- 1Institutional Analytics – Research and Analytics, Indiana University, Bloomington, IN, United States
- 2Undergraduate Education, College of Literature, Science, and the Arts, University of Michigan, Ann Arbor, MI, United States
- 3Combined Program in Education and Psychology, University of Michigan, Ann Arbor, MI, United States
- 4Center for Academic Innovation, University of Michigan, Ann Arbor, MI, United States
- 5Physics, College of Literature, Science, and the Arts, University of Michigan, Ann Arbor, MI, United States
- 6College of Education and Human Development, University of Delaware, Newark, DE, United States
- 7Division of Teaching Excellence and Innovation, University of California, Irvine, Irvine, CA, United States
- 8Department of Neurobiology, Physiology and Behavior, University of California, Davis, Davis, CA, United States
- 9Center for the Study of Higher and Postsecondary Education, University of Michigan, Ann Arbor, MI, United States
Institutions of higher learning are characterized by multiple, often intersecting, social-educational structures aimed at regulating the conditions by which a degree is ultimately granted. The sequence of courses that students must take for a degree is one such structure. Building on the Sloan Equity and Inclusion in STEM Introductory Courses (SEISMIC) Collaboration’s prior work, we provide a comparative view of students’ pathways through selected curricula at two participating institutions. We apply process analytics to students’ course enrollments as a tool to reveal features of the curricula and the associated impacts on students’ progressions to degree. Given the high enrollment in biology-related degree programs at these institutions, we focus on those and ask two questions: (1) Is the intended progression through the curriculum the one most commonly experienced by the students? and (2) does the maintenance of coherence and socialization into the discipline act in a similar way on individuals of different socio, economic and demographic backgrounds? Curriculum analytics tends to be driven by a reductionist view of its structure. Instead, we view the curriculum as a tool for disciplinary acculturation, revealing aspects of students’ transitions through educational systems not captured by commonly applied course or retention analyses. Curricular structures and the constraints they impose impact the way individual students become members of a scholarly community by acting as a cultural and social homogenizing agent. Across the curricula and institutions in this study, we find that this process results in minoritization, hampering student progression through the curriculum and contributing to disciplinary exclusion in favor of traditionally advantaged socio-demographic groups. We call for curricular restructuring that (1) reduces or alters the depth of the hierarchical course sequences, changing the way progression is established; and (2) encourages adoption of pedagogical approaches in the courses that adapt to the learning community to which they cater; ultimately incorporating an asset-based approach to the acquisition of knowledge inclusive of students’ diversity of backgrounds, experiences, and ways of being.
1. Introduction
United States’ higher education institutions are embedded with structural characteristics that hinder minoritized students from obtaining science, technology, engineering and mathematics (STEM) bachelor degrees (Benitez, 2010; National Science Foundation, 2021). Minoritized students are those who are affected by historical and contemporary processes that limit educational access and participation (Benitez, 2010). Prior work has illustrated examples of how structural inequities in STEM higher education offer advantages in degree attainment to students who hold privileged status with respect to their racial, gender, economic, and educational backgrounds (Seymour and Hunter, 2019; McGee, 2020; Blair-Loy and Cech, 2022). This suggests the existence of a continuum between the conditions experienced by minoritized students in the K-12 system and the higher education context. For example, students who do not come from higher economic status backgrounds or continuing-generation families tend to experience conditions in their educational environment not conducive to pursuing a STEM degree. These factors include psycho-social processes reinforcing the idea of non-belonging (Bottia et al., 2021; O’Hara, 2022), the quality and offering of the STEM curriculum, and the availability and access to advanced placement courses (Mensah and Jackson, 2018; Bottia et al., 2021). Additionally, women and students of color discuss how STEM higher education environments are embedded with gendered and race-based stereotypes that limit their access to resources and recognition from peers and colleagues (Steele and Aronson, 1995; Herzig, 2004; Du, 2006; McGee and Martin, 2011; De Grandi et al., 2021; Nishi, 2021; Tomeh and Sackett, 2022). Thus, active and passive forms of exclusion hinder minoritized undergraduate students from advancing through the higher education system. The goal of this paper is to extend our understanding of minoritization by exploring the ways in which another STEM higher education structure – curriculum – contributes to creating additional structural barriers to who can obtain a STEM bachelor’s degree. Focusing on curriculum and student pathways is a currently emerging and important area of investigation (Kizilcec et al., 2023). Traditionally, the curricular structure is perceived as a neutral component for individuals who pursue a higher education degree. However, research has long documented how curricular structures in K-12 education are racialized, gendered, and classed that promote the learning of students from privileged social positions (Oakes, 1985; Tyson, 2011; Lewis and Diamond, 2015). Moreover, recent research documents how academic tracking continues from K-12 education into higher education through differing levels of support and university policies and practices guiding students down different degree pathways and extending beyond the oft-focused “gatekeeping” courses (Stich, 2021). In this paper, we offer evidence of how curricular structures in higher education operate in ways that afford undergraduate students who belong to privileged social groups better chances of obtaining a STEM degree; thereby, hindering the progression of minoritized undergraduate students.
While all college majors have a core set of course requirements, there is heterogeneity across institutions on the exact courses required for each major (Cheesman et al., 2007). Even within the same university, where the core sequence of courses required for each major is set, a variety of factors result in variations in how students with similar degree goals progress through course sequences. Institutions often encourage or require students to take a particular version of a course or even enroll in a related preparatory course depending on their proficiency level upon entry to the university. Rules surrounding Advanced Placement (AP) credits and other test-related and transfer credits also vary across institutions (Fischer et al., 2023) and build on the inequitable access to AP classes in the K-12 system (Lewis and Diamond, 2015; Hirschl and Smith, 2023). In recent years, the field of Learning Analytics has started to examine the structure of curricula for different majors and institutions (e.g., Dawson and Hubball, 2014; Wigdahl et al., 2014; Aldrich, 2015; Heileman et al., 2018; Hilliger et al., 2020), with the goal of optimizing curricula to streamline overall progression to degree. However, other aspects of curricula, among them how they may differentially impact outcomes for students of different backgrounds, remain understudied.
One common feature of curricula is that they define social spaces aimed at empowering students to utilize, acquire and practice social and cultural capitals necessary to belong and perform in an academic community (Naidoo, 2004; McCoy et al., 2017; Reinholz et al., 2019). Only those who complete the course of study can claim membership in the group (O’Connor et al., 2015). Curricula contribute in structuring participation and engagement in an acculturation process that reinforces hierarchical power relations and imposes dominant models of success (Weatherton and Schussler, 2021). Existing qualitative research demonstrates how STEM curricula bestow advantages to students who belong to privileged social groups (Masta, 2019; Hales, 2020; Leyva et al., 2021a). For instance, Masta (2019) illustrated how STEM higher education classrooms socialize indigenous students to abandon the lived experiences of their communities and adopt white settler colonial perspectives. Masta (2019) argues that the result is the marginalization and exclusion of forms of social and cultural capital that are assets of nondominant groups (see also Yosso, 2005; O’Shea, 2016). Therefore, existing qualitative work demonstrates how STEM higher education curricula negatively contribute to the minoritization of undergraduate students. Inspired by this work, this paper seeks to interrogate the relationship between curricula and undergraduate student outcomes across multiple university contexts. Specifically, this paper suggests that curricula are equitably designed only when the ability to reach the end of a curriculum path is not related to the social position of a student (i.e., race, gender, education and economic background) (however, see Rodriguez et al., 2012; Pearson et al., 2022 for a critique of this minimum requirement).
Clearly, the COVID-19 pandemic caused a series of disruptions in higher education, the implications of which are still unclear and unfolding. The data here presented intentionally excludes the most recent time period to allow for a comparison of curricular systems in a somewhat more homeostatic and stable state. It is yet unclear the extent that lessons learned from the impact the pandemic had on our students will result in significant changes in curricular structures, or if institutions will return to ‘business as usual’ after the emergency passes (Matz et al., 2023). Focusing on how curricula acted on students before the pandemic will, hopefully, contribute to addressing systemic inequities that characterized higher education up until then and may continue after.
We use a methodological approach called Educational Process Mining (Trčka and Pechenizkiy, 2009) to map students’ transitions through biology and chemistry course sequences required by undergraduate bachelor’s biology degree programs at two large, public, research-intensive universities in the U.S. In particular, we show how the required course sequences are structures that create funnels rather than alternative pathways for students of diverse backgrounds (see, e.g., Maltese et al., 2014 for a discussion of STEM pipelines), thus homogenizing and reducing the social and economic diversity of STEM majors. Such marginalizing curricular structures reproduce the existing broader structures of racial minoritization (Benitez, 2010) and exclusion in STEM, unintentionally continuing tracking processes characteristic of K-12 environments (Mensah and Jackson, 2018; Bottia et al., 2021). The following research questions (RQs) guide the study:
RQ 1: Is the intended progression through the curriculum the one most commonly experienced by the students?
RQ 2: Does the maintenance of coherence and socialization into the discipline act in a similar way on individuals of different socio, economic and demographic backgrounds?
This paper concludes with a discussion of the opportunities that this type of analysis affords in revealing commonalities of curriculum-based inequities.
2. Theoretical framework and literature review
2.1. Departmental curricula are educational structures
Academic fields are often referred to as academic disciplines, connecting an area of knowledge to practices of acquisition, maintenance, and (re-)production of that knowledge (see Reinholz et al., 2019 for a recent discussion related to STEM). According to the Oxford English Dictionary, “disciplines,” in the sense of training and instruction, can be defined as:
“Instruction or teaching intended to [mold] the mind and character and instill a sense of proper, orderly conduct and action; training to behave or act in a controlled and effective manner; mental, intellectual, moral, or spiritual training or exercise.” (OED, 2023).
Curricula serve a significant role in this process of structuring academic training and practice. Faculty in academic disciplines work with university administrators to construct a layout of course requirements for undergraduate (and graduate) students. These course layouts reflect institutional and disciplinary expectations about which content, modalities of knowledge acquisitions, and milestones need to be achieved to obtain a particular bachelor’s degree (O’Connor et al., 2015). Students who successfully progress through required coursework are characterized as having the foundational knowledge and practices of an academic discipline (Pfeffer and Langton, 1988; Konrad and Pfeffer, 1990; Bowen, 1999; O’Connor et al., 2015). These practices are internalized and reproduced by members of the community while acting in the system. Within Gramsci’s theoretical approach, this is how hegemony operates, and how traditional intellectuals participate in maintaining and reinforcing the dominant ideological system. In the words of Bates (1975, p. 616):
“The concept of hegemony is really a very simple one. It means political leadership based on the consent of the led, a consent which is secured by the diffusion and popularization of the world view of the ruling class [sic]. [...]It is possible to affirm that the essential feature of the most modern philosophy of praxis consists precisely in the historico-political concept of hegemony.”
Acculturation in the system places the actors in an active/passive relationship vis a vis how the system operates, and it is maintained. Here, in part, we see a convergence with structuralists’ analysis of education systems. Academic disciplinary curricula are the core educational structure that shapes students’ cultural and social capitals necessary to fulfill specific roles in society. Institutions of higher education evolved to serve students who had achieved the necessary requirements to advance in the educational system. Expectations in this sense are relevant for understanding student experiences and their opportunities to perform in the social-educational context of universities, as well as how the socio-educational context operates in enforcing the needed capital for integrating students into the profession, and middle and upper classes (see Webb et al., 2017 for a discussion of Bourdieu’s contribution to these insights).
This poststructuralist understanding of higher education has evolved and been integrated into more recent theoretical frameworks and analysis of the roles that structures have in shaping student opportunities in higher education. For example, McCoy et al. (2017) apply the concepts of the field of practice, social and cultural capital to compare the experience of STEM students in predominantly white colleges versus historically Black colleges, describing how in the former context, Black students struggle in securing advisors’ support for building the needed social and cultural capital to be effective in their aspirational field. In Critical Race Theory, the system of power relations experienced by these students is also shaping systems where “tracking and grouping practices that differentiate curricula and instruction” hamper the opportunities for persisting in STEM (Mensah and Jackson, 2018; Bottia et al., 2021, p. 618), or to become active participants in programs that support students in the STEM pipeline (Dixson and Rousseau, 2005; O’Hara, 2022). This chronic unequal distribution of resources places minoritized students at a disadvantage when interacting with a system that portrays itself as being meritocratic (Carnevale et al., 2020; O’Hara, 2022). At the same time, the system is unable to accommodate and empower diverse forms of social and cultural capital (Yosso, 2005).
Hence, the need to properly understand structures as elements regulating, shaping and constraining human activities emerges from the realization that they tend to work to the advantage of historically privileged groups and to the detriment of others (Webb et al., 2017), ultimately affecting opportunities for diverse students to enact and contribute their aspirational professional roles in society (see, e.g., Østergaard et al., 2011; Nielsen et al., 2018; Koning et al., 2021).
In higher education institutions, curricula exist as structures that have a significant impact on students’ retention and progression to a degree, particularly when obtaining a STEM degree (O’Connor et al., 2015). One example of curricula as structures is the way STEM introductory courses in higher education provide unequal access to higher level curricula for undergraduate students across social groups. Oftentimes, STEM courses formally build on each other, requiring a passing grade in each course before a student is allowed to take the following course in the sequence. Failing one course in the sequence not only sets the student back by at least one academic term, but also segregates the student from that cohort of peers and, depending on the frequency of course offering, potentially derails a student’s academic progression. For instance, the lack of diversity in introductory STEM courses creates conditions in which minoritized students are provided fewer resources and agency than their counterparts (Ray, 2019). Within the classroom context, racial and gendered stereotypes of who is considered a STEM person results in women and students of color having to prove their intellectual abilities to their peers and instructors, making it harder for them to find peer study groups and reinforcing feelings of not belonging (Strayhorn, 2011; Seymour and Hunter, 2019; Leyva et al., 2021b; McGee, 2021). Thus, introductory STEM courses often act as “gatekeepers” or “filters,” as failing an introductory STEM course is highly correlated with students, particularly minoritized students, switching out of STEM majors (Matz et al., 2017; Seymour and Hunter, 2019; Castle et al., 2021; Thompson, 2021; Whitcomb et al., 2021; Hatfield et al., 2022).
2.2. Critique of existing analytic approaches for curricula as structures
The structures that emerge from a major’s curricular requirements and the interdependency across the introductory courses lead to students following a variety of curricular paths with different outcomes (graduating in that major, switching to another major, leaving the university, etc.). Given their complexity, the quantitative characterization of these curricular paths has been an analytical challenge for quite some time (e.g., Dawson and Hubball, 2014; Wigdahl et al., 2014; Aldrich, 2015; Heileman et al., 2018; Hilliger et al., 2020).
One of the most common approaches for the study of curricular structures and student paths to graduation has been the application of graph theory-based analytical frameworks, which in the field of Learning Analytics is generally referred to as Curriculum Analytics (CA) (Dawson and Hubball, 2014). In Aldrich (2015), the structure is revealed through an analysis of the Curriculum Prerequisite Network (CPN) as a Directed Acyclical Graph (DAG). In this approach, courses are represented as nodes and prerequisite relationships define the graph’s edges. The goal is to represent information flows and highlight the interconnections within and between courses across various disciplines, with specific courses acting as information bridges or conduits connecting knowledge domains. In Aldrich’s case study, interconnected courses were a common feature in STEM disciplines and were especially observed in mathematics and chemistry (Aldrich, 2015, p. 175). Courses in these two STEM areas were also characterized by the longest disciplinary depth (i.e., course prerequisite chains that build on each other). Based on Aldrich (2015), long (versus short) course chains are most likely affected by information loss, and hence are problematic for knowledge retention. Course chains are also affected by the impact that gatekeeping courses have in hampering students’ progressions, acting and contributing to the tracking of students away from STEM career pathways, as discussed above.
Heileman et al. (2018, see also Wigdahl et al., 2014) analyze the degree plans as DAG, developing metrics that highlight intrinsic structural attributes of a curriculum by quantifying overall rigidity as well as other elements such as course-related constraints on the student flow through the requirements. Similar to Aldrich, the approach taken in this case is built on established rules that define what courses determine a curriculum of study and how they are interconnected in prerequisite chains. In the words of Heileman et al. (2018, p. 2), they modeled the curriculum by adopting a “reductionist approach to the study of student success, akin to how those in the natural sciences often explain biological phenomena in terms of the underlying chemistry, which in term might be explained more fundamentally using the laws of physics” (cf. Krist et al., 2019). Instead of interpreting curricula graphs in a reductionist way, we interpret these structures as the product of socio-cultural constructions that result in features that are structuring the processes that shape an academic community.
Some of the limitations recognized in the approaches above, and discussed by Heileman et al. (2018), have been addressed by building analytics that rely on the students’ actual enrollments (e.g., Dawson and Hubball, 2014; Aiken et al., 2019) or adopting a system-level perspective on curriculum analytics and development (Hilliger et al., 2020). Dawson and Hubball (2014) apply Social Network Analysis to student enrollments to reveal dominant underlying curricular structures and student pathways. By developing an interactive tool able to customize the data visualization to show demographic and other characteristics of students and programs, they showed the potential of this approach to inform quality assurance and connect curricular paths to student employability (Dawson and Hubball, 2014).
Hilliger et al. (2020) propose an approach to CA based on an Integrative Learning Design (ILD) framework that involves phases of continuous improvement and evaluation, and conceive of CA as a system of stakeholders, technology and knowledge. Their work overcomes the limitations inherent with graph-based characterizations of curriculum structure and its characteristics, and the associated risk of reducing the curriculum to its basic course relationship elements. In this view of CA as a process (Hilliger et al., 2020), faculty, administrators, and students are involved in facilitating the production and evaluation of actionable knowledge. When the curriculum is conceptualized in this way, then its structure should be understood as emerging from a network of ‘actants’ (following Latour, 1996), meaning, not just a set of nodes (courses) connected by edges (requirements, prerequisite relationships, and enrollment sequences) that result in a set of attributes (path length and centrality), but instead as a network of relationships that include the actors producing and experiencing them.
In this paper, we represent students flows through curriculum structures using two analytical elements of the Process Mining methodology: (1) process maps, (2) the traces students leave while interacting with course requirements (Trčka and Pechenizkiy, 2009; Cairns et al., 2015; Schulte et al., 2017; Bogarín et al., 2018; Caballero-Hernández et al., 2018; Janssenswillen et al., 2019; Salazar-Fernandez et al., 2021). These representations ought to be interpreted as lived experiences of each individual student in interaction with others (as intersubjective selves, Fuchs, 2021). The students are transformed by experiencing a system of knowledge, culture, capital, and people (faculty, students, staff) that are actants in the socio-educational environment, constituting a network of actants interconnected and interacting (Latour, 1996) in a field of practice.
3. Materials and methods
3.1. Data
This study relies on institutional student records routinely collected by higher education institutions. These data consist of student enrollments, intended or declared major, degree received and student socioeconomic status, race/ethnicity, gender, and first- versus continuing-generation status. This administrative source of data affords the opportunity for the analysis presented here to be easily reproduced by other institutions in the U.S. higher education context.
Using institutional data, we approximate dimensions of student diversity by adopting the approach outlined by Castle et al. (2021), who built on critical race and intersectional theory to highlight commonalities in how students with different intersectional identities experience the educational context. In particular, students with different identities were found to have to contend with a delivery of educational resources and a social context that is potentially disadvantageous. The methodology relied on defining the Systemic Advantage Index (SAI), an additive representation of advantages potentially experienced by students due to their race/ethnicity, gender, socioeconomic status, and first-generation status (Castle et al., 2021). Building on work by Wilder (2013) and Byrd (2021), among others, which discuss how the U.S. higher education context was historically developed to serve male, white, and upper-class students, the index assigns a score of four to continuing-generation students with these characteristics, and removes advantages to the individuals depending on differences in these elements. Hence, a student who is first-generation, low-income, female, and non-white experiences no systemic advantages according to this index, and a student who is first-generation, low-income, male, and non-white has an SAI of one.
3.2. Institutional context
Two institutions participated in this study. Both are located in the Great Lakes region and are research-intensive (R1) public universities with undergraduate enrollments around 31,000 students. One of these universities has an acceptance rate of 80% and a four-year graduation rate of 79% (institution A) and the other has an acceptance rate of about 26% and a four-year graduation rate of 92% (institution B). Both universities enroll low numbers of students from historically marginalized racial/ethnic backgrounds (Hispanic and Black students together represent 8 and 4% of the undergraduate student body, respectively). In addition, approximately 18.5% of the students enrolled at these universities receive Pell grants (grants intended for low-income students) (U.S. Department of Education College Scorecard, 2023). First generation students are approximately 10% of the undergraduate degree seeking students at institution A and 16% at institution B.
Here, we focus on students who intended to graduate with an undergraduate biology degree. This intention is derived from the declared major of the student, when available, or inferred by the student enrolling in a key introductory course required by biology-related degree programs. Furthermore, we limit the population under study to first-year, non-transfer students. The population is further limited to those who started at the institutions in the Fall term cohorts between 2012 and 2016 (inclusive). This time frame guarantees that students can be followed for at least 6 years to establish whether they earn a degree in biology. Data was right censored, that is, limited to 6 years plus one term so that outcomes and paths for students were observed within the same time window, and certification for degrees granted by the end of the academic year was complete (see Supplementary Table S1 for descriptive statistics of the final populations at institution A and B). The final status of the students were classified as: earned a biology degree (Biology D), earned a degree in STEM (defined by the NSF LSAMP program’s list of majors; National Science Foundation – Louis Stokes Alliance for Minority Participation, 2023) but not biology (STEM D), earned a degree in a non-STEM field (Other D), or left the institution without a degree or is still enrolled after 6 years (Left-Enrl). By the sixth year, the majority of students tend to have graduated or left an institution.
Pathways to majors in biology vary among the two institutions we examined. Where possible, we standardize common structural elements of the pathways for comparison purposes, excluding the representation of other ways of fulfilling requirements, like using tests, advanced placement or transfer credits. We acknowledge the importance that differences among the institutions have on the experience of individual students, as well as the fact that each institution has unique aspects of the structures that students must navigate. Nevertheless, our focus is on the relationship between structures pervasive in the education system and students sharing social and economic characteristics.
The courses investigated in this study include the biology and chemistry courses that are required by the different biology degrees at each institution and which are suggested to be taken by the end of the second academic year. We also included preparatory courses, not counting as degree requirements, as they impact students’ opportunities to follow the canonical disciplinary path. We separately analyze course-taking patterns for biology courses and chemistry courses. The analysis of chemistry course pathways complement the analysis of the biology sequences because it highlights the impact that service courses have on other disciplines within STEM.
At institution A we focused our analysis on the eight main undergraduate degrees offered in biology: the Bachelor of Arts (BA) and Bachelor of Science (BS) in Biology, Microbiology, Biotechnology, and Human Biology. All degrees are offered in the same college, and all name introductory chemistry and/or biology courses among their requirements. The introductory Chemistry sequence consists of General Chemistry (GC), Organic Chemistry I (OC I), and Organic Chemistry II (OC II), each of which is delivered in large lecture format. Separately graded laboratory courses are associated with the lectures. Students have to pass a test in order to enroll in General Chemistry. If the requirements are not met, the student is asked to enroll in a pre-Chemistry course (PGC). The biology sequence includes Biology I (BIO I), Biology II (BIO II), which can be taken as a sequence or off sequence and is also associated with an introductory Biology lab, and Biology III (BIO III) with prerequisites being Biology II and General Chemistry. Similarly to the other institution in this study, the courses are not exclusive for biology students. Intending biology majors are required to engage with the chemistry requirements in their second or third term. Students at this institution declare a major when they enroll for the first time, and have the option of changing their major at any time in their career. For the purpose of this study, students are defined as intending a degree in biology if they selected this intent in their first term.
Institution B offers a diverse set of 18 undergraduate four-year degrees that we consider herein broadly as biology-related degrees, and that were available to these cohorts. The degrees most frequently earned by students are in Neuroscience, Biology, Cell and Molecular Biology, Biomedical Engineering, and Biophysics, Cognition & Neuroscience. All but the engineering degrees are offered in the same college and all name introductory chemistry and/or biology courses among their requirements, and most are Bachelor of Science degrees, although Bachelor of Arts are also included. Those courses comprise part of a sequence, where enrollment in the next course requires successful completion of the previous course. The introductory Chemistry sequence comprises General Chemistry (GC), Organic Chemistry I (OC I), and Organic Chemistry II (OC II). Each is a large lecture with an associated but separately graded laboratory course. The Biology sequence includes Biology I (BIO I), Biology II (BIO II), and a Biology Lab (BIO L), as with Chemistry. While intending biology majors may enroll in these courses, they are not exclusive to biology students. For instance, General Chemistry has an especially broad reach that includes a large population of engineering students. At institution B, students’ intent to major in biology is not immediately known. They are admitted as first-year students to a college or school, and often by the end of their second year they declare a major. However, this is not the rule. Without surveying students directly, we often can only estimate their intention until they formally declare. Therefore, at institution B, enrollment in the first of one of these sequences is taken as “intent” for the purposes of this study, with the understanding that this practice creates a large false positive rate. However, our focus on the pervasive impact of structures in affecting students’ experiences of the curriculum reduces the influence of this latter aspect on the outcomes of the analysis.
3.3. Educational process mining – path mapping and trace exploration
Process Mining methodologies in education (Educational Process Mining, EPM) have found increasing popularity and application in Educational Data Mining (EDM) (see Bogarín et al., 2018 for a review of EPM). This popularity is due to the pervasive use of information technology and the record that it generates in the process. EPM, building on event logs generated in the course of computer-supported instruction, administration and record-keeping, is a process-centric approach that aims at making explicit patterns and relationships existing between events linked to activities (Bogarín et al., 2018, p. 1). Hence, EPM can be applied to a variety of educational contexts and activities; for example, using data from learning management systems to explore quiz taking behavior (Juhaňák et al., 2019) or self-regulated learning (Cerezo et al., 2020). We limit the scope of this work to the application of what Bogarín et al. (2018, p. 4) call process discovery, that is, the representation of the “process model able to reproduce the behavior seen in the log file.” Here, we also base our analysis on a qualitative form of conformance checking, where discrepancies between expected curricular progressions and actual student progression, if observed, reflect the active process of disciplinary acculturation (Reinholz et al., 2019) and student tracking (Tyson, 2011; Lewis and Diamond, 2015; Bottia et al., 2021; Stich, 2021) described previously.
This approach is in line with earlier applications of EPM by Trčka and Pechenizkiy (2009) based on domain knowledge (i.e., domain-driven EPM), where pattern discovery is replaced in the early phase of the analysis by a predefined template that reflects expert knowledge or the established rules the investigated process should follow; in the words of Trčka and Pechenizkiy (2009, p. 1115), establishing “a precise and unambiguous semantics to the study rules.” Once this is established, analysis can focus on verifying conformance with the rules, helping students audit progression, identify efficient paths (e.g., Caballero-Hernández et al., 2018), or see violations of the rules.
Applications of EPM to curriculum analytics have shown the potential of this approach, and acknowledged the challenges that the complexity of educational systems and the variety of student behavior introduce to the analysis (see, e.g., Trčka and Pechenizkiy, 2009; Cairns et al., 2015; Schulte et al., 2017). Focus on identifying system-level inefficiencies and optimizations to enhance students flow through the curriculum tends to adopt what Heileman et al. (2018) acknowledged as being a reductionist approach to students’ success. The introduction of a domain-driven approach, as suggested by Trčka and Pechenizkiy (2009), or casting the EPM analysis within retention theory literature as in Salazar-Fernandez et al. (2021) can overcome the theoretical limitations of a reductionist approach to curriculum analytics.
We build on an understanding of institutions of higher education as social educational systems to provide our theoretical framework and guide our application of EPM. The domain-rules structure the maintenance and reproduction of the disciplinary domain (Reinholz et al., 2019) to maintain the system as is (Wilder, 2013; Ray, 2019; Byrd, 2021; Pearson et al., 2022). EPM allows us to reveal whether such structures tend to work to the advantage of certain groups and to the detriment of others, as discussed by Webb et al. (2017) among others.
To respond to RQ1 we rely on EPM’s process mapping to represent students course taking and transitions from cohort to outcomes. Once the requirements of the curriculum are defined in terms of the biology and chemistry lower-division courses and the order in which they have to be taken, that is, defining the process using domain knowledge, we should expect that the majority of transitions follow the established rules. Divergence from the required curricular path would be indication of experienced advantage, that is, in cases where students skip a required course, or disadvantage, that is, in cases where students leave the curricular path and do not earn a biology-related degree. To ease representation, we limit the representations of students’ paths to the most common accounting for 60% of students taking at least a course in the biology sequence or chemistry sequence, respectively. This threshold ensures that more than half of the population is included in the sample.
To address RQ2, we focus on the top traces representing 50% of the students at each institution by Systemic Advantage Index (SAI). In particular, we compare the traces of white, male, continuing-generation and higher income students (SAI = 4), with students who are non-white, female, first-generation, and low income (SAI = 0) or have an advantage in any of these characteristics (SAI = 1).
Process mining, as process maps and trace explorations, is performed using the Business Process Analytics toolkit BupaR (Janssenswillen et al., 2019). Conformance checking consists of a qualitative analysis highlighting discrepancies in how the curriculum is experienced by different students via the traces they leave in the pursuit of their academic aspirations. The focus is on observable differences in ways that students do (or do not) transition through the required curriculum while engaging in the acculturation process that builds and transforms the knowledge and capital required to properly perform in the disciplinary field of practice.
If the curriculum was neutral to the intersectional identities of the students and if it were not a selective active agent of transformation that advantages some and disadvantages others, then different students (i.e., of different demographics and life pathways) would progress through it in the same way.
4. Results
4.1. Research question 1: is the intended progression through the curriculum the one most commonly experienced by the students?
In Figures 1, 2 we show example process maps for students majoring in biology at the two participating institutions. Figure 1 represents the curricular paths in the core biology sequence required of all biology majors at each institution and Figure 2 shows the curricular paths in the core chemistry sequences in those majors. For clarity, we limit the maps to the top most frequent pathways, accounting for (i.e., covering, also referred to as coverage in EPM) 60% of the students and for students who had an enrollment in at least one of the courses in the path.
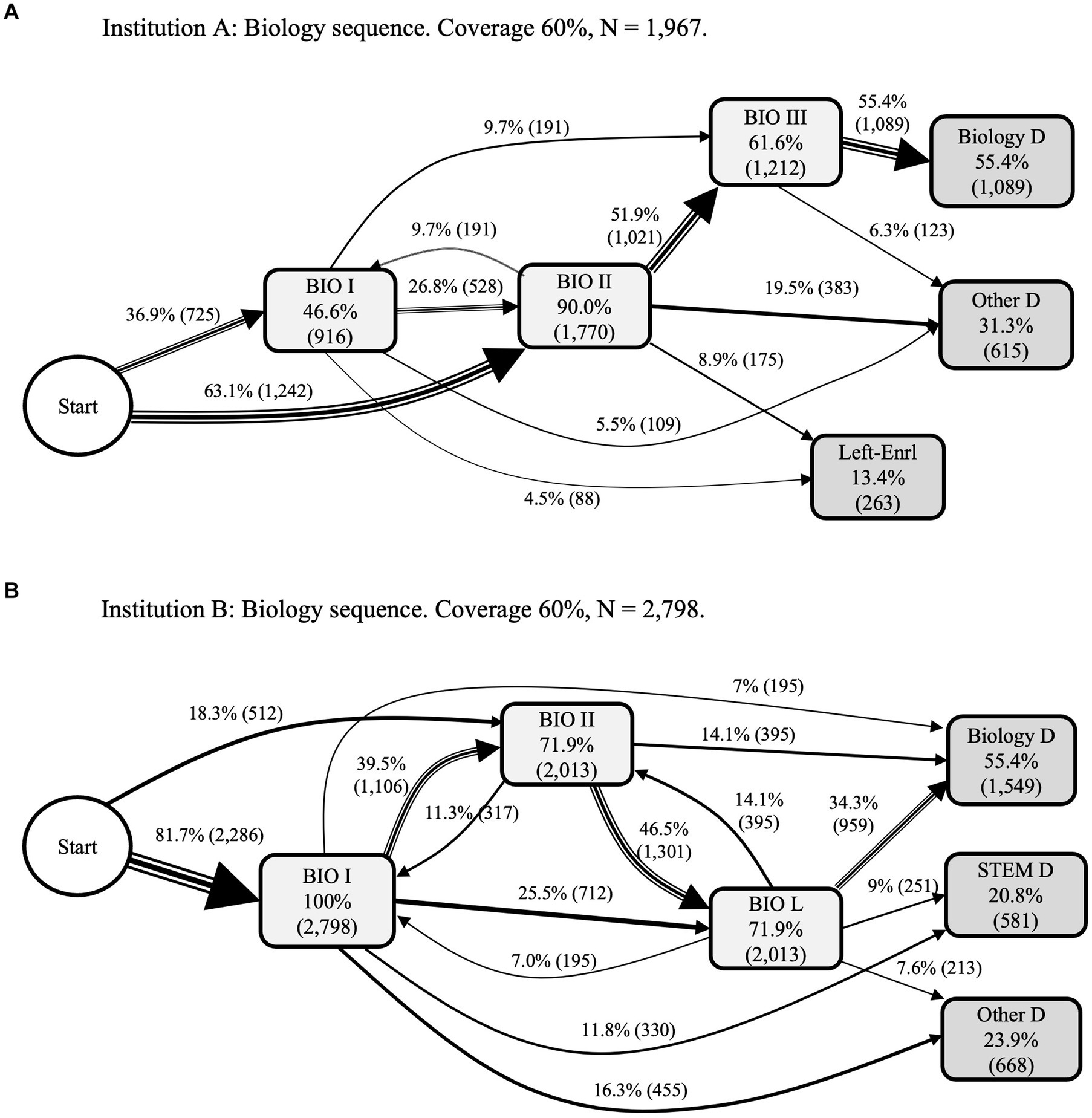
Figure 1. Process maps displaying course-taking in lower-level biology courses by students intending a biology degree in the first semester (institution A, A) or indicating an intention to pursue a biology degree (institution B, B). The pathways represent the topmost common paths accounting for 60% of the students who had at least one enrollment in any of the required courses. The coverage limitation allows for getting as many pathways represented without making the maps too complicated. The percentages represent the relative number of cases over the total sample per activity (i.e., course) and flow (i.e., arrows connecting the courses). For example, among the students in this sample at Institution A (N = 1,967), 916 (46.6%) enrolled in BIO I. Of these, 725 (36.5% of the total) had BIO I as their first enrollment, while 191 (9.7%) enrolled in BIO I after enrolling in BIO II. The end points of the paths represent students’ outcomes after 6 years from cohort (Biology D: students who earned a degree in biology; STEM D: students who earned a degree in STEM but not biology; Other D: students who earned a degree not in STEM; and Left-Enrl: students who by the sixth year had left without a degree or are still enrolled).
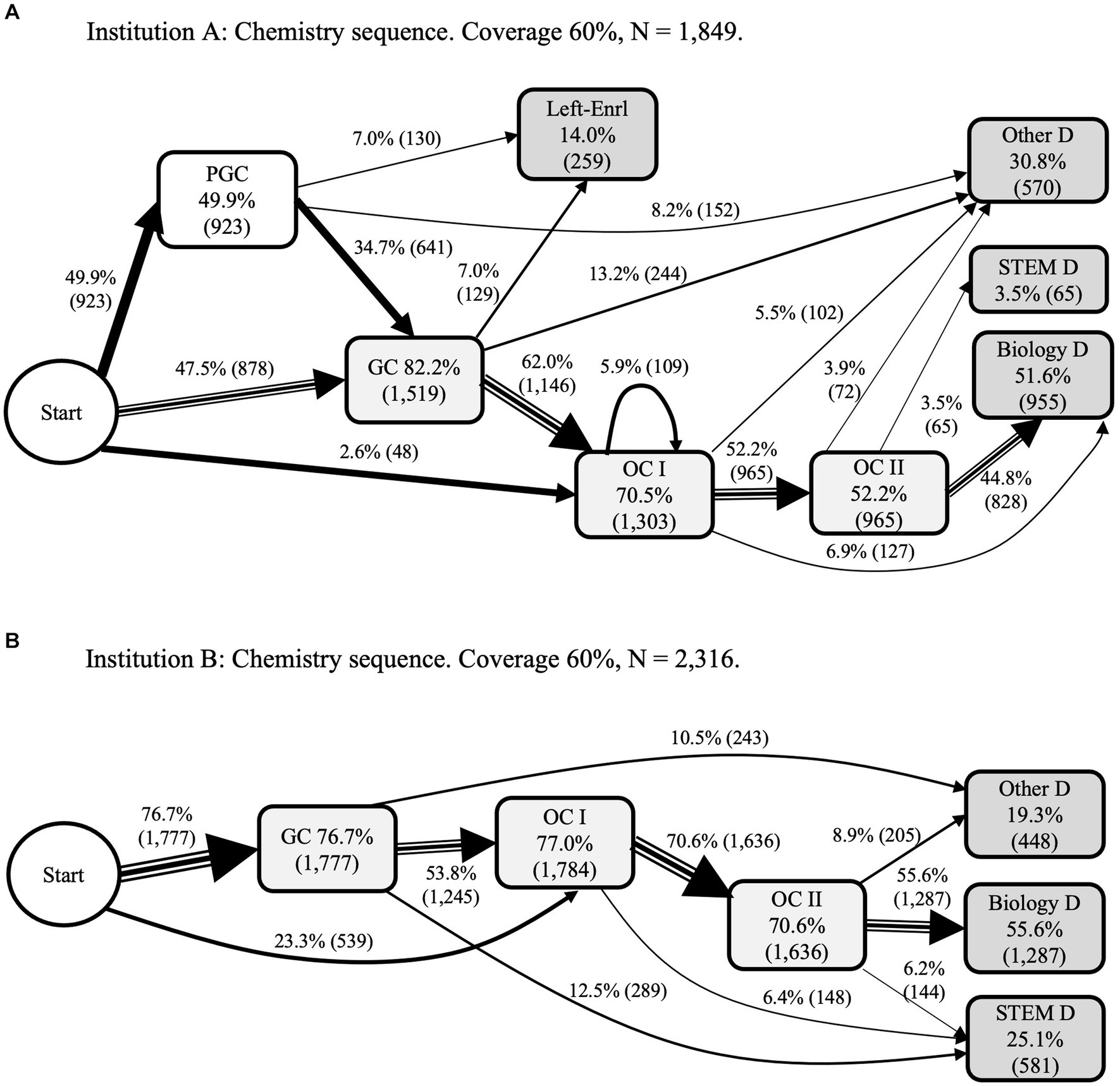
Figure 2. Process maps displaying course-taking in lower-level chemistry courses by students intending a biology degree in the first semester (institution A, A) or indicating an intention to pursue a biology degree (institution B, B). The pathways represent the topmost common paths accounting for 60% of the students who had at least one enrollment in any of the required courses. The percentages represent the relative number of cases per activity (i.e., course) and flow (i.e., arrows connecting the courses). For example, among the students in this sample at Institution A, there were 923 (49.9% of the sample) enrolled in the pre-Chemistry course (PGC). Of the 1,519 (82.2%) of students enrolling in general Chemistry (CG), 878 (47.5%) did that directly, while 641 (34.7%) enrolled in GC after enrolling in PGC. The end points of the paths represent students outcomes after 6 years from cohort (Biology D: students who earned a degree in biology; STEM D: students who earned a degree in STEM but not biology; Other D: students who earned a degree not in STEM; and Left-Enrl: students who by the sixth year had left without a degree or are still enrolled).
Most students transition through the curriculum as expected. For example, if we focus on Figure 1, institution A, we see how students fulfill lower level courses before enrolling in the last course in the lower division sequence and eventually moving on to earn a biology degree. The representation also highlights opportunities for skipping a course, for example via placement test or advanced placement credits. At institution A, the most common biology path skips the BIO I course and moves directly to the BIO II course, which is also the most common course by enrollments. Skipping the first biology course is less common for institution B. At institution A, BIO I and BIO II can be taken off sequence; Figure 1 top panel reveals this choice as well, although it is less common for students to follow BIO II with BIO I. Throughout the progression from Start to the final status, students leave the path leading to a biology degree (Biology D) after enrollments in any of the lower-division courses. When they leave, they either leave the institution or delay the degree completion (Left-Enrl) or opt for a non-STEM degree (Other D). Students at institution B, the more selective of the two institutions, manage to maintain a path to a STEM degree at higher rates than those at institution A.
Figure 2 shows similar patterns for students while they are engaging with the introductory chemistry sequence. Pathways for institution A reveal the importance that the required enrollment in general chemistry (GC) has in affecting students’ progression to the degree. Here, the majority of students experience their first encounter with chemistry in a preparatory course (PGC), affecting the progression to GC and potentially the enrollment in BIO III, for which GC is a prerequisite, delaying the student for at least one semester. For institution A, both the preparatory and the general chemistry course are a significant roadblock to earning a biology or a STEM degree, evidenced by the outflow of students toward the Lef-Enrl and Other D nodes associated with these two courses. The more selective institution B appears to maintain greater retention on the path to a biology degree or a STEM degree, and overall to degree completion.
These results confirm the presence of what has been referred to in the literature as the ‘leaking pipeline’ of STEM education (following a common metaphor for STEM careers, see, e.g., Maltese et al., 2014) and referred as tracking (Oakes, 1985; Tyson, 2011; Lewis and Diamond, 2015; Mensah and Jackson, 2018; Bottia et al., 2021, p. 618; Stich, 2021). The pattern is also expected considering neo-structuralist and constructivist understandings of higher education, because the curriculum structure acts as a selecting and homogenizing agent of acculturation into biology, disciplining the students to perform the knowledge and norms of behavior expected of biologists (Reinholz et al., 2019). Once the individual does not conform or fit within the expected cultural system, they opt for an alternative path.
In the next section we analyze whether progressing through an academic curriculum and the passive and active structures associated with that process result in students’ minoritization.
4.2. Research question 2: does the maintenance of coherence and socialization into the discipline act in a similar way on individuals of different socio, economic and demographic backgrounds?
Addressing RQ1 allowed us to establish that the curriculum acts on students intending to earn a degree in biology by requiring the acquisition of knowledge contained in milestone courses. This curriculum pathway also acts by shaping the community. The diversity of pathways we observed above could just represent different modalities of disciplinary participation or acculturation, for example, different students who start with a preparatory course, or who retake a course, maintain the same chances and opportunities to build the knowledge and capital that the disciplinary community sets as the standard for belonging.
EPM allows us to explore and represent the paths students leave/follow on their progression to the degree (traces). In Figure 3 we compare the topmost common traces followed by at least 50% of the students with a Systemic Advantage Index (SAI) of 4 (top panel) versus the combination of students with SAI of 0 or 1 (bottom panel) for institution B. The figure represents the decreasing frequency of importance of the course sequences followed by students. For both groups of students, the required biology sequence is experienced by the highest share of students at a rate of ~15% and ~ 12% for an SAI of 4 and of 0 or 1, respectively (row of Order 1 in top and bottom panels). If we combine rows 1, 3 and 5 on the top panel (comprising the allowed variations in order for completion for the core), the rate increases to 33%. In comparison, the combination of the comparable traces from SAI 0.1 results in a 24.3% rate, a rate 8.7% lower than for SAI 4 group (rows of Order 1, 5, and 6 in the lower panel). Of the traces characterizing students with an SAI of 4, all lead to a degree. Specifically, the majority lead to a biology degree (33%), and only 10.1% lead to a non-STEM degree. In contrast, on the traces characterizing students with an SAI of 0 or 1 we do see common traces leading to a non-STEM degree for a total rate of 18.6% (rows 2 and 3, lower panel). We want to highlight the 3rd trace on the SAI 0/1 group. In all other cases, students in either group switched to a non-STEM degree after taking the first Biology course. Yet the group in the 3rd trace attempted to complete the whole series before making the change. These students represent a lost opportunity. They obviously had the interest and commitment to make it through a full year of biology before changing majors. This pattern is not observed in SAI = 4.
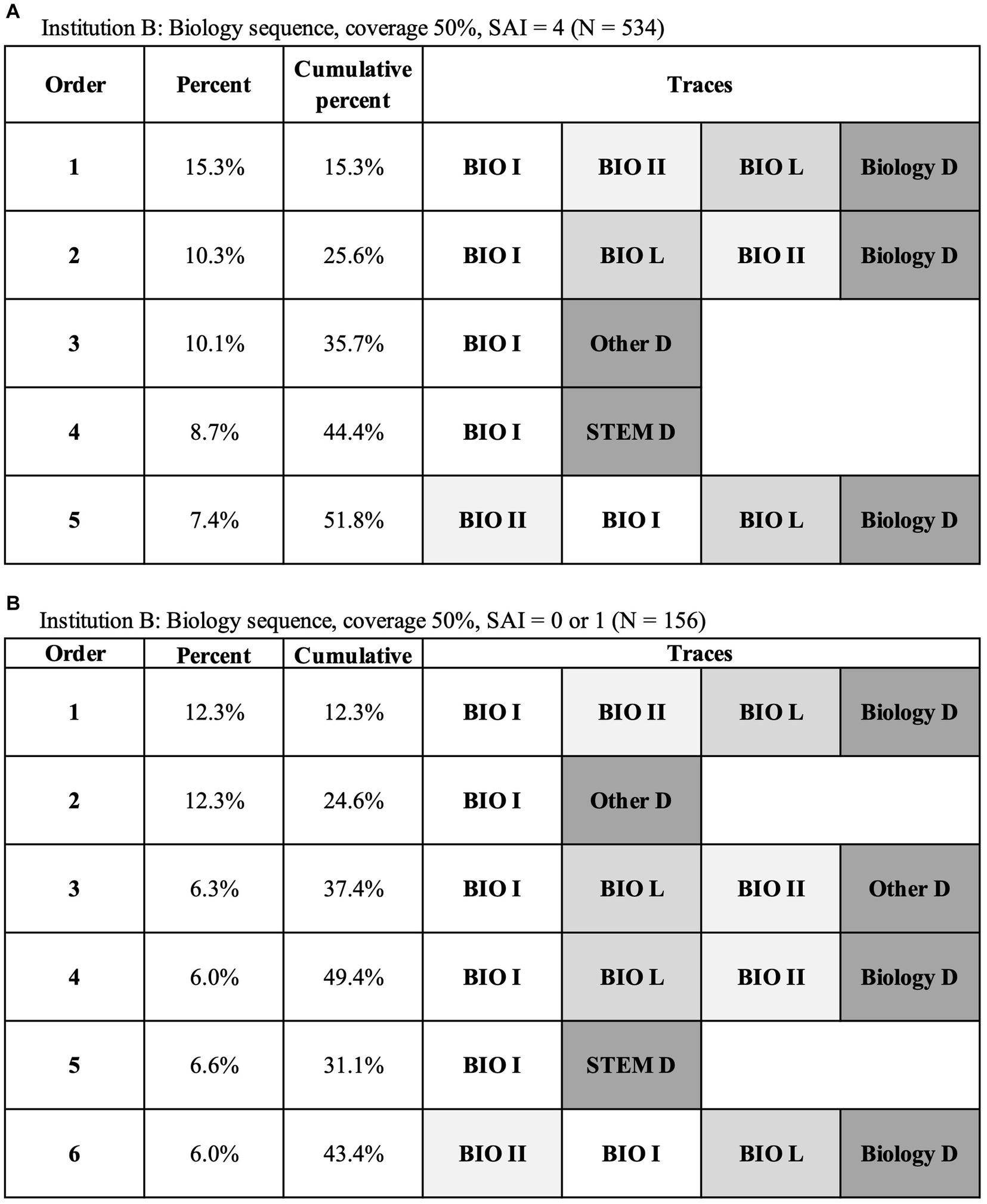
Figure 3. Top traces for biology courses at institution B, the more selective institution among the two compared here. Traces represent the sequence of courses taken by students and outcome after 6 years. The figure represents in decreasing order the most common traces and accounts for at least 50% of the students by group. Traces on the top (A) and bottom (B) are those from students with an SAI of 4 or of 0 or 1, respectively. Percentage represents the rate of students by group who experienced the trace.
Figure 4 represents paths taken by students at institution A when trying to fulfill the required core lower-division biology courses. At this institution the top 3 most common biology traces for students with an SAI of 4 lead to a biology degree and account for 40% of the students. For the SAI 0/1 group, only 22.3% of students followed top traces that led them to a biology degree. Both groups presented instances of students who had not arrived at any major (left or still enrolled). This comprised 4.8% for SAI-4 (row 5, top panel) and 8.5% for SAI-0/1 (row 3, lower panel). This pattern had not been observed in institution B. Also, in contrast with institution B none of the students in the 50% majority switched to a different STEM degree.
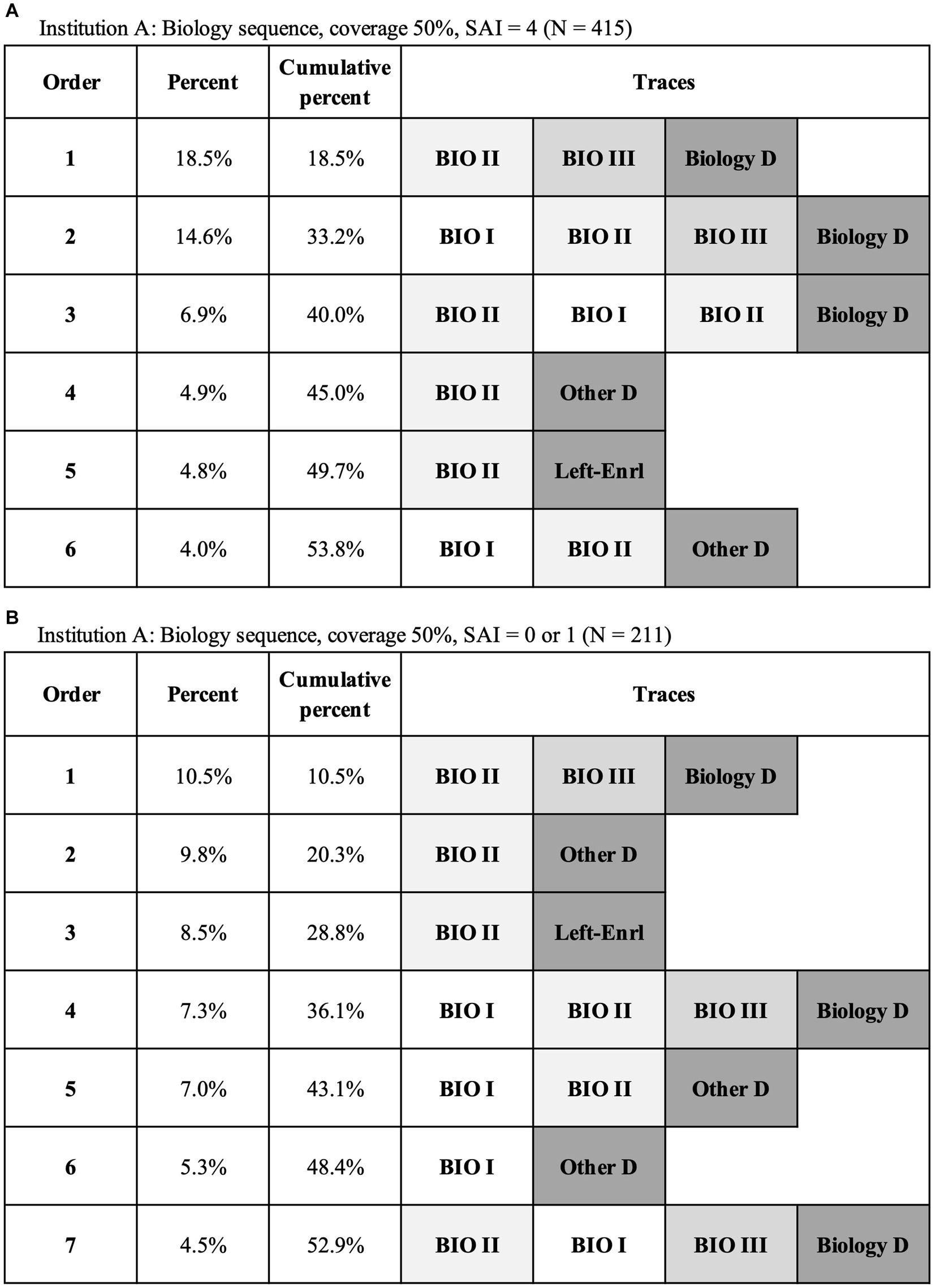
Figure 4. Top traces for biology courses at institution A, the more selective institution among the two compared here. Traces represent the sequence of courses taken by students and outcome after 6 years. The figure represents in decreasing order the most common traces and accounts for at least 50% of the students by group. Traces on the top (A) and bottom (B) are those from students with an SAI of 4 or of 0 or 1, respectively. Percentage represents the rate of students by group who experienced the trace.
Figure 5 represents the traces representing the courses taken by students following the chemistry course sequence at institution A. The standard progression from general chemistry to a biology degree characterizes the experience of 20.4% of students with an SAI of 4 against 8.2% of the students with an SAI of 0 or 1 (see top trace in both panels). Moreover, the alternative required option, that is, when a student has to take a preparatory course and eventually earn a biology degree, is the second most common for students with an SAI of 4 (10.3% of the students in this group) and only the fifth most common pathway for an SAI of 0 or 1 (fifth trace from the top). The curriculum structure in place precludes students in the latter group from progressing to a biology degree as it is indicated by the second to fourth most common traces followed by these students (rows of order 2 to 4, lower panel), that is, starting with a preparatory course and ending with a non-STEM degree (rows 2 and 4, lower panel) or having left or still being enrolled after 6 years (rows 3, lower panel). While no re-enrollments were seen for students with SAI of 4, 5 traces showed re-enrollments for SAI of 0 or 1 (rows 7, 8, 10, 13, lower panel). Barriers at institution A act on students with an SAI of 0 or 1 despite their efforts to persist on the degree path, indicated by the abundance of traces characterized by re-enrollments.
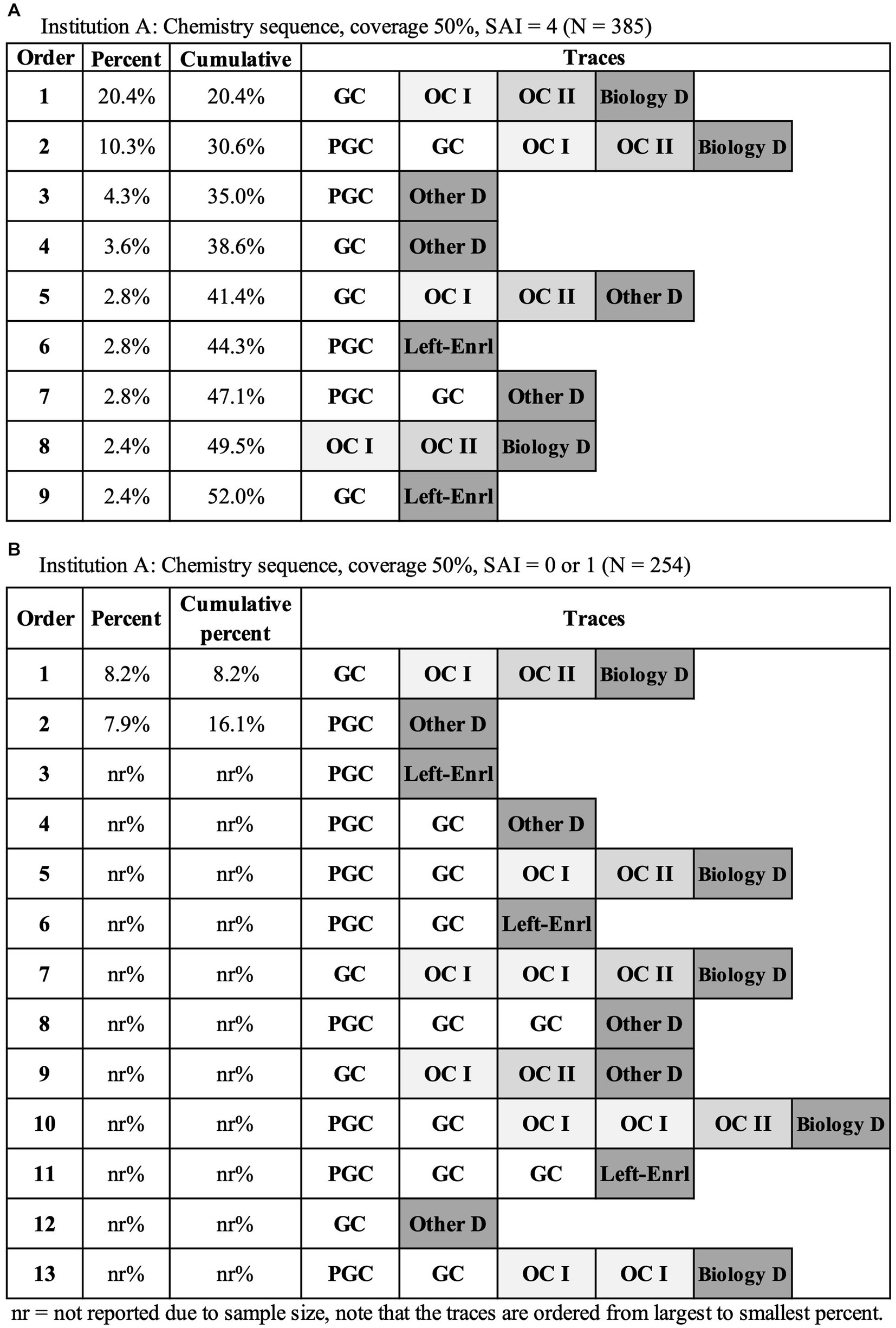
Figure 5. Top traces for chemistry courses at institution A, the more selective institution among the two compared here. Traces represent the sequence of courses taken by students and outcome after 6 years. The figure represents in decreasing order the most common traces and accounts for at least 50% of the students by group. Traces on the top (A) and bottom (B) are those from students with an SAI of 4 or of 0 or 1, respectively. Percentage represents the rate of students by group who experienced the trace. Due to sample size, percentages for traces that would have reveal a size below 10 students are not reported.
Figure 6 represents the traces representing the courses taken by students following the chemistry course sequence at institution B. Although more selective, some of the patterns experienced by students at institutions A, are present at institution B. The most common traces for students with a SAI of 4 lead to either a biology degree or a STEM degree. This is not the case for students with a SAI of 0/1. In this group common outcomes lead to leaving a biology or STEM degree (row 2 and 3) (see Supplementary Figures 1–4 for separate traces for SAI of 3, 2, 1 or 0).
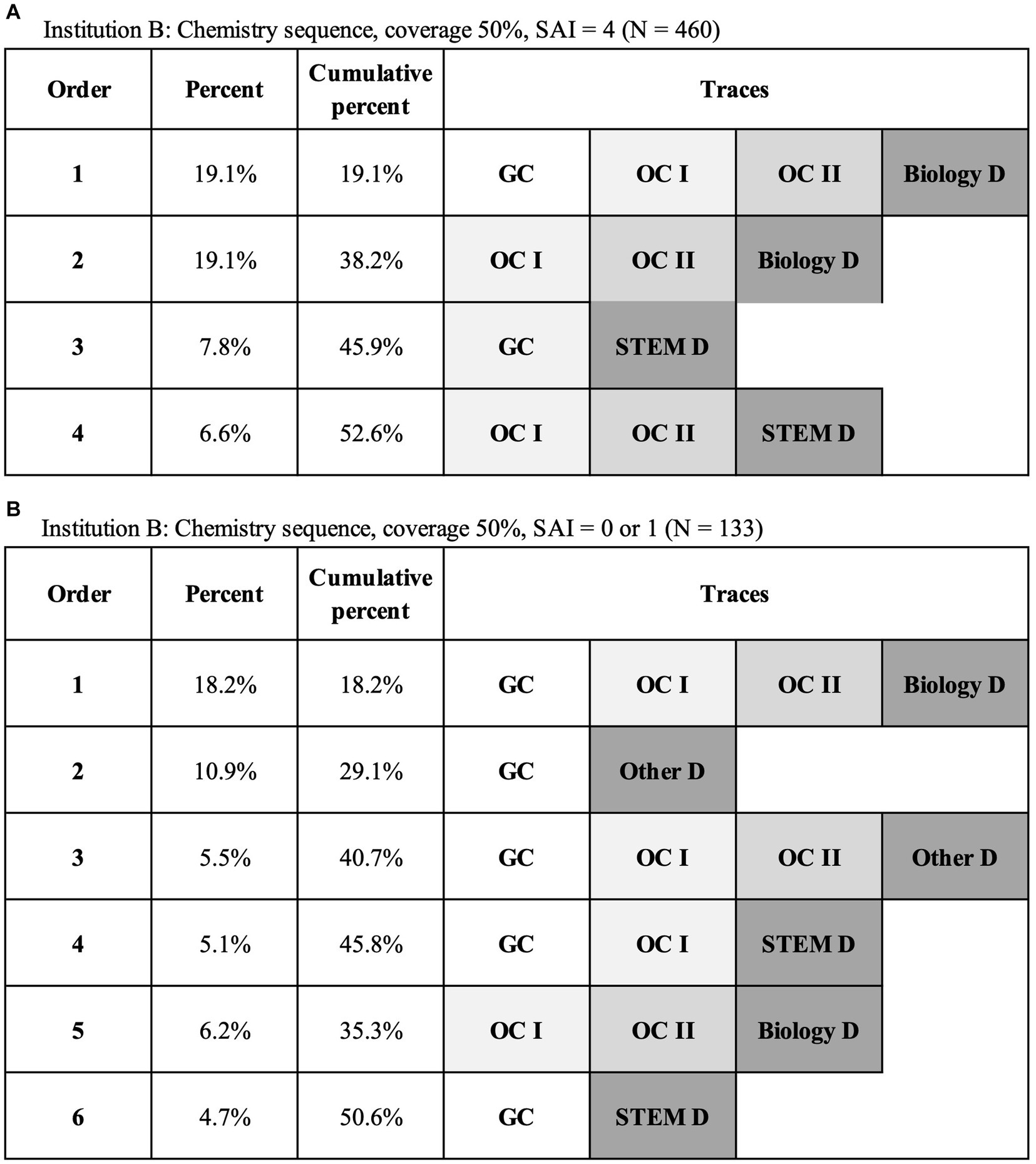
Figure 6. Top traces for chemistry courses at institution B, the more selective institution among the two compared here. Traces represent the sequence of courses taken by students and outcome after 6 years. The figure represents in decreasing order the most common traces and accounts for 50% of the students by group. Traces on the top (A) and bottom (B) are those from students with an SAI of 4 or of 0 or 1, respectively. Percentage represents the rate of students by group who experienced the trace.
These trace explorations indicate that at the two institutions in this study, the students with a SAI of 0 or 1 who engaged in the process of acculturation into biology were minoritized. This result follows what we would expect based on current and past analysis of the role and effect of higher education in society; that is, students with historically marginalized race, economic or demographic backgrounds tend to be negatively affected by the curriculum structure in place, and eventually end up ‘socially constructed’ out of certain academic disciplines (to follow Benitez, 2010, but also see Webb et al., 2017).
5. Discussion
Curricular structures for STEM degree programs in higher education, that is, the ordered sequencing of courses and pace for progression to a degree, are often portrayed as a neutral phenomenon in the curriculum analytics literature. Each student who is admitted for an undergraduate degree should have an equal opportunity to navigate through disciplinary coursework. The results from this study indicate that a majority of students experience biology and chemistry undergraduate curricula in different ways from their degree requirements. The findings also reveal that students across social positions navigate the biology and chemistry curricular structures in different ways, compounding prior results showing significant differences between groups of students in their awareness of different major paths (Baker and Orona, 2020). Our results suggest that these differences contribute to structural inequities in who can obtain a STEM undergraduate degree.
When attempts are made to condense the course enrollment path followed by students in pursuit of a degree, what emerges is that each institutional landscape is associated with different choices and outcomes across students—different paths are required to reach the same destination, that is, a degree in the chosen subject. This diversity of paths is inevitably associated with a diversity of lived student experiences, affecting the way students acquire disciplinary knowledge and acculturate into a disciplinary field.
Importantly, the traditional default curricula do not seem to be working for everyone, including students from traditionally privileged backgrounds, as in both these two cases less than 50% of these students followed the expected path. Various reasons can be brought forth to explain this. For example, disciplinary or departmental culture and practice might yield introductory and lower-division courses that are perceived as selective spaces where ‘weed out’ practices are accepted or unaddressed (Weston et al., 2019) to the detriment of enhancing belonging and inclusion, and reinforcing minoritization in the discipline (Seymour et al., 2019; Leyva et al., 2021b; McGee, 2021). Or lower-division courses might be centered on theory and foundational knowledge, reserving applications and practical relevance of the disciplinary knowledge to upper-division courses, alienating students who place more value on these latter aspects of a disciplinary experience. Certainly, the common practice of giving low grades in introductory STEM courses is a contributing factor (Seymour and Hunter, 2019).
Curricula are particularly not working for those who belong to minoritized backgrounds confirming the presence of structural inequities in our education systems (Webb et al., 2017; Mensah and Jackson, 2018; Bottia et al., 2021; O’Hara, 2022), more extensively discussed in the introduction and theoretical sections. Inequities might emerge from the required pace and order set by the course sequence. The content of the courses still tend to be centered on the dominant group, affecting an individual’s sense of belonging or overlooking social and cultural assets that were not traditionally the norm for an academic discipline or higher education more broadly (Yosso, 2005; O’Shea, 2016). Lack of access to peers or advisers who experienced minoritization themselves, makes it harder to navigate the social educational context and acquire or contribute to shaping the dominant social norms of a field (Park et al., 2020). Exclusionary practices (e.g., less support, discouragement, discrimination) from peers and faculty reinforce feelings of estrangement among minoritized students (McCoy et al., 2017; Park et al., 2020; De Grandi et al., 2021). Racial and gendered stereotypes in STEM provides additional social and cognitive burdens on women and students of color, as we stated above, requiring these students the onus to prove themselves vis a vis peers and faculty and affecting their ability to belong (Strayhorn, 2011; Seymour et al., 2019; Leyva et al., 2021b; McGee, 2021). It is likely that the processes and structures that shape the STEM curricula similarly affect non-STEM educational contexts due to historical and present-day exclusion in higher education institutions. Their adverse impact on students of minoritized backgrounds will be likely mediated by the historical roots and cultural trajectory of each academic discipline and its relationship to the surrounding social context. Future research should expand evidence for non-STEM majors with our proposed approach.
The structural organization of human activities has important implications for people’s livelihoods, determining experiences and opportunities. This is true for different areas of our life and has been demonstrated by work applying a critical race theory approach in different contexts and in the educational context in particular (Webb et al., 2017; Seymour and Hunter, 2019; McGee, 2020; Blair-Loy and Cech, 2022), showing how structures maintain, consolidate, and reinforce power relations resulting in and exacerbating inequalities in society.
Structures shape and are learned already in the earlier phases of our life, affecting how we experience, relate and respond to the social context surrounding us (Fuchs, 2021). There is evidence that the family and especially the community context experienced in childhood impacts an individual’s social and cultural capital and affects their opportunities in adulthood (Chetty et al., 2020). The forms of capital learned throughout an individual’s development, affect students experiences and their ability to express their potential in the higher education context. The inability of the educational context to build and empower different forms of capital adversely affects students of different minoritized social, economic and demographic backgrounds. We concur with the calls in the literature for partnering with students, building on their diversity of experiences by adopting an asset-based approach where cultural capital(s) are not placed in hierarchical relationships (that is, where a way of doing or behaving in a social group supersedes other equally acceptable options) but instead are viewed as providing assets that support achieving individual’s goals and benefit the educational community at large (O’Shea, 2016; following Yosso, 2005; see also Mercer-Mapstone et al., 2017). This commitment requires an intentional restructuring of curricula to the benefit of all students, especially those from minoritized backgrounds.
Data availability statement
The data analyzed in this study is subject to the following licenses/restrictions: data is not publicly available and is FERPA protected and cannot be accessed by third parties. Requests to access these datasets should be directed to c2Zpb3JpbmlAaXUuZWR1.
Ethics statement
The studies involving human subjects data were approved by Indiana University – Bloomington and University of Michigan. The studies were conducted in accordance with the local legislation and institutional requirements. Written informed consent for participation was not required from the participants or the participants’ legal guardians/next of kin in accordance with the national legislation and institutional requirements.
Author contributions
MP, MV, RM, JL, HRL, BK, NT, SF, KD, NC, and WB: developed and framed research questions – originated idea for product and contributed significantly to framing the ideas in the product and wrote and presented the product – wrote or presented part of the product. MV, BK, SF, and KD: analyzed the data – generated and evaluated the models (conceptual, statistical, and mathematical), figures, tables, etc. that are included in the product, contributed to data analyses – provided comments, suggestions, and code for data analysis. NT, RM, and SF: coordinated project – contributed substantially to network level activities such as management of network data, recruiting and assisting of new sites, or finding funding for the project. MV, BK, SF, and KD: managed the data – organized, tailored, and collated project data from multiple original sources. All authors contributed to the article and approved the submitted version.
Funding
This work received support from the Sloan Equity and Inclusion in STEM Introductory Courses (SEISMIC) project (seismicproject.org) funded by the Alfred P. Sloan Foundation and participating institutions. SEISMIC is managed at the University of Michigan for the participating institutions which include Arizona State University, Indiana University, Michigan State University, Purdue University, University of California, Davis, University of California, Irvine, University of California, Santa Barbara, University of Michigan, University of Minnesota, and University of Pittsburgh.
Acknowledgments
We thank Ashley Atkinson for assistance, Tim McKay for bringing and supporting the community that collaborated for this manuscript, and the students, faculty, staff, and administrators at our institutions for providing organizational support, access, and insights related to the information used for this study. Finally, in the spirit of Data Feminism (D’Ignazio and Klein, 2020), we acknowledge the “many hands” – well beyond those recognized here – that have made this study possible.
Conflict of interest
The authors declare that the research was conducted in the absence of any commercial or financial relationships that could be construed as a potential conflict of interest.
Publisher’s note
All claims expressed in this article are solely those of the authors and do not necessarily represent those of their affiliated organizations, or those of the publisher, the editors and the reviewers. Any product that may be evaluated in this article, or claim that may be made by its manufacturer, is not guaranteed or endorsed by the publisher.
Supplementary material
The Supplementary material for this article can be found online at: https://www.frontiersin.org/articles/10.3389/feduc.2023.1176876/full#supplementary-material
References
Aiken, J. M., Henderson, R., and Caballero, M. D. (2019). Modeling student pathways in a physics bachelor’s degree program. Phys. Rev. Phys. Educ. Res. 15:010128. doi: 10.1103/PhysRevPhysEducRes.15.010128
Aldrich, P. R. (2015). The curriculum prerequisite network: Modeling the curriculum as a complex system. Biochem. Mol. Biol. Educ. 43, 168–180. doi: 10.1002/bmb.20861
Baker, R., and Orona, G. A. (2020). Gender and racial differences in awareness and consideration of curricular programs: exploring a multistage model of major choice. AERA Open 6:233285842093702. doi: 10.1177/2332858420937023
Bates, T. (1975). Gramsci and the theory of hegemony. J. Hist. Ideas 36, 351–366. doi: 10.2307/2708933
Benitez, M. Jr. (2010). “Resituating culture centers within a social justice framework” in Culture centers in higher education: perspectives on identity, theory, and practice. ed. L. D. Patton (New York), 119–134.
Blair-Loy, M., and Cech, E. A. (2022). Misconceiving merit: Paradoxes of excellence and devotion in academic science and engineering. Chicago, IL: University of Chicago Press
Bogarín, A., Cerezo, R., and Romero, C. (2018). A survey on educational process mining. WIREs Data Mining Knowledge Discovery 8:e1230. doi: 10.1002/widm.1230
Bottia, M. C., Mickelson, R. A., Jamil, C., Moniz, K., and Barry, L. (2021). Factors associated with college STEM participation of racially minoritized students: a synthesis of research. Rev. Educ. Res. 91, 614–648. doi: 10.3102/00346543211012751
Bowen, G. M. R. (1999). Trajectories of legitimate peripheral participation: ethnographic case studies of learning ecology. Doctoral dissertation, University of Victoria.
Byrd, W. C. (2021). Behind the diversity numbers: Achieving racial equity on campus. Boston: Harvard Education Press.
Caballero-Hernández, J. A., Dodero, J. M., Ruiz-Rube, I., Palomo-Duarte, M., Argudo, J. F., and Domínguez-Jiménez, J. J. (2018). Discovering bottlenecks in a computer science degree through process mining techniques. In 2018 International Symposium on Computers in Education (SIIE)
Cairns, A. H., Gueni, B., Fhima, M., Cairns, A., David, S., and Khelifa, N. (2015). Process mining in the education domain. Int. J. Intell. Syst. 8, 219–232.
Carnevale, A. P., Schmidt, P., and Strohl, J. (2020). The merit myth: How our colleges favor the rich and divide America. New York: The New Press.
Castle, S. D., Byrd, W. C., Koester, B. P., Bonem, E. M., Caporale, N., Cwik, S., et al. (2021). Equity in the STEM landscape: a multi-institutional approach to mapping systemic advantages within STEM courses. American Educational Research Association (AERA) Annual Meeting, April 9–12, virtual.
Cerezo, R., Bogarín, A., Esteban, M., and Romero, C. (2020). Process mining for self-regulated learning assessment in e-learning. J. Comput. High. Educ. 32, 74–88. doi: 10.1007/s12528-019-09225-y
Cheesman, K., French, D., Cheesman, I., Swails, N., and Thomas, J. (2007). Is there any common curriculum for undergraduate biology majors in the 21st century? Bioscience 57, 516–522. doi: 10.1641/B570609
Chetty, R., Hendren, N., Jones, M. R., and Porter, S. R. (2020). Race and economic opportunity in the United States: an intergenerational perspective*. Q. J. Econ. 135, 711–783. doi: 10.1093/qje/qjz042
Dawson, S., and Hubball, H. (2014). Curriculum analytics: application of social network analysis for improving strategic curriculum decision-making in a research-intensive university. Teach Learn Inq 2, 59–74. doi: 10.2979/teachlearninqu.2.2.59
De Grandi, C., Smithline, Z. B., Reeves, P. M., Goetz, T. G., Barbour, N., Hairston, E., et al. (2021). STEM climate survey developed through student–faculty collaboration. Teach. High. Educ. 26, 65–80. doi: 10.1080/13562517.2019.1636219
Dixson, A. D., and Rousseau, C. K. (2005). And we are still not saved: critical race theory in education ten years later. Race Ethn. Educ. 8, 7–27. doi: 10.1080/1361332052000340971
Du, X.-Y. (2006). Gendered practices of constructing an engineering identity in a problem-based learning environment. Eur. J. Eng. Educ. 31, 35–42. doi: 10.1080/03043790500430185
Fischer, C., Witherspoon, E., Nguyen, H., Feng, Y., Fiorini, S., Vincent-Ruz, P., et al. (2023). Advanced placement course credit and undergraduate student success in gateway science courses. J. Res. Sci. Teach. 60, 304–329. doi: 10.1002/tea.21799
Fuchs, T. (2021). In defence of the human being: foundational questions of an embodied anthropology. Oxford: Oxford University Press.
Hales, K. G. (2020). Signaling inclusivity in undergraduate biology courses through deliberate framing of genetics topics relevant to gender identity, disability, and race. CBE Life Sci Educ 19:es2. doi: 10.1187/cbe.19-08-0156
Hatfield, N., Brown, N., and Topaz, C. M. (2022). Do introductory courses disproportionately drive minoritized students out of STEM pathways? PNAS Nexus 1:pgac167. doi: 10.1093/pnasnexus/pgac167
Heileman, G. L., Abdallah, C. T., Slim, A., and Hickman, M. (2018). Curricular analytics: a framework for quantifying the impact of curricular reforms and pedagogical innovations. arXiv preprint. arXiv:1811.09676.
Herzig, A. H. (2004). ‘Slaughtering this beautiful math’: graduate women choosing and leaving mathematics. Gend. Educ. 16, 379–395. doi: 10.1080/09540250042000251506
Hilliger, I., Aguirre, C., Miranda, C., Celis, S., and Pérez-Sanagustín, M. (2020). Design of a curriculum analytics tool to support continuous improvement processes in higher education. In Proceedings of the Tenth International Conference on Learning Analytics & Knowledge LAK’20. (New York, NY, USA: Association for Computing Machinery), 181–186.
Hirschl, N., and Smith, C. M. (2023). Advanced placement gatekeeping and racialized tracking. Sociol. Educ. 96, 190–210. doi: 10.1177/00380407231161334
Janssenswillen, G., Depaire, B., Swennen, M., Jans, M., and Vanhoof, K. (2019). bupaR: enabling reproducible business process analysis. Knowl.-Based Syst. 163, 927–930. doi: 10.1016/j.knosys.2018.10.018
Juhaňák, L., Zounek, J., and Rohlíková, L. (2019). Using process mining to analyze students’ quiz-taking behavior patterns in a learning management system. Comput. Hum. Behav. 92, 496–506. doi: 10.1016/j.chb.2017.12.015
Kizilcec, R., Baker, R., Bruch, E., Cortes, K., Hamilton, L., Nathan Lang, D., et al. (2023). From pipelines to pathways in the study of academic progress. Science 380, 344–347. doi: 10.1126/science.adg5406
Koning, R., Samila, S., and Ferguson, J.-P. (2021). Who do we invent for? Patents by women focus more on women’s health, but few women get to invent. Science 372, 1345–1348. doi: 10.1126/science.aba6990
Konrad, A. M., and Pfeffer, J. (1990). Do you get what you deserve? Factors affecting the relationship between productivity and pay. Adm. Sci. Q. 35, 258–285. doi: 10.2307/2393391
Krist, C., Schwarz, C. V., and Reiser, B. J. (2019). Identifying essential epistemic heuristics for guiding mechanistic reasoning in science learning. J. Learn. Sci. 28, 160–205. doi: 10.1080/10508406.2018.1510404
Lewis, A. E., and Diamond, J. B. (2015). Despite the best intentions: How racial inequality thrives in good schools. Oxford: Oxford University Press.
Leyva, L. A., McNeill, R. T., Marshall, B. L., and Guzmán, O. A. (2021a). “It seems like they purposefully try to make as many kids drop”: an analysis of logics and mechanisms of racial-gendered inequality in introductory mathematics instruction. J. High. Educ. 92, 784–814. doi: 10.1080/00221546.2021.1879586
Leyva, L. A., Quea, R., Weber, K., Battey, D., and López, D. (2021b). Detailing racialized and gendered mechanisms of undergraduate precalculus and calculus classroom instruction. Cogn. Instr. 39, 1–34. doi: 10.1080/07370008.2020.1849218
Maltese, A. V., Melki, C. S., and Wiebke, H. L. (2014). The nature of experiences responsible for the generation and maintenance of interest in STEM. Sci. Educ. 98, 937–962. doi: 10.1002/sce.21132
Masta, S. (2019). Challenging the relationship between settler colonial ideology and higher education spaces. Berkeley Rev Educ 8, 179–194. doi: 10.5070/B80037547
Matz, Rebecca, Hayward, Caitlin, and Fishman, Barry (2023). What happened when a university retained a COVID-era flexible course withdrawal policy? Exploring relationships between grading policy and equity. In Proceedings of the 13th International Conference on Learning Analytics and Knowledge (LAK23) Arlington, TX.
Matz, R. L., Koester, B. P., Fiorini, S., Grom, G., Shepard, L., Stangor, C. G., et al. (2017). Patterns of gendered performance differences in large introductory courses at five research universities. AERA Open 3:233285841774375. doi: 10.1177/2332858417743754
McCoy, D. L., Luedke, C. L., and Winkle-Wagner, R. (2017). Encouraged or weeded out: perspectives of students of color in the STEM disciplines on faculty interactions. J. Coll. Stud. Dev. 58, 657–673. doi: 10.1353/csd.2017.0052
McGee, E. O. (2020). Interrogating structural racism in STEM higher education. Educ. Res. 49, 633–644. doi: 10.3102/0013189X20972718
McGee, E. O. (2021). Black, brown, bruised: How racialized STEM education stifles innovation. Harvard Education Press. Cambridge, Massachussets.
McGee, E. O., and Martin, D. B. (2011). “You would not believe what i have to go through to prove my intellectual value!” Stereotype management among academically successful black mathematics and engineering students. Am. Educ. Res. J. 48, 1347–1389. doi: 10.3102/0002831211423972
Mensah, F. M., and Jackson, I. (2018). Whiteness as property in science teacher education. Teach. Coll. Rec. 120, 1–38. doi: 10.1177/016146811812000108
Mercer-Mapstone, L., Dvorakova, S. L., Matthews, K., Abbot, S., Cheng, B., Felten, P., et al. (2017). A systematic literature review of students as partners in higher education. Int J Students Partners 1, 1–23. doi: 10.15173/ijsap.v1i1.3119
Naidoo, R. (2004). Fields and institutional strategy: Bourdieu on the relationship between higher education, inequality and society. Br. J. Sociol. Educ. 25, 457–471. doi: 10.1080/0142569042000236952
National Science Foundation – Louis Stokes Alliance for Minority Participation (2023). NSF LSAMP. NSF STEM classification of instructional programs crosswalk. LSAMP Program. Available at: https://www.lsamp.org/help/help_stem_cip.cfm#bio (Accessed February 26, 2023).
Nielsen, M. W., Bloch, C. W., and Schiebinger, L. (2018). Making gender diversity work for scientific discovery and innovation. Nat. Hum. Behav. 2, 726–734. doi: 10.1038/s41562-018-0433-1
Nishi, N. W. (2021). It’s only micro when you don’t experience it: stealth racist abuse in college algebra. J. Divers. High. Educ. 16, 509–519. doi: 10.1037/dhe0000359
O’Connor, K., Peck, F. A., and Cafarella, J. (2015). Struggling for legitimacy: trajectories of membership and naturalization in the sorting out of engineering students. Mind Cult. Act. 22, 168–183. doi: 10.1080/10749039.2015.1025146
O’Hara, R. M. (2022). STEM (ing) the tide: a critical race theory analysis in STEM education1. J. Constr. Psychol. 35, 986–998. doi: 10.1080/10720537.2020.1842825
O’Shea, S. (2016). Avoiding the manufacture of “sameness”: first-in-family students, cultural capital and the higher education environment. High. Educ. 72, 59–78. doi: 10.1007/s10734-015-9938-y
Oakes, J. (1985). Keeping track: how schools structure inequality. New Haven & London: Yale University Press.
Østergaard, C. R., Timmermans, B., and Kristinsson, K. (2011). Does a different view create something new? The effect of employee diversity on innovation. Res. Policy 40, 500–509. doi: 10.1016/j.respol.2010.11.004
Park, J. J., Kim, Y. K., Salazar, C., and Hayes, S. (2020). Student–faculty interaction and discrimination from faculty in STEM: the link with retention. Res. High. Educ. 61, 330–356. doi: 10.1007/s11162-019-09564-w
Pearson, M. I., Castle, S. D., Matz, R. L., Koester, B. P., and Byrd, W. C. (2022). Integrating critical approaches into quantitative STEM equity work. LSE 21:es1. doi: 10.1187/cbe.21-06-0158
Pfeffer, J., and Langton, N. (1988). Wage inequality and the organization of work: the case of academic departments. Adm. Sci. Q. 33, 588–606. doi: 10.2307/2392645
Ray, V. (2019). A Theory of Racialized Organizations. American Sociological Review, 84, 26–53. doi: 10.1177/0003122418822335
Reinholz, D. L., Matz, R. L., Cole, R., and Apkarian, N. (2019). STEM is not a monolith: a preliminary analysis of variations in STEM disciplinary cultures and implications for change. LSE 18:mr4. doi: 10.1187/cbe.19-02-0038
Rodriguez, I., Brewe, E., Sawtelle, V., and Kramer, L. H. (2012). Impact of equity models and statistical measures on interpretations of educational reform. Phys. Rev. ST Phys. Educ. Res. 8:020103. doi: 10.1103/PhysRevSTPER.8.020103
Salazar-Fernandez, J. P., Sepúlveda, M., Munoz-Gama, J., and Nussbaum, M. (2021). Curricular analytics to characterize educational trajectories in high-failure rate courses that Lead to late dropout. Appl. Sci. 11:1436. doi: 10.3390/app11041436
Schulte, J., Fernandez de Mendonca, P., Martinez-Maldonado, R., and Buckingham Shum, S. (2017). Large scale predictive process mining and analytics of university degree course data. In Proceedings of the Seventh International Learning Analytics & Knowledge Conference Vancouver British Columbia Canada: ACM, 538–539.
Seymour, E., Hunter, A. B., and Weston, T. J. (2019). Why we are still talking about leaving. In Talking about leaving revisited: Persistence, relocation, and loss in undergraduate STEM education. Eds. E. Seymour and A.-B. Hunter (Cham: Springer International Publishing), 1–53.
Seymour, E., and Hunter, A.-B. (Eds). (2019). Talking about leaving revisited: Persistence, relocation, and loss in undergraduate STEM education. Cham: Springer International Publishing.
Steele, C. M., and Aronson, J. (1995). Stereotype threat and the intellectual test performance of African Americans. J. Pers. Soc. Psychol. 69, 797–811. doi: 10.1037/0022-3514.69.5.797
Stich, A. E. (2021). Beneath the white noise of postsecondary sorting: a case study of the “low” track in higher education. J. High. Educ. 92, 546–569. doi: 10.1080/00221546.2020.1824481
Strayhorn, T. L. (2011). Chapter 10 Sense of belonging and African-American student success in STEM: comparative insights between men and women. In Beyond stock stories and folktales: African Americans& paths to STEM fields. Emerald Group Publishing Limited, (pp. 213–226).
Thompson, M. E. (2021). Grade expectations: the role of first-year grades in predicting the pursuit of STEM majors for first-and continuing-generation students. J. High. Educ. 92, 961–985. doi: 10.1080/00221546.2021.1907169
Tomeh, D. H., and Sackett, P. R. (2022). On the continued misinterpretation of stereotype threat as accounting for black-white differences on cognitive tests. Pers. Assess. Decis. 8:1. doi: 10.25035/pad.2022.01.001
Trčka, N., and Pechenizkiy, M. (2009). From local patterns to global models: towards domain driven educational process mining. In 2009 Ninth International Conference on Intelligent Systems Design and Applications, 1114–1119.
Tyson, K. (2011). Integration interrupted: tracking, black students, and acting white after brown. New York, NY: Oxford University Press.
U.S. Department of Education College Scorecard (2023). College Scorecard. Available at: https://collegescorecard.ed.gov/ (Accessed February 26, 2023).
Weatherton, M., and Schussler, E. E. (2021). Success for all? A call to re-examine how student success is defined in higher education. CBE Life Sci. Educ. 20:es3. doi: 10.1187/cbe.20-09-0223
Webb, S., Burke, P. J., Nichols, S., Roberts, S., Stahl, G., Threadgold, S., et al. (2017). Thinking with and beyond Bourdieu in widening higher education participation. Stud. Contin. Educ. 39, 138–160. doi: 10.1080/0158037X.2017.1302926
Weston, T. J., Seymour, E., Koch, A. K., and Drake, B. M. (2019). “Weed-out classes and their consequences” in Talking about leaving revisited: Persistence, relocation, and loss in undergraduate STEM education. eds. E. Seymour and A.-B. Hunter (Cham, Switzerland), 197–243.
Whitcomb, K. M., Cwik, S., and Singh, C. (2021). Not all disadvantages are equal: racial/ethnic minority students have largest disadvantage among demographic groups in both STEM and non-STEM GPA. AERA Open 7:233285842110598. doi: 10.1177/23328584211059823
Wigdahl, J., Heileman, G., Slim, A., and Abdallah, C. (2014). Curricular efficiency: what role does it play in student success? ASEE Annual Conference and Exposition, Conference Proceedings. doi: 10.18260/1-2--20235
Wilder, C. S. (2013). Ebony and ivy: Race, slavery, and the troubled history of America’s universities. Bloomsbury Publishing USA Available at: https://www.bloomsbury.com/us/ebony-and-ivy-9781608193837/ (Accessed February 7, 2023).
National Science Foundation (2021). Women, Minorities, and Persons with Disabilities in Science and Engineering. Available at: https://ncses.nsf.gov/pubs/nsf21321/report (Accessed February 7, 2023).
Keywords: minoritization, biology, chemistry, STEM, attrition, process analytics, curriculum analytics
Citation: Fiorini S, Tarchinski N, Pearson M, Valdivia Medinaceli M, Matz RL, Lucien J, Lee HR, Koester B, Denaro K, Caporale N and Byrd WC (2023) Major curricula as structures for disciplinary acculturation that contribute to student minoritization. Front. Educ. 8:1176876. doi: 10.3389/feduc.2023.1176876
Edited by:
Sheila S. Jaswal, Amherst College, United StatesReviewed by:
I-Chien Chen, Michigan State University, United StatesBryan Dewsbury, Florida International University, United States
Alo Basu, College of the Holy Cross, United States
Copyright © 2023 Fiorini, Tarchinski, Pearson, Valdivia Medinaceli, Matz, Lucien, Lee, Koester, Denaro, Caporale and Byrd. This is an open-access article distributed under the terms of the Creative Commons Attribution License (CC BY). The use, distribution or reproduction in other forums is permitted, provided the original author(s) and the copyright owner(s) are credited and that the original publication in this journal is cited, in accordance with accepted academic practice. No use, distribution or reproduction is permitted which does not comply with these terms.
*Correspondence: Stefano Fiorini, c2Zpb3JpbmlAaXUuZWR1