- 1Section of Educational Sciences, and LEARN! Research Institute, Faculty of Behavioural and Movement Sciences, Vrije Universiteit Amsterdam, Amsterdam, Netherlands
- 2Hogeschool IPabo, Amsterdam, Netherlands
- 3Behavioural Science Institute, Radboud University Nijmegen, Nijmegen, Netherlands
The primary aim of this study was to identify how elementary school students’ individual differences are related to their learning outcomes and learning process in science and technology (S&T) education, using a mixed methods design. At the start of the study, we assessed the reading comprehension, math skills, science skills, executive functions, coherence of speech, science curiosity and attitude toward S&T of 73 fifth and sixth graders. The students then received a four-lesson inquiry- and design-based learning unit on the concept of sound. Learning outcomes were measured through a pre- and post-test regarding students’ conceptual knowledge of sound, a practical assessment of design skills and a situational interest measure. A factor score regression model (N = 62) showed significant influence from prior conceptual knowledge and the latent factor “academic abilities” (reading, math, and science skills) on post conceptual knowledge. The latent factor “affective” (curiosity and attitude toward S&T) and to a lesser extend also prior conceptual knowledge were predictive of situational interest. Learning process was measured through individual interviews and student worksheets within a subsample (N = 24). We used latent profile analysis to identify three profiles based on students’ individual differences, from which the subsample for qualitative analyses was selected. Codes and themes that emerged from the qualitative analyses revealed differences between students from the three profiles. The results of this study show how different types of students succeed or struggle within S&T education, which is essential for teachers in order to differentiate their instruction and guidance. Differentiation aimed at supporting language and the integration of science into design, while facilitating a variety of learning activities and assessments that move beyond written assignments, could help achieve the most optimal learning conditions for each student.
Introduction
Every student should learn science and technology (S&T) content and skills in primary education in order to participate in a technology-rich, knowledge-based society (Rocard et al., 2007). Providing effective S&T-education to every student is not unequivocal, however, since students’ individual differences (e.g., differences in academic abilities or motivation) are a complex source of individual variation in learning behavior (Van Schijndel et al., 2018). While previous studies have gained insights into the effect of these individual differences to some extent, their results also indicate that the exact roles of individual differences in S&T-education still remain unclear (Wagensveld et al., 2015; van Dijk et al., 2016; Schlatter et al., 2020). Moreover, these previous studies are mainly focused on science education as opposed to S&T education. In pursuance of suitable S&T-education for every student, the current study makes use of quantitative and qualitative analyses to understand how individual differences are related to both learning process and learning outcomes.
Before elaborating on the literature regarding relevant individual differences for S&T learning, it is important to consider the S&T-classroom context and corresponding terminology. Greater consistency and understanding of what defines S&T-education can facilitate future research synthesis and promote consensus on which approaches are most effective (Furtak et al., 2012; Martín-Páez et al., 2019). Comparable to other countries, S&T-education in the Dutch educational context (i.e., the context of the current study) typically entails inquiry- and design-based learning. These learning approaches are also a core component of STEM (science, technology, engineering, and mathematics) education (Sanders, 2009). According to Lewis (2006), “the complementarities we see between science and technology in society can be exploited in schools through the interplay of design and inquiry” (p. 274). Core processes of inquiry-based learning include experimenting and drawing conclusions (Pedaste et al., 2015), while core processes of design-based learning include defining problems by identifying criteria for possible solutions and optimizing a solution by systematically testing and refining (English et al., 2017). Nonetheless, these processes should not be seen as exclusively belonging to either inquiry- or design-based learning. Design converges with inquiry in two central ways: they both include reasoning processes, such as analogical reasoning used to solve a problem, and they both have uncertainty as a starting position (Purzer et al., 2015). Taken together, inquiry- and design-based learning combines both technological/engineering design and scientific inquiry in the context of technological problem-solving (Sanders, 2009).
Up to now, research on individual differences within S&T-education is typically focused on the specific core processes of (most often) inquiry- or (less often) design-based learning alone. For example, Koerber and Osterhaus (2019) have examined the influence of intelligence, language abilities, and advanced theory of mind on scientific thinking, and Schlatter et al. (2020) have looked into the effects of mathematical skillfulness and reading comprehension on scientific reasoning. The combination of inquiry and design could, however, make a difference in students’ learning experiences, as it poses several advantages for students: (1) “making things function” could help students frame their activity as more productive (when compared to constructing an answer to a science question), (2) it has the potential to make science education feel more approachable to students who are intimidated by more traditional forms of science, (3) design could offer a more tangible context and motivation to reason about physical processes, and (4) integrating scientific inquiry into design can help achieve higher science concept learning (Roth, 2001; Lewis, 2006; Mehalik et al., 2008; Wendell and Rogers, 2013). The current study will therefore relate multiple individual differences to both inquiry- and design-based learning processes and outcomes, in order to obtain a comprehensive picture of the role of individual differences in S&T education. When teachers know what differences to attend to, they can differentiate their instruction and guidance in order to optimize students’ individual potential (Tomlinson, 2000). For this purpose, combining inquiry with design provides a more accurate representation of the S&T-learning environment and the related individual differences that teachers can target. Since the literature on relevant individual differences is mostly specific to either inquiry- or design-based learning, below we will discuss these learning approaches separately (as far as they can be seen as separate approaches) when providing an overview of the most common studied individual differences in S&T education.
Individual Differences in Inquiry-Based Learning
Reading Comprehension and Linguistic Abilities
A higher level of reading comprehension has frequently been linked to greater performance in science education or inquiry-based learning (e.g., Osterhaus et al., 2017; Stender et al., 2018; Schlatter et al., 2020). In fact, reading comprehension seems to be one of the most prominent individual differences that affect science achievement. Lazonder et al. (2020) mapped the development of scientific reasoning among primary school students in a 3-year longitudinal study and found four distinct developmental patterns; while these patterns were mostly independent of students’ (other) cognitive and sociodemographic characteristics, they largely complied with the course of development in reading comprehension. Furthermore, Wagensveld et al. (2015) found reading comprehension and verbal reasoning skills to be the only predictors of learning the control of variables strategy (CVS), when also taking scientific knowledge and non-verbal reasoning into account. Similarly, based on their multidimensional model of scientific reasoning, Van de Sande et al. (2019) claim that linguistic abilities are essential in research on individual differences in scientific reasoning skills.
Lazonder et al. (2020) offer some conjectures as to why scientific reasoning might be of such a linguistic nature, such as that “scientific reasoning and reading comprehension share a set of problem-solving processes and sense-making strategies” (p. 13). However, these conjectures have not yet been verified and little is known regarding differences in the learning processes of students with high or low reading abilities. A study by van Dijk et al. (2016), using a computer-simulated task, found that “average- or low ability students” (indicated among others by reading comprehension) made little use of written prompts that were available in the task. These prompts were specifically intended as support that could reduce differences between students, and the authors could not pinpoint the rationale behind the finding that low-ability students abstained from the use of prompts. These results, together with a relatively new-found importance of linguistic abilities in science education, show that it is important to gain more insight into how students with different levels of reading abilities approach the inquiry learning process. Especially since measures for assessing science achievement usually rely heavily on reading comprehension (Mayer et al., 2014), solely looking at achievement differences gives little clue about how to optimize the learning process for low-ability students.
Math Skills
Closely related to S&T, as it is one of the other two letters in STEM education, is mathematics. Yet, few studies have looked into the influence of math skills as an individual difference on learning outcomes in primary science education (Byrnes et al., 2018). Recent findings have established a positive relationship between math skills or knowledge of mathematical concepts with scientific reasoning or science achievement (Byrnes et al., 2018; Koerber and Osterhaus, 2019; Schlatter et al., 2020). The longitudinal study by Lazonder et al. (2020) did not replicate these findings, which they speculate might be due to the limited set of skills that was included in their study. Both Byrnes et al. (2018) and Lazonder et al. (2020) highlight that the assessment for math should measure a broad set of skills such as number sense and spatial ability.
Executive Functions
Executive functions are a set of mental processes such as inhibitory control, working memory, and cognitive flexibility, which are needed for concentration, planning or self-control (Diamond, 2013). In the context of science education, inhibitory control is thought to be needed in the process of experimentation in order to inhibit the influence of existing beliefs on the interpretation of newly presented data (Kuhn and Pease, 2006; Mareschal, 2016). In addition, inhibitory control can suppress irrelevant thoughts and keep the mental workspace free from too much clutter, thus functioning as an aid for working memory (Diamond, 2013). Working memory, in turn, is crucial for inquiry-based learning, as its storage capacity and control efficiency is strongly related to reasoning ability (Chuderski and Jastrzebski, 2018).
Recent experimental studies have indeed shown positive relations between executive functions and science achievement. For example, van der Graaf et al. (2018) found that inhibition and verbal working memory predicted evidence evaluation and experimentation abilities, and indirectly scientific reasoning, among kindergartners. Osterhaus et al. (2017) found that inhibition, next to intelligence and language skills, predicted scientific thinking among 8- to 10-year-olds. In a study by Wilkinson et al. (2020), better inhibitory control was linked to greater performance on a counterintuitive reasoning task and science achievement scores among 7- to 10-year-olds. However, Wilkinson et al. (2020) place the important side note that possible confounding effects of a common causal factor, such as reading ability, could not be ruled out. It is therefore essential for further research to include a broad palette of individual differences, including executive functions.
Affective Factors
Students’ attitude toward S&T, a complex construct consisting of several components such as anxiety toward science and enjoyment of science (Osborne et al., 2003), is an important addition to the individual differences mentioned so far. Students’ attitude toward S&T has consistently been shown to decline as they grow older, which affects learning and later career choices (Potvin and Hasni, 2014a; Denessen et al., 2015; van Aalderen-Smeets and Walma van der Molen, 2018). A recent study modeled science interest as a dynamic relational network, in which different interest components, such as attitude, are shown to reinforce one another (Sachisthal et al., 2019). Central components within this network are most influential in positively affecting the network, and are thus interesting targets for interventions. Among 15-year-olds in the Netherlands, enjoyment seems to be a central component that influences other components such as behavior and self-efficacy (Sachisthal et al., 2019). Furthermore, it is quite possible that the decline in interest or motivation is not inevitable; it seems that the school environment is a more determining factor for whether students are willing to learn science rather than the home environment (Vedder-Weiss and Fortus, 2012). Therefore, gaining a better understanding of students’ enjoyment in S&T education and how school curricula can improve or reinforce this is a crucial starting point for further interventions.
With regard to attitude toward S&T, gender differences are important to consider as well. Especially at a later age, when choosing courses in secondary or tertiary education, girls tend to be less positive toward S&T than boys (Driessen and van Langen, 2013). In fact, the Netherlands is second-last of 159 countries with regard to the percentage of females in tertiary science programs (Hanson et al., 2017). This educational and career choice behavior seems to be crucially related to girls’ self-efficacy and stereotypical beliefs (van Aalderen-Smeets and Walma van der Molen, 2018) and might be a consequence of how science education is shaped (DeWitt and Archer, 2015). According to the review by Brotman and Moore (2008), girls have different approaches in science learning in comparison to boys, such as that girls are more cooperative and less competitive, seem to strive for deep conceptual understanding, and may particularly benefit from hands-on or inquiry-based learning. This benefit Brotman and Moore (2008) refer to, however, is with respect to achievement. Even though boys have outperformed girls in science for several years in the Netherlands, differences were small and the last two TIMSS (Trends in International Mathematics and Science Study, at the age of 10) results show that boys’ achievement has declined, resulting in a narrowing of the gap in achievement and a (non-significant) very slight advantage for girls (Martin et al., 2016; Mullis et al., 2020). Even when girls perform just as well as boys, their self-efficacy can be lower and effective methods to boost these self-efficacy beliefs are still unknown (van Aalderen-Smeets and Walma van der Molen, 2018). Gender is therefore an important confounding variable to consider when looking more closely at individual differences in S&T education.
Although sometimes grouped with attitude or interest, another affective construct that may have distinct importance is curiosity (Jirout and Klahr, 2012; Weible and Zimmerman, 2016). Specifically, scientific curiosity is a construct related to information seeking behavior and can be seen as a preference for uncertainty (Jirout and Klahr, 2012). Since uncertainty is a starting condition for both inquiry- and design-based learning (Purzer et al., 2015), curiosity is, on top of attitude, an interesting construct for S&T education. It has been positively linked to recognizing both effective and ineffective questions, and generating more questions about a science topic, even when controlling for verbal ability (Jirout and Klahr, 2012). Furthermore, a study by Van Schijndel et al. (2018) found curiosity to be positively related to learning outcomes (as an added effect on intelligence), and in a specific way related to learning process: more curious children experimented shorter, yet learned more than less curious children, possibly because of a more efficient reflection on experiments. Looking more closely at the learning process thus seems pivotal in understanding the differences between students.
Individual Differences in Design-Based Learning
The role of individual differences in elementary design-based learning is a rather unexplored field, leading to mixed results. For example, Lie et al. (2019) found achievement gaps on an engineering assessment in both elementary and middle school samples. On the elementary level, language capabilities were found to be predictive, while this was not the case for the middle school level where only ethnicity was found to be a predictor. Gender was also a factor in students’ attitudes toward engineering, with males having a more positive attitude. A study by Mehalik et al. (2008) in a middle school sample found differentiated performance effects when comparing scripted inquiry learning with the use of an authentic design task: all students’ scores on science concept learning were improved in favor of the design task, but improvement was largest among low-achieving African-American students in terms of science knowledge. A study on motivational factors within a design-based learning makerspace found students’ self-efficacy to be related to their situational interest (Vongkulluksn et al., 2018). In other words, students who had more positive evaluations of their learning progress were more likely to maintain an interest toward their design projects. Other studies also point to a possible role of executive functions within design-based learning. Unfamiliarity with engineering design and therefore a lack of prior knowledge and skills may lead to cognitive fixation in the design problem-solving process (Luo, 2015; McFadden and Roehrig, 2019). Students with more developed executive skills might overcome fixation more easily, as the ability to change perspectives and “think outside the box” is a core executive function (Diamond, 2013). Furthermore, Vaino et al. (2018), who examined 8th-grade students’ solutions for an ice cream making device, suggest that some of the unrealistic design ideas by students were caused by a working memory overload.
Other differences found between students in design-based learning relate to prior misconceptions (Marulcu and Barnett, 2013), how they apply disciplinary knowledge in solving an engineering problem (English and King, 2015), or their ability to iterate back and forth in testing multiple solutions (Kelley et al., 2015). These studies show that students differ on multiple aspects of the learning process within design-based learning. What lies at the heart of these differences is still unclear, however, since a comprehensive overview of students’ individual differences in design-based learning is missing.
Current Study
Taken together, research in inquiry- and design-based learning points to the need of further examining how different types of students struggle or succeed. The current study will therefore address the following research questions:
1. How are students’ individual differences in academic abilities, executive functioning and affective factors related to their learning outcomes in S&T education?
2. How are students’ individual differences in academic abilities, executive functioning and affective factors related to their learning process during S&T education?
Based on our literature review, we can hypothesize for our main effects that academic abilities (e.g., Lazonder et al., 2020; Schlatter et al., 2020), executive functioning (e.g., van der Graaf et al., 2018), and affective factors (e.g., Van Schijndel et al., 2018) have a positive influence on learning outcomes. Our research questions are still formulated exploratively, however, since our focus is to gain a deeper understanding of the relationship between students’ individual differences and their learning process and outcomes in S&T education.
To explore these relationships, a lesson unit of four lessons regarding the concept of sound was taught to six classes of students in the age of 9–12. The topic of sound was chosen as this is a physics phenomenon that students have received little education about (in the Netherlands), but do have everyday experience with. Furthermore, the results by Lautrey and Mazens (2004), who have studied the organization of children’s naïve knowledge on sound and heat, suggest that “naive knowledge is constrained by some foundational principles” (p. 420) and that the gradual process of conceptual change is similar between sound and heat. We therefore expect the influence of individual differences during this lesson unit (on top of prior knowledge) to be similar to other physics phenomena. For learning outcomes, we’ve included measures of content knowledge, skills and situational interest. Situational interest is seen as an important affective component related to the focus and attention someone has to the task at hand (Rotgans and Schmidt, 2011a). It is proposed to be evoked by certain features of environmental stimuli (Hidi, 1990), and can eventually lead to the psychological state of interest and the more long-lasting predisposition to reengage with particular content (Hidi and Renninger, 2006; Palmer et al., 2017). As an important goal of S&T education is to spark students’ interest for a lifelong learning in S&T (Rocard et al., 2007), situational interest is included as an outcome measure in the current study. For learning process, we’ve included interviews and worksheets that can more specifically illustrate the aspects of inquiry- and design-based learning that students perceive to be, for example, enjoyable or difficult.
Materials and Methods
Design
A mixed methods design was chosen for complementarity purposes (Alexander et al., 2008). By combining both quantitative and qualitative measures, we intend to provide a more enriched understanding of S&T education and how individual differences come into play.
The study design consists of four phases: (a) measurement of individual differences, (b) pre-test measure of content knowledge, (c) the lesson unit, from which worksheets and subsequent interviews in a subsample were used for qualitative analysis (i.e., learning process), and (d) a final quantitative phase after completion of the lesson unit, with post-test measures of content knowledge, design skills and situational interest (i.e., learning outcomes). The design of this study is displayed in Figure 1.
Participants
The sample consisted of 73 students (37 girls), with a mean age of 10.7 (SD = 0.70). Fifty-six students were from grade 5, and 17 from grade 6. In the Netherlands, students from the fifth and sixth grade are comparable in experience in S&T-learning in terms of procedural and conceptual knowledge. Grade 5 and 6 are also often combined in one classroom, as was the case in our sample; therefore, both grades were included in the sample. Students were recruited from two suburban primary schools in the Netherlands. Both schools claimed to provide a similar amount and type of S&T-education, in the form of projects/series of lessons a few times a year. Qualitative analyses were conducted on an a priori selected subsample of 24 students. Stratified sampling was used as sampling procedure. This procedure is further described under the analyses section.
Procedure
This research was approved by the ethics committee of the Vrije Universiteit Amsterdam (VCWE-2019-114R1). Parents and/or caregivers were contacted through the teachers for active informed consent. In agreement with schools, the lesson unit was taught to all students in the participating classes in order to maintain a realistic classroom setting (each class consisted of approximately 30 students, which is typical for Dutch elementary classrooms). Personal data was only collected from students who had parental consent. Prior to the lesson unit, individual differences were tested through parent and student questionnaires.
The parent questionnaire was presented after completing the consent form, and consisted of the inhibition and working memory items from the Behavior Rating Inventory of Executive Function (BRIEF; Huizinga and Smidts, 2012) and items concerning coherence of speech from The Children’s Communication Checklist (CCC-2-NL; Geurts, 2007). Gender and age of the student were also attained through the parent questionnaire.
The student questionnaire was administered simultaneously to all participating students of one class, in exam-like setting, and took on average 30 min to complete. The student questionnaire consisted of the Science Curiosity In Learning Environments (SCILE; Weible and Zimmerman, 2016) and an adapted attitude questionnaire (Denessen et al., 2015). The student questionnaire also contained the pretest measures of content knowledge regarding the concept of sound as well as a science skills test (Kruit et al., 2018).
The lesson unit started 1 week later and consisted of four weekly lessons of approximately 1 h. The first author, who is also a certified teacher, taught all classes, while the regular teacher was present in the classroom. The regular teachers were instructed to not provide any assistance, in order to maintain equal conditions for all classes as much as possible. The lesson unit was largely based on an existing lesson unit that was developed for the European project ENGINEER in collaboration with a Dutch Science Museum (ENGINEER, 2014; Nemo, n.d.). The ENGINEER project was a consortium of 26 partners from 12 countries, aiming to propose an innovative inquiry-based learning methodology inspired by the problem-solving approach of engineering, similar to the widely used and reviewed U.S. program “Engineering is Elementary” (Anyfandi et al., 2016). Furthermore, since attention in the lesson unit was placed on “promoting the constructive aspects of learning, facilitating collaboration and providing opportunities for meaningful activities,” following general principles for constructing an effective science learning environment (Vosniadou et al., 2001, p. 397), the lesson unit was deemed an appropriate representation of a typical S&T learning environment that holds both national as international standards.
The objective for the students was to design a musical string instrument that could produce different sounds. Throughout the four lessons, learning objectives for students included “sound is a vibration” or “sound needs a medium to travel through.” In the first lesson of the lesson unit, the problem of designing a string instrument was introduced, with activation of prior knowledge on musical (string) instruments. Differences and similarities between instruments were discussed, both in visual and auditory terms. Students were probed to write down questions that could help them solve the problem, such as “How can I create a loud instrument?” and to hypothesize about the answers to these questions. The second lesson consisted first of a plenary discussion on “what is sound,” with the help of some visual demonstrations, such as making sugar placed on top of a cup move by producing sound and using a slinky to model a sound wave. The largest part of the second lesson consisted of students undertaking small experiments in pairs regarding sound, for example by investigating the difference between the sound of a tight and a less tight rubber band. After students had handed in their worksheet, conclusions of the experiments were discussed in class. In the third lesson, students individually designed and created their own musical string instrument. At the start of the lesson, design demands were discussed in class and agreed upon in correspondence with the students, in order to keep involvement high. Some direction was given by the teacher in order to keep design demands similar between classes. Then, the material with which the instrument could be designed was shown, but not provided until students had completed their design drawing. The fourth and final lesson consisted of presenting the instruments to each other. Students were prompted to evaluate the instrument of their peers regarding the design demands and to discuss the improvements made to the instrument. Each lesson contained at least one worksheet to guide students in the process; these worksheets are described in more detail under the instruments section. Guidance was intentionally provided through worksheets as opposed to by the teacher, in order to keep the influence from teacher interference as small as possible and obtain a clear view of the influence of individual differences.
The post-test, also in the form of a student questionnaire, took place 1 week after the final lesson. It contained another version of the content knowledge test regarding the concept of sound, another version of the science skills test and a situational interest questionnaire (Rotgans and Schmidt, 2011b). The pre- and post-test versions were counterbalanced.
Instruments
Individual Differences
Reading Comprehension and Math Skills
For math skills and reading comprehension, students’ standardized assessment scores were requested from the teachers with permission from parents. These standardized assessment scores are developed by the Dutch National Institute for Educational Testing and Assessment which monitors students’ achievement through semi-annual assessments.1 The scores for math and reading comprehension are used by teachers to advise students for further education and are considered a valid indication of general cognitive ability (Kruit et al., 2018). The scores for the latest assessment were used, which were administered a few months prior to the lesson unit.
Coherence of Speech
As an additional language measure, the subscale Coherence from The Children’s Communication Checklist (CCC-2-NL) was administered. The CCC-2-NL can be used to give a quantitative estimate of pragmatic language impairments in children (Geurts, 2007). The subscale Coherence consists of seven items (Cronbach’s α = 0.75 in the current sample), such as “Can give an understandable report of something that happened, for example what he/she did at school.” Parents had to indicate for each item how often this behavior occurred, on a 4-point scale ranging from “Less than once a week (or never)” to “Several times (more than twice) a day (or always).”
Executive Functions
Students’ inhibition and working memory were measured through the Dutch version of the parent-reported BRIEF (Huizinga and Smidts, 2012). This inventory examines executive function in children and adolescents (ages 5–18 years; Gioia et al., 2000). For items such as “When given three things to do, remembers only the first or last” (working memory) and “Interrupts others” (inhibition), parents had to indicate on a three-point scale (Never, Sometimes, Often) how often their child exhibited this behavior over the last 3 months. The working memory (Cronbach’s α = 0.87 in the current sample) and inhibition scale (Cronbach’s α = 0.80 in the current sample) both consisted of 10 items each.
Attitude
In this study, the construct attitude is defined as enjoyment and self-efficacy toward S&T. Based on the literature review above, these two subcomponents were deemed appropriate as core elements of attitude. The questionnaire developed for measuring attitude in the current study consisted of 10 multiple choice items (included as Supplementary Material) on which students had to indicate their agreement on a scale of 1–4, ranging from “totally disagree” to “totally agree.” Five items pertained to self-efficacy, such as “I think I’m better at solving puzzles than most children” (Cronbach’s α = 0.69), and five items pertained to enjoyment, such as “I like learning about science and technology” (Cronbach’s α = 0.84). The self-efficacy items were self-developed, while the enjoyment items were derived from a previous study by Denessen et al. (2015). Somewhat similar to their study, our attitude questionnaire consisted of examples and pictograms of important concepts and skills related to S&T, such as analyzing, experimenting, and designing, in order to ensure that students’ shared a basic understanding of what S&T entails.
Science Curiosity
Students’ science curiosity was measured with a translated version of the SCILE questionnaire developed by Weible and Zimmerman (2016). This questionnaire consists of 12 self-report items, such as “I like to work on problems or puzzles that have more than one answer.” Students reported on a 5-point scale ranging from “never” to “always.” The level of internal consistency was high (Cronbach’s α = 0.91) in the sample of Weible and Zimmerman (2016) and good in the current sample (Cronbach’s α = 0.77).
Science Skills
Initially, the intention was to include a pre- and post-test measure of students’ science related skills. An existing measure developed and validated by Kruit et al. (2018) was adjusted for the purposes of the current study. Their paper-and-pencil science skills test contained items that measure “thinking” (e.g., reasoning) and “science-specific” (e.g., formulating a research question) skills. The original version included 18 multiple-choice items and 5 open-ended items and administration took about 45 min. Since the lesson unit in the current study was not specifically focused on teaching “science-specific” skills and administration time was limited, we only selected the multiple-choice “thinking” items of the science skills test. Ultimately, based on the data on the pre- and post-test, we decided it was not sensible to include the science skills test as a learning gain measure. The skills measured are more general for science than for example the skills and knowledge needed to design a musical string instrument. Therefore, we included the pre-test measure of science skills as an individual difference.
Learning Outcomes (Quantitative)
Content Knowledge Gain
The lesson unit focused on learning content knowledge regarding the concept of sound. To our knowledge, there was no existing appropriate form of assessment to measure primary school students’ understanding of this concept. Based on the learning goals of the lesson unit and existing assessments for older children, 10 multiple choice items were developed for the purpose of this study (included as Supplementary Material). Two teacher educators, specialized in S&T content, reviewed the items and provided feedback, after which the items were revised and again reviewed. The items intended to measure knowledge that was covered during the lesson unit, for example the influence of vibration on key pitch or the influence of string material on vibration.
In order to prevent a testing effect, two slightly different versions of the content knowledge test were created. These differences were kept as minimal as possible. For example, an item of version 1 of the test was “If you tighten the strings on a guitar, the sound will be…,” with answer options being (a) louder, (b) higher, (c) softer, (d) lower. On the post-test, the comparable item was “If you loosen the strings on a guitar, the sound will be…,” with the same answer options as the version 1 item. The two versions were counterbalanced across the pre- and post-test.
Unfortunately, the reliability of this measure was below an acceptable level (Cronbach’s α = 0.26 for both version 1 and 2). Based on item correlations and “Cronbach’s alpha if item deleted,” several items were excluded. For both versions, this came down to the same five items. Cronbach’s alpha was improved to 0.53 for version 1 and to 0.49 for version 2. This still points to a low reliability, which is why we conducted a latent class analysis to further inspect the possible heterogeneity in the data. The specifications of this analysis are described in the Supplementary Material. The outcomes of the latent class analysis led us to conclude that the five items that were retained after the reliability analysis are more sufficient in distinguishing between knowledgeable and less knowledgeable students than the initial 10 items were. In the Supplementary Material regarding the latent class analysis, excluded items are denoted with an asterisk.
Design Skills
To our knowledge, no standardized assessment was available for measuring students design skills. The skills involved in design are multifaceted and therefore difficult to grasp in one unidimensional assessment. Based on Crismond and Adams’ Informed Design Teaching and Learning Matrix (2012) and the study by Kelley and Sung (2017), we selected a few skills that we believe are central to design-based learning and that we also deemed feasible to measure through the design sketches and final products of the students. Although rarely used as assessment, Kelley and Sung (2017) propose sketching to be an appropriate form of assessing students design abilities, especially when compared to the final design solution skills.
Initially, we selected seven different skills that were measurable through the design sketch or product. After a first round of coding the worksheets (on which students had sketched their design), we felt that some of these skills, such as “originality” or “use of annotations,” were not done justice when quantifying them into a unidimensional score. The final selection of design skills was therefore: (1) writing down the demands of the design (sketch), (2) meeting the demands of the design (sketch + product), and (3) agreement between sketch and product (sketch + product). It should be strongly noted that this measure of design skills consists of components of design skills and is not an exhaustive measure of design skills.
The coding of these design skills was commenced by the first author, and shared, checked and revised in collaboration with the other authors. This led to a coding protocol, which was adhered to by two student researchers who coded all design sketches and final design products. The inter-coder reliability was assessed using Krippendorff’s alpha, which pointed to a high level of agreement (α = 0.92; Krippendorff, 2004). Inconsistencies in coding were discussed until agreement was reached. This resulted in a final sum score for all students regarding design skills. An example design sketch and product are shown in Figure 2.
Situational Interest
Six situational interest items were developed, based on Rotgans and Schmidt (2011b). Items were: (1) I want to learn more about the topic of sound; (2) I think the topic of sound is interesting; (3) I was bored during the S&T-lessons (recoded), (4) I would like to continue working on the topic of sound; (5) I expect to master the topic of sound well; (6) I was fully focused during the S&T-lessons; I was not distracted by other things. The items were scored on a 5-point Likert scale ranging from “not true at all” to “very true for me” (Cronbach’s α = 0.78 in the current sample).
Learning Process (Qualitative)
Worksheets
Worksheets were essential in providing structure and support for the students during their learning process, and provided information for quantitative and qualitative analysis. Worksheet 2a and 2b were used as source of data triangulation for the interviews. For example, if a student mentioned something in the interview that was unclear, the worksheets could be consulted for further information. Worksheet 2a and 2b also provided instructions for experimenting during lesson 2 and contained open or multiple-choice questions regarding the conclusion of the experiments. On worksheet 3, during lesson 3, students had to draw their design sketch and write down the corresponding design demands that were agreed upon in the class discussion. They were also prompted to list the materials they needed and to incorporate how they planned to attach the strings. Worksheet 1 and 4 were supportive to the lesson unit, and not used in analyses. For lesson 2, there was an extra worksheet in case students were finished; the assignment was to play a well-known Dutch song with a ruler.
Interviews
The semi-structured interviews were held after lesson 2 and 3 in order to obtain more descriptive insights into students’ learning process during experimenting (lesson 2) and designing (lesson 3). The interview protocol (included as Supplementary Material) included three main questions regarding (1) what they did during the lesson, (2) what they learned from the lesson, and (3) what they liked/did not like and what they perceived as easy/difficult. Probes for each question were specified in the protocol, to prompt the children to explicate their thoughts and feelings. All interviews were audio-recorded and transcribed verbatim.
Analyses
Quantitative Analyses (Research Question 1)
The aim of the main quantitative analyses was to identify the effect of several individual differences on learning outcomes (i.e., content knowledge gain, design skills, and situational interest). These individual differences are hypothesized constructs (i.e., latent variables), which is why structural equation modeling (SEM) was deemed appropriate. However, SEM is not suitable for a small sample size (Kline, 2011). A proposed alternative is factor score regression (FSR), with a correction for biased parameter estimates using the method of Croon (Devlieger et al., 2019). We used FSR to calculate the models, with the lavaan-package in R (Rosseel, 2012). The full maximum likelihood procedure was used to account for missing data. Students that had missing data on the pre-test scores due to absence were excluded from the analysis (n = 11).
Prior to the main FSR analysis, a principal component analysis (PCA) was conducted to verify the structure of the latent variables and corresponding indicators. Specifics of this PCA are included as Supplementary Material.
The initial FSR model included reading comprehension, math skills, science skills, inhibition, working memory, coherence of speech, enjoyment and self-efficacy toward S&T, science curiosity, and pre-content knowledge as predictors (i.e., exogenous variables). Their influence was assessed on the outcome variables (i.e., endogenous) post-content knowledge, design skills and situational interest. For model comparison, non-significant predictors were removed from the initial model. The general characteristics age and gender were added subsequently to the best fitting model, to see if model fit improved.
Model fit was assessed using common guidelines for global fit indices: the chi-square statistic, the comparative fit index (CFI), the Root Mean Square Error of Approximation (RMSEA), and Standardized Root Mean Square Residual (SRMR) (Schreiber et al., 2006). Model fit is deemed adequate when CFI is ≥ 0.90, though ideally ≥ 0.95, RMSEA < 0.06–0.08, and SRMR ≤ 0.08 (Sheu et al., 2018). For model comparison, the Akaike information criterion (AIC) and the Bayes information criterion (BIC) were used to assess the most optimal model, where smaller values indicate more adequate fit. However, these indices are not a “gold standard” and the ultimate decision on which model fits the data best should have a solid basis in theory as well (Kline, 2011). Furthermore, because FSR uses a two-step estimation for the parameters, the fit indices produced by FSR are not identical to the standard global fit indices and should be seen as “pseudo” fit indices (Y. Rosseel, personal communication, July 2020).
Qualitative Analyses (Research Question 2)
Sampling Procedure (Latent Profile Analysis)
For the purpose of qualitative analyses, a subsample of students was selected. In order to identify a meaningful subsample of students who might represent a larger group within S&T-education, a stratified sampling procedure was applied (Robinson, 2014). The rationale for this stratification was based on latent profile analysis (LPA). This analysis can be used to “trace back the heterogeneity in a group to a number of underlying homogenous subgroups” (subgroups henceforth called classes; Hickendorff et al., 2018, p. 4). Averaged reading comprehension and math scores, inhibition, working memory, average attitude (enjoyment and self-efficacy), science curiosity, and coherence of speech were included as predictor variables in the LPA. For a clearer interpretation, scores on inhibition, working memory and coherence of speech were recorded in order for high scores to indicate good functioning on all variables. To determine the appropriate number of latent profiles, the steps described in Hickendorff et al. (2018) were followed. Starting with a one-class model, where all students are in the same class, one additional class was added iteratively to see which model (i.e., number of classes) was deemed most appropriate. Models with more than three classes no longer resulted in a stable solution. The decision for the final three-profile model was based on a combination of statistical fit measures, parsimony and theoretically meaningful interpretations (Hickendorff et al., 2018). Fit indices for the three models are depicted in Table 1. A cautionary statement should be made regarding the LPA results: the small sample size brings about some risks regarding the power of the analysis and stability of the profiles. Since the LPA is not intended to provide a final answer regarding the number of profiles that exist in the population, but to guide our selection process of students for qualitative analysis, we do believe results to be useful and informative. Additionally, the overall posterior classification probabilities of the three profiles gave some assurance that differences between profiles were distinct. The results of the LPA should, however, be interpreted with caution.
The first profile consisted of 61% of students and was characterized by averages on almost all measures, except for attitude and curiosity which were lowest. We labeled this profile as the “average majority.” The second profile consisted of 21% of the students, and was characterized by very high scores on inhibition, working memory, and coherence of speech, indicating good executive functioning and no problems with speaking coherently. Furthermore, this profile was defined by high scores on combined math and reading comprehension and on attitude and curiosity. Thus, we labeled this profile as “successful science enthusiasts.” The third and final profile consisted of 18% of the students and was characterized by low scores for inhibition, working memory, and coherence of speech, indicating problems with executive functioning and speaking coherently. Furthermore, this profile was characterized by a low score on combined math skills and reading comprehension, indicating problems with academic abilities, and average, but high, scores for attitude and curiosity that indicated a positive attitude toward S&T. We labeled this profile as the “struggling science enthusiasts,” as this profile seemed to be mirrored to the “successful scientist enthusiasts” in regard to academic and executive abilities, while being similar in a positive and curious attitude. The LPA estimates per predictor variable and class are depicted in Figure 3.
Subsequently, students from each profile were selected for qualitative analysis by taking several considerations into account: (1) a maximum of four students per classroom could be selected because of available time for interviewing, (2) the matching of student’s scores on individual difference measures with the profile characterization, (3) permission from parents to be interviewed for qualitative analysis, and (4) obtaining an approximate equal distribution of boys and girls. This resulted in a subsample of 24 students: 11 students belonging to the profile “average majority,” 8 students belonging to the profile “successful science enthusiasts” and 5 students belonging to the profile “struggling science enthusiasts.”
Coding of Interviews
The interviews held after lesson 2 and 3 were open coded by the first author, using the software package “Atlas.ti.” Following the constant comparative method (Glaser and Strauss, 1967), the interview transcripts were first reviewed in search of themes and concepts, with descriptive codes emerging inductively as themes were identified (Campbell et al., 2013). After the first cycle of coding, all transcripts were revisited in order to reorganize and categorize the codes in development of a coherent coding scheme (Saldana, 2009). This iterative process was in close consultation with the other authors, who had no personal contact with the participants; this reflexivity (considering the bias as a qualitative researcher) is an important aspect of qualitative research that helps understand how the analytic process is influenced by personal relationships (O’Brien et al., 2014).
The coding for lesson 2 resulted in four overarching codes or themes, of which some could be specified in two or three subgroups. These codes were (1) specific actions; (2a) difficulty/easy; (2b) difficulty/hard; (3a) knowledge/correct; (3b) knowledge/incorrect; (3c) knowledge/unclear; (4a) opinion/negative; and (4b) opinion/positive. For lesson 3, the coding resulted in seven overarching codes or themes, of which some could be specified in two or three subgroups. These codes were (1a) design demands/as discussed; (1b) design demands/partly as discussed, (1c) design demands/other; (2) specific materials, (3a) difficulty/easy/crafting, (3b) difficulty/easy/ideas, (3c) difficulty/easy/drawing, (3d) difficulty/easy/other, (4a) difficulty/hard/crafting, (4b) difficulty/hard/ideas, (4c) difficulty/hard/other, (5a) knowledge/correct; (5b) knowledge unclear, (6a) opinion/positive, (6b) opinion/negative, (7a) suggestions for improvement; (7b) no suggestions for improvement. A more detailed description of the codes for lesson 2 and 3, with example fragments, are depicted in Tables 2, 3.
Using this final coding scheme, all transcripts were then coded by a second coder. Quotations were pre-defined, although the second coder was instructed to read all assertions and code extra quotations if deemed necessary. For lesson 2, overall agreement was high (Krippendorff’s alpha = 0.86). However, one code category (“specific actions”) did not reach sufficient agreement (0.44). In accordance with the second coder, this code category was then redefined more specifically. For lesson 3, overall agreement was also high (Krippendorff’s alpha = 0.89). Disagreements on any of the codes were discussed until agreement was reached. In the final step of analysis, the frequencies of the codes were compared between latent profiles to answer the question how students’ individual differences are related to their learning process in S&T-education.
Results
The descriptive statistics for the main variables, and per latent profile, are depicted in Table 4.
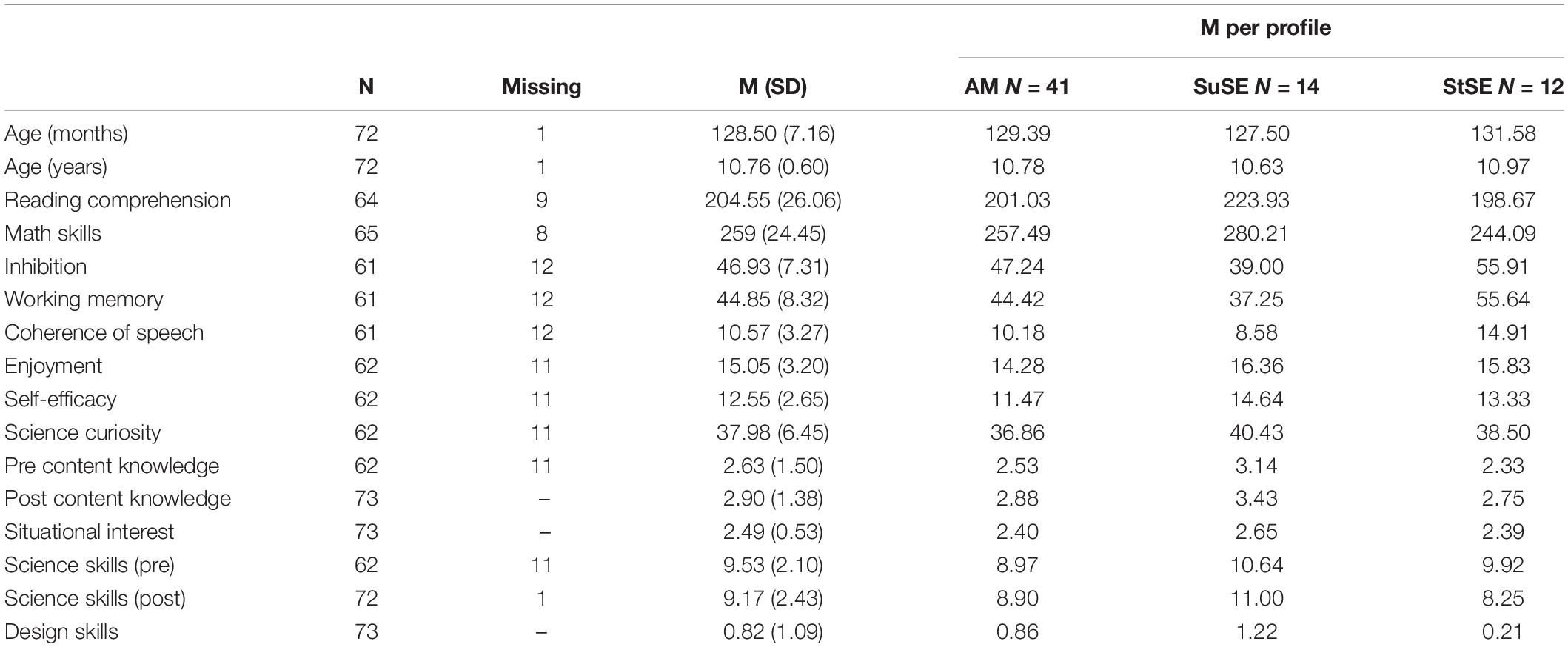
Table 4. Descriptive statistics for the main variables and per latent profile: average majority (AM), successful science enthusiasts (SuSE), and struggling science enthusiasts (StSE).
Learning Outcomes
The fit indices for the initial FSR model, the subsequent comparison models and the final model are shown in Table 5. Design skills was removed from the initial model, based on low and non-significant regression coefficients and a more parsimonious model when removed. The latent variable “executive functioning” was removed from the structural model, to simplify model structure and improve model fit. The final model is displayed in Figure 4 and model parameter estimates in Table 6. The pseudo fit indices for the final model: X2(47) = 63.07, p = 0.06, CFI = 0.902, SRMR = 0.106, RMSEA = 0.074. This model was deemed most appropriate as final model considering the theoretical substantiation and since cut-off criteria were met for the majority of the fit indices. Gender and age were not included in the final model, as the addition of these variables did not improve model fit.
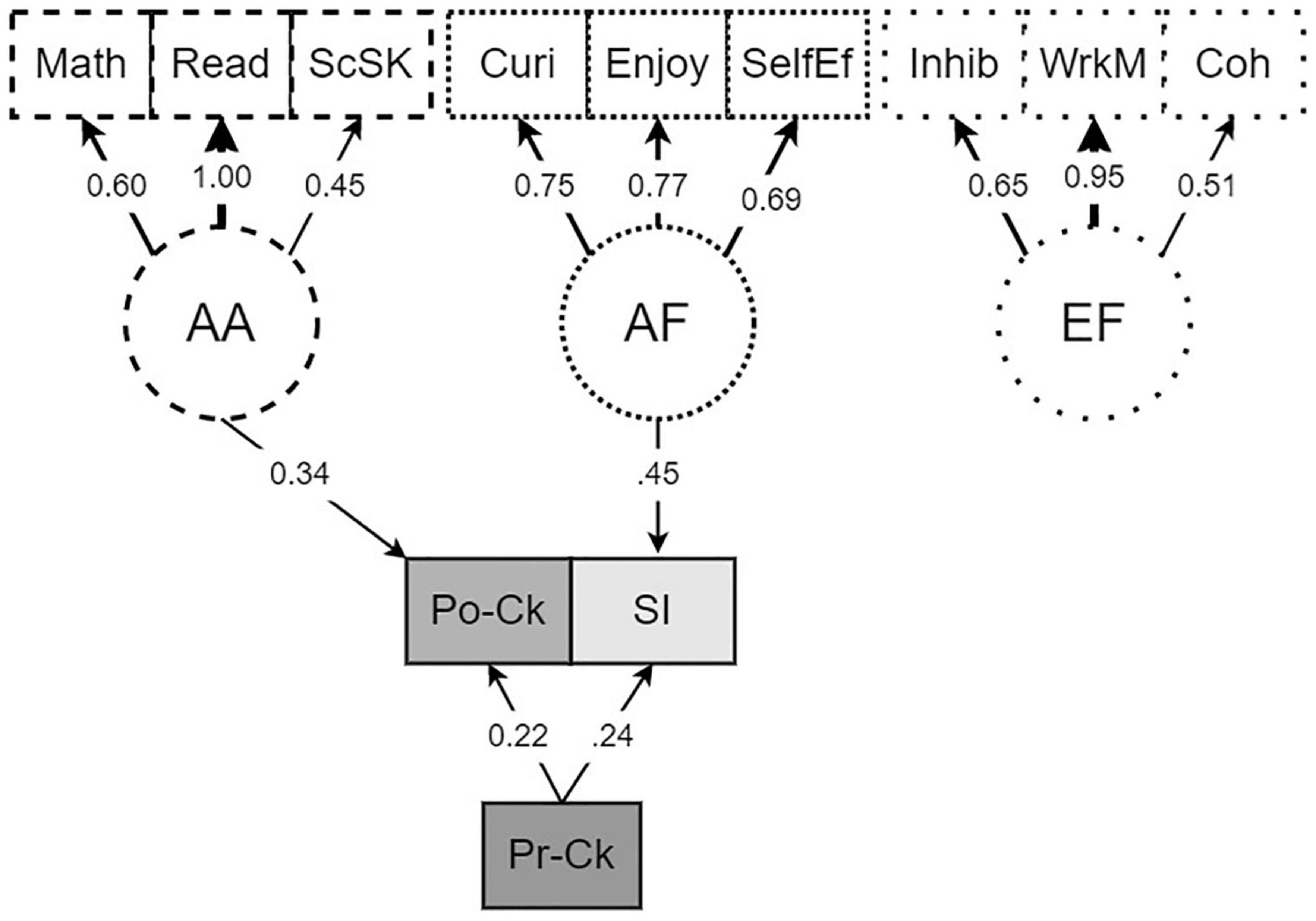
Figure 4. Final factor score regression model. The final model was specified with direct paths on the outcome variables post content knowledge (Po-Ck) and situational interest (SI). Predictors are pre content knowledge (Pr-Ck) and latent variables (circles): academic abilities (AA), indicated by science skills (ScSk), reading (Read), and math; affective (AF), indicated by self-efficacy (SelfEf), enjoyment (Enjy), and curiosity (Curi); executive functioning (EF), indicated by coherence of speech (Coh), working memory (WrkM), and inhibition (Inhib). Arrows that are missing between latent and outcome variables indicate non-significant paths.
The measurement part of the final model consisted of three latent variables: “academic abilities,” “affective,” and “executive functioning.” Each of these three latent variables was defined by three observed indicators. Positive predictors for the post-content knowledge score were the latent variable “academic abilities,” as indicated by reading comprehension, math skills and science skills (β = 0.33, p = 0.02), and the pre-content knowledge score (β = 0.33, p = 0.005). Positive predictors for situational interest were the latent variable “affective,” as indicated by enjoyment, self-efficacy, and science curiosity (β = 0.33, p = 0.003), and the pre-content knowledge score (β = 0.33, p = 0.045), although the latter was marginally significant.
Learning Process
For the second research question, regarding learning process, qualitative analyses were used to gain more in-depth insight into the role of individual differences. Coding was done separately for lesson 2 (experimenting) and lesson 3 (designing). The learning process results will be discussed based on differences between the latent profiles (which are described under the method section).
Learning Process: Experimenting
The frequencies of codes per student, per profile, for lesson 2 (experimenting), are depicted in Table 7. Since the latent profiles were unequal in sample size, all frequencies of codes are divided by the number of students in the profile. Most strikingly are differences for the codes (3a) “knowledge” and (1) “specific actions,” which we will discuss separately. The code groups (2) difficulty and (4) opinion are discussed more briefly.

Table 7. Code frequencies of the code groups per profile, divided by the profile sample size, for lesson 2 “experimenting”.
Knowledge
A remarkable outcome is that the “struggling science enthusiasts,” who were characterized by low scores on academic abilities (math skills and reading comprehension) but high on attitude and curiosity, have the most frequencies for the code (3a) “knowledge correct.” This outcome is remarkable as quantitative analyses showed academic abilities to be a predictor for content knowledge gain. The interviews show that these students are able to reproduce the knowledge that was covered in the lesson unit. On the other hand, the students in this profile also had the most code frequencies for (3c) “knowledge unclear.” This latter code was ascribed when it was unclear what students meant. For example, when students used too many different terms or contradictory terms in one assertion: “If you put the ruler […] if you make it shorter than you will hear it a bit softer and louder,” or because the exact terms used were unclear in combination with wat the student described: “Pitch ehm. Yeah, if it’s, I don’t know, if it’s smaller then it’s higher than if it’s lower and larger.” In this last assertion, it’s not clear what the student meant by “smaller”; it could refer to the wavelength of the sound, which would indeed be smaller at a higher frequency, but it could also refer to the amplitude of the sound wave, which would be related to volume instead of pitch. Thus, it’s unclear whether this knowledge is correct or incorrect. Since these students also have the highest number of the code “knowledge unclear,” next to the highest number of “knowledge correct,” it remains ambiguous to what extent these students have developed their knowledge on the concept of sound.
Three students (two belonging to the profile “struggling science enthusiasts” and one to “average majority”) seemed to mistakenly pair a high pitch with louder volume and a low pitch with a softer volume. For example, one student mentioned with regard to volume, “the louder, the higher,” but afterward repeated his answer again and said “The louder you hit the table, the louder the sound. And the more soft, the softer the sound,” where he did not repeat anything about high or low. When observing the worksheets of these students, it was noticed that a certain question triggered this combination of “soft–low” and “loud–high.” The question was: “Pluck the ruler hard first and then soft. Do you hear a difference? Circle the correct underlined word (1 word per sentence): If I pluck the ruler hard, the sound gets higher/lower/louder/softer. If I pluck the ruler soft, the sound gets higher/lower/louder/softer.” Two of these three students underlined two words per sentence instead of one and repeated these exact combinations in the interviews when asked what they had learned with regard to volume or sound pitch. It seems that the way the question was formulated caused confusion for these students.
Specific Actions
The code (1) specific actions was ascribed when students systematically described the experiments. For example, an assertion that was coded as specific action was: “And two different rubber bands, a thick and a thin one and then find out what the difference in sound is if you put it, if you for example make a thick rubber band loose or really tight. And when you do the thin rubber band really loose or really tight.” The student describes a comparison between two different types of rubber bands and how she experimented with these rubber bands to produce different sounds. Again, the “struggling science enthusiasts” were ascribed the most frequencies of code (1) specific actions, while one might expect the “successful science enthusiasts,” who were characterized by students having higher scores on coherent speech and academic abilities, to be more systematic in their descriptions. The interviews show that students that generally had lower school- or speech abilities were also able to give detailed descriptions of inquiry activities and recall the experimental conditions that were necessary to conclude something about volume or pitch.
Difficulty and Opinion
Differences between students on the remaining code groups (2) difficulty and (4) opinion were less apparent. Even though differences between profiles were small, it is noteworthy that the “struggling science enthusiasts” had most mentions of “difficulty/easy,” which seems incongruent with the characterization of their profile. In general, students mentioned more aspects they perceived to be difficult than easy (4.27 against 3.80), and far more positive opinions than negative (10.28 against 1.24). In fact, the “average majority,” who were characterized by having a less positive attitude than the other two profiles, had given most positive opinions, although differences were negligible. Most often mentioned as difficult, was the extra assignment where students had to produce a song with a ruler, possibly because this assignment was less structured than the other assignments. As a student described it: “[the extra assignment] was a bit more difficult, because you had to see where the good sounds, good pitch was and where you had to put it […]. I found that a bit more difficult than for example if you, eh, “put your ruler 10 centimeters on the table and the end 10 centimeters from the edge. And what do you hear” […] I found that a bit easier to do.”
With respect to aspects that were perceived as enjoyable, multiple students described the fact that they could use the objects hands-on (e.g., “I especially enjoyed it because you had all these objects and you could produce sounds with these objects” or “The plunking and with the cup. Just plunking a bit, because it made music and that was really fun”), which could also be novel and surprising (e.g., “I did not know you could do this with a ruler, I just figured that if you put it down it would be the same all the time, but it turns out it wasn’t. And also the rubber band really surprised me”). The aspect of working together was mentioned multiple times as positive as well (e.g., “Together with [classmate] I enjoyed it quite a bit […] And then we were like ‘no, that is… no, but this many centimeters, no but that much centimeters. And that was actually quite fun because it’s… a good discussion”), although collaboration was also mentioned as negative twice (e.g., “Because without working together, everything is much quicker and you make less mistakes”).
Learning Process: Designing
Depicted in Table 8, are the frequencies of the code groups per student, per profile, for lesson 3 (designing). Below we will discuss the code groups (1) design demands, (2) specific materials, (7) suggestions for improvement, (3 + 4) difficulty, and (6) opinion. The code group (5) knowledge was not often ascribed, and differences between students were negligible, which is why this code group will not be discussed in further detail.
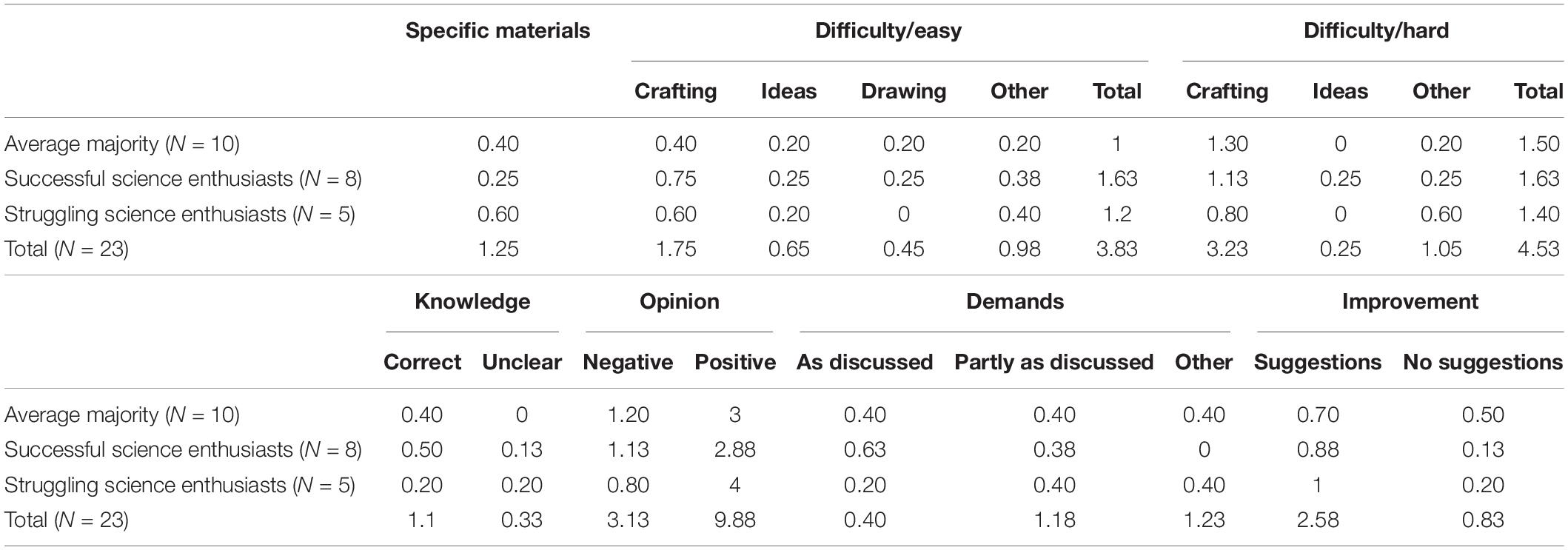
Table 8. Code frequencies of the code groups per profile, divided by the profile sample size, for lesson 3 “designing”.
Design Demands and Specific Materials
An important process within design-based learning is meeting the “design challenge” with its corresponding constraints, requirements or demands (Crismond and Adams, 2012). The “successful science enthusiasts” were most often able to recall all of the design demands as they were discussed in class, whereas the “struggling science enthusiasts” did so least often. This latter profile more often mentioned “other” design demands (e.g., “I wanted nobody to interfere”) as compared to the discussed “correct” design demands. However, frequencies are reversed for the code “specific materials”: the “struggling science enthusiasts” most often described materials in a specific way. This code was ascribed when students, after being asked what materials they used, mentioned an aspect of the material that was relevant for the design challenge. For example, assertions like “I chose the sound box how large I, you know… how loud [.] I wanted it to be” or “I used a thick rubber band and a thin rubber band” show that students thought of their materials as a way of producing a certain sound, which was one of the requirements of the design. Therefore, the “struggling science enthusiasts” students could still be engaged with a relevant design challenge and apply the knowledge they had learned through experimenting, while not being concerned with meeting the specific design demands that were discussed in class or were imposed by a teacher. Another example for this is a student who firstly answered: “I didn’t really have any demands,” when asked about the design demands. When the interviewer asked which design demands were discussed in class, the student was then able to mention some of the discussed design demands. Thus, it seems necessary to look more closely at how a student is engaged with his design, rather than looking solely at quantified performance differences.
Suggestions for Improvement
The “average majority” seemed to have the most difficulty in naming suggestions for improvement of the design, however, differences were small. In general, students were able to provide suggestions when asked about this, such as “You can add more sounds” or “Try something but not attach it, and then see how it sounds” or “If for example the pitch is really out of key, you can tighten or loosen the rubber band.”
Difficulty and Opinion
For lesson 3, the code groups difficulty/easy and difficulty/hard were further categorized into crafting, ideas, drawing (only for code group easy), and other. This distinction was made, as it was noticeable that many students mentioned practical aspects of designing, both as being easy or hard. This might be an indication that the focus of this lesson was not so much on higher-order thinking skills such as problem-solving or the application of science, but was rather an “arts and crafts project” which English and King (2019) warn about. A focus more on crafting rather than science application might explain why the “successful science enthusiasts” were less enthusiastic regarding this lesson, as compared to the other profiles and regarding experimenting. The struggling science enthusiasts on the other hand, were most positive with regard to this lesson. Assuming that these students were merely engaged in a crafting project and hence more positive, does not do justice to their learning process, as the previous described code “specific materials” showed that these students also considered the scientific knowledge needed to optimize their instrument.
In general, several students mentioned the autonomy of designing as a positive aspect. For example: “that you’re mainly busy with working on something and trying things for yourself” or “Well just ehm… the figuring it out for yourself, how it works and stuff. I liked that.” Aspects mentioned as negative were failings in the design (e.g., “Well that it kept failing” or “It didn’t really work”), or firstly having to draw the design (e.g., “I really wanted to start right away, because I have an idea in my mind and I’m afraid that I will lose it”).
Discussion
The current study examined how students’ individual differences are related to their learning outcomes and learning process in S&T-education, during and after an inquiry- and design-based learning lesson unit. To this end, both an extensive quantitative model, to analyze the influence of multiple individual differences on learning outcomes (content knowledge and situational interest), as well as a more in-depth qualitative analysis, to understand the role of individual differences within the learning process, were used. This study is the first to take individual differences into account for both inquiry- and design-based learning, related didactic approaches within S&T-education in the Netherlands (Van Graft and Klein Tank, 2018).
Who Struggles and Who Succeeds?
Our results indicated that reading comprehension, math skills, science skills, and prior knowledge were positively related to content knowledge following the lesson unit on the topic of sound. The importance of these individual differences were also shown in previous studies that have either looked at science achievement or specific subskills of science education (e.g., Mayer et al., 2014; Wagensveld et al., 2015; van der Graaf et al., 2018; Koerber and Osterhaus, 2019; Schlatter et al., 2020). The current study adds to these results by combining multiple individual differences into one model, using both a factor and regression analysis, therefore elucidating the complex interplay of the individual differences and their role in S&T education.
With respect to situational interest, our results indicated that prior knowledge, curiosity and attitude toward S&T (enjoyment and self-efficacy) were positive predictors. This is in line with prior research that has shown that these concepts are greatly interconnected (Schraw et al., 2001; Krapp and Prenzel, 2011; Vongkulluksn et al., 2018; Schmidt and Rotgans, 2020). As an extension to these results, our model shows that affective factors and prior knowledge can affect situational interest. Schraw et al. (2001) have previously revealed the importance of prior knowledge for increasing situational interest. Increasing situational interest, in turn, is important, as situational interest can develop into or contribute to a more long-lasting personal interest or more positive attitude (Hidi, 1990; Palmer, 2004; Palmer et al., 2017; Rotgans and Schmidt, 2017).
Our findings did not replicate earlier studies in regard to the influence of executive functions on science learning (e.g., Osterhaus et al., 2017; van der Graaf et al., 2018). In the study by van der Graaf et al. (2018), it was stated that “executive functions boost knowledge acquisition indirectly via other abilities” (p. 7). Perhaps the model of the current study and/or the small sample size were insufficient in detecting the interplay of executive functions with other cognitive abilities. The same holds true for the influence of affective measures on learning outcomes, although previous studies have shown mixed results and even some disagreement exists regarding the nature of causality (Osborne et al., 2003; Potvin and Hasni, 2014b). Future research is needed to explicate the role of executive functions and affective measures in both inquiry- and design-based learning.
Based on our and prior results, we expected to find similar differences within the learning process. In other words, students with lower academic abilities were expected to struggle more than students with higher academic abilities, and students with a more positive attitude and higher levels of curiosity were expected to be more positive about their learning process. The qualitative analysis of the learning process, however, did not entirely support this expectation, which we will illustrate next.
In order to portray the learning process of a representative selection of struggling and succeeding students, the subsample used for the analyses of the learning process was stratified based on the results of an LPA. The three latent profiles resulting from this analysis were interpreted as (1) “average majority,” (2) “successful science enthusiasts,” and (3) “struggling science enthusiasts.” There was a clear distinction between profiles based on the scores on reading comprehension, math skills, executive functioning and coherence of speech, which is reflected in the first part of the profile names (i.e., average, successful, and struggling). The second and third profile were considerably smaller than the first profile (hence the first profile is called “majority”), and both had relatively high levels of curiosity and attitude toward S&T (hence these profiles are both called “science enthusiasts”).
The students belonging to the “average majority” profile, who had the least positive attitude toward S&T, indeed gave more negative opinions than the other two profiles. This seems in correspondence with the influence of the affective component on situational interest. Nevertheless, these students (essentially) had just as much positive opinions regarding experimenting as the other two profiles and their amount of positive opinions for both experimenting and designing was substantially more than their amount of negative opinions. Thus, there is room to work with: utilizing the aspects that these students find enjoyable to optimize the S&T learning environment can possibly elicit situational interest and ultimately a more stable individual interest or positive attitude (Hidi and Renninger, 2006; Palmer et al., 2017). Aspects of the learning environment that were perceived as enjoyable by multiple students in the current study, which have also been mentioned in previous research, were working hands-on (Palmer, 2009), collaboration with peers (Dohn, 2013) and a sense of autonomy (Hidi and Renninger, 2006).
The second profile, the “successful science enthusiasts” did not show as much of an advantage over the other two profiles as expected. They did not (always) give the most correct knowledge statements, the most positive opinions or the most specific descriptions. When they did, differences with the other profiles were small. The number of positive opinions for designing was even lowest compared to the other profiles. A possible explanation for this is that the lesson regarding designing might have had too much of a focus on constructing the musical instrument, rather than it being a rich context that supports the development of problem-solving skills and a deeper understanding of scientific concepts (English and King, 2019). It might especially be important for these students, who have above-average academic abilities, curiosity and attitudes toward S&T, to be able to connect science with technology and engineering. Additionally, if S&T education falls short in moving beyond a “trial-and-error tinkering process,” students will develop a false image of what a scientist or engineer entails (Clough and Olson, 2016).
The qualitative results for the “struggling science enthusiasts” profile stood out most in comparison to the quantitative results. On the one hand, these students enjoyed both experimenting and designing, made use of their scientific knowledge in selection of design materials, were able to provide suggestions for design improvements, and gave the most systematic descriptions of their experiments as well as the most correct knowledge statements as compared to the other profiles. On the other hand, these students gave many incorrect knowledge statements as well and gave most “unclear” knowledge statements (which indicated confusion of terms or misunderstanding), making the result of “most correct knowledge statements” more ambiguous. They were also least able to reproduce the design demands that were discussed in class. These latter examples, combined with the positive opinions, are in concurrence with the quantitative results and endorse the idea of “struggling science enthusiasts.” Nonetheless, the prior examples also portray a range of successes these students have experienced. Merely classifying these students as “struggling” based on their academic abilities, and tailoring instruction and teacher support accordingly, disregards the possibilities and potential these students have.
These results are in line with a case-study by Doppelt et al. (2008), in which the results from a knowledge test were combined with observations and portfolios. It was found that “the observations and the portfolios showed that the low-achievers reached similar levels of understanding scientific concepts despite doing poorly on the pen-and-paper test” (p. 34). Together with the results of the current study, it thus seems that “struggling” students might not be struggling when other forms of assessment (i.e., other than a paper-pencil-test) are used. This finding is important for teachers and policy makers, in order to determine the most optimal form of differentiation that complements the knowledge students already possess.
In conclusion, by looking more closely at both learning outcomes and learning process of not just inquiry-based learning but also design-based learning, the results of the current study have clarified how S&T education can be tailored to be more inclusive for different types of students.
Limitations and Future Research
A first limitation in the current study is the small sample size. Although we have accounted for the small sample size in our quantitative analysis by using an alternative approach to SEM, a larger sample size would have provided more certainty to our results. Nevertheless, we do feel that, considering this is a mixed-method research, valuable implications can be made for practice as well as further research.
Second, the assessments used in the current study have some limitations. The variables science skills and design skills were not included in our final model as learning outcomes as originally intended. The design skills measure was found to decrease model fit and was excluded to attain a more parsimonious model. This may have been a result of the small sample size, but the validity of the instrument may also be at cause. The self-developed measure of design skills was based on, among other aspects, how well students could represent their ideas compared to the final design solution and meet the demands of the design in their sketch and product. Given that these aspects are assessable through students’ sketches, worksheets and final product, and therefore limit the assessment load for students’, it serves as an efficient way to map students’ design capability. In addition, we observed that students made use of annotations in their design sketches or indicated how their material use would contribute to meeting the design demands. This shows, in concurrence with the results by English et al. (2017), that elementary school students are capable of planning and drawing and applying disciplinary knowledge in their design. However, it may be crucial to also include skills such as troubleshooting, weighing options, revising and iterating or reflection on the process (Crismond and Adams, 2012). It is possible that our selection of skills was too much a function of students’ level of reading comprehension, as the worksheets were a key aspect in assessing design skills. Furthermore, Kelley and Sung emphasize that “design sketching is a skill that needs proper instruction and practice […] and how teachers introduce the role of sketching is critical to how students engage in the process of sketching as a way of thinking” (p. 381). In our lesson unit, only one lesson was focused on designing, which might not be sufficient for students’ design skills to be manifested.
The other intended outcome measure, science skills, was eventually deemed more appropriate in the current study as a measure of individual difference than as a pre- and post-test measure. The skills that this measure assessed are more general science skills instead of skills specifically taught through the current study’s lesson unit. As students’ performance on scientific thinking items can differ substantially, it is important to include a broad-scale of items (Koerber and Osterhaus, 2019). Moreover, as students are often novices in inquiry-based learning, assessing science skills too generally or holistically instead of structured by subskill may fail in measuring the progress of students’ skill ability (Kruit et al., 2018).
Future research can extend the findings of the current study by including more validated assessment measures that take account of the multifaceted skills and knowledge that S&T education comprises. As our qualitative results show, it is complex and possibly undesirable to determine which students are “struggling” or “successful” purely on the score of a written knowledge assessment. In addition, the use of LPA to distinguish students who might represent a larger group of students provided interesting insights into the interplay of individual differences. It thus seems worthwhile to replicate the LPA in a larger sample and pursue this as an effective technique to examine the role of individual differences in S&T education in future approaches.
Implications
The findings of this study provide several implications for education. First, the results of the quantitative analysis stress the important role of academic abilities, such as reading comprehension and math skills, in S&T education. As Schlatter et al. (2020) point out, the monitoring data of students’ academic abilities is thus an easily accessible tool for teachers that can support decisions for differentiation within their S&T lessons. Second, the qualitative results show that students can be (academically) engaged in the S&T learning environment in a variety of ways. Purely adhering to worksheets that must be completed or pre-defined goals of what a student must achieve during a lesson might result in overlooking the students’ effort and successes. Third, the current findings endorse earlier findings on what students find enjoyable about S&T education, such as hand-on activities, novelty or collaboration (Palmer, 2009). This information is essential for tailoring learning environments such that they can trigger interest in S&T (Loukomies et al., 2015). Ultimately, interest could reinforce other affective components and science-related behaviors (Palmer et al., 2017; Sachisthal et al., 2019; Vu et al., 2021). Fourth, supported by both our quantitative and qualitative results, is that language can be a hindering factor in S&T learning; students sometimes explicitly indicated their troubles with the terms used in the lesson unit. Integrating language more prominently in the S&T classroom is thus vital in supporting students’ learning (Smit et al., 2018). Lastly, the current results also point to the need of supporting and guiding students in the inquiry- and design-based learning process. Especially for design-based learning, it is not self-evident for students to use higher-order thinking skills; teachers are needed to support students in developing a deeper understanding of the S&T content (English and King, 2019). Wendell and Rogers (2013) propose practices such as “problematizing phenomena that seemed self-evident, using physical artifacts to embody science ideas, and consulting one-on-one with students about engineering journal work” (p. 533) as supportive to students’ learning of science concepts.
Conclusion
In conclusion, this study is the first to combine both quantitative and qualitative analysis with regard to individual differences in S&T-education. The quantitative results build and extend upon prior research and show the importance of academic abilities and affective factors in gaining content knowledge and affecting situational interest, respectively. The qualitative analysis added a more detailed look into the learning process and revealed the many potentials students have with regard to inquiry- and design based learning. Taken together, the findings of the current study clarify how different types of students succeed or struggle within S&T education. Differentiation aimed at supporting language and the integration of science into design, while facilitating a variety of learning activities and assessments that move beyond written assignments, could help achieve the most optimal learning conditions for each student.
Data Availability Statement
The datasets presented in this article are not readily available because of privacy restrictions. Requests to access the datasets should be directed to TS, dC5zbGltQHZ1Lm5s.
Ethics Statement
The studies involving human participants were reviewed and approved by the Ethics Committee of the Vrije Universiteit Amsterdam (VCWE-2019-114R1). Written informed consent to participate in this study was provided by the participants’ legal guardian/next of kin.
Author Contributions
TS and JS conducted the data collection. TS, JS, AH, and MR conducted the data analysis. TS wrote the manuscript draft. JS, AH, and MR have edited the manuscript draft. All authors contributed to the conception and the design of the study and approved the submitted version.
Conflict of Interest
The authors declare that the research was conducted in the absence of any commercial or financial relationships that could be construed as a potential conflict of interest.
Publisher’s Note
All claims expressed in this article are solely those of the authors and do not necessarily represent those of their affiliated organizations, or those of the publisher, the editors and the reviewers. Any product that may be evaluated in this article, or claim that may be made by its manufacturer, is not guaranteed or endorsed by the publisher.
Acknowledgments
This research was supported by the ‘Stichting tot behartiging van de belangen van RK opleidingsscholen Amsterdam e.o.’ and ‘Stichting tot Steun bij opleiding en begeleiding van leerkrachten in het christelijk basisonderwijs in Amsterdam e.o.’. We greatly appreciate Prof. Yves Rosseel for personal communication about the factor score regression analysis, and Pascalle Ruigrok, Elsje Landman, and Nicky de Vries for help in data collection and analysis.
Supplementary Material
The Supplementary Material for this article can be found online at: https://www.frontiersin.org/articles/10.3389/feduc.2022.842537/full#supplementary-material
Supplementary Data Sheet 1 | Attitude questionnaire items.
Supplementary Data Sheet 2 | Interview protocol.
Supplementary Data Sheet 3 | Factor analysis.
Supplementary Data Sheet 4 | Correlation table.
Supplementary Data Sheet 5 | Latent class analysis-content knowledge test.
Supplementary Data Sheet 6 | Content knowledge test items.
Footnotes
References
Alexander, V. D., Thomas, H., Cronin, A., Fielding, J., and Moran-Ellis, J. (2008). “Mixed methods,” in Researching Social Life, 4th Edn, eds N. Gilbert and P. Stoneman (Thousand Oaks, CA: Sage).
Anyfandi, G., Filippoupoliti, A., and Alexopoulous, I. (2016). “ENGINEER project: Breaking new ground in the science education realm,” in Electronic Proceedings of the ESERA 2015 Conference. Science Education Research: Engaging Learners for a Sustainable Future, eds J. Lavonen, K. Juuti, J. Lampiselkä, A. Uitto, and K. Hahl (Helsinki: University of Helsinki).
Brotman, J. S., and Moore, F. M. (2008). Girls and science: a review of four themes in the science education literature. J. Res. Sci. Teach. 45, 971–1002. doi: 10.1002/tea.20241
Byrnes, J. P., Miller-Cotto, D., and Wang, A. H. (2018). Children as mediators of their own cognitive development: the case of learning science in kindergarten and first grade. J. Cogn. Dev. 19, 248–277. doi: 10.1080/15248372.2018.1470975
Campbell, J. L., Quincy, C., Osserman, J., and Pedersen, O. K. (2013). Coding in-depth semistructured interviews: problems of unitization and intercoder reliability and agreement. Sociol. Methods Res. 42, 294–320. doi: 10.1177/0049124113500475
Chuderski, A., and Jastrzebski, J. (2018). Much ado about aha!: Insight problem solving is strongly related to working memory capacity and reasoning ability. J. Exp. Psychol. Gen. 147, 257–281. doi: 10.1037/xge0000378
Clough, M. P., and Olson, J. K. (2016). “Final Commentary: Connecting Science and Engineering Practices: A Cautionary Perspective,” in Connecting Science and Engineering Education Practices in Meaningful Ways, Vol. 44, eds L. A. Annetta and J. Minogue (Berlin: Springer International Publishing), doi: 10.1007/978-3-319-16399-4
Crismond, D. P., and Adams, R. S. (2012). The informed design teaching and learning matrix. J. Eng. Educ. 101, 738–797.
Denessen, E., Vos, N., Hasselman, F., and Louws, M. (2015). The Relationship between Primary School Teacher and Student Attitudes towards Science and Technology. Educ. Res. Int. 2015, 1–7. doi: 10.1155/2015/534690
Devlieger, I., Talloen, W., and Rosseel, Y. (2019). New Developments in Factor Score Regression: Fit Indices and a Model Comparison Test. Educ. Psychol. Meas. 79, 1017–1037. doi: 10.1177/0013164419844552
DeWitt, J., and Archer, L. (2015). Who aspires to a science career? A comparison of survey responses from primary and secondary school students. Int. J. Sci. Educ. 37, 2170–2192. doi: 10.1080/09500693.2015.1071899
Diamond, A. (2013). Executive Functions. Annu. Rev. Psychol. 64, 135–168. doi: 10.1146/annurev-psych-113011-143750
Dohn, N. B. (2013). Situational interest in engineering design activities. Int. J. Sci. Educ. 35, 2057–2078. doi: 10.1080/09500693.2012.757670
Doppelt, Y., Schunn, C. D., Mehalik, M. M., Silk, E., and Krysinski, D. (2008). Engagement and achievements: a case study of design-based learning in a science context. J. Technol. Educ. 19, 22–39.
Driessen, G., and van Langen, A. (2013). Gender differences in primary and secondary education: Are girls really outperforming boys? Int. Rev. Educ. 59, 67–86. doi: 10.1007/s11159-013-9352-6
ENGINEER (2014). Music to the Ears: Designing and Creating a Sound Generator. Available online at: http://www.engineer-project.eu/download/acoustics/index.html (accessed June 25, 2020).
English, L. D., and King, D. (2019). STEM Integration in Sixth Grade: desligning and Constructing Paper Bridges. Int. J. Sci. Math. Educ. 17, 863–884. doi: 10.1007/s10763-018-9912-0
English, L. D., King, D., and Smeed, J. (2017). Advancing integrated STEM learning through engineering design: sixth-grade students’ design and construction of earthquake resistant buildings. J. Educ. Res. 110, 255–271. doi: 10.1080/00220671.2016.1264053
English, L. D., and King, D. T. (2015). STEM learning through engineering design: fourth-grade students’ investigations in aerospace. Int. J. STEM Educ. 2, 1–18. doi: 10.1186/s40594-015-0027-7
Furtak, E. M., Seidel, T., Iverson, H., and Briggs, D. C. (2012). Experimental and quasi-experimental studies of inquiry-based science teaching: a meta-analysis. Rev. Educ. Res. 82, 300–329. doi: 10.3102/0034654312457206
Geurts, H. M. (2007). CCC – 2 – NL: Children’s Communication Checklist – 2 (Bishop D.M.V.). Amsterdam: Pearson Assessment and Information B.V.
Gioia, G. A., Isquith, P. K., Guy, S. C., Kenworthy, L., and Baron, I. S. (2000). Behavior rating inventory of executive function. Child Neuropsychol. 6, 235–238. doi: 10.1076/chin.6.3.235.3152
Glaser, B. G., and Strauss, A. L. (1967). The Discovery of Grounded Theory. Strategies for Qualitative Research. Mill Valley, CA: Sociology Press.
Hanson, S. L., Sykes, M., and Pena, L. B. (2017). Gender equity in science: the global context. Int. J. Soc. Sci. Stud. 6, 33–47. doi: 10.11114/ijsss.v6i1.2704
Hickendorff, M., Edelsbrunner, P. A., Mcmullen, J., Schneider, M., and Trezise, K. (2018). Informative tools for characterizing individual differences in learning: latent class, latent profile, and latent transition analysis. Learn. Individ. Dif. 66, 4–15. doi: 10.1016/j.lindif.2018.06.001
Hidi, S. (1990). Interest and its contribution as a mental resource for learning. Rev. Educ. Res. 60, 549–571. doi: 10.3102/00346543060004549
Hidi, S., and Renninger, A. (2006). The four-phase model of interest development. Am. J. Psychiatry 41, 111–127. doi: 10.1207/s15326985ep4102
Huizinga, M., and Smidts, D. P. (2012). BRIEF Vragenlijst Executieve Functies voor 5- tot 18-Jarigen: Handleiding. Amsterdam: Hogrefe Uitgevers.
Jirout, J., and Klahr, D. (2012). Children’s scientific curiosity: in search of an operational definition of an elusive concept. Dev. Rev. 32, 125–160. doi: 10.1016/j.dr.2012.04.002
Kelley, T. R., Capobianco, B. M., and Kaluf, K. J. (2015). Concurrent think-aloud protocols to assess elementary design students. Int. J. Technol. Design Educ. 25, 521–540. doi: 10.1007/s10798-014-9291-y
Kelley, T. R., and Sung, E. (2017). Sketching by design: teaching sketching to young learners. Int. J. Technol. Design Educ. 27, 363–386. doi: 10.1007/s10798-016-9354-3
Kline, R. B. (2011). Principles and Practice of Structural Equation Modeling. New York, NY: Guilford Press.
Koerber, S., and Osterhaus, C. (2019). Individual differences in early scientific thinking: assessment, cognitive influences, and their relevance for science learning. J. Cogn. Dev. 20, 510–533. doi: 10.1080/15248372.2019.1620232
Krapp, A., and Prenzel, M. (2011). Research on Interest in Science: theories, methods, and findings. Int. J. Sci. Educ. 33, 27–50. doi: 10.1080/09500693.2010.518645
Krippendorff, K. (2004). Reliability in Content Analysis. Hum. Commun. Res. 30, 411–433. doi: 10.1111/j.1468-2958.2004.tb00738.x
Kruit, P. M., Oostdam, R. J., van den Berg, E., and Schuitema, J. A. (2018). Assessing students’ ability in performing scientific inquiry: instruments for measuring science skills in primary education. Res. Sci. Technol. Educ. 36, 413–439. doi: 10.1080/02635143.2017.1421530
Kuhn, D., and Pease, M. (2006). Do children and adults learn differently? J. Cogn. Dev. 7, 279–293. doi: 10.1207/s15327647jcd0703_1
Lautrey, J., and Mazens, K. (2004). Is children’s naive knowledge consistent? A comparison of the concepts of sound and heat. Learn. Instr. 14, 399–423. doi: 10.1016/j.learninstruc.2004.06.011
Lazonder, A. W., Janssen, N., Gijlers, H., and Walraven, A. (2020). Patterns of development in children’s scientific reasoning: results from a three-year longitudinal study. J. Cogn. Dev. [Epub ahead of print]. doi: 10.1080/15248372.2020.1814293
Lewis, T. (2006). Design and inquiry: bases for an accommodation between science and technology education in the curriculum? J. Res. Sci. Teach. 43, 255–281. doi: 10.1002/tea.20111
Lie, R., Selcen Guzey, S., and Moore, T. J. (2019). Implementing engineering in diverse upper elementary and middle school science classrooms: student learning and attitudes. J. Sci. Educ. Technol. 28, 104–117. doi: 10.1007/s10956-018-9751-3
Loukomies, A., Juuti, K., and Lavonen, J. (2015). Investigating Situational Interest in Primary Science Lessons. Int. J. Sci. Educ. 37, 3015–3037. doi: 10.1080/09500693.2015.1119909
Luo, Y. (2015). Design fixation and cooperative learning in elementary engineering design project: a case study. Int. Electron. J. Elem. Educ. 8, 601–614.
Mareschal, D. (2016). The neuroscience of conceptual learning in science and mathematics. Curr. Opin. Behav. Sci. 10, 114–118. doi: 10.1016/j.cobeha.2016.06.001
Martin, M. O., Mullis, I. V. S., Foy, P., and Hooper, M. (2016). TIMSS 2015 International Results in Science. Boston, MA: TIMSS & PIRLS International Study Center.
Martín-Páez, T., Aguilera, D., Perales-Palacios, F. J., and Vílchez-González, J. M. (2019). What are we talking about when we talk about STEM education? A review of literature. Sci. Educ. 103, 799–822. doi: 10.1002/sce.21522
Marulcu, I., and Barnett, M. (2013). Fifth Graders’ learning about simple machines through engineering design-based instruction using LEGO™ Materials. Res. Sci. Educ. 43, 1825–1850. doi: 10.1007/s11165-012-9335-9
Mayer, D., Sodian, B., Koerber, S., and Schwippert, K. (2014). Scientific reasoning in elementary school children: assessment and relations with cognitive abilities. Learn. Instr. 29, 43–55. doi: 10.1016/j.learninstruc.2013.07.005
McFadden, J., and Roehrig, G. (2019). Engineering design in the elementary science classroom: supporting student discourse during an engineering design challenge. Int. J.Technol. Design Educ. 29, 231–262. doi: 10.1007/s10798-018-9444-5
Mehalik, M. M., Doppelt, Y., and Schuun, C. D. (2008). Middle-school science through design-based learning versus scripted. Inquiry: better overall science concept learning and equity gap reduction. J. Eng. Educ. 97, 71–85. doi: 10.1002/j.2168-9830.2008.tb00955.x
Mullis, I. V. S., Martin, M. O., Foy, P., Kelly, D. L., and Fishbein, B. (2020). TIMSS 2019 International Results in Mathematics and Science. Boston, MA: TIMSS & PIRLS International Study Center.
Nemo (n.d.). Maakkunde: Module Muziek. Available online at: https://maakkunde.nl/themas/geluid/ (accessed June 25, 2020).
O’Brien, B. C., Harris, I. B., Beckman, T. J., Reed, D. A., and Cook, D. A. (2014). Standards for reporting qualitative research: a synthesis of recommendations. Acad. Med. 89, 1245–1251. doi: 10.1097/ACM.0000000000000388
Osborne, J., Simon, S., and Collins, S. (2003). Attitudes towards science: a review of the literature and its implications. Attitude Towards Sci. 25, 1049–1079. doi: 10.1080/0950069032000032199
Osterhaus, C., Koerber, S., and Sodian, B. (2017). Scientific thinking in elementary school: children’s social cognition and their epistemological understanding promote experimentation skills. Dev. Psychol. 53, 450–462. doi: 10.1037/dev0000260
Palmer, D. H. (2004). Situational interest and the attitudes towards science of primary teacher education students. Int. J. Sci. Educ. 26, 895–908. doi: 10.1080/0950069032000177262
Palmer, D. H. (2009). Student interest generated during an inquiry skills lesson. J. Res. Sci. Teach. 46, 147–165. doi: 10.1002/tea.20263
Palmer, D. H., Dixon, J., and Archer, J. (2017). Using Situational Interest to Enhance Individual Interest and Science-Related Behaviours. Res. Sci. Educ. 47, 731–753. doi: 10.1007/s11165-016-9526-x
Pedaste, M., Mäeots, M., Siiman, L. A., de Jong, T., van Riesen, S. A. N., Kamp, E. T., et al. (2015). Phases of inquiry-based learning: definitions and the inquiry cycle. Educ. Res. Rev. 14, 47–61. doi: 10.1016/j.edurev.2015.02.003
Potvin, P., and Hasni, A. (2014a). Analysis of the Decline in Interest Towards School Science and Technology from Grades 5 Through 11. J. Sci. Educ. Technol. 23, 784–802. doi: 10.1007/s10956-014-9512-x
Potvin, P., and Hasni, A. (2014b). Interest, motivation and attitude towards science and technology at K-12 levels: a systematic review of 12 years of educational research. Stud. Sci. Educ. 50, 85–129. doi: 10.1080/03057267.2014.881626
Purzer, Ş, Goldstein, M. H., Adams, R. S., Xie, C., and Nourian, S. (2015). An exploratory study of informed engineering design behaviors associated with scientific explanations. Int. J. STEM Educ. 2:9. doi: 10.1186/s40594-015-0019-7
Robinson, O. C. (2014). Sampling in interview-based qualitative research: a theoretical and practical guide. Qual. Res. Psychol. 11, 25–41. doi: 10.1080/14780887.2013.801543
Rocard, M., Csermely, P., Jorde, D., Lenzen, D., Walberg-Henriksson, H., and Hemmo, V. (2007). Science Education NOW: A Renewed Pedagogy for the Future of Europe. Lakewood, CO: RTD info.
Rosseel, Y. (2012). Lavaan: an R package for structural equation modeling. J. Stat. Softw. 48, 1–36. doi: 10.18637/jss.v048.i02
Rotgans, J. I., and Schmidt, H. G. (2011a). Situational interest and academic achievement in the active-learning classroom. Learn. Instr. 21, 58–67. doi: 10.1016/j.learninstruc.2009.11.001
Rotgans, J. I., and Schmidt, H. G. (2011b). The role of teachers in facilitating situational interest in an active-learning classroom. Teach. Teach. Educ. 27, 37–42. doi: 10.1016/j.tate.2010.06.025
Rotgans, J. I., and Schmidt, H. G. (2017). Interest development: arousing situational interest affects the growth trajectory of individual interest. Contemp. Educ. Psychol. 49, 175–184. doi: 10.1016/j.cedpsych.2017.02.003
Roth, W. M. (2001). Learning science through technological design. J. Res. Sci. Teach. 38, 768–790. doi: 10.1002/tea.1031
Sachisthal, M. S. M., Jansen, B. R. J., Peetsma, T. T. D., Dalege, J., van der Maas, H. L. J., and Raijmakers, M. E. J. (2019). Introducing a science interest network model to reveal country differences. J. Educ. Psychol. 111, 1063–1080. doi: 10.1037/edu0000327
Saldana, J. (2009). The Coding Manual for Qualitative Researchers. Thousand Oaks, CA: Sage Publications.
Schlatter, E., Molenaar, I., and Lazonder, A. W. (2020). Individual differences in children’s development of scientific reasoning through inquiry-based instruction: Who Needs Additional Guidance? Front. Psychol. 11:904. doi: 10.3389/fpsyg.2020.00904
Schmidt, H. G., and Rotgans, J. I. (2020). Epistemic curiosity and situational interest: Distant Cousins or Identical Twins? Educ. Psychol. Rev. 33, 325–352. doi: 10.1007/s10648-020-09539-9
Schraw, G., Flowerday, T., and Lehman, S. (2001). Increasing situational interest in the classroom. Educ. Psychol. Rev. 13, 211–224. doi: 10.1023/A:1016619705184
Schreiber, J. B., Nora, A., Stage, F. K., Barlow, E. A., and King, J. (2006). Reporting structural equation modeling and confirmatory factor analysis results: a review. J. Educ. Res. 99, 323–338. doi: 10.3200/JOER.99.6.323-338
Sheu, H.-B., Lent, R. W., Miller, M. J., Penn, L. T., Cusick, M. E., and Truong, N. N. (2018). Sources of self-efficacy and outcome expectations in science, technology, engineering, and mathematics domains: a meta-analysis. J. Vocat. Behav. 109, 118–136. doi: 10.1016/j.jvb.2018.10.003
Smit, J., Gijsel, M., Hotze, A., and Bakker, A. (2018). Scaffolding primary teachers in designing and enacting language-oriented science lessons: Is handing over to independence a fata morgana? Learn. Cult. Soc. Interact. 18, 72–85. doi: 10.1016/j.lcsi.2018.03.006
Stender, A., Schwichow, M., Zimmerman, C., and Härtig, H. (2018). Making inquiry-based science learning visible: the influence of CVS and cognitive skills on content knowledge learning in guided inquiry. Int. J. Sci. Educ. 40, 1812–1831. doi: 10.1080/09500693.2018.1504346
Tomlinson, C. A. (2000). Differentiation of Instruction in the Elementary Grades. ERIC Digests. Available online at: https://files.eric.ed.gov/fulltext/ED443572.pdf (accessed December 12, 2021).
Vaino, K., Vaino, T., and Ottander, C. (2018). Designing an ice cream making device: a design-based science learning approach. Sci. Educ. Int. 29, 149–162.
van Aalderen-Smeets, S. I., and Walma van der Molen, J. H. (2018). Modeling the relation between students’ implicit beliefs about their abilities and their educational STEM choices. Int. J. Technol. Design Educ. 28, 1–27. doi: 10.1007/s10798-016-9387-7
Van de Sande, E., Kleemans, T., Verhoeven, L., and Segers, E. (2019). The linguistic nature of Children’s scientific reasoning. Learn. Instr. 62, 20–26. doi: 10.1016/j.learninstruc.2019.02.002
van der Graaf, J., Segers, E., and Verhoeven, L. (2018). Individual differences in the development of scientific thinking in kindergarten. Learn. Instr. 56, 1–9. doi: 10.1016/j.learninstruc.2018.03.005
van Dijk, A. M., Eysink, T. H. S., and De Jong, T. (2016). Ability-related differences in performance of an inquiry task: the added value of prompts. Learn. Individ. Dif. 47, 145–155. doi: 10.1016/j.lindif.2016.01.008
Van Graft, M., and Klein Tank, M. (2018). Wetenschap en Technologie in het Basis- en Speciaal Onderwijs: Richtinggevend Leerplankader bij het Leergebied Oriëntatie op Jezelf en de Wereld. Enschede: SLO.
Van Schijndel, T. J. P., Jansen, B. R. J., and Raijmakers, M. E. J. (2018). Do individual differences in children’s curiosity relate to their inquiry-based learning? Int. J. Sci. Educ. 40, 996–1015. doi: 10.1080/09500693.2018.1460772
Vedder-Weiss, D., and Fortus, D. (2012). Adolescents’ declining motivation to learn science: a follow-up study. J. Res. Sci. Teach. 49, 1057–1095. doi: 10.1002/tea.21049
Vongkulluksn, V. W., Matewos, A. M., Sinatra, G. M., and Marsh, J. A. (2018). Motivational factors in makerspaces: a mixed methods study of elementary school students’ situational interest, self-efficacy, and achievement emotions. Int. J. STEM Educ. 5:43. doi: 10.1186/s40594-018-0129-0
Vosniadou, S., Ioannides, C., Dimitrakopoulou, A., and Papademetriou, E. (2001). Designing learning environments to promote conceptual change in science. Learn. Instr. 11, 381–419. doi: 10.1016/S0959-4752(00)00038-4
Vu, T., Magis-Weinberg, L., Jansen, B. R. J., van Atteveldt, N., Janssen, T. W. P., Lee, N. C., et al. (2021). Motivation-Achievement Cycles in Learning: a Literature Review and Research Agenda. Educ. Psychol. Rev. 31, 121–162.
Wagensveld, B., Segers, E., Kleemans, T., and Verhoeven, L. (2015). Child predictors of learning to control variables via instruction or self-discovery. Instr. Sci. 43, 365–379. doi: 10.1007/s11251-014-9334-5
Weible, J. L., and Zimmerman, H. T. (2016). Science curiosity in learning environments: developing an attitudinal scale for research in schools, homes, museums, and the community. Int. J. Sci. Educ. 38, 1235–1255. doi: 10.1080/09500693.2016.1186853
Wendell, K. B., and Rogers, C. (2013). Engineering design-based science, science content performance, and science attitudes in elementary school. J. Eng. Educ. 102, 513–540. doi: 10.1002/jee.20026
Keywords: science education, individual differences, mixed methods, inquiry-based learning, design-based learning, stem education
Citation: Slim T, van Schaik JE, Dobber M, Hotze ACG and Raijmakers MEJ (2022) Struggling or Succeeding in Science and Technology Education: Elementary School Students’ Individual Differences During Inquiry- and Design-Based Learning. Front. Educ. 7:842537. doi: 10.3389/feduc.2022.842537
Received: 23 December 2021; Accepted: 24 May 2022;
Published: 13 June 2022.
Edited by:
Peter Adriaan Edelsbrunner, ETH Zürich, SwitzerlandReviewed by:
Julie Vaiopoulou, Aristotle University of Thessaloniki, GreeceFrank Reinhold, University of Education Freiburg, Germany
Copyright © 2022 Slim, van Schaik, Dobber, Hotze and Raijmakers. This is an open-access article distributed under the terms of the Creative Commons Attribution License (CC BY). The use, distribution or reproduction in other forums is permitted, provided the original author(s) and the copyright owner(s) are credited and that the original publication in this journal is cited, in accordance with accepted academic practice. No use, distribution or reproduction is permitted which does not comply with these terms.
*Correspondence: Tessa Slim, dC5zbGltQHZ1Lm5s
†These authors have contributed equally to this work and share last authorship