- 1State Grid Energy and Power Planning Laboratory, State Grid Energy Research Institute Co., Ltd., Beijing, China
- 2School of Electrical Engineering, Southeast University, Nanjing, China
Hydrogen production by electrolysis is considered an essential means of consuming renewable energy in the future. However, the current assessment of the potential of renewable energy electrolysis for hydrogen production is relatively simple, and the perspective is not comprehensive. Here, we established a Combined Wind and Solar Electrolytic Hydrogen system, considering the influence of regional wind-solar-load characteristics and transmission costs to evaluate the hydrogen production potential of 31 provincial-level regions in China in 2050. The results show that in 2050, the levelized cost of hydrogen (LCOH) in China’s provincial regions will still be higher than 10 ¥/kg, which is not cost-competitive compared to the current hydrogen production from fossil fuels. It is more cost-effective to deploy wind turbines than photovoltaic in areas with similar wind and solar resources or rich in wind resources. Wind-solar differences impact LCOH, equipment capacity configuration, and transmission cost composition, while load fluctuation significantly impacts LCOH and electricity storage configuration. In addition, the sensitivity analysis of 11 technical and economic parameters showed differences in the response performance of LCOH changes to different parameters, and the electrolyzer conversion efficiency had the most severe impact. The analysis of subsidy policy shows that for most regions (except Chongqing and Xizang), subsidizing the unit investment cost of wind turbines can minimize LCOH. Nevertheless, from the perspective of comprehensive subsidy effect, subsidy cost, and hydrogen energy development, it is more cost-effective to take subsidies for electrolysis equipment with the popularization of hydrogen.
1 Introduction
With the depletion of traditional fossil energy and the increasingly severe environmental problems it brings, countries worldwide are gradually falling into the dilemma of traditional fossil energy, and it is imminent to promote the reform of traditional energy structure (Pan et al., 2021a; Bertram et al., 2021; Wu et al., 2022). Countries have promulgated regulations and policies to promote the vigorous development of renewable energy sources such as wind and solar to achieve the goal of carbon neutrality (Holdmann et al., 2019; O’Malley et al., 2020; Yang et al., 2021). The International Energy Agency (IEA) states that renewable energy generation needs to grow at an annual rate of nearly 12% over the 2021–2030 period to put the world on track for net-zero emissions by 2050 (International Energy Agency, 2021). How to absorb a high proportion of renewable energy power generation will become a fundamental research problem (Yang et al., 2018; Wu Y et al., 2021).
As one of the essential means of consuming renewable energy, electrolytic hydrogen has received extensive attention worldwide in recent years (Wang et al., 2021; Wu Y J et al., 2021). Compared with electricity, the advantage of hydrogen energy is that it can be used as long-term energy storage to achieve cross-regional and cross-season energy mutual assistance (Pan et al., 2020; Zhang et al., 2021). Currently, the research on hydrogen production from renewable energy focuses on feasibility (Fereidooni et al., 2018; Lin et al., 2021; Lucas et al., 2022) and cost analysis (Glenk and Reichelstein, 2019; Guerra et al., 2019; Pan et al., 2021b), multi-subject cooperation (Wu et al., 2020; Ma et al., 2021), and the operation planning and marketization of systems such as electric-hydrogen hybrid energy storage and hydrogen-mixed natural gas (Xiao et al., 2017; Tao et al., 2020). However, at present, the evaluation of hydrogen production potential from renewable energy is mainly based on specific regions, and only independent evaluation of hydrogen production from wind power or photovoltaic hydrogen production is conducted. For example, Lucas et al. (2022) and Lin et al. (2021) analyzed the feasibility of hydrogen production from wind power. Fereidooni et al. (2018) analyzed the feasibility of solar hydrogen production and evaluated the potential of photovoltaic hydrogen production in Iran. Pan et al., 2021a) analyzes China’s cost and low-carbon competitiveness of hydrogen production from grids and photovoltaics. (Guerra et al. (2019). and (Glenk and Reichelstein, 2019) focus on the economics and cost competitiveness of hydrogen production from renewable energy sources. In addition, the current research is relatively simple in considering the electrolytic hydrogen model, lacks comprehensive system modeling, and lacks the analysis of the wind-solar combination and regional load characteristics.
Because of the above shortcomings, this paper predicts and evaluates the levelized hydrogen production cost of 31 provincial-level regions in China in 2050 and establishes a Combined Wind and Solar Electrolytic Hydrogen system (CWSEHs). On this basis, we thoroughly evaluate the impact of the combination of wind and solar and the energy consumption characteristics of each provincial region on the CWSWHs and give a system sensitivity analysis and a variety of subsidy policy evaluations. In particular, based on the characteristics of future marketization and the consideration of promoting the development of hydrogen production from renewable energy, wind and solar power generation is expected to use the existing power network to transmit and supply electricity to the hydrogen production system by paying a transmission fee, which can not only promote the process of marketization but also significantly reduce the investment cost of the line. Therefore, we have established a more refined model for the CWSEHs and fully consider the influence of factors such as transmission fee and inflation rate in the calculation of levelized cost of hydrogen (LCOH), hoping to provide a helpful reference and thinking for hydrogen production from renewable energy in 2050.
2 Materials and methods
2.1 Wind and solar capacity factor and electrical load data
To obtain the capacity factor (CF) of wind and solar power generation in each provincial region, we simulated through the renewables. ninja platform jointly developed by ETH Zurich and Imperial College of Technology (Pfenninger and Staffell, 2016), and obtained 8760 h of wind and solar power generation CF in each provincial region throughout the year, as the upper limit of wind turbine (WT) and photovoltaic (PV) power generation output. Specifically, for different provincial-level regions, we select five cities distributed in five directions to calculate the CF of wind energy and solar energy, respectively, and take the average value as the CF of wind energy and solar power generation in the provincial-level region. Similarly, the national average wind and solar CFs were averaged across 31 provincial-level regions. Due to data limitations, hourly provincial electric loads were obtained from provincial power grid companies or fitted based on typical provincial daily and annual load curves (National Energy Administration, 2020).
In addition, in order to make the article easy to understand and without losing rigor, we use abbreviated form for the names of 31 provincial-level regions in China. The specific correspondence is shown in Table 1 below.
2.2 Optimization model of CWSEHs
2.2.1 Objective function
In this paper, we define LCOH as the hydrogen production cost obtained after the cost of wind and solar power generation equipment, electrolysis-related equipment investment, operation and maintenance, and transmission fee and hydrogen production amount are levelized. A series of factors such as investment, operation, and maintenance costs, transmission fees, equipment life, wind and solar power generation CF, electrolyzer conversion efficiency, discount rate, inflation rate, and electrical load in the system will affect LCOH. Among the above parameters, the parameters with regional differences are the CF of wind and solar power generation and the regional electricity load. The following optimization model can be established based on this, with the objective function of minimizing LCOH.
As shown in Eq. 1, the established optimization model aims to minimize the LCOH of the system, where Cinv is the annual investment cost of PV, WT, electricity storage (ES), and electrolysis (ELY) equipment. Com is the annual operation and maintenance cost of the system. Ctp is the transmission fee paid for transmitting photovoltaic and wind turbine power generation through the grid. N is the system’s life, i is the inflation rate, r is the discount rate, ηELY is the conversion efficiency of ELY, PELY t is the electric power consumed by electrolysis at time t, and LHV is the low calorific value of hydrogen.
Cinv, Com and Ctp are given by Eqs 2–4, where λ is the hydrogen to electricity ratio and represents the power consumed by hydrogen production to the power consumed by the electrical load. CPV, CWT, CES and CELY are PV, WT, ES, and ELY’s optimal configuration capacities. cPV inv, cWT inv, cES inv and cELY inv are the unit investment costs of PV, WT, ES, and ELY, respectively. cPV om, cWT om, cES om and cELY om are the unit annual fixed operation and maintenance costs of PV, WT, ES, and ELY, respectively. σPV and σWT are the unit power transmission fees paid by PV and WT, respectively, and PPV t and PWT t are the actual power generated by PV and WT at time t.
2.2.2 Constraints
The optimization model also needs to satisfy the investment and equipment operation constraints of Eqs 5–17:
2.2.2.1 Electric power balance constraint
Equation 5 ensures the hour-level electric power balance of the CWSEHs, where PES+ t and PES- t respectively represent ES's charge and discharge power at time t, and PLOAD t represents the regional electrical load at time t. PELY t represents the electrical power consumed by ELY at time t, ΦT is the time series set, ΦT = {1,2,3,…,8760}.
2.2.3 Hydrogen production power constraint
Equation 6 ensures that ELY’s electricity consumed is λ times the electric load daily. According to Hydrogen Council (2017) and Institute of Climate Change and Sustainable Development, Tsinghua University (2021), it is estimated that hydrogen energy and electric energy will account for 18% and 55% of the final energy demand in 2050, respectively. Based on this, we calculated the value of λ.
2.2.4 Equipment investment capacity constraints
Equations 7–10 ensure that the investment capacity of PV, WT, ES and ELY equipment is non-negative.
2.2.5 PV and WT operating constraints
Equations 11 and 12 and ensure that the output electric power of PV and WT is non-negative and not greater than the upper limit of output power, where pPV t and pWT t are the upper limits of output electric power of unit capacity PV and unit WT at time t, respectively.
2.2.6 ES operating constraints
Equations 13 and 14 respectively ensure that the ES charge and discharge power is non-negative and not greater than the ES configuration capacity. Eq. 15 ensures that the actual storage capacity of the ES is not greater than the upper limit of the storage capacity, where SES t is the actual storage capacity of the ES at time t. Eq. 16 simulates ES’s charging and discharging process and ensures the same storage capacity at the beginning and end of ES, where ηES+ and ηES- are ES's charging and discharging efficiencies, respectively.
2.2.7 ELY operating constraint
Equation 17 is the ELY operating constraint, which limits the minimum operating power of ELY, where εELY t is the operating state of ELY at time t, its value 1 means ELY is working, and 0 means ELY is not working.
2.3 Model parameters
In this model, the relevant techno-economic parameters of CWSEHs are unified in 2050, and the specific data are given in Table 2 below. Among them, the annual operation and maintenance (OM) cost of PV, WT, and ES are 0.01 of the unit investment cost of each piece of equipment, and the annual OM cost of ELY is 0.04 of the unit investment cost of it.
3 Results
3.1 System structure
Figure 1 shows the schematic diagram of the CWSEHs structure. On the energy supply side, renewable energy power generation, such as wind and solar energy, and electric energy storage modules are considered dispatchable electric power to compensate for the imbalance between supply and demand. The network side considers wind and solar power generation through the existing power network for transmission and pays a certain transmission fee. On the load side, we consider that electric energy can be used to simultaneously meet the electrical load and the operation of power to hydrogen (P2H) equipment. The produced hydrogen energy can be used as fuel in transportation, power generation, and heating and can also be used as raw materials for oil refining and steel production.
3.2 LCOH assessment of wind solar electrolytic hydrogen in China
Figure 2 shows the LCOH of wind-solar combined hydrogen production in 31 provincial-level regions in China. The darker the background color of each region, the higher the LCOH (See Supplementary Figure S1 in Supplementary Material for the composition of LCOH in each provincial region). Overall, there are significant regional differences in the LCOH of wind-solar combined hydrogen production in 31 provincial-level regions. It is estimated that in 2050, the highest provincial LCOH in China will be CQ and the lowest is Inner Mongolia, and the span between the two can reach 16 ¥/kg. The eight provinces with the highest LCOH are CQ, ZJ, HB, SC, XJ, GD, YN, and FJ, all of which are greater than 15 ¥/kg, and the main parts are located in the eastern coastal areas and central areas. While northern regions such as HL, LN, JL, and IM have lower LCOH, it can be found that the LCOH of different provincial regions has significant geographic differences, which are essentially related to the level of wind and solar resources and power generation in each region.
The above results show that the differences between LCOH and wind and solar resources are significantly correlated. In order to further study the impact of the two on LCOH, Figure 3 shows the relationship between LCOH and the proportion of PV and WT configuration capacity in each provincial region. The definition of PV and WT capacity ratio is shown in Eq. 18. It can be found that compared with WT, there is a higher correlation between the PV capacity ratio and LCOH. Moreover, regions with higher PV capacity ratios have higher LCOH. The results show that from the perspective of reducing LCOH to a certain extent, the configuration of WT is more cost-effective than the configuration of PV. Of course, this is also related to the wind and solar CF in each provincial area, and the configuration of WT in areas with low wind power CF will be of limited significance.
3.3 The influence of wind and solar characteristics on CWSEHs
In order to compare the impact of wind and solar characteristics on the system, we selected four representative provinces for typical analysis to ensure that they are under similar load fluctuations, as shown in the first four rows in Table 3.
Figure 4A shows that HB has the highest LCOH of 17.38 ¥/kg, SH has the lowest LCOH of only 8.48 ¥/kg, the difference is nearly 10 ¥/kg, and the LCOH of TJ and XZ are in the middle. Comparing the data in Table 3, we can see that HB has the lowest wind and solar CF, SH has the highest wind and wind CF, and TJ and XZ have higher wind and photovoltaic CF, respectively has a significant correlation with their LCOH. In addition, comparing TJ and XZ again shows that wind resources can reduce LCOH more than solar resources.
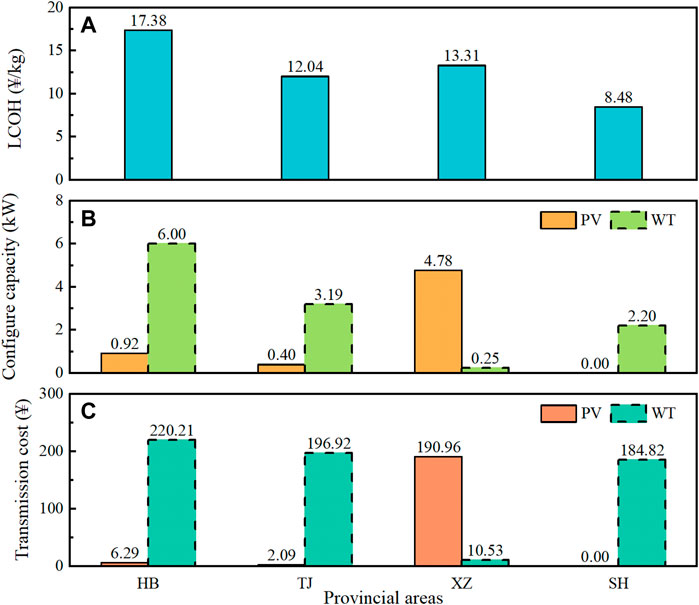
FIGURE 4. Comparison of (A) LCOH (B) PV and WT configuration capacity (C) PV and WT transmission costs in four representative regions.
Figure 4B shows the configuration capacity of PV and WT in four regions. The configuration capacity of PV and WT has a positive relationship with the CF of PV and WT. The CF of TJ wind power is higher, so its WT configuration capacity is larger, XZ can configure more PV because of higher PV power generation CF. It is worth noting that the CF of wind and solar power generation of HB and SH are both higher or lower, and the system is more inclined to configure WT instead of PV at this time, which is consistent with the above analysis. The PV and WT transmission cost in Figure 4C is similar to the equipment configuration capacity in Figure 4B. Because the more WT or PV equipment capacity is equipped, the greater the power generation and the higher the transmission cost. Wind and solar differences significantly impact LCOH, WT, and PV capacity allocation and transmission costs. In areas with similar wind and solar resources, prioritizing WT equipment can further reduce LCOH, making it more competitive than PV equipment.
3.4 The influence of power load volatility on CWSEHs
When studying the influence of regional electrical load fluctuations on system parameters, in order to avoid the influence of dimensions, we have normalized the electrical loads in different regions and selected two regions with similar wind and solar resources and significant differences in electrical load fluctuations as shown in the last two rows in Table 3.
The left panel in Figure 5A shows that the LCOH of XJ is higher than that of SA, but in Figure 5B, it is shown that the ES configuration capacity of SA is significantly higher than that of XJ, even more than two times that of XJ, which is due to the electrical load fluctuation of SA is bigger than XJ. Specifically, when the electrical load is low, the PV and WT power generation can meet the electrical load and the P2H system, and only a small amount or no ES is needed for compensation. While at a high electrical load, most or all of the PV and WT power generation is absorbed by the electrical load, and the P2H system needs to be compensated by ES. In addition, regardless of the fluctuation of the electrical load, the WT configuration capacity is higher than the PV configuration capacity (Figure 5B), and WT also occupies an absolute advantage in the transmission fee (Figure 5A), which highlighted that the configuration of WT has significant advantages and universality compared to PV again.
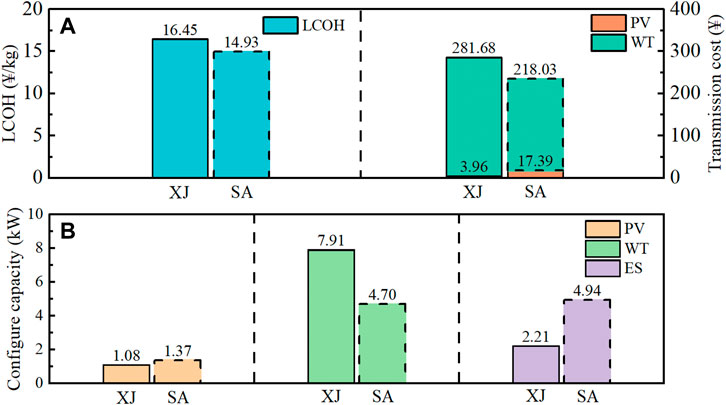
FIGURE 5. Comparison of (A) LCOH and PV and WT transmission costs (B) PV, WT and ES configuration capacity for two representative regions.
3.5 LCOH sensitivity analysis
Figure 6 presents the LCOH sensitivity analysis of CWSEHs under different parameters. We calculated the LCOH at this time by making each parameter ±25% based on the benchmark scenario. It can be seen from Figure 6 that the average LCOH in China under the benchmark scenario is 9.82 ¥/kg, and the change in electrolyzer conversion efficiency has the most significant impact on LCOH, and a ±25% fluctuation will cause LCOH to change from 7.86 ¥/kg to 13.10 ¥/kg. The impact of the unit investment cost of WT, system lifetime, and discount rate on LCOH is gradually reduced but still has a significant impact. Changes in other parameters have a limited impact on LCOH, especially the unit investment cost of PV, and the transmission costs of PV can hardly make LCOH change, which is also related to the low PV configuration capacity. It is worth noting that as λ increases, the LCOH will gradually decrease, which means that when the hydrogen demand (electric power consumed by hydrogen production) increases, the LCOH will further decrease, which is consistent with the scale effect of system development. In addition, system lifetime, electrolyzer conversion efficiency, inflation rate, and λ remain negatively correlated with LCOH changes, which is also consistent with common sense.
3.6 Subsidy policy analysis
Currently, the LCOH of hydrogen production using traditional fossil energy (such as coal and natural gas) is about 10 ¥/kg (National Alliance of Hydrogen and Fuel Cell, 2019), and according to our prediction in 2050, 27 of China’s 31 provincial-level regions have LCOH higher than 10 ¥/kg. It means that even by 2050, the competitiveness of renewable energy electrolysis hydrogen production is still limited compared with traditional fossil energy hydrogen production. Therefore, we considered six different subsidy policies, adopting a 50% investment cost subsidy for PV, WT, ELY, ES, and a 50% cost subsidy for PV and WT transmission costs. The subsidy effect is shown in Figure 7. The results show that, except for the two regions of CQ and XZ, subsidizing WT can reduce LCOH to the greatest extent, so that the LCOH in most regions is reduced to less than 10 ¥/kg, while CQ and XZ have the best subsidy effect on PV, which is mainly closely related to the landscape resources in different regions. Generally, the effect of subsidizing ES and PV is better than the other three subsidy policies except for the WT subsidy, which can significantly reduce LCOH. The ELY subsidy and the PV and WT transmission cost subsidy have the worst effect on reducing LCOH, which can only reduce about 0.5 ¥/kg.
Further, Figure 8 (the upper part) shows the annual cost of the six subsidy policies. Overall, the annual cost of various subsidy policies is proportional to the subsidy effect. The better the subsidy effect, the higher the annual subsidy cost. It is worth noting that CQ subsidizes PV with the best effect, but the annual cost of PV subsidy is less than that of WT subsidy, which is a very shocking situation because it shows that adopting PV subsidy in CQ can not only minimize LCOH, but also it can ensure that the annual subsidy cost is not the highest, which is a very cost-effective subsidy policy.
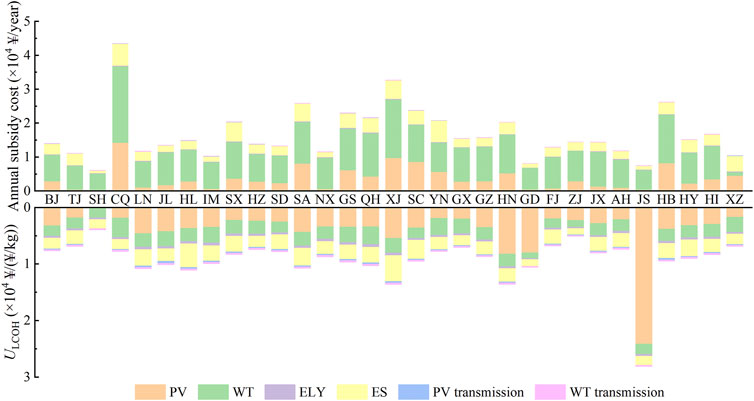
FIGURE 8. Annual subsidy costs and unit LCOH reduction costs for each provincial-level region under six subsidy policies.
In response to the particular situation of CQ, we comprehensively considered the subsidy effect of various policies and the annual subsidy cost and proposed the concept of unit LCOH decrease cost, whose definition is shown in Eq. 19. In the equation, UM,i LCOH, CostM,i, and ΔLCOHM,i are the unit LCOH decrease cost, annual subsidy cost, and LCOH decrease of the ith provincial region under the Mth subsidy policy, respectively, ΦCOMP and ΦPRO are the subsidy policy set and the provincial set, respectively. Figure 8 (the lower part) shows each provincial region’s unit LCOH decrease cost. It can be found that PV, WT, and ES subsidies have their advantages and disadvantages in different regions, but generally, the ULCOH subsidized by PV, WT, and ES will be higher than that subsidized by ELY and transmission costs. In particular, although subsidizing ELY can obtain lower ULCOH, it still has a limited effect on reducing LCOH because the predicted terminal hydrogen demand in 2050 is still small, and the required ELY equipment configuration capacity is limited. However, in the long run, there is excellent potential to subsidize ELY after the terminal hydrogen demand increases in the future. In addition, if the PV and WT transmission costs can be further reduced, this will benefit LCOH.
4 Discussion
In the context of emission reduction, hydrogen production from renewable energy electrolysis has received extensive attention from countries worldwide. In this paper, we build CWESHs, evaluate the LCOH of 31 provincial-level regions in China in 2050, and analyze the advantages and disadvantages of using PV or WT power generation in detail. Starting from regional wind and solar energy characteristics and load characteristics, we evaluate the impact of regionally differentiated resource characteristics and load characteristics on CWESHs. Finally, the system sensitivity is analyzed, and the differences between the six subsidy policies are compared from different aspects, which is expected to provide helpful guidance for developing and popularizing hydrogen energy.
In China’s provincial-level LCOH assessment, 27 of the 31 provincial-level regions are expected to have still LCOH higher than 10 ¥/kg in 2050, which is limited compared to traditional fossil energy hydrogen production. In this context, if a specific carbon tax is charged for hydrogen production from fossil energy at the policy level, it will further enhance the cost competitiveness of hydrogen production from renewable energy. In addition, we also found that deploying WT is more cost-effective than deploying PV and can widely promote wind power development in areas with abundant wind resources. In particular, when analyzing the influence of wind and solar characteristics and regional electrical load characteristics on the system, it was once again found that the configuration of WT in areas with similar wind and solar resources or areas with abundant wind resources is more cost-effective than PV, and the analysis of 31 provincial-level regions shows that the results are generalizable.
Finally, in the analysis of the system’s sensitivity, it is pointed out that the conversion efficiency of the electrolyzer, the unit investment cost of WT, the system lifetime, and the discount rate are the four most critical factors affecting LCOH. With the further development of electrolyzer-related technologies in the future, even a slight increase in electrolysis efficiency can significantly impact reducing LCOH. Subsidy policy analysis shows that WT subsidies can reduce LCOH to the greatest extent in most provinces, but the annual cost is also the highest, but CQ and XZ are exceptional cases. With the further development of hydrogen energy in the future, subsidies for ELY equipment will have more tremendous application potential.
The above research has comprehensively assessed China’s future renewable energy electrolysis hydrogen business, which is of great significance for promoting the development and popularization of hydrogen energy. In the future, we will further explore the unique role of hydrogen energy in carbon reduction from the perspective of deep coupling between the power system and the hydrogen energy system.
Data availability statement
The original contributions presented in the study are included in the article/Supplementary Material, further inquiries can be directed to the corresponding author.
Author contributions
GL and ZG conceived and designed the research. GL, BY, and ZG developed the framework and formulated the theoretical model. GL, BY, and ZG conducted the data search. GL, BY and ZG performed the simulations and analyses. All authors contributed to the discussions on the methods and the writing of this article.
Funding
This work was supported by State Grid Corporation of China Headquarters Science and Technology Project (1400-202257460A-2-0-ZN).
Conflict of interest
Authors GL, BY, HC, CW, and PX were employed by the company State Grid Energy Research Institute Co., Ltd.
The remaining author declares that the research was conducted in the absence of any commercial or financial relationships that could be construed as a potential conflict of interest.
The authors declare that this study received funding from State Grid Corporation of China Headquarters Science and Technology Project. The funder had the following involvement in the study: data collection, interpretation, analysis and the writing of this article.
Publisher’s note
All claims expressed in this article are solely those of the authors and do not necessarily represent those of their affiliated organizations, or those of the publisher, the editors and the reviewers. Any product that may be evaluated in this article, or claim that may be made by its manufacturer, is not guaranteed or endorsed by the publisher.
Supplementary material
The Supplementary Material for this article can be found online at: https://www.frontiersin.org/articles/10.3389/fenrg.2022.1046140/full#supplementary-material
Abbreviations
CWSEHs, Combined wind and solar electrolytic hydrogen system; LCOH, Levelized cost of hydrogen; CF, Capacity factor; WT, Wind turbine; PV, Photovoltaic; ES, Electricity storage; ELY, Electrolysis; OM, Operation and maintenance; P2H, Power to hydrogen.
References
Bertram, C., Riahi, K., Hilaire, J., et al. (2021). Energy system developments and investments in the decisive decade for the Paris Agreement goals. Environ. Res. Lett. 16, 074020. doi:10.1088/1748-9326/ac09ae
Fereidooni, M., Mostafaeipour, A., Kalantar, V., and Goudarzi, H. (2018). A comprehensive evaluation of hydrogen production from photovoltaic power station. Renew. Sustain. Energy Rev. 82, 415–423. doi:10.1016/j.rser.2017.09.060
Glenk, G., and Reichelstein, S. (2019). Economics of converting renewable power to hydrogen. Nat. Energy 4, 216–222. doi:10.1038/s41560-019-0326-1
Gu, Z., Pan, G., and Gu, W. (2022). “Portfolio of wind-photovoltaic-loads toward green hydrogen development,” in 2022 IEEE 5th International Electrical and Energy Conference (CIEEC).
Guerra, O. J., Eichman, J., Kurtz, J., and Hodge, B. M. (2019). Cost competitiveness of electrolytic hydrogen. Joule 3, 2425–2443. doi:10.1016/j.joule.2019.07.006
Holdmann, G. P., Wies, R. W., and Vandermeer, J. B. (2019). Renewable energy integration in Alaska’s remote islanded microgrids: Economic drivers, technical strategies, technological niche development, and policy implications. Proc. IEEE 107, 1820–1837. doi:10.1109/jproc.2019.2932755
Hydrogen Council (2017). Hydrogen, scaling up. Available at: https://hydrogencouncil.com/en/study-hydrogen-scaling-up/(Accessed September 14, 2022).
Institute of Climate Change and Sustainable Development, Tsinghua University (2021). Research on China's long-term low-carbon development strategy and transformation path. Beijing: Tsinghua University.
International Energy Agency (IEA) (2021). Renewable power. Available at: https://www.iea.org/reports/renewable-power (Accessed September 14, 2022).
International Renewable Energy Agency (IRENA) (2020). Green hydrogen cost reduction: Scaling up electrolysers to meet the 1.5⁰C climate goal. Available at: https://www.irena.org/publications/2020/Dec/Green-hydrogen-cost-reduction (Accessed September 14, 2022).
Kempler, P. A., Slack, J. J., and Baker, A. M. (2022). Research priorities for seasonal energy storage using electrolyzers and fuel cells. Joule 6, 280–285. doi:10.1016/j.joule.2021.12.020
Lin, H., Wu, Q., Chen, X., Yang, X., Guo, X., Lv, J., et al. (2021). Economic and technological feasibility of using power-to-hydrogen technology under higher wind penetration in China. Renew. Energy 173, 569–580. doi:10.1016/j.renene.2021.04.015
Lucas, T. R., Ferreira, A. F., Pereira, R. S., and Alves, M. (2022). Hydrogen production from the WindFloat atlantic offshore wind farm: A techno-economic analysis. Appl. Energy 310, 118481. doi:10.1016/j.apenergy.2021.118481
Ma, T., Pei, W., Xiao, H., Li, D., Lyu, X., and Hou, K. (2021). Cooperative operation method for wind-solar-hydrogen multi-agent energy system based on Nash bargaining theory. Proceeding CSEE 41, 25–39.
National Alliance of Hydrogen and Fuel Cell (2019). White paper of China’s hydrogen energy and fuel cell industry (2019 Edition). Beijing: National Alliance of Hydrogen and Fuel Cell.
National Energy Administration (NEA) (2020). Notice on doing a good job in the signing of medium- and long-term electric power contracts in 2021. Available at: https://www.ndrc.gov.cn/xwdt/tzgg/202012/t20201202_1252095.html?code=&state=123 (Accessed September 14, 2022).
O’Malley, M. J., Anwar, M. B., Heinen, S., Kober, T., McCalley, J., McPherson, M., et al. (2020). Multicarrier energy systems: Shaping our energy future. Proc. IEEE 108, 1437–1456. doi:10.1109/jproc.2020.2992251
Pan, G., Gu, W., Hu, Q., Wang, J., Teng, F., and Strbac, G. (2021a). Cost and low-carbon competitiveness of electrolytic hydrogen in China. Energy Environ. Sci. 14, 4868–4881. doi:10.1039/d1ee01840j
Pan, G., Gu, W., Lu, Y., Qiu, H., Lu, S., and Yao, S. (2020). Optimal planning for electricity-hydrogen integrated energy system considering power to hydrogen and heat and seasonal storage. IEEE Trans. Sustain. Energy 11, 2662–2676. doi:10.1109/tste.2020.2970078
Pan, G., Hu, Q., Gu, W., Ding, S., Qiu, H., and Lu, Y. (2021b). Assessment of plum rain’s impact on power system emissions in Yangtze-Huaihe River basin of China. Nat. Commun. 12, 6156–6210. doi:10.1038/s41467-021-26358-w
Pfenninger, S., and Staffell, I. (2016). Long-term patterns of European PV output using 30 years of validated hourly reanalysis and satellite data. Energy 114, 1251–1265. doi:10.1016/j.energy.2016.08.060
Qiu, C. (2022). Analysis and suggestions on the pricing mechanism of transmission and distribution Fee of pilot distributed power generation nearby trading. Water Power, 1. doi:10.3969/j.issn.0559-9342.2022.09.020
State Grid Energy Research Institution (2019). China energy & electricity outlook. Beijing: China Electric Power Press.
Tao, Y., Qiu, J., Lai, S., and Zhao, J. (2020). Integrated electricity and hydrogen energy sharing in coupled energy systems. IEEE Trans. Smart Grid 12, 1149–1162. doi:10.1109/tsg.2020.3023716
Wang, W., Huang, S., Zhang, G., Liu, J., and Chen, Z. (2021). Optimal operation of an integrated electricity-heat energy system considering flexible resources dispatch for renewable integration. J. Mod. Power Syst. Clean Energy 9, 699–710. doi:10.35833/mpce.2020.000917
Wu, X., Li, H., Wang, X., and Zhao, W. (2020). Cooperative operation for wind turbines and hydrogen fueling stations with on-site hydrogen production. IEEE Trans. Sustain. Energy 11, 2775–2789. doi:10.1109/tste.2020.2975609
Wu, Y., Lin, Z., Liu, C., Huang, T., Chen, Y., Ru, Y., et al. (2022). Resilience enhancement for urban distribution network via risk-based emergency response plan amendment for ice disasters. Int. J. Electr. Power & Energy Syst. 141, 108183. doi:10.1016/j.ijepes.2022.108183
Wu, Y, Y., Lin, Z., Liu, C., Chen, Y., and Uddin, N. (2021). A demand response trade model considering cost and benefit allocation game and hydrogen to electricity conversion. IEEE Trans. Ind. Appl. 58, 2909–2920. doi:10.1109/tia.2021.3088769
Wu, Y. J, Y. J., Liang, X. Y., Huang, T., Lin, Z. W., Li, Z. X., and Hossain, M. F. (2021). A hierarchical framework for renewable energy sources consumption promotion among microgrids through two-layer electricity prices. Renew. Sustain. Energy Rev. 145, 111140. doi:10.1016/j.rser.2021.111140
Xiao, Y., Wang, X., Pinson, P., and Wang, X. (2017). A local energy market for electricity and hydrogen. IEEE Trans. Power Syst. 33, 3898–3908. doi:10.1109/tpwrs.2017.2779540
Yang, J., Zhang, N., Wang, Y., and Kang, C. (2018). Multi-energy system towards renewable energy accommodation: Review and prospect. Automation Electr. Power Syst. 42, 11. doi:10.7500/AEPS20171002004
Yang, Y., Wu, W., Wang, B., Li, M., and Zhu, T. (2021). Optimal decomposition of stochastic dispatch schedule for renewable energy cluster. J. Mod. Power Syst. Clean Energy 9, 711–719. doi:10.35833/mpce.2020.000620
Keywords: electrolytic hydrogen, renewable energy, transmission cost, wind-solar-load characteristics, techno-economic assessment (TEA), sensitivity analysis A(t), subsidy policy analysis
Citation: Lu G, Yuan B, Gu Z, Chen H, Wu C and Xia P (2023) Techno-economic assessment of electrolytic hydrogen in China considering wind-solar-load characteristics. Front. Energy Res. 10:1046140. doi: 10.3389/fenrg.2022.1046140
Received: 16 September 2022; Accepted: 31 October 2022;
Published: 16 January 2023.
Edited by:
Yingjun Wu, Hohai University, ChinaCopyright © 2023 Lu, Yuan, Gu, Chen, Wu and Xia. This is an open-access article distributed under the terms of the Creative Commons Attribution License (CC BY). The use, distribution or reproduction in other forums is permitted, provided the original author(s) and the copyright owner(s) are credited and that the original publication in this journal is cited, in accordance with accepted academic practice. No use, distribution or reproduction is permitted which does not comply with these terms.
*Correspondence: Zhongfan Gu, Z3pmMjIwNkAxNjMuY29t