- 1Earth System and Mitigation Science, Climate Impacts Modelling, Met Office Hadley Centre, Exeter, UK
- 2School of Earth and Environment, University of Leeds, Leeds, UK
- 3Department of Engineering, Centre for Sustainable Development, University of Cambridge, Cambridge, UK
The increasing use of multi-member climate model ensembles for making future climate impact assessments presents both opportunities for understanding uncertainties, and challenges for interpreting the results. We outline current approaches to assessing uncertainties in climate impacts, statistical methods for assessing uncertainties, issues regarding model integration and complexity, and ways in which uncertainty frameworks can be used to inform adaptation decisions, with case studies focused on agriculture. Finally, we highlight future research needs and provide recommendations for making further progress.
Introduction
Robust assessments of climate change impacts are important for assessing the scale of adaptation required, and for estimating the implications of climate mitigation pathways (Collins, 2007; IPCC, 2014). A comprehensive understanding of uncertainties in projected impacts is a key element of making robust assessments (Challinor et al., 2013; Katz et al., 2013). Uncertainties arise from a range of sources in climate projections (model structural differences, initial conditions, scenarios, parameters and resolution/bias-correction), climate impact models (CIMs) and observations (e.g., Challinor et al., 2009a,b; Hawkins and Sutton, 2009; Osborne et al., 2013). Multi-member model ensembles (Collins et al., 2010, for example) and model intercomparison projects (MIPs) are used to assess uncertainties in future climate and climate impacts. These studies include the Coupled MIP (CMIP—Taylor et al., 2012), Water MIP (WaterMIP—Haddeland et al., 2011), the Agricultural MIP (AgMIP—Rosenzweig et al., 2013), and the Inter-Sectoral Impacts MIP (ISI-MIP—Warszawski et al., 2013), which contributed to the IPCC reports (IPCC, 2013, 2014).
The use of ensemble projections and the outputs of MIPs in impact assessments raises the issue of how to interpret the resulting uncertainty ranges (e.g., Smith et al., 2009; Knutti et al., 2010; Tebaldi et al., 2011), which are dependent on experimental design. For instance, “high-end” impacts (e.g., under global mean temperature changes >4 K) are less sampled (Challinor et al., 2009a, 2010). Uncertainty ranges may be interpreted differently by scientists and decision-makers, potentially resulting in poor decision making.
The appropriate treatment of uncertainty ranges will vary according to the nature of adaptation required. In agriculture for example, adaptation could mean coping (altering planting dates or crop varieties), adjusting (new crops or livestock species), or transforming (new production systems, livelihoods, migration). Bottom-up approaches (beginning by assessing the decision-making context) are important for incremental (coping) strategies while top-down approaches (beginning with climate scenarios) are important for transformative strategies (Vermeulen et al., 2013; Figure 1). Our aim is to highlight challenges in using ensembles to assess uncertainties in future climate impacts and identify priorities for making further progress.
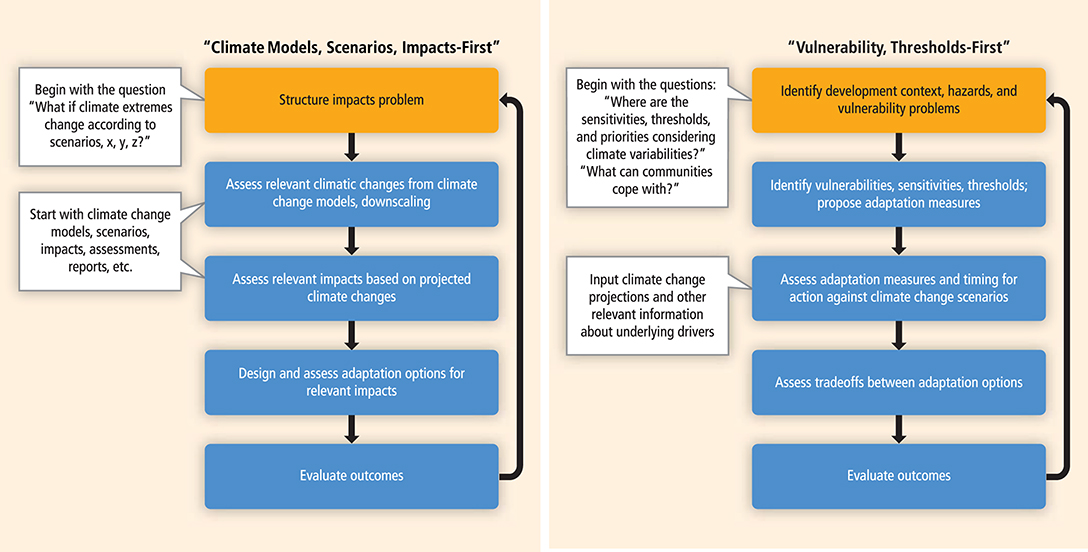
Figure 1. Top-down scenario, impacts-first approach (left panel) and bottom-up vulnerability, thresholds-first approach (right panel)—comparison of stages involved in identifying and evaluating adaptation options under changing climate conditions (© IPCC, 2012; from Lal et al., 2012).
Statistical Methods for Understanding and Reducing Uncertainty
Large-scale models of natural and physical systems inherently contain uncertainties. These uncertainties originate from the complex nature of the system, and from our limited knowledge of it. Uncertainty is described in a variety of ways, from qualitative statements such as “likely” and “unlikely,” or “low,” “medium” and “high” confidence (IPCC, 2012, 2013, 2014) to quantitative representations like a range of plausible values, a standard deviation or a full probability distribution providing confidence bounds.
Multi-Model Diversity
For models of large-scale complex systems, a common approach to assessing model uncertainty is the use of MIPs (see introduction). This involves taking a selection of models which often differ in their representation of system processes, and evaluating their outputs for a range of scenarios. The resulting comparisons allow uncertainty in predictions due to model diversity to be quantified. However, these estimates must be used with caution if the models compared are not independent, or contain mutual errors and omissions (e.g., regional precipitation biases), as this can lead to unrepresentative estimates of uncertainty. It can also be difficult to determine the causes of uncertainty since the resulting ensemble contains little information on how process representations affect the outputs (Davie et al., 2013).
By focusing on a single model, sources of uncertainty within that model may be evaluated more rigorously (Deser et al., 2012). While uncertainty is quantified for only one model, evaluation of how the uncertainty in the processes and parameters within that model affects the outputs can be very informative, leading to more focused model development.
Parametric Uncertainty
There are many statistical tools for understanding model parametric uncertainty. Classical methods involve direct Monte Carlo simulation, where the entire parameter space is explored by running the simulator for a large number of input parameter combinations and evaluating the model response (e.g., the Generalized Likelihood Uncertainty Estimation: GLUE (Beven, 2007) method used in hydrology). For a complex model with a large run time this may become unfeasible, although distributed experiments such as climateprediction.net are pioneering such approaches (Stainforth et al., 2005).
Bayesian statistical framework-based approaches have been used to overcome such computational barriers. For example, Lee et al. (2013) explored the sensitivity of cloud condensation nuclei (CCN) concentration estimates to parameters in a global aerosol model following the Bayesian approach of Oakley and O'Hagan (2004). Here, Gaussian process emulation (O'Hagan, 2006) was used to reduce the computational cost and probabilistic variance-based sensitivity analysis (Saltelli et al., 2000) was applied to evaluate which inputs were driving uncertainty in the outputs. This approach is rarely used for assessing climate change impacts, in comparison to projections of climate.
Inverse modeling approaches involving Markov chain Monte Carlo simulation to evaluate a full Bayesian posterior parameter distribution have been used to assess the effects of parameter uncertainty for aerosol-cloud interactions (Partridge et al., 2012) and hydrology (Vrugt et al., 2008). The comparison of model simulations to observations (model calibration) and the use of observations to reduce an uncertain parameter space (history matching) can help to assess model uncertainty. For example, McNeall et al. (2013) show the potential of these methods to constrain a complex ice sheet model.
Treatment of Uncertainty in Impact Studies: A Case Study for Wheat
Climate impact studies depend on the choice of climate data and the impact model used. Recent impact studies have used climate model ensembles to account for the uncertainty due to global climate models (GCMs) and their parameterizations, building on earlier approaches using small numbers of scenarios or models. Less emphasis has been placed in making progress via impact studies using raw GCM output (e.g., Falloon et al., 2011; Taylor et al., 2013; Betts et al., 2013; Mathison et al., 2013), and in understanding uncertainty introduced through downscaling methods, bias-correction methods or the use of weather generators. Bias-correction methods for GCM output may be as important as GCM uncertainty (e.g., Done et al., 2013), and potentially greater than uncertainty due to scenarios as shown for the number of summer days where maximum temperature exceeds a certain threshold across Europe (Hawkins et al., 2013).
There is considerable diversity in present crop models (Rivington and Koo, 2010): e.g., statistical models based on observed relationships, dynamic process-based models for particular crop types, and generalized large and field scale process-based models. Crop models vary in their complexity, how they simulate dynamic processes (e.g., crop development), and which processes they simulate (e.g., high temperature stress around anthesis and/or microclimate). Crop models based on observed relationships have set parameters for a given cultivar (“genetic coefficients”), determined through field experiments; thus not accounting for parametric uncertainty. Regional scale crop models may be optimized to observed yield data and a parameter ensemble may be used (Challinor et al., 2009a). Impact model studies are limited by the number of observable output variables that can be used for parameterization, regardless of the approach taken and the application.
Several studies have assessed the importance of different sources of uncertainties in crop models. An ensemble of wheat models performed well compared to experimental data from four contrasting growing environments but only when provided with sufficient calibration data (Asseng et al., 2013). With increasing temperatures, crop development may make a large contribution to uncertainties in simulated impact, both between models (Asseng et al., 2013) and in one model exploring a range of common functions and cardinal temperature settings (Koehler et al., 2013). Changes in growing season precipitation affected simulated yield but showed little change in the variation between models in simulated yield change (Asseng et al., 2013). Warming may expose crops to more high temperature stress around anthesis (the onset of flowering). However, not all models include a direct temperature effect during anthesis, and accounting for anthesis may not result in correctly simulating the effect (Asseng et al., 2013), illustrating the importance of understanding model behavior for predictive uses. For processes where threshold values are important (e.g., heat stress), ignoring microclimate may lead to large systematic errors, since in irrigated systems panicle (loose branching flower cluster) temperatures may vary strongly from air temperature depending on vapor pressure deficit (Julia and Dingkuhn, 2013).
Lessons learnt from the AgMIP-wheat pilot study (Asseng et al., 2013) were taken forward into the next set of simulations, which cover a wide temperature range during different growth stages with non-limited conditions for nutrients and water (Ottman et al., 2012). Crop models are often developed for a specific region and/or purpose and may depend on regional characteristics. With the strong focus on global studies, the same crop model may be applied outside its “design region,” so key processes for crop growth need to be identified, and contrasting regions (e.g., hot humid versus hot dry) should be prioritized in MIPs.
Does Uncertainty Increase with Greater Integration of Impacts?
A more comprehensive integration of sectors and processes in Earth System Models (ESMs) and CIMs should provide a more complete picture of system behavior since impacts and the earth system are interlinked in reality. There are also numerous feedbacks between impacts and weather and climate (Falloon and Betts, 2010). Critical thresholds and non-linear responses also exist between impacts and climate drivers, such as the effect of extreme temperature on crop flowering (Wheeler et al., 2000).
To illustrate the interactions between climate impacts, we describe how water systems may be affected by climate change with implications for agriculture (Falloon and Betts, 2010). Direct climate effects on water include changing location, amounts and timing of precipitation, snowmelt, runoff, evaporation and groundwater recharge. Indirect effects include altered water management practices, responding to the changing climate and direct effects. For example, higher summer temperatures may increase industrial and domestic water demand, increase abstraction and reduce river flows. This may increase inter-user competition for water, affecting agricultural water availability. Higher summer temperatures may increase agricultural water demands, further increasing inter-sectoral pressures.
Feedbacks between climate and impacts include both changes in local and remote weather and climate, and biophysical and biogeochemical effects. For example, Falloon et al. (2012) used an ESM to investigate the effect of future vegetation change on the climate itself, finding warming of ~1 K in high latitudes where forest expansion reduced albedo, and over the Amazon where reduced tree cover reduced evaporative cooling. Carbon storage increased in the high latitudes but was reduced over Amazonia. McCarthy et al. (2010) showed that the inclusion of cities in a climate model led to increased frequency of extreme hot nights, due to both the urban land surface and due to increased anthropogenic heat sources.
Several approaches may be taken to integrate climate impacts. Bio-physical impacts may be linked in “online” approaches where impacts are included within weather/climate models. “Offline” approaches may link different impacts in stand alone models driven by climate model outputs (e.g., Krysanova et al., 2007; Mahmood et al., 2007; Davie et al., 2013). Integrating biophysical impacts with socioeconomic factors is complex but approaches include integrated assessment models (e.g., Warren et al., 2008), global economic models (e.g., linking crops, trade, irrigation and river flows—Calzadilla et al., 2013), and loose linkages (e.g., Barthel et al., 2008).
There are a range of potential issues to consider when integrating climate impacts. In some cases, meaningful comparisons between models and observations may be challenging. For instance, ESMs provide a wide range of estimates of contemporary soil C stocks (510–3040 Pg C), and observational estimates vary widely (500–1260 Pg C). There are also differences in what is represented by models (Todd-Brown et al., 2013). It may be difficult to find data to parameterize impact models for all the processes needed to realistically reproduce observed behavior (Challinor et al., 2013). Finite computing resources mean that tradeoffs will need to be made between complexity/breadth, detail, and risk assessment—the ability to represent multiple sectors, processes and sample uncertainties using large ensembles. The ISI-MIP study suggested that ensembles of both impact and climate models are needed for making robust future assessments, although the bias-correction applied to the climate model output may alter the impacts (e.g., Ehret et al., 2012; Hawkins et al., 2013). Finally, interactions between biophysical impacts and socioeconomic drivers are important, including decisions made on small space/time scales.
Several approaches may be taken to assessing the appropriate level of model integration and complexity for a particular purpose. Testing for relationships between observed impact (e.g., crop yield) and weather would provide a top-level justification for combining models of impact and climate (e.g., Challinor et al., 2003), as would the existence of significant feedbacks between impact and climate (e.g., river flow impact on ocean circulation; carbon cycle; or albedo). Several authors have selectively removed model components or fixed parameters, and then retested model performance (e.g., Crout et al., 2009; Tarsitano et al., 2011). This may be challenging with large, complex models. The appropriate level of model integration will also depend on the time and space scale in question, the location, climate and needs of stakeholders (Challinor et al., 2009a).
How Should Uncertainty be Treated for Adaptation?
Adapting to a changing climate is a complex societal process of activities, actions, decisions and attitudes that reflect existing social norms and processes (Adger et al., 2005). Systems and actors do not adapt to climate change in isolation—adaptation happens in a world of multiple stresses and drivers of which climate is one. The significance of the climate driver depends on the adaptation context.
There are two approaches for evaluating climate adaptation options (IPCC, 2012; Figure 1): “top-down” and “bottom-up.” The top-down climate models, scenario, impacts-first approach starts with the question: “how will future climate change?” (Dessai and van der Sluijs, 2011), and the assessment of uncertainty at each stage led Schneider (2001) to coin the phrase: “cascade and explosion of uncertainty” (Figure 2). Once relevant impacts have been assessed, adaptation options can be designed and assessed. Given the uncertainties involved in climate impact assessments, a more fruitful approach may be to begin with the decision-making context (Dessai et al., 2009a,b; Wilby and Dessai, 2010). Such bottom-up, vulnerability, threshold-first approaches first identify vulnerabilities, sensitivities, and thresholds to proposed adaptation measures. These measures and their timing are assessed against present and future uncertain drivers of which climate is but one. The characterization of climate uncertainty should be commensurate with that of other uncertain drivers in adaptation assessments. Rigorous sensitivity analysis of models that inform adaptation decisions (e.g., Saltelli et al., 2000) can produce a more robust assessment of relevant uncertainties, which is more fit-for-purpose for decision making. However, individual responses to climate change and adaptation may themselves be a significant source of uncertainty.
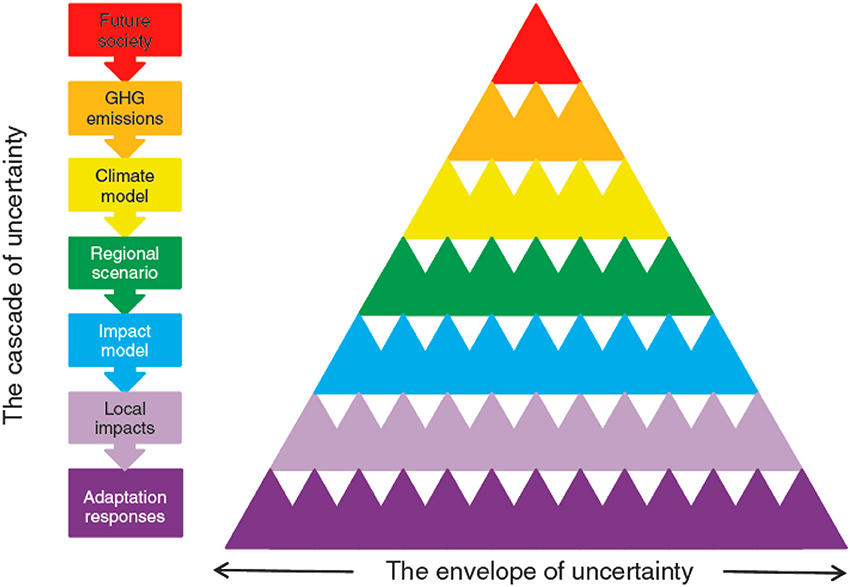
Figure 2. The cascade of uncertainty from illustrating the growth of the envelope of uncertainty from various sources going from future society to adaptation response. Used with permission from Wilby and Dessai, 2010, (© Royal Meteorological Society, 2010).
The top-down approach has been dominated by climate model projections. A bottom-up approach can use climate model information to characterize climate uncertainty. However, other approaches (that are potentially more appropriate alongside other uncertain drivers) include climate change narratives (e.g., a description of how circulation changes will affect climate in a location), expert judgment and theory based principles. The bottom-up approach enables the assessment of trade-offs between different adaptation options due to different uncertainties (e.g., climate, socio-economic, land use changes) and multiple criteria (e.g., maximizing expected utility, saving lives, protecting the environment).
While the top-down approach is still very common, there is an emerging body of work which applies the bottom up approach, particularly in water resources (Risbey, 1998; Lempert and Groves, 2010; Prudhomme et al., 2010; Korteling et al., 2013). The bottom-up approach requires information providers to work and communicate closely with decision makers (Dilling and Lemos, 2011) to understand their plans and goals, before tailoring the uncertainty description to focus on key factors. This can be very effective, but often needs to be individually customized for each decision context (Lempert and Kalra, 2011; Lempert et al., 2012).
Recommendations and Future Research Needs
While the design and implementation of MIPs tends to focus on comparing model responses (outputs), there is a need to consider how they can be better used to improve and develop models, and to synthesize knowledge more effectively (Challinor et al., 2014). For instance, multiple variables (e.g., nitrogen availability, water use, crop quality, yield) could be assessed within single (e.g., crop model) impact studies.
The interlinked nature of climate and impacts requires comprehensive treatments of uncertainty including relationships/feedbacks between climate and its impacts. A more consistent approach should be taken to assessing the effect of model integration, particularly considering “offline” and “online” approaches. Key issues include the ability to model the individual components at the right level of complexity, whether the components need to be modeled individually or together, impacts on model performance, and whether integration provides more insight into system behavior. There is a need to assess the benefits and tradeoffs between model complexity, resolution and ensemble size for making impact assessments.
The use of statistical methods to assess uncertainty should be promoted. The growing use of statistical methods to evaluate, understand and reduce model uncertainty means that more robust conclusions can be obtained from model applications, including an assessment of uncertainties. This can also lead to an improved understanding of model behavior, indicating where a model can be most improved and where greatest confidence can be placed in results. Impact modeling approaches may benefit from experiences gained in the climate modeling community (and potentially vice-versa).
The usability of impact assessments for decision making could be improved through:
• Greater clarity in the methods and assumptions used (e.g., use of GCMs, scenarios, timescales, ensembles, bias correction, downscaling, and impact models), and assessment of their impact on results (e.g., Watson and Challinor, 2013).
• Appropriate selection of assessment approaches—for instance a top-down framing when uncertainties are shallow (and for broad scoping assessments), or a bottom-up framing when uncertainties are deep (Dessai et al., 2009a).
• Common, clear ways of reporting and describing uncertainties (e.g., Challinor and Visman, 2014).
• The use of models as tools from which information is extracted, rather than as competing attempts to represent reality (Challinor et al., 2003).
• Assessment of methods which go directly from climate model to decision parameter, removing intermediate steps and potentially reducing embedded uncertainty (e.g., Holland et al., 2010).
Conflict of Interest Statement
The authors declare that the research was conducted in the absence of any commercial or financial relationships that could be construed as a potential conflict of interest.
Acknowledgments
This article was supported the Joint DECC/Defra Met Office Hadley Centre Climate programme (GA01101) and the EUPORIAS project, funded by the European Commission 7th Framework Programme for Research, grant agreement 308291. Jill Johnson is funded by the NERC project “Aerosol-Cloud Interactions—a Directed Programme to Reduce Uncertainty in Forcing.” (ACID-PRUF), grant ref. NE/I020059/1. Suraje Dessai is supported by the European Research Council (ERC) under the European Union’s Seventh Framework Programme for Research (FP7/2007-2013), ERC Grant agreement 284369. This paper resulted from discussions during a Met Office Group at the University of Leeds (MOGUL) workshop on ensembles and uncertainty in climate impacts, held in Leeds on 12th December 2013.
References
Adger, W. N., Arnell, N. W., and Tompkins, E. L. (2005). Successful adaptation to climate change across scales. Glob. Environ. Change 15, 77–86. doi: 10.1016/j.gloenvcha.2004.12.005
Asseng, S., Ewert, F., Rosenzweig, C., Jones, J. W., Hatfield, J. L., Ruane, A. C., et al. (2013). Uncertainty in simulating wheat yields under climate change. Nat. Clim. Change 3, 827–832. doi: 10.1038/nclimate1916
Barthel, R., Janisch, S., Schwarz, N., Trifkovic, A., Nickel, D., Schulz, C., et al. (2008). An integrated modelling framework for simulating regional-scale actor responses to global change in the water domain. Environ. Model. Softw. 23,1095–1121. doi: 10.1016/j.envsoft.2008.02.004
Betts, R. A., Golding, N., Gonzalez, P., Gornall, J., Kahana, R., Kay, G., et al. (2013). Climate and land use change impacts on global terrestrial ecosystems, fire, and river flows in the HadGEM2-ES Earth system model using the representative concentration pathways. Biogeosci. Discuss. 10, 6171–6223. doi: 10.5194/bgd-10-6171-2013
Beven, K. J. (2007). Towards integrated environmental models of everywhere: uncertainty, data and modelling as a learning process. Hydrol. Earth Syst. Sci. 11, 460–467. doi: 10.5194/hess-11-460-2007
Calzadilla, A., Rehdanz, K., Betts, R., Falloon, P., Wiltshire, A., and Tol, R. S. J. (2013). Climate change impacts on global agriculture. Clim. Change 120, 357–374. doi: 10.1007/s10584-013-0822-4
Challinor, A., Martre, P., Asseng, P., Thornton, P., and Ewert, F. (2014). Making the most of climate impacts ensembles. Nat. Clim. Change 4, 77–80. doi: 10.1038/nclimate2117
Challinor, A., and Visman, E. (2014). Climate Impacts: Taking Action in the Face of Uncertainty, LWEC Policy and Practice Note 05. LWEC, Swindon. Available online at http://www.lwec.org.uk/sites/default/files/attachments_biblio/15433%20LWEC%20P%26P%20Note%2005_WEB.pdf
Challinor, A. J., Ewert, F., Arnold, S., Simelton, E., and Fraser, E. (2009b). Crops and climate change: progress, trends, and challenges in simulating impacts and informing adaptation. J. Exp. Bot. 60, 2775–2789. doi: 10.1093/jxb/erp062
Challinor, A. J., Simelton, E. S., Fraser, E. D. G., Hemming, D., and Collins, M. (2010). Increased crop failure due to climate change: assessing adaptation options using models and socio-economic data for wheat in China. Environ. Res. Lett. 5:034012. doi: 10.1088/1748-9326/5/3/034012
Challinor, A. J., Slingo, J. M., Wheeler, T. R., Craufurd, P. Q., and Grimes, D. I. F. (2003). Toward a combined seasonal weather and crop productivity forecasting system: determination of the working spatial scale. J. Appl. Meteorol. 42, 175–192. doi: 10.1175/1520-0450(2003)042<0175:TACSWA>2.0.CO;2
Challinor, A. J., Thornton, P., and Smith, M. S. (2013). Use of agro-climate ensembles for quantifying uncertainty and informing adaptation. Agric. For. Meteorol. 170, 2–7. doi: 10.1016/j.agrformet.2012.09.007
Challinor, A. J., Wheeler, T., Hemming, D., and Upadhyaya, H. D. (2009a). Ensemble yield simulations: crop and climate uncertainties, sensitivity to temperature and genotypic adaptation to climate change. Clim. Res. 38, 117–127. doi: 10.3354/cr00779
Collins, M. (2007). Ensembles and probabilities: a new era in the prediction of climate change. Philos. Trans. R. Soc. A 365, 1957–1970. doi: 10.1098/rsta.2007.2068
Collins, M., Booth, B. B. B., Bhaskaran, B., Harris, G. R., Murphy, J. M., Sexton, D. M. H., et al. (2010). Climate model errors, feedbacks and forcings: a comparison of perturbed physics and multi-model ensembles. Clim. Dyn. 36, 1737–1766. doi: 10.1007/s00382-010-0808-0
Crout, N. M. J., Tarsitano, D., and Wood, A. T. A. (2009). Is my model too complex? Evaluating model formulation using model reduction. Environ. Model. Softw. 24, 1–7. doi: 10.1016/j.envsoft.2008.06.004
Davie, J. C. S., Falloon, P. D., Kahana, R., Dankers, R., Betts, R., Portmann, F. T., et al. (2013). Comparing projections of future changes in runoff from hydrological and biome models in ISI-MIP. Earth Syst. Dyn. 4, 359–374. doi: 10.5194/esd-4-359-2013
Deser, C., Phillips, A. S., Bourdette, V., and Teng, H. (2012). Uncertainty in climate change projections: the role of internal variability. Clim. Dyn. 38, 527–546. doi: 10.1007/s00382-010-0977-x
Dessai, S., Hulme, M., Lempert, R., and Pielke, R. Jr. (2009a). “Climate prediction: a limit to adaptation?,” in Adapting to Climate Change: Thresholds, Values, Governance, eds W. N. Adger, I. Lorenzoni, and K. L. O'Brien (Cambridge: Cambridge University Press), 64–78.
Dessai, S., Hulme, M., Lempert, R., and Pielke, R. (2009b). Do we need better predictions to adapt to a changing climate? Eos Trans. Am. Geophys. Union 90, 111–112. doi: 10.1029/2009EO130003
Dessai, S., and van der Sluijs, J. (2011). “Modelling climate change impacts for adaptation assessments,” in Simplicity, Complexity and Modelling, eds M. Christie, A. Cliffe, P. Dawid and S. Senn (Chichester: John Wiley & Sons Ltd), 83–102.
Dilling, L., and Lemos, M. C. (2011). Creating usable science: opportunities and constraints for climate knowledge use and their implications for science policy. Glob. Environ. Change 21, 680–689. doi: 10.1016/j.gloenvcha.2010.11.006
Done, J. M., Holland, G. J., Bruyere, C. L., Ruby Leung, L., and Suzuki-Parker, A. (2013). Modeling high-impact weather and climate: lessons from a tropical cyclone perspective. Clim. Change. doi: 10.1007/s10584-013-0954-6
Ehret, U., Zehe, E., Wulfmeyer, V., Warrach-Sagi, K., and Liebert, J. (2012). HESS opinions “Should we apply bias correction to global and regional climate model data?”. Hydrol. Earth Syst. Sci. 16, 3391–3404. doi: 10.5194/hess-16-3391-2012
Falloon, P., Betts, R., Wiltshire, A., Dankers, R., Mathison, C., McNeall, D., et al. (2011). Validation of river flows in HadGEM1 and HadCM3 with the TRIP river flow model. J. Hydrometeorol. 12, 1157–1180. doi: 10.1175/2011JHM1388.1
Falloon, P. D., and Betts, R. A. (2010). Climate impacts on European agriculture and water management in the context of adaptation and mitigation - the importance of an integrated approach. Sci. Tot. Environ. 408, 5667–5687. doi: 10.1016/j.scitotenv.2009.05.002
Falloon, P. D., Dankers, R., Betts, R. A., Jones, C. D., Booth, B. B. B., and Lambert, F. H. (2012). Role of vegetation change in future climate under the A1B scenario and a climate stabilisation scenario, using the HadCM3C Earth system model. Biogeosciences 9, 4739–4756. doi: 10.5194/bg-9-4739-2012
Haddeland, I., Clark, D. B., Franssen, W., Ludwig, F., Voß, F., Arnell, N. W., et al. (2011). Multimodel estimate of the global terrestrial water balance: setup and first results. J. Hydrometeorol. 12, 869–884. doi: 10.1175/2011JHM1324.1
Hawkins, E., Osborne, T. M., Ho, C. K., and Challinor, A. J. (2013). Calibration and bias correction of climate projections for crop modelling: an idealised case study over Europe. Agric. For. Meteorol. 170, 19–31. doi: 10.1016/j.agrformet.2012.04.007
Hawkins, E., and Sutton, R. (2009). The potential to narrow uncertainty in regional climate predictions. Bull. Am. Meteor. Soc. 90, 1095–1107. doi: 10.1175/2009BAMS2607.1
Holland, G. J., Done, J., Bruyere, C., Cooper, C., and Suzuki-Parker, A. (2010). “Model investigations of the effects of climate variability and change on future gulf of mexico tropical cyclone activity,” in Offshore Technology Conference (Houston, TX: ASCE, OTC20690), 1–13.
IPCC. (2012). “Managing the risks of extreme events and disasters to advance climate change adaptation,” in A Special Report of Working Groups I and II of the Intergovernmental Panel on Climate Change, eds C. B Field, V. Barros, T. F. Stocker, D. Qin, D. J. Dokken, K. L. Ebi, et al. (Cambridge; New York: Cambridge University Press), 522.
IPCC. (2013). “Climate change 2013: the physical science basis,” in Contribution of Working Group I to the Fifth Assessment Report of the Intergovernmental Panel on Climate Change, eds T. F. Stocker, D. Qin, G.-K. Plattner, M. Tignor, S. K. Allen, J. Boschung, et al. (Cambridge; New York: Cambridge University Press), 1535.
IPCC. (2014). “Climate change 2014: impacts, adaptation, and vulnerability. Part A: global and sectoral aspects,” in Contribution of Working Group II to the Fifth Assessment Report of the Intergovernmental Panel on Climate Change, eds C. B. Field, V.R. Barros, D. J. Dokken, K. J. Mach, M. D. Mastrandrea, T. E. Bilir, et al. (Cambridge; New York: Cambridge University Press).
Julia, C., and Dingkuhn, M. (2013). Predicting temperature induced sterility of rice spikelets requires simulation of crop-generated microclimate. Eur. J. Agron. 49, 50–60. doi: 10.1016/j.eja.2013.03.006
Katz, R. W., Craigmile, P. F., Guttorp, P., Haran, M., Sansó, B., and Stein, M. L. (2013). Uncertainty analysis in climate change assessments. Nat. Clim. Change 3, 769–771. doi: 10.1038/nclimate1980
Knutti, R., Furrer, R., Tebaldi, C., Cermak, J., and Meehl, G. A. (2010). Challenges in combining projections from multiple climate models. J. Clim. 23, 2739–2758. doi: 10.1175/2009JCLI3361.1
Koehler, A.-K., Challinor, A. J., Hawkins, E., and Asseng, S. (2013). Influences of increasing temperature on Indian wheat: quantifying limits to predictability. Environ. Res. Lett. 8:034016. doi: 10.1088/1748-9326/8/3/034016
Korteling, B., Dessai, S., and Kapelan, Z. (2013). Using information-gap decision theory for water resources planning under severe uncertainty. Water Resour. Manage. 27, 1149–1172. doi: 10.1007/s11269-012-0164-4
Krysanova, V., Hattermann, F., and Wechsung, F. (2007), Implications of complexity and uncertainty for integrated modelling and impact assessment in river basins. Environ. Model. Softw. 22, 701–709. doi: 10.1016/j.envsoft.2005.12.029
Lal, P. N., Mitchell, T., Aldunce, P., Auld, H., Mechler, R., Miyan, A., et al. (2012). “National systems for managing the risks from climate extremes and disasters,” in Managing the Risks of Extreme Events and Disasters to Advance Climate Change Adaptation. A Special Report of Working Groups I and II of the Intergovernmental Panel on Climate Change (IPCC), eds C. B. Field, V. Barros, T. F. Stocker, D. Qin, D. J. Dokken, K. L. Ebi, et al. (Cambridge, UK; New York, NY: Cambridge University Press), 339–392.
Lee, L. A., Pringle, K. J., Reddington, C. L., Mann, G. W., Stier, P., Spracklen, D. V., et al. (2013). The magnitude and causes of uncertainty in global model simulations of cloud condensation nuclei. Atmos. Chem. Phys. 13, 8879–8914. doi: 10.5194/acp-13-8879-2013
Lempert, R., and Groves, D. (2010). Identifying and evaluating robust adaptive policy responses to climate change for water management agencies in the American West. Technol. Forecast. Soc. Change 77, 960–974. doi: 10.1016/j.techfore.2010.04.007
Lempert, R., Sriver, R. L., Keller, K., and Commission, C. E. (2012). Characterizing Uncertain Sea Level Rise Projections to Support Investment Decisions. Sacramento, CA: California Energy Commission.
Lempert, R. J., and Kalra, N. (2011). “Managing climate risks in developing countries with robust decision making,” in World Resources Report EP-201100-254 (Washington, DC: World Resources Institute), 9.
Mahmood, R., Hubbard, K. G., and Pielke Sr, R. (2007). Effect of human activities on the atmosphere. Eos Trans. Am. Geophys. Union 88, 580. doi: 10.1029/2007EO520007
Mathison, C., Wiltshire, A., Kumar, P., Dimri, A. P., Ridley, J., Jacob, D., et al. (2013). Regional projections of south asian climate for adaptation studies. Sci. Tot. Environ. 468, 4–17. doi: 10.1016/j.scitotenv.2012.04.066
McCarthy, M. P., Best, M. J., and Betts, R. A. (2010). Climate change in cities due to global warming and urban effects. Geophys. Res. Lett. 37:L09705. doi: 10.1029/2010GL042845
McNeall, D. J., Challenor, P. G., Gattiker, J. R., and Stone, E. J. (2013). The potential of an observational data set for calibration of a computationally expensive computer model. Geosci. Model Dev. 6, 1715–1728. doi: 10.5194/gmd-6-1715-2013
O'Hagan, A. (2006). Bayesian analysis of computer code outputs: a tutorial. Reliab. Eng. Syst. Safe. 91, 1290–1300. doi: 10.1016/j.ress.2005.11.025
Oakley, J. E., and O'Hagan, A. (2004). Probabilistic sensitivity analysis of complex models: a Bayesian approach. J. R. Statist. Soc. B. 66, 751–769. doi: 10.1111/j.1467-9868.2004.05304.x
Osborne, T., Rose, G., and Wheeler, T. (2013). Variation in the global-scale impacts of climate change on crop productivity due to climate model uncertainty and adaptation. Agric. For. Meteorol. 170, 183–194. doi: 10.1016/j.agrformet.2012.07.006
Ottman, M. J., Kimball, B. A., White, J. W., and Wall, G. W. (2012). Wheat growth response to increased temperature from varied planting dates and supplemental infrared heating. Agron. J. 104, 7. doi: 10.2134/agronj2011.0212
Partridge, D. G., Vrugt, J. A., Tunved, P., Ekman, A. M. L., Struthers, H., and Sorooshian, A. (2012). Inverse modelling of cloud-aerosol interactions – Part 2: sensitivity tests on liquid phase clouds using Markov chain Monte Carlo based simulation approach. Atmos. Chem. Phys. 12, 2823–2847. doi: 10.5194/acp-12-2823-2012
Prudhomme, C., Wilby, R. L., Crooks, S., Kay, A. L., and Reynard, N. S. (2010). Scenario-neutral approach to climate change impact studies: application to flood risk. J. Hydrol. 390, 198–209. doi: 10.1016/j.jhydrol.2010.06.043
Risbey, J. S. (1998). Sensitivities of water supply planning decisions to streamflow and climate scenario uncertainties. Water Policy 1, 321–340. doi: 10.1016/S1366-7017(98)00023-3
Rivington, M., and Koo, J. (2010). “Report on the meta-¬ analysis of crop modelling for climate change and food security survey,” CGIAR Program on Climate Change, Agriculture and Food Security (CCAFS) (Copenhagen), 73. Available online at: http://r4d.dfid.gov.uk/PDF/Outputs/CCAFS/meta-analysis_of_crop_modelling_for_ccafs.pdf
Rosenzweig, C., Jones, J. W., Hatfield, J. L., Ruane, A. C., Boote, K. J., Thorburn, P. J., et al. (2013). The Agricultural Model intercomparison and Improvement Project (AgMIP): protocols and pilot studies. Agric. For. Meteorol. 170, 166–182. doi: 10.1016/j.agrformet.2012.09.011
Saltelli, A., Chan, K., and Scott, E. M. (2000). Sensitivity Analysis. Chichester: John Wiley & Sons Ltd.
Schneider, S. H. (2001). What is ‘dangerous’ climate change? Nature 411, 17–19. doi: 10.1038/35075167
Smith, R. L., Tebaldi, C., Nychka, D., and Mearns, L. O. (2009). Bayesian modeling of uncertainty in ensembles of climate models. J. Am. Stat. Assoc. 104, 97116. doi: 10.1198/jasa.2009.0007
Stainforth, D. A., Aina, T., Christensen, C., Collins, M., Faull, N., Frame, D. J., et al. (2005). Uncertainty in predictions of the climate response to rising levels of greenhouse gases. Nature 433, 403–406. doi: 10.1038/nature03301
Tarsitano, D., Young, S. D., and Crout, N. M. J. (2011). Evaluating and reducing a model of radiocaesium soil-plant uptake. J. Environ. Radioact. 102, 262–269. doi: 10.1016/j.jenvrad.2010.11.017
Taylor, I. H., Burke, E., McColl, L., Falloon, P. D., Harris, G. R., and McNeall, D. (2013). The impact of climate mitigation on projections of future drought. Hydrol. Earth Syst. Sci. 17, 2339–2358. doi: 10.5194/hess-17-2339-2013
Taylor, K. E., Stouffer, R. J., and Meehl, G. A. (2012). An overview of CMIP5 and the experiment design. Bull. Am. Meteor. Soc. 93, 485–498. doi: 10.1175/BAMS-D-11-00094.1
Tebaldi, C., Arblaster, J. M., and Knutti, R. (2011). Mapping model agreement on future climate projections. Geophys. Res. Lett. 38, L23701. doi: 10.1029/2011GL049863
Todd-Brown, K. E. O., Randerson, J. T., Post, W. M., Hoffman, F. M., Tarnocai, C., Schuur, E. A. G., et al. (2013). Causes of variation in soil carbon simulations from CMIP5 Earth system models and comparison with observations. Biogeosciences 10, 1717–1736. doi: 10.5194/bg-10-1717-2013
Vermeulen, S. J., Challinor, A. J., Thornton, P. K., Campbell, B. M., Eriyagam, N., Vervoort, J. M., et al. (2013). Addressing uncertainty in adaptation planning for agriculture. Proc. Natl. Acad. Sci. U.S.A. 110, 8357–8362. doi: 10.1073/pnas.1219441110
Vrugt, J. A., Ter. Braak, C. J. F., Clark, M. P., Hyman, J. M., and Robinson, B. A. (2008). Treatment of input uncertainty in hydrologic modelling: doing hydrology backward with Markov chain Monte Carlo simulation.Water Resour. Res. 44, W00B09. doi: 10.1029/2007WR006720
Warren, R., de la Nava Santos, S., Bane, M., Barker, T., Barton, C., Ford, R., et al. (2008). Development of the Community Integrated Assessment System (CIAS), a multi-institutional modular integrated assessment approach for modelling climate change, and of SoftIAM, its supporting software. Environ. Model. Softw. 23, 592–610. doi: 10.1016/j.envsoft.2007.09.002
Warszawski, L., Frieler, K., Huber, V., Piontek, F., Serdeczny, O., and Schewe, J. (2013). The Inter-Sectoral Impact Model Intercomparison Project (ISI–MIP): project framework. Proc. Natl. Acad. Sci.U.S.A. 111, 3228–3232. doi: 10.1073/pnas.1312330110
Watson, J., and Challinor, A. (2013), The relative importance of rainfall, temperature and yield data for a regional-scale crop model. Agric. For. Meteorol.170, 47–57. doi: 10.1016/j.agrformet.2012.08.001
Wheeler, T. R., Craufurd, P. Q., Ellis, R. H., Porter, J. R., and Vara Prasad, P. V. (2000). Temperature variability and the yield of annual crops. Agric. Ecosys. Environ. 82, 159–167. doi: 10.1016/S0167-8809(00)00224-3
Keywords: climate change, climate impacts, ensembles, uncertainty, modeling
Citation: Falloon P, Challinor A, Dessai S, Hoang L, Johnson J and Koehler A-K (2014) Ensembles and uncertainty in climate change impacts. Front. Environ. Sci. 2:33. doi: 10.3389/fenvs.2014.00033
Received: 11 June 2014; Accepted: 14 July 2014;
Published online: 31 July 2014.
Edited by:
Veerasamy Sejian, Indian Council of Agricultural Research, IndiaReviewed by:
Saumitra Mukherjee, Jawaharlal Nehru University, IndiaKazuya Nishina, National Institute for Environmental Studies, Japan
Mari Rachel Tye, National Center for Atmospheric Research, USA
Copyright © 2014 Falloon, Challinor, Dessai, Hoang, Johnson and Koehler. This is an open-access article distributed under the terms of the Creative Commons Attribution License (CC BY). The use, distribution or reproduction in other forums is permitted, provided the original author(s) or licensor are credited and that the original publication in this journal is cited, in accordance with accepted academic practice. No use, distribution or reproduction is permitted which does not comply with these terms.
*Correspondence: Pete Falloon, Earth System and Mitigation Science, Climate Impacts Modelling, Met Office Hadley Centre, Fitzroy Road, Exeter, EX1 3PB, UK e-mail:cGV0ZS5mYWxsb29uQG1ldG9mZmljZS5nb3YudWs=