- Educational Testing Service, Princeton, NJ, United States
Because dropout from PhD programs is common and is a problem with serious consequences for both students and institutions, identifying indicators of likely dropout would be very valuable. Scores on admissions tests might be useful, but existing data on their utility is contradictory and typically based on highly restricted small samples from just a single institution or a small set of institutions. Programs dropping the GRE as an admissions requirement noted this lack of convincing evidence that the test was useful in predicting the criterion of primary interest–persistence in graduate school PhD programs versus early dropout. HLM and quartile analyses were used to provide that evidence with a sample of 1,672 graduate programs containing 157,924 students. GRE Verbal and Analytical Writing scores, but not Quantitative scores, are shown to predict persistence versus dropout in a variety of majors with especially strong results in business, engineering, and the physical sciences (e.g., in the physical sciences only 40% of the students with low GRE Analytical Writing scores in their programs persist while 78% of the students with high scores do so).
Introduction
About 50% of students who begin doctoral programs drop out before receiving the degree (Cassuto, 2013). Being able to identify students who are at greatest risk of dropping out would have clear benefits for both students and graduate institutions. Both students and institutions make a significant financial investment in a graduate education and early drop out wastes these investments. Institutions that identified students with a higher risk of dropping out could commit more resources to helping these students succeed in graduate school. Scores on graduate admissions tests such as the GRE would seem to be one way of identifying these at-risk students, but the existing evidence on the value of such tests in identifying students likely to persist in PhD programs is equivocal and typically based on small samples with severe range restriction.
Showing a relationship between scores on a cognitive test such as the GRE and dropout from graduate school is challenging for several reasons. First, most dropout is for reasons other than a lack of cognitive ability. A study of students who dropped out of graduate programs by the National Center for Educational Statistics (Nevill and Chen, 2007) indicated that the top reasons for leaving were: change in family status, conflict with job or military, dissatisfied with program, needed to work, personal problems, other financial reasons, taking time off, and other career interests. A cognitive test could not be expected to predict a change in family status or job conflict. Second, most graduate programs have already selected students based on strong indicators of cognitive skill, so it is impossible to predict how students without those skills would have done had they been admitted. Third, generalizing from studies done in a single institution or a small handful of institutions is difficult and can lead to contradictory and confusing results.
Despite these challenges, a number of studies have attempted to evaluate the relationship of GRE scores to persistence in graduate programs. One study noted that GRE scores were higher for men who dropped out of PhD STEM programs than for those who remained enrolled (Petersen et al., 2017). But because this study used data from four flagship state universities that had selected students based on strong GRE quantitative (GRE-Q) scores virtually no one in the sample had low scores. The men who dropped out had average scores of 742 on the old 200–800 GRE scale and the students who persisted had average scores of 723. Thus, the results cannot tell us anything about the likely success of students with low or mediocre scores, or the potential value of considering GRE scores as part of a holistic review process. A study of biomedical graduate programs at the University of North Carolina (Hall et al., 2017) similarly reached a conclusion of no relationship of GRE scores to persistence but had the same problem of attempting to reach conclusions in a sample from highly selective programs that include few if any students with low scores. The title of another recent study (Miller et al., 2019), “Typical physics Ph.D. admissions criteria limit access to underrepresented groups but fail to predict doctoral completion,” seemed to suggest no relationship between GRE scores and persistence in a doctoral program. Nevertheless, the text concluded that significant associations exist. Using a multivariate logistic model with the 3,692 physics students in their sample, the study abstract noted, “Significant associations with completion were found for undergraduate GPA in all models and for GRE Quantitative in two of four studies models.” Specifically, in the model that included all students the significant odds ratio for GRE-Q was 1.013 with a standard error of 0.004. The “fail to predict doctoral completion” in the original article title is actually contradicted in the article text that notes, “the traditional admissions metrics of undergraduate grade point average (GPA) and the Graduate Record Examination (GRE) Quantitative, Verbal, and Physics Subject Tests do not predict completion as effectively [as] admissions committees presume.” “Fail to predict” and not predicting as effectively as committees presume are not the same thing. Furthermore, a number of flaws in the original analysis that tended to underestimate the effects for GRE-Q were identified (Weisman, 2019). Some of these flaws were addressed in a response by the authors (Miller et al., 2020) but a major concern remained–the severe range restriction on GRE-Q scores. As the authors acknowledged in their response to the comment, “Undergraduate physics majors’ GRE-Q scores are nearly all within just a few standard errors (SEs) of a perfect score. This strong range restriction necessarily limits the strength of any correlation between GRE-Q and any other variable.”
A study of 344 applicants to a top-five PhD program in economics (Grove and Stephen, 2007) indicated a significant positive relationship of both GRE verbal (GRE-V) and quantitative (GRE-Q) scores to program completion. The Probit for GRE-V was 0.064 and for GRE-Q the Probit was 0.152 with standard errors of 0.031 and 0.064 for V and Q, respectively. A study using data from a flagship public university and an Ivy League university (Bridgeman and Cline, 2022) indicated practically and statistically significant predictions of persistence for students in PhD programs in chemistry (n = 315) from GRE-Q, but not from GRE-V. Among students in the top quartile of GRE-Q scores in the chemistry programs 14% dropped out compared to 30% dropout in the bottom quartile of GRE scores, yielding a 2(drop/stay)x2(top quartile/bottom quartile) Chi-square with Yates correction of 5.28 with a p < 0.03. A study of 203 applicants (over a 7-year period) to a math PhD program at a Tier I public university in California noted significant GRE-V correlations with program completion (Ma et al., 2018); the coefficient from the logistic regression was 0.051 (p = 0.02). For GRE-Q, the authors reported a coefficient of 0.065 (p = 0.07) and noted that the coefficient “is only marginally significant, possibly due to the small variation in the GREQ scores among math PhD students (all scores tend to be near the maximum possible score of 166, with about a half within 2 points, and three quarters within 7 points of the maximum).”
In summary, some studies find a significant relationship of GRE scores to PhD program completion while others do not, and there are substantial limitations in all of these studies. Attempting to generalize from the existing studies is difficult because they may focus on only a single program at one university or data from just a few highly selective universities. The current study examines early dropout versus persistence to a PhD degree with a very large sample of programs and students by using comprehensive data from the National Student Clearinghouse.
Method
Sample
From ETS files, we identified GRE test takers with scores from 2012 to 2016 so that sufficient time would have elapsed for them to enroll in graduate programs and make some progress toward a degree. Names were sent to the National Student Clearinghouse that then provided data on where and when these students were enrolled in higher education institutions. These data did not indicate whether students’ postsecondary enrollment was specific to undergraduate or graduate studies, and therefore we implemented a data selection procedure that would allow us to select students who were likely enrolled in a graduate program. Specifically, using data from the biographical questionnaire that students complete when they register to take the GRE, only students who indicated a doctoral degree goal and who were enrolled in a higher education institution within 2 years of taking the GRE were retained in the sample.
Procedures
We identified students who were still enrolled 4 years after taking the GRE (persisters) or were no longer enrolled (early dropouts). Note that some of the dropouts may have obtained master’s degrees in less than 4 years after taking the GRE, but because they had stated a doctoral degree goal they could still appropriately be labeled as dropouts from their stated degree goal. Students were classified according to their intended graduate majors on the biographical questionnaire. It is possible that some students may have switched from their original stated intentions, but because the intention was recorded just as the students were about to apply to graduate school we assume that most students stayed with their stated intentions. We defined these intentions within institutions as programs, and only programs with at least 20 students were retained in the sample because analyses had to be conducted at the program-within-institution level before taking the sample size weighted average over programs. As indicated in Table 1, the sample consisted of 1,672 graduate programs containing 157,924 students. Although students are expected to have scores on the three GRE sections, Verbal (GRE-V), Quantitative (GRE-Q), and Analytical Writing (GRE-AW), occasionally a student would leave one section blank resulting in no score for that section and explaining the very slight variations in sample sizes across sections in Table 1. The Mean column is based on the weighted average of the means within each program/institution. The highest GRE-V mean, as might be expected, was in the humanities, but there was relatively little variation across the program means from a low of 153 to a high of 159. On the other hand, there was considerable variation in the GRE-Q mean scores from a low of 149 to a high of 162 with the highest means in business, engineering, and the physical sciences. We also looked at the median scores in each program/institution and noted that on-average medians were within one point of the means.
Analyses
Two methods were used to analyze the results. First, we conducted a series of hierarchical linear models (HLM) predicting the 0–1 criterion of persisting or dropping out from a binary indicator of whether the test takers were international or domestic (United States citizens or resident aliens), GRE-V, GRE-Q, and GRE-AW scores. Then, to make these results easier to visualize, within each program/institution we divided the students by GRE score quartile separately for V, Q, and AW, and identified the percent of students in each quartile who were still enrolled 4 years after taking the GRE (persisters) and took the within-institution sample size weighted average over all institutions. Note that both the HLM and quartile analyses account for students embedded in different programs/institutions; the GRE quartiles reflected different score levels in different programs/institutions and persistence v dropout was unique to each program/institution.
Hierarchical linear models
To account for the clustering of test takers within institutions, we conducted a series of generalized estimating equation logistic regressions in each of the study majors: business, education, engineering, humanities, life sciences, physical sciences, and social sciences. For each major, first an unconditional random effects analysis of variance (ANOVA) with PhD persistence as the outcome variable was conducted to compute the ICC for dichotomous outcomes (Snijders and Bosker, 1999; O’Connell et al., 2008) and determine the proportion of variance due to institutional variability. ICC values above 0.10 indicate the need to use multi-level modeling to account for the clustering of the data (Lee, 2000). In a second set of models, we added a binary variable for international versus domestic student status and each of the three GRE scores. Based on recommendations by Enders and Tofighi (2007), the continuous predictors were group mean centered. To be consistent with the sample selection for the quartile analysis, we restricted the analysis to programs within institutions with at least 20 individuals.
Quartile analysis
Within each program/institution, and separately for each of the three GRE scores, we identified students the bottom score quartile through the top score quartile. We computed the mean GRE score in each quartile in each program/institution and took the sample size weighted average of these means. These means and their standard deviations are in Table 2. Note that in the full sample of GRE test-takers the standard deviations for V, Q, and AW are 8.6, 9.6, and 0.9 (GRE, 2022), so the differences in means between the first and fourth quartiles are substantial. We also used the same procedure with undergraduate point average (UGPA) and with combined scores, e.g., students who were in top quartile on three measures (UGPA, GRE-V, and GRE-AW) or in the bottom quartile in all three measures.
Results
Hierarchical linear models
Although the unconditional models revealed that the proportion of between institution variance was over the suggested value of 0.10 (Lee, 2000) only for business (ICC = 0.12), we nevertheless ran HLM models for all of the programs. The results indicate an improvement in better model fit on the models including the predictors over the unconditional model (Tables 3–9). Overall, the results from the model with predictors indicate that GRE-V was a significant positive predictor and GRE-Q was a significant negative predictor of PhD persistence in every major. While the effect of GRE-AW was positive in every major (except in life sciences where GRE-AW had a significant negative relationship), its relationship was statistically significant only in Education, Engineering and Physical Sciences. It should be noted that while the regression coefficients for GRE-V and GRE-Q represents the single point increase on a scale that goes from 130 to 170, the GRE-AW scale is from 0 to 6, hence, appearing to have a larger effect in some majors.
Quartile analysis
The quartile results for persistence in graduate school are in Figures 1–3. For both GRE-V and GRE-W the percent of persisters in the fourth GRE quartile was always larger, often much larger, than the percent of persisters in the first quartile. For example, in engineering only 25% of the students in the first GRE-AW quartile persisted while 73% of the fourth quartile students were still enrolled 4 years after taking the GRE; in the physical sciences the percent persisting was 40% in the first quartile and 78% in the fourth. Surprisingly (but consistent with the HLM analysis), the results were reversed for GRE-Q with lower scoring students more likely to persist. For example, in the physical sciences 75% of the first quartile students persisted while only 50% of the fourth quartile students did so. Programs show a monotonic increase from the first to forth quartiles with the exception of GRE-AW in the life sciences with a decline from the first to third quartiles, but then a positive jump in the fourth quartile. This odd fit could explain the negative Logit weight and odds ratio less than 1.0 for GRE-AW in the HLM analyses for the life sciences while the weight is positive in all other majors.
Another way to look at these data is to determine the percent of programs in each program area in which there are more persisters in the top GRE score quartile than in the bottom quartile. These percents are in Table 10. Results mirrored the conclusions from Figures 1–3 with especially strong results for programs in business, engineering, and physical sciences for both GRE-V and GRE-AW scores, but results in the opposite direction for GRE-Q.
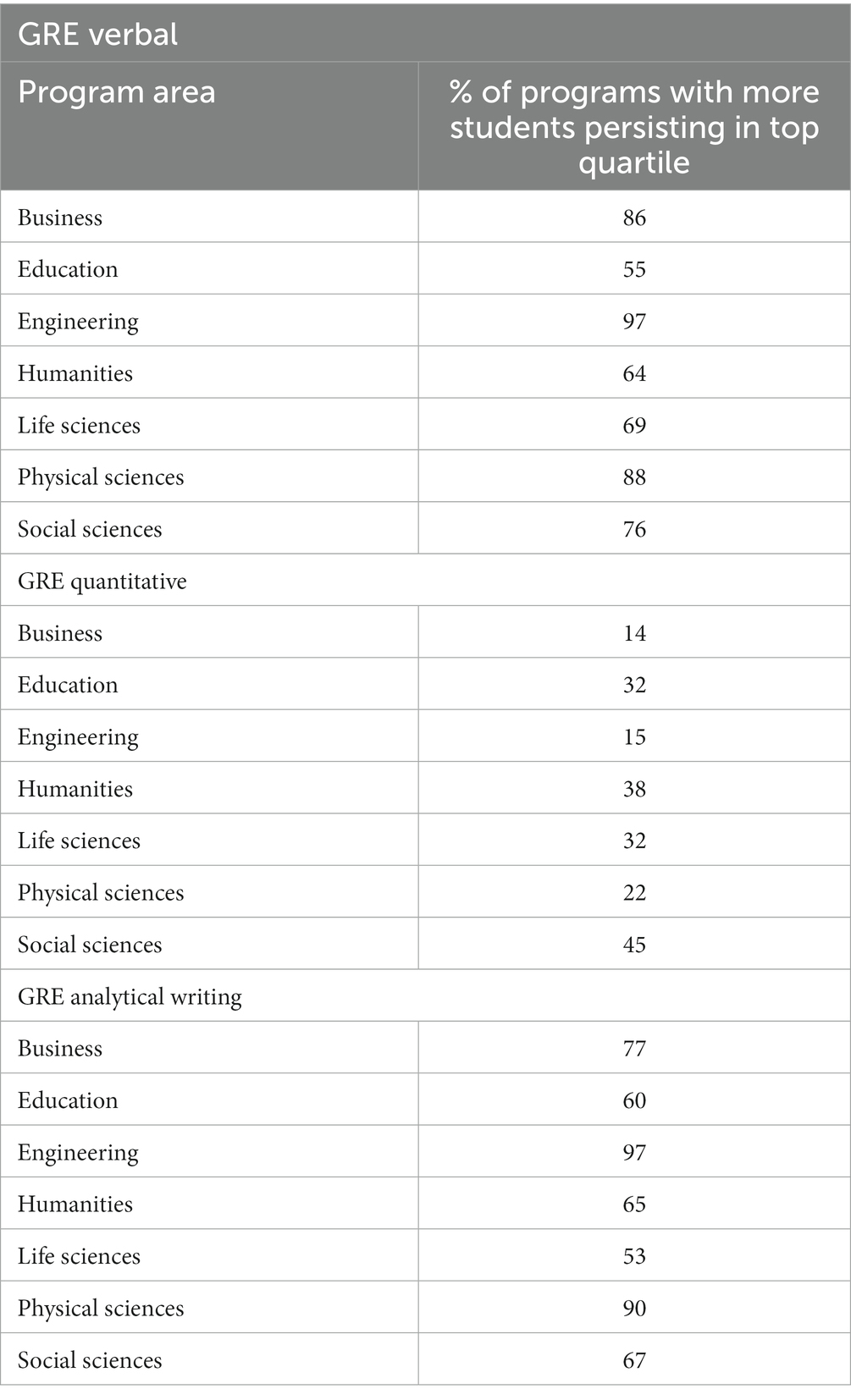
Table 10. Percent of Programs in which the percent of persisters is higher in the fourth quartile than in the first quartile.
We wondered if the pattern would be the same in more and less selective institutions, so we divided programs in thirds based on mean GRE-Q scores at the institution (i.e., highly selective, average, and less selective institutions). But the pattern was essentially the same for the more and less selective institutions.
Next we evaluated persistence for top and bottom quartiles of UGPA and for students who were in the top or bottom quartiles on multiple measures, that is, for example, a student would need to be in the top quartile in their program/institution on GRE-V and GRE-AW and GRE-Q or in the bottom quartile on all three scores. These results are in Table 11. Note that because results for GRE-Q are in the opposite direction there is more of an increase in persistence for the combination of just GRE-V and GRE-AW than for the combination that includes all three GRE scores. Also note that UGPA has a very limited impact as an indicator of persistence. This may be due to the very restricted range and generally high scores for UGPA with mean UGPAs of 3.6 or higher on the 0–4 scale for all majors.
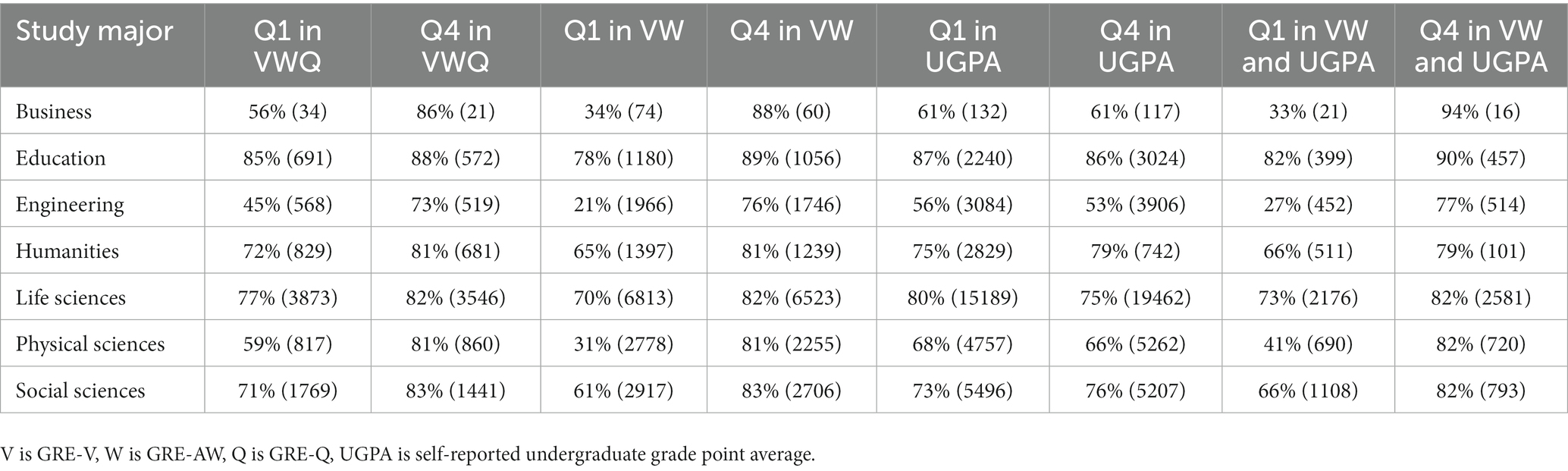
Table 11. Percent persisting in first and fourth quartiles for combined predictors with cell sizes in parentheses.
Discussion and conclusions
In both the HLM and quartile analyses, and with a much larger sample than in any prior research, greater persistence rates were associated with higher GRE-V and GRE-AW scores, but with lower GRE-Q scores. We do not have enough information to fully understand this result for GRE-Q, but one speculation is that students with very strong quantitative skills may drop out with just a master’s degree (despite their originally stated intention to get a PhD) when they realize that they already have the potential to earn a high salary without putting in the additional years needed to earn a PhD. Although exact data on the number of students choosing this path are not available, anecdotal reports are common. For example, a CNN report focused on the number of PhD dropouts getting high-paying jobs in Silicon Valley and noted:
Dropouts are nothing new to the Valley. Quite the opposite: The tech turk–characteristically, someone too brilliant, too arrogant, too obsessed for the classroom–is key to the Valley's creation myth and the stories it tells about itself. Sergey Brin, Larry Page and Jerry Yang dropped out of graduate programs. (Ozy, 2014)
Consistent with this speculation are the large declines in persistence in business, engineering, and physical sciences for students where there are many employment opportunities for students with strong quantitative skills, and the relatively flat profiles for education, humanities, and the social sciences.
Additional research is also needed to better understand the apparently low persistence rates for international students. Our data did not indicate whether students left graduate school after receiving a master’s degree. Although we considered any such students to be non-persisters given a stated degree goal of a doctorate, international students may have been more likely to intentionally or unintentionally indicate a doctoral degree goal when they were actually seeking a master’s degree.
These results suggest that GRE scores could have a place as part of a holistic review of potential PhD candidates. We fully support the conclusions in a 2014 Nature article (Miller and Stassun, 2014) that GRE scores should not have a disproportionate weight in admissions decisions; many factors should be considered in making holistic admissions decisions, and relatively low GRE scores should not be used to reject an otherwise clearly qualified candidate. But the relationship of GRE scores to persistence demonstrated in this research suggests that while GRE scores should not have disproportionate weight they also should not have zero weight whether used as part of an admissions decision or in identifying admitted students who may need extra support to avoid early dropout.
Data availability statement
The raw data supporting the conclusions of this article will be made available by the authors, without undue reservation.
Ethics statement
The studies involving human participants were reviewed and approved by ETS IRB. Written informed consent for participation was not required for this study in accordance with the national legislation and the institutional requirements.
Author contributions
BB, MO-A, and SH contributed to the conceptualization, analyses, and manuscript writing for this study. All authors contributed to the article and approved the submitted version.
Funding
The study received funding from ETS.
Conflict of interest
ETS researchers are encouraged to freely express their professional judgment. Therefore, points of view or opinions stated in this report do not necessarily reflect ETS positions or policies.
Publisher’s note
All claims expressed in this article are solely those of the authors and do not necessarily represent those of their affiliated organizations, or those of the publisher, the editors and the reviewers. Any product that may be evaluated in this article, or claim that may be made by its manufacturer, is not guaranteed or endorsed by the publisher.
References
Bridgeman, B., and Cline, F. (2022). Can the GRE predict valued outcomes? Dropout and writing skill. PLoS One 17:e0268738. doi: 10.1371/journal.pone.0268738
Cassuto, L. (2013). Ph.D. attrition: how much is too much? The Chronicle of Higher Education. Available at: https://www.chronicle.com/article/ph-d-attrition-how-much-is-too-much/.
Enders, C. K., and Tofighi, D. (2007). Centering predictor variables in cross-sectional multilevel models: a new look at an old issue. Psychol. Methods 12, 121–138. doi: 10.1037/1082-989X.12.2.121
GRE . (2022). Interpreting your GRE scores: 2022-23. Available at: https://www.ets.org/pdfs/gre/interpreting-gre-scores.pdf.
Grove, W. A., and Stephen, W. (2007). The search for economics talent: doctoral completion and research productivity. Am. Econ. Rev. 97, 506–511. doi: 10.1257/aer.97.2.506
Hall, J. D., O’Connell, A. B., and Cook, J. G. (2017). Predictors of student productivity in biomedical graduate school applications. PLoS One 12:e0169121. doi: 10.1371/journal.pone.0169121
Lee, V. E. (2000). Using hierarchical linear modeling to study social contexts: the case of school effects. Educ. Psychol. 35, 125–141. doi: 10.1207/S15326985EP3502_6
Ma, T., Wood, K. E., Xu, D., Guidotti, P., Pantano, A., and Komarova, N. L. (2018). Admission predictors for success in a mathematics graduate program. ArXiv 2018. doi: 10.48550/arXiv.1803.00595
Miller, C., and Stassun, K. (2014). A test that fails. Nature 510, 303–304. doi: 10.1038/nj7504-303a(2014)
Miller, C. W., Zwickl, B. M., Posselt, J. R., Silvestrini, R. T., and Hodapp, T. (2019). Typical physics Ph.D. admissions criteria limit access to underrepresented groups but fail to predict doctoral completion. Sci. Adv. 5:eaat7550. doi: 10.1126/sciadv.aat7550
Miller, C. W., Zwickl, B. M., Posselt, J. R., Silvestrini, R. T., and Hodapp, T. (2020). Response to comment on typical physics Ph.D. admissions criteria limit access to underrepresented groups but fail to predict doctoral completion. Sci. Adv. 6:4647. doi: 10.1126/sciadv.aba4647
Nevill, S. C., and Chen, X. (2007). The path through graduate school: a longitudinal examination 10 years after bachelor’s degree. (NCES No. 2007-162). Washington, DC: National Center for Education Statistics, US Department of Education.
O’Connell, A. A., Goldstein, J., Rogers, H. J., and Peng, C. Y. J. (2008). “Multilevel logistic models for dichotomous and ordinal data” in Multilevel modeling of educational data. eds. A. A. O’Connell and D. B. McCoach (Charlotte, NC: Information Age Publishing, Inc), 199–242.
Ozy, P. B . (2014). PhD dropout? Lucrative Silicon Valley career. Available at: https://money.cnn.com/2014/09/09/news/economy/ozy-dropout-career/index.html.
Petersen, S. L., Erenrich, E. S., Levine, D. L., Vigoreaux, J., and Gile, K. (2017). Multi-institutional study of GRE scores as predictors of STEM PhD degree completion: GRE gets a low mark. PLoS One 13:e0206570. doi: 10.1371/journal.pone.0206570
Snijders, T. A. B., and Bosker, R. J. (1999). Multilevel analysis An introduction to basic and advanced multilevel modeling. Thousand Oaks: Sage Publications.
Weisman, M. B. (2019).Do GRE scores help predict getting a physics Ph.D.: a comment on a paper by Miller et al. Available at: https://arxiv.org/ftp/arxiv/papers/1902/1902.09442.pdf.
Keywords: dropout, persistence, graduate school, PhD, HLM
Citation: Bridgeman B, Olivera-Aguilar M and Holtzman S (2023) The GRE as a predictor of persistence to a PhD. Front. Educ. 8:1182508. doi: 10.3389/feduc.2023.1182508
Edited by:
Anastasiya A. Lipnevich, The City University of New York, United StatesReviewed by:
Terrence Calistro, The City University of New York, United StatesDrew Gitomer, Rutgers, The State University of New Jersey, United States
Arminda Wey, Rutgers University, United States
Copyright © 2023 Bridgeman, Olivera-Aguilar and Holtzman. This is an open-access article distributed under the terms of the Creative Commons Attribution License (CC BY). The use, distribution or reproduction in other forums is permitted, provided the original author(s) and the copyright owner(s) are credited and that the original publication in this journal is cited, in accordance with accepted academic practice. No use, distribution or reproduction is permitted which does not comply with these terms.
*Correspondence: Brent Bridgeman, YnJpZGdlOEBtc24uY29t