- 1Department of Space, Earth and Environment, Chalmers University of Technology, Göteborg, Sweden
- 2Department of Energy Technology, KTH Royal Institute of Technology, Stockholm, Sweden
- 3Department of Industrial Economics and Management, KTH Royal Institute of Technology, Stockholm, Sweden
- 4Stockholm Exergi AB, Stockholm, Sweden
The upscaling of novel carbon dioxide removal, such as bioenergy carbon capture and storage (BECCS), to gigatonne scales is an urgent priority if global warming is to be limited to well below 2 °C. But political, economic, social, technological, environmental and regulatory uncertainty permeates BECCS projects and deters investors. To address this, we explore options to improve the robustness of BECCS deployment strategies in the face of multi-dimensional uncertainties. We apply Dynamic Adaptive Planning (DAP) through expert interviews and Robust Decision Making (RDM) through exploratory modelling, two decision making under deep uncertainty methods, to the case of Stockholm Exergi, an early mover aiming to deploy BECCS at a combined heat and power plant in the capital of Sweden. The main contributions of the research are to 1) illustrate how a quantification of robustness against uncertainty can support an investment decision to deploy BECCS 2) comprehensively cover uncertain vulnerabilities and opportunities of deploying BECCS, and 3) identify critical scenarios and adaptations to manage these uncertainties. The main conclusions are: investing in BECCS is relatively robust if assessing performance across many scenarios and if comparing the worst-cases of either investing, or not doing so. Not investing could miss out on up to € 3.8 billion in terms of net present value. The critical uncertainties of BECCS can be managed by strengthening biomass sustainability strategies and by gaining support for negative emission trading regulation on carbon markets, e.g., voluntary or Paris Agreement Article 6. Even in vulnerable scenarios of average electricity prices above 82 €/MWh, if trading regulation is implemented before 2030 and if negative emission prices exceed 151 €/CO2, investing in BECCS performs better than not doing so in 96% of cases. We suggest that facility-level parameters and cost-reductions are of little importance for BECCS investments and upscaling. It is regulatory certainty of operating revenues, e.g., through negative emission markets, that needs to be provided by policymakers.
1 Introduction
The recent IPCC assessment shows that carbon dioxide removal (CDR) technologies and negative emissions (NEs) are becoming increasingly important for limiting global warming to well below 2 °C (IPCC, 2023). CDRs can compensate for sectors with hard-to-abate emissions, which is especially important to achieve net-zero greenhouse gas emissions by 2050, or to undo any temperature overshoot from exhausting the 2 °C carbon budget. Some prominent examples of CDRs include afforestation/reforestation, soil carbon sequestration, biochar, bioenergy carbon capture and storage (BECCS), direct air carbon capture and storage (DACCS) and enhanced weathering. However, the IPCC conclude that the gap between deployed and projected CDR capacity is considerable, and rapid upscaling is necessary. This notion has been comprehensively assessed by Smith et al. (2023), who also conclude that CDR upscaling is an urgent priority that requires accelerated efforts, not in the least for BECCS. They estimate current BECCS projects to remove around 1.82 MtCO2 p.a., which should be compared with the gigatonne capacities in many low-temperature scenarios of the IPCC. “Novel” CDR, such as BECCS, may need to grow by a factor of 1,300 by mid-century.
Considering accelerated BECCS deployment specifically, many researchers have highlighted the gap between scenario projections and real-life projects and suggested potential pathways forward, e.g., Fridahl et al. (2018) and Fuss and Johnsson (2021). Some notable examples of such BECCS projects and actors include: Drax Power Station (Drax Global, 2022), Summit Carbon Solutions (2023), Amager Bakke (ARC, 2023) and Asnæs and Avedøre power stations (Ørsted, 2023). This study contributes by exploring the bottom-up perspective of another early BECCS mover: the Swedish energy utility company Stockholm Exergi (Stockholm Exergi AB, 2020). The study focuses the analysis of hands-on activities and investment decisions of actors addressing the CDR gap, underlined by the IPCC.
BECCS can be described as a chain of integrated technological processes that captures and stores biogenic CO2 emissions, in connection with conversion of biomass (IEA, 2022a). How BECCS is implemented is highly context dependent, but it typically includes the steps listed below (Möllersten and Naqvi, 2022):
1. Biomass production, which removes atmospheric CO2 during growth of the biomass.
2. Conversion of biomass into electricity, heat or fuels.
3. Application of a CO2 capture technology, to generate a CO2 stream of high purity.
4. CO2 compression and transportation to a storage site.
5. CO2 injection into a suitable geological formation for long-term storage.
When the CO2 is intentionally removed from the atmosphere and permanently stored negative emissions (NEs) are supposedly achieved (Minx et al., 2018). This reduces the atmospheric CO2 concentration and counteracts climate change, if the complete value-chain stores more CO2 than what is emitted. These conditions apply for the BECCS project of Stockholm Exergi (Hammar and Levihn, 2020; Stockholm Exergi AB, 2020), which is used as a case study in this work. The case is of particular interest as Stockholm Exergi see themselves as BECCS frontrunners, aiming to serve as an example for other carbon capture and storage (CCS) actors to follow suit, and to accelerate the development of a new market for NEs. The project has been discussed in the literature as an early mover (Bellamy and Geden, 2019; Fuss and Johnsson, 2021), and was in 2022 selected to receive funding by the European Union’s Innovation Fund (European Commission, 2022).
In Section 3, the Stockholm Exergi case is described further as it serves as a basis to analyse the many uncertainties of BECCS deployment more generally. As will be illustrated below, there is potential in systematically and comprehensively analysing these uncertainties. Such an analysis could help address the implementation gap between BECCS capacities in IPCC scenarios and actual BECCS projects, or showcase the infeasibility of such scenarios and the necessity for scaling other CDR solutions. Bevan (2022) have presented different definitions of uncertainty in the literature. Many of these could be valid, but the definition used in this article is the one below:
Uncertainty is “… limited (incomplete or imperfect) knowledge and information about current or future environmental, social, economic, technological, political and institutional conditions, states and outcomes and the implications or consequences of these current or future conditions, states and outcomes” (Brouwer and De Blois, 2008).
Applying this definition, many multi-dimensional uncertainties surrounding BECCS deployment, i.e., economic, technological, social, etc., can be identified from the literature. Levihn et al. (2019) studied the Stockholm Exergi case and found that uncertain policy support for BECCS makes the business model ambiguous. Costs related to the capture unit and storage infrastructure were also identified as uncertain. Other uncertainties include, e.g., the design of economic incentives, legal and acceptance barriers and the consequences of potentially increased biomass usage (SOU, 2020:4). In addition, Lefvert et al. (2022) identified companies’ investment cycles and CO2 storage capacity bottlenecks as possible BECCS barriers, to name a few examples. Rodriguez et al. (2021) highlighted the lack of reliable customer demand for NEs, the uncertainty of companies’ perceived responsibility to mitigate climate change, and the uncertain effects on process efficiency when deploying BECCS. Fuss and Johnsson (2021) found uncertainty in the future use of biomass, in social preferences for BECCS, the accounting rules of NEs and the lack of coherence between pricing fossil fuel emissions and NEs. Möllersten et al. (2021) highlighted, among other uncertainties, policy incentives, regulatory barriers, and the challenge in developing the complete BECCS value chain jointly. Moreover, Minx et al. (2018) raises the issues of early-stage incentives for BECCS, the ethical and policy discourses surrounding bioenergy usage, and the overall ability of BECCS to be deployed in-line with top-down modelling expectations. Section 2.1 elaborates further on current research on the policy incentives for BECCS.
A case can be made for managing the uncertainties of BECCS deployment by applying decision making under deep uncertainty (DMDU) approaches (Marchau et al., 2019), as such approaches improve strategies’ adaptivity and resilience to uncertainty. Deep uncertainty is when analysts and decisionmakers do not know or cannot agree on “....” i) The external context of the system, ii) how the system works and its boundaries, and/or iii) the outcomes of interest from the system and/or their relative importance” (Lempert et al., 2003; Marchau et al., 2019). Sovacool (2021) mentions the deep uncertainties surrounding CDR cost profiles and performance attributes, and Workman et al. (2021) made explicit the need to consider deep uncertainty in CDR strategies to avoid overreliance on BECCS in mitigation strategies.
To the authors’ knowledge, and despite the potential, few studies have been made that explicitly apply DMDU methods to BECCS deployment. One such study was performed by Lindroos et al. (2019) which aimed to develop a robust strategy for BECCS in Helsinki. Within DMDU, robustness refers to the ability of a strategy to perform well, relative to alternatives, across a wide range of possible futures (Lempert et al., 2006). The Helsinki study focused on the techno-economic dimensions of BECCS, but as was illustrated above, more uncertainty dimensions would need to be considered to support accelerated and robust BECCS deployment strategies broadly.
Given the implementation gap of BECCS to deliver on net-zero targets, and the uncertainties surrounding BECCS deployment, the overall objective of this research is to explore options to improve the robustness of BECCS deployment strategies. The explicit research questions supporting this objective are:
1) How could an investment decision to deploy BECCS be supported by a quantification of robustness?
2) What are the major and uncertain vulnerabilities and opportunities of deploying BECCS?
3) How can adaptations be made to address the major and uncertain vulnerabilities and opportunities of deploying BECCS?
The study answers these research questions by applying quantitative and qualitative DMDU methods to the specific case of Stockholm Exergi’s BECCS project. As DMDU approaches are shaped by the stakeholders involved, potentially biased findings from this case are critically discussed where appropriate. While recognizing this possible flaw, the case study provides an opportunity to appropriately represent the organizational context of a deeply uncertain decision, which has been raised as a limitation to DMDU (Stanton and Roelich, 2021).
After this introductory Section 1, where the role and uncertainties of BECCS, the potential of applying DMDU and the research questions were outlined, the article is structured as follows: Section 2 covers the materials and methods used and findings from the narrative review, and puts emphasis on the uncertain policy drivers for BECCS, the Stockholm Exergi case study and the DMDU methods and data. Section 3 covers first the qualitative, then the quantitative results. Section 4 covers a discussion where the study is recapped, put into its research context, and the findings are critically discussed for each research question. Section 5 covers the conclusions of the study.
2 Materials and methods
This section first describes how DMDU was integrated with the methodology. Then, the policy drivers for BECCS are conceptualized, and the case study of Stockholm Exergi’s BECCS project is described. Lastly, the implementation of Dynamic Adaptive Planning (DAP) and Robust Decision Making (RDM) methods is described.
2.1 Methodology
The methodology applied was both linear and iterative, as illustrated below in Figure 1. DMDU methods generally consist of a frame, explore and choose phase (Marchau et al., 2019). The framing phase involved formulating the triggering issue, i.e., the BECCS deployment gap, and specifying the system, objectives and potential strategies, grounded in a case study of Stockholm Exergi. A literature study was performed simultaneously and in a narrative review style, which was suitable given the exploratory research objective (Sovacool et al., 2018). The review included both peer-reviewed and grey literature. Grey literature, i.e., reports, dissertations, manuscripts, etc. Which have not been formally published as scholarly articles, were considered credible if they were published by a recognized organization, e.g., the IPCC or government authorities. Furthermore, during the framing phase, two DMDU approaches were chosen, guided by Kwakkel and Haasnoot (2019), to provide qualitative and quantitative inputs to the research: DAP (Walker et al., 2019) through expert interviews and RDM (Lempert, 2019) by exploratory modelling. DAP emphasises defending an existing plan, which makes it a good fit for Stockholm Exergi’s BECCS project, and allows us to assess non-quantifiable uncertainties and adaptations. RDM quantifies robustness, and allows us to assess the relative importance of uncertainties and identify vulnerabilities or opportunities through scenario discovery, quantitatively. The methods are thus complementary and are further described in Sections 2.4, 2.5.
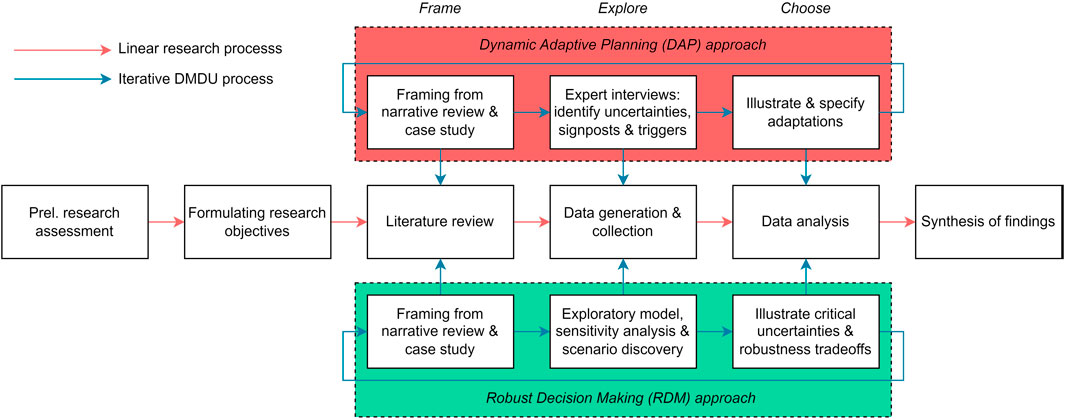
FIGURE 1. Schematic illustration of the methodology. The iterative frame, explore and choose phases of DMDU gave inputs to the otherwise linear research process. Developed by authors.
The explore phase, which was considered the data generation step of the study, involved specifying uncertainties or disagreements on BECCS deployment and exploring different strategies given the uncertainties. Moreover, the results analysis was covered by the DMDU choose phase, which involved illustrating trade-offs and critical uncertainties and identifying adaptive actions for the BECCS strategy. Finally, the findings were synthesised into this article.
2.2 The uncertain incentives for bioenergy carbon capture and storage
A common theme in the literature is that economic incentives need to be in place for Stockholm Exergi, or other actors, to deploy BECCS. Unfortunately, the future realities of economic incentives for BECCS are highly uncertain (Levihn et al., 2019; SOU, 2020:4; Lefvert et al., 2022; Fuss and Johnsson, 2021; Möllersten et al., 2021). For this reason, and since these incentives are key features of the RDM and DAP analyses, this section conceptualizes promising policy or market models providing such incentives. Multiple studies have analysed such models, e.g., Bellamy et al. (2021), Möllersten et al. (2021) or Honegger et al. (2021), but the options described below, including Figure 2, were adapted from Zetterberg et al. (2021) unless otherwise specified. These models considered are: 1) state guarantees, 2) quota obligations, 3) BECCS credits integrated with EU Emission Trading System (ETS), 4) private voluntary markets and 5) other states acting as buyers.
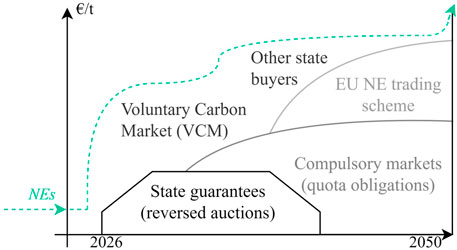
FIGURE 2. Possible policy models for NEs and their sequencing, supporting an uncertain price per tonne CO2. Adapted from Zetterberg et al. (2021).
Firstly, the state (through taxpayers) could guarantee financing for a certain amount of CO2 removal by BECCS, i.e., a state guarantee (Zetterberg et al., 2021). Such a model is currently being implemented in Sweden through a reversed auctioning system where, starting from 2026, the Swedish state will distribute funds to actors who can deliver NEs at the lowest cost (Regeringskansliet, 2022). This model provides favourable conditions for BECCS since long-term contracts, including agreed-upon prices and volumes of NEs, increases predictability for BECCS operators.
Secondly, sectors with hard-to-abate fossil emission could be forced to finance BECCS through state-imposed quota obligations. These emitters would then buy an amount of NEs corresponding to a share of their fossil-based emissions, e.g., at an initial level of 10%, which is then ramped up over time (Zetterberg et al., 2021). Such a mechanism, through a carbon takeback obligation, has been shown to be effective and comparable in cost to mitigation scenarios dominated by demand-side measures, while the combination of an obligation and demand-side measures would enable a low-risk pathway to net-zero by 2050 (Jenkins et al., 2021). Furthermore, this extended producer responsibility of the primary beneficiaries of fossil fuels could deconflict energy security and climate policy (Jenkins et al., 2022).
Thirdly, as the EU aims for climate neutrality by 2050, likely the EU ETS cap will be tightened to zero allowances in 2050 or earlier (Zetterberg et al., 2021). The system was revised in 2023, resulting in an increase in reduction levels and sector coverage (European Council, 2023). As the cap approaches zero, some residual hard-to-abate emissions could be efficiently compensated for by NE “credits,” a commodity representing tonnes of removed CO2, if these were integrated with the trading system or some linked EU scheme, as discussed by Rickels et al. (2021). Fossil based CCS can already be applied to avoid emissions and thus avoid buying ETS allowances; this ruling could be extended to NEs from BECCS. Furthermore, the EU is developing a certification framework for CDR credits (European Commission, n.d.). This framework would enable NE integration into EU climate policy, e.g., by trading under the common EU Nationally Determined Contributions, but could also serve as a reference point to establish NE accounting and trading praxis on voluntary carbon markets (VCMs), as introduced below.
Fourthly, NE credits from BECCS could be sold on a VCM, i.e., to individuals or companies that wish to offset their emissions without any legal requirement to do so. A motivation for this could be to achieve net-zero greenhouse gas targets. VCMs could be crucial to mobilize necessary private financing for CDRs (Zetterberg et al., 2021). Notably, large actors like Microsoft, Stripe and Shopify have expressed intentions to buy significant quantities of carbon credits (Honegger et al., 2021). However, along with other issues highlighted by early VCM failures, there is no unified approach for assessing non-permanence risks of CDR activities eligible for such credits. It is possible that more standardized NE accounting practices, along with the mitigation claims that these represent, would strengthen the position of BECCS credits on VCM markets. Such standardization could be informed by EU best practices, or by the operationalisation of Article 6 of the Paris Agreement (UNFCCC, 2015).
Lastly, as indicated, Article 6 sets a foundation for the transfer of CDR credits between nations. It is intended to increase climate ambition by regulating mechanisms for voluntary cooperation between countries and international trading of emissions (Swedish Energy Agency, 2022). This is relevant for private VCMs, but also fundamental for the case of states exchanging credits to comply with their Nationally Determined Contributions targets. For example, Country A could fund carbon removal investments in country B, and then A claim parts (or all) of the corresponding NEs towards their national targets (Zetterberg et al., 2021). Article 6.2 enables such transfers of mitigation outcomes between nations, while Article 6.4 outlines a market mechanism (Swedish Energy Agency, 2022) which also engage private corporations.
Detailed rules of Article 6 need to be agreed upon, e.g., the interpretation of a “corresponding adjustment” of a country surrendering NE credits to avoid double claiming of the same emission reduction, especially in Article 6.4 applications. In Stockholm Exergi’s view, it should be possible for a company to buy and claim NEs in its climate reporting system, while the NE producing country is also benefiting from the same NEs in its national climate accounting. They frame this as in-line with principles for fossil emission accounting, and as an enabler of public and private co-financing of NEs (Stockholm Exergi AB, 2022). However, other NE claiming and respective trading regulation could be established. Exactly how Article 6.4 will be implemented is still uncertain.
How does this policy landscape relate to individual BECCS operators? It is conceivable that any, or a combination, of the policy models discussed could support an uncertain selling price of NEs, Figure 2. Building on this, the following sections describes the case of Stockholm Exergi’s BECCS facility and illustrates how their project could deliver NEs as a service, given these policy models.
2.3 Framing a case study of Stockholm Exergi’s bioenergy carbon capture and storage project
Stockholm Exergi AB is the largest actor in the multi-energy system of Stockholm municipality, Sweden. As is common in Swedish cities, about half of the heating demand of the region is provided via district heating. For Stockholm municipality, this corresponds to about 12 TWh. Here, Stockholm Exergi has a key role and utilizes about 6 TWh of biogenic-origin fuel for co-generation of heat and power in several combustion facilities. About 3 TWh is the biogenic fraction of municipal solid waste, which mostly is utilized in dedicated waste-incineration plants. The remaining 3 TWh is based on Swedish forestry industry residues, most of which is incinerated in the KVV8 circulating fluidized-bed plant. KVV8 is among the largest co-generation plants in the world originally designed for 100% biomass as fuel (Levihn, 2017; Levihn et al., 2019).
Stockholm Exergi is currently (winter 2023) planning to deploy BECCS at the aforementioned combined heat and power plant KVV8 (Stockholm Exergi AB, 2020). As previously indicated, this case serves as a basis for analyzing general conditions for BECCS deployment. Previous research has shown that KVV8 shows significant potential for BECCS retrofitting using the Hot Potassium Carbonate (HPC) process (Levihn et al., 2019). The choice of the HPC process for CO2 capture rather than absorption in aqueous amine solution should be commented, as the HPC process has often been deemed as unsuitable for CCS and BECCS. The main drawback of the HPC process is its high internal power demand, which results in much reduced electric efficiency for dedicated power generation plants. However, for an operator whose main product is district heating, this drawback is not as pronounced since power consumed internally can be recovered as sellable heat. Indeed, it has been shown that the KVV8 BECCS value chain would achieve considerable volumes of NEs and a climate cooling effect (Hammar and Levihn, 2020) and that the energy penalty of the capture process could be significantly reduced by recovering waste heat (Gustafsson et al., 2021). Currently, steps are being taken for a possible investment decision in 2024, which could lead to operation of the facility in 2026.
The planned BECCS facility is illustrated in Figure 3. The carbon pathway (green) can be described as 1) upstream biomass production, pre-treatment into pellets, and ship or rail transport to KVV8, 2) energy conversion through circulating fluidized bed combustion of the biomass into CO2 containing (18–19 vol%) flue gas, 3) compression of flue gases, 4) absorption of CO2 from flue gases into the HPC absorbent, 5) stripping of gaseous CO2 from absorbent, 6) compression, cooling and intermediate storage of liquid CO2 and 7) downstream ship transportation and final storage. A saline aquifer in the North Sea west of Norway is the planned location for final storage. After certification of the stored CO2, the physical stream of CO2 is transformed into a sellable NE commodity and can be sold at some price level, as was introduced in the previous section. This can be thought of as Stockholm Exergi delivering NEs as a service.
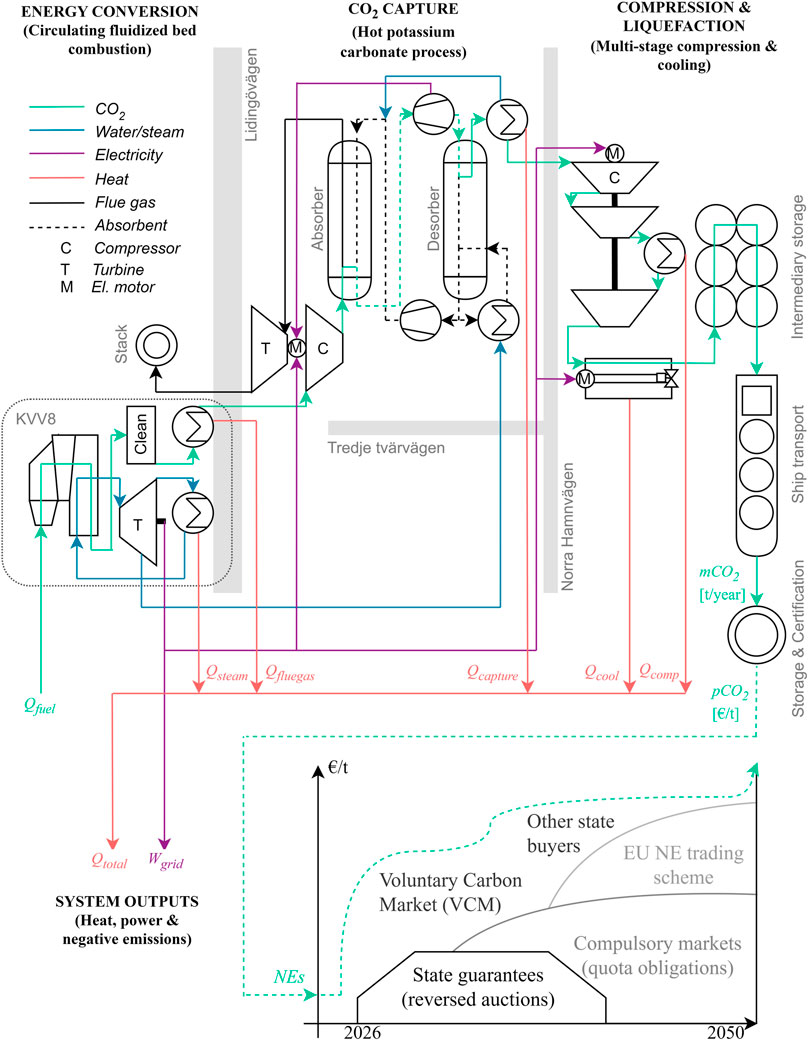
FIGURE 3. Simplified schematic of KVV8 and the planned BECCS system of Stockholm Exergi, as incentivized by NE policy models of Figure 2. Arrows indicate mass, energy or NE flows. Grey bars are roads separating boiler, capture and compression steps. Figure developed by authors and adapted from Gustafsson et al. (2021) and Zetterberg et al. (2021).
Furthermore, (Gustafsson et al., 2021), has described operating conditions for this BECCS configuration, while the following values were given by Stockholm Exergi (Levihn, 2023). Under normal operations, KVV8 can convert 400 MW (∼500 MW higher heating value) biomass into 118 MW electricity and 330 MW district heating, implying a power-to-heat ratio of α = 0.36. After a BECCS retrofit, the same biomass input fuel would instead be converted into around 40 MW net electricity and 424 MW district heating, thus yielding α = 0.09 while capturing NEs of ∼850,000 tCO2 p.a., assuming a capture rate of 140 tCO2/h and ∼6,200 h of operation. Notably, the energy conversion efficiency is sometimes presented as above 100%. This is because the 400 MW thermal input refers to the lower heating value of the fuel, while steam present in the flue gas is condensed to generate district heating.
Naturally, making the deployment decision of this BECCS facility is conditional on managing the many uncertainties faced by Stockholm Exergi. Here, this decision was analyzed using both DAP and RDM methods, which is described in the following sections.
2.4 Dynamic Adaptive Planning through expert interviews
DAP is a DMDU approach to designing strategies that are inherently adaptive to evolving uncertain conditions. The approach has been described in detail by, e.g., Walker et al. (2019) or Jittrapirom et al. (2018). In short, it involves identifying uncertain vulnerabilities and opportunities and adaptive actions to manage these, together with appropriate signposts and triggers. A signpost is information that should be tracked to monitor conditions for success, while triggers are critical threshold values of signposts. For example, the uncertainty “the VCM demand for NEs could be lower than expected” could have the signpost “monitor expected NE price levels” and the trigger “prices reach X level.” If a trigger is reached due to an observed development, an adaptive action should be taken.
In this study, DAP was implemented through semi-structured interviews of eight Stockholm Exergi BECCS experts or employees. Since we answer our research questions by applying DMDU to the Stockholm Exergi case, this narrow organizational focus ensured that, collectively, the interviewees would have both broad and in-depth knowledge of this case, e.g., of the HPC technology choice or of possible financing through Article 6 trading. This selection of interviewees had two important implications: 1) the conclusions from the interviews could clearly be biased, which must be recognized and managed, and 2) the participants to the BECCS deployment decision were deliberating on the analysis, which is a central aspect of DMDU approaches (Kwakkel and Haasnoot, 2019; Lempert, 2019). The interviews varied between 45 min and 1.5 h. Interviewees were asked to participate in the study based on their complementary knowledge. More specifically, some interviewees possessed knowledge on the BECCS policy landscape, others on the technologies, or on regulation, biomass sourcing, etc., so the combined insights from the interviews were comprehensive. The common questions for all interviews are given below, translated from Swedish. The questions were asked with some variation and not exactly as written.
• “What are potential vulnerabilities that could cause the BECCS strategy to fail?”
• “What are potential actions that could be taken to manage these vulnerabilities?”
• “What are potential opportunities that could increase the BECCS strategy’s chance of success?”
• “What are potential actions that could be taken to capitalize on these opportunities?”
Then, different follow-up questions were asked depending on the conditions and actions discussed. Some examples include: “Could you explain X condition in greater detail?,” or “What actions could your company then take, more concretely?.” Furthermore, questions often highlighted specific areas where the interviewees were more knowledgeable, or where previous interviewees had not elaborated much.
The interviews resulted in a list of vulnerabilities and opportunities, the main conditions these would depend upon, and possible adaptive actions. This data was cleaned by removing duplicate statements and by concretizing vaguer statements. This concretization, which was largely interpretive and thus a potential source of biased results, aimed to fit the findings to the DAP framework applied to Stockholm Exergi. Thus, if an expert urged policymakers to support NE co-claiming rules, this support was not interpreted as an adaptive action; instead, the urging or negotiation with policymakers was interpreted as the adaptive action. Similarly, signposts and triggers were interpreted based on the, sometimes not strictly defined, conditions surrounding any given vulnerability or opportunity. For example, an expert could indicate that the integration of NEs into EU policy would be signaled through different communication channels, and therefore require general monitoring of, and presence in, policy and regulatory developments. The interpreted signposts and triggers were in these cases more generally formulated, to both reflect the perceptions of the interviewees while still fitting the DAP framework. And, notably, if an expert indicated that an uncertainty could lead to the complete reassessment of the BECCS strategy, this uncertainty was given extra emphasis in the presentation of results in Section 3.
The uncertainties, i.e., the vulnerabilities and opportunities, were then categorized according to their dimension: political, economic, social, technological, regulatory or environmental. This is similar to Khatiwada et al. (2022) and Song et al. (2017) who base their analysis on an extended PEST framework introduced by Aguilar (1967). In this study, the framework is not used as a methodological basis, but is merely used to characterize and group the uncertainties identified. This was done by considering the pre-conditions of each uncertainty, and not their effects. For example, although establishing an Article 6 market would enable economic incentives for BECCS, this uncertainty was considered mainly political, as its realization would be the result of political negotiations.
Finally, the uncertainties were categorized according to their level, as described by Marchau et al. (2019):
• Level 1 uncertainties lean towards determinism, can be described in one possible future state of the world and be estimated with probabilities.
• Level 2 uncertainties could realize as alternative futures but can still be characterized by confidence intervals and probabilities.
• Level 3 uncertainties can be captured in a limited set of futures, but without assigning probabilities, and traditional scenario analysis is then often used.
• Deep uncertainty, Level 4, is present when it is difficult, Level 4a, or impossible, Level 4b, to representatively specify the system model, variable interactions, probability distributions and/or value of outputs.
The authors evaluated each uncertainty against these criteria and categorized them accordingly.
2.5 Robust Decision Making by exploratory modelling
In parallel with the DAP process, RDM was implemented to analyze the Stockholm Exergi BECCS project. RDM is a DMDU approach that utilizes computational tools and stakeholder deliberation to produce critical scenarios and robust adaptive strategies. A detailed description has been given by Lempert (2019) but put briefly, RDM consists of iteratively framing the decision to be taken, stress testing alternative strategies and choosing a strategy that performs well over a wide range of future scenarios. How these steps were implemented in this study is described below.
2.5.1 Framing the deployment decision
It was concluded that the BECCS deployment decision of Stockholm Exergi principally consists of two options: either invest in a full-scale BECCS facility coupled to KVV8, or not. These alternative strategies are named Invest or Wait and are illustrated in Figure 4. Notably, the model does not analyze later investments than 2024. The logic of investing or waiting is similar to the fossil CCS analysis performed by Oda and Akimoto (2011).
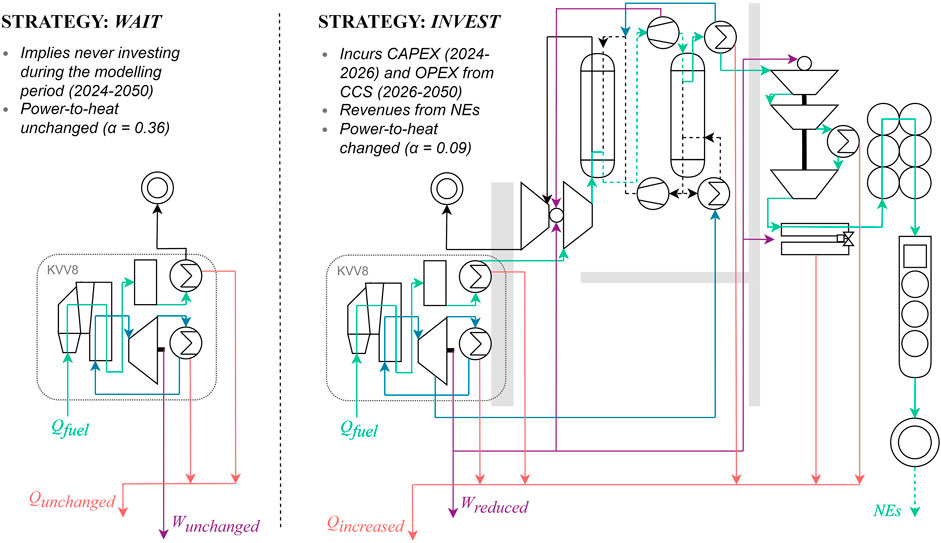
FIGURE 4. Key differences between the two alternative strategies: Wait, or Invest in BECCS. Developed by authors.
A model was developed to evaluate the two strategies. This was done using Rhodium (Hadjimichael et al., 2020), which is an open-source Python library with supporting tools for RDM and exploratory modelling. The main output of the model was the Net Present Value (NPV) of the two strategies, which was used as the measure of performance and was assumed to be an appropriate proxy for the business case. Basically, it tells us which decision was the better one. NPV was calculated using Eq. 1. Full equations and more detailed descriptions can be found as Supplementary Material.
Here, t tracks the year, which increases from 2024 until 2050, when the assumed economic lifetime of 26 years ends. DR is the discount rate. CFenergy includes the different costs of running the combined heat and power plant and the revenues of selling electricity and district heating. CFCO2 includes the different costs of running the carbon capture and storage value chain, possible costs of buying ETS allowances, and revenues from selling NEs. The policy variable represents multiple rows of code which alter the revenues from NEs, based on different policy uncertainties. These represent both vulnerabilities and opportunities of the BECCS decision, and their implementation is described in Table 1. Furthermore, in-code, capital expenditures were incurred during the first two modelling years.
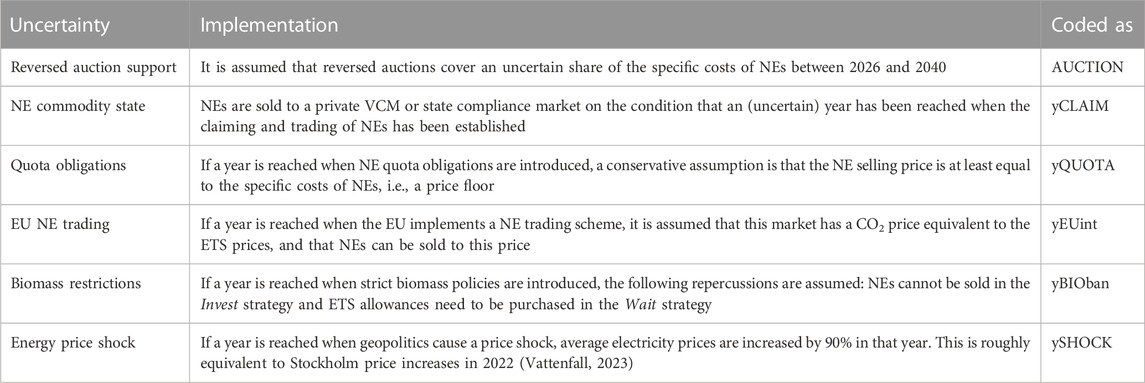
TABLE 1. Overview of the assumptions for and implementation of policy uncertainties. Refer to Section 2.1 for additional background and the Supplementary Material and RDM model for the in-code implementation.
The final framing step was to determine how to evaluate the robustness of the decision i = Invest, or i = Wait. This required the calculation of regret for every model evaluation. The regret of strategy i in future j is the difference between the performance of that strategy in that future, and the performance of the optimal strategy i' in that future (Lempert et al., 2006). This can be formalized using Eq. 2:
Which was calculated for each future using Eq. 3, for the case of investing in BECCS or not:
2.5.2 Evaluating the deployment decision
After framing the decision to Invest in BECCS or Wait, the performance of these strategies was explored in a multitude of future scenarios. Each future was generated using Rhodium by Latin hypercube sampling from the uncertainty ranges provided in Table 2. In simple terms, samples are thus not taken at random from probability distributions: samples are selected to maximize the “spread” between futures. See Preece and Milanovic (2016) for additional information.
In Table 2, wherever a source is not provided, the range of data values were assumed. Wherever a source provided one specific value, an interval was created based on a +-50% change of this value. Sources marked with asterisk (*) are discussed in Supplementary Material.
Notably, many parameters are unique to the case studied, e.g., heat and electricity prices represent energy prices of Stockholm. furthermore, some parameters were not modelled as uncertainties and were instead fixed across future scenarios, see Table 2. The rationale was that any variation in these parameters would either be very small or have little impact on the overall results.
In total, the NPV and regret of the BECCS investment decision were evaluated in 100,000 futures by sampling from the uncertainty ranges. From these evaluations the robustness of both the Investing and Waiting strategies could be quantified using satisficing and domain criteria from DMDU theory (Marchau et al., 2019). Savage’s criterion selects the strategy that minimizes the maximum regret across all plausible future scenarios (Schneller and Sphicas, 1983). Satisficing metrics (Simon, 1959) recognise that decisionmakers often prioritize strategies that do not optimize against any objective, but that meet one or more performance requirements in many futures. An application of this is the domain criteria, which selects the strategy that meets a given performance threshold across most future scenarios (Schneller and Sphicas, 1983; Marchau et al., 2019). These metrics attempt to select a robust strategy that performs well over a wide range of futures, either by avoiding worst cases (Savage’s criteria) or performing adequately across many futures (satisficing domain criteria). Given this, three robustness criteria for the decision to Invest in BECCS or Wait were implemented in the following ways:
• Satisficing domain criteria: a robust strategy performs satisficing in many futures. A strategy was considered satisficing in one future if NPV >0 in that future.
• Relative satisficing domain criteria: a robust strategy performs satisficing, relative to other strategies, in many futures. A strategy was considered satisficing in one future if NPV >0 and regret = 0 in that future.
• Savage’s criteria: a robust strategy avoids worst cases. A strategy was considered robust if it minimizes the maximum regret across all futures.
2.5.3 Identifying critical uncertainties and scenarios
Rhodium’s methods for scenario discovery and sensitivity analysis were applied to identify key conditions and drivers of the BECCS deployment decision. Classification and Regression Trees was used for scenario discovery and has been described in detail by Krzywinski and Altman (2017). The method was used to identify futures of interest, i.e., where the Invest strategy performed well (zero regret) or poorly (non-zero regret). The resulting scenarios were characterized as uncertainty ranges with a certain quality, i.e., coverage and density. Coverage represents the ratio of futures of interest within a given scenario subset to the total number of futures. Density represents the ratio of futures of interest to the number of futures within a scenario subset (Kwakkel et al., 2016). Simply put, high-coverage scenarios explain many of the futures of interest, and high-density scenarios represent many interesting and few non-interesting futures.
Furthermore, Sobol’s method was used for global and variance-based sensitivity analysis. The method has been described in detail by Rosolem et al. (2012). Using Rhodium, the method was used to attribute variance in regret of the Invest strategy to the input uncertainties of Table 2. The algorithm produces first, second, and total order sensitivity indices for each uncertainty. Respectively, these represent the uncertainty’s main effect, second order interaction effects, and the combination of main effect and interaction effects of any order. The total order sensitivity indices were used to rank the uncertainties in order of importance, where uncertainties with a higher index cause greater variance in regret.
3 Results
In Section 3.1 we present the results of the expert interviews and in Section 3.2 the modelling results.
3.1 Interview results on deploying bioenergy carbon capture and storage
From the expert interviews and by applying the DAP framework, 29 vulnerabilities, 19 opportunities and 79 corresponding adaptive actions of the BECCS deployment strategy were identified. Critical vulnerabilities, i.e., uncertainties which could require a complete reassessment of the BECCS strategy, are listed in Table 3. Additionally, a selection of vulnerabilities and opportunities of different dimensions, i.e., economic, social, etc., are listed in Table 4. Refer to the Supplementary Material for all results. Uncertainties are color-coded as follows: political (red), economic (green), social (yellow), technological (purple), regulatory (blue) and environmental (pink). Furthermore, note that different triggers can be mapped to different adaptive actions: these should not be read row-by-row.
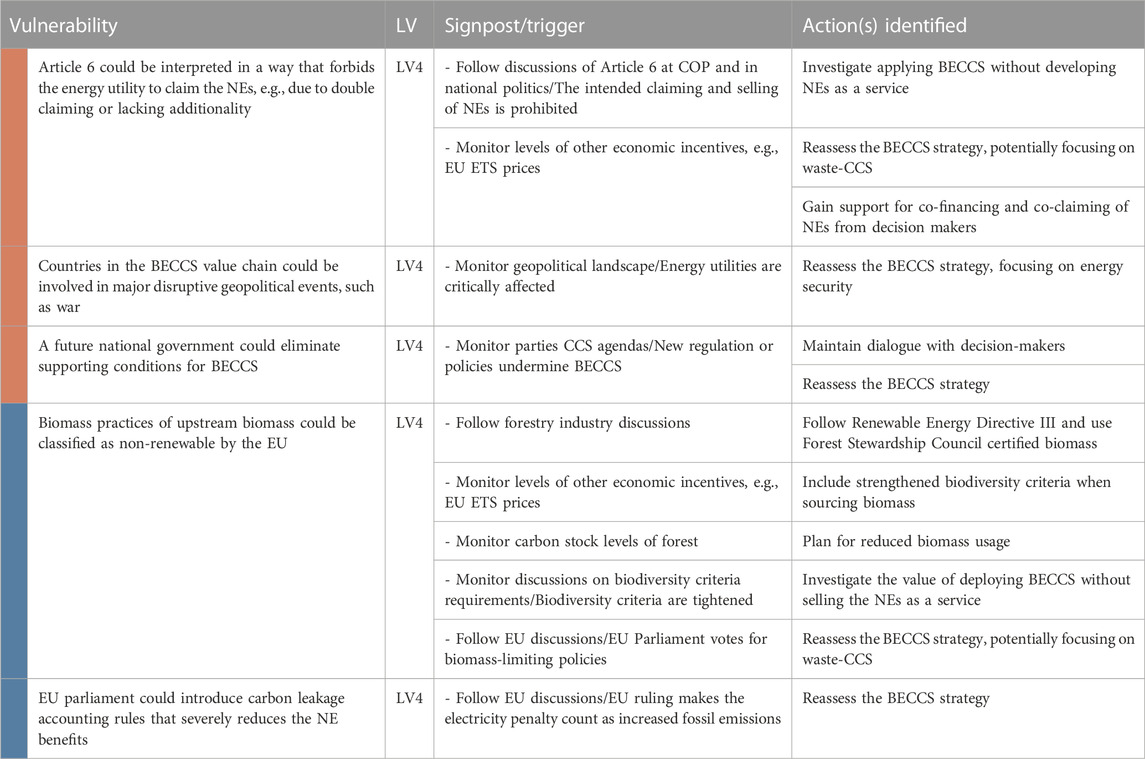
TABLE 3. Critical vulnerabilities and corresponding adaptive actions, including signposts and triggers, of deploying BECCS. LV refers to uncertainty level, see Section 2.3.
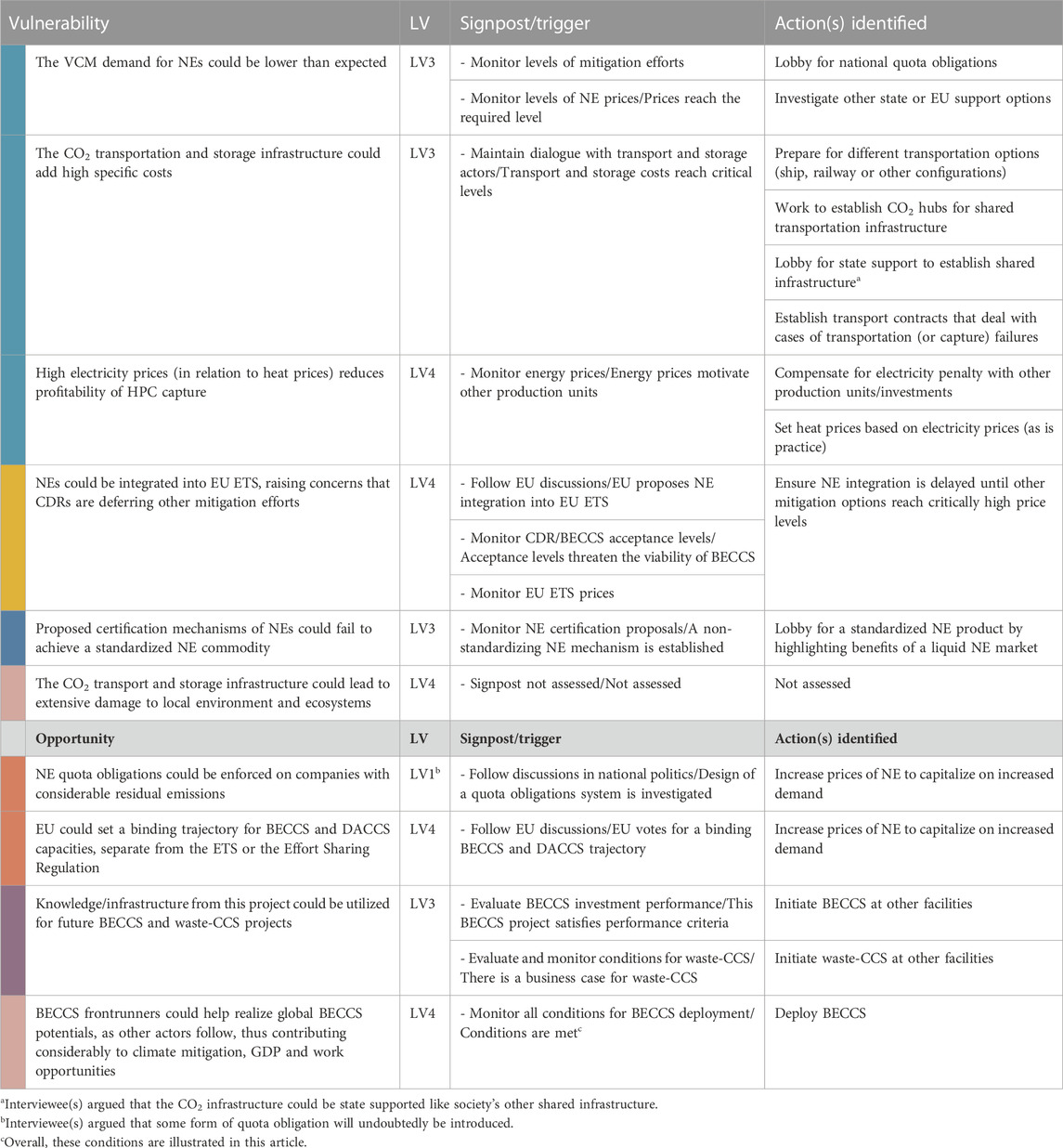
TABLE 4. A selection of multi-dimensional vulnerabilities and opportunities, corresponding adaptive actions, signposts and triggers of deploying BECCS. LV refers to uncertainty level, see Section 2.3.
Table 3 shows that the critical uncertainties of deploying BECCS, as identified by Stockholm Exergi, are political or regulatory, as categorized by the authors. Establishing appropriate NE claiming and trading rules under Article 6 of the Paris Agreement and strengthening the biomass sustainability strategy are key adaptations to manage these uncertainties. Table 4 illustrates the multi-dimensionality of BECCS uncertainties. To name a few examples, an energy utility deploying BECCS needs to ensure social acceptance, actively engage with politicians and other CCS actors, and engage in regulation and policymaking processes.
3.2 Modelling results on deploying bioenergy carbon capture and storage
The interview findings are complemented by the modelling results, which consist of 100,000 evaluated scenarios represented by colored points. Colors represent regret [€ billion] of the strategy to Invest in BECCS. In Figure 5 the NPV of Invest and Wait is illustrated. Notably, due to the sampling technique used we do not make probabilistic analyses of these results.
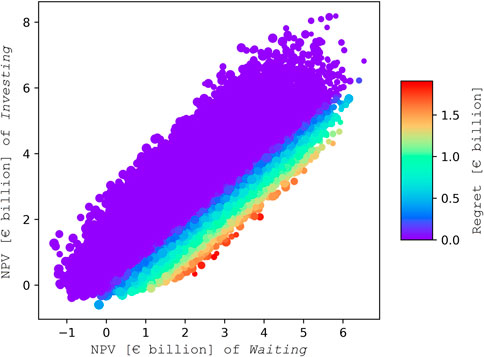
FIGURE 5. NPV of Investing in BECCS or Waiting in all model evaluations. Color represents regret of Investing.
Figure 5 indicates that Invest performs better than Wait in terms of NPV in many futures, and vice versa, that NPV varies greatly across model evaluations. The overall performance of these two strategies was quantified using three robustness criteria. The results are illustrated in Table 5. From this analysis, Invest is the more robust strategy across all evaluated robustness criteria, as the strategy is both satisficing and relative satisficing in a higher number of futures, and as it has lower maximum regret compared to the Wait strategy. See Section 2.5.2 for the implementation of these criteria.
Furthermore, Figure 6 illustrates how the average selling price and specific costs of NEs achieved between 2024–2050 correlates to the NPV of Investing, in panels (A) and (B) respectively. “Average” refers to the price average over the modelling period. This price and costs are calculated at the end of each model run and thus aggregate multiple input uncertainties, including e.g., policies and ETS prices, and operating, transport and storage costs.
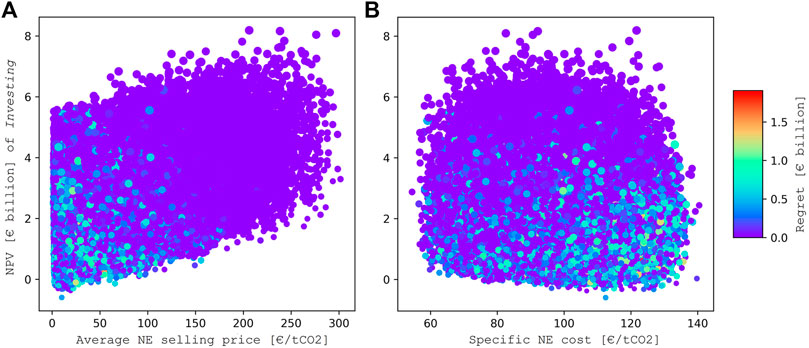
FIGURE 6. NPV of Investing in BECCS against average selling price (A), and specific costs (B), of NEs.
From Figure 6A it can be concluded that higher NPV and lower regret of Investing is achieved at higher average selling prices of NEs. For example, at average NE prices higher than 120 €/tCO2, 94% of evaluated futures have zero regret, implying Investing in BECCS performs better than Waiting. Such a correlation is less clear between the NPV and the specific cost of NEs, Figure 6B, possibly due to the narrower cost interval.
To understand what uncertainties are driving regret, Sobol’s method (Rosolem et al., 2012) for global sensitivity analysis was performed. The results are illustrated in the spider plot of Figure 7. First, second and total order sensitivity indices are respectively represented by the grey circle radii, spider leg “width,” and white circle radii. Numerical values of total order indices are given in parenthesis. Refer to the Supplementary Material for other indices.
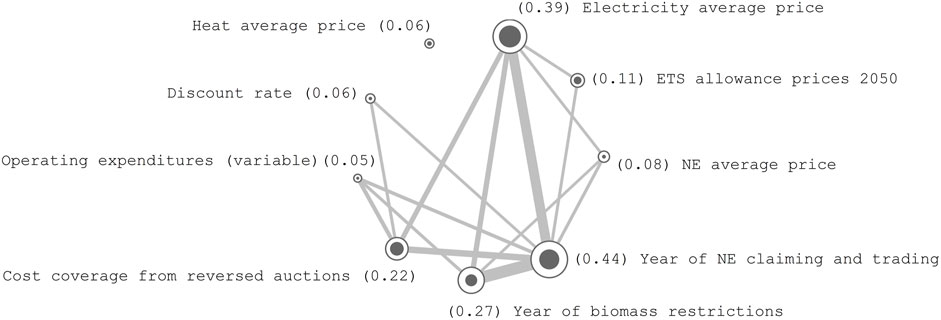
FIGURE 7. Sensitivity indicies of 9 out of 23 input uncertainties of deploying BECCS, illustrated in a spider plot.
The spider plot illustrates 9 out of 23 uncertainties that drive regret of Investing in BECCS, and is helpful to show the relative importance of uncertainties. Given their high total sensitivity indices (white circle radii), four key uncertainties are: the year when NE claiming rules are established, the average electricity prices between 2024–2050, the year when biomass usage could be restricted, and the specific NE costs covered by a reversed auction policy. While these are elaborated upon in the discussion, Figure 8 showcases an example of how regret of Investing is affected by the timing of NE claiming rules and the electricity prices. Clearly, higher values of these uncertainties cause greater regret.
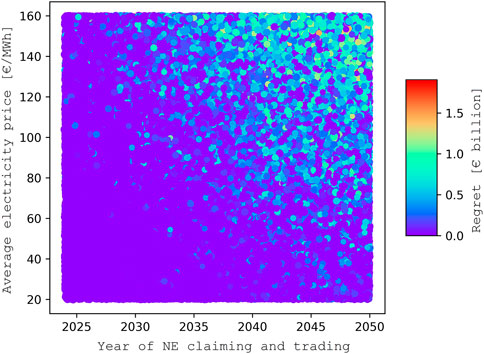
FIGURE 8. The year when NE claiming rules are established and the mean electricity price are key drivers of regret when deploying BECCS.
In addition, Classification and Regression Trees was used for scenario discovery to identify combinations of uncertainty ranges where Investing in BECCS performs well, and where this strategy is vulnerable. A selection of these scenarios is illustrated in Figure 9. Panel (A) shows a scenario (yellow box) where good performance of BECCS, i.e., zero regret, was sought after. If mean electricity prices are below 110 €/MWh, and if NE claiming rules are established before 2034, Investing in BECCS performs better than not doing so in 92% of cases evaluated.
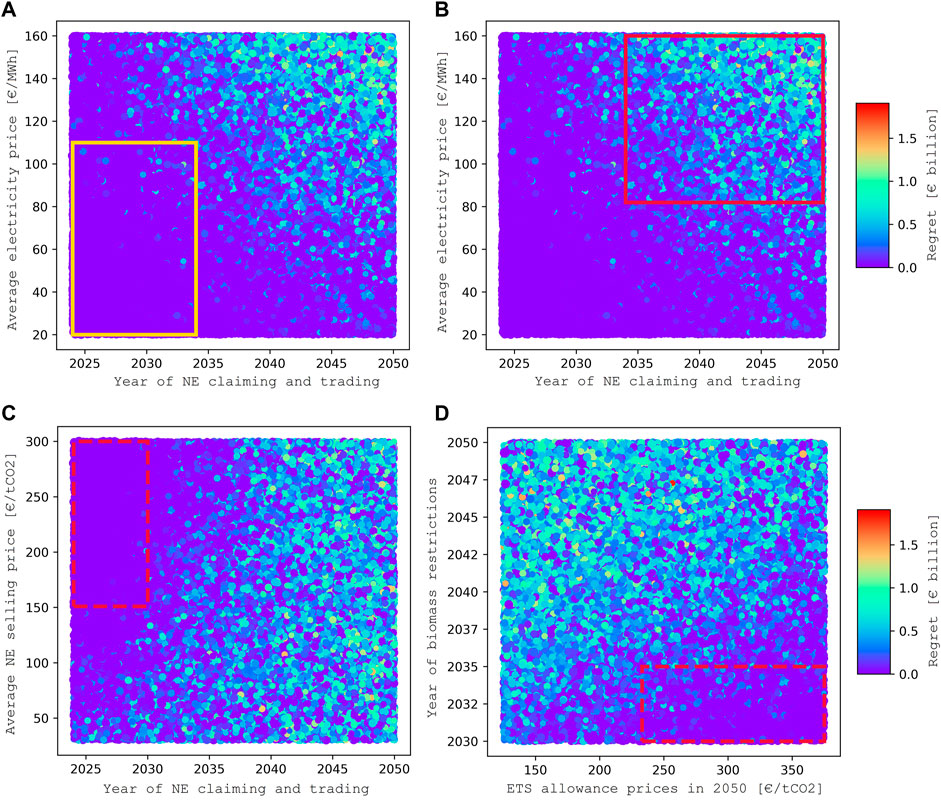
FIGURE 9. (A) a scenario of low electricity prices and early establishment of NE claiming rules has low regret of investing in BECCS. (B) a scenario of high electricity prices and late establishment of NE claiming rules causes regret of investing in BECCS. (C) a scenario of high NE selling prices and early establishment of NE claiming rules has low regret despite high electricity prices. (D) a scenario of high mean ETS prices in 2050 and early biomass restrictions has low regret despite high electricity prices and late establishment of NE claiming rules.
Panel (B) shows a scenario (red box) where poor performance of BECCS, i.e., non-zero regret, was sought after. If mean electricity prices are above 82 €/MWh, and if NE claiming rules are established after 2034, Investing in BECCS performs worse than not doing so in 75% of cases evaluated. To identify possible adaptations of the BECCS strategy under such vulnerable conditions, i.e., electricity prices above 82 €/MWh, a zero-regret scenario was sought after in panel (C). It was found that under this electricity price, Investing still performs better than not doing so in 96% of cases if NE prices are above 151 €/tCO2 and if NE claiming rules are established before 2030 (red dashed box). Panel (D) also has these high electricity prices, and adds the second vulnerable condition, i.e., that NE claiming rules are established sometime after 2034. In this scenario, Investing in BECCS can still perform better than not doing so in 70% of cases if mean ETS prices are above 233 €/tCO2 in 2050, and if restrictions on biomass are imposed sometime before 2035 (red dashed box).
A full list of discovered scenarios and sensitivity indices can be found in the Supplementary Material.
4 Discussion
The objective of this article is to explore options to improve the robustness of BECCS deployment strategies. DMDU methods were applied to the early BECCS mover Stockholm Exergi, who served as a basis of analysis. The authors assessed many of the critical uncertainties of their BECCS project as deeply uncertain, or Level 4, in Tables 3, 4. This is in-line with the definition of deep uncertainty (Lempert et al., 2003; Marchau et al., 2019), since it is either unknown how the uncertainties i) form the external context of the BECCS system, ii) interact with the BECCS system, or iii) affect the outcomes of the BECCS system. The same comparison can be made for the quantified uncertainties, Table 2. It would, e.g., be difficult to assign probabilities to or project NE and energy prices or the implementation of BECCS policy support. Naturally, it is possible to perform such analyses, but the results would be strongly dependent on the probabilistic assumptions made. While, as was illustrated in Figures 7–9, these uncertainties have a major impact on the case studied. Also, it is likely that these uncertainties have large impact on BECCS deployment cases in general, as carbon or energy prices are very relevant to other applications of BECCS, e.g., in pulp and paper, biorefinery or waste sectors. Applying DMDU to systematically explore these uncertainties in a wide range of plausible, non-probabilistic, futures was therefore a natural methodological fit for the BECCS deployment problem.
Furthermore, this study illustrated how DMDU approaches can support the development of CDR strategies, e.g., by exploring alternative integration options of NEs in climate policy and targets, or by showcasing how different policy options perform in terms of enabling BECCS investments. Workman et al. (2021) suggested how DMDU approaches, adopted by policymakers, could be key for robust CDR policy design. Similarly, this study expanded on possible applications of the DMDU field, when adopted by CDR industry actors. This provided an opportunity to better represent the organizational context of decision making, which has been raised as a limitation of DMDU (Stanton and Roelich, 2021). The consideration of CDR policy from these different viewpoints, i.e., from policymakers or from industry actors, represents both novel and useful applications of DMDU.
By implementing DAP, 29 vulnerabilities, 19 opportunities and 79 corresponding adaptive actions of deploying BECCS were identified. These covered both political, economic, social, technological, regulatory and environmental dimensions. This was an exploration of options to improve BECCS robustness and provided qualitative answers to the second and third research questions. By implementing RDM, the NPV and regret of deploying BECCS, or not doing so, was explored in 100,000 futures, and critical uncertainties and scenarios were identified. This exploration provided quantitative answers to the first, second, and to some extent the third research question. The two methods were thus complementary. A strength of this approach is that qualitative and quantitative findings can be integrated, discussed and compared, which is done below, ordered by research question. The Supplementary Material includes further results.
An investment decision to deploy BECCS can be supported by a quantification of robustness. This was done using NPV and regret. Using these studied metrics, it was shown that investing in BECCS is more robust than not doing so across three robustness criteria, Table 5. Investing had NPV >0 in more than 99% of futures evaluated, regret = 0 in 58% of futures, and a maximum regret of € 1.90 billion. However, not investing performed similarly well, with NPV >0 in more than 98% of futures, regret = 0 in 42% of futures, and a maximum regret of € 3.8 billion. If just relying on NPV calculations, an energy utility with conditions similar to the studied case may therefore find that the strategies of deploying BECCS, or not, may perform similarly against domain robustness criteria, although slightly favored towards investing. But, considering robustness criteria that avoid worst-cases, not investing could have around two times higher regret, which here implied missed out profits of € 3.8 billion. These worst-case scenarios occur at high mean NE prices, which in this analysis was maximized at 300 €/tCO2. Likely, higher CO2 prices would further increase the maximum regret of not investing.
There is high agreement between the qualitative and quantitative findings on what conditions are critical for BECCS deployment. Although currently uncertain, agreement and clarity on how NEs are claimed, accounted for and traded would enable critical economic incentives for BECCS projects to be developed. This concerns cases of private or state buyers, either on a VCM or a compliance market within the EU, or under Article 6 of the Paris Agreement. The qualitative findings suggest that such conditions would enable BECCS operators to deliver NEs as a service, and that the economic uncertainties then become manageable. For example, by establishing long-term contracts at an agreed-upon NE price level, or by selling to a future liquid NE market where other CDR operators could set high marginal prices. Similarly, the quantitative findings strongly suggest that the timing of establishing NE claiming rules is of critical importance: it is the uncertainty of highest total order sensitivity index, Figures 7, 8, and the uncertainty is the main driver of regret in key scenarios, Figure 9.
Future biomass regulation was identified as a critical uncertainty of BECCS deployment. A comprehensive assessment of such regulation was beyond the scope of this research, but the qualitative findings suggest that extreme cases of EU imposed biomass regulation could necessitate the reassessment of BECCS deployment strategies. For example, biomass could be treated as non-renewable or unsustainable, thus incurring an obligation to give up ETS allowances, or dismissing the presumed climate mitigating effects of NEs. Furthermore, sustainability criteria could be perpetually tightened to reduce deforestation and risks of unsustainable bioenergy production. Ultimately, such regulation threatens biomass-fired combined heat and power plants in general, with or without CCS. However, framing biomass regulation as a threat could be a result of views reported by the Stockholm Exergi interviewees. That said, the quantitative analysis in Figure 9D also showcases the issue: at vulnerable conditions for investing in BECCS, not investing is vulnerable to early biomass bans, as ETS allowances would need to be bought for the CO2 not captured. In practice though, this scenario does not necessarily result in NEs if a switch to other means of producing district heating occurs, e.g., heat pumps. However, this finding is dependent on how this uncertainty was implemented, see Table 1. Different implementations of biomass regulation would give different outcomes, and could be the focus of future research. Taken together, the qualitative and quantitative findings still suggest that responsible and sustainable bioenergy supply is essential to managing the vulnerabilities and capitalizing on the opportunities of deploying BECCS.
Considering the importance of energy prices on BECCS deployment, the findings are nuanced. Qualitatively, high electricity prices were seen as a clear vulnerability by some experts, and not by others. The electricity price shock of 2022, in combination with the electricity penalty incurred by the HPC capture process, was the main cause of concern. But some experts instead argued that in response to the crisis, society would “overinvest” in renewable generation capacity. A reversed situation with high supply and low marginal prices of electricity would then favor the HPC investment. An energy utility can deploy/withdraw other production units to adapt to such situations and balance the reduced electricity and increased heat output of a BECCS facility. Although not quantitatively assessed in this research, similar dynamics have previously been analyzed and discussed by Levihn (2017).
Furthermore, potentially rising biomass prices were not seen as a critical vulnerability of the BECCS project, although this likely would affect profitability of biomass-fired combined heat and power in general. Moreover, heat prices were seen as less uncertain. This could be due to the lower fluctuations of district heating prices in Stockholm. The difference between heat and electricity prices was however understood as important for the relative performance of deploying BECCS, but these prices are also interdependently set. The authors conclude that the experts acknowledged energy prices as highly uncertain and important, but not critical, for the chosen BECCS deployment pathway. This could either be due to an unwillingness to recognize futures where the HPC choice would be a vulnerable strategy, or to legitimate confidence in the energy utility’s ability to adapt to different energy price settings.
Notably, BECCS deployment with CO2 capture by amine absorption would showcase different dynamics, as compared to this case here with CO2 capture by HPC. In general, the electric efficiency of the plant and the α-factor would be higher, while heat production would be lower, thus entailing a different sensitivity to energy prices. But for this HPC case, the importance of energy prices could also be quantitatively illustrated. Notably, heat and biomass prices were not critical drivers of regret. The future electricity price shock uncertainty, which incurred a 90% increase over 1 year, also had negligible impact on the performance of the BECCS investment, contrary to some of the experts’ concerns. The average electricity price was however shown to be a key driver of regret in the sensitivity analysis, Figure 8, and the scenarios discovered, Figure 9. In part, this is due to the wide range of mean electricity prices explored, i.e. 20-160 €/MWh. The higher values of this interval could be considered unlikely, yet they give substantial weight to the analysis. This implies that extreme electricity prices can be significant for a robust BECCS deployment strategy which avoids worst cases, which is in-line with the concerns raised by some of the experts. But, such worst cases could be considered unlikely. Thus, there is agreement between the quantitative and qualitative findings that average electricity prices are important for the performance of the BECCS strategy, while only the quantitative findings suggest that this could be a critical uncertainty. This can be explained by the wide price range explored and by the limitations of the model, as it does not include adaptations to different energy price settings.
Of the identified key scenarios and adaptations to manage the uncertainties of BECCS deployment, most critical uncertainties are external to Stockholm Exergi, or other BECCS operators. This was shown, e.g., in the Sobol results, Figure 7. NE claiming rules and the consecutive establishment on a VCM or Article 6 compliance market requires actions outside the normal system boundaries of an energy utility. These require external adaptive actions: gaining support in regional, national and global forums for NE regulation, or ensuring certification mechanisms and targets for CDR are developed at EU level. Additional actions on policy level include the promotion of NE quota obligations or of (careful) integration of NEs into the ETS. These adaptations aim to secure the revenue stream of delivering NEs as a service. Notably, even in a vulnerable scenario of average electricity prices exceeding 82 €/MWh, illustrated in Figure 9C, investing in BECCS performs better than not doing so in 96% of futures evaluated if NE claiming rules are established before 2030 and the NE price exceeds 151 €/tCO2. To reflect on limitations of this study, the mix of revenue options for NEs is here modelled without much correlation between the options. In real life, some correlation would likely exist, e.g., high ETS prices might correlate with high VCM prices.
Other adaptations of the BECCS strategy should address the uncertainty of future biomass regulation. European BECCS operators can follow the Renewable Energy Directive III, use Forest Stewardship Council certified biomass and adopt stricter biodiversity criteria when sourcing biomass. Possible adaptations also include planning for reduced or modified biomass feedstock. The quantitative findings suggest that such actions are more important for the decision not to invest in BECCS, compared to investing. An early biomass ban implies low regret of investing, Figure 9D, and conversely, high regret of not investing. Taken together, the qualitative and quantitative findings suggest that bioenergy utilities should take a range of actions to strengthen the sustainability of their biomass sourcing strategy, no matter if BECCS is deployed or not.
Moreover, as previously discussed, investing in BECCS can be vulnerable to high mean electricity prices, Figure 9B. Some possible adaptations to this uncertainty were already discussed, e.g., the deployment/withdrawal of other production units, but these were not represented in the exploratory modelling. A future RDM iteration could specifically analyze the electricity price uncertainty and expand on possible adaptations to address it. Other limitations of the study include the selection of parameter ranges. When applying RDM, wide ranges should be explored. But their exact selection impacts the findings. For example, the wide electricity price range gave this uncertainty a greater weight in the quantitative analysis. If reducing this range to 20–80 €/MWh and re-running the model, most of the findings remain the same, but the reversed auction uncertainty grows in importance. While such an analysis can be performed for all parameters of the study and other uncertainties could be shown to be more significant, this range selection should be recognized as a limitation of our research.
For a different case than Stockholm Exergi, who intend to deploy BECCS in the near future, a new RDM iteration could evaluate different timings of investing. This should also be relevant for Stockholm Exergi, who in reality would have the option of a postponed investment. Such a postponed decision could then be better tailored to future regulatory or political conditions. This is another limitation of our research, but also a suggested topic for future research: how different policy mixes impact the timing of robust BECCS investment decisions. Also, CO2 capture technologies with lower power demand than HPC could be considered, even though all options have their own set of uncertainties and drawback. Finally, future research could incorporate probabilistic analyses to complement the findings from ours. Due attention would then need to be paid to any assumed probabilities, while the justification of these probabilities would be difficult for deep uncertainties. A potentially promising method to address this has been proposed by Stroombergen and Lawrence (2022).
The research identified critical uncertainties and adaptations of an energy utility deploying BECCS. But interestingly, it also shows what uncertainties are not important, at least for the application to biomass-fired combined heat and power plants. Notably, capital expenditures were considered a certain vulnerability. The cost range is wide, but this was in-line with the experts’ expectations, and adaptations were already made to address this, e.g., through support from the EU Innovation Fund. Emphasis was instead put on securing the highly (or deeply) uncertain revenues of delivering NEs as a service. This was also supported by the quantitative findings: capital expenditure is not driving regret substantially and is not key in scenarios discovered, Figures 7–9. Although raised by the experts as important, operating, transport and storage costs were similarly shown to have limited quantitative impact. As was previously indicated, it is regulatory and political certainty of operational revenues that enable actors to manage the high risk and capital expenditure of BECCS projects. This suggests that future research aiming to accelerate BECCS deployment, in-line with mitigation scenarios of the IPCC, should look beyond facility-level efficiency or cost optimizations, and instead support business development of these technologies in the context of regulation and policy. This study illustrated that such regulatory and policy interventions to establish an NE market, as advocated by Stockholm Exergi, could be a viable way forward. But given the prevalence of other BECCS support models in the NE literature and policy debates, and their simplified representation in this study, the effectiveness of these models should be researched further.
5 Conclusion
This study expands on existing DMDU and CDR research, as previous studies which apply these methods have not adequately captured the multi-dimensionality of uncertainties surrounding BECCS deployment, nor has this been done by application to specific BECCS case studies. Furthermore, we reason that industry actors adopting DMDU approaches effectively illustrates the performance of CDR policies. This would be complementary to policymakers adopting these approaches to cope with the deep uncertainties surrounding CDR strategies, which has been suggested in previous research.
The main conclusions of the research are:
(1) Investing in BECCS is relatively robust against robustness criteria which rely on satisficing performance across many scenarios and which avoid worst-cases. Not investing is also relatively robust, but could miss out on € 3.8 billion in terms of NPV. This worst-case is two times higher than that of investing. Although this finding could support a BECCS investment decision, not all non-quantifiable uncertainties, e.g., some political, regulatory or social, were reflected in the robustness calculations.
(2) From the viewpoint of an energy utility, the critical vulnerabilities of BECCS deployment are political or regulatory, while this deployment is more robust to economic, social, technological and environmental uncertainties. The major uncertainties are the future NE claiming and trading rules, which would enable private or state compliance markets, e.g., within EU or under Article 6 of the Paris Agreement, and the future of biomass regulation. Average electricity prices were also shown to be of great importance at high ranges, although these were not shown to require the complete reassessment of the BECCS deployment decision. Finally, we suggest that facility-level uncertainties, such as capital expenditures or process parameters, are of lesser importance, and that future research supporting BECCS deployment instead should analyze business development of these technologies in the context of policy and regulation.
(3) To manage the major uncertainties of BECCS deployment, some key adaptations are to gain support broadly for negative emission claiming and trading, and to proactively strengthen biomass sustainability strategies, e.g., through tightened sourcing criteria or diversifying the biomass supply. Even at vulnerable conditions, i.e., at electricity prices above 82 €/MWh, if actions are taken to allow the claiming and selling of negative emissions before 2030, and if these are sold at a price above 151 €/CO2, investing in BECCS performs better than not doing so in 96% of scenarios evaluated.
For energy utilities, the main implications of the research are that companies with favorable conditions for BECCS may be missing out on substantial economic benefits of deploying BECCS, if not taking action to deploy these technologies. To assess the effects of (in) action, these energy utilities could strengthen their uncertainty analysis of CCS investment options and of biomass supply, as was illustrated in this research.
For policymakers, the main implication was the need for interventions to establish the mechanisms for claiming, accounting and trading of negative emissions. This concerns both private carbon markets and states utilizing bilateral trading to comply with emissions targets, e.g., through Article 6 of the Paris Agreement, or EU trading under the common Nationally Determined Contributions. From an investor perspective, removing regulatory uncertainties is key for increased robustness of developing new BECCS projects. Although the effectiveness of other policy models should be studied further, such regulatory interventions could enable the necessary incentives for BECCS up-scaling and, conceivably, the achievement of global net-zero emissions.
Data availability statement
The datasets presented in this study can be found in online repositories. The names of the repository/repositories and accession number(s) can be found below: https://doi.org/10.5281/zenodo.8086484.
Ethics statement
Ethical review and approval was not required for the study on human participants in accordance with the local legislation and institutional requirements. Written informed consent from the participants was not required to participate in this study in accordance with the national legislation and the institutional requirements.
Author contributions
OS, DK, and FL contributed to conception and design of the study. OS performed interviews. OS performed quantitative modelling, with support from WU. OS was responsible for analysis of data, with support from all co-authors. OS wrote the first draft of the manuscript. OS and MR wrote sections of the manuscript. MR, DK, and FL contributed with supervision, project administration and funding acquisition. All authors contributed to the article and approved the submitted version.
Funding
This work received funding from the Swedish Energy Agency via the project “Below zero–Responsible and adaptive realization of sociotechnical bioenergy systems with carbon capture and storge,” grant no. P2022-00172.
Acknowledgments
The authors are grateful for the discussions and support provided by the researchers affiliated to KTH Climate Action Centre, in particular Francesco Fuso-Nerini and Karin Larsdotter. At the time of submitting this manuscript, Stockholm Exergi are planning to take an investment decision during the first quarter of 2024, for possible operation of the BECCS facility in 2026.
Conflict of interest
This research was made possible through collaboration between academia and industry. Any potential conflict of interest would be represented by the affiliations of the authors. OS and MR are employed at Chalmers University of Technology. FL is employed at Stockholm Exergi AB and at KTH Royal Institute of Technology. DK and WU are employed at KTH Royal Institute of Technology.
Publisher’s note
All claims expressed in this article are solely those of the authors and do not necessarily represent those of their affiliated organizations, or those of the publisher, the editors and the reviewers. Any product that may be evaluated in this article, or claim that may be made by its manufacturer, is not guaranteed or endorsed by the publisher.
Supplementary material
The Supplementary Material for this article can be found online at: https://www.frontiersin.org/articles/10.3389/fenrg.2023.1250537/full#supplementary-material
Abbreviations
BECCS, Bioenergy Carbon Capture and Storage; CAPEX, Capital Expenditures; CCS, Carbon Capture and Storage; CDR, Carbon Dioxide Removal; DACCS, Direct Air Carbon Capture and Storage; DAP, Dynamic Adaptive Planning; DMDU, Decision Making under Deep Uncertainty; ETS, Emissions Trading System; HPC, Hot Potassium Carbonate; IPCC , Intergovernmental Panel on Climate Change; NE, Negative Emission; NPV, Net Present Value; OPEX, Operational Expenditures; RDM, Robust Decision Making; VCM, Voluntary Carbon Market.
References
ARC (2023). Carbon capture - ARC. [online] Available at: https://a-r-c.dk/english/carbon-capture/ (Accessed November 28, 2023).
Bellamy, R., Fridahl, M., Lezaun, J., Palmer, J., Rodriguez, E., Lefvert, A., et al. (2021). Incentivising bioenergy with carbon capture and storage (BECCS) responsibly: comparing stakeholder policy preferences in the United Kingdom and Sweden. Environ. Sci. Policy 116, 47–55. doi:10.1016/j.envsci.2020.09.022
Bellamy, R., and Geden, O. (2019). Govern CO2 removal from the ground up. Nat. Geosci. 12 (11), 874–876. doi:10.1038/s41561-019-0475-7
Bevan, L. D. (2022). The ambiguities of uncertainty: a review of uncertainty frameworks relevant to the assessment of environmental change. Futures 137, 102919. doi:10.1016/j.futures.2022.102919
Brouwer, R., and De Blois, C. (2008). Integrated modelling of risk and uncertainty underlying the cost and effectiveness of water quality measures. Environ. Model. Softw. 23 (7), 922–937. doi:10.1016/j.envsoft.2007.10.006
Drax Global (2022). BECCS and negative emissions - Drax Global. [online] Available at: https://www.drax.com/about-us/our-projects/bioenergy-carbon-capture-use-and-storage-beccs/ (Accessed November 28, 2023).
European Commission (2022). Brussels. https://climate.ec.europa.eu/system/files/2022-03/c_2022_1571_annex_en.pdf.ANNEX to the Commission Decision on the award of grants under the Innovation Fund – first call for large-scale proposals
European Commission (n.d.). Carbon removal certification. [online] climate.ec.europa.eu. Available at: https://climate.ec.europa.eu/eu-action/sustainable-carbon-cycles/carbon-removal-certification_en (Accessed May 29, 2023).
European Council (2023). Fit for 55’: Council adopts key pieces of legislation delivering on 2030 climate targets. [online] Consilium.europa.eu. Available at: https://www.consilium.europa.eu/en/press/press-releases/2023/04/25/fit-for-55-council-adopts-key-pieces-of-legislation-delivering-on-2030-climate-targets/ (Accessed May 29, 2023).
Fridahl, M., Hansson, A., Arbman Hansing, A., Gustafsson, K., Lehtveer, M., Bellamy, R., et al. (2018). Bioenergy with carbon capture and storage - from global potentials to domestic realities. Brussels: European Liberal Forum.
Fuss, S., and Johnsson, F. (2021). The BECCS implementation gap–A Swedish case study. Front. Energy Res. 8. doi:10.3389/fenrg.2020.553400
Gustafsson, K., Sadegh-Vaziri, R., Grönkvist, S., Levihn, F., and Sundberg, C. (2021). BECCS with combined heat and power: assessing the energy penalty. Int. J. Greenh. Gas Control 108, 103248. doi:10.1016/j.ijggc.2020.103248
Hadjimichael, A., Gold, D., Hadka, D., and Reed, P. (2020). Rhodium: Python library for many-objective robust decision making and exploratory modeling. J. Open Res. Softw. 8, 12. doi:10.5334/jors.293
Hammar, T., and Levihn, F. (2020). Time-dependent climate impact of biomass use in a fourth generation district heating system, including BECCS. Biomass Bioenergy 138, 105606. doi:10.1016/j.biombioe.2020.105606
Honegger, M., Poralla, M., Michaelowa, A., and Ahonen, H.-M. (2021). Who is paying for carbon dioxide removal? Designing policy instruments for mobilizing negative emissions technologies. Front. Clim. 3. doi:10.3389/fclim.2021.672996
IEA (2022a). Bioenergy with carbon capture and storage – analysis. [online] IEA. Available at: https://www.iea.org/reports/bioenergy-with-carbon-capture-and-storage (Accessed May 30, 2023).
IEA (2022b). Global energy and climate model. www.iea.org. Paris: International Energy Agency. Available at: https://iea.blob.core.windows.net/assets/2db1f4ab-85c0-4dd0-9a57-32e542556a49/GlobalEnergyandClimateModelDocumentation2022.pdf [Accessed 31 May 2023].
IPCC (2023). in Climate change 2023: synthesis report. A report of the intergovernmental panel on climate change. Contribution of working groups I, II and III to the sixth assessment report of the intergovernmental panel on climate change. [Core writing team. Editors H. Lee, and J. Romero (Geneva, Switzerland: IPCC). (in press.
Jenkins, S., Kuijper, M., Helferty, H., Girardin, C., and Allen, M. (2022). Extended producer responsibility for fossil fuels. Environ. Res. Lett. 18, 011005. doi:10.1088/1748-9326/aca4e8
Jenkins, S., Mitchell-Larson, E., Ives, M. C., Haszeldine, S., and Allen, M. (2021). Upstream decarbonization through a carbon takeback obligation: an affordable backstop climate policy. Joule 5 (11), 2777–2796. doi:10.1016/j.joule.2021.10.012
Jittrapirom, P., Marchau, V., van der Heijden, R., and Meurs, H. (2018). Dynamic adaptive policymaking for implementing mobility-as-a service (MaaS). Res. Transp. Bus. Manag. 27, 46–55. doi:10.1016/j.rtbm.2018.07.001
Khatiwada, D., Vasudevan, R. A., and Santos, B. H. (2022). Decarbonization of natural gas systems in the EU – costs, barriers, and constraints of hydrogen production with a case study in Portugal. Renew. Sustain. Energy Rev. 168, 112775. doi:10.1016/j.rser.2022.112775
Krzywinski, M., and Altman, N. (2017). Classification and regression Trees. Nat. Methods 14 (8), 757–758. doi:10.1038/nmeth.4370
Kwakkel, J. H., and Haasnoot, M. (2019). “Supporting DMDU: a taxonomy of approaches and tools,” in Decision making under deep uncertainty: from theory to practice (cham: Springer).
Kwakkel, J. H., Haasnoot, M., and Walker, W. E. (2016). Comparing robust Decision Making and dynamic adaptive policy pathways for modelbased decision support under deep uncertainty. Environmental Modelling and Software 86, 168–183. doi:10.1016/j.envsoft.2016.09.017
Lefvert, A., Rodriguez, E., Fridahl, M., Grönkvist, S., Haikola, S., and Hansson, A. (2022). What are the potential paths for carbon capture and storage in Sweden? A multi-level assessment of historical and current developments. Energy Research and Social Science 87, 102452. doi:10.1016/j.erss.2021.102452
Lempert, R. J. (2019). “Robust decision making (RDM),” in Decision making under deep uncertainty: from theory to practice (Cham: Springer).
Lempert, R. J., Groves, D. G., Popper, S. W., and Bankes, S. C. (2006). A general, analytic method for generating robust strategies and narrative scenarios. Manag. Sci. 52 (4), 514–528. doi:10.1287/mnsc.1050.0472
Lempert, R. J., Popper, S. W., and Bankes, S. C. (2003). Shaping the next one hundred years: new methods for quantitative, long-term policy analysis. Santa Monica, CA: RAND Corporation.
Levihn, F. (2017). CHP and heat pumps to balance renewable power production: lessons from the district heating network in Stockholm. Energy 137, 670–678. doi:10.1016/j.energy.2017.01.118
Levihn, F., Linde, L., Gustafsson, K., and Dahlén, E. (2019). Introducing BECCS through HPC to the research agenda: the case of combined heat and power in Stockholm. Energy Rep. 5, 1381–1389. doi:10.1016/j.egyr.2019.09.018
Lindroos, T. J., Rydén, M., Langørgen, Ø., Pursiheimo, E., and Pikkarainen, T. (2019). Robust decision making analysis of BECCS (bio-CLC) in a district heating and cooling grid. Sustainable Energy Technologies and Assessments 34, 157–172. doi:10.1016/j.seta.2019.05.005
Marchau, V. A. W. J., Walker, W. E., Bloemen, P. J. T. M., and Popper, S. W. (2019). Decision making under deep uncertainty: from theory to practice. 1st ed. Cham: Springer.
Minx, J. C., Lamb, W. F., Callaghan, M. W., Fuss, S., Hilaire, J., Creutzig, F., et al. (2018). Negative emissions—Part 1: research landscape and synthesis. Environ. Res. Lett. 13 (6), 063001. doi:10.1088/1748-9326/aabf9b
Möllersten, K., and Naqvi, R. (2022). Technology readiness assessment, costs, and limitations of five shortlisted NETs • accelerated mineralisation, biochar as soil additive, BECCS, DACCS, wetland restoration. [online] Researchgate.net. Available at: https://www.researchgate.net/publication/359427009_Technology_Readiness_Assessment_Costs_and_Limitations_of_five_shortlisted_NETs_Accelerated_mineralisation_Biochar_as_soil_additive_BECCS_DACCS_Wetland_restoration (Accessed November 15, 2022).
Möllersten, K., Zetterberg, L., Nielsen, T., Torvanger, A., Siikavirta, H., Kujanpää, L., et al. (2021). Policies for the promotion of BECCS in the nordic countries. TemaNord: Nordic Council of Ministers. doi:10.6027/temanord2021-538
Oda, J., and Akimoto, K. (2011). An analysis of CCS investment under uncertainty. Energy Procedia 4, 1997–2004. doi:10.1016/j.egypro.2011.02.081
Ørsted, (2023). Ørsted awarded contract – will capture and store 430,000 tonnes of biogenic CO2. [online] Orsted.com. Available at: https://orsted.com/en/media/newsroom/news/2023/05/20230515676011 (Accessed November 28, 2023).
Preece, R., and Milanovic, J. V. (2016). Efficient estimation of the probability of small-disturbance instability of large uncertain power systems. IEEE Trans. Power Syst. 31 (2), 1063–1072. doi:10.1109/tpwrs.2015.2417204
Regeringskansliet, (2022). Stor satsning görs på infångning av biogen koldioxid. [online] Regeringen.se. Available at: https://www.regeringen.se/pressmeddelanden/2022/11/stor-satsning-gors-pa-infangning-av-biogen-koldioxid/ (Accessed February 29, 2023).
Rickels, W., Proelß, A., Geden, O., Burhenne, J., and Fridahl, M. (2021). Integrating carbon dioxide removal into European emissions trading. Front. Clim. 3. doi:10.3389/fclim.2021.690023
Rodriguez, E., Lefvert, A., Fridahl, M., Grönkvist, S., Haikola, S., and Hansson, A. (2021). Tensions in the energy transition: Swedish and Finnish company perspectives on bioenergy with carbon capture and storage. J. Clean. Prod. 280, 124527. doi:10.1016/j.jclepro.2020.124527
Rosolem, R., Gupta, H. V., James, S. W., Zeng, X., and Gustavo, L. (2012). A fully multiple-criteria implementation of the Sobol′ method for parameter sensitivity analysis. J. Geophys. Res. Atmos. 117. doi:10.1029/2011JD016355
Schneller, G. O., and Sphicas, G. P. (1983). Decision making under uncertainty: starr’s domain criterion. Theory Decis. 15 (4), 321–336. doi:10.1007/BF00162111
Simon, H. A. (1959). Theories of decision-making in economics and behavioral science. Am. Econ. Rev. 49 (3), 253–283. Available at: https://www.jstor.org/stable/1809901.
Smith, S. M., Geden, O., Nemet, G. F., Gidden, M., Lamb, W. F., Powis, C., et al. (2023). The state of carbon dioxide removal. 1st Edition. [online] The State of Carbon Dioxide Removal. doi:10.17605/OSF.IO/W3B4Z
Song, J., Sun, Y., and Jin, L. (2017). PESTEL analysis of the development of the waste-to-energy incineration industry in China. Renew. Sustain. Energy Rev. 80 (1), 276–289. doi:10.1016/j.rser.2017.05.066
SOU (2020). Vägen till en klimatpositiv framtid [The Pathway to a Climate-PositiveFuture]. Stockholm: Statens Offentliga Utredningar.
Sovacool, B. K. (2021). Reckless or righteous? Reviewing the sociotechnical benefits and risks of climate change geoengineering. Energy Strategy Rev. 35, 100656. doi:10.1016/j.esr.2021.100656
Sovacool, B. K., Axsen, J., and Sorrell, S. (2018). Promoting novelty, rigor, and style in energy social science: towards codes of practice for appropriate methods and research design. Energy Res. Soc. Sci. 45, 12–42. doi:10.1016/j.erss.2018.07.007
Stanton, M. C. B., and Roelich, K. (2021). Decision making under deep uncertainties: a review of the applicability of methods in practice. Technol. Forecast. Soc. Change 171, 120939. doi:10.1016/j.techfore.2021.120939
Stenström, O., and Usher, W. (2023). ostenst/BECCS-Stockholm: review release (v.1.0). Zenodo. doi:10.5281/zenodo.8086484
Stockholm Exergi AB (2020). About BECCS Stockholm. [online] beccs.se. Available at: https://beccs.se/about-beccs-stockholm-2/ (Accessed April 27, 2023).
Stockholm Exergi AB (2022). Q&A about article 6 of the Paris agreement - Stockholm Exergi. [online] Stockholmexergi.se. Available at: https://www.stockholmexergi.se/en/cop27/qa-about-article-6-of-the-paris-agreement/ (Accessed May 30, 2023).
Stroombergen, A., and Lawrence, J. (2022). A novel illustration of real options analysis to address the problem of probabilities under deep uncertainty and changing climate risk. Clim. Risk Manag. 38, 100458. doi:10.1016/j.crm.2022.100458
Summit Carbon Solutions (2023). About us - Summit carbon solutions. [online] Available at: https://summitcarbonsolutions.com/about-us/ (Accessed November 28, 2023).
Swedish Energy Agency (2022). Article 6 of the Paris agreement. www.energimyndigheten.se. Available at: https://www.energimyndigheten.se/en/cooperation/swedens-program-for-international-climate-initiatives/paris-agreement/article-6-of-the-paris-agreement/#:∼:text=Article%206%20of%20the%20Paris%20Agreement%20allows%20for%20countries%20to [Accessed 30 May 2023].
UNFCCC (2015). Paris agreement. [online] Available at: https://unfccc.int/files/meetings/paris_nov_2015/application/pdf/paris_agreement_english_.pdf (Accessed September 26, 2022).
Vattenfall (2023). Historik över elpriserna på elbörsen - vattenfall. www.vattenfall.se. Available at: https://www.vattenfall.se/elavtal/elpriser/rorligt-elpris/prishistorik/ [Accessed 31 May 2023].
Walker, W., Marchau, V. A. W., and Kwakkel, J. H. (2019). “Dynamic adaptive planning (DAP),” in Decision making under deep uncertainty: from theory to practice (Cham: Springer).
Workman, M., Darch, G., Dooley, K., Lomax, G., Maltby, J., and Pollitt, H. (2021). Climate policy decision making in contexts of deep uncertainty - from optimisation to robustness. Environ. Sci. Policy 120, 127–137. doi:10.1016/j.envsci.2021.03.002
World Bank (2023). State and trends of carbon pricing 2023. ©. [online] Available at: http://hdl.handle.net/10986/39796 (Accessed May 31, 2023).
Keywords: carbon capture and storage, bioenergy, deep uncertainty, robust decision making, dynamic adaptive planning, case study, carbon dioxide removal, policy
Citation: Stenström O, Khatiwada D, Levihn F, Usher W and Rydén M (2024) A robust investment decision to deploy bioenergy carbon capture and storage—exploring the case of Stockholm Exergi. Front. Energy Res. 11:1250537. doi: 10.3389/fenrg.2023.1250537
Received: 04 July 2023; Accepted: 12 December 2023;
Published: 05 January 2024.
Edited by:
Edmundo Molina-Perez, Monterrey Institute of Technology and Higher Education (ITESM), MexicoReviewed by:
Arnaldo Walter, State University of Campinas, BrazilSteve Pye, University College London, United Kingdom
Tomi Lindroos, VTT Technical Research Centre of Finland Ltd., Finland
Copyright © 2024 Stenström, Khatiwada, Levihn, Usher and Rydén. This is an open-access article distributed under the terms of the Creative Commons Attribution License (CC BY). The use, distribution or reproduction in other forums is permitted, provided the original author(s) and the copyright owner(s) are credited and that the original publication in this journal is cited, in accordance with accepted academic practice. No use, distribution or reproduction is permitted which does not comply with these terms.
*Correspondence: Oscar Stenström, b3NjYXIuc3RlbnN0cm9tQGNoYWxtZXJzLnNl