- Department of Psychology, Faculty of Health Sciences, UiT The Arctic University of Norway, Tromsø, Norway
Why do students leave universities? The current study addresses the problem of academic attrition from the perspective of students’ intentions. Specifically, we focus on the roles of academic self-efficacy and procrastination in exploring their relationships with attrition intentions. Based on existing research, we expected a negative relationship between academic self-efficacy and attrition intentions, with procrastination as a possible mediator. Furthermore, it was expected that this relationship would differ depending on the type of attrition (i.e., drop-out, transfer university, transfer study field). These hypotheses were investigated among Norwegian students in a questionnaire study (N = 693). Results showed that procrastination partially mediated the relationship between academic self-efficacy and three attrition intentions categories. Although procrastination was a significant mediator of self-efficacy for all types of intentions, the sizes of the direct and indirect effects were different. We conclude that academic procrastination is important in understanding the relationship between students’ self-efficacy beliefs and attrition intentions.
Introduction
The rates of students’ departure before degree completion (i.e., academic attrition) remain relatively high across Europe, with 24% of students leaving higher education before obtaining formal degree qualifications (OECD, 2019). Internationally, academic attrition remains on the agenda of higher education stakeholders. The increased importance of formal education, detrimental societal and personal consequences of academic attrition are among the main reasons for increased attention to the issue. For example, personal consequences might include short- and long-term economic consequences (i.e., needing to pay back study loans while earning lower wages due to the lack of formal qualifications) as well as reduced physical health and general well-being (Mayhew et al., 2016; Zajacova and Lawrence, 2018; Kirp, 2019). The leading social consequence is an inefficient use of government funding which might have more detrimental consequences in countries with state-funded higher education systems (OECD, 2021a,c). Therefore, research providing good explanations seems required to facilitate more effective solutions.
Academic attrition has usually been addressed from the perspective of students’ actual behavior, despite research evidence on the role of intentions in explaining human behavior (e.g., Sheeran, 2002; Morwitz and Munz, 2020). Although some theoretical models address the role of attrition intentions (e.g., Bean, 1982; Tinto, 1993; Bean and Eaton, 2000), they do not differentiate between types of students’ attrition (e.g., leaving permanently, changing university). However, evidence shows that the predictive ability of intentions might be dependent on the behavior in question (e.g., Sheeran, 2002). Although intentions might be a good predictor of students’ permanent departure from the university, the same might not be the case for changing university.
In turn, focusing on different types of students’ attrition intentions might enable institutions to better address and assist students during the process of disengagement. For example, students intending to change the place of education or study field but continue their education may receive better support before (e.g., considering alternative solutions, providing information about the process) and during (e.g., grades transfer assistance) the actual transfer which might be beneficial for time spent on obtaining a degree (Li, 2010; Spencer, 2021). Students intending to leave altogether might need different types of support. Hence, counselors and university staff might adjust assistance or intervention strategies accordingly by knowing students’ intentions. Interventions based on a vague definition of the target population and their intentions, on the other hand, may be limited in their effectiveness (e.g., Hovdhaugen, 2011).
The present study aims to address some of the central student-related factors and mechanisms involved in the process of attrition intentions formation. Understanding the mechanisms involved may assist researchers and practitioners in developing, assessing, and refining the assistance programs. In particular, the present study aims to assess the relationship of two psychologically grounded factors (i.e., academic self-efficacy and procrastination) with different types of attrition intentions. As will be discussed, academic self-efficacy is related to procrastination and students’ attrition. However, few studies have investigated the relationship between procrastination and academic attrition. Further, these factors have not been examined accounting for different attrition intentions (e.g., leaving entirely, changing an academic institution). Hence, we first present a brief overview of academic attrition and the role of behavioral intentions. Then we proceed with an overview of the factors of interest in the present paper, self-efficacy and procrastination.
Academic Attrition and Its Variability
Researchers have used different terminology to describe that some students leave their studies before getting an official degree qualification. The operationalization of the phenomenon varied from “wastage” (e.g., Cross and Hall, 1954) to more recent “attrition.” However, a common feature shared by both operationalizations is their negative connotation1. Although neither wastage nor attrition are appropriate to fully describe student departure, we will use the term academic attrition, an umbrella term for all types of academic discontinuations.
However, it is important to acknowledge that there are different forms of academic attrition. The notion that all students leaving higher education are not the same can be traced back to Tinto (1993). In his seminal work, Tinto (1993, Chapter 2) provides a synthesis of research and, importantly, distinguishes between two main categories of students’ departure, institutional and system departure. The first type of departure describes a pattern of attrition when students switch academic institutions (i.e., transferring out), while the second distinguishes students who leave the wider education system altogether (i.e., dropping out). The categorization was primarily based on the registry data and pattern of students’ behavior after leaving university. This distinction was seen as crucial since different factors were assumed to be involved. If an academic institution aims to handle departure, it is essential to know which type of departure a university is dealing with, institutional or system.
The institutional-system distinction is supported by research evidence indicating non-uniformity of the student population (Hoyt and Winn, 2004; Hovdhaugen, 2009; Jones-White et al., 2010; Kehm et al., 2019). For example, previous and current academic performance, or “problems related to meeting academic standards,” are reported more frequently as reasons for leaving by drop-out than by transfer-out students (Hoyt and Winn, 2004; Hovdhaugen, 2009, 2011; Hovdhaugen and Aamodt, 2009). Indeed, transfer-out students have comparable performance with direct-entry students (Aulck and West, 2017; Quinn-Nilas et al., 2019). Also, Hovdhaugen (2009) found that background characteristics such as age, gender, and school grades are significantly related to dropping out, but not so for transfer-out behaviors. Transfer-out was more strongly related to students’ motivation, educational goals, and field of study.
Behavioral Intentions to Leave Education
Behavioral intention is one of the most studied factors in basic and applied research on human behavior (Morwitz and Munz, 2020). Based on a meta-analysis by Sheeran (2002), intentions explain 28% of the variance in behaviors including alcohol consumption, weight loss, seatbelt use, training, smoking, and cancer screening, to name but a few. These findings align with the assumptions of the Theory of Reasoned Action (TRA)/Theory of Planned Behavior (TPB) stating that intentions are the closest antecedents of actual behaviors (Fishbein and Ajzen, 1975; Ajzen, 1991). According to TRA and TPB, intentions capture the motivational factors influencing actual behaviors. Intentions are indicators of how hard people are willing to try and how much effort they are planning to exert to perform behaviors. It is assumed that the stronger the intention to perform a behavior is, the more likely a person is to perform the behavior.
However, behavioral intentions or intentions to leave education have been rarely included in a theoretical discussion on academic attrition. This can be partially explained by the predominance of the sociological perspective on the issue (for review, see Melguizo, 2011; Aljohani, 2016; Behr et al., 2020). Nevertheless, some classical theories of academic attrition and their reevaluations acknowledged the importance of students’ intentions. For example, the ideas from TRA/TPB (i.e., intentions as antecedents of behaviors) were implemented in the student attrition models by Bean (1982) and Cabrera et al. (1993). The authors found that intentions to leave were the best predictor of students’ actual attrition. Also, the importance of student’s attrition intention as an antecedent of actual behavior is asserted in the models by Tinto (1993) and Bean and Eaton (2000). Although the models agree on the role of intentions, they do not address the variability of academic attrition. As discussed, different factors are related to the different types of attrition, and thus it might be the case for students’ intentions. Moreover, based on the analysis of items used to measure students’ intentions, the classical studies might have assessed students’ persistence intentions (e.g., “Do you expect to return to this university next fall”; Bean, 1982). Still, it is evident that reasons for staying can differ from reasons for leaving.
To summarize, emerging evidence shows that transfer-out and drop-out students leave universities for different reasons. Thus, operationalizing and measuring students’ departure in general terms such as wastage or attrition may lead to imprecise results and conclusions. For example, the overrepresentation of drop-out students in a study sample might lead to findings that are hardly applicable to transfer-out students, and the other way around. Further, few studies investigated differences in factors related to students’ intentions. The central assumption of the majority of proposed theoretical models and frameworks is that students’ attrition results from their interaction with the academic environment. Still, what is lacking in the interactionalist perspective and research on academic attrition is factors that are relevant for students and their learning. Further, relatively few studies have focused on factors that are malleable and for which evidence on possible interventions is available. In the present study, we aim to address these issues by assessing the relationship of academic self-efficacy and procrastination with students’ drop-out, transfer university, and transfer study field intentions. As will be discussed, both factors may have theoretical and practical utility.
Factors and Mechanisms Involved in Academic Attrition
Academic Self-Efficacy
From a student’s perspective, attrition can be seen as a manifestation of a flaw in motivation. According to results of multiple meta-analyses and reviews (e.g., Robbins et al., 2004; Richardson et al., 2012; Schneider and Preckel, 2017), academic self-efficacy shows the strongest relationship with both academic performance2 and persistence. Also, indirect evidence shows that self-efficacy might be related to both dropping and transferring out behaviors. According to the Social Cognitive Theory (Bandura, 1997), individuals’ confidence in their ability to perform a required course of action to solve a problem or achieve a desired goal (i.e., self-efficacy) is important for understanding human motivation and behavior. The basic principle behind self-efficacy is that individuals are more likely to engage, exert more effort, and persist in activities for which they have high self-efficacy. By and large, the evidence supports the theoretical predictions on the relationship of self-efficacy beliefs with the amount of effort devoted to and persistence on a certain task (Van Dinther et al., 2011; Jackson et al., 2012; Komarraju and Nadler, 2013; Puente−Diaz and Cavazos−Arroyo, 2018). In turn, students’ efforts are related to both drop-out and transfer-out behaviors (Hovdhaugen, 2009).
Further, self-efficacy beliefs play a major role in Bean and Eaton’s (2000) model of academic attrition. Similar to Tinto’s (1975, 1993) and related theoretical models, student-university interaction is an important part of the model by Bean and Eaton (2000). Nevertheless, it adds an individual perspective or students’ self-assesments of their interaction with university into the explanation of the attrition process. In particular, Bean and Eaton (2000) assumed that as the result of interaction with the university’s environment, students’ academic and social self-efficacy increases or decreases facilitating persistence or attrition intentions and actual behavior. Hence, the relationship between self-efficacy and students’ attrition intentions can be assumed. Also, according to the Theory of Planned Behavior, self-efficacy as a dimension of behavioral control is a crucial aspect in the formation of behavioral intentions and has a direct relationship with actual behavior (Ajzen, 1991, 2002, 2020). According to TPB (Ajzen, 1991, 2002), behavior is primarily determined by attitudes toward behavior, subjective social norms or pressure from significant others, and perceived behavioral control (PBC). Individual’s attitudes, subjective norms, and PBC influence behavior by facilitating intention to act. The theory assumes that behavioral intentions, which summarize the motivational forces (i.e., attitudes, subjective norms, and PBC), are the most approximate predictors of behaviors. In addition, the theory also suggests that PBC can have a direct impact on behavior.
Hence, academic self-efficacy is related to students’ attrition intentions and actual attrition behaviors. Although the results of Robbins et al. (2004) meta-analysis support the importance of self-efficacy for students’ retention, the size of the relationship was only moderate. Nevertheless, we argue that this relationship is crucial and has a great theoretical and practical utility. First, from a practical perspective, self-efficacy is a cognitive belief that is malleable to change (Bandura, 1997; Van Dinther et al., 2011; Bartimote-Aufflick et al., 2016). Second, from a theoretical perspective, the evidence on the relationship of self-efficacy with different categories of students’ attrition (i.e., drop-out, transfer-out) is scarce. Third, according to Weissberg and Owen (2005), the findings of Robbins et al. (2004) might not be equally applicable to commuter students, which is the case for many European universities and our study sample. Thus, research on the importance of students’ self-efficacy for different attrition intentions is of particular interest.
Malleability of Self-Efficacy
As noted, self-efficacy is assumed to effect engagement, effort, and persistence in tasks and behaviors (Bandura, 1997; Van Dinther et al., 2011). According to the Social Cognitive Theory (Bandura, 1997), there are four primary sources of information that influence or create self-efficacy: mastery experience (previous success experience), vicarious (observational) experience, social persuasion, and physical/affective states. The common characteristic describing these four sources of self-efficacy is that they are based on personal experience meaning that self-efficacy may be improved. Indeed, the evidence supports the theory’s assertion. For example, Bartimote-Aufflick et al. (2016) reviewed 64 articles indicating 17 intervention studies investigating if certain teaching strategies or approaches can improve students’ self-efficacy. Among these studies, ten interventions demonstrated improvement in participants’ self-efficacy beliefs. In particular, facilitating opportunities to work with peers, helping students identify their misconceptions, including multimedia into the learning process, providing additional resources and activities for challenging concepts, and encouraging students to share their personal experiences were effective. Also, Van Dinther et al. (2011) note that interventions based on the Social Cognitive Theory are more effective with mastery experiences having the most powerful influence on self-efficacy beliefs. Here, providing practical experience such as performing a task while applying knowledge and skills in a demanding situation is argued to facilitate mastery experience. In addition, goal setting combined with self-reflection (i.e., self-regulation components) may influence students’ perception of progress leading to mastery experience.
Procrastination and Academic Attrition
Procrastination has been defined as a voluntary delay of an intended course of action despite expecting to be worse off for doing so (Steel, 2007; Klingsieck, 2013). Procrastination can occur in all possible areas but is especially prevalent in the academic context (i.e., academic procrastination; Steel, 2007). Poor academic achievement, perceived stress, depression, and anxiety are among the potential outcomes of students’ tendency to procrastinate (Steel, 2007; Klassen et al., 2008; Kim and Seo, 2015; Rozental et al., 2015; Sirois, 2016). To the best of our knowledge, only few studies have investigated the role of procrastination in academic attrition. For example, Grau and Minguillon (2013) demonstrated that students taking online programs who procrastinated in returning to university after taking a break from studies were more likely to leave permanently (i.e., drop out). Further, Bäulke et al. (2018) found that procrastination is related to drop-out intentions and mediated the relationship between motivational regulation and students’ intentions. Also, results of a qualitative study by Visser et al. (2018) indicated that students scoring high on academic procrastination reported that they considered quitting their studies. Finally, Herrmann and Brandstätter (2015) found that an action crisis was predictive of disengagement from academic goals (i.e., dropout). An action crisis is a decisional conflict between continuing and disengaging from the pursuit of a personal goal. As defined by Herrmann and Brandstätter (2015), this conflict is characterized by six dimensions, including procrastination. However, to the best of our knowledge, there is no evidence on whether procrastination is related to other types of academic attrition (i.e., transfer-out intentions and behaviors).
Malleability of Procrastination
Similar to self-efficacy beliefs, research evidence shows that academic procrastination can be ameliorated (see meta-analysis by Van Eerde and Klingsieck, 2018, Malouff and Schutte, 2019). According to Van Eerde and Klingsieck (2018), cognitive-behavior therapy is the most effective approach. Still, such interventions are usually either ad hoc, time-consuming or require the involvement of professionals. Thus, interventions that would enable educators to support students effectively within their natural academic environment with little additional effort are of particular interest. According to Wäschle et al. (2014), one of such approaches may be strengthening students’ self-efficacy beliefs. These authors argued that high self-efficacy facilitates students’ achievement by increasing their motivation and application of effective learning strategies. Achievement, in turn, contributes to and raises self-efficacy which should facilitate students’ motivation and achievement during the next learning cycle (i.e., virtuous cycle of self-efficacy). The results of the study supported these assumptions and indicated that self-efficacy beliefs have an important role in counteracting procrastination.
Procrastination as a Mediator
The research shows a close relationship between self-efficacy and procrastination. According to the Social Cognitive Theory (Bandura, 1997), high self-efficacy should increase students’ effort and persistence devoted to a task. Hence, a negative relationship between self-efficacy and procrastination characterized by reduced effort and persistence is not unexpected (Van Eerde, 2003; Klassen et al., 2008; Wu and Fan, 2017). In addition, experimental evidence shows that altering students’ negative and irrational thoughts (e.g., low self-efficacy) may be effective in reducing procrastination (Visser et al., 2017). The findings can be explained by the Temporal Motivational Theory (TMT; Steel and König, 2006). According to TMT, self-efficacy (an indicator of the expectancy construct) is crucial in explaining procrastination. In particular, motivation to perform a behavior (i.e., utility) is increased when people are confident of acquiring the desired reward (i.e., expectancy) or outcome (i.e., value). In turn, increased motivation should increase task performance or reduce task delay (i.e., procrastination).
In addition, although direct evidence on the environmentally driven nature of procrastination is scarce, different lines of research suggest that procrastination may be ingrained into the academic environment (Klingsieck, 2013; Svartdal et al., 2020). Hence, procrastination might represent an unintended environmental characteristic (i.e., academic system; Tinto, 1993) facilitating students’ attrition intentions and actual attrition behaviors (Bean and Eaton, 2000). Likewise, evidence on the negative relationship of procrastination with academic performance is well-established (Steel, 2007; Kim and Seo, 2015). In turn, students’ performance is a central aspect of the student-university interaction perspective where performance is commonly defined as a mediating factor in the process of academic attrition (Aljohani, 2016). Finally, seen from a different perspective, academic attrition can be seen as a result of a goal-disengagement process (Brandstätter and Bernecker, 2021). In turn, action crisis characterized by delaying a goal pursuit (i.e., procrastination) has been commonly found to precede actual goal-disengagement (Herrmann and Brandstätter, 2015). Action crisis typically arises when individuals suffer from repeated setbacks. In the case of students, the setbacks may be determined by their self-efficacy beliefs (for review, see Honicke and Broadbent, 2016).
In sum, different lines of research suggest that having low self-efficacy beliefs may be detrimental to students’ academic success and persistence. In this study, we will investigate whether this relationship can be explained (i.e., mediated) by students’ tendency to procrastinate. As discussed, although the assumption is reasonable, there is no evidence on whether procrastination is related to other types of academic attrition beyond dropout (i.e., transfer-out intentions and behaviors). Hence, we aim to elucidate this aspect which may have practical utility for universities since both academic self-efficacy and procrastination are malleable to change (e.g., Van Dinther et al., 2011; Wäschle et al., 2014; Bartimote-Aufflick et al., 2016; Van Eerde and Klingsieck, 2018).
Background Factors
Also, we considered several potentially relevant covariates including gender, age, high-school GPA, study field, university affiliation, years studied, parents’ education, and history of changing study field or university. Previous empirical research suggests a relationship between students’ background factors and actual attrition. For example, Hovdhaugen (2009) found that females, younger students, students whose parents have higher education, and students having better high-school GPAs are less likely to drop out. In contrast, transferring to another university is less likely when students are older and study natural sciences. Also, some evidence shows that females are more likely to switch majors (i.e., transfer study field) than males (Astorne-Figari and Speer, 2018; Meyer et al., 2021). Still, based on the findings of Ishitani and Flood (2018a), females may be less prone to change university (i.e., transfer university). Further, researchers note that attrition, including transferring out, varies across study fields and programs (DesJardins et al., 2003; Danaher et al., 2008; Ishitani and Flood, 2018b; Korhonen and Rautopuro, 2019). According to Wolter et al. (2014), students who have previously changed their study field or major are more likely to drop out. Similarly, changing university (i.e., history of changing university) was found to be negatively related to students’ degree attainment and persistence (Ishitani, 2008; Li, 2010). Finally, Willcoxson (2010), Willcoxson et al. (2011), and Ishitani and Flood (2018b) found that different factors may drive students to drop and transfer out depending on their study year and university affiliation.
The findings that background factors (i.e., age, gender, high-school GPA) are important in the process of students attrition are in line with available theoretical models and frameworks (e.g., Tinto, 1975, 1993; Pascarella et al., 1983; Bean and Metzner, 1985). Still, the described associations are primarily found for students’ actual behavior while evidence on students’ intentions is scarce. Based on TPB, stating that intentions are the closest antecedents of actual behaviors, we assumed that the described factors are important for students’ attrition intentions and, therefore, appropriate to control for in the analyses.
The Current Study
Academic attrition and persistence have been commonly viewed as the result of interaction between students and their academic environment leading to either persistence or attrition. Still, the mechanisms involved in the process of student-university interaction have rarely been addressed explicitly. In the present paper, we focus on the relationship of students’ self-efficacy with different categories of attrition intentions (i.e., drop-out, transfer university, and transfer study field). As discussed, the relationship between self-efficacy beliefs and students’ persistence/attrition is well-documented in the research literature (Robbins et al., 2004). Still, the evidence on the relationship of self-efficacy with other types of departure (i.e., transfer university or study field) is less clear. Also, there is little evidence on the mechanisms that explain this relationship. In the present study, we investigated if procrastination is one of such mechanisms. Self-efficacy beliefs are relatively strongly related to procrastination (Van Eerde, 2003; Klassen et al., 2008) which, in turn, is related to students’ drop-out intentions (Bäulke et al., 2018). As discussed, low self-efficacy may incline students to delay and devote less effort to academic tasks facilitating students’ attrition intentions (Van Eerde, 2003; Klassen et al., 2008; Hovdhaugen, 2009; Wu and Fan, 2017). In addition, seen as an environmental characteristic, procrastination may be important in the student-environment interaction process traditionally used to explain academic attrition (Tinto, 1993; Bean and Eaton, 2000; Svartdal et al., 2020). Finally, although it remains unknown whether interventions aimed at self-efficacy and procrastination substantially reduce academic attrition, the literature suggests that both factors are amenable to change. In this study, we assume that self-efficacy is negatively related to procrastination and attrition intentions (Hypothesis 1). Further, the relationship between self-efficacy and attrition intentions is mediated by students’ procrastination tendency (Hypothesis 2). Finally (Hypothesis 3), we aim to explore if the observed relationships (i.e., direct and indirect) would differ for three types of attrition intentions (i.e., drop-out, transfer university, and transfer study field). It is expected that the observed relationships would differ for three types of intentions.
Materials and Methods
Sample and Setting
Participants were 693 students (65% females) in different stages of their education: first-year (26%), second-year (25%), third-year (19%), fourth-year (13%), fifth-year (10%), and sixth-year or more (7%). Age ranged from 19 to 54 with a mean of 23.9 years (SD = 4.79). The data was collected at the beginning of the spring semester (January- March) 2020 before the COVID restriction. The response and completion rates were satisfactory (41.2 and 88.5%, respectively).
Assessment and Measurement
Procedure and Ethics
Students were contacted via the university’s e-mail and received an invitation to the study containing a brief study summary. Following the link, respondents were presented with a consent form, informed that they were anonymous and could refrain from answering or withdraw from the study at any time. Participants agreed to participate in the web-based survey by pressing a start survey button after reading information about the study. Uncompleted and suspicious responses (e.g., fast completion time) were excluded from analyses. The study was approved by the Norwegian Center for Research Data (NSD) in accordance with the requirements of data protection legislation (reference code 651244). The data for the present study is available on Open Science Framework (OSF)3. Participants could also participate in a random tracking of a gift card with a value of 1000 NOK. These participants provided their phone numbers which were recorded and stored separately from the rest of the data. Phone numbers were deleted when a winner had been chosen.
Covariates of Attrition Intentions
Students were asked to report their gender, age, high-school GPA, study field, university affiliation, years studied, parents’ education, and history of changing study field or university (see Supplementary Table 8 for descriptive data). Age was an open-ended question. High-school GPA was a categorical variable consisting of six categories (1 = Lowest grade; 6 = Highest grade). Study field was recorded into five categories: psychology; humanities and social science; science, technology, engineering and math (STEM); medicine and health science; biology and fishery. Parents’ education included four categories: lower-secondary education, upper-secondary education, higher education, and other. Responses of students who chose “other” were recorded as missing. Parents’ education was not distinguished into the mother’s and father’s levels of education based on data privacy considerations. University affiliation consisted of two categories: University of Tromsø (UiT) and Norwegian University of Science and Technology (NTNU). Only 18 participants were from other universities and, thus, were recorded as missing. Number of years studied at university was a six-categories variable (1 = 1 year; 6 = 6 years or more). Participants who have studied for 4 years and above were merged into one category due to the small sample size in the last two categories (i.e., 5, 6 years, and more). We also included two questions about students’ previous history of changing study fields and history of changing academic institutions (0 = No; 1 = Yes). Parents’ education (with university’s education as the reference group), university affiliation (with students from NTNU as the reference group), number of years studied (with 1 year as the reference category), and study field (with medicine as the reference group) were dummy coded for subsequent mediation analyses. The reference category was chosen based on the easiness of interpretation (e.g., years studied). The medicine field was chosen as the reference group based on present results showing the most differences with other study fields. High-school GPA was subsequently excluded from the mediation analysis. According to the Social Cognitive Theory (Bandura, 1997), high school GPA is the antecedent of self-efficacy (i.e., previous or mastery experiences). In the study, it was related to students’ academic self-efficacy beliefs (i.e., independent variable) and was insignificantly related to attrition intentions. Exclusion of high-school GPA did not lead to substantial changes in the estimated relationships.
Academic Self-Efficacy
The measurement index was borrowed from a Danish study by Herrmann et al. (2017). The scale is based on MSLQ (Motivated Strategies for Learning Questionnaire) by Pintrich (1991). Three items were chosen based on the reported highest factor loadings (Herrmann et al., 2017). An example item is: “I am confident that I can acquire the skills necessary to excel within my field of study” with higher scores indicating stronger self-efficacy beliefs (1 = Totally disagree; 5 = Totally agree). The items were translated to Norwegian with forward-back translation. Internal reliability (Cronbach’s alpha) was 0.80. The measure was significantly related to students’ self-reported academic performance (r = 0.39) and three study strategies subscales (relating ideas, r = 0.32; time-management, r = 0.38; unrelated memorizing, r = –0.39) consistent with the research literature (Robbins et al., 2004; Diseth, 2011; Richardson et al., 2012). This particular scale was chosen since the pure self-efficacy scale (i.e., task- or subject-specific) was deemed inappropriate in the context of the present study (i.e., students from different study fields). Still, it is worth mentioning that such decision could raise some questions about the construct validity (i.e., self-concept/self-efficacy distinction; Marsh et al., 2019).
Procrastination
A subset of four items from the Academic Procrastination Scale (APS; Mccloskey and Scielzo, 2015; Yockey, 2016) measured academic procrastination (e.g., “I know that I should work on a school work, but I just don’t’ do it”; “Cramming and last-minute studying is the best way that I study for a big test”). Based on the exploratory factor analysis performed before the main analysis, one item was excluded due to factor loading below 0.40 and low communality. The items were translated to Norwegian with forward-back translation. All items are rated on a 5-point scale with higher scores indicating more procrastination (1 = Totally disagree; 5 = Totally agree). Cronbach’s alpha for 25 items was 0.94 (Mccloskey and Scielzo, 2015). The three items used in this study had Cronbach’s alpha of 0.85. The measure was significantly related to students’ self-reported academic performance (r = –0.20) and three study strategies subscales (relating ideas, r = –0.08; time-management, r = –0.71; unrelated memorizing, r = 0.23) consistent with the research literature (Richardson et al., 2012; Saele et al., 2017).
Attrition Intentions
In the present study, we used four-item measure of students’ intentions to drop out, transfer to another university, and transfer to another study field. Although the research on behavioral intentions is extensive (Sheeran, 2002), there is scarce evidence on validated and psychometrically sound measures of intentions (Fishman et al., 2020). Based on findings that intentions/thoughts of performing an action can vary in the degree of their specificity (Mashburn, 2000; Gollwitzer, 2012; Bäulke et al., 2021), we borrowed the first two items from the study by Hardre and Reeve (2003). Based on the face validity, they represented the first two (i.e., deliberation; intention or Rubicon) mindset phases of goal pursuit (Gollwitzer, 2012). The items were: “I sometimes consider dropping out of university before graduation,” “I intend to drop out of school before graduation.” Further, we designed two additional items for the study: “I sometimes think that other job opportunities suit me better than those I can get with my current education”; “I know what I am going to do if I withdraw from my studies.” The items were intended to measure the deliberation and planning phases. Similar items were designed for transfer university intentions: “I sometimes think about how my life would be if I change my study place”; “I have a plan for when and how I will change my study place.” The second pair of items measuring transfer study field intentions were the following: “I sometimes think about advantages and disadvantages of changing study field”; “I am waiting for the possibility to change my study field.” Participants were also presented with a descriptive text for transfer study field intentions specifying the high-cost transfer (e.g., history → science; Meyer et al., 2021). Exploratory factor analysis was performed to test the dimensionality of the items. Based on the results, only two items for each type of intention were retained. All items are rated on a 5-point scale with higher scores indicating higher intentions. Spearman-Brown coefficient for drop-out, transfer university and transfer study field intentions were 0.73, 0.76, and 0.82 (Eisinga et al., 2013).
Analysis
Model Specification and Estimation
A structural equation model (SEM) using weighted least squares parameter (WLSMV) estimation was employed. The WLSMV estimation is appropriate when manifest variables are categorical or ordinal, and the sample size is relatively large (Muthén and Muthén, 2017). Model fit data were examined using the chi-square test (χ2), Comparative Fit Index (CFI), Tucker-Lewis Fit Index (TLI), Root Mean Square Error of Approximation (RMSEA), and Standardized Root Mean Square Residual (SRMR). For a more detailed description and discussion of the fit indices, the reader is referred to Hu and Bentler (1999) and Brown (2015). Standard fit cut-off values were applied: CFI, TLI > 0.95, SRMR < 0.08, and RMSEA < 0.06 (Hu and Bentler, 1999). Values equal to or lesser/higher than cut-off values indicate good or close fit. Although the traditional approach to mediation using ordinary least squares or Baron and Kenny’s (1986) stepwise approach is widely used, we chose the SEM alternative. Based on the recent evaluations of the approaches to mediation analysis (e.g., Iacobucci et al., 2007; Kline, 2015), SEM seems to be superior to Baron and Kenny’s (1986) regression approach. For example, SEM provides more accurate or less biased estimations due to adjustment for measurement error which is not possible with traditional mediation approaches. Confirmatory factor analysis (CFA) was performed to assess the validity of the measurement model (see Supplementary Materials). The results of CFA indicated an excellent fit: χ2 = 94.737, df = 44, p < 0.001; CFI = 0.993; TLI = 0.989; RMSEA = 0.041 (90% CI 0.029 – 0.052); SRMR = 0.028.
The results of observed indirect effects were interpreted in concordance with Zhao et al. (2010) approach to mediation analysis. The main characteristic and the difference of this approach from the traditionally applied Baron and Kenny’s (1986) mediation analysis is the dependent-independent variables relationship. In particular, Zhao et al. (2010) argue that a zero-order relationship between dependent and independent variables should not necessarily be significant for proceeding with the mediation analysis. Under certain conditions (e.g., presence of mediator variables with opposite effects, presence of suppressing variables, temporal distance), a mediator variable may be exercising its effect even when no significant dependent-independent variables relationship is found. The main requirement for mediation is the significant interaction effect (i.e., indirect effect). Further, consistent with the proposed mediation approach, the authors provided an alternative to the «full, partial, and no mediation» categorization of mediation patterns. Complementary mediation is present when mediated and direct effects are significant and point in the same direction. In contrast, competitive mediation assumes that the same effects are present but point in the opposite direction. Indirect-only mediation describes a pattern when the mediated effect is significant while the direct effect is not. Direct-only non-mediation and no-effect non-mediation are patterns when either only direct effect is significant or all the relationships between variables are insignificant.
Results
Academic Self-Efficacy and Drop-Out Intentions via Procrastination
The chi-square test was significant (χ2 = 99.820, df = 44, p < 0.01) for the model without covariates. However, the chi-square test statistics is sensitive to sample size and is usually significant in large samples (Hooper et al., 2008). Other fit indices indicated a very good model fit, CFI = 0.991; TLI = 0.986; RMSEA = 0.043 (90% CI 0.032–0.054); SRMR = 0.028. As seen in Figure 1, academic self-efficacy was negatively related to procrastination (β = –0.265, boot SE = 0.047, p < 0.001), which in turn was positively related to drop-out intentions (β = 0.277, boot SE = 0.054, p < 0.001). The direct effect from academic self-efficacy to drop-out intentions was significant and in expected direction (β = –0.395, boot SE = 0.052, p < 0.001). The indirect effect via procrastination was also significant and in the same direction as the direct effect (β = –0.074, boot SE = 0.019, p < 0.001), indicating complementary mediation (Zhao et al., 2010). The total effect was significant (β = –0.469, boot SE = 0.048, p < 0.001). Hence, procrastination “partially” mediated the relationship between academic self-efficacy and drop-out intentions. All additional estimates are provided in the Supplementary Table 1. Including covariates into the model did not substantially alter either model fit or mediation model relationships (see Supplementary Table 2).
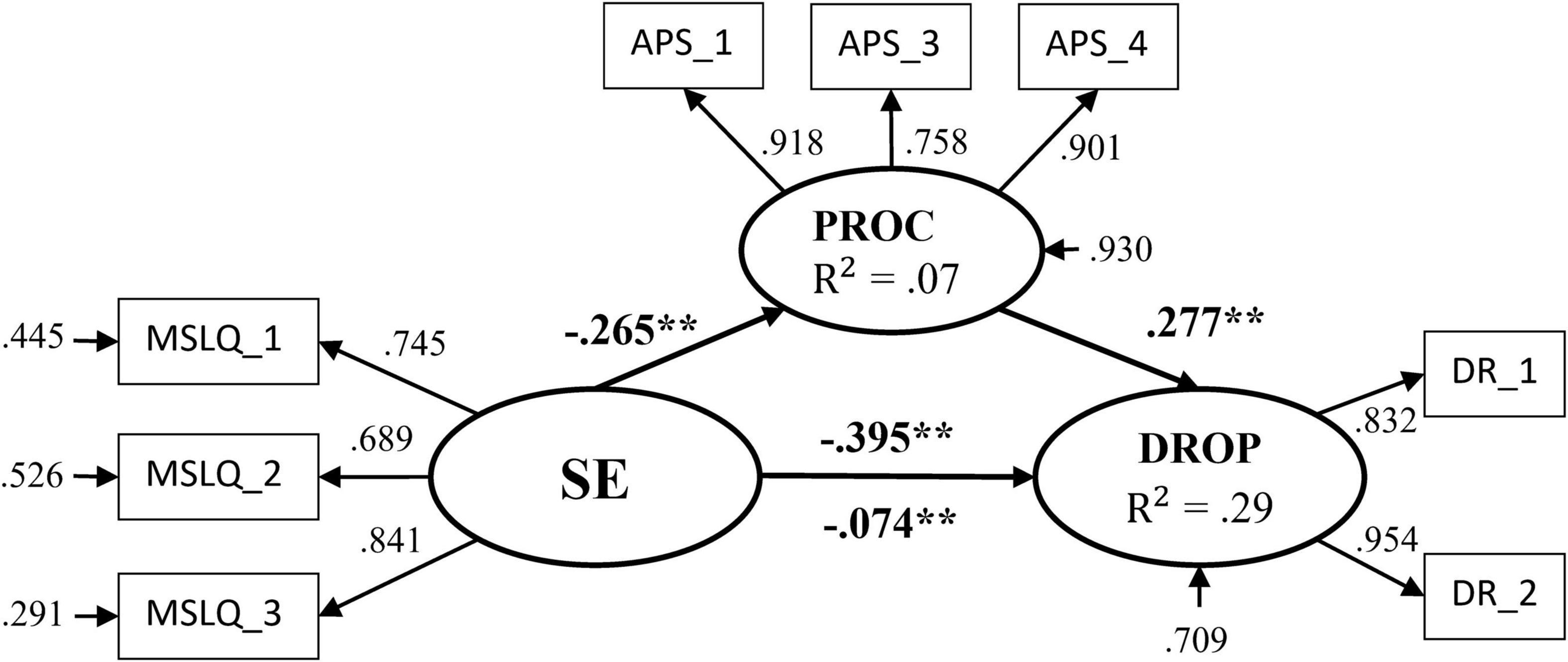
Figure 1. Mediation model for drop-out intentions (n = 693). Indirect effect is provided below the path line. SE, academic self-efficacy; PROC, procrastination; DROP, drop-out intentions. **p ≤ 0.001.
Academic Self-Efficacy and Transfer University Intentions via Procrastination
The overall model fit for transfer university intentions without covariates was very good. The chi-square test was significant (χ2 = 99.820, df = 44, p < 0.01); CFI = 0.991; TLI = 0.986; RMSEA = 0.043 (90% CI 0.032–0.054); SRMR = 0.028. As seen in Figure 2, academic self-efficacy was negatively related to procrastination (β = –0.265, boot SE = 0.047, p < 0.001), which in turn was positively related to transfer university intentions (β = 0.168, boot SE = 0.066, p < 0.01). The direct effect from academic self-efficacy to transfer university intentions was insignificant and in expected direction (β = –0.102, boot SE = 0.063, p = 0.11). Still, the indirect effect via procrastination was significant and in the same direction as the direct effect (β = –0.045, boot SE = 0.020, p < 0.01), indicating indirect-only mediation. The total effect was significant (β = –0.212, boot SE = 0.049, p < 0.001). Hence, procrastination “fully” mediated the relationship between academic self-efficacy and transfer university intentions. All additional estimates are provided in the Supplementary Table 3. Including covariates into the model did not substantially change the overall model fit: χ2 = 274.910, df = 188, p < 0.001; CFI = 0.982; TLI = 0.975; RMSEA = 0.029 (90% CI 0.021 – 0.036); SRMR = 0.054. However, type of mediation changed from the indirect-only to complementary (see Supplementary Table 4). In particular, the direct relationship between academic self-efficacy and transfer university intentions became significant (β = –0.204, boot SE = 0.069, p < 0.01).
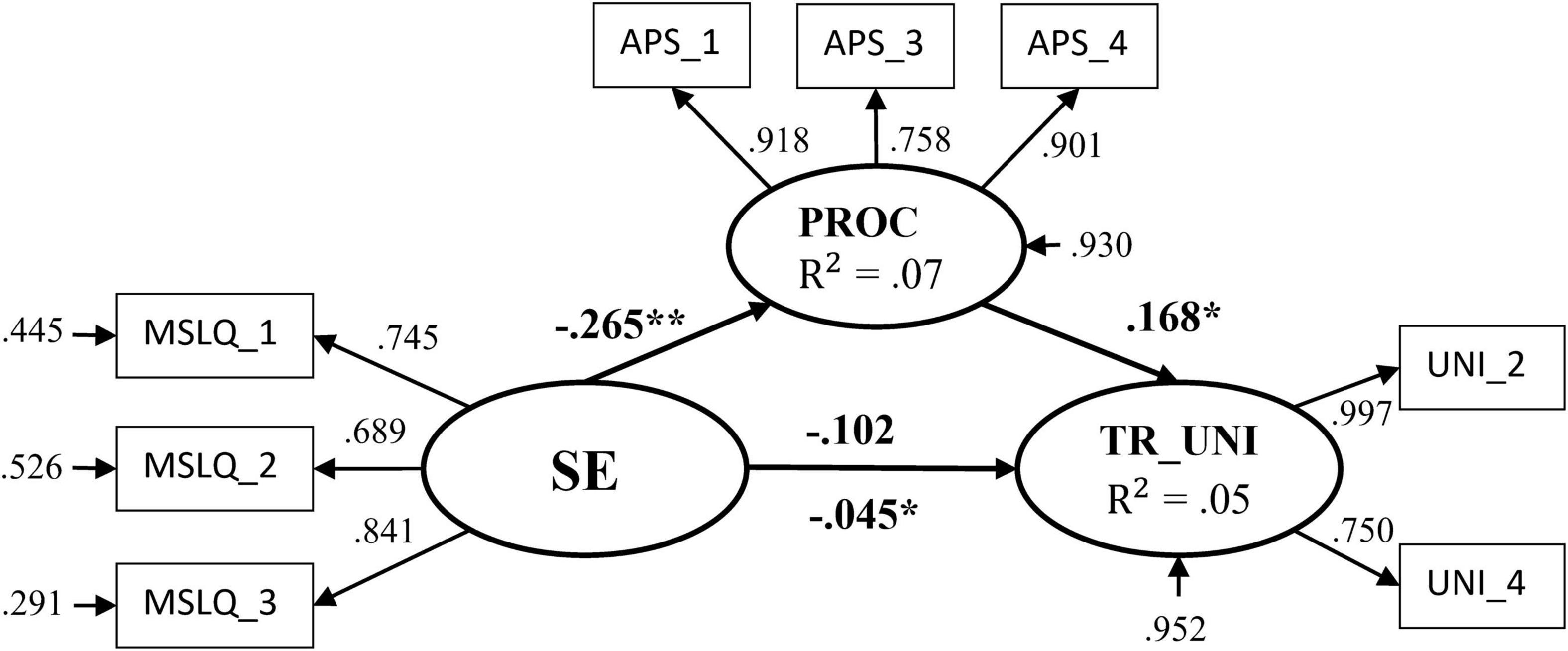
Figure 2. Mediation model for transfer university intentions (n = 693). Indirect effect is provided below the path line. SE, Academic self-efficacy; PROC, procrastination; TR_UNI, transfer university intentions. *p ≤ 0.01, **p ≤ 0.001.
Academic Self-Efficacy and Transfer Study Field Intentions via Procrastination
The overall model fit for transfer study field intentions without covariates was very good. The chi-square test was significant (χ2 = 99.820, df = 44, p < 0.01); CFI = 0.991; TLI = 0.986; RMSEA = 0.043 (90% CI 0.032–0.054); SRMR = 0.028. As seen in Figure 3, academic self-efficacy was negatively related to procrastination (β = –0.265, boot SE = 0.047, p < 0.001), which in turn was positively related to transfer study field intentions (β = 0.181, boot SE = 0.057, p < 0.001). The direct effect from academic self-efficacy to transfer study field intentions was significant and in expected direction (β = –0.229, boot SE = 0.053, p < 0.001). The indirect effect via procrastination was also significant and in the same direction as the direct effect (β = –0.048, boot SE = 0.018, p < 0.001), indicating complimentary mediation. The total effect was significant (β = –0.276, boot SE = 0.049, p < 0.001). Hence, procrastination “partially” mediated the relationship between academic self-efficacy and transfer study field intentions. All additional estimates are provided in the Supplementary Table 5. Including covariates into the model did not substantially alter either model fit or mediation model relationships (see Supplementary Table 6).
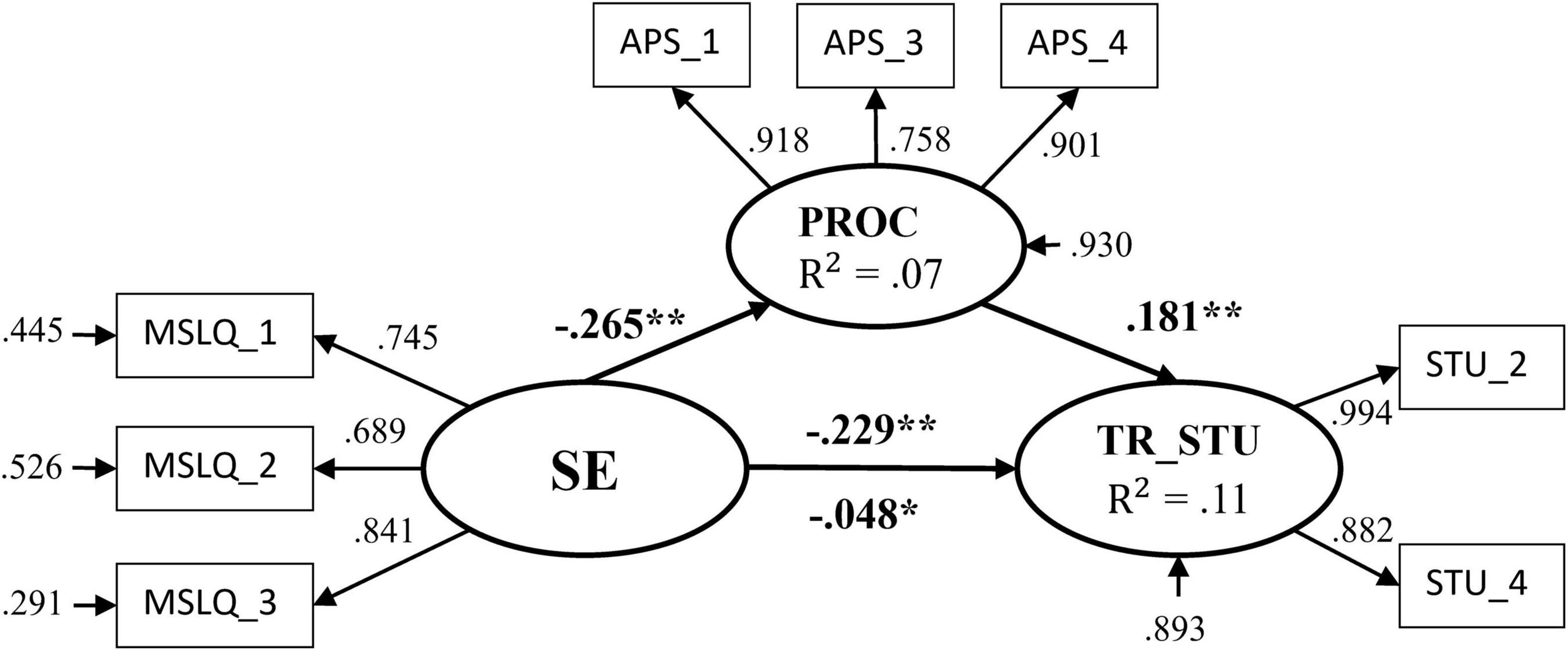
Figure 3. Mediation model for transfer study field intentions (n = 693). Indirect effect is provided below the path line. SE, academic self-efficacy; PROC, procrastination; TR_STU, transfer study field intentions. *p ≤ 0.01, **p ≤ 0.001.
Results Summary
The results of the three mediatory analyses supported Hypothesis 1 that academic self-efficacy is negatively related to procrastination and attrition intentions. Also, Hypothesis 2 was supported by results showing that the relationship of self-efficacy with drop-out and transfer study field intentions was complementary (partially) mediated by academic procrastination. These findings may indicate that the investigated models have an omitted mediator. In turn, the relationship between self-efficacy and transfer study field intentions was complementary mediated only when covariates were included in the model. Without covariates, procrastination indirect-only or fully mediated the investigated relationship. Hence, the inclusion of covariate variables into the model was reasonable. Finally, Hypothesis 3 was supported by results indicating stronger relationships (i.e., direct and indirect) between self-efficacy and drop-out intentions than it was the case for two types of transfer-out intentions. Also, self-efficacy and procrastination accounted for a larger amount of variance in drop-out intentions (R2 = 29%) than in transfer university (R2 = 5%) and transfer study field intentions (R2 = 11%). The inclusion of control variables did not substantially change the observed relationships for drop-out and transfer study field intentions. In contrast, the relationship between academic self-efficacy and transfer university intentions has become complementary after the inclusion of covariates. In addition, different covariates turned out significant depending on the type of attrition intention. For instance, students’ intentions to drop out and transfer study field differed between medicine and STEM fields with medicine students having fewer intentions. In contrast, no significant difference was found across the study fields for transfer university intentions (see Supplementary Tables 2, 4, 6). In sum, the results indicated the importance of the distinction between different categories of attrition intentions.
Discussion
The present paper aimed to investigate the significance of the distinction between different categories of students’ attrition intentions. Although students’ motivation in general (Demetriou and Schmitz-Sciborski, 2011) and self-efficacy in particular (Bean and Eaton, 2000; Robbins et al., 2004; Willcoxson, 2010; Willcoxson et al., 2011; Tinto, 2017) are important for academic success and persistence, there is scarce evidence on the role of procrastination in academic attrition. In turn, understanding the involved mechanisms might assist researchers and practitioners in developing, assessing, and refining the assistance programs. Further, to the best of our knowledge, none of the previous studies investigated whether these relationships are present when accounting for the variability of academic attrition (i.e., dropping out, transferring out). The present study set out to investigate whether the relationship between academic self-efficacy and procrastination with attrition intentions would differ for drop-out and transfer-out intentions. It was also hypothesized that students’ tendency to procrastinate would mediate the relationship between academic self-efficacy and attrition intentions.
The general pattern of results is in line with previous research. Academic self-efficacy was negatively related to procrastination (Van Eerde, 2003; Steel and König, 2006; Klassen et al., 2008) and attrition intentions (Robbins et al., 2004; Willcoxson, 2010; Willcoxson et al., 2011). Further, procrastination showed a positive relationship with attrition intentions, as in the study by Bäulke et al. (2018). However, our findings supplement Bäulke et al.’s (2018) results by indicating that procrastination is also related to transfer-out intentions. Importantly, when the relationships are considered separately for each category, our findings align with prior evidence on the greater importance of academic factors for dropping out (Tinto, 1993; Hovdhaugen, 2009, 2011; Quinn-Nilas et al., 2019). This is represented by the larger amount of variance accounted for by academic self-efficacy and procrastination in drop-out intentions and larger relationships between academic self-efficacy and drop-out compared to transfer-out intentions. Hence, universities aiming to reduce academic attrition should adjust their strategies accordingly. For example, providing academic mentoring programs focusing on academic skills to reduce transfer university rates may prove less effective than expected.
In addition, our study demonstrates that students’ academic self-efficacy significantly relates to attrition intentions through academic procrastination. It has been traditionally assumed that students’ pre-entry characteristics or previous experiences determine the nature of student-university interaction (Aljohani, 2016). Likewise, past experiences also determine students’ academic self-efficacy beliefs that have a well-established relationship with students’ academic success (Robbins et al., 2004; Richardson et al., 2012). In turn, students who enter university with low self-efficacy might be at a considerable disadvantage compared to students with firm beliefs in their abilities. In particular, students with low self-efficacy tend to devote less effort, persistence to a given task, and procrastinate (Bandura, 1986, 1997; Steel, 2007). According to Wäschle et al. (2014), low self-efficacy may be involved in a vicious circle of procrastination (low self-efficacy, procrastination → poor performance → low self-efficacy → procrastination). Over time, in the face of recurrent setbacks (i.e., low performance), students may start to question the desirability and feasibility of their degree attainment goal leading to subsequent goal disengagement or attrition (Brandstätter and Bernecker, 2021). Even if students enter university with firm self-efficacy beliefs, many students lack the required competencies or abilities to succeed at university such as critical thinking or information literacy (Dunlosky et al., 2013). Lack of such skills in a students’ toolbox puts them at a disadvantage causing poor achievement and, as described, might lead to procrastination and academic attrition.
Nevertheless, procrastination partially mediated the relation of academic self-efficacy with drop-out and transfer-out intentions. Obviously, other mechanisms associated with academic self-efficacy should be explored in future studies. One of the candidates for the role of a mediator is academic performance. According to the Social Cognitive Theory, self-efficacy beliefs influence which course of action a person takes, the amount of effort devoted to a task, resilience, and perseverance in the face of obstacles (Bandura, 1986, 1997). Unsurprisingly, empirical evidence shows a medium-strong relationship between self-efficacy and academic performance (e.g., Robbins et al., 2004; Richardson et al., 2012; Schneider and Preckel, 2017). However, as discussed, performance comes up to be a non-significant determinant of transferring out while it does predict drop-out behaviors. Further, according to social cognitive theory (Bandura, 1997), self-efficacy influences behavior through motivational processes. In turn, Hovdhaugen’s (2009) study shows a significant relationship between students’ motivation (i.e., intrinsic and extrinsic) and transferring out and a non-significant association with dropping out. Finally, self-efficacy is related to students’ effort and commitment (Bandura, 1986, 1997; Weng et al., 2015). Both factors have been found important for students’ drop-out and transfer-out behaviors (Tinto, 1993; Hovdhaugen, 2009). Hence, students’ effort and goal commitment might be additional contributors (i.e., omitted mediators) in explaining the observed relationship of academic self-efficacy with drop-out and transfer-out intentions.
Further, in the present study, we performed the exploratory analysis with a set of covariates to investigate their relationship with attrition intentions and their influence on mediation relationships. The investigated covariates have been found important in relation to actual attrition behaviors. Still, these factors have not been addressed in the context of students’ intentions. Although intentions are good approximators of actual behaviors, still, they do not account for the whole variance in actual behaviors meaning that the factors are not identical (Webb and Sheeran, 2006). Hence, it can be assumed that differences found for actual attrition behaviors (e.g., gender differences) might be absent in the case of students’ intentions. The results of the present study supported this assumption. As discussed, previous findings show that female students are less prone to drop out and switch universities than males (Hovdhaugen, 2009; Ishitani and Flood, 2018a) while they are more likely to switch majors (Astorne-Figari and Speer, 2018; Meyer et al., 2021). However, we did not find any significant gender differences in drop-out, transfer university, and transfer study field intentions. Among investigated covariates, only years studied, study field, and history of changing university were significantly related to attrition intentions in the present study. In line with the findings by Willcoxson (2010), Willcoxson et al. (2011), and Ishitani and Flood (2018b), we found that students’ attrition intentions differed by year of study. In particular, the longer the students studied, the fewer attrition intentions they had. Hence, assisting and paying extra attention to students during their first year at university seems crucial (Willcoxson et al., 2011). Further, it was found that students reporting that they have previously changed university had more transfer university intentions. In addition, student assistance may be less of a concern for some study fields than others. In particular, it was found that medical students have fewer drop-out and transfer-out intentions than students from other study fields (see Supplementary Tables 2, 4, 6). This might be related to higher enrollment standards and programs’ structure (e.g., same students, closer follow-up of the students) than it is the case for other study majors. In sum, our findings show that although academic self-efficacy and procrastination are related to the three types of attrition intentions, adressing the attrition issue should be tailored to specific study programs and student characteristics. Also, considering students’ characteristics such as year of education and previous history of changing study place might be more relevant in the case of transfer-out students based on the results showing a change in mediated relationships after the inclusion of covariates (indirect-only → complementary mediation).
To sum up, the present study shows that academic self-efficacy and procrastination are related to students’ intentions to drop out, change their field of study, and change university. Our results indicate that procrastination might be detrimental not only to traditionally investigated academic performance but also to other aspects of academic success (i.e., persistence). Hence, procrastination might have much more extensive consequences considering the negative relationship of attrition with students’ future economic success and well-being (Hout, 2012; Mayhew et al., 2016). In addition, the size of the relationships, the nature of mediation, and the amount of variance accounted for were dependent on the type of intentions being considered indicating the relevance of the distinction among students’ attrition intentions. Hence, future studies and interventions should be cautious when defining and drawing conclusions about academic attrition and attrition intentions.
Finally, the present study contributes to the current research by investigating the factors that are malleable and may be influenced by universities. For example, Van Dinther et al.’s (2011) literature review shows that self-efficacy interventions based on social cognitive theory are the most effective in improving self-efficacy. Some researchers (e.g., Bartimote-Aufflick et al., 2016) provide research-based best practice suggestions on how students’ self-efficacy can be improved via teaching, learning support, and curriculum design. Similarly, evidence shows that procrastination can be ameliorated, with self-efficacy being one of the proposed alternatives for intervention (Wäschle et al., 2014; Van Eerde and Klingsieck, 2018). Nevertheless, counselors and university staff might need to adjust assistance or intervention strategies. As discussed, evidence and result of the present study show that students switching to another university may do it less due to performance-related problems (Hovdhaugen and Aamodt, 2009; Quinn-Nilas et al., 2019). Thus, assisting students in improving their self-efficacy beliefs when they intend to change university might be a less effective or appropriate solution for these students. In this case, universities might be better of adjusting their strategy based on students’ intentions and known reasons for why these intentions occur. Still, it is worth mentioning that external factors (e.g., work, child care, illness, finances) are also responsible for students’ attrition (Bean, 1985; Bean and Metzner, 1985; Leveson et al., 2013; Hovdhaugen, 2015; Behr et al., 2021). For example, Behr et al. (2021) identified a separate cluster of students who left university for personal (e.g., illness, stay abroad) or family (e.g., child care) reasons. Family or personal reasons were rarely decisive for dropping out and were reported by a small proportion of participants. Still, universities can hardly address these student difficulties directly. Hence, institutional ability to reduce student attrition may be limited indicating the need for more complex state interventions such as financial support or child-care arrangements. Finally, although improving students’ self-efficacy and reducing procrastination may be a prospective approach to tackle students’ attrition, its effectiveness for the students leaving primarily due to external reasons can be questioned.
Limitations and Future Research
One of the main limitations of the current study is the validity of the attrition intentions scale. The measure of students’ intentions used in the present study should be cautiously evaluated since it lacks validation other than face validity. Also, factors with only two indicators are prone to estimation problems when the sample size is small (Kline, 2015). Hence, future psychometric studies developing and validating the attrition intentions scale that is applicable irrespective of statistical analysis are required. Further, although intentions represent the closest antecedent of behavior, they cannot substitute students’ actual behavior (Webb and Sheeran, 2006). Thus, examination of the mechanisms found in the current study when students’ actual behavior is also considered represents a prospective line for future research. In this regard, measures of intentions that depict students’ firm resolution or concrete action plan can be considered for inclusion since they may be more predictive of actual behaviors (Brandstätter et al., 2015; Achtziger and Gollwitzer, 2018; Gollwitzer, 2018). However, implementation intentions (i.e., concrete if-then plans) might be problematic to measure in the context of academic attrition considering the lack of measurement scales and ethical considerations related to experimental designs. Still, future studies might test whether less concrete measures such as action planning would serve as a substitute and better predictor of students’ behaviors (Hagger and Luszczynska, 2014).
Second, the causality of the proposed mediatory mechanisms should be cautiously evaluated due to the correlational study design. In the present study, the directional relationships were derived from the available research literature and theory (Bandura, 1997; Steel and König, 2006; Wu and Fan, 2017; Bäulke et al., 2018). It is reasonable to assume that self-efficacy determines students’ attrition intentions and not the other way around. The results of the meta-analysis of experimental evidence indicate that changes in self-efficacy beliefs lead to changes in health-related intentions and behaviors (Sheeran et al., 2016). Nevertheless, future studies should account for alternative models (Danner et al., 2015) since the relationship between self-efficacy and procrastination may be bi-directional (Wäschle et al., 2014).
Third, the non-probability based sampling method (i.e., convenience sampling) has been used for data collection purposes due to the exploratory nature of the present study. Thus, generalization of the results to the student population should be made with caution. Future studies should preferably acquire the probability-based sampling methods to make more valid inferences about the whole population of Norwegian students.
Fourth, the self-efficacy measure used in the present study can be questioned in terms of its validity. According to Marsh et al. (2019), relatively “pure” self-efficacy measures are characterized by the future orientation and purely descriptive nature of response items and clear frame-of-reference. In particular, the present measure lacks a clear frame of reference such as being confident in obtaining a top grade in a certain course. Although achieving such a standard in the present context (i.e., participants from different study fields) was nearly impossible, future research should clarify this aspect of the present study and if the observed relationships are better explained by a more pure self-efficacy measure. In addition, investigating the role of students’ social self-efficacy may be a prospective line for future research. Based on the classical perspective on academic attrition (i.e., Tinto, 1975, 1993), Bean and Eaton’s (2000) model suggests that academic and social self-efficacy are important in explaining student attrition. Still, to the best of our knowledge, we are not aware of any study which addressed the role of students’ social self-efficacy in explaining different types of academic attrition.
Finally, in the present paper, we investigated the relationships between academic self-efficacy and procrastination with high-cost transfer study field intentions. The high-cost transfer is described by Meyer et al. (2021) as situations when students switch between broad categories of academic disciplines (e.g., history → science). In contrast, a low-cost transfer means situations when students switch within the same academic discipline (e.g., sociology → political science). The distinction is worth noting since Meyer et al. (2021) found that two categories might be related to different factors. In particular, high-school final grades were related to switching across disciplines (i.e., high-cost transfer), while misfit between student’s occupational interests and major’s content was mainly related to switching within disciplines (i.e., low-cost transfer). Thus, the results of the present study are only applicable to the high-cost transfer intentions. Future studies are encouraged to investigate the generalizability of the present findings to low-cost transfer intentions.
Data Availability Statement
The datasets presented in this study can be found in online repositories. The names of the repository/repositories and accession number(s) can be found below: https://osf.io/k8ax4/?view_only=f8cf1a2b15ab4da7b552e4a20a79e125.
Ethics Statement
Ethical review and approval was not required for the study on human participants in accordance with the local legislation and institutional requirements. The patients/participants provided their written informed consent to participate in this study.
Author Contributions
EN wrote the draft and did the statistical analyses under the supervision of FS. TG-K assisted with data analyses. RS, TG-K, and FS edited the manuscript. All authors contributed to the article and approved the submitted version.
Funding
This research was facilitated by funds from the UiT The Arctic University of Norway and publication charges were funded by a grant from the publication fund of UiT The Arctic University of Norway.
Conflict of Interest
The authors declare that the research was conducted in the absence of any commercial or financial relationships that could be construed as a potential conflict of interest.
Publisher’s Note
All claims expressed in this article are solely those of the authors and do not necessarily represent those of their affiliated organizations, or those of the publisher, the editors and the reviewers. Any product that may be evaluated in this article, or claim that may be made by its manufacturer, is not guaranteed or endorsed by the publisher.
Supplementary Material
The Supplementary Material for this article can be found online at: https://www.frontiersin.org/articles/10.3389/feduc.2022.768959/full#supplementary-material
Footnotes
- ^ Still, it is worth mentioning that not all types of students’ departures are necessarily negative or, at least, not for everyone and not in every case (e.g., Faas et al., 2018). For example, changing university might be perceived as something positive from a student’s perspective since he/she is presumably aiming for a degree qualification, only in a more suitable institution/place. Also, some students might take only specific courses to increase their qualifications while being employed. In addition, students may take a break from their studies for one or another reason and subsequently re-enroll to receive their academic degrees. Yet another group might find that higher education is not for them but may go on to something else without any negative consequences.
- ^ Academic performance is the most stable predictor of drop behaviors (Tinto, 1975, 1993; Bean, 1982; Bean and Metzner, 1985; Bean and Eaton, 2000; Robbins et al., 2004). In addition, as discussed in the section on the variability of academic attrition, performance may be important in the distinction of drop-out and transfer-out students.
- ^ https://osf.io/k8ax4/?view_only=f8cf1a2b15ab4da7b552e4a20a79e125
References
Achtziger, A., and Gollwitzer, P. M. (2018). “Motivation and volition in the course of action,” in Motivation and Action, eds J. Heckhausen and H. Heckhausen (Berlin: Springer International Publishing), 485–527. doi: 10.1007/978-3-319-65094-4_12
Ajzen, I. (1991). The theory of planned behavior. Organ. Behav. Hum. Decis. Process. 50, 179–211. doi: 10.1016/0749-5978(91)90020-t
Ajzen, I. (2002). Perceived behavioral control, self−efficacy, locus of control, and the theory of planned behavior. J. Appl. Soc. Psychol. 32, 665–683. doi: 10.1111/j.1559-1816.2002.tb00236.x
Ajzen, I. (2020). The theory of planned behavior: Frequently asked questions. Hum. Behav. Emerg. Technol. 2, 314–324. doi: 10.1002/hbe2.195
Aljohani, O. (2016). A comprehensive review of the major studies and theoretical models of student retention in higher education. High. Educ. Stud. 6, 1–18. doi: 10.5539/hes.v6n2p1
Astorne-Figari, C., and Speer, J. D. (2018). Drop out, switch majors, or persist? The contrasting gender gaps. Econ. Lett. 164, 82–85. doi: 10.1016/j.econlet.2018.01.010
Aulck, L., and West, J. (2017). Attrition and performance of community college transfers. PLoS One 12:e0174683. doi: 10.1371/journal.pone.0174683
Bandura, A. (1986). Social Foundations of Thought and Action: A Social Cognitive Theory. Hoboken, NJ: Prentice-Hall.
Baron, R. M., and Kenny, D. A. (1986). The moderator-mediator variable distinction in social psychological research: conceptual, strategic, and statistical considerations. J. Pers. Soc. Psychol. 51, 1173–1182. doi: 10.1037/0022-3514.51.6.1173
Bartimote-Aufflick, K., Bridgeman, A., Walker, R., Sharma, M., and Smith, L. (2016). The study, evaluation, and improvement of university student self-efficacy. Stud. High. Educ. 41, 1918–1942. doi: 10.1080/03075079.2014.999319
Bäulke, L., Eckerlein, N., and Dresel, M. (2018). Interrelations between motivational regulation, procrastination and college dropout intentions. Unterrichtswissenschaft 46, 461–479. doi: 10.1007/s42010-018-0029-5
Bäulke, L., Grunschel, C., and Dresel, M. (2021). Student dropout at university: a phase-orientated view on quitting studies and changing majors. Eur. J. Psychol. Educ. 24, 1–24. doi: 10.1007/s10212-021-00557-x
Bean, J. (1982). Student attrition, intentions, and confidence: interaction effects in a path model. J. Assoc. Inst. Res. 17, 291–320. doi: 10.1007/bf00977899
Bean, J. P. (1985). Interaction effects based on class level in an explanatory model of college student dropout syndrome. Am. Educ. Res. J. 22, 35–64. doi: 10.3102/00028312022001035
Bean, J. P., and Eaton, S. B. (2000). “A psychological model of college student retention,” in Reworking the Student Departure Puzzle, ed. J. M. Braxton (Nashville: Vanderbilt University Press).
Bean, J. P., and Metzner, B. S. (1985). A conceptual model of nontraditional undergraduate student attrition. Rev. Educ. Res. 55, 485–540. doi: 10.3102/00346543055004485
Behr, A., Giese, M., Kamdjou, H. D. T., and Theune, K. (2020). Dropping out of university: a literature review. Rev. Educ. 8, 614–652. doi: 10.1002/rev3.3202
Behr, A., Giese, M., Kamdjou, H. D. T., and Theune, K. (2021). Motives for dropping out from higher education—an analysis of bachelor’s degree students in Germany. Eur. J. Educ. 56, 325–343. doi: 10.1111/ejed.12433
Brandstätter, V., and Bernecker, K. (2021). Persistence and disengagement in personal goal pursuit. Annu. Rev. Psychol. 73, 271–299. doi: 10.1146/annurev-psych-020821-110710
Brandstätter, V., Giesinger, L., Job, V., and Frank, E. (2015). The role of deliberative versus implemental mindsets in time prediction and task accomplishment. Soc. Psychol. 46, 104–115. doi: 10.1027/1864-9335/a000231
Brown, T. A. (2015). Confirmatory Factor Analysis for Applied Research, 2nd Edn. New York, NY: Guilford Press.
Cabrera, A. F., Nora, A., and Castaneda, M. B. (1993). College persistence: structural equations modeling test of an integrated model of student retention. J. High. Educ. 64, 123–139. doi: 10.2307/2960026
Cross, K. W., and Hall, D. L. A. (1954). Survey of entrants to nurse training schools and of student-nurse wastage in the Birmingham region. Brit. J. Prev. Soc. Med. 8, 70–76. doi: 10.1136/jech.8.2.70
Danaher, P. A., Bowser, D., and Somasundaram, J. (2008). The student departure puzzle: Do some faculties and programs have answers? High. Educ. Res. Dev. 27, 271–280. doi: 10.1080/07294360802183820
Danner, D., Hagemann, D., and Fiedler, K. (2015). Mediation analysis with structural equation models: combining theory, design, and statistics. Eur. J. Soc. Psychol. 45, 460–481. doi: 10.1002/ejsp.2106
Demetriou, C., and Schmitz-Sciborski, A. (2011). “Integration, motivation, strengths and optimism: retention theories past, present and future,” in Proceedings of the 7th National Symposium on Student Retention, (Charleston, SC), 300–312.
DesJardins, S. L., Kim, D.-O., and Rzonca, C. S. (2003). A nested analysis of factors affecting bachelor’s degree completion. J. Coll. Stud. Retent. 4, 407–435. doi: 10.2190/bgmr-3ch7-4k50-b5g3
Diseth, Å (2011). Self-efficacy, goal orientations and learning strategies as mediators between preceding and subsequent academic achievement. Learn. Individ. Differ. 21, 191–195. doi: 10.1016/j.lindif.2011.01.003
Dunlosky, J., Rawson, K. A., Marsh, E. J., Nathan, M. J., and Willingham, D. T. (2013). Improving students’ learning with effective learning techniques. Psychol. Sci. Public. Interest 14, 4–58. doi: 10.1177/1529100612453266
Eisinga, R., Grotenhuis, M., and Pelzer, B. (2013). The reliability of a two-item scale: pearson, Cronbach, or Spearman-Brown? Int. J. Public Health 58, 637—-642. doi: 10.1007/s00038-012-0416-3
Faas, C., Benson, M. J., Kaestle, C. E., and Salva, J. (2018). Socioeconomic success and mental health profiles of young adults who drop out of college. J. Youth Stud. 21, 669–686. doi: 10.1080/13676261.2017.1406598
Fishbein, M., and Ajzen, I. (1975). Belief, Attitude, Intention and Behavior: An Introduction to Theory and Research. Boston, MA: Addison-Wesley.
Fishman, J., Lushin, V., and Mandell, D. S. (2020). Predicting implementation: comparing validated measures of intention and assessing the role of motivation when designing behavioral interventions. Implement. Sci. Commun. 1:81. doi: 10.1186/s43058-020-00050-4
Gollwitzer, P. M. (2012). “Mindset theory of action phases,” in Handbook of Theories of Social Psychology, eds P. Van Lange, A. W. Kruglanski, and E. T. Higgins (London: Sage), 526–546. doi: 10.3389/fpsyg.2020.00922
Gollwitzer, P. M. (2018). The goal concept: a helpful tool for theory development and testing in motivation science. Motiv. Sci 4, 185–205. doi: 10.1037/mot0000115
Grau, J., and Minguillon, J. (2013). When procrastination leads to dropping out: analysing students at risk. eLC Res. Pap. Ser. 6, 63–74.
Hagger, M. S., and Luszczynska, A. (2014). Implementation intention and action planning interventions in health contexts: state of the research and proposals for the way forward. Appl. Psychol. Health Well Being 6, 1–47. doi: 10.1111/aphw.12017
Hardre, P. L., and Reeve, J. (2003). A motivational model of rural students’ intentions to persist in, versus drop out of, high school. J. Educ. Psychol. 95, 347–356. doi: 10.1037/0022-0663.95.2.347
Herrmann, K. J., Bager–Elsborg, A., and McCune, V. (2017). Investigating the relationships between approaches to learning, learner identities and academic achievement in higher education. High. Educ. 74, 385–400. doi: 10.1007/s10734-016-9999-6
Herrmann, M., and Brandstätter, V. (2015). Action crises and goal disengagement: longitudinal evidence on the predictive validity of a motivational phase in goal striving. Motiv. Sci. 1, 121–136. doi: 10.1037/mot0000016
Honicke, T., and Broadbent, J. (2016). The influence of academic self-efficacy on academic performance: a systematic review. Educ. Res. Rev. 17, 63–84. doi: 10.1016/j.edurev.2015.11.002
Hooper, D., Coughlan, J., and Mullen, M. R. (2008). Structural equation modelling: guidelines for determining model fit. Electron. J. Bus. Res. Methods 6, 53–60.
Hout, M. (2012). Social and economic returns to college education in the United States. Annu. Rev. Sociol. 38, 379–400. doi: 10.1146/annurev.soc.012809.102503
Hovdhaugen, E. (2009). Transfer and dropout: different forms of student departure in Norway. Stud. High. Educ. 34, 1–17. doi: 10.1080/03075070802457009
Hovdhaugen, E. (2011). Do structured study programmes lead to lower rates of dropout and student transfer from university? Irish Educ. Stud. 30, 237–251. doi: 10.1080/03323315.2011.569143
Hovdhaugen, E. (2015). Working while studying: the impact of term-time employment on dropout rates. J. Educ. Work 28, 631–651. doi: 10.1080/13639080.2013.869311
Hovdhaugen, E., and Aamodt, P. O. (2009). Learning environment: relevant or not to students’ decision to leave university? Qual. High. Educ. 15, 177–189. doi: 10.1080/13538320902995808
Hoyt, J. E., and Winn, B. A. (2004). Understanding retention and college student bodies: differences between drop-outs, stop-outs, opt-outs, and transfer-outs. NASPA J. 41, 395–417. doi: 10.2202/1949-6605.1351
Hu, L.-T., and Bentler, P. M. (1999). Cutoff criteria for fit indexes in covariance structure analysis: conventional criteria versus new alternatives. Struct. Equ. Model. Multidiscip. J. 6, 1–55. doi: 10.1080/10705519909540118
Iacobucci, D., Saldanha, N., and Deng, X. (2007). A meditation on mediation: evidence that structural equations models perform better than regressions. J. Consum. Psychol. 17, 139–153. doi: 10.1016/s1057-7408(07)70020-7
Ishitani, T., and Flood, L. (2018a). Reverse and horizontal transfer-out behavior at four-year institutions. Coll. Univ. 93, 16–28.
Ishitani, T., and Flood, L. (2018b). Student transfer-out behavior at four-year institutions. Res. High. Educ. 59, 825–846. doi: 10.1007/s11162-017-9489-4
Ishitani, T. T. (2008). How do transfers survive after “transfer shock”? a longitudinal study of transfer student departure at a four-year institution. Res. High. Educ. 49, 403–419. doi: 10.1007/s11162-008-9091-x
Jackson, B., Myers, N. D., Taylor, I. M., and Beauchamp, M. R. (2012). Relational efficacy beliefs in physical activity classes: a test of the tripartite model. J. Sport Exerc. Psychol. 34, 285–304. doi: 10.1123/jsep.34.3.285
Jones-White, D. R., Radcliffe, P. M., Huesman, R. L., and Kellogg, J. P. (2010). Redefining student success: applying different multinomial regression techniques for the study of student graduation across institutions of higher education. Res. High. Educ. 51, 154–174. doi: 10.1007/s11162-009-9149-4
Kehm, B. M., Larsen, M. R., and Sommersel, H. B. (2019). Student dropout from universities in Europe: a review of empirical literature. Hung. Educ. Res. J. 9, 147–164. doi: 10.1556/063.9.2019.1.18
Kim, K. R., and Seo, E. H. (2015). The relationship between procrastination and academic performance: a meta-analysis. Pers. Indivd. Differ. 82, 26–33. doi: 10.1016/j.paid.2015.02.038
Klassen, R. M., Krawchuk, L. L., and Rajani, S. (2008). Academic procrastination of undergraduates: low self-efficacy to self-regulate predicts higher levels of procrastination. Contemp. Educ. Psychol. 33, 915–931. doi: 10.1016/j.cedpsych.2007.07.001
Kline, R. B. (2015). Principles and Practice of Structural Equation Modeling, 4 Edn. New York, NY: Guilford Press.
Klingsieck, K. B. (2013). Procrastination: when good things don’t come to those who wait. Eur. Psychol. 18, 24–34. doi: 10.1027/1016-9040/a000138
Komarraju, M., and Nadler, D. (2013). Self-efficacy and academic achievement: Why do implicit beliefs, goals, and effort regulation matter? Learn. Individ. Differ. 25, 67–72. doi: 10.1016/j.lindif.2013.01.005
Korhonen, V., and Rautopuro, J. (2019). Identifying problematic study progression and “at-risk” students in higher education in Finland. Scand. J. Educ. Res. 63, 1056–1069. doi: 10.1080/00313831.2018.1476407
Leveson, L., McNeil, N., and Joiner, T. (2013). Persist or withdraw: the importance of external factors in students’ departure intentions. High. Educ. Res. Dev. 32, 932—-945. doi: 10.1080/07294360.2013.806442
Li, D. (2010). They need help: transfer Students from Four-Year to Four-Year Institutions. Rev. High. Educ. 33, 207–238. doi: 10.1353/rhe.0.0131
Malouff, J. M., and Schutte, N. S. (2019). The efficacy of interventions aimed at reducing procrastination: a meta−analysis of randomized controlled trials. J. Couns. Dev. 97, 117–127. doi: 10.1002/jcad.12243
Marsh, H. W., Pekrun, R., Parker, P. D., Murayama, K., Guo, J., Dicke, T., et al. (2019). The Murky distinction between self-concept and self-efficacy: beware of lurking jingle-jangle fallacies. J. Educ. Psychol. 111, 331–353. doi: 10.1037/edu0000281
Mashburn, A. J. (2000). A psychological process of college student dropout. J. Coll. Stud. Retent. 2, 173–190. doi: 10.2190/u2qb-52j9-ghgp-6lee
Mayhew, M. J., Rockenbach, A. N., Bowman, N. A., Seifert, T. A., Wolniak, G. C., Pascarella, E. T., et al. (2016). How College Affects Students: 21st Century Evidence that Higher Education Works. San Francisco, CA: Jossey-Bass.
Mccloskey, J., and Scielzo, A. S. (2015). Finally!: The Development and Validation of the Academic Procrastination Scale. Arlington, TX: University Of Texas. doi: 10.13140/RG.2.2.23164.64640
Melguizo, T. (2011). “A review of the theories developed to describe the process of college persistence and attainment,” in Higher Education: Handbook of Theory and Research, eds J. Smart and M. Paulsen (Berlin: Springer), 395–424. doi: 10.1007/978-94-007-0702-3_10
Meyer, J., Leuze, K., and Strauss, S. (2021). Individual achievement, person-major fit, or social expectations: Why do students switch majors in German higher education? Res. High. Educ. 63, 222–247. doi: 10.1007/s11162-021-09650-y
Morwitz, V. G., and Munz, K. P. (2020). Intentions. Consum. Psychol. Rev. 4, 26–41. doi: 10.1002/arcp.1061
Muthén, L. K., and Muthén, B. O. (2017). Mplus User’s Guide, 8th Edn. Los Angeles, CA: Muthén & Muthén.
OECD (2021a). Education at a Glance 2021: OECD Indicators. Paris: OECD Publishing, doi: 10.1787/b35a14e5-en
OECD (2021c). How much is Spent per Student on Educational Institutions?. Paris: OECD Publishing, doi: 10.1787/be9806af-en
Pascarella, E. T., Duby, P. B., and Iverson, B. K. (1983). A test and reconceptualization of a theoretical model of college withdrawal in a commuter institution setting. Sociol. Educ. 56, 88–100. doi: 10.2307/2112657
Pintrich, P. R. (1991). A Manual for the Use of the Motivated Strategies for Learning Questionnaire (MSLQ). Ann Arbor, MI: National Center for Research to Improve Post-Secondary Teaching.
Puente−Diaz, R., and Cavazos−Arroyo, J. (2018). An exploration of some antecedents and consequences of creative self−efficacy among College Students. J. Creat. Behav. 52, 256–266. doi: 10.1002/jocb.149
Quinn-Nilas, C., Kennett, D. J., and Maki, K. (2019). Examining explanatory style for failure of direct entry and transfer students using structural equation modelling. Educ. Psychol. 39, 749–767. doi: 10.1080/01443410.2019.1574340
Richardson, M., Abraham, C., and Bond, R. (2012). Psychological correlates of university students’ academic performance: a systematic review and meta-analysis. Psychol. Bull. 138, 353–387. doi: 10.1037/a0026838
Robbins, S. B., Lauver, K., Le, H., Davis, D., Langley, R., and Carlstrom, A. (2004). Do psychosocial and study skill factors predict college outcomes? A meta-analysis. Psychol. Bull. 130, 261–288. doi: 10.1037/0033-2909.130.2.261
Rozental, A., Forsell, E., Svensson, A., Forsström, D., Andersson, G., and Carlbring, P. (2015). Differentiating procrastinators from each other: a cluster analysis. Cogn. Behav. Therapy 44, 480–490. doi: 10.1080/16506073.2015.1059353
Saele, R. G., Dahl, T. I., Sørlie, T., and Friborg, O. (2017). Relationships between learning approach, procrastination and academic achievement amongst first-year university students. High. Educ. 74, 757–774. doi: 10.1007/s10734-016-0075-z
Schneider, M., and Preckel, F. (2017). Variables associated with achievement in higher education: a systematic review of meta-analyses. Psychol. Bull. 143, 565–600. doi: 10.1037/bul0000098
Sheeran, P. (2002). Intention—behavior relations: a conceptual and empirical review. Eur. Rev. Soc. Psychol. 12, 1–36. doi: 10.1080/14792772143000003
Sheeran, P., Maki, A., Montanaro, E., Avishai-Yitshak, A., Bryan, A., Klein, W. M. P., et al. (2016). The impact of changing attitudes, norms, and self-efficacy on health-related intentions and behavior: a meta-analysis. Health Psychol. 35, 1178–1188. doi: 10.1037/hea0000387
Sirois, F. M. (2016). “Procrastination, stress, and chronic health conditions: a temporal perspective,” in Procrastination, Health, and Well-Being, eds F. M. Sirois and T. Pychyl (Amsterdam: Elsevier), 67–92. doi: 10.1016/B978-0-12-802862-9.00004-9
Spencer, G. (2021). Off the beaten path: Can statewide articulation support students transferring in nonlinear directions? Am. Educ. Res. J. 58, 1070–1102. doi: 10.3102/0002831221999782
Steel, P. (2007). The nature of procrastination: a meta-analytic and theoretical review of quintessential self-regulatory failure. Psychol. Bull. 133, 65–94. doi: 10.1037/0033-2909.133.1.65
Steel, P., and König, C. J. (2006). Integrating theories of motivation. Acad. Manage. Rev. 31, 889–913. doi: 10.5465/amr.2006.22527462
Svartdal, F., Dahl, T. I., Gamst-Klaussen, T., Koppenborg, M., and Klingsieck, K. B. (2020). How study environments foster academic procrastination: overview and recommendations. Front. Psychol. 11:540910. doi: 10.3389/fpsyg.2020.540910
Tinto, V. (1975). Dropout from higher education: a theoretical synthesis of recent research. Rev. Educ. Res. 45, 89–125. doi: 10.3102/00346543045001089
Tinto, V. (1993). Leaving College: Rethinking the Causes and Cures of Student Attrition, 2nd Edn. Chicago, IL: The University of Chicago Press.
Tinto, V. (2017). Through the eyes of students. J. Coll. Stud. Retent. Res. Theory Pract. 19, 254–269. doi: 10.1177/1521025115621917
Van Dinther, M., Dochy, F., and Segers, M. (2011). Factors affecting students’ self-efficacy in higher education. Educ. Res. Rev. 6, 95–108. doi: 10.1016/j.edurev.2010.10.003
Van Eerde, W. (2003). A meta-analytically derived nomological network of procrastination. Pers. Indivd. Differ. 35, 1401–1418. doi: 10.1016/s0191-8869(02)00358-6
Van Eerde, W., and Klingsieck, K. B. (2018). Overcoming procrastination? A meta-analysis of intervention studies. Educ. Res. Rev. 25, 73–85. doi: 10.1016/j.edurev.2018.09.002
Visser, L., Korthagen, F. A. J., and Schoonenboom, J. (2018). Differences in learning characteristics between students with high, average, and low levels of academic procrastination: students’ views on factors influencing their learning. Front. Psychol. 9:808. doi: 10.3389/fpsyg.2018.00808
Visser, L., Schoonenboom, J., and Korthagen, F. A. J. (2017). A field experimental design of a strengths-based training to overcome academic procrastination: short- and long-term effect. Front. Psychol. 8:1949. doi: 10.3389/fpsyg.2017.01949
Wäschle, K., Allgaier, A., Lachner, A., Fink, S., and Nückles, M. (2014). Procrastination and self-efficacy: tracing vicious and virtuous circles in self-regulated learning. Learn. Instr. 29, 103–114. doi: 10.1016/j.learninstruc.2013.09.005
Webb, T. L., and Sheeran, P. (2006). Does changing behavioral intentions engender behavior change? A meta-analysis of the experimental evidence. Psychol. Bull. 132, 249–268. doi: 10.1037/0033-2909.132.2.249
Weissberg, N. C., and Owen, D. R. (2005). Do psychosocial and study skill factors predict college outcomes? Comment on Robbins et al. (2004). Psychol. Bull. 131, 407–409. doi: 10.1037/0033-2909.131.3.407
Weng, F., Cheong, F., and Cheong, C. (2015). Modelling is student retention in Taiwan: extending Tinto and Bean’s model with self-efficacy. Innov. Teach. Learn. Inf. Comput. Sci. 9, 1–12. doi: 10.11120/ital.2010.09020012
Willcoxson, L. (2010). Factors affecting intention to leave in the first, second and third year of university studies: a semester−by−semester investigation. High. Educ. Res. Dev. 29, 623–639. doi: 10.1080/07294360.2010.501071
Willcoxson, L., Cotter, J., and Joy, S. (2011). Beyond the first-year experience: the impact on attrition of student experiences throughout undergraduate degree studies in six diverse universities. Stud. High. Educ. 36, 331–352. doi: 10.1080/03075070903581533
Wolter, S. C., Diem, A., and Messer, D. (2014). Drop−outs from Swiss Universities: an empirical analysis of data on all students between 1975 and 2008. Eur. J. Educ. 49, 471–483. doi: 10.1111/ejed.12096
Wu, F., and Fan, W. (2017). Academic procrastination in linking motivation and achievement-related behaviours: a perspective of expectancy-value theory. Educ. Psychol. 37, 695–711. doi: 10.1080/01443410.2016.1202901
Yockey, R. D. (2016). Validation of the short form of the Academic Procrastination Scale. Psychol. Rep. 118, 171–179. doi: 10.1177/0033294115626825
Zajacova, A., and Lawrence, E. M. (2018). The relationship between education and health: reducing disparities through a contextual approach. Annu. Rev. Public Health 39, 273–289. doi: 10.1146/annurev-publhealth-031816-044628
Keywords: academic attrition, attrition intentions, drop-out, transfer-out, academic self-efficacy, procrastination, mediation
Citation: Nemtcan E, Sæle RG, Gamst-Klaussen T and Svartdal F (2022) Academic Self-Efficacy, Procrastination, and Attrition Intentions. Front. Educ. 7:768959. doi: 10.3389/feduc.2022.768959
Received: 29 September 2021; Accepted: 28 April 2022;
Published: 24 May 2022.
Edited by:
Marcos Cupani, Consejo Nacional de Investigaciones Científicas y Técnicas (CONICET), ArgentinaReviewed by:
Aude Villatte, University of Quebec in Outaouais, CanadaValeria Moran, Siglo 21 Business University, Argentina
Javier Sanchez Rosas, National University of Córdoba, Argentina
Copyright © 2022 Nemtcan, Sæle, Gamst-Klaussen and Svartdal. This is an open-access article distributed under the terms of the Creative Commons Attribution License (CC BY). The use, distribution or reproduction in other forums is permitted, provided the original author(s) and the copyright owner(s) are credited and that the original publication in this journal is cited, in accordance with accepted academic practice. No use, distribution or reproduction is permitted which does not comply with these terms.
*Correspondence: Efim Nemtcan, ZWZpbS5uZW10Y2FuQHVpdC5ubw==