- 1Department of Molecular Biology and Biochemistry, Simon Fraser University, Burnaby, BC, Canada
- 2Department of Biological Sciences, Simon Fraser University, Burnaby, BC, Canada
- 3Palmer Environmental Consulting Group, Vancouver, BC, Canada
Due to the environmental persistence, bioaccumulation, and toxicity of metals released by mining activities, mitigation methods are crucial to minimize impacts on aquatic environments. Bioremediation is one mitigation strategy used to reduce the potential for metal accumulation and toxicity in aquatic organisms. At a potential mine site in Yukon, Canada, elevated copper (Cu) concentrations and low pH are found in a water course near a naturally mineralized area; however, Cu concentrations and acidity are greatly reduced downstream. Physicochemical processes do not appear to explain this natural remediation and it is suggested that unique microbial communities may be responsible through Cu immobilization. To investigate the role of microbes in sequestering or transforming Cu in the water, biofilm samples were collected from five sites along a natural copper gradient: upstream of Cu introduction, on a Cu-rich tributary, 30 m downstream of Cu introduction, where Cu levels were reduced, and 2 and 7 km further downstream, where Cu concentrations were low. Taxonomic profiles of microbial communities (microbiomes) were compiled using DNA sequencing of 16S rRNA gene amplicons. Clear relationships between total Cu concentrations, pH and the microbiomes were evident. In the most Cu-affected samples, communities were dominated by bacteria from the Gallionellaceae family. Metagenomic sequencing profiled the genes present in microbiomes from the most Cu-contaminated sampling location and the area immediately upstream and showed that microbes in this area are well adapted to tolerate heavy metals. This study provides fundamental knowledge of microbial communities at a potential mine site and characterizes the genes likely involved in providing tolerance to an acidic and metals-rich environment. These results inform hypotheses for future experiments to support the development of bioremediation approaches that incorporate the use of native microorganisms at mining sites.
Introduction
Mining is an important natural resource extraction method that typically requires mitigation strategies to reduce or eliminate resulting adverse environmental impacts. If impacts to aquatic environments are predicted during the design and assessment phases of mine development, water treatment is often considered. However, current water treatment strategies and the management of resulting sludges can be extremely costly and time consuming (Perales-Vela et al., 2006). Bioremediation, an alternative to conventional physicochemical treatment processes, uses biological organisms to metabolize, alter, or capture contaminants of concern in an engineered project or at a contaminated site. Bioremediation examples include: toluene-degrading bacteria in a Massachusetts, United States of America (USA) watershed (Tay et al., 2001); mercury-accumulating periphyton in Boreal Canadian Shield Lakes (Desrosiers et al., 2011); and naphthenic acid-removing bacteria in oil sands processing water, Alberta, Canada (Islam et al., 2015). Bioremediation may be the preferred option for reducing contaminant concentrations in circumstances where access is difficult, habitat will be destroyed, species at risk will be disrupted, or the area is simply too large to be feasibly physically remediated. Hence, bioremediation, along with geochemical processes, can contribute to the attenuation of environmental impacts and may be an innovative option to solve some contamination issues.
In Northern Canada (Yukon Territory), a copper-gold-silver-molybdenum mine has been proposed near Casino Creek by the Casino Mining Corporation (CMC)1. Baseline environmental assessments of the aquatic environment have shown that the background concentrations of metals in some areas are elevated above Canadian Council of Ministers of the Environment (CCME) water quality guideline values for the protection of aquatic life2, which are concentrations used by the Yukon Government to protect the environment. More specifically, preliminary studies found that copper (Cu) concentrations were naturally elevated in some parts of Casino Creek but reduced immediately downstream. Physical and chemical evaluations (e.g., hydrogeological modeling of dilution and calculations of solubility limits for common minerals [e.g., tenorite (CuO)]) offered no indication as to the cause of reductions in Cu concentrations, suggesting that this may be a case of natural Cu bioremediation.
Metals bioremediation is performed by many types of organisms, including bacteria, fungi, and algae (Tay et al., 1998; Desrosiers et al., 2011; Arini et al., 2012). Across a wide range of environments, including aquatic ecosystems, these organisms naturally organize into biofilm communities (Malik, 2004) in which cells adhere to each other on a surface and produce a matrix of extracellular polymeric substance (EPS). Some biofilms can withstand high concentrations of metals (Orell et al., 2010 and references therein) and acidic conditions (Baker and Banfield, 2003; Arini et al., 2012), which make them a useful tool in developing new biotechnologies for mining-related areas of research. The science of strategically using biofilms is continuously developing (ITRC, 2008), including their use for bioremediation purposes.
Biofilms are complex and difficult to cultivate artificially, and new tools to study microbiomes in situ have enabled the profiling of biofilm community structures based on their DNA sequences (Besemer et al., 2012), greatly enhancing the knowledge base regarding their natural compositions. The objective of this study was to use new approaches to characterize native microorganisms from a proposed mine site and investigate their potential involvement in Cu bioremediation. This study used microbiome profiling and metagenomic techniques to investigate biofilms collected from an artificial substrate placed in situ at five sites in the Casino Creek watershed to describe how the microbiome varies concurrently with Cu concentrations and acidic conditions. Microbial taxonomic profiles were compiled using amplicon sequencing of the 16S rRNA genes present in extracted DNA and community profiles were compared between sites. Genes from select samples were profiled using shotgun methods for metagenomic DNA sequencing to characterize the entire microbial community and identify metal-associated genes that could possibly explain elevated Cu-tolerance and the hypothesized ability to reduce Cu concentrations in the water column. The results of this study may be useful to inform future development of bioremediation strategies using native microbes to mitigate potential increases in environmental metals concentrations from mining operations. This is one of the first studies to investigate the microbial community structure and gene content of microorganisms from a naturally mineralized area prior to the initiation of mining operations and therefore represents an example of microbial investigations that can support natural resource extraction processes.
Materials and Methods
Sampling Locations
Five locations for biofilm sampling were selected in the Casino Mine watershed, Yukon, Canada (Figure 1; Table 1). Site A is located furthest upstream on Casino Creek, just prior to the introduction of Cu to the system (via the Proctor Gulch tributary). Site B is on the copper-rich tributary in Proctor Gulch, immediately upstream of the confluence with Casino Creek, and has the highest Cu concentrations with visible dark orange staining on rocks. Site C is on the Casino Creek mainstem, 30 m downstream of Site A, and Cu concentrations were slightly elevated and some orange staining on rocks was visible. Site D is ~2 km downstream of Site C and is located in the proposed mine tailings pond area. Site E is furthest downstream, ~5 km south of Site D, and sits at the toe of the proposed tailings pond.
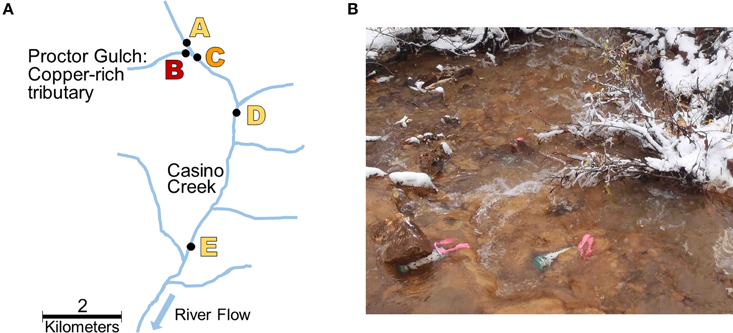
Figure 1. Sampling site locations. (A) Map of biofilm sampling locations (Sites A–E) along Casino Creek, Yukon, Canada. (B) Picture of triplicate biofilm samplers deployed at Site B.
Casino Creek Biofilm Sample Collection
Biofilm samplers were deployed in triplicate at each sampling location on the same day. Samplers consisted of PVC pipes, 20 cm in length and 5 cm in diameter (approximate volume 400 ml), containing 5 mm glass beads inside a nylon bag as the substrate for biofilm colonization (Besemer et al., 2012). Plastic netting was placed over the ends of the PVC pipes to exclude large debris while allowing for water flow through the sampler. Samplers were tethered and submerged at depths of 0.3–0.4 m. Median water hardness measured during baseline environmental assessments in Casino Creek was 111 mg/L CaCO3.
The biofilm samplers were left in situ for 18 d during late summer/early fall. On the final day, water quality parameters (dissolved oxygen, pH, conductivity) and substrate information were noted (Table 1) and samplers were retrieved and placed in sterile plastic bags with site water and kept cool on ice until processed. To harvest the biofilms (colonized organisms), glass beads were removed from the nylon bag into a glass beaker containing 0.5 volumes of corresponding site water and sonicated ~10 min. To collect and concentrate the biofilm organisms, the water was filtered through a Super200 0.22 μm filter (Pall Corporation, VWR, Mississauga, ON, Canada). This process was repeated using the remaining 0.5 volume of corresponding site water. Filters containing the organisms were stored in 15 ml tubes and kept frozen during shipping (via air from Whitehorse, Yukon to Burnaby, BC) until transfer to −80°C.
Whole, dry filters were cut using sterilized scissors into 10–15 mm wide strips. Filter segments were placed into a Powerlyzer® glass bead tube containing 0.75 ml bead solution and DNA was extracted using the Powerlyzer® PowerSoil® DNA isolation kit (Mo Bio Laboratories Inc., Carlsbad, CA, USA) following the manufacturer's instructions. The elution step was performed twice for a total final volume of 0.12 ml of DNA. The DNA samples were stored at −20°C. DNA concentrations were evaluated using a NanoDrop 2000 spectrophotometer (Thermo Scientific, Wilmington, DE, USA). A sample was considered acceptable if it had a DNA concentration >5 ng/μl.
Amplicon Library Preparation and Sequencing
DNA concentrations were adjusted to 5 ng/μl. Each Polymerase Chain Reaction (PCR) contained: 5 ng of biofilm DNA, 13 μL of molecular biology grade water, 10 μL of 5 Prime Hot Master Mix (5 Prime, Gaithersburg, MD, USA), and 0.5 μL of each forward and reverse primer (10 μM final concentration) as described in Caporaso et al. (2011). PCR was performed in triplicate on a TGradient Thermocycler (Biometra, Horsham, PA, USA) with the following program: 94°C for 3 min; 35 cycles of 94°C for 45 s, 50°C for 60 s, and 72°C for 90 s; and finally an extension at 72°C for 10 min. Primers that amplify the V4, V5 region of the 16S rRNA gene were obtained from Klindworth et al. (2012), forward S-D-Bact-0564-a-S-15: 5′-AYT GGG YDT AAA GNG-3′ (520F) and reverse S-D-Bact-0785-b-A-18: 5′-TAC NVG GGT ATC TAA TCC-3′ (802R). A 2% agarose gel was run on each PCR sample to confirm amplification occurred. PCR cleanup of the 16S amplified samples was performed as described in the 16S Metagenomic Sequencing Library Preparation instructions (Illumina, San Diego, CA, USA).
Indices were incorporated into the 16S amplified samples as per the Illumina 16S Metagenomic Sequencing Library Preparation instructions. Each index reaction used 10 μL of DNA, 5 μL of Index primer 1 (N701–N705), 5 μL of index primer 2 (S502–S504 or S517), 25 μL of 5 Prime Hot Master Mix, and 10 μL of molecular biology grade water. PCR was performed to anneal indices to the 16S rRNA amplicon with the following conditions: 95°C for 3 min; eight cycles of 95°C for 30 s, 55°C for 30 s, and 72°C for 30 s; a final extension at 72°C for 5 min. Several control samples were included at this stage: (1) a MilliQ water-only negative control, (2) a MilliQ water and DNase negative control, (3) a filter-only negative control, and (4) a spiked positive control. Negative controls showed undetectable (<0.4 ng/μL) amounts of DNA prior to amplification. The spiked positive control contained an in-house laboratory mixture of DNA extracted from cultures of: Bacillus amyloliquefaciens (FZB4Z), Escherichia coli (K12), Pseudomonas aeruginosa (PAO1), Pseudomonas putida (KTZ440), and Rhodobacter capsulatus (SB1003).
A second PCR cleanup of the indexed 16S amplified samples occurred as described in the Illumina 16S Metagenomic Sequencing Library Preparation instructions. Prior to input on the Illumina MiSeq cartridge, DNA concentrations were assessed using a Qubit® 2.0 Fluorometer (Life Technologies, Grand Island, NY, USA), and DNA quality and size (to confirm 390 bp products) were assessed using a DNA 1000 chip on a Bioanalyzer 2100 instrument (Agilent Technologies, Santa Clara, CA, USA). DNA libraries were normalized to 4 nM with 10 mM Tris, pH 8.5. All samples and controls were pooled and run on the Illumina MiSeq Sequencer, following the Illumina 16S Metagenomic Sequencing Library Preparation instructions. All raw sequences are deposited in the NCBI Sequence Read Archive under BioProject ID: PRJNA297682.
Metagenomic Library Preparation and Sequencing
Two samples were selected for further analysis using shotgun sequencing: one from Site B, where the Cu concentration is highest, and one from Site A, upstream of Cu introduction (Table 1). The same in-house produced positive control as described above was also processed with these samples.
Prior to input on the Illumina MiSeq cartridge, DNA concentrations were assessed using a Qubit® 2.0 Fluorometer (Life Technologies) to check for appropriate concentration (0.2 ng/μL) and cluster density for the Illumina instrument. Tagmentation was performed according to the Nextera XT DNA Sample Preparation Kit (Illumina, San Diego, CA, USA) instructions. The amount of DNA for each sample varied slightly but was at least 1 ng. Indices were incorporated into the samples for metagenomic sequencing as per the Illumina Nextera XT DNA Sample Preparation Kit instructions. Each index reaction contained 25 μL DNA, 5 μL of Index primer 1 (N701 or N702), 5 μL of index primer 2 (S502 or S504), and 15 μL of NPM master mix. To anneal index primers to tagmented DNA, PCR was performed as follows: 72°C for 3 min; 95°C for 30 s; 12 cycles of 95°C for 10 s, 55°C for 30 s, and 72°C for 30 s; a final extension at 72°C for 5 min.
PCR cleanup was performed for the metagenomic libraries following the procedure from the Illumina Nextera XT DNA Sample Preparation Guide. For the metagenomic libraries, DNA quality and size (to verify the appropriate range of 100–1000 bp) were assessed using a DNA 1000 chip on a Bioanalyzer 2100 instrument (Agilent Technologies). DNA concentrations were assessed using a Qubit® 2.0 Fluoromoter (Life Technologies). Each library was diluted to 4 nM with 10 mM Tris, pH 8.5, and pooled with 0.1X volume of positive control before being run on the Illumina MiSeq Sequencer, following Illumina Nextera XT DNA Sample Preparation Guide. All raw sequences are deposited in the NCBI Sequence Read Archive under BioProject ID: PRJNA297682.
Amplicon Data Analysis
Amplicon sequence reads were preprocessed according to best practices (Schirmer et al., 2015). Low confidence bases were trimmed by Phred score using Trimmomatic (Bolger et al., 2014), with a sliding window of length 3 and a minimum Phred score of 20. Error correction was performed using BayesHammer (Nikolenko et al., 2013), followed by merging of overlapping paired reads using PEAR (Zhang et al., 2014), both with default settings. Reads containing ambiguous bases and reads that were >5% longer or shorter than expected were discarded. All samples were rarefied to 100,000 reads before using QIIME v 1.9 (Caporaso et al., 2010a) to perform open-reference OTU picking. Reads were clustered using uclust (Edgar, 2010) at 97% identity against the Greengenes v13_8 16S rRNA database (DeSantis et al., 2006). Reads that did not match to a reference sequence were clustered de novo at 97% identity. Where possible, OTUs were taxonomically annotated using uclust (Edgar, 2010) against Greengenes reference sequences (DeSantis et al., 2006). OTUs with <3 reads were removed to avoid noise. A PyNAST (Caporaso et al., 2010b) alignment of OTUs was used to build a phylogenetic tree using FastTree (Price et al., 2009). OTU diversity and abundances were analyzed in R 3.0 using PhyloSeq (McMurdie and Holmes, 2013) and Vegan (Oksanen et al., 2002).
In the positive control, 40,095 reads were assigned to 1087 OTUs, with 99.7% of reads assigned to OTUs from the four expected families. The most abundant OTU assigned to the incorrect family contained 0.015% of the sample's reads and was assigned to the most abundant family from the experimental samples (Gallionellaceae). This indicates that OTUs with extremely low abundance may be due to a small amount of sample cross-contamination. In the experimental samples, 299,356 reads were assigned to 6767 OTUs. After OTUs with abundances lower than 0.015% were discarded, 251,783 reads were assigned to 1579 OTUs. To compare across samples, all samples were sub-sampled to the lowest per-sample read count (2432 reads), such that 36,480 reads were assigned to 1128 OTUs across all samples.
Alpha(α)-diversity was measured using Shannon-Weiner index and β-diversity was measured using weighted Unifrac distances. Correlations between OTU abundances and Cu concentration were measured by first discarding low-variance OTUs (variance in proportional abundance across samples <1E-7). The remaining OTUs were tested for correlation using Pearson correlation and p-values were adjusted for false discovery rate using Benjamini-Hochberg method. Statistical significance was determined when q-values were <0.05.
Metagenomic Data Analysis
Metagenome reads were trimmed to remove low confidence bases using Trimmomatic (Bolger et al., 2014), with a sliding window of length 5 and a minimum Phred score of 20. Sequencing adapters were removed using cutadapt (Martin, 2011), overlapping paired-end reads were merged using PEAR (Zhang et al., 2014), and reads shorter than 100 bp were discarded. After this processing, 3 and 10 million reads remained in samples from Sites A and B, respectively. Reads were then compared against BacMet, a database of metal-associated genes using BlastX with a 60% identity threshold (Pal et al., 2014). Assembly of reads from Site B was performed using SPAdes with default parameters (Bankevich et al., 2012). Experimental samples were subsampled down to 1.8 million reads to be compared against nr (downloaded January 22, 2015) using RAPSearch2 (Zhao et al., 2012). Protein alignments with an e < 0.01 and length greater than 30 amino acids were analyzed using MEGAN version 5.10 (Huson et al., 2011) to determine the taxa and gene families present. MEGAN was run using default parameters to assign reads using the March 2015 taxonomic reference file and the SEED database (Overbeek et al., 2005) mapping file (most recent version: January 2014).
Results
Cu-Rich Samples Were Dominated by Gallionellaceae
Total Cu concentrations at sampling sites ranged from 0.03 to 1.02 mg/L (Table 1) and were highly correlated with specific conductivity and pH (Pearson's Rho: 1.0 and 0.91, respectively), and not correlated with temperature (data not shown) or percent saturation of dissolved oxygen (Table 2). Taxonomic compositions of sampled microbiomes (the community of microorganisms) were predicted from DNA sequences of the 16S rRNA gene. Overall, Proteobacteria was the most abundant phylum across samples, with the remainder of the communities being composed of Bacteroidetes in Sites D and E and a combination of phyla in Site A [Figure 2; class and order data are presented in Figures S1A,B]. Sites B and C were dominated by Proteobacteria, except for a small amount of Cyanobacteria in Site B, which was classified down to the order level as Stramenopiles. The dominant taxonomic family (87–93%) present in the Cu-exposed microbiomes at Sites B and C was Gallionellaceae (Figure 2). Across all other samples, 0.2–23% of reads were assigned to Gallionellaceae. Site A was the least characterized at the family level, with the majority of the community belonging to an unknown family, while the most abundant family at Sites D and E was Comamonadaceae (Figure 2).
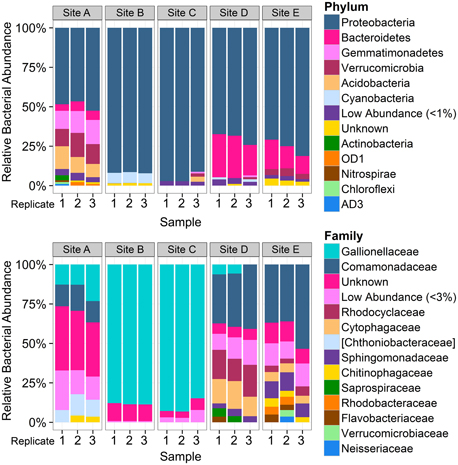
Figure 2. Overview of taxonomic composition of each sample by phylum and family. Bar plots represent the proportion of reads assigned to the taxonomic composition of each sample by phylum (top) and by family (bottom), grouped by sampling site (n = 3 per site). Colors do not correspond between figures. Low abundance taxa (<1% at the phylum level and 3% at the family level) were collapsed to improve readability. Square brackets indicate proposed taxonomy based on Greengenes phylogenetic analysis. Phylum level plot shows that Site A was distinct from other low-copper sites and that cyanobacteria were present in Site B. Family level analysis shows dominance of Gallionellaceae at Sites B and C. The higher number of OTUs that could not be assigned to a family at Site A indicates that those bacteria were more distantly related to known bacteria than those present in the other sites.
Of all the reads assigned to the 593 Gallionellaceae operational taxonomic units (OTUs), 99.99% were assigned to 591 OTUs in the Gallionella genus. While the high number of Gallionella OTUs indicates that there was some species diversity present in these samples, most of these were present in very low abundance and could also have been due to noise from sequencing errors. One of the Gallionella OTUs was by far the most abundant, comprising 81% of all Gallionella-assigned reads: OTU 830064 (Figure 3). This OTU was most abundant in Cu-rich Sites B and C, where it accounted for 76–83% of reads, but it was also seen at low levels in all other samples (0.2–4%). In contrast, the reads assigned to Gallionella in Site A were mostly from a different OTU: OTU 4342654, which was the second most abundant Gallionella OTU overall. This OTU was also present across all other sampling sites but in very low abundance (0.02–0.1%).
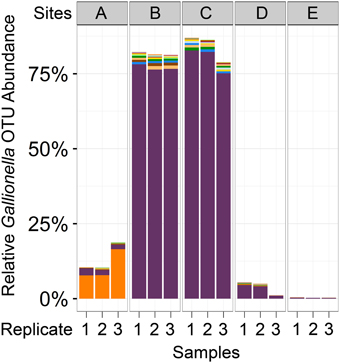
Figure 3. Abundance of Gallionella OTUs across samples. Bar plots represent the number of reads assigned to all OTUs classified as Gallionella. Each color represents a different OTU. The two most abundant Gallionella OTUs (purple and orange) represented 87% of all reads assigned to any Gallionella OTU. Purple, OTU 830064; orange, OTU 4342654 (Greengenes IDs).
To identify OTUs with abundances correlated with Cu concentration, OTUs with low variance in abundance across Sites A–E were first discarded, leaving 227 OTUs to be tested. Six of these OTUs were significantly correlated with Cu concentration and had at least 1% abundance in any sample: four belong to the Gallionella genus, one was unclassifiable, although similar to a sequence found in an arctic stream (Larouche et al., 2012), and one was from the order Stramenopiles (Table 3).
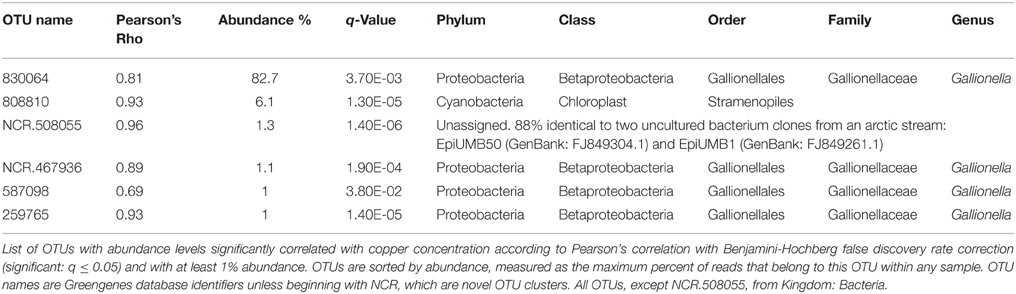
Table 3. OTUs positively correlated with copper concentration are from Gallionellaceae and Stramenopiles.
While 16S data indicates that one OTU belonging to the genus Gallionella dominates Site B, metagenomic sequencing results from Site B indicate that the DNA present is most closely related to sequences from multiple genera in the Gallionellaceae family (Figure 4). Reads assigned in this family at the species level are split between three genera: Gallionella, with 62.5% of reads, Sideroxydans, with 34% of reads, and Ferriphaselus, with 3.5% of reads. De novo assembly of the metagenome from Site B resulted in an N50 of 835. Although this is low, many long contigs were constructed: 4 longer than 100 kbp and 558 longer than 10 kbp. Further sequencing that targets genome completion may be required to improve this assembly.
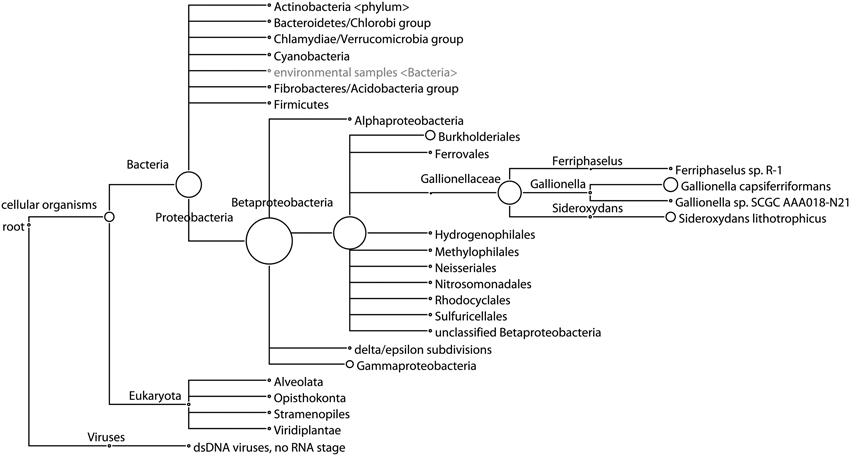
Figure 4. Phylogenetic tree of taxa at Site B predicted from shotgun sequencing analysis. Circle size represents the number of reads assigned to each taxon, showing predominance of sequences classified as multiple genera in the Gallionellaceae family. Taxa only shown when at least 0.1% of reads are assigned.
Bacterial Communities Recover in Phylogenetic Diversity But not Composition After Cu is Depleted
Within-sample bacterial diversity was measured on OTU counts using the Shannon–Wiener index, which takes into account richness and abundance. Biofilm samples from Sites B and C (high total Cu concentrations: 1.02 and 0.37 mg/L, respectively), had the lowest diversity, while biofilm samples from Sites A, D, and E (low total Cu concentrations: 0.12, 0.03, and 0.07 mg/L, respectively), had higher diversity (Figure 5A). Between-sample bacterial diversity was calculated using weighted-UniFrac distances to measure diversity between samples. This method is phylogenetically sensitive, such that it measures the distance between samples based both on the abundance and relatedness of shared and unshared OTUs. The pair-wise distance matrix is represented in Figure 5B using principle coordinates analysis (PCoA). Samples clustered closer together had more similar microbiomes. Samples from the Cu-affected Sites B and C clustered together, as did samples from the two downstream Sites D and E where Cu had been depleted, while the samples from the site before Cu was introduced (Site A) were distinct from both groups (Sites B and C, or D and E). This suggested that while diversity levels recovered after Cu concentrations returned to baseline values, the composition of the community did not return to a pre-Cu exposure state. However, other factors that were not evaluated in detail in this study (e.g., stream topography or substrate type) may account for the microbiome differences between sampling locations A and D or E.
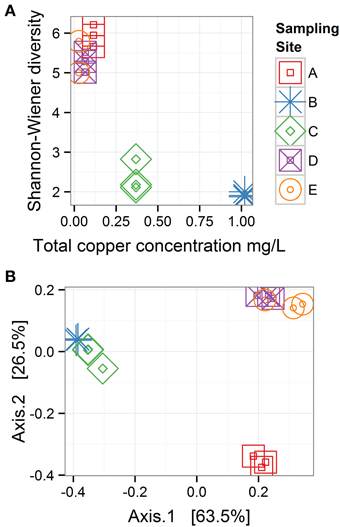
Figure 5. Bacterial diversity across sampling sites. (A) Within-sample diversity (α-diversity) is measured with the Shannon-Wiener index. (B) Between-sample diversity (β-diversity) is measured with weighted UniFrac distances and represented with a principle coordinates analysis plot, in which 63.5 and 26.5% of the variability among samples is projected on Axis 1 and 2, respectively; n = 3 for all sampling sites. These plots show when copper concentrations were high (Sites B and C), α diversity was low, and bacterial communities recover in α-diversity after copper concentrations decline, but community composition does not return to the pre-copper state.
Eukaryotic Community Also Differs Between Sites with Low and High Cu Concentrations
The taxonomic analysis of metagenomic sequences showed that bacteria were the main component of the biofilms examined and that Sites A and B differed in their eukaryotic and archaeal compositions (Figure 4; list of taxa abundances in Table S1). In Site A, 52% of reads were classified taxonomically. Reads may not have been classified due to low sequence complexity or high evolutionary distance from reference genomes. Of those reads that were classified, 99% were classified as bacterial, 1% as eukaryotic, and 0.5% as archaeal. The eukaryotes were mostly Opisthokonta (0.6%), including Metazoa (0.3%) and Fungi (0.2%). The archaea were mostly Euryarchaeota (0.2%), with few reads classified down to the class level; the most abundant classes were Methanomicrobia (0.08%) and Halobacteria (0.04%). Viruses were detected at very low abundance (0.07%), as expected due to the pore-size of the filters used. In Site B, 61% of reads were classified taxonomically, of which 96% were bacterial, 4% were eukaryotic, and very few were archaeal (0.06%). The most abundant eukaryotic taxa identified in Site B were Opisthokonta (1.7%), including Fungi (1%) and Metazoa (0.5%), and two types of algae: Stramenopiles (0.5%) and Viridiplantae (0.4%). Viruses were again detected at low abundance (0.2%), half of which were Phycodnaviridae, which infect aquatic eukaryotic algae. These results show that Sites A and B differed beyond their bacterial communities, with archaea and Metazoa more abundant in Site A, while Fungi, algae, and algal viruses were more abundant in Site B.
Metal-Associated Genes and Pathway-Level Differences Occur Between Sites with High and Low Cu Concentrations
More reads from the Cu-rich Site B than from Site A were assigned to metal-associated genes, with 0.7 and 0.09% assigned, respectively, (Table S3). Of the 22 metal-associated genes with at least 10 reads per million assigned in either sample, all were more abundant in Site B compared to Site A, with nine having at least a 20-fold higher abundance (Table 4). Of the remaining 13 lower abundance genes, 12 had at least a 2-fold higher abundance in Site B compared to Site A (Table S3).
More reads from Site B were assigned to a SEED subsystem functional group than from Site A, with 27 and 18% of reads assigned, respectively (Table S2). This was likely due to fewer closely-related reference genomes being available for the upstream site. Table 5 lists the 8 SEED subgroups that were abundant, with at least 0.1% reads assigned, and differential, with at least a four-fold difference in abundance between Sites A and B. Most of these subgroups were more abundant in Site B than Site A, with a larger ratio than could be accounted for by the difference in number of reads classified (1.5-fold difference).
Discussion
Microbial communities are being intensively studied for their environmental applications, including bioremediation to reduce undesirable contaminant concentrations that result from anthropogenic activities. During the environmental assessment of a proposed metals mine in Northern Canada, an unusual feature of the area was discovered: aqueous Cu concentrations that were high in one reach of a creek were quickly reduced downstream with data suggesting that the causative factor was biological. In this study, microbiome samples were collected from several reaches of the creek, including those high in metal concentrations and low in pH, for metagenomic analysis in order to characterize their taxonomic and genetic compositions. Microbial profiles at sampling locations with high Cu concentrations were highly similar and dominated by the Gallionellaceae bacterial family, suggesting that one or more species of Gallionellaceae may be involved in the observed reduction in aqueous Cu concentrations. The findings of this study provides further evidence that Gallionella-like species can thrive in environments that are acidic and high in metal concentrations, as previously described by Fabisch et al.(2013). This study contributes to the characterization of native microorganisms that can potentially be cultivated for in situ bioremediation.
Metagenomic sequencing of DNA from biofilm samples from Sites A and B revealed that these communities were dominated by bacteria, with >99 and >96% of taxonomically classified reads assigned to bacteria, respectively; thus, eukaryotic and archaeal proportions were minimal (Table S1). Fungi are known to sequester or uptake metals by biosorption but since fungi were proportionally quite low in the assigned reads from the Site A and Site B biofilms (0.2 and 1%, respectively), their contribution to Cu sequestration/transformation was assumed to be low. The same assumption may be true for archaea and algae found at Site B (0.06 and 0.9%, respectively). Thus, due to the dominance of bacteria in the biofilms obtained, these results suggest that the bacteria present at Site B and/or Site C (which was not evaluated for its proportions of eukaryotes, archaea, and bacteria) are the most likely candidates for the removal of aqueous Cu from the water column, if bioremediation was occurring. A low proportion of eukaryotes in an acidic, alkaline, and/or metals-rich environment does not appear to be unusual, although studies of these environments often focus on microbial community structures and thus the eukaryotic proportion is not evaluated or reported (Bier et al., 2014; Tsitko et al., 2014; Liljeqvist et al., 2015). It is possible that the biofilm collection period of 18 days may have affected the final composition of the communities through succession limitations (i.e., limited colonization of eukaryotes). Additionally, the materials and structure of the biofilm sampling devices may also have had an effect on colonization. However, similar apparatuses have been used successfully elsewhere to study biofilm development in freshwater lotic environments (Besemer et al., 2012), with similar timing (21 day collection) and materials. Further investigation of the riverbed biofilms at Sites B and C will be needed to confirm the bacterial dominance and microbial contributions to Cu sequestration/transformation.
The bacterial communities present at Cu-affected Sites B and C were dominated by one OTU belonging to the genus Gallionella (Figure 3). However, the metagenomic sequencing results from Site B showed reads assigned to Gallionella capsiferriformans ES-2, Sideroxydans lithotrophicus ES-1, and Ferriphaselus sp. R-1 (Figure 4). G. capsiferriformans ES-2 and S. lithotrophicus ES-1 are the only complete genomes in the Gallionellaceae family and were recently sequenced (Emerson et al., 2013). The 16S genes for these two species are quite different (93% sequence identity, which is well below the 97% OTU threshold used in this study) but the genomes are fairly similar, with 40% of genes homologous at 60% identity (Emerson et al., 2013). This supports assigning the bacteria at Sites B and C as belonging to the Gallionellaceae family, however, the Gallionella-specific label may be incorrect. The contradiction between the 16S rRNA results and the metagenomic sequencing results was informative, suggesting that either the dominant OTU observed from Site B does belong to Gallionella and that G. capsiferriformans ES-2 has undergone considerable gene-loss since Gallionella diverged from the other Gallionellaceae genera, or that the 16S rRNA-driven genus classification is unreliable in this clade, in which case this OTU may represent a new genus in Gallionellaceae that is related to both G. capsiferriformans ES-2 and S. lithotrophicus ES-1. Future studies could focus on identifying and isolating this member of Gallionellaceae, as well as the distinctly different member of the Gallionellaceae family found upstream at Site A (Figure 3). Comparisons of the genomes of these two Gallionellaceae populations may provide insights into microbial adaptations for extreme environments. Furthermore, isolating and culturing the Gallionellaceae from Site B would allow for exploration of bioremediative potential, which could result in the development of a new product for remediation activities.
From the data collected in this study, hypotheses can be drawn about potential bioremediation mechanisms that may be occurring. In general, bacteria can manage metals through active entrapment (biosorption or sequestration) and/or metabolic transformation into precipitates (Malik, 2004; Andreazza et al., 2010; Ghosh and Saha, 2013), some of which bind to microbial surfaces via adsorption (Gadd, 2010). Biosorption and metal precipitation can even co-occur, making it difficult to determine the contribution of each process to metal immobilization (Glasauer et al., 2001). Microbial transformational processes that alter the speciation of metals (e.g., from Fe2+ to Fe3+) may achieve detoxification, enabling survival (Perales-Vela et al., 2006; Orell et al., 2010). Such transformations can result in the production of insoluble products, such as the orange-colored iron (III) oxide precipitate. When iron oxide production appears to be the dominant detoxification process occurring, studies have shown that heavy metals can be co-precipitated by bacteria, including Gallionellaceae (Cu, cadmium, nickel, and zinc: Fabisch et al., 2013; manganese: Akob et al., 2014). The presence of Gallionellaceae and orange staining at Sites B and C indicate the possibility that copper could be co-precipitating with iron oxides. While sediments and precipitates (i.e., crystalline structures of minerals) were not examined for the presence of other metal oxides in this study, this could be performed in the future. In addition, riverbed biofilm samples could be evaluated in future studies to determine if Cu co-locates with biofilm mass.
The gene content of the biofilms collected from Site B were examined to look for insights into possible mechanisms that would explain the lowered aqueous Cu concentration downstream. One of the most well-known natural precipitation mechanisms for dissolved Cu is performed by sulfate-reducing bacteria, which precipitate metals as highly insoluble sulfides. For example, sulfate-reducing bacterial biofilms have been shown to accumulate Cu on their surfaces as metal-sulfides (White and Gadd, 2000). The most abundant family present at Sites B and C, Gallionellaceae, has not been reported to reduce sulfate. Of the typical, non-thermophilic sulfate-reducing bacterial groups, such as the three families and eight genera within Deltaproteobacteria and three genera within Clostridia (Muyzer and Stams, 2008), none were present with at least 0.01% abundance at the site with the highest Cu concentrations (Site B). Downstream at Site C, where Cu concentrations were still elevated, Syntrophobacteraceae was the most abundant sulfate-reducing bacterial clade with at most 0.3% abundance (note, such a low abundance can be difficult to distinguish from sequencing noise). Sulfate-reduction might be occurring in this environment; however, genetic evidence to indicate that this mechanism functions at this site was not found in this study. Another major mechanism by which bacteria can immobilize metals is through intracellular binding with polyphosphate bodies, a process that has been observed in cyanobacteria (Malik, 2004). Although this has not been observed in Gallionellaceae, a high abundance of two genes from the pstSACB operon (pstA and pstS), an ATP binding cassette (ABC) phosphate transporter, were found in the metals-rich Site B (Table 2). However, this operon has also been associated with arsenate transport (Li et al., 2013) and so these bacteria could be equipped with these genes either to import phosphate, tolerate arsenate, or for some other unknown reason. Further study including measurements of the metals concentrations collocated with biofilms and possibly a characterization of the planktonic community will help to elucidate the processes occurring. Additional sequencing of the microbial community transcriptome (metatranscriptomics) under controlled conditions could also give insight into the specific immobilization or transformational mechanisms involved.
Metagenome sequencing of the biofilm sample from Site B gave insight into the metals-tolerance genes in these organisms. The most abundant metal-associated gene identified in the Site B biofilm was cusA/ybdB, which is involved in a cation efflux system that mediates resistance to Cu and silver (Table 4). The second most abundant metal-associated gene observed was mdtC, which is involved in zinc efflux. Zinc was not identified during the mine development process as a current or future metal of environmental concern but it is present in the water column at relatively low levels. Potentially, the high abundance of this gene relates to its involvement as an efflux pump for more than one cationic metal. Since genes were counted as present with a 60% identity threshold, these results more generally suggest that heavy-metal efflux ATPases were present in this metagenome. One of the best characterized P-type ATPases related to Cu resistance, copA (Orell et al., 2010), was detected only at very low abundance in this sample, which was expected due to the lack of annotated copA genes in the two available sequenced Gallionellaceae genomes. The metal-associated gene with the largest fold change between Site B and Site A was chrB (Table 4), which belongs to the chrBACF operon and has been shown to be involved in resistance to high concentrations of chromium (Branco et al., 2008). ChrA, which also belongs to this operon, also had a high fold change at Site B over Site A (Table 4). It is possible that the abundance of these two chr genes was not indicating chromium or chromate resistance, but rather a resistance against harmful reactive oxygen species that can be produced during the detoxification of other metals (e.g., Cu[I]) because genes in the chrBACF operon have also been shown to enable resistance to superoxide anions (Branco et al., 2008). Genes from the chrBACF operon have not been annotated in G. capsiferriformans or S. lithotrophicus but chrA is present in Ferriphaselus sp. R-1, which is an incomplete genome from the Gallionellaceae family. This further supports our hypothesis that this study has identified a new genus within Gallionellaceae, one that has a unique profile of metals tolerance genes, enabling adaptation to this environment. Overall, the metagenomic sequencing results identified numerous well-known metal-related tolerance genes; thus, the Site B biofilm was resistant to the heavy metal cations in the water and is likely well situated to tolerate conditions in a mining operations environment.
This study represents a first stage investigation at a future mining site into the native microbial communities present in a stream affected by acidic and high metals concentration conditions. It is an important step in characterizing the diversity of microbes adapted to extreme environments and the naturally-evolved microbial potential for beneficial and effective future uses, before mining activities begin and disturb the natural environment. These findings provide important baseline data regarding microbes present and the effect of metals on the community composition, as well as supporting future sampling and investigations of their potential utility in bioremediation.
Conclusions
A native microbial community from a main watercourse in a proposed mining operation in Yukon, Canada is dominated by bacteria in the family Gallionellaceae. This microbiome tolerates elevated heavy metals concentrations and may be providing beneficial bioremedial actions by reducing aqueous Cu concentrations, a metal of environmental concern. The 16S rRNA gene and metagenomic investigations in this study have identified the taxonomic and functional profiles of key microbial communities and may enable the assembly of one or more genomes in the Gallionellaceae family from this unique site. The shotgun sequencing of DNA from biofilm samples provides insight into the mechanisms that may be providing resistance to heavy metals, by the identification of genes that encode for efflux pumps, cell wall components, and metabolic processes for metals tolerance. This study provides important baseline data that indicates a potential bacterial component in a case of natural Cu depletion. It provides a pathway for future studies to specifically investigate the biofilm community (or the most abundant bacteria within) that may be responsible for this transformation. Fundamentally, these analyses are useful for investigations into the potential use of a novel native biofilm to bioremediate contaminants and reduce adverse effects in aquatic organisms. This study is one of the first investigations to profile the taxa and genes in a stream microbiome from the Yukon, Canada, an area rich in valuable metals, and therefore provides support for investigations on novel organisms useful to the mining industry and for environmental protection.
Author Contributions
TV analyzed results with bioinformatics techniques, wrote parts of Materials and Methods and Discussion and majority of Results Sections of manuscript (MS), and prepared figures and tables. HO aided with method of sample collection, contributed to interpretation of results, and wrote portions of MS. MP, EG, and RL performed laboratory work: DNA extractions, library preparations, and sequencing runs. MP did background research and wrote some of the MS. NL, MQ, and RP aided with study design and performed field collection of samples. FB supervised TV, EG, and RL, contributed to interpretation of results, and edited the MS. CK supervised HO and MP, designed study, contributed to interpretation of results, and edited the MS.
Conflict of Interest Statement
The authors declare that the research was conducted in the absence of any commercial or financial relationships that could be construed as a potential conflict of interest.
Casino Mining Corporation (CMC) provided supportive co-funding for the GenomeBC study. The findings and conclusions presented by the authors are their own and do not necessarily reflect the view or position of CMC.
Acknowledgments
This study was funded by a GenomeBC grant #UPP004 to CK in partnership with the CMC and Palmer Environmental Consulting Group (PECG). We acknowledge additional funding to TV from the Natural Sciences and Engineering Research Council of Canada (NSERC) and the Molecular Biology and Biochemistry Department of Simon Fraser University (SFU; Burnaby, BC, Canada), and to MP from the Biological Sciences Department of SFU. Help from Sydney Love and other staff and students in the FB and CK laboratories at SFU was greatly appreciated, as well as from Dr. P. Tang and Dr. M. Uyaguari-Diaz from the BC Centre for Disease Control (Vancouver, BC, Canada) and Dr. K. Besemer from the University of Vienna (Vienna, Austria).
Supplementary Material
The Supplementary Material for this article can be found online at: http://journal.frontiersin.org/article/10.3389/fenvs.2015.00084
Table S1. Number of metagenomic reads assigned to taxa for samples from Sites A and B.
Table S2. Number of metagenomic reads assigned to SEED functional groups for samples from Sites A and B.
Table S3. Number of reads per million assigned to genes associated with metal-resistance in the BacMet database.
Figure S1. Bar plots of microbial taxa profiles (class and order) in each replicate for each biofilm sampling site. Bar plots represent a summary of the taxonomic composition of each sample by (A) Class, and (B) Order, grouped by sampling Sites A–E (n = 3 per site). Colors do not correspond between figures. Square brackets indicate proposed taxonomy based on Greengenes phylogenetic analysis.
Footnotes
1 ^http://www.casinomining.com/project/proposed_mine/.
2 ^http://www.ccme.ca/en/resources/canadian_environmental_quality_guidelines/index.html.
References
Akob, D. M., Bohu, T., Beyer, A., Schäffner, F., Händel, M., Johnson, C. A., et al. (2014). Identification of Mn(II)-oxidizing bacteria from a low-pH contaminated former uranium mine. Appl. Environ. Microbiol. 80, 5086–5097. doi: 10.1128/AEM.01296-14
Andreazza, R., Pieniz, S., Wolf, L., Lee, M.-K., Camargo, F. A. O., and Okeke, B. C. (2010). Characterization of copper bioreduction and biosorption by a highly copper resistant bacterium isolated from copper-contaminated vineyard soil. Sci. Total Environ. 408, 1501–1507. doi: 10.1016/j.scitotenv.2009.12.017
Arini, A., Feurtet-Mazel, A., Morin, S., Maury-Brachet, R., Coste, M., and Delmas, F. (2012). Remediation of a watershed contaminated by heavy metals: a 2-year field biomonitoring of periphytic biofilms. Sci. Total Environ. 425, 242–253. doi: 10.1016/j.scitotenv.2012.02.067
Baker, B. J., and Banfield, J. F. (2003). Microbial communities in acid mine drainage. FEMS Microbiol. Ecol. 44, 139–152. doi: 10.1016/S0168-6496(03)00028-X
Bankevich, A., Nurk, S., Antipov, D., Gurevich, A. A., Dvorkin, M., Kulikov, A. S., et al. (2012). SPAdes: a new genome assembly algorithm and its applications to single-cell sequencing. J. Comput. Biol. 19, 455–477. doi: 10.1089/cmb.2012.0021
Besemer, K., Peter, H., Logue, J. B., Langenheder, S., Lindström, E. S., Tranvik, L. J., et al. (2012). Unraveling assembly of stream biofilm communities. ISME J. 6, 1459–1468. doi: 10.1038/ismej.2011.205
Bier, R. L., Voss, K. A., and Bernhardt, E. S. (2014). Bacterial community responses to a gradient of alkaline mountaintop mine drainage in Central Appalachian streams. ISME J. 9, 1378–1390. doi: 10.1038/ismej.2014.222
Bolger, A. M., Lohse, M., and Usadel, B. (2014). Trimmomatic: a flexible trimmer for illumina sequence data. Bioinformatics 30, 2114–2120. doi: 10.1093/bioinformatics/btu170
Branco, R., Chung, A. P., Johnston, T., Gurel, V., Morais, P., and Zhitkovich, A. (2008). The chromate-inducible chrBACF operon from the transposable element TnOtChr confers resistance to chromium (VI) and superoxide. J. Bacteriol. 190, 6996–7003. doi: 10.1128/JB.00289-08
Caporaso, J. G., Bittinger, K., Bushman, F. D., DeSantis, T. Z., Andersen, G. L., and Knight, R. (2010b). PyNAST: a flexible tool for aligning sequences to a template alignment. Bioinformatics 26, 266–267. doi: 10.1093/bioinformatics/btp636
Caporaso, J. G., Kuczynski, J., Stombaugh, J., Bittinger, K., Bushman, F. D., Costello, E. K., et al. (2010a). QIIME allows analysis of high-throughput community sequencing data. Nat. Methods 7, 335–336. doi: 10.1038/nmeth.f.303
Caporaso, J. G., Lauber, C. L., Walters, W. A., Berg-Lyons, D., Lozupone, C. A., and Turnbaugh, P. J. (2011). Global patterns of 16S rRNA diversity at a depth of millions of sequences per sample. PNAS 108, 4516–4522. doi: 10.1073/pnas.1000080107
DeSantis, T. Z., Hugenholtz, P., Larsen, N., Rojas, M., Brodie, E. L., Keller, K., et al. (2006). Greengenes, a Chimera-Checked 16S rRNA gene database and workbench compatible with ARB. Appl. Environ. Microbiol. 72, 5069–5072. doi: 10.1128/AEM.03006-05
Desrosiers, M., Planas, D., and Mucci, A. (2011). Total mercury and methylmercury accumulation in periphyton of Boreal Shield Lakes: influence of watershed physiographic characteristics. Sci. Total Environ. 355, 247–258. doi: 10.1016/j.scitotenv.2005.02.036
Edgar, R. C. (2010). Search and clustering orders of magnitude faster than blast. Bioinformatics 26, 2460–2461. doi: 10.1093/bioinformatics/btq461
Emerson, D., Field, E. K., Chertkov, O., Davenport, K. W., Goodwin, L., Munk, C., et al. (2013). Comparative genomics of freshwater Fe-oxidizing bacteria: implications for physiology, ecology, and systematics. Front. Microbiol. 4:254. doi: 10.3389/fmicb.2013.00254
Fabisch, M., Beulig, F., Akob, D. M., and Küsel, K. (2013). Surprising abundance of Gallionella-related iron oxidizers in creek sediments at pH 4.4 or at high heavy metal concentrations. Front. Microbiol. 4:390. doi: 10.3389/fmicb.2013.00390
Gadd, G. M. (2010). Metals, minerals and microbes: geomicrobiology and bioremediation. Microbiology 156, 609–643. doi: 10.1099/mic.0.037143-0
Ghosh, A., and Saha, P. D. (2013). Optimization of copper bioremediation by Stenotrophomonas maltophilia PD2. J. Environ. Chem. Eng. 1, 159–163. doi: 10.1016/j.jece.2013.04.012
Glasauer, S., Langley, S., and Beveridge, T. J. (2001). Sorption of Fe (hydr)oxides to the surface of Shewanella putrefaciens: cell-bound fine-grained minerals are not always formed de novo. Appl. Environ. Microbiol. 67, 5544–5550. doi: 10.1128/AEM.67.12.5544-5550.2001
Huson, D. H., Mitra, S., Ruscheweyh, H. J., Weber, N., and Schuster, S. C. (2011). Integrative analysis of environmental sequences using MEGAN4. Genome Res. 21, 1552–1560. doi: 10.1101/gr.120618.111
Islam, M. S., Zhang, Y., McPhedran, K. N., Liu, Y., and El-Din, M. G. (2015). Granular activated carbon for simultaneous adsorption and biodegradation of toxic oil sands process-affected water organic compounds. J. Environ. Manage. 152, 49–57. doi: 10.1016/j.jenvman.2015.01.020
ITRC (Interstate Technology Regulatory Council) (2008). In Situ Bioremediation of Chlorinated Ethene: DNAPL Source Zones. BioDNAPL-3. Washington DC: Interstate Technology and Regulatory Council, Bioremediation of DNAPLs Team. Available online at: www.itrcweb.org (Accessed April 12, 2015).
Klindworth, A., Pruesse, E., Schweer, T., Peplies, J., Quast, C., Horn, M., et al. (2012). Evaluation of general 16S ribosomal RNA gene PCR primers for classical and next-generation sequencing-based diversity studies. Nucleic Acid Res. 41, 1–11. doi: 10.1093/nar/gks808
Larouche, J. R., Bowden, W. B., Giordano, R., Flinn, M. B., and Crump, B. C. (2012). Microbial biogeography of arctic streams: exploring influences of lithology and habitat. Front. Microbiol. 3:309. doi: 10.3389/fmicb.2012.00309
Li, H., Li, M., Huang, Y., Rensing, C., and Wang, G. (2013). In silico analysis of bacterial arsenic islands reveals remarkable synteny and functional relatedness between arsenate and phosphate. Front. Microbiol. 4:347. doi: 10.3389/fmicb.2013.00347
Liljeqvist, M., Ossandon, F. J., González, C., Rajan, S., Stell, A., Vales, J., et al. (2015). Metagenomic analysis reveals adaptations to a cold adapted lifestyle in a low temperature acid mine drainage stream. FEMS Microbiol. Ecol. 91:fiv011. doi: 10.1093/femsec/fiv011
Malik, A. (2004). Metal bioremediation through growing cells. Environ. Int. 30, 261–278. doi: 10.1016/j.envint.2003.08.001
Martin, M. (2011). Cutadapt removes adapter sequences from high-throughput sequencing reads. EMBnet J. 17, 10–12. doi: 10.14806/ej.17.1.200
McMurdie, P. J., and Holmes, S. (2013). Phyloseq: an R package for reproducible interactive analysis and graphics of microbiome census data. PLoS ONE 8:e61217. doi: 10.1371/journal.pone.0061217
Muyzer, G., and Stams, A. J. (2008). The ecology and biotechnology of sulphate-reducing bacteria. Nat. Rev. Microbiol. 6, 441–454. doi: 10.1038/nrmicro1892
Nikolenko, S. I., Korobeynikov, A. I., and Alekseyev, M. A. (2013). BayesHammer: Bayesian clustering for error correction in single-cell sequencing. BMC Genomics 14:S7. doi: 10.1186/1471-2164-14-S1-S7
Oksanen, J., Blanchet, F. G., Kindt, R., Legendre, P., Minchin, R., O'Hara, B., et al. (2002). Vegan: Community Ecology Package. R package version 2.2-1. Available online at: http://cran.r-project.org/web/packages/vegan/index.html
Orell, A., Navarro, C. A., Arancibia, R., Mobarec, J. C., and Jerez, C. A. (2010). Life in blue: copper resistance mechanisms of bacteria and Archaea used in industrial biomining of minerals. Biotechnol. Adv. 28, 839–848. doi: 10.1016/j.biotechadv.2010.07.003
Overbeek, R., Begley, T., Butler, R. M., Choudhuri, J. V., Chuang, H. Y., Cohoon, M., et al. (2005). The subsystems approach to genome annotation and its use in the project to annotate 1000 genomes. Nucl. Acids Res. 33, 5691–5702. doi: 10.1093/nar/gki866
Pal, C., Bengtsson-Palme, J., Rensing, C., Kristiansson, E., and Larsson, D. G. J. (2014). BacMet: Antibacterial biocide and metal resistance genes database. Nucleic Acids Res. 42, D737–D743. doi: 10.1093/nar/gkt1252
Perales-Vela, H. V., Peña-Castro, J. M., and Cañizares-Villanueva, R. O. (2006). Heavy metal detoxification in eukaryotic microalgae. Chemosphere 64, 1–10. doi: 10.1016/j.chemosphere.2005.11.024
Price, M. N., Dehal, P. S., and Arkin, A. P. (2009). FastTree: computing large minimum evolution trees with profiles instead of a distance matrix. Mol. Biol. Evol. 26, 1641–1650. doi: 10.1093/molbev/msp077
Schirmer, M., Ijaz, U. Z., D'Amore, R., Hall, N., Sloan, W. R., and Quince, C. (2015). Insight into biases and sequencing errors for amplicon sequencing with the Illumina MiSeq platform. Nucl. Acid Res. 43:e37. doi: 10.1093/nar/gku1341
Tay, S. T.-L., Hemond, H. F., Krumholz, L., Cavanaugh, C. M., and Polz, M. F. (2001). Population dynamics of two toluene degrading bacterial species in a contaminated stream. Microbiol. Ecol. 41, 124–131. doi: 10.1007/s002480000089
Tay, S. T.-L., Hemond, H. F., Polz, M. F., Cavanaugh, C. M., and Krumholz, L. (1998). Two new Mycobacterium strains and their role in toluene degradation in a contaminated stream. Appl. Environ. Microbiol. 64, 1715–1720.
Tsitko, I., Lusa, M., Lehto, J., Parviainen, L., Ikonen, A. T., Lahdenperä, A. M., et al. (2014). The variation of microbial communities in a depth profile of an acidic, nutrient-poor boreal bog in southwestern Finland. Open J. Ecol. 4, 832. doi: 10.4236/oje.2014.413071
White, C., and Gadd, G. M. (2000). Copper accumulation by sulphate-reducing bacterial biofilms. FEMS Microbiol. Lett. 183, 313–318. doi: 10.1111/j.1574-6968.2000.tb08977.x
Zhang, J., Kobert, K., Flouri, T., and Stamatakis, A. (2014). PEAR: a fast and accurate Illumina paired-end reAd mergeR. Bioinformatics 30, 614–620. doi: 10.1093/bioinformatics/btt593
Keywords: microbiome, copper, bioremediation, Gallionellaceae, mine, metagenomics, metals, biofilm
Citation: Van Rossum T, Pylatuk MM, Osachoff HL, Griffiths EJ, Lo R, Quach M, Palmer R, Lower N, Brinkman FSL and Kennedy CJ (2016) Microbiome Analysis Across a Natural Copper Gradient at a Proposed Northern Canadian Mine Site. Front. Environ. Sci. 3:84. doi: 10.3389/fenvs.2015.00084
Received: 12 October 2015; Accepted: 11 December 2015;
Published: 06 January 2016.
Edited by:
Belinda Ferrari, University of New South Wales, AustraliaReviewed by:
Bharath Prithiviraj, The Samuel Roberts Noble Foundation Inc., USABrendan Paul Burns, University of New South Wales, Australia
Copyright © 2016 Van Rossum, Pylatuk, Osachoff, Griffiths, Lo, Quach, Palmer, Lower, Brinkman and Kennedy. This is an open-access article distributed under the terms of the Creative Commons Attribution License (CC BY). The use, distribution or reproduction in other forums is permitted, provided the original author(s) or licensor are credited and that the original publication in this journal is cited, in accordance with accepted academic practice. No use, distribution or reproduction is permitted which does not comply with these terms.
*Correspondence: Christopher J. Kennedy, Y2tlbm5lZHlAc2Z1LmNh
†These authors have contributed equally to this work.