- 1Human-Computer Interaction Center (HCIC)/Chair of Communication Science, RWTH Aachen University, Aachen, Germany
- 2Risk Perception and Communication, RWTH Aachen University, Aachen, Germany
- 3Junior Professorship of Management Science, Faculty of Business and Economics, TU Dresden, Dresden, Germany
- 4Chair of Operations Management, School of Business and Economics, RWTH Aachen University, Aachen, Germany
To abate climate change and ameliorate the air quality in urban areas, innovative solutions are required to reduce CO2 and pollutant emissions from traffic. Alternative fuels made from biomass or CO2 and hydrogen can contribute to these goals by substituting fossil gasoline or diesel in combustion engines. Using a conjoint analysis approach, the current study investigates preferences of laypeople (n = 303) for fuel production facilities in terms of siting location, plant size, raw material used in the production, and raw material transport. The location was most decision-relevant, followed by raw material transport, whereas plant size and type of raw material played a less prominent role for the preference choice. The best-case scenario from the point of view of acceptance would be the installation of a rather small bio-hybrid fuel production plant in an industrial area (instead of an agricultural or pristine environment). No transport or transport via underground pipeline were preferred over truck/tank car or overground pipeline. The findings can be used as a basis for planning and decision-making for designing production networks for new fuel types.
1 Introduction
Climate change and the constant release of emissions into the atmosphere have been recognized as a major threat to the environment and the well-being of living on our planet by the parties who signed the Paris Agreement in 2016. Consequently, the goal was formulated to combat global warming by limiting anthropogenic climate change to a maximum increase of 1.5° compared to pre-industrial levels (United Nations, 2015). In 2018, the transport sector was responsible for 27% of global CO2 emissions. Although the demand for oil for both individual and long-distance transportation (e.g., aircraft, trucks) has historically declined during the COVID-19 crisis in 2020 (IEA, 2020a; IEA, 2020b), forecasts indicate that global energy demand in 2030 could increase to 110% of 2019 demand (IEA, 2020c). During the COVID-19 pandemic, a reduction of worldwide CO2 emissions was observed as a reaction to decreased mobility, industrial production, and economy (Nguyen et al., 2021). The CO2 emissions per day in April 2020 were 17% lower than average CO2 levels in 2019 (Le Quere et al., 2020) and for the year 2020 they decreased by 6.4% compared to pre-crisis times (Tollefson, 2021a). However, the decreasing effect of the COVID-19 pandemic was only a temporary one and it is estimated that CO2 emission levels will rise again and meet or augment the CO2 emissions before the pandemic (Smith et al., 2021), which is called the “Pandemic rebound” (Tollefson, 2021b). For the transport sector, several technologies are available that can contribute to achieving the climate targets mentioned, e.g., electric vehicles, but also liquid fuels obtained from biomass, hydrogen, or CO2 (Stančin et al., 2020).
In the context of this paper, we define alternative fuels as “any gaseous or liquid transportation fuel for light-duty vehicles other than gasoline or diesel” (Linzenich et al., 2019). These can include biofuels (produced from biomass), e-fuels (produced from CO2, water and renewable electricity), and bio-hybrids (a combination of biofuel and e-fuel pathway). The production of renewable fuels requires large amounts of renewable electricity, biomass, and carbon dioxide, depending on the production pathway. Wind and solar power generation facilities define the primary sources of renewable electricity. To obtain carbon dioxide, there is the possibility to use point sources or direct air capture. Further, many types of biomass can be used to produce renewable fuels (Ruiz et al., 2019). Since there is limited availability of resource potentials and a highly heterogeneous spatial distribution, a combination of the different pathways and inherent technologies will be used in the future.
This paper focuses on a production network for renewable fuels in Germany. Figure 1 gives an overview of the three stages of a renewable fuel production network: resource potentials, production of renewable fuels, and distribution of these fuels to demand regions. The main decisions in a renewable fuel production network include decisions on production facility locations and logistics activities. In addition to the location of a production facility, the size and production pathway have to be determined. In the planning of renewable fuel supply chains, the aim is to determine a particular production network configuration which meets the fuel demand at minimum cost.
Herein, it is essential to take into account that plants and modes of transport never exist in a vacuum—they are embedded into an environment with existing properties, infrastructure, inhabitants, and various types of land use. Therefore, positive public perception and acceptance are crucial for the social diffusion of alternative fuels. Herein, positive perception and acceptance are not limited to the end product of alternative fuels but must also include the production and transportation infrastructure (Offermann-van Heek et al., 2018). In addition, refusal potentials differ for various types of alternative energy generation. Refusal potentials were found to be linked to the acceptance of the respective type of renewable energy generation (Liebe and Dobers, 2019). For this reason, it is crucial to investigate the general perception of fuel production facilities and, particularly, the local acceptance of potential construction sites as well as the influence of different installation conditions.
To examine the acceptance and preferences for the local installation of a production plant for alternative fuels, the current study uses data from a conjoint analysis study with 303 German laypeople. The aim is to reveal what influences decisions regarding production facilities and to identify the best-case scenario for the production of alternative fuels from the point of view of acceptance. The novelty of the work lies in the analysis of social acceptance requirements for technically relevant parts of the production network for alternative fuel types. Although crucial for energy infrastructure siting decisions, the question of how to design socially accepted production networks for novel fuel types has been scarcely addressed in the relevant literature [e.g., (Arning et al., 2019; Offermann-van Heek et al., 2020)]. The scope of the current analysis is demonstrated taking Germany as an example, but the findings can be applied to global fuel production networks when adjusting for local topography and infrastructure, country-specific energy and transport systems, environmental policies, and cultural value orientations. The results of this analysis quantify the social acceptance of various technically feasible renewable fuel production plant designs. In planning models, these results can be used to directly consider social acceptance when selecting production sites and configurations for a renewable fuel production network.
The paper is structured as follows: In Section 2, the current state of research on the social acceptance of alternative fuels is presented in line with the research questions guiding this research. In Section 3, the methodology and the survey sample are described. Section 4 contains the study results. First, general findings on the acceptance of alternative fuels are presented, followed by an analysis of laypeople’s preferences for the configuration of fuel production facilities. In a last step, a sensitivity analysis is conducted on different location scenarios for alternative fuels plants. The study findings are discussed in Section 5 and include the development of recommendations for action for policy makers. The paper closes with the conclusion in Section 6.
2 Social acceptance of alternative fuels and their production infrastructure
2.1 Acceptance and preferences for alternative fuel production and other types of infrastructure technologies
Considering the many uses of the term, acceptance is defined as the (active or passive) approval of the development, implementation, and use of technologies (Dethloff, 2004). The multidimensional construct of acceptance has an attitude dimension, which can range from approval to rejection, and a behavioural dimension which can be expressed in accepting behaviour (purchase, recommendation, use) or rejecting behaviour (protest, boycott, legal action). Especially for novel and unknown technologies, an indifferent or tolerating attitude can often be found among the public (Schweizer-Ries, 2008). Acceptance is shaped by individual technology assessments (such as perceived costs and benefits), but also by external contextual factors (social norms, media coverage, political and cultural climate). Also, an indifferent attitude can change over time into an accepting or rejecting attitude.
A conceptualisation of acceptance that is useful in the context of energy system infrastructures was developed by Wüstenhagen (Wüstenhagen et al., 2007), who distinguishes between three dimensions of social acceptance in the context of renewable energy projects: socio-political, market, and local acceptance. Socio-political acceptance comprises the general societal acceptance of the technology, including the legal framework conditions. Market acceptance refers to the process by which all market participants, i.e., operators, investors but also consumers, adopt and support an innovative energy technology. Community acceptance refers to the specific projects at the local level and thus to the stakeholders acting on the ground and the population affected by them. A positive market acceptance is only one building block for the successful diffusion of alternative fuels; a positive general or socio-political and, above all, local or community acceptance is also required to expand the necessary production and transport infrastructure. As the expansion of sustainable energy system infrastructures has shown, protests occur primarily at the local level, which is directly affected by the expansion [e.g., against the introduction of wind farms (Kontogianni et al., 2014), the construction of power lines (Devine-Wright and Batel, 2013), or storage sites for CO2 (Carbon Capture and Storage) (Terwel and Daamen, 2012)].
The state of knowledge about the acceptance of alternative fuels and their production and transport infrastructures is still very limited. So far, alternative fuels have been studied from a social science perspective mainly in terms of their market acceptance (Schulte et al., 2004; Chin et al., 2014; Linzenich et al., 2019). From a consumer perspective, alternative fuels are perceived positively as long as they are not significantly more expensive than conventional fuels in terms of price (Hackbarth and Madlener, 2016) and there are no high requirements for the conversion of the fuel tank (Linzenich et al., 2019). Studies show that the level of vehicle emissions impacts the process of choosing an alternative fuel type (Bunch et al., 1993), it is not yet known whether this also applies to feared emissions from fuel production facilities, which could lead to a rejection of facilities near homes.
While the economic and environmental impacts of alternative fuel production facilities have been studied elaborately, [e.g., (Santibañez-Aguilar et al., 2014; Borning et al., 2020)] and respective requirements and factors of success are derived, only little is known so far about the factors that determine an acceptable design of alternative fuel plants from the perspective of the public and potentially affected residents. Only few studies have focused on the issue of site selection and ways to achieve community acceptance (Tigges and Noble, 2012; Fortenbery et al., 2013). In a study on the general and local acceptance of CCU technology (e.g., for fuel production) it was found that local acceptance for a plant tended to be neutral, with the strongest (negative) influencing factor being affective risk perception, but this did not affect general acceptance (Arning et al., 2020).
The results on the perception of distance between one’s home/residence and an industrial facility of the energy and environmental sector and associated risks show differences across different studies, e.g., depending on the type of facility to be placed. In case of waste facilities, Johnson et al. (2012) reported that while people who perceive their own residence as being (rather) far from a facility have fewer concerns about safety risks that it may pose. However, it cannot be confirmed that living (very) close to a facility is a significant factor in assessing its hazardousness (Johnson and Scicchitano, 2012). An analysis of ethanol plant siting decisions found that population density is negatively related to siting probabilities, while feedstock availability and transportation infrastructure availability are important factors in siting decisions (Haddad et al., 2010). The acceptance of renewable energy infrastructure and network can also vary depending on the type of feedstock used, as shown by varying acceptance scores for proximity to different plants. For example, the construction of solar or wind power plants is accepted at a lower distance from one’s own home than biomass power plants, which perform on the same level as natural gas power plants. Similar results are seen for spatial acceptance of infrastructure like electrical grid and pipelines, where underground grid expansion is more acceptable than overground infrastructure elements. In the same study, the perceived degree of landscape modification was identified as the greatest influencing factor on local acceptance (Bertsch et al., 2016), which leaves room for consideration of whether an interplay of the factors of space, visibility, proximity, and the type of space used (e.g., natural or already industrially built environment) could be influential here. Finally, when comparing perceptions of different energy technologies (e.g., CCS, biomass) in a study it was concluded that the evaluation categories can vary widely and that each technology brings its own pattern of relevant factors. Therefore, no one-fits-all solution for renewable energy implementation can exist (Scheer et al., 2017).
From these considerations it is clear that when planning sites for fuel production, from the point of view of the affected population, various factors must be taken into account. These include raw material, location, and transport. For the end product ‘alternative fuels’ and their properties, this has been done in the past using choice-based conjoint studies to determine which property is most important for society to evaluate or where tradeoffs exist (Tanaka et al., 2014; Byun et al., 2018; Linzenich et al., 2019).
There is still no study that combines the relevant aspects regarding an acceptable roll-out of the production and transport infrastructure of different alternative fuel types. As the introduction of renewable energy technologies has shown, public perceptions are not always positive and supportive. However, these perceptions and reactions of the public and affected communities should not be dismissed as troublesome and irrational, but should be taken into account as valuable and informative indicators of individual and social needs that enable a tailor-made technology development and communication.
2.2 Framing of the study and reasoning of the experimental approach
This section regards the reasoning of the study and the approach, and the long-term vision of using the social acceptance data for technical and economic planning. One could argue that the planning of alternative fuel facilities and their infrastructure can only be assessed by technical and policy experts, as this is a complex and multifactorial problem that requires a high domain knowledge and expertise. Based on this assumption, it could be concluded that the investigation of average citizens’ needs and preferences is useless as laypeople’s views on such planning processes might be naive, lacking the required expert knowledge, and, as a consequence, the complexity of the planning problem cannot be “correctly” assessed by them due to their restricted view. However, it is important to consider that these very citizens or local politicians are supposed to accept the placement solutions in the end in their neighborhood or community. Therefore, it is an essential cornerstone for a successful roll-out to assess the acceptance patterns and the preferences for different planning scenarios from the perspective of laypeople.
Our goal, which is pursued in this work, is to capture and quantify the preference patterns of laypersons in order to be able to use the results for planning based on acceptance criteria. This approach requires that 1) laypeople’s preferences are assessed in scenarios with different (technically) realistic planning characteristics and 2) that tipping points between the tolerated and non-tolerated scenarios are determined in order to integrate the tolerated scenarios into the set of planning alternatives for economic modelling. As relevant criteria for the planning of fuel production networks we examined the siting location, the plant size, the type of raw material used in the production, and different transport modes of the raw materials. In order to enable laypersons to carry out these complex evaluations, two strategies were pursued (United Nations, 2015): Understandability of the problem. It was important to convey in advance the basic concepts and principles of the criteria studied. For this reason, the participants received an introduction to the topic before the acceptance evaluation began, which was optimized by experts in terms of technical correctness and comprehensibility. In addition, the abstract information (e.g., the type of transport or raw material) was illustrated by pictograms so that the scenarios could be understood better and distinguished from each other. This type of information presentation made it possible to make complex technical scenarios accessible—even for laypersons. (IEA, 2020a). Manageability of the complexity. In order to cut down the complexity of the decisions we presented experimental scenarios representing different versions of planning and siting scenarios that had to be assessed as acceptable or not. Each of the scenarios consisted of different levels of the main attributes, i.e., siting location, plant size, type of raw material used in the production, and different transport modes of the raw material (see 3.1 for a detailed description of the method). In this way, the complexity in each scenario was manageable and it was finally calculated which of the scenario characteristics were the main drivers of (non) acceptance.
2.3 Research questions
Based on the current state of research and the identified research gap, this study investigates the following research questions:
1. Which decision criteria are relevant for laypeople when judging alternative fuel production plants?
2. Which is the preferred roll-out scenario for alternative fuels from an acceptance perspective?
3. Which are the focal points for the acceptance of different siting location scenarios?
3 Methods
In this section, we first justify the conjoint method, then describe the attributes of the production plant infrastructure in detail, followed by the description of the structure of the questionnaire (including the listing of all items used in line with their theoretical foundation and source), which is followed by a description of the sample and data analysis.
3.1 Conjoint methodology
In order to examine consumers’ decisions or choices, different methods (multidimensional scaling, conjoint procedures, e.g., (Carmone et al., 1978; Malhotra, 1982)) can be applied. As shown in a number of methodological validity studies (Baier et al., 2014; Selka et al., 2014; Baier et al., 2015), the choice-based conjoint study (CBC) was superior in predicting accurate consumer choices and was therefore chosen to investigate preferences of laypeople for alternative fuel production plants. CBC is a type of conjoint analysis in which the respondents select in multiple choice tasks their preferred product or scenario out of different options, which consist of the selected attributes and their varying levels (Orme, 2019). In a conjoint study, participants do not evaluate single aspects of a product or technology in isolation. Instead, they evaluate the product or technology as a whole (i.e., as a combination of different characteristics). The basic assumption of conjoint analysis is that the total utility (meaning the overall preference judgement or acceptance) of a product or scenario is composed linearly additive by the part-worth utilities for each attribute level (Rao, 2014). The individual part-worth utilities are estimated by Hierarchical Bayesian (HB) estimation. Conjoint analysis provides basically two types of results: the decision-relevance of the evaluation criteria for choosing a product/scenario (the relative importance scores) and the attractiveness of each attribute level (the part-worth utilities) (Orme, 2019). Herein, the part-worth utilities provide information whether an attribute level has a positive or negative contribution to the choice decision. The relative importance of an attribute indicates how much the attribute contributes to the attractiveness of a scenario and is calculated based on the range between the highest and lowest part-worth utility of the levels of this attribute for each respondent (Orme, 2019). Based on the HB analysis data, sensitivity analyses can be conducted. These sensitivity analyses produce preference shares, which can be understood as an indicator for the relative attractiveness of one scenario compared to other scenarios and allow identifying focal points for increasing the acceptance of a product or technology (Orme, 2019).
Thus, conjoint studies simulate real-life decision situations, in which multiple decision criteria are involved, e.g., consumers selecting which product they want to buy and use. This is why conjoint analysis is a method that is frequently applied in the marketing sector to analyze preferences of consumers for products and brands, to identify the preferred product configurations, and to uncover possible trade-offs between evaluation criteria (Green and Srinivasan, 1978; Arning, 2017). Conjoint studies are an established research method in different disciplines [e.g., in the healthcare sector (Marshall et al., 2010) and environmental science (Alriksson and Öberg, 2008)]. In technology acceptance research, the benefits of conjoint analyses for identifying preferences for the planning of energy projects [e.g., wind farms (Knapp and Ladenburg, 2015)] and mobility innovations [such as alternative fuel vehicles (Linzenich et al., 2019)] are increasingly acknowledged.
In this study, conjoint analysis allows to evaluate the relevance of planning criteria for production plants for alternative fuels with regard to their acceptance and to identify designs for an accepted roll-out of alternative fuel production plants in Germany.
3.2 Conjoint study design and attributes
In the following, the conjoint design and the selected attributes and levels are described. The fuel production plant options were characterized by four attributes: plant size, location, raw material used, and raw material transport. Table 1 gives an overview of the attributes and levels used in the study.
The attribute plant size was chosen because it is an important parameter from the planning perspective, and larger plants might increase the perceived visual impact of the plant. In previous studies on large-scale infrastructure acceptance, e.g., in the fuel production and wind farm context, the size of plants or height of turbines was frequently considered, although the relevance of size for acceptance and preferences was found to be limited (Langer et al., 2017; Arning et al., 2019; Offermann-van Heek et al., 2020).
Plant size was modeled as the size of the production plant compared to a village of 530 m × 630 m. On top of that, information about the production capacity was given as the percentage of the total demand of diesel in Germany that could be replaced by the amount of alternative fuels produced by the respective plant size.
There is a range of possible plant sizes that could be selected. This range is determined by available resource potentials and fuel demand. To satisfy a meaningful fraction of Germany’s demand for liquid fuels in transportation, large-scale production facilities are required. Therefore, we have chosen 150 kilotons Fischer-Tropsch-liquids/year as the lower bound for the capacity of any fuel production facility. We refer to this size as a small production facility. As the upper bound for production capacity we have chosen 6.500 kilotons FT-liquids/year (large production facility). We have estimated the required land area of such a production facility based on a large-scale gas-to-liquids plant. We assumed a linear relationship between production capacity and required land area. Further, we added two more levels to the attribute plant size: The size rather small refers to a production capacity of 500 kilotons FT-liquids/year and rather large denotes a size of 1.500 kilotons FT-liquids/year. We are able to represent the set of potential facility sizes by the use of these four capacity levels. The resulting levels are similar to levels used by Arning et al. (Arning et al., 2019) and Offermann-van Heek et al. (Offermann-van Heek et al., 2020) and ranged between a small plant (producing 0.4% of the total demand in Germany) and a large plant (producing 18.6% of the total demand in Germany). However, in contrast to these studies, we did not link plant size to the (de)centralized distribution of the plants.
Also, past research has identified the siting location as an important parameter for the acceptance of energy infrastructure projects. Previous studies have found that visibility from residential areas and placement in open countryside (e.g., in pristine areas such as forests or an installation near nature protection areas) influence preferences and acceptance (Ek and Persson, 2014; Kontogianni et al., 2014; Bertsch et al., 2016). To represent a variety of landscape types, four levels for location were selected using a K-means clustering approach on CORINE Land Cover data (Büttner and Kosztra, 2011) in line with previous acceptance research [e.g., (Zaunbrecher et al., 2017)]: industrial area, field, field near residential area, and forest/pristine area. We chose to include the distinction between the landscapes field and field near residential area to also capture the distance aspect and whether the plant would be visible from residents’ homes.
The levels of the attribute raw material represent the three production pathways of alternative fuels: the biofuel pathway (raw material biomass), the electrofuel pathway (raw materials CO2 + hydrogen), and the bio-hybrid fuel pathway (raw materials biomass + hydrogen). We let the participants evaluate the raw material basis instead of the production pathway because: 1. Production plants of all fuel types are based on the Fischer-Tropsch process and thus the differences are only tangible for laypeople in terms of the raw materials provided. 2. The production pathway is a multidimensional and multifaceted attribute that would have made it impossible to understand on which basis laypeople formed their evaluations (e.g., did they evaluate the different raw materials, the production processes, or the resulting fuels?). Raw materials were selected as attributes because previous research (Offermann-van Heek et al., 2020) has shown that for CO2-based fuels the source of CO2 was crucial for acceptance and we wanted to shed light on the issue of whether the type of raw material used is important for laypeople. We assumed that fuel production facilities are based on Fischer-Tropsch synthesis. The three levels of the raw material attribute directly correspond to the alternative fuel production pathways analyzed by Albrecht et al. (Albrecht et al., 2017).
Moreover, the raw material transport to the fuel production plant was considered and varied between truck/tank car, overground/underground pipeline, a combination of both, and no transport.
Production facilities require sufficient raw materials for the production of renewable fuels. Depending on the regional availability of raw materials and production capacities, the raw material transportation plays an important role for the production network efficiency. By use of the aforementioned attribute levels, we capture the transportation of biomass and hydrogen. We considered biomass transportation by truck (Ruiz et al., 2015) and hydrogen transportation by tank truck (Zoulias, 2014). A transportation of biomass via pipelines was not considered as this is currently not considered technically feasible. Further, we considered hydrogen transportation by buried and overground pipeline (Reuß et al., 2017).
We chose to include the transport attribute because previous studies in the context of Carbon Capture Storage and Utilization (CCS and CCU) revealed transport of CO2, especially via pipeline, as a crucial aspect for acceptance (Wallquist et al., 2012; Offermann-van Heek et al., 2020). It was distinguished between overground and underground pipelines since in the electricity transmission context, underground cables are more accepted than overhead power lines (Devine-Wright and Batel, 2013; Lienert et al., 2018).
We used a design with a reduced number of stimuli because a fully orthogonal design, in which respondents judge all combinations of the attribute levels, would have included 4 × 4 × 3 × 5 = 240 options (by considering the aforementioned constraints between biomass and pipeline transport, 208 possible options remained). Respondents completed 10 choice tasks in total. That means, they were asked ten times to select their preferred fuel production plant from three randomly developed options that varied in the four attributes. To avoid presenting respondents with unrealistic scenarios, we excluded any combination of the raw material “biomass” with the transport levels “underground/overground pipeline” [this option is technically improbable and only feasible in a few cases from an economic point of view (Muradin and Kulczycka, 2020)]. Figure 2 shows a sample conjoint task. Based on the obtained choice data, Hierarchical Bayes estimation (HB) via Lighthouse Studio (Sawtooth Software, 2020) was used to calculate the preferences for all possible level combinations. The RLH (root likelihood), as measure for the HB model’s goodness of fit, for the reduced CBC design was 0.616, which indicates a sufficient model fit [the random probability for a choice between three simultaneously presented scenarios is 0.33, the maximum possible value is 1 (Orme, 2019)].
3.3 Structure of the questionnaire
The questionnaire consisted of three parts. First, demographic data was collected to screen the participants according to previously defined quotas for age, gender, educational level, and inhabited federal state. Thereafter, the topic of the survey was introduced along general information regarding data use and privacy. After giving their consent and confirming their legal age, participants answered a few general questions concerning their location of residence (e.g., living in the city center or in the countryside and the zip code of their place of residence) as well as attitudinal questions. The latter included items retrieved from Spence et al., 2010, Dunlap, 2000, and Eurobarometer, 2008, to measure people’s attitudes toward climate and environmental change (Dunlap et al., 2000; European Commission, 2008; Spence and Pidgeon, 2010) using four items (Cronbach’s α = 0.86, e.g., “If we continue as we are, we are heading for an environmental catastrophe.”, see Supplementary Table A4 for a list of items used in constructs)1. The subsequent series of questions revolved around car ownership and vehicle use, including information on the vehicle and type of propulsion used, as well as the frequency of usage (before and during the COVID-19 pandemic). In order to avoid forming an unstable pseudo-opinion (de Best-Waldhober et al., 2009) among our participants, who were laypersons regarding alternative fuels and the required production infrastructure, respondents also received a detailed introduction which described the purpose of the study as well as information on alternative fuels and fuel production plants (Supplementary Appendix). Further, they received an introduction into the decision scenario, in which the specific decision criteria under study (transport, location, size, raw material) and the respective levels with their visual representation icons were explained (Supplementary Appendix). The introductory information and the scenario introduction were developed in close cooperation with technical experts and pretested with a sub-sample of laypeople, to ensure factual correctness and comprehensibility. In a previous study (Offermann-van Heek et al., 2020) we demonstrated that laypeople, even with little prior knowledge, are able to evaluate more complex issues in the format of decision choice tasks when using this kind of detailed instructions.
In the second part of the survey, respondents’ attitudes toward alternative fuels were queried using questions by Engelmann et al., 2020, regarding their interest in and knowledge of alternative fuels (Engelmann et al., 2020) (α = 0.83, e.g., “I know a lot about alternative fuels.”). Also, participants were asked to indicate their evaluation of alternative fuel use in road traffic (Linzenich et al., 2019; Engelmann et al., 2020), resulting in a construct for the market acceptance of alternative fuels (α = 0.91, e.g., “I would prefer alternative over conventional fuels when driving.”) based on items by Engelmann et al., 2020 and Linzenich et al., 2019. To gain a deeper understanding of people’s acceptance of alternative fuel production plants, two sets of five questions each were implemented in the survey to assess the general acceptance of production plants using items by Soland et al., 2013, Lienert et al., 2018, and Zaunbrecher et al., 2014 (Soland et al., 2013; Zaunbrecher et al., 2014; Lienert et al., 2018) (α = 0.89, e.g., “I think alternative fuel production plants make sense.”) as well as the local acceptance of production plants, i.e., people’s acceptance of a plant in the vicinity of their place of residence (O’Garra et al., 2008; Soland et al., 2013; Lienert et al., 2018) (α = 0.92, e.g., “I would support the construction of such a facility in my neighborhood.”), measured with items originally used by Soland et al., 2013, Lienert et al., 2018, and O’Garra et al., 2008.
At the beginning of the third part of the survey, the scenario for the subsequent conjoint choice tasks was presented. The scenario asked the participants to imagine the establishment of a production plant for alternative fuels in the vicinity of their homes, followed by separate explanations of the attributes (plant size, location, raw material, and raw material transport) and their respective levels used in the survey (Table 1). Then, the respondents chose the most favourable of three options in ten subsequent choice tasks (Figure 2). The survey closed by thanking respondents for their participation and allowing to leave comments or feedback on the contents and topic of the survey2.
It might seem reasonable at first glance that explaining the conjoint study scenarios in person would help laypeople to better understand the complex decision scenarios. However, there are several reasons that advised us to conduct the study online instead of conducting it in person:
1. Comparability and instruction effect: As the fuel infrastructure configurations had to be randomly generated for each participant, a comparable instruction for each respondent could not have been assured by conducting the study in person. Moreover, explaining the scenarios in person could have evoked an instruction effect and an effect of social desirability.
2. Data protection and anonymity: Conducting the study online helped to ensure the anonymity of the respondents.
3. Complexity of the choice tasks: Choice tasks in a conjoint analysis are cognitively challenging to solve for laypeople since they condense a complex decision space into pictorial and textual data. Providing additional auditory information at the same time might thus overtax laypeople and distract them.
4. Feasibility: Conducting the study online was necessary since the fuel infrastructure configurations had to be randomly generated (it would not have been feasible for participants to evaluate all possible combinations of attribute levels). Also, conducting the study online was required to access a study sample representative for Germany in terms of geographical distribution.
3.4 Sample
Data was collected via an online questionnaire in fall 2020. The respondents were recruited from an online panel and were rewarded by a market research institute for their participation. Quotas were set to aim for a quota-representative sample for Germany in terms of age, gender, education, and federal state. Respondents were informed that their participation in the survey was voluntary and that they could terminate their participation at any point. Participants were also informed about the use of the survey data and that their data was anonymized and only used for scientific purposes. At the beginning of the survey, they actively consented to the use of their data. Prior to conducting the survey, ethical approval by the ethics committee of our university was sought and granted. In total, 387 respondents participated in the survey. After quality control (removing incomplete data sets, speeders, and inconsistent answering patterns), 303 data sets remained for further analysis. We determined the sample size by three different criteria. First of all, in conjoint analysis it is important that the sample size exceeds a critical value, which is determined by the maximum number of attribute levels, the number of choice tasks, and the number of choice options shown per choice task
The mean age of the sample was 45.1 years (SD = 14.6). Participants ranged between 18 and 70 years and there was an equal distribution of women (49.5%) and men (50.5%). The socio-demographic characteristics of the sample are displayed in Table 2. The sample reported a high awareness for the environment and climate change (M = 4.61, SD = 1.10) as well as a positive self-confidence in their own technical competency (M = 4.21, SD = 1.01). In contrast, the awareness and interest in alternative fuels were comparatively lower (M = 3.16, SD = 1.15)3.
With 80.9%, the large majority of the sample owned a car. Table 3 gives an overview of the sample’s self-reported driving behavior before and during the COVID-19 pandemic. As you can see, the driving behavior has not changed significantly: 66.0% of respondents reported that they used a car daily or several times per week before the COVID-19 pandemic compared to 60.1% during the COVID-19 pandemic.
3.5 Data analysis
First, results for general and local acceptance of alternative fuels and their production were analyzed by calculating mean values over the acceptance scales and conducting t-tests to compare acceptance levels.
In a second step, the results of the conjoint analysis were investigated. Part-worth utilities and relative importance scores of the attributes and levels were calculated by HB estimation in Sawtooth Software Lighthouse Studio (Sawtooth Software, 2020). Zero-centered diffs were used so that the sum of part-worth utilities of an attribute adds to zero to enable a direct comparison of part-worth utilities within the attribute. However, zero-centered diffs do not allow comparisons between different attributes because the rescaling is specific to each attribute (Orme, 2019).
Finally, to gain a deeper look into preferences for different location scenarios for alternative fuel plants, a sensitivity analysis was run based on the HB estimations using the Sawtooth Choice Simulator integrated in Lighthouse Studio (Sawtooth Software, 2020). In the sensitivity analysis, different scenarios (in this case different types of location) are defined and then compared. The sensitivity analysis produces preference shares as measure of the relative attractiveness of a scenario compared to the other predefined scenarios, as they indicate the percentage of participants who would opt for each scenario (Orme, 2019). Sensitivity analyses allow the simulation of changes in preference shares if one attribute level changes while all others remain constant to discover possible trade-offs and identify focal points for acceptance that can be considered by planners and decision-makers.
4 Results
In this section, the results for acceptance of alternative fuels and their production as well as laypeople’s preferences for the installation of fuel production plants are presented.
4.1 Acceptance of alternative fuels and their production
First, mean values for the market acceptance of alternative fuels (including attitude towards and willingness to use alternative fuels) and the general and local acceptance of their production were calculated (Figure 3). The general acceptance of alternative fuels and their production was positive and equally high (Malternative fuels = 4.46, SDalternative fuels = 1.07; Mproduction plants = 4.46, SDproduction plants = 0.97). In contrast, the local acceptance of alternative fuel production plants, i.e., the acceptance towards a hypothetical installation of a production plant in a respondent’s neighborhood, was significantly lower than the general acceptance (t (302) = − 12.00, p
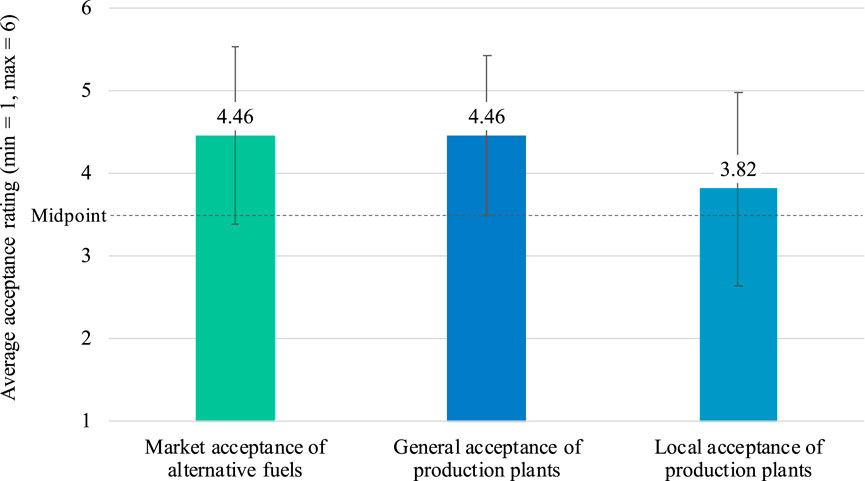
FIGURE 3. Acceptance ratings of alternative fuels and their production plants (n = 303). Mean values on top of the bars, error bars indicate standard deviations.
4.2 Decision criteria and preferences for the planning of alternative fuel production plants
Next, the conjoint data was analyzed to reveal 1) the criteria that are most decision-relevant for laypeople when choosing which alternative fuel production plant should be installed in their neighborhood and 2) the most preferred roll-out scenario for the production of new fuel types.
Using HB estimation, the relative importance scores for the four considered attributes (Figure 4) and the part-worth utilities for all attribute levels (Figure 5) were calculated. The relative importance scores show that the location where the plant would be installed was the most relevant criterion in respondents’ choices for an alternative fuel production plant (39.8%), followed by the raw material transport (28.5%). In contrast, the type of raw material (16.2%) and the plant size (15.5%) played a less prominent role in the choice decisions.
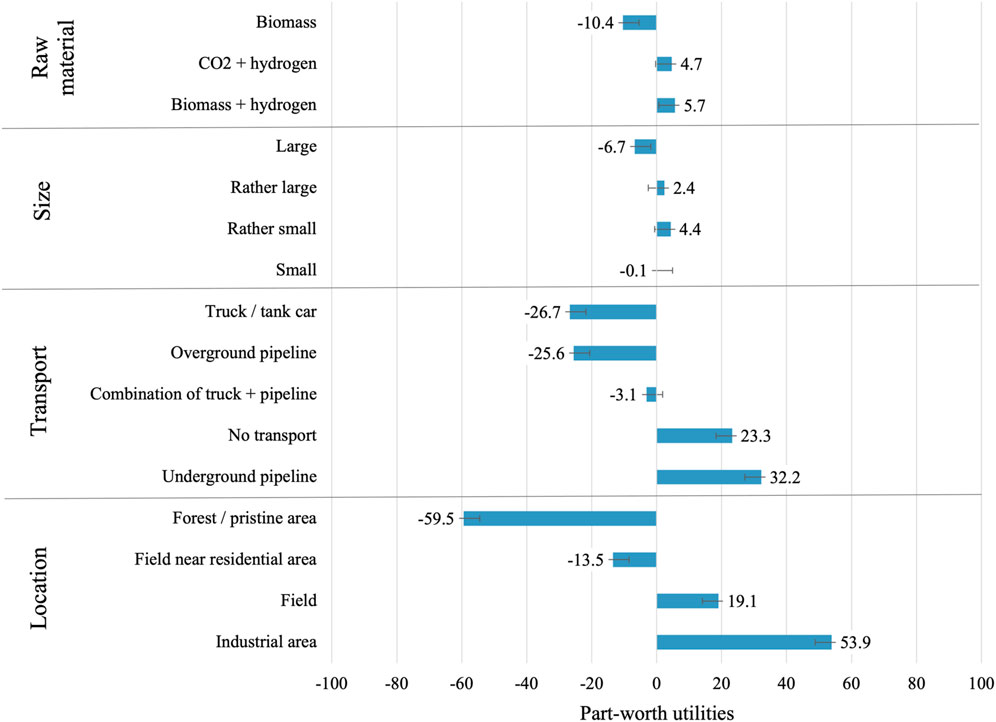
FIGURE 5. Part-worth utilities (zero-centered diffs) for the attribute levels (n = 303). Error bars indicate standard deviations.
Looking at the part-worth utilities for the attribute levels (Figure 5), the most preferred location was an installation in an industrial area (+53.9), followed by the installation on a field (+19.1). Both contributed positively to the preference for the production plant. Installing a fuel production plant on a field with no visibility from a residential area was preferred over construction on a field near a residential area (−13.5). The forest/pristine area was the least accepted option (−59.5). The negative signs indicate that choosing a forest/pristine area or a field near a residential area lowers the preference for the fuel production plant.
For the raw material transport, the underground pipeline obtained the highest preference rating (+32.2) and had a positive influence on the choice of the fuel plant scenario. The same held for no transport (+23.3). In contrast, transport by truck/tank car (−26.7) or an overground pipeline (−25.6) were less accepted and contributed negatively to the decision. The combination of truck/tank car and pipeline ranged in the middle (−3.1).
As raw materials, biomass + hydrogen (+5.7), i.e., bio-hybrid fuel production, and CO2 + hydrogen (+4.7), i.e., CO2-based fuel production, were more preferred than biomass (−10.4), i.e., bio-based fuel production.
Results for plant size showed that medium-sized [rather small (+4.4) and rather large plants (+2.4)] were preferred to small plants (−0.1), and the large plant received the lowest utility score (−6.7).
Overall, the best case scenario from an acceptance perspective would be a rather small fuel plant installed in an industrial area with biomass + hydrogen as raw material. Hydrogen would then be transported by an underground pipeline whereas local resources would be used for biomass. Contrary to this, the worst-case scenario would be installing a large plant in a forest or a similar pristine area using biomass as raw material provided by truck/tank car.
4.3 Towards a decision aid for planners: Sensitivity analysis of location scenarios for alternative fuel plants
The conjoint analysis results unveiled the fuel plant location as the most relevant decision criterion. To dive deeper into the preferences for different location scenarios, a sensitivity analysis was conducted in the Sawtooth Market Simulator (Sawtooth Software, 2020). The sensitivity analysis produced preference shares that allow a comparison of the scenarios in terms of their relative attractiveness. The aim was to identify focal points for the planning of an accepted fuel production plant.
Four scenarios (one for each location type) were defined to investigate how preferences change for the different locations: the industrial area scenario, the field scenario, the field near residential area scenario, and the forest scenario. In the base case, the four scenarios only varied on the attribute location. For all other attributes the best case levels identified in Section 4.2 were chosen (rather small plant using the raw materials biomass + hydrogen with the raw material being provided by an underground pipeline). Looking at the base case, the industrial area scenario obtained the highest preference share (54.4%), followed by the field with 21.9% and the field near residential area with 14.0%. The forest scenario was the least preferred since only 9.7% would have voted for this option.
Starting from the base case scenario, we then varied the levels of the attributes plant size, raw material, and transport while holding the location conditions constant to investigate if there are trade-offs or turning points that make the other three locations ‘competitive’ to an installation in an industrial area. Results of the sensitivity analysis are shown in Figure 6.
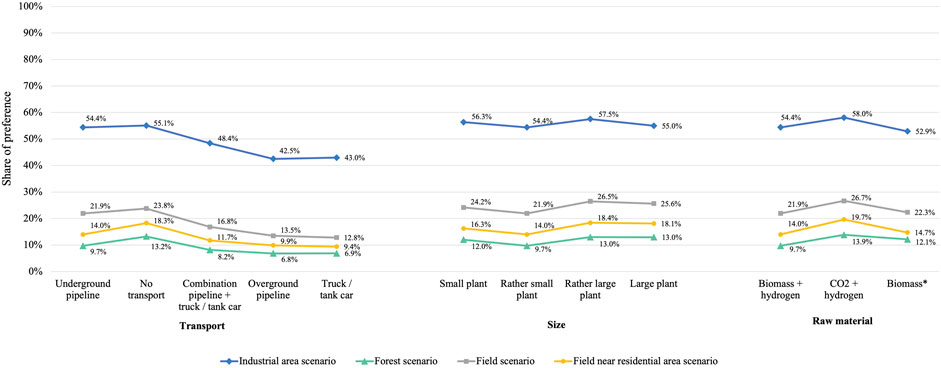
FIGURE 6. Sensitivity analysis for location scenarios (location levels held constant) (n = 303). *Preference shares for biomass are affected by the constraints between biomass and pipeline transport described in Section 3.2.
It can be seen that the industrial area scenario was by far the most preferred option for each transport, size, and raw material configuration. For all single attribute levels, the preference share for the industrial area exceeded that of the other three scenarios. It was always higher than 50%, except for the transport options truck/tank car (43.0%), overground pipeline (42.5%), and the truck-pipeline mix (48.4%).
In contrast, the preference shares for the other three location types were more close to each other. The field scenario was favored over the field near residential area.
For some transport configurations, there were interesting trade-offs between visual impacts and/or environmental effects. When the field scenario was changed to one of the less preferred transport options, e.g., an overground pipeline (13.5%) or trucks/tank cars (12.8%), it became less attractive for respondents than the field near residential area combined with no transport (18.3%) or an underground pipeline (14.0%). Further, the field near residential area with no transport had a higher preference share (18.3%) than the field scenario using a combination of truck/tank car and pipeline (16.8%). However, variations in size and raw material did not lead to a preference shift between the two field scenarios due to their lower decision relevance4.
For any constellation, the forest scenario remained the least preferred location that was only ‘competitive’ to the field or the field near residential area when it required no transport (13.2%). This configuration was favored over the location on a field combined with transport by truck/tank car (preference share for field: 12.8%, preference share for field near residential area: 9.4%). In case of the less favored field near residential area, the overground pipeline (9.9%) and combination of truck/tank car and pipeline (11.7%) were also less attractive than the forest case with no transport. Again, the results revealed a trade-off between landscape impacts and environmental impacts due to traffic. Focusing on the different raw materials, a fuel plant using CO2 + hydrogen in a forest location (13.9%) was as accepted as a plant on a field near a residential area using biomass + hydrogen (14.0%).
The sensitivity analysis showed that no single measure would suffice to make respondents select the other locations over the industrial area. Therefore, a further preference simulation was performed between the industrial area and the field as the second most preferred option. Herein, we investigated whether changing all other attributes to the least preferred option for the industrial area case would lead to a preference shift towards the field scenario consisting of the most preferred levels for the three remaining attributes. Figure 7 shows the trade-offs between the industrial area, where a large plant using biomass is installed and raw material is provided by truck/tank car, and the field, where a rather small plant is installed with biomass + hydrogen transported by an underground pipeline. In this case, the field scenario would be preferred over the industrial area but the industrial area would still have a preference share of 41.7%, although all other attributes were changed to the least preferred levels. This underlines the high relevance of the siting decision for alternative fuel production plants.
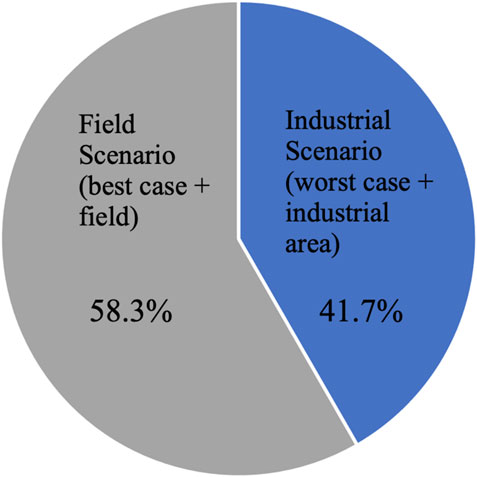
FIGURE 7. Preference simulation for the locations industrial area and field. (The industrial area + worst case scenario was defined as: Industrial area, large plant, biomass, truck/tank car. The field + best case scenario was defined as: Field, rather small plant, biomass + hydrogen, underground pipeline).
5 Discussion
In this chapter, the study findings and their methodological limitations are discussed and future research duties are outlined.
5.1 Acceptance and preferences for alternative fuel production plants
We examined the social acceptance of alternative fuels and their production, and identified decision-relevant criteria for the planning of alternative fuel production plants.
The plant location was most decision-relevant in preferences of laypeople for alternative fuel production plants, followed by raw material transport. In contrast, plant size and type of raw material were less important. The low impact of size, especially compared to transport, is in line with findings from past research on fuel production (Arning et al., 2019; Offermann-van Heek et al., 2020) and wind power (Langer et al., 2017).
For the attribute location, preferences were higher for industrial and agricultural areas than for pristine areas, which is corroborating previous research (Ek and Persson, 2014; Zaunbrecher et al., 2017). For transport options, a lower visual impact (no transport or underground pipeline) was favored over visible transport options (overground pipeline and truck/tank car), which is in line with past research on acceptance of power lines [e.g., (Lienert et al., 2018)].
The best-case scenario (= most preferred fuel plant configuration) from an acceptance point of view was a rather small bio-hybrid fuel production plant in an industrial area with the raw material transported by an underground pipeline. Since biomass cannot be transported through pipelines, the resources must be available locally and must not require extensive transport. This requires to find locations where a complex mix of requirements is fulfilled: The production plant should be installed in an area already used for industrial purposes, where a sufficient amount of biomass is locally available on a long-term basis.
However, this scenario appears less likely from an economic perspective. Economies of scale play an important role in the planning of fuel production facilities, and thus higher capacities are often preferred from an economic point of view. Also, it is more plausible to use regional supplies of hydrogen or to rely on hydrogen transportation by tank truck instead of building expensive underground pipelines for plants of small or rather small capacities. Additionally, the utilization of expensive hydrogen within bio-hybrid fuel production plants results in high operating costs. However, the bio-hybrid fuel pathway shows a higher efficiency at utilizing biomass potentials. In combination with the rather small production capacity, local biomass resources could provide sufficient supply to ensure the utilization of the production facility. In the end, the economics of this pathway depend on the overall system design, the system-wide fuel production quantities, and the local availability of raw materials.
In contrast, the worst case scenario from an acceptance perspective refers to a large biofuel plant in a forest or a similar pristine area, which is provided with raw material by truck/tank car. This option was clearly rejected.
The worst-case scenario could be feasible from an economic perspective. However, the construction of a large production facility that relies on the biofuel production pathway requires the transportation of biomass over long distances. When relying on the biofuel production pathway, a higher number of smaller production facilities could be more favorable to avoid biomass transportation over long distances.
The results of the sensitivity analysis on different location scenarios showed that the installation of a production plant in an industrial area dominates all other locations (field, forest). In public perception, a production scenario in which a less popular installation site has been chosen cannot be compensated or enhanced by other planning characteristics.
To ensure acceptance, it should therefore be assessed if it is possible to install production facilities within existing industrial areas at reasonable costs. However, this requires a detailed analysis of the complete renewable fuel supply chain, as the plant location influences the availability and transportation of raw materials and the distribution of liquid fuels.
Comparing preference shares for the other location types (field, field near residential area, and forest/pristine area) revealed a trade-off between location and transport options. This trade-off can either result from a trade-off between different visual or landscape impacts (overground pipeline vs. an installation in a more natural area) or from weighing of different environmental impacts (negative impacts by installing a plant in a more natural area vs. impacts caused by truck transport and pipeline construction and operation). On the one hand, visual impacts could be the main motive for explaining the differences between a location on a field vs. a location on a field near a residential area. On the other hand, landscape destruction and environmental effects of transport could be the main motives for the low acceptance of the forest scenario.
Previous studies also analyzed the connection between place attachment and acceptance (Devine-Wright, 2011). They pointed out that regional characteristics can be of relevance with regard to pre-existing industry and the knowledge of residents that some level of industrial manufacturing is necessary to produce goods such as energy or fuel (van der Horst, 2007). These underlying issues may also have led to differences in the evaluation of visual impact of plant and transportation modes. Thus, future research needs to look deeper into the underlying motives for these trade-offs.
5.2 Discussion of the methodology
Examining complex human decisions, like the preferences of laypeople for a renewable fuel production network, requires a careful selection of an experimental methodology which is (United Nations, 2015) reliable and valid in terms of methodological quality (IEA, 2020a), manageable in terms of understandability by laypeople, and, finally (IEA, 2020b), representative in terms of sample selection. In the following we discuss the quality and appropriateness of the methodology.
Human decision making for real-world multidimensional ‘problems’ is based on the cognitive and affective balancing of a number of attributes that may contribute differently to the final decision. If we want to learn about the impact of these attributes, we need a method that allows experimental variation of attribute levels and separation of their relative weight on the final decision. One such methodology is conjoint analysis, a well-established and across many application scenarios validated methodology [e.g., (Alriksson and Öberg, 2008; Marshall et al., 2010)]. It has been shown that the choice-based conjoint methodology delivers highly accurate, robust and valid results [e.g., (Baier et al., 2014; Baier et al., 2015)], provided that the number of attributes that must be weighed against each other in the decission making process does not exceed the limits of attention [e.g., (Carmone et al., 1978; Pullman et al., 1999)].
Another crucial factor is the way in which decision tasks are introduced to laypeople, i.e., people that do not have much techno-economic knowledge and expertise. The choice tasks used in this study reflected real-world decisions, therefore the content-related complexity was at an appropriate level. Prior to running the questionnaire, we had several pre-tests in which the semantic transparency of the explanations in combination with the pictorial transparency of the graphics were under study. As found, laypeople had no difficulty understanding instructions and the decision problem. We targeted laypeople in the conjoint tasks as we wanted to collect unbiased opinions of “normal” deciders which are confronted with such infrastructural decisions on a communal level. Finally, the selection of a census-representative sample was essential. In order to avoid potential biases of ad-hoc samples, we collected answers of a census-representative sample of German respondents (representative for age, education, gender, place and region of residence in Germany). Therefore, the findings reflect an unbiased sample of laypeople in Germany. Even though the check of the method and the procedures revealed a high quality of both, data and procedure, still, the question how far the findings can be generalized to other national cultures and countries remains. On the one hand, the findings reflect the well-known NIMBY phenomenon (e.g., (Wallquist et al., 2012; Devine-Wright and Batel, 2013)), which shows that there is a basic generalisability of the findings. On the other hand, the results are not fully transferable, as the question of lay preferences for a renewable fuel production network has not yet been investigated further. Therefore, we think that it could be worthwhile to replicate and cross-check the findings in other national cultures and countries.
5.3 Limitations
Through the current study, valuable insight was gained into the preferences of laypeople regarding alternative fuel production facilities in Germany. However, the study has some methodological limitations that should be addressed in future research.
The findings on the low importance of plant size to the total preference of alternative fuel production plants are in line with previous research [e.g., (Langer et al., 2017; Arning et al., 2019; Offermann-van Heek et al., 2020)]. However, it remains unclear if our depiction of plant size could have influenced the low decision relevance. The depiction used in the survey was tested for comprehensibility and imaginability in pretests with laypeople and was iterated several times. Still, we cannot exclude that the realistic plant size was not fully imaginable for laypeople in a conjoint study with limited space for depiction. This raises the methodological question on how to most appropriately convey the size dimensions of large infrastructure elements in a survey so that respondents can imagine their visual impact. For a particular project, one solution could be to place the fuel plant on a picture of the region where it will be located.
The combination truck/tank car + pipeline was preferred to the single transportation options truck/tank car and overground pipeline. Here, it remains unclear what respondents understood by “combination of truck/tank car + pipeline”. In the questionnaire design, we did not distinguish between an overground and underground pipeline when asking for combinations of transport options. This was done to avoid an overload of the attribute “raw material transport” and a cognitive overtaxing of the respondents. In retrospective we are not able to explain why respondents favored the combination over the single components. One explanation might be that respondents automatically associated their favorite pipeline option (underground) with the word “pipeline”. Thus, the positive preference for the combination of truck + pipeline could refer to the positive evaluation of the underground pipeline. Another explanation could be that respondents assumed a synergy effect between pipeline and truck, which offsets possible disadvantages of each transport type. These explanations should be examined in future research.
5.4 Future research
The results point towards several interesting research topics that should be investigated in future studies.
It was found that fuels made from biomass + hydrogen and CO2 + hydrogen were preferred over fuels produced from biomass. Future research should investigate the underlying mental models and beliefs of this evaluation: Is the more negative evaluation of biofuels based on disadvantages associated with biomass production (e.g., land use or competition to the production of food crops, despite our notion in the instructions that only agricultural residues would be used)? Or is it based on perceived differences in the fuel production process [e.g., concerns about smell and noise, which have been identified as acceptance-relevant in previous research (Arning et al., 2019)]? Or does the low preference refer to reservations regarding biofuels as the ‘end-product’?
The present study focused on the local acceptance of alternative fuel production plants and identified decision-relevant criteria for the local installation of production plants. Since acceptance entails multiple dimensions (Wüstenhagen et al., 2007), future studies should also examine acceptance-relevant parameters for the design of an entire production network for alternative fuels in Germany (socio-political acceptance of alternative fuel production as a general technological concept). On top of that, the study should be replicated in different European countries with different energy supply systems and different energy and mobility cultures to get a Europe-wide perspective. This holds even more since the production and use of alternative fuels will not be limited to Germany, but will play a role on a European or rather a global level.
Finally, research on social acceptance should not stop at its own results. Here, interdisciplinary efforts are needed to bridge the gap to technical research and development: Social acceptance needs to be directly integrated as a decision parameter in alternative fuel supply chain planning as done in previous studies on wind power and CCS (Höfer et al., 2016; d’Amore et al., 2020). Methodologically, future research should take acceptance parameters explicitly into account in the objective function, which allows to analyse the trade-offs between total costs and social acceptance. Such models could provide a valuable decision support tool for political decision-makers and planners helping to achieve a high acceptance towards production networks for alternative fuels in Germany.
6 Conclusion
The present conjoint study investigated the preferences of laypeople for alternative fuel production plants in Germany. The location where the plant is sited was most relevant for the choice of a fuel plant, followed by the raw material transport. In contrast, the raw material used and the size of the plant were less decision-relevant. Laypeople would prefer rather small bio-hybrid fuel production plants that should preferably be installed in an industrial area. Moreover, respondents favored either an underground pipeline to supply the raw materials needed in fuel production or no transport (meaning the raw materials are locally available).
Future research should expand the current perspective by developing a method for directly integrating acceptance as a decision parameter into the supply chain design for alternative fuels. This can be achieved by transferring the acceptance parameters into an objective function and optimizing the fuel production network in terms of costs and acceptance. This would provide an important planning tool for decision makers to find the optimal trade-off between social acceptance and feasibility of planned projects.
The overall findings have a strong implication for policy recommendations in both, managerial and practical implications for policy planners.
6.1 Managerial implications for policy: What planners can learn from laypeople
For planners it might appear counter-intuitive to take laypeople’s view on technology development and infrastructure planning into account due to their anticipated lack of technical expertise. However, there are crucial reasons why the perspective of laypeople should not be dismissed as ‘irrelevant’ or ‘not qualified’ by decision-makers:
Previous experience has shown that publicly contested technology projects were postponed or cancelled (Temper et al., 2020). Thus, it cannot be assumed or anticipated that solutions that are deemed promising from technical perspective (because they are ecologically and economically favorable) will automatically lead to a favorable reception by the public.
Integrating acceptance early in the technological development and planning is not only advantageous for the society as a whole as it leads to more accepted and democratic technology innovations (with ‘democratic’ referring to the equal consideration of the viewpoints of all groups and stakeholders) (Batel et al., 2013) but also for developers and planners because it helps to reduce uncertainty and prevents large investments (and public subsidies) for technology routes with a bleak future due to missing acceptance.
If transferred into an objective function, the acceptance parameters for fuel production plants obtained in this study can be used to simultaneously consider acceptance, costs, and environmental effects in the supply chain optimization for renewable fuels and can thereby not only inform but also facilitate technological development and political decision-making. Herein, it is crucial that political decision-makers acknowledge the lay perspective as legitimate as their own view on technology and risks, and adequately address the concerns and requirements by dialogue and/or by adjusting the development and planning (Wolsink, 2007; Batel et al., 2013; Brunsting et al., 2013). Integrating acceptance should thus not be regarded as an obstacle to innovation or additional requirement to fulfill but rather as a chance to fuel innovations since it can highlight promising technological pathways and uncover new technological applications.
6.2 Practical implications: Guidelines for decision makers
The findings of the study hold the potential to contribute to recommendations for decision makers on a general and a specific level.
Generally, in any infrastructure planning and management of production networks decisions on production facility locations and logistics activities have to be drawn, and the size and production pathway of location of a production facility is of essential impact for all stakeholders involved, be it industry, communes which mostly concentrate on technical feasibility, market demand and, of course, logistics industry calculating transport routes and costs. This includes careful planning of the supply of a whole region or a country with renewable fuel types, the planning of appropriate fuel production plants and a network of transport options for input materials (e.g., biomass), intermediate products (e.g., hydrogen), and fuel as final product. However, this is only one side of the coin. The other side is that land use issues come to the fore, which are also influenced by the positive perception and acceptance by the public. The investigation of social acceptance of fuel innovations is not limited to the end-product of alternative fuels as such, but has to include production and transport infrastructure, as well as different perceptions on land use scenarios, to develop reliable public trust in deciders, technical experts, policy, and governance (Offermann-van Heek et al., 2018; Linzenich et al., 2021). As taken from local protest movements in the context of infrastructure decisions [e.g., (Takahashi and Gaber, 1998; van der Horst, 2007; Moss et al., 2015; Neukirch, 2016)], we know that local residents are not only interested in a timely information and communication strategy about upcoming energy infrastructure [e.g., (Zaunbrecher et al., 2017; Linzenich et al., 2019; Linzenich et al., 2020)], but rather they demand to be integrated into the decision making process [e.g., (Zaunbrecher and Ziefle, 2016; Kluge et al., 2021)]. This integration of the public in early phases of technology development is a necessary requirement of forming shared common values [e.g., (Pelletier et al., 1999)] and also fosters the trust in the transparency of the process, the information, and the public authority [e.g., (Offermann-van Heek et al., 2018; Kluge et al., 2021)]. In addition, one should be aware that the group of laypeople is not limited to the general public, but also includes many people in policy, institutions, and governance, who are in charge of taking such far-reaching infrastructure decisions [e.g., (Offermann-van Heek et al., 2018; Kluge et al., 2021; Simons et al., 2021)]. Therefore it seems to be a mandatory claim of fair decision-making for renewable infrastructure decisions that the lay public is not only transparently informed during the technical development process, but rather, that acceptance factors and public perceptions are studied in line with the conceptualization and the design of infrastructure technology. On a more specific level, the findings of this study provide valuable information for decision-makers in politics and industry. In the following, five key guidelines for the socially accepted planning of renewable fuel production plants are presented:
1. Location planning for fuel production plants should receive special consideration: If possible, production facilities should be located in industrial areas. A rejection of the installation of a fuel production plant is more likely the closer to nature and the less industrialized a location is.
2. Siting a plant in visual proximity to residential areas should be avoided, since a production facility within sight is less preferred than a facility out of sight.
3. The production of e-fuels and bio-hybrids is more popular than the production of pure biofuels.
4. Transportation should either be avoided or underground pipeline transportation should be used5.
5. Since the construction of new pipelines is very planning intensive and expensive, we recommend—from an acceptance perspective—to keep transportation routes short, which requires that raw materials are locally available on a long-term basis.
In designing and implementing a renewable fuel production network, economic criteria also play an important role. Therefore, it is important to analyze the trade-offs between social acceptance and economic aspects like the total costs of constructing and operating a renewable fuel production network. In this study, we propose a conjoint study design describing the preferences for various technically feasible renewable fuel plant locations and configurations. This structure allows direct integration of the results into planning models if each possible plant configuration can be mapped to a specific set of levels in terms of the attributes of the conjoint study. As a result, planning models would be able to not only evaluate renewable fuel production networks in economic terms but also in terms of their social acceptance. This allows for detailed analyses of the trade-offs of economic and social acceptance criteria in designing a complete renewable fuel production network, potentially providing valuable insights into the price of social acceptance.
Respecting these guidelines, political and legal frameworks for the planning and siting of production plants for alternative fuels should ensure that sufficient distances to housing areas are maintained and that an installation in more natural landscapes is prevented. On the contrary, an installation in industrial areas should be fostered and made attractive for investors. As existing industrial areas usually already provide essential infrastructure connections, a siting in industrial areas may reduce the need for new infrastructure and keep costs lower for investors while at the same time reducing the disturbance for residents. On top of that, in pre-existing industrial areas the raw materials needed (biomass, CO2, and hydrogen) can possibly be locally provided by other industrial plants and thereby reduce the need for transport of raw material. Strictly speaking from acceptance perspective, policy measures should prioritize e-fuels and bio-hybrid fuels since they were preferred over biofuels. However, as the reason for the lower preference of biofuels is not completely resolved, information campaigns which objectively inform about the different fuel types and their raw material basis could help laypeople to develop an informed understanding of differences between fuel types (regarding their environmental impacts and usability) and their production.
6.3 Reconciling laypeople’s requirements with experts’ perspective on renewable fuel production
The current study has revealed laypeople’s preferences for renewable fuel production infrastructure. However, it was revealed that the most accepted solution goes hand in hand with high costs indicating possible trade-offs between the optimal production network from acceptance perspective and from economic perspective. Thus, it is important for political decision-making to reconcile the requirements of the public with the expert perspective on fuel production to identify the most feasible and promising production network for renewable fuels. In the following, a decision aid for political decision-making and project planning for specific fuel types (biofuels and e-fuels) is given.
6.3.1 Biofuels
From the perspective of experts, biofuels are less costly to produce than e-fuels and biohybrid fuels. At the same time, they are least accepted by laypeople. However, since the fuel type was less acceptance-relevant than production plant location and raw material transport, the rather low acceptance of biofuel production could be compensated by an acceptance-optimized site selection for the production plants. In case of biofuel production, installing the production plant near industrial infrastructure that produces biomass and hydrogen would be a cost-efficient measure to achieve both, the most preferred plant location (industrial area) and a minimization of transport due to the local availability of raw materials. Because plant size was also of low decision-relevance for laypeople, the plant size could be adjusted in planning to either achieve a more cost-efficient or more accepted solution. This decision should be made based on available budgets and a consultation of the local public living near a proposed fuel production site to meet the specific requirements of the project.
6.3.2 E-fuels
E-fuels are more expensive than biofuels but more accepted by laypeople as shown in the current study. Moreover, they are also promising from expert perspective due to their possibility for reusing CO2 emissions and their lower carbon loss and global warming potential compared to biofuels (König et al., 2019). The high production costs warrant a cost-optimization of the production process and/or subsidies for the production and purchase of e-fuels, which need to be considered by political decision-making. Because of this, a costly transport of raw materials should be avoided, which means that the most accepted transport option for CO2 (underground pipelines) are not economically feasible. Thus, also in the case of e-fuels the local availability of raw materials would be most reasonable, which can be achieved by placing the e-fuel production plants near suitable industrial CO2 sources such as chemical and power plants (von der Assen et al., 2016). Again, this solution would also fulfill laypeople’s requirement for placing the production infrastructure in an existing industrial area.
Data availability statement
The raw data supporting the conclusion of this article will be made available by the authors, without undue reservation.
Ethics statement
The studies involving human participants were reviewed and approved by the Ethikkommission der Fachgruppe “Empirische Humanwissenschaften” der Philosophischen Fakultät der Rheinisch-Westfälischen Technischen Hochschule Aachen. The patients/participants provided their written informed consent to participate in this study.
Author contributions
AL: Conceptualization, Methodology, Validation, Formal analysis, Investigation, Writing—Original Draft, Writing—Review and Editing, Visualization. LE: Conceptualization, Methodology, Validation, Writing—Original Draft, Writing—Review and Editing. KA: Conceptualization, Methodology, Validation, Writing—Original Draft, Writing—Review and Editing. TB: Conceptualization, Methodology, Writing—Original Draft, Writing—Review and Editing. MW: Conceptualization, Methodology, Writing—Original Draft, Writing—Review and Editing. GW: Writing—Original Draft, Writing—Review and Editing, Supervision, Project administration, Funding acquisition. MZ: Data Curation, Writing—Original Draft, Writing—Review and Editing, Supervision, Project administration, Funding acquisition.
Funding
The work was funded by the Deutsche Forschungsgemeinschaft (DFG, German Research Foundation) under Germany’s Excellence Strategy—Cluster of Excellence 2186 “The Fuel Science Center” ID: 390919832.
Acknowledgments
Tremendous thanks go to Felix Glawe for support in study conceptualization and visualization and quality control of datasets. Furthermore, we would like to thank Mona Frank, Verena Grouls, Insa Menzel, and Valerie Michaux for general research support.
Conflict of interest
The authors declare that the research was conducted in the absence of any commercial or financial relationships that could be construed as a potential conflict of interest.
Publisher’s note
All claims expressed in this article are solely those of the authors and do not necessarily represent those of their affiliated organizations, or those of the publisher, the editors and the reviewers. Any product that may be evaluated in this article, or claim that may be made by its manufacturer, is not guaranteed or endorsed by the publisher.
Supplementary material
The Supplementary Material for this article can be found online at: https://www.frontiersin.org/articles/10.3389/fenrg.2022.989553/full#supplementary-material
Footnotes
1The items that were used to calculate a construct are reported. Items with a poorer fit to the overall scale were excluded.
2In order to ensure that the attention of the participants remains constantly high, attention questions were used within the survey.
3All attitudinal variables were measured on a Likert scale from min = 1 to max = 6.
4The low relevance of the attributes size and raw material also explains why the (rather) large plant and the biomass option were among the most preferred in the sensitivity analysis whereas they were the least preferred according to part-worth utilities in Figure 5.
5However, this study cannot address the question of whether new pipeline construction is acceptable or whether existing pipelines should be used.
References
Albrecht, F. G., König, D. H., Baucks, N., and Dietrich, R. U. (2017). A standardized methodology for the techno-economic evaluation of alternative fuels – a case study. Fuel 194 (4), 511–526. doi:10.1016/j.fuel.2016.12.003
Alriksson, S., and Öberg, T. (2008). Conjoint analysis for environmental evaluation. Environ. Sci. Pollut. Res. 15 (3), 244–257. doi:10.1065/espr2008.02.479
Arning, K. (2017). “Conjoint measurement,” in The international encyclopedia of communication research methods (American Cancer Society), 1–10. doi:10.1002/9781118901731.iecrm0040
Arning, K., Offermann-van Heek, J., Sternberg, A., Bardow, A., and Ziefle, M. (2020). Risk-benefit perceptions and public acceptance of carbon capture and utilization. Environ. Innovation Soc. Transitions 35, 292–308. doi:10.1016/j.eist.2019.05.003
Arning, K., Zaunbrecher, B., Borning, M., van Bracht, N., Ziefle, M., and Moser, A. (2019). “Does size matter? Investigating laypeoples’ preferences for roll-out scenarios of alternative fuel production plants,” in Proceedings of the 8th international conference on smart cities and green ICT systems (Heraklion, Crete, Greece: SCITEPRESS - Science and Technology Publications), 91–99. doi:10.5220/0007697100910099
Baier, D., Pełka, M., Rybicka, A., and Schreiber, S. (2015). “Ratings-/rankings-based versus choice-based conjoint analysis for predicting choices,” in Data science, learning by latent structures, and knowledge discovery. studies in classification, data analysis, and knowledge organization. Editors B. Lausen, S. Krolak-Schwerdt, and M. Böhmer (Berlin, Heidelberg: Springer). doi:10.1007/978-3-662-44983-7_18
Baier, D., Pełka, M., Rybicka, A., and Schreiber, S. (2014). Tca/hb compared to cbc/hb for predicting choices among multi-attributed products. Archives Data Sci. 1 (1), 1–11.
Batel, S., Devine-Wright, P., and Tangeland, T. (2013). Social acceptance of low carbon energy and associated infrastructures: A critical discussion 58. Energy Policy 58, 1–5. doi:10.1016/j.enpol.2013.03.018
Bertsch, V., Hall, M., Weinhardt, C., and Fichtner, W. (2016). Public acceptance and preferences related to renewable energy and grid expansion policy: Empirical insights for Germany. Energy 114, 465–477. doi:10.1016/j.energy.2016.08.022
Borning, M., Doré, L., Wolff, M., Walter, J., Becker, T., Walther, G., et al. (2020). Opportunities and challenges of flexible electricity-based fuel production for the European power system. Sustainability 12 (23), 9844. doi:10.3390/su12239844
Brunsting, S., de Best-Waldhober, M., and Terwel, B. W. (2013). ‘I reject your reality and substitute my own!’ why more knowledge about CO2 storage hardly improve public attitudes. Energy Procedia 37, 7419–7427. doi:10.1016/j.egypro.2013.06.684
Bunch, D. S., Bradley, M., Golob, T. F., Kitamura, R., and Occhiuzzo, G. P. (1993). Demand for clean-fuel vehicles in California: A discrete-choice stated preference pilot project, special issue energy and global climate change. Transp. Res. Part A Policy Pract. 27 (3), 237–253. doi:10.1016/0965-8564(93)90062-P
Büttner, G., and Kosztra, B. (2011). CLC2018 technical guidelines. Available at: https://land.copernicus.eu/user-corner/technical-library/clc2018technicalguidelines_final.pdf.
Byun, H., Shin, J., and Lee, C.-Y. (2018). Using a discrete choice experiment to predict the penetration possibility of environmentally friendly vehicles. Energy 144, 312–321. doi:10.1016/j.energy.2017.12.035
Carmone, F. J., Green, P. E., and Jain, A. K. (1978). Robustness of conjoint analysis: Some Monte Carlo results. J. Mark. Res. 15 (2), 300–303. doi:10.2307/3151267
Chin, H.-C., Choong, W.-W., Alwi, S. R. W., and Mohammed, A. H. (2014). Issues of social acceptance on biofuel development. J. Clean. Prod. 71, 30–39. doi:10.1016/j.jclepro.2013.12.060
d’Amore, F., Lovisotto, L., and Bezzo, F. (2020). Introducing social acceptance into the design of CCS supply chains: A case study at a European level. J. Clean. Prod. 249, 119337. doi:10.1016/j.jclepro.2019.119337
de Best-Waldhober, M., Daamen, D., and Faaij, A. (2009). Informed and uninformed public opinions on co2 capture and storage technologies in the Netherlands. Int. J. Greenh. Gas Control 3 (3), 322–332. doi:10.1016/j.ijggc.2008.09.001
Dethloff, C. (2004). Akzeptanz und Nicht-Akzeptanz von technischen Produktinnovationen [Acceptance and non-acceptance of technical product innovations]. Berlin, Germany: Pabst Science Publication.
Devine-Wright, P., and Batel, S. (2013). Explaining public preferences for high voltage pylon designs: An empirical study of perceived fit in a rural landscape. Land Use Policy 31, 640–649. doi:10.1016/j.landusepol.2012.09.011
Devine-Wright, P. (2011). Place attachment and public acceptance of renewable energy: A tidal energy case study. J. Environ. Psychol. 31 (4), 336–343. doi:10.1016/j.jenvp.2011.07.001
Dunlap, R. E., Van Liere, K. D., Mertig, A. G., and Jones, R. E. (2000). New trends in measuring environmental attitudes: Measuring endorsement of the new ecological paradigm: A revised NEP scale. J. Soc. Issues 56, 425–442. doi:10.1111/0022-4537.00176
Ek, K., and Persson, L. (2014). Wind farms — where and how to place them? A choice experiment approach to measure consumer preferences for characteristics of wind farm establishments in Sweden. Ecol. Econ. 105, 193–203. doi:10.1016/j.ecolecon.2014.06.001
Engelmann, L., Arning, K., Linzenich, A., and Ziefle, M. (2020). Risk assessment regarding perceived toxicity and acceptance of carbon dioxide-based fuel by laypeople for its use in road traffic and aviation. Front. Energy Res. 8, 579814. doi:10.3389/fenrg.2020.579814
European Commission (2008). Europeans’ attitudes towards climate change. Special Eurobarometer 300/Wave 69.2. https://europa.eu/eurobarometer/surveys/detail/2592.
Fortenbery, T. R., Deller, S. C., and Amiel, L. (2013). The location decisions of biodiesel refineries. Land Econ. 89 (1), 118–136. doi:10.3368/le.89.1.118
Green, P. E., and Srinivasan, V. (1978). Conjoint analysis in consumer research: Issues and outlook. J. Consumer Res. 5 (2), 103–123. doi:10.1086/208721
Hackbarth, A., and Madlener, R. (2016). Willingness-to-pay for alternative fuel vehicle characteristics: A stated choice study for Germany. Transp. Res. Part A Policy Pract. 85, 89–111. doi:10.1016/j.tra.2015.12.005
Haddad, M. A., Taylor, G., and Owusu, F. (2010). Locational choices of the ethanol industry in the midwest corn belt. Econ. Dev. Q. 24 (1), 74–86. doi:10.1177/0891242409347722
Höfer, T., Sunak, Y., Siddique, H., and Madlener, R. (2016). Wind farm siting using a spatial analytic hierarchy process approach: A case study of the Städteregion Aachen. Appl. Energy 163, 222–243. doi:10.1016/j.apenergy.2015.10.138
IEA (2020a). Oil demand in the long-distance transport sector in the stated policies scenario, 2019-2030. Available at: https://www.iea.org/data-and-statistics/charts/oil-demand-in-the-long-distance-transport-sector-in-the-stated-policies-scenario-2019-2030 (Accessed 03 08, 2021).
IEA (2020b). Oil demand for passenger vehicles in the stated policies scenario, 2019-2030. Available at: https://www.iea.org/data-and-statistics/charts/oil-demand-for-passenger-vehicles-in-the-stated-policies-scenario-2019-2030 (Accessed 03 08, 2021).
IEA (2020c). Global energy demand and CO2 emissions trends in the Stated Policies Scenario, 2019–2030. Available at: https://www.iea.org/data-and-statistics/charts/global-energy-demand-and-co2-emissions-trends-in-the-stated-policies-scenario-2019-2030 (Accessed 03 08, 2021).
Johnson, R. J., and Scicchitano, M. J. (2012). Don’t call me nimby: Public attitudes toward solid waste facilities. Environ. Behav. 44 (3), 410–426. doi:10.1177/0013916511435354
Kluge, J., Offermann-van Heek, J., and Ziefle, M. (2021). How will i know about local energy projects? A conjoint-analysis of communication preferences in Germany. Energy Res. Soc. Sci. 75, 102016. doi:10.1016/j.erss.2021.102016
Knapp, L., and Ladenburg, J. (2015). How spatial relationships influence economic preferences for wind power—A review. Energies 8 (6), 6177–6201. doi:10.3390/en8066177
König, A., Ulonska, K., Mitsos, A., and Viell, J. (2019). Optimal applications and combinations of renewable fuel production from biomass and electricity. Energy fuels 33 (2), 1659–1672. doi:10.1021/acs.energyfuels.8b03790
Kontogianni, A., Tourkolias, C., Skourtos, M., and Damigos, D. (2014). Planning globally, protesting locally: Patterns in community perceptions towards the installation of wind farms. Renew. Energy 66, 170–177. doi:10.1016/j.renene.2013.11.074
Langer, K., Decker, T., and Menrad, K. (2017). Public participation in wind energy projects located in Germany: Which form of participation is the key to acceptance? Renew. Energy 112, 63–73. doi:10.1016/j.renene.2017.05.021
Le Quere, C., Jackson, R., Jones, M., Smith, A., Abernethy, S., Andrew, R., et al. (2020). Temporary reduction in daily global co2 emissions during the Covid-19 forced confinement. Nat. Clim. Chang. 10, 647–653. doi:10.1038/s41558-020-0797-x
Liebe, U., and Dobers, G. M. (2019). Decomposing public support for energy policy: What drives acceptance of and intentions to protest against renewable energy expansion in Germany? Energy Res. Soc. Sci. 47, 247–260. doi:10.1016/j.erss.2018.09.004
Lienert, P., Sütterlin, B., and Siegrist, M. (2018). Public acceptance of high-voltage power lines: The influence of information provision on undergrounding. Energy Policy 112, 305–315. doi:10.1016/j.enpol.2017.10.025
Linzenich, A., Arning, K., Bongartz, D., Mitsos, A., and Ziefle, M. (2019). What fuels the adoption of alternative fuels? Examining preferences of German car drivers for fuel innovations. Appl. Energy 249, 222–236. doi:10.1016/j.apenergy.2019.04.041
Linzenich, A., Arning, K., and Ziefle, M. (2021). Acceptance of energy technologies in context: Comparing laypeople’s risk perceptions across eight infrastructure technologies in Germany. Energy Policy 152, 112071. doi:10.1016/j.enpol.2020.112071
Linzenich, A., Zaunbrecher, B. S., and Ziefle, M. (2020). Risky transitions?” risk perceptions, public concerns, and energy infrastructure in Germany. Energy Res. Soc. Sci. 68, 101554. doi:10.1016/j.erss.2020.101554
Malhotra, N. K. (1982). Structural reliability and stability of nonmetric conjoint analysis. J. Mark. Res. 19 (2), 199–207. doi:10.2307/3151620
Marshall, D., Bridges, J. F. P., Hauber, B., Cameron, R., Donnalley, L., Fyie, K., et al. (2010). Conjoint analysis applications in health — how are studies being designed and reported? The Patient: Patient-Centered Outcomes Research 3 (4), 249–256. doi:10.2165/11539650-000000000-00000
Moss, T., Becker, S., and Naumann, M. (2015). Whose energy transition is it, anyway? Organisation and ownership of the Energiewende in villages, cities and regions. Local Environ. 20 (12), 1547–1563. doi:10.1080/13549839.2014.915799
Muradin, M., and Kulczycka, J. (2020). The identification of hotspots in the bioenergy production chain. Energies 13 (21), 5757. doi:10.3390/en13215757
Neukirch, M. (2016). Protests against German electricity grid extension as a new social movement? A journey into the areas of conflict. Energy sustain. Soc. 6 (1), 4–15. doi:10.1186/s13705-016-0069-9
Nguyen, X. P., Hoang, A. T., Ölçer, A. I., and Huynh, T. T. (2021). Record decline in global co2 emissions prompted by Covid-19 pandemic and its implications on future climate change policies. Energy Sources, Part A Recovery, Util. Environ. Eff., 1–4. doi:10.1080/15567036.2021.1879969
Offermann-van Heek, J., Arning, K., Linzenich, A., and Ziefle, M. (2018). Trust and distrust in carbon capture and utilization industry as relevant factors for the acceptance of carbon-based products. Front. Energy Res. 6, 73. doi:10.3389/fenrg.2018.00073
Offermann-van Heek, J., Arning, K., Sternberg, A., Bardow, A., and Ziefle, M. (2020). Assessing public acceptance of the life cycle of CO2-based fuels: Does information make the difference? Energy Policy 143, 111586. doi:10.1016/j.enpol.2020.111586
O’Garra, T., Mourato, S., and Pearson, P. (2008). Investigating attitudes to hydrogen refuelling facilities and the social cost to local residents. Energy Policy 36 (6), 2074–2085. doi:10.1016/j.enpol.2008.02.026
Orme, B. K. (2019). Getting started with conjoint analysis: Strategies for product design and pricing research. 4th Edition. Madison, Wis: Research Pub LLC.
Pelletier, D., Kraak, V., McCullum, C., Uusitalo, U., and Rich, R. (1999). The shaping of collective values through deliberative democracy: An empirical study from New York’s north country. Policy Sci. 32 (2), 103–131. doi:10.1023/a:1004641300366
Pullman, M. E., Dodson, K. J., and Moore, W. L. (1999). A comparison of conjoint methods when there are many attributes. Mark. Lett. 10 (2), 125–138.
Rao, V. R. (2014). Applied conjoint analysis. Berlin Heidelberg: Springer-Verlag. doi:10.1007/978-3-540-87753-0
Reuß, M., Grube, T., Robinius, M., Preuster, P., Wasserscheid, P., and Stolten, D. (2017). Seasonal storage and alternative carriers: A flexible hydrogen supply chain model. Appl. Energy 200, 290–302. doi:10.1016/j.apenergy.2017.05.050
Ruiz, P., Nijs, W., Tarvydas, D., Sgobbi, A., Zucker, A., Pilli, R., et al. (2019). Enspreso - an open, EU-28 wide, transparent and coherent database of wind, solar and biomass energy potentials. Energy Strategy Rev. 26, 100379. doi:10.1016/j.esr.2019.100379
Ruiz, P., Sgobbi, A., Nijs, W., Thiel, C., Dalla Longa, F., Kober, T., et al. (2015). Alterra, the JRC-EU-TIMES model - bioenergy potentials for EU and neighbouring countries. Available at: https://publications.europa.eu/en/publication-detail/-/publication/b291a7c5-a878-11e5-b528-01aa75ed71a1/language-en.
Santibañez-Aguilar, J. E., González-Campos, J. B., Ponce-Ortega, J. M., Serna-González, M., and El-Halwagi, M. M. (2014). Optimal planning and site selection for distributed multiproduct biorefineries involving economic, environmental and social objectives. J. Clean. Prod. 65, 270–294. doi:10.1016/j.jclepro.2013.08.004
Scheer, D., Konrad, W., and Wassermann, S. (2017). The good, the bad, and the ambivalent: A qualitative study of public perceptions towards energy technologies and portfolios in Germany. Energy Policy 100, 89–100. doi:10.1016/j.enpol.2016.09.061
Schulte, I., Hart, D., and Van der Vorst, R. (2004). Issues affecting the acceptance of hydrogen fuel. Int. J. Hydrogen Energy 29 (7), 677–685. doi:10.1016/j.ijhydene.2003.09.006
Schweizer-Ries, P. (2008). Energy sustainable communities: Environmental psychological investigations. Energy Policy 36 (11), 4126–4135. doi:10.1016/j.enpol.2008.06.021
Selka, S., Baier, D., and Kurz, P. (2014). “The validity of conjoint analysis: An investigation of commercial studies over time,” in Data analysis, machine learning and knowledge discovery. Studies in classification, data analysis, and knowledge organization. Editors M. Spiliopoulou, L. Schmidt-Thieme, and R. Janning (Cham: Springer). doi:10.1007/978-3-319-01595-8_25
Simons, L., Engelmann, L., Arning, K., and Ziefle, M. (2021). Two sides of the same coin—Explaining the acceptance of co2-based fuels for aviation using pls-sem by considering the production and product evaluation. Front. Energy Res. 9, 491. doi:10.3389/fenrg.2021.742109
Smith, L. V., Tarui, N., and Yamagata, T. (2021). Assessing the impact of Covid-19 on global fossil fuel consumption and co2 emissions. Energy Econ. 97, 105170. doi:10.1016/j.eneco.2021.105170
Soland, M., Steimer, N., and Walter, G. (2013). Local acceptance of existing biogas plants in Switzerland. Energy Policy 61, 802–810. doi:10.1016/j.enpol.2013.06.111
Spence, A., and Pidgeon, N. (2010). Framing and communicating climate change: The effects of distance and outcome frame manipulations. Glob. Environ. Change 20 (4), 656–667. doi:10.1016/j.gloenvcha.2010.07.002
Stančin, H., Mikulčić, H., Wang, X., and Duić, N. (2020). A review on alternative fuels in future energy system. Renew. Sustain. Energy Rev. 128, 109927. doi:10.1016/j.rser.2020.109927
Takahashi, L. M., and Gaber, S. L. (1998). Controversial facility siting in the urban environment: Resident and planner perceptions in the United States. Environ. Behav. 30 (2), 184–215. doi:10.1177/0013916598302004
Tanaka, M., Ida, T., Murakami, K., and Friedman, L. (2014). Consumers’ willingness to pay for alternative fuel vehicles: A comparative discrete choice analysis between the us and Japan. Transp. Res. Part A Policy Pract. 70, 194–209. doi:10.1016/j.tra.2014.10.019
Temper, L., Avila, S., Bene, D. D., Gobby, J., Kosoy, N., Billon, P. L., et al. (2020). Movements shaping climate futures: A systematic mapping of protests against fossil fuel and low-carbon energy projects. Environ. Res. Lett. 15 (12), 123004. doi:10.1088/1748-9326/abc197
Terwel, B. W., and Daamen, D. D. (2012). Initial public reactions to carbon capture and storage (CCS): Differentiating general and local views. Clim. Policy 12 (3), 288–300. doi:10.1080/14693062.2011.637819
Tigges, L. M., and Noble, M. (2012). Getting to yes or bailing on no: The site selection process of ethanol plants in Wisconsin. Rural. Sociol. 77 (4), 547–568. doi:10.1111/j.1549-0831.2012.00092.x
Tollefson, J. (2021a). Covid curbed carbon emissions in 2020 – but not by much. Nature 589 (7842), 343. doi:10.1038/d41586-021-00090-3
Tollefson, J. (2021b). Carbon emissions rapidly rebounded following Covid pandemic dip. Nature. doi:10.1038/d41586-021-03036-x
United Nations (2015). Paris agreement. Available at: https://treaties.un.org/Pages/ViewDetails.aspx?src=TREA TY& mtdsg_no=XXVII-7-d& chapter=27& clang=_en (Accessed 03 08, 2021).
van der Horst, D. (2007). Nimby or not? Exploring the relevance of location and the politics of voiced opinions in renewable energy siting controversies. Energy Policy 35 (5), 2705–2714. doi:10.1016/j.enpol.2006.12.012
von der Assen, N., Müller, L. J., Steingrube, A., Voll, P., and Bardow, A. (2016). Selecting co2 sources for co2 utilization by environmental-merit-order curves. Environ. Sci. Technol. 50 (3), 1093–1101. doi:10.1021/acs.est.5b03474
Wallquist, L., Seigo, S. L., Visschers, V. H. M., and Siegrist, M. (2012). Public acceptance of CCS system elements: A conjoint measurement. Int. J. Greenh. Gas Control 6, 77–83. doi:10.1016/j.ijggc.2011.11.008
Wolsink, M. (2007). Wind power implementation: The nature of public attitudes: Equity and fairness instead of ‘backyard motives’. Renew. Sustain. Energy Rev. 11 (6), 1188–1207. doi:10.1016/j.rser.2005.10.005
Wüstenhagen, R., Wolsink, M., and Bürer, M. J. (2007). Social acceptance of renewable energy innovation: An introduction to the concept. Energy Policy 35 (5), 2683–2691. doi:10.1016/j.enpol.2006.12.001
Zaunbrecher, B. S., Kowalewski, S., and Ziefle, M. (2014). “The willingness to adopt technologies: A cross-sectional study on the influence of technical self-efficacy on acceptance,” in Human-computer interaction. Applications and services, lecture notes in computer science. Editor Kurosu M. (Cham: Springer International Publishing), 764–775. doi:10.1007/978-3-319-07227-2_73
Zaunbrecher, B. S., Linzenich, A., and Ziefle, M. (2017). A mast is a mast is a mast...? Comparison of preferences for location-scenarios of electricity pylons and wind power plants using conjoint analysis. Energy Policy 105, 429–439. doi:10.1016/j.enpol.2017.02.043
Zaunbrecher, B. S., and Ziefle, M. (2016). Integrating acceptance-relevant factors into wind power planning: A discussion. Sustain. Cities Soc. 27, 307–314. doi:10.1016/j.scs.2016.08.018
Zoulias, M. (2014). Energy technology system analysis programme: Hydrogen production & distribution, technology brief P12. Available at: www.etsap.org.
Keywords: acceptance, preferences, conjoint analysis, renewable fuels, fuel production plants
Citation: Linzenich A, Engelmann L, Arning K, Becker T, Wolff M, Walther G and Ziefle M (2022) On the road to sustainable transport: Acceptance and preferences for renewable fuel production infrastructure. Front. Energy Res. 10:989553. doi: 10.3389/fenrg.2022.989553
Received: 08 July 2022; Accepted: 15 August 2022;
Published: 14 September 2022.
Edited by:
Ao Xia, Chongqing University, ChinaReviewed by:
Andrés Armando Mendiburu Zevallos, Federal University of Rio Grande do Sul, BrazilEric Okonkwo, Hamad bin Khalifa University, Qatar
Copyright © 2022 Linzenich, Engelmann, Arning, Becker, Wolff, Walther and Ziefle. This is an open-access article distributed under the terms of the Creative Commons Attribution License (CC BY). The use, distribution or reproduction in other forums is permitted, provided the original author(s) and the copyright owner(s) are credited and that the original publication in this journal is cited, in accordance with accepted academic practice. No use, distribution or reproduction is permitted which does not comply with these terms.
*Correspondence: Anika Linzenich, bGluemVuaWNoQGNvbW0ucnd0aC1hYWNoZW4uZGU=