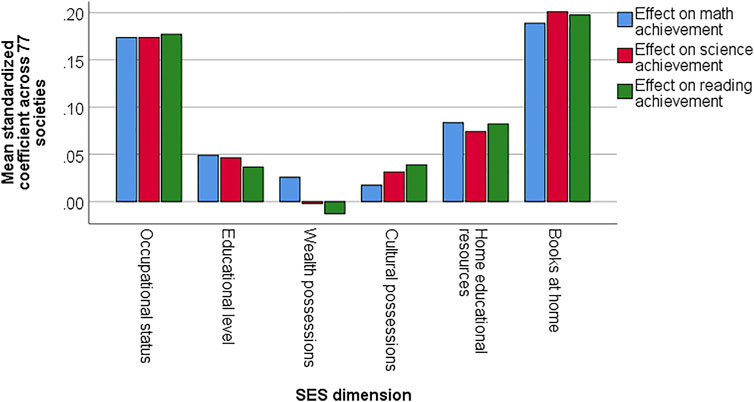
FIGURE 1. Estimates of the independent effects on achievement of six SES components. Bars show mean values of standardized coefficients across 77 societies. Different colors denote different achievement domains: math (blue), science (red), and reading (green).
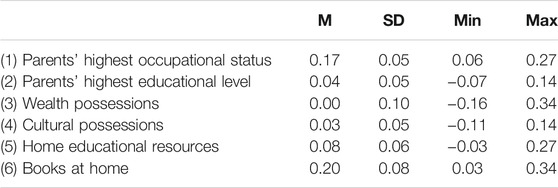
TABLE 2. Descriptive statistics of the standardized coefficients of different SES components, averaged across three academic domains, in 77 societies.
Special Importance of Books at Home and Parents’ Occupations Status
A second thing to note in Figure 1 is that books at home and parents’ occupational status had much larger average effects on student achievement than the other SES components. This is an important finding. Among other things, it suggests that a useful composite SES index could be based on only these two components. We turn to this topic next.
Alternative Measures of the SES Achievement Gap
In their executive report, PISA reports two different measures of the SES achievement gap in a society. One measure is the mean difference in achievement between advantaged students and disadvantaged students, operationalized as the highest and lowest quarter of students on the ESCS index, respectively. Another measure is the proportion of variance in achievement (R2) explained by the ESCS index. The ESCS index adds together the different SES components in a way that does not reflect their relative effects on achievement. Estimates of the SES achievement gap that are based on ESCS index will therefore underestimate the SES achievement gap. To illustrate this underestimation, we construct an alternative SES index based on the two SES components that had the largest effects on student achievement: books at home and parents’ occupational status. After standardization (across the whole dataset) of these two SES components, we average them into a two-item SES index. We compare the estimate of SES achievement gaps when SES was measured by the ESCS vs. the two-item index.
The Two-Item Index Yields Larger Estimates of the Achievement Gap
Figure 2 shows that when using the two-item SES index instead of ESCS, the mean difference in achievement between advantaged students and disadvantaged students increased by about 11% (or 9 points on the test score) in the average society. The largest achievement gap in any society increased even more, by about 19% (or 23 points). Results were similar across all academic domains.
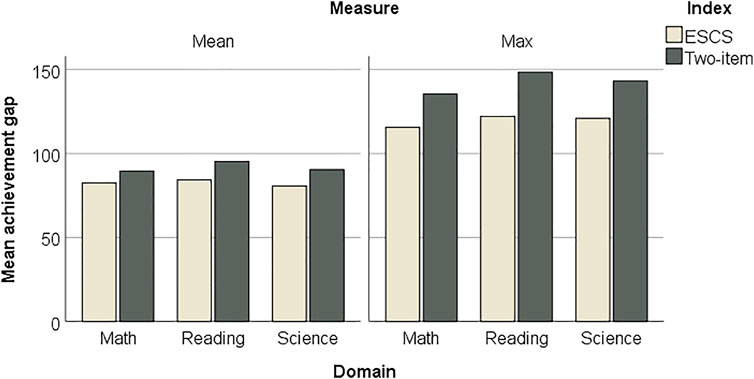
FIGURE 2. SES achievement gaps for math, reading, and science, estimated either using the composite measure provided by PISA (light bars) or using the new two-item SES index (dark bars). The latter index yielded larger gap estimates, whether looking at mean values across 77 societies (left panel) or the maximal value among all 77 societies (right panel).
The Two-Item Index Explains More Variance in Achievement
In Table 3 we report the proportion of variance in student achievement explained by the ESCS index and the two-item SES index, respectively. Consistent with the previous analysis, the two-item SES index explained more variance than the ESCS index in the average society (15 percent vs. 12-13 percent), and the difference was even larger in societies with the largest SES achievement gap (29-31 vs. 21-24 percent variance explained).

TABLE 3. The proportion of variance (R2) in achievement explained by socioeconomic status when SES is operationalized either by PISA’s composite measure (ESCS), or by the new two-item index, or by multiple predictors.
By using all six components as multiple predictors instead we will inevitably be able to explain even more variance. This analysis will produce the optimal weighting of all six components. Because we run separate analyses for each society, weightings will be optimized specifically for every society. The results of this analysis are reported in the last column of Table 3. Despite the inherent advantage of this method in accounting for variance, the proportion of variance explained only increased marginally compared to the simple two-item index. This was particularly evident in the societies with the largest SES achievement gap, where the two-item index accounted for 31 percent of the variance and the six components together accounted for 32 percent, a negligible difference. As usual, similar results were obtained across all three academic domains.
The Multi-Predictor Model is Preferred by the Bayesian Information Criterion
Although the use of multiple predictors allows more variance to be explained, this gain will to some extent reflect overfitting. To assess whether the more complex model is in fact warranted, researchers use model selection criteria such as the Bayesian Information Criterion (BIC). The model that yields a smaller BIC value is preferred. BIC values are not provided in analyses produced by the IDB Analyzer. However, BIC values are provided by SPSS for standard linear regressions. We therefore used SPSS to perform linear regressions corresponding to those presented in Table 3. These alternative analyses replicated the pattern of results in Table 3, that is, ESCS explained less variance than the two-item index, which in turn explained slightly less variance than the multi-predictor model. Moreover, they showed that the same pattern holds for BIC, that is, the ESCS model had a higher BIC value (M = 42,729 across countries and domains) than the two-item index model (M = 39,767), which in turn had a slightly higher BIC value than the multi-predictor model (M = 38,423). We conclude that using SES components as multiple predictors is warranted.
Cross-Societal Variation in the Effects of SES on Achievement
We now turn to how the specific effects of different SES components on student achievement varies across societies. The spread between the minimum and maximum effects among the 77 societies in the study (Table 2) indicates that the cross-societal variation in the size of SES effects is substantial. If we want to order societies on the size of the SES achievement gap, does it matter which SES component we use? To answer this question, we calculated the correlations between the effects of different SES components. See Table 4. Note that these correlations are often negative, such as between the effect of books at home and the effect of wealth possessions. This means that we get completely different lists if we order societies by the size of the achievement gap between students with few vs. many books at home or by the size of the achievement gap between students with few vs. many wealth possessions.
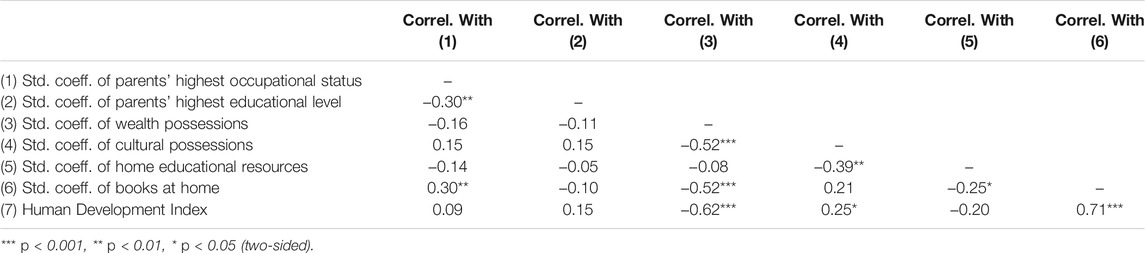
TABLE 4. Mean (SD) Pearson correlations among the standardized coefficients (averaged across academic domains) of six SES components and the Human Development Index in 77 societies.
The Effects of Books at Home and Wealth Vary in Opposite Ways With Development Level
To better understand this discrepancy, we consider the development level of societies, operationalized by the Human Development Index (HDI). We use the latest values available from the United Nations Development Programme (http://hdr.undp.org/) and the Subnational Human Development Database (Smits and Permanyer, 2019). The last row of Table 4 reports how the effects of the six SES components correlate with the HDI. In Table 4 we see that the effect of books at home exhibited a very strong positive correlation with HDI whereas the exact opposite held for the effect of wealth possessions. This means that in societies with a lower level of human development, student achievement is less strongly associated with books at home but more strongly associated with wealth possessions. To illustrate these findings, Figures 3, 4 present scatterplots of societies’ HDI plotted against the effects on achievement (as measured by the average standardized coefficient) of books at home and wealth possessions, respectively. Figure 3 shows that the effect of books at home on achievement was positive everywhere but it was weaker in low-developed societies. Figure 4 shows that while the effect of wealth possessions on achievement was negative in most of the societies in this study, it was nonetheless strongly positive in several low-developed societies.
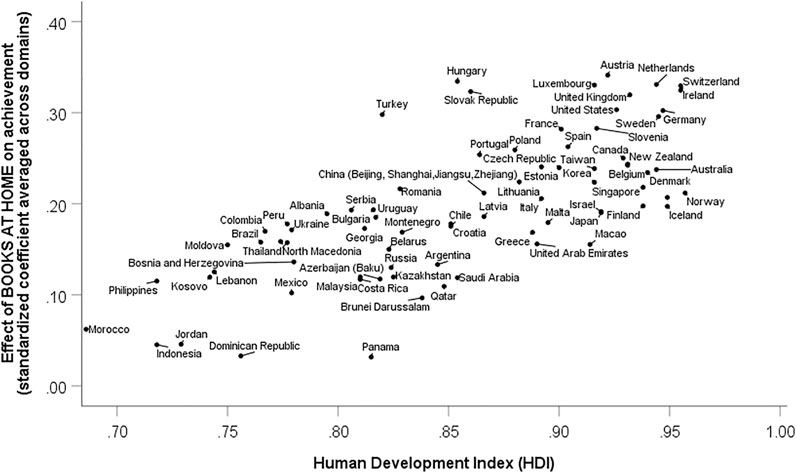
FIGURE 3. Societies’ HDI plotted against the independent effect of books at home on achievement as measured by standardized coefficients averaged across the domains of math, science, and reading.
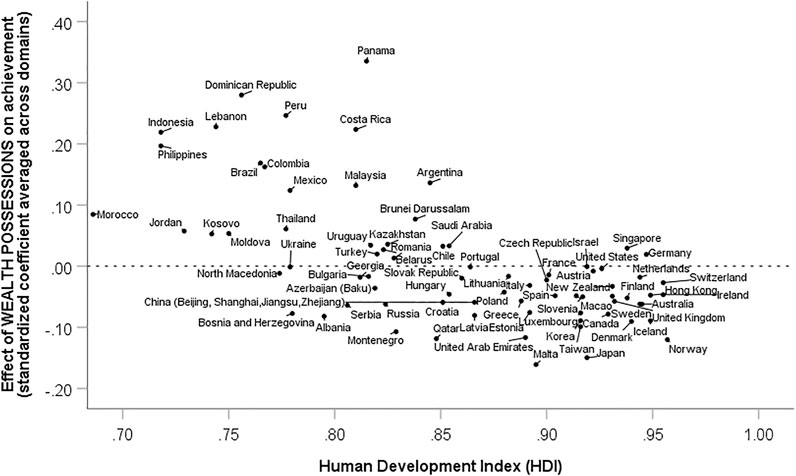
FIGURE 4. Societies’ HDI plotted against the independent effect of wealth possessions on achievement as measured by standardized coefficients averaged across the domains of math, science, and reading.
Discussion
An influential recommendation has been that a multidimensional approach to socioeconomic status should be avoided in education research because it complicates interpretation of results (Cowan et al., 2012). The working assumption behind the current study was that a multidimensional approach can nonetheless be valuable, and that the complexity of the results thus obtained will be a source of insight. We used the six components of PISA’s composite measure of socioeconomic status as multiple predictors of achievement. Analyses of data for almost 600,000 students in 77 societies yielded several interesting findings that crucially rely on the multidimensional approach.
First and foremost, we found very clear results with respect to the relative importance of different SES components. In the average society, the single item on the number of books at home was the strongest predictor of achievement, closely followed by the parents’ highest occupational status. The other four components (parents’ highest educational attainment, home educational resources, cultural possessions, and wealth possessions) tended to contribute little, if at all, to prediction of student achievement. These findings offer a novel way of assessing the validity of theories about the effect of SES on student achievement: Can they account for the primacy of books at home and occupational status? We return to this question below.
Our second finding concerned the size of the SES achievement gap, a topic to which PISA devotes considerable attention. PISA reports achievement gaps estimated using a composite SES measure that is based on the six SES components. Compared to an analysis based on multiple separate components, the use of a composite measure will always underestimate the achievement gap (except when the component weights of the composite measure are chosen to exactly match the multiple regression coefficients). Indeed, by using multiple predictors instead of PISA’s composite measure, the proportion of variance in achievement (R squared) explained by SES increased by 40% in the average society and even more in the society with the largest achievement gap. This finding illustrates that estimates of achievement gaps, whether based on composite measures or single measures, are likely to substantially underestimate the total effect of socioeconomic factors on student achievement. This is important to be aware of when interpreting meta-analyses of such estimates (e.g., Sirin, 2005; Harwell et al., 2017). In the literature there appear to be some misunderstandings around this issue, including unwarranted warnings that a use of a single SES component may somehow overestimate the effect of SES on achievement (Sirin, 2005).
To improve estimations of the achievement gap, it is not necessary to use multiple predictors. It is sufficient to improve the composite measure by adjusting the weights of components to reflect their relative importance. We illustrated this by replacing PISA’s composite measure by a two-item composite measure based only on the two most important components (number of books at home and parents’ highest occupational status). This simple composite measure performed almost as well as the multidimensional approach, especially in societies with large achievement gaps. Compared to using multiple predictors, an advantage of using a single composite measure is that it allows the SES achievement gap to be illustrated in more intuitive ways than as the proportion of variance explained. Following the PISA reports, we illustrated achievement gaps by the mean difference in achievement between the highest and lowest quarter of students on the SES measure. We observed a substantial increase in this gap when it was estimated using the two-item index instead of PISA’s composite measure. We conclude that to grasp the extent of the SES achievement gap, the two-item index does a better job. It may also serve the purpose of making researchers aware that books at home and parents’ occupational status are especially important predictors of achievement in the average society. Thus, for researchers who require a single measure of SES that is relevant across many countries (though not all, see below), we recommend the two-item index over the index provided by PISA.
Last, we examined how results varied across societies. These analyses showed a large systematic influence of societies’ level of human development. Specifically, books at home had a much more positive effect on achievement in the most developed societies than in the least developed societies, where instead wealth possessions had a substantial positive effect on achievement (while having no positive effect at all in the most developed societies). An important conclusion is that the special importance of books at home and parents’ occupational status is not universal. Researchers should be aware that cross-societal comparisons of the SES achievement gap may yield completely different results depending on how SES is operationalized. This underscores the value of taking the multidimensional approach to SES and applying it separately to each country. We recommend that researchers take the multidimensional approach using those SES components that are available to them, which ideally would include occupational status, books at home, and economic resources. In this study we have used the SES components available in PISA, which were limited in that there was only a proxy measure of economic resources (wealth possessions) instead of a direct measure such as household disposable income.
Theories About SES Effects: Direct Causation vs. Trait Transfer
So far, we have discussed our findings from a methodological perspective. However, as we mentioned, they also have implications for theories about why SES is related to achievement. We shall consider two broad classes of potential mechanisms: direct causation and trait transfer. By direct causation we mean that the parental possessions that SES measures are used to directly benefit children’s achievement in school. For example, more wealth allows more monetary input into children’s education, while more education may allow more non-financial input, such as quality help with schoolwork (Jerrim and Macmillan, 2015; Rözer and van de Werfhorst, 2019). If direct causation is an important mechanism, student achievement could be raised by giving parents more money and more education. However, direct causation does not seem to account for our finding that, in most countries, the SES effect is not attributable to parents’ educational attainment and wealth possessions but mainly to their occupational status and the number of books at home. It is difficult to see how parents’ occupational status could directly cause higher achievement. A direct effect of the number of books at home on reading achievement could arise if children tend to read the books they find at home, but this hypothesis does not account for our finding of an equally strong effect of books at home on achievement in mathematics. For these reasons, our findings suggest that direct causation is not the main reason behind the SES effect. This conclusion is in line with studies of adopted children finding no clear influence of socioeconomic factors among adoptive parents, such as their education, on children’s educational attainment (Kendler et al., 2015; Ludeke et al., 2021).
An alternative theory is trait transfer. This theory builds on the combination of two assumptions that are well supported by studies. The first assumption is that achievement in school and achievement of high socioeconomic status (in terms of educational attainment and high-status and high-paying jobs) partly rely on a common set of traits, such as intelligence, self-efficacy, and a conscientious personality (Briley et al., 2014; Krapohl et al., 2014). The second assumption is that these achievement-promoting traits are, to a large extent, genetically transferred from parents to children (Krapohl et al., 2014; Ayorech et al., 2017; Garon-Carrier et al., 2017). Transfer of achievement-promoting traits would readily account for the observed relation between children’s achievement in school and parents’ occupational status. That the independent effect of parents’ educational attainment was much smaller is consistent with educational attainment being a less reliable indicator of achievement-promoting traits, especially in societies where most people get a long education (Chmielewski, 2019).
Parents’ wealth possessions (e.g., cars and mobile phones) were found to have a positive relation to children’s achievement in school only in countries with low levels of development. To see how trait transfer may account for this finding, consider that high economic development makes wealth possessions affordable for most people (Pokropek et al., 2017) and appears to lead to post-materialist values where wealth possessions are no longer what people strive for (Ahuvia and Wong, 2002). For these reasons, wealth possessions would be a poor indicator of achievement-promoting traits specifically in societies with high economic development. In addition, it is plausible that the direct effects of monetary input and economic hardship are more substantial in societies with low economic development.
A remaining puzzle is why books at home is so strongly related to student achievement, and especially so in countries with high levels of development. Here we speculate that, to the extent that people can afford buying books they desire, the number of books at home indicates parents’ general interest in, and enjoyment of, reading. It is plausible that these traits facilitate schoolwork and that they are subject to genetic transfer. This would account for the observed main effect. In poorer countries, those who would like to read books may not afford to buy them, however. This would make the number of books at home a poorer indicator of parents’ reading enjoyment, consistent with the decrease of the observed effect of books at home at lower levels of economic development. Moreover, the reported number of books at home may be less accurate in countries with lower development level (Rutkowski and Rutkowski, 2010), which would also contribute to the decrease of the observed effect.
In sum, although the main aim of this research was to contribute to the methodological debate, we believe that our empirical findings also may inform theories about the pathways of the SES effect on achievement. This important issue requires much more research.
Conclusion
To conclude, this study took a multidimensional approach to socioeconomic status, thereby revealing a striking variation in the effect of socioeconomic factors on achievement. The effect varies both across different factors and across societies, so that higher development is associated with increased importance of some factors and decreased importance of other factors. These findings have implications for how the SES achievement gap should be measured as well as for how it may be explained.
Data Availability Statement
The datasets presented in this study can be found in online repositories. The names of the repository/repositories and accession number(s) can be found below: osf.io/69tpu/.
Ethics Statement
Ethical review and approval was not required for the study on human participants in accordance with the local legislation and institutional requirements. Written informed consent from the participants’ legal guardian/next of kin was not required to participate in this study in accordance with the national legislation and the institutional requirements.
Author Contributions
KE conceived of the study, performed the analyses, and wrote the paper. JL, OH, and AR assisted with the survey of the literature and the interpretation of results. All authors read and approved the submitted version.
Funding
This work was supported by the Swedish Research Council under Grants 2014-2008 and 2014-2468.
Conflict of Interest
The authors declare that the research was conducted in the absence of any commercial or financial relationships that could be construed as a potential conflict of interest.
Publisher’s Note
All claims expressed in this article are solely those of the authors and do not necessarily represent those of their affiliated organizations, or those of the publisher, the editors and the reviewers. Any product that may be evaluated in this article, or claim that may be made by its manufacturer, is not guaranteed or endorsed by the publisher.
Supplementary Material
The Supplementary Material for this article can be found online at: https://www.frontiersin.org/articles/10.3389/feduc.2021.731634/full#supplementary-material
Footnotes
1https://www.oecd.org/pisa/data/pisa2018technicalreport/PISA2018_Technical-Report-Chapter-16-Background-Questionnaires.pdf
References
Ahuvia, A. C., and Wong, N. Y. (2002). Personality and Values Based Materialism: Their Relationship and Origins. J. Consumer Psychol. 12 (4), 389–402. doi:10.1016/S1057-7408(16)30089-4
Avvisati, F. (2020). The Measure of Socio-Economic Status in PISA: a Review and Some Suggested Improvements. Large-scale Assess. Educ. 8, 1–37. doi:10.1186/s40536-020-00086-x
Ayorech, Z., Krapohl, E., Plomin, R., and von Stumm, S. (2017). Genetic Influence on Intergenerational Educational Attainment. Psychol. Sci. 28 (9), 1302–1310. doi:10.1177/0956797617707270
Blömeke, S., Olsen, R. V., and Suhl, U. (2016). “Relation of Student Achievement to the Quality of Their Teachers and Instructional Quality,” in Teacher Quality, Instructional Quality and Student Outcomes. Editors T. Nilsen, and J. E. Gustafsson (Berlin: Springer), 21–50. doi:10.1007/978-3-319-41252-8_2
Briley, D. A., Domiteaux, M., and Tucker-Drob, E. M. (2014). Achievement-relevant Personality: Relations with the Big Five and Validation of an Efficient Instrument. Learn. Individ Differ. 32, 26–39. doi:10.1016/j.lindif.2014.03.010
Chmielewski, A. K. (2019). The Global Increase in the Socioeconomic Achievement gap, 1964 to 2015. Am. Sociol. Rev. 84 (3), 517–544. doi:10.1177/0003122419847165
Coleman, J. S., Campbell, E. Q., Hobson, C. J., McPartland, J., Mood, A. M., Weinfeld, F. D., et al. (1966). Equality of Educational Opportunity. Washington, DC: U.S. Department of Health Education and Welfare, U.S. Government Printing Office.
Cowan, C. R., Hauser, R. M., Kominski, R. A., Levin, H. M., Lucas, S. R., Morgan, S. L., et al. (2012). Improving the Measurement of Socioeconomic Status for the National Assessment of Educational Progress: A Theoretical Foundation. Washington, DC: National Center for Education Statistics.
Eriksson, K., Helenius, O., and Ryve, A. (2019). Using TIMSS Items to Evaluate the Effectiveness of Different Instructional Practices. Instr. Sci. 47 (1), 1–18. doi:10.1007/s11251-018-9473-1
Ganzeboom, H. B. G. (2010). “A New International Socio-Economic Index [ISEI] of Occupational Status for the International Standard Classification of Occupation 2008 [ISCO-08] Constructed with Data from the ISSP 2002-2007,” in In paper presented at annual conference of international social survey programme, Lisbon, Portugal. Retrieved June 2021. Available at: http://www.harryganzeboom.nl/pdf/2010-Ganzeboom-ISEI08-ISSP-Lisbon-%28paper%29.
Garon-Carrier, G., Boivin, M., Kovas, Y., Feng, B., Brendgen, M., Vitaro, F., et al. (2017). Persistent Genetic and Family-wide Environmental Contributions to Early Number Knowledge and Later Achievement in Mathematics. Psychol. Sci. 28 (12), 1707–1718. doi:10.1177/0956797617721480
Harwell, M., Maeda, Y., Bishop, K., and Xie, A. (2017). The Surprisingly Modest Relationship between SES and Educational Achievement. J. Exp. Educ. 85 (2), 197–214. doi:10.1080/00220973.2015.1123668
Jerrim, J., and Macmillan, L. (2015). Income Inequality, Intergenerational Mobility, and the Great Gatsby Curve: Is Education the Key? Social Forces 94 (2), 505–533. doi:10.1093/sf/sov075
Jerrim, J., Volante, L., Klinger, D. A., and Schnepf, S. V. (2019). “Socioeconomic Inequality and Student Outcomes across Education Systems,” in Socioeconomic Inequality and Student Outcomes. Education Policy & Social Inequality. Editors L. Volante, S. Schnepf, J. Jerrim, and D. Klinger (Singapore: Springer), Vol. 4, 3–16. doi:10.1007/978-981-13-9863-6_1
Kendler, K. S., Turkheimer, E., Ohlsson, H., Sundquist, J., and Sundquist, K. (2015). Family Environment and the Malleability of Cognitive Ability: A Swedish National home-reared and Adopted-Away Cosibling Control Study. Proc. Natl. Acad. Sci. U S A. 112 (15), 4612–4617. doi:10.1073/pnas.1417106112
Kim, S. W., Cho, H., and Kim, L. Y. (2019). Socioeconomic Status and Academic Outcomes in Developing Countries: A Meta-Analysis. Rev. Educ. Res. 89 (6), 875–916. doi:10.3102/0034654319877155
Krapohl, E., Rimfeld, K., Shakeshaft, N. G., Trzaskowski, M., McMillan, A., Pingault, J. B., et al. (2014). The High Heritability of Educational Achievement Reflects many Genetically Influenced Traits, Not Just Intelligence. Proc. Natl. Acad. Sci. U S A. 111 (42), 15273–15278. doi:10.1073/pnas.1408777111
Lien, N., Friestad, C., and Klepp, K. I. (2001). Adolescents' Proxy Reports of Parents' Socioeconomic Status: How Valid Are They? J. Epidemiol. Community Health 55 (10), 731–737. doi:10.1136/jech.55.10.731
Ludeke, S. G., Gensowski, M., Junge, S. Y., Kirkpatrick, R. M., John, O. P., and Andersen, S. C. (2021). Does Parental Education Influence Child Educational Outcomes? A Developmental Analysis in a Full-Population Sample and Adoptee Design. J. Pers Soc. Psychol. 120 (4), 1074–1090. doi:10.1037/pspp0000314
Marks, G. N. (2011). Issues in the Conceptualisation and Measurement of Socioeconomic Background: Do Different Measures Generate Different Conclusions? Soc. Indic Res. 104 (2), 225–251. doi:10.1007/s11205-010-9741-1
Mueller, C. W., and Parcel, T. L. (1981). Measures of Socioeconomic Status: Alternatives and Recommendations. Child. Develop. 52 (1), 13–20. doi:10.2307/1129211
OECD (2018). Equity in Education: Breaking Down Barriers to Social Mobility. Paris: PISA, OECD Publishing.
Pokropek, A., Borgonovi, F., and McCormick, C. (2017). On the Cross-Country Comparability of Indicators of Socioeconomic Resources in PISA. Appl. Meas. Educ. 30 (4), 243–258. doi:10.1080/08957347.2017.1353985
Rözer, J. J., and van de Werfhorst, H. G. (2019). “Achievement Inequalities and the Impact of Educational Institutions,” in ISOTIS Report (D 1.4a) (Amsterdam: University of Amsterdam).
Rutkowski, L., and Rutkowski, D. (2010). Getting it 'better': the Importance of Improving Background Questionnaires in International Large‐scale Assessment. J. Curriculum Stud. 42 (3), 411–430. doi:10.1080/00220272.2010.487546
Schulz, W. (2005). Measuring the Socio-Economic Background of Students and its Effect on Achievement in PISA 2000 and PISA 2003. San Francisco, CA: Paper presented at the annual meeting of the American Educational Research Association.
Sirin, S. R. (2005). Socioeconomic Status and Academic Achievement: A Meta-Analytic Review of Research. Rev. Educ. Res. 75 (3), 417–453. doi:10.3102/00346543075003417
Smits, J., and Permanyer, I. (2019). The Subnational Human Development Database. Sci. Data 6 (1), 190038. doi:10.1038/sdata.2019.38
Strietholt, R., Gustafsson, J.-E., Hogrebe, N., Rolfe, V., Rosén, M., Steinmann, I., et al. (2019). “The Impact of Education Policies on Socioeconomic Inequality in Student Achievement: A Review of Comparative Studies,” in Socioeconomic Inequality and Student Outcomes: Cross National Trends, Policies and Practices. Editors L. Volante, S. V. Schnepf, J. Jerrim, and D. A. Klinger (Singapore: Springer), 17–38. doi:10.1007/978-981-13-9863-6_2
White, K. R. (1982). The Relation between Socioeconomic Status and Academic Achievement. Psychol. Bull. 91 (3), 461–481. doi:10.1037/0033-2909.91.3.461
Keywords: student achievement, achievement gap, socioeconomic status, human development, composite measure
Citation: Eriksson K, Lindvall J, Helenius O and Ryve A (2021) Socioeconomic Status as a Multidimensional Predictor of Student Achievement in 77 Societies. Front. Educ. 6:731634. doi: 10.3389/feduc.2021.731634
Received: 27 June 2021; Accepted: 26 October 2021;
Published: 22 November 2021.
Edited by:
Ana E. Azpilicueta, Consejo Nacional de Investigaciones Científicas y Técnicas (CONICET), ArgentinaReviewed by:
Kim Koh, University of Calgary, CanadaLokman Akbay, Istanbul University-Cerrahpasa, Turkey
Copyright © 2021 Eriksson, Lindvall, Helenius and Ryve. This is an open-access article distributed under the terms of the Creative Commons Attribution License (CC BY). The use, distribution or reproduction in other forums is permitted, provided the original author(s) and the copyright owner(s) are credited and that the original publication in this journal is cited, in accordance with accepted academic practice. No use, distribution or reproduction is permitted which does not comply with these terms.
*Correspondence: Kimmo Eriksson, a2ltbW8uZXJpa3Nzb25AbWRoLnNl